- 1Department of Biostatistics, School of Public Health, Cheeloo College of Medicine, Shandong University, Jinan, China
- 2National Institute of Health Data Science of China, Shandong University, Jinan, China
- 3Shandong Mental Health Center, Shandong University, Jinan, China
Intrinsically disordered proteins (IDPs) participate in many biological processes by interacting with other proteins, including the regulation of transcription, translation, and the cell cycle. With the increasing amount of disorder sequence data available, it is thus crucial to identify the IDP binding sites for functional annotation of these proteins. Over the decades, many computational approaches have been developed to predict protein-protein binding sites of IDP (IDP-PPIS) based on protein sequence information. Moreover, there are new IDP-PPIS predictors developed every year with the rapid development of artificial intelligence. It is thus necessary to provide an up-to-date overview of these methods in this field. In this paper, we collected 30 representative predictors published recently and summarized the databases, features and algorithms. We described the procedure how the features were generated based on public data and used for the prediction of IDP-PPIS, along with the methods to generate the feature representations. All the predictors were divided into three categories: scoring functions, machine learning-based prediction, and consensus approaches. For each category, we described the details of algorithms and their performances. Hopefully, our manuscript will not only provide a full picture of the status quo of IDP binding prediction, but also a guide for selecting different methods. More importantly, it will shed light on the inspirations for future development trends and principles.
1 Introduction
With the rapid development in the protein field, there are increasingly number of intrinsically disordered proteins (IDPs) and intrinsically disordered protein regions (IDRs) identified in viruses, bacteria, archaea, and eukaryotes (Dubreuil et al., 2019).
IDPs lack stable tertiary structure under physiological conditions and are highly flexible compared with globular proteins (Uversky et al., 2008). Therefore, when interacting with other proteins, IDP can participate in various physiological processes (Uversky et al., 2005) including cell signal transduction and regulation through conformational changes. IDPs are also widely involved in diseases. For example, type 1 susceptible protein (BRCA1) involved in the occurrence of breast cancer participates in the interaction mainly through disordered regions (Uversky et al., 2008), and α -synuclein folds from disordered state in acidic or high temperature environment, which leads to neurodegenerative diseases (Uversky et al., 2008).
IDPs take part in protein-protein interactions through one-to-many or many-to-one mode (Uversky et al., 2008). Studies found that the protein-protein interaction in which IDP participates is often achieved through coupled folding and binding (Dyson and Wright, 2002). For example, CREB protein forms a spiral structure by binding CBP protein (Radhakrishnan et al., 1997), and the binding of p53 protein with MDM2 protein leads to the protein folding from coil to spiral (Kussie et al., 1996). It has been found that the binding of IDP with corresponding proteins will lead to the transition from disorder to order. For instance, the disordered regions of E-cadherin turn to order format after interacting with β-catenin (Dyson and Wright, 2002); the disordered protein DFF45 interacts with DFF40 to transform into an ordered state (Zhou et al., 2001); the Furin-like cleavage site exists at the S1/S2 junction of the SARS-CoV-2 Spike (FLCSSpike), and the “disorder-to-order transition” of Spike-Furin complex has been found (Roy et al., 2022). In addition, some ordered proteins interacting with other molecules leads to the unfolding of self-inhibiting domains and activates biological functions (Uversky, 2013). All these examples above show that protein interaction sites play an important role in the transition between disordered protein and ordered protein.
The identification of protein-protein interaction sites is a key to deciphering the functional relationship between proteins and biological processes, which is one of the most important tasks for both experimental and computational approaches. The commonly used experimental methods to pinpoint binding sites in disordered proteins include nuclear magnetic resonance spectroscopy (NMR) and non-equilibrium transient kinetic techniques (Mollica et al., 2016). NMR identifies binding sites through the changes in chemical shifts and residual dipole coupling (RDC) caused by changes in the magnetic environment during binding (Jensen et al., 2011); non-equilibrium transient kinetic technique refers to identifying binding sites by measuring the changes in optical signals that occur during the binding process of disordered proteins (Mollica et al., 2016). Large efforts have also been devoted to gaining a better knowledge of disordered protein interactions by high throughput methodologies through amino acid substitutions, such as binding energetics study in CcdA (Chandra et al., 2022), mutational studies in c-Myb (Giri et al., 2013), ACTR (transcriptional co-activator for thyroid hormone and retinoid receptors) (Dogan et al., 2013), NCBD domain of CBP (CREB binding protein) (Dogan et al., 2013), Hif 1α (hypoxia inducible factor 1α) (Lindström et al., 2018) as well as MazE6 antitoxin (Chandra et al., 2021). These studies greatly contributed to obtaining a thorough understanding of disordered protein interactions.
Besides the experiment methods, there are a series of computational approaches developed for IDP protein interaction sites (IDP-PPIS) prediction such as MoRFpred (Disfani et al., 2012), SLiMPred (Mooney et al., 2012), ANCHOR (Dosztanyi et al., 2009; Mészáros et al., 2009) and SPINE-D (Zhang et al., 2013). With the increasing amount of disordered protein data available, computational approaches to predict IDP-PPIS are becoming more and more important to aid the expensive and time-consuming experiments to annotate the functional properties of disordered proteins. Predictors on IDP-PPIS are mainly designed for predicting several sub-types of disorder binding sites including molecular recognition features (MoRFs), short linear motifs (SLiMs), disordered protein-binding regions (DPBRs), and semi-disordered regions (Katuwawala et al., 2019b). Molecular recognition features (MoRFs) are short disordered fragments involved in state transitions through four types (α-MoRFs, β-MoRFs, ι-MoRFs and complex-MoRFs) during disordered protein binding activities (Mohan et al., 2006). Short linear motifs (SLiMs) are short disordered protein fragments that bind to the structural domains of proteins, consisting of 3–10 amino acid residues, and the disordered binding regions operated by SLiMs and MoRFs are highly overlapping (Mooney et al., 2012; Weatheritt and Gibson, 2012). Disordered protein-binding regions (DPBRs) are more general binding fragments that include not only short binding regions but also longer fragments (Katuwawala et al., 2019b). Semi-disordered regions refer to regions with a 50% probability of being predicted to be disordered regions, and its functional properties can be used to further predict MoRFs (Katuwawala et al., 2019b).
There are several review articles (Katuwawala et al., 2019a; Katuwawala et al., 2019b) on the predictors of IDP-PPIS have been published previously, but most lack systematic descriptions of the factors affecting the performance of the predictors. Moreover, it is time to conclude the latest predictors due to the rapid update of the IDP interaction site predictors. For these purposes, we select 30 predictors of IDP-PPIS published up to January 2022 and provide a comprehensive description of the database, features, and algorithms used in the predictor construction process. This paper gives a detailed overview of the status of the predictors of IDP-PPIS and thus provides new insights and inspiration for the development and application of new computational approaches.
2 Overview of the predictors of intrinsically disordered protein-protein interaction sites
Figure 1 illustrates the common scheme for developing an IDP-PPIS predictor. Firstly, datasets are curated and selected from large databases and/or published papers. Then, features are extracted from protein sequences using different methods. Then, different algorithms are applied to train and optimize the predictors to output a real-valued amino acid propensity score or binary prediction. Here, we classify the predictors into three categories based on the algorithms used: scoring functions, machine learning-based methods, and consensus-based approaches.
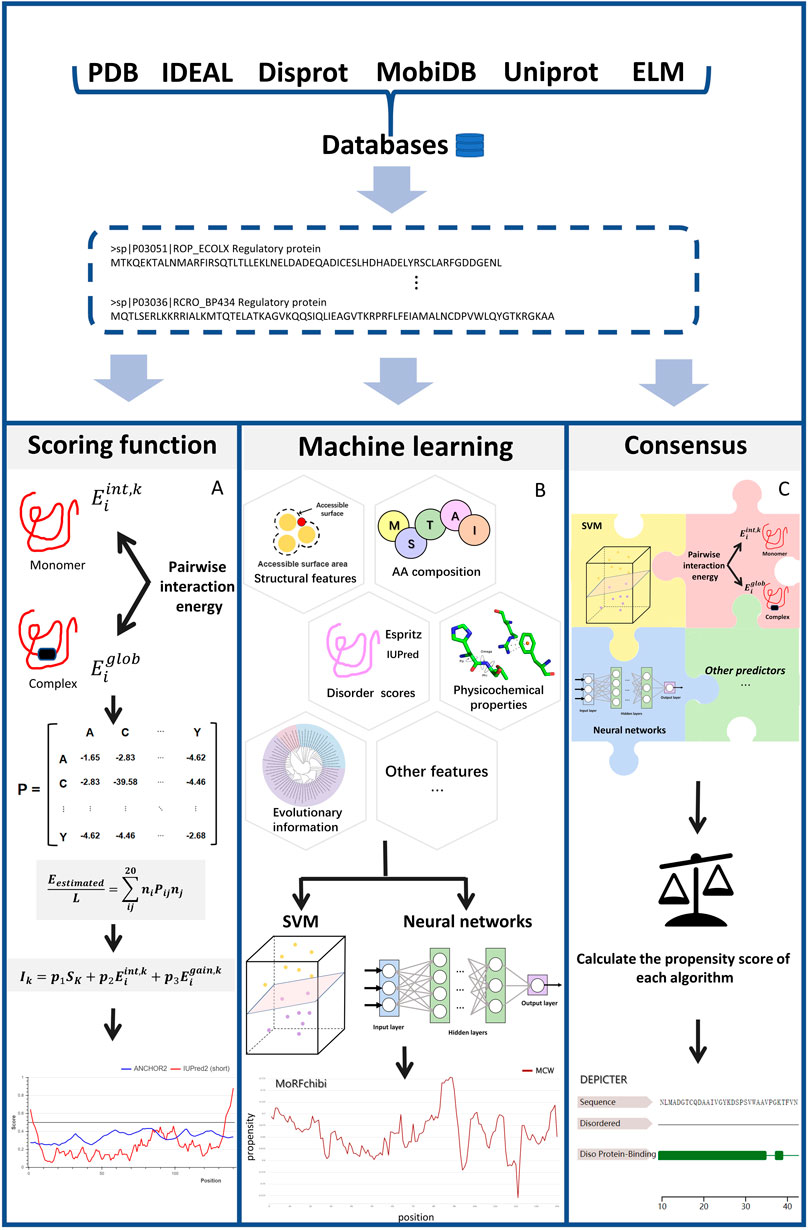
FIGURE 1. The work flow of each type of methods Figure 1 illustrates main three categories described in this article: (A) scoring function-based methods (B) machine learning-based methods and (C) consensus-based methods. The key steps for each type of methods are depicted in the diagram. Scoring function-based methods in (A), we use ANCHOR work flow to represent how scoring function works. Machine learning-based methods perform the prediction using various types of machine learning models like SVM and Neural Network based on features extracted from different perspectives. Consensus-based methods can predict IDP binding site by weighting different prediction models and combining them optimally. The final results show in (A) was processed by (Mészáros et al., 2018). The final results show in (B) was processed by (Malhis et al., 2016), The final results show in (C) was processed by (Barik et al., 2020).
2.1 Databases
From Figure 1, the first key aspect of developing the predictor is the selection of a sufficient amount of high-quality data in a standardized format. We present databases widely used in predictors and describes some relevant databases.
The Database of Protein Disorder (DisProt) (Sickmeier et al., 2007) is the first public available database on IDPs and IDRs which includes experimentally annotated information on multiple types of proteins. DisProt is one of the common databases for IDP-PPIS predictors, and the latest version is DisProt 9, covering more than 2000 proteins and nearly 5,000 functional annotations (Quaglia et al., 2021). DisProt is available at https://www.disprot.org/.
Disordered protein regions are often characterized by the missing electron density found by X-ray (DeForte and Uversky, 2016). Several different X-ray experiments are used to examine the same protein to achieve a more stable definition of disordered regions (Monzon et al., 2020). Therefore, The Protein Data Bank (PDB) (Burley et al., 2018), the largest protein 3D structure database is often used for obtaining the contact details of disorder regions in structured proteins. PDB is available at https://www.rcsb.org/.
The missing annotations are related to the inherently disordered protein regions. Several methods implemented UniProt (Apweiler et al., 2010) for disordered prediction, e.g., MobiDB-lite (Bateman et al., 2021). UniProtKB (Apweiler et al., 2010) is a protein database managed by experts, which consists of UniProtKB/Swiss-Prot containing manually annotated data. UniProt (Apweiler et al., 2010) is available at https://www.uniprot.org/.
Intrinsically Disordered proteins with Extensive Annotations and Literature (IDEAL) (Fukuchi et al., 2011) is another commonly used database for disordered protein studies, covering reliable evidence of disordered proteins. In particular, annotations for protein binding regions are helpful for binding sites prediction. There are more than 10,000 non-redundant IDRs in IDEAL as of October 2021. IDEAL (Fukuchi et al., 2013) is available at http://www.ideal.force.cs.is.nagoya-u.ac.jp/IDEAL/.
The Disordered Binding Site (DIBS) (Schad et al., 2017) is a large database storing disordered protein complexes, primarily in combination with ordered proteins. The synthesized information provided on protein interactions is an important resource for studying binding sites. DIBS is available at http://dibs.enzim.ttk.mta.hu/.
The Eukaryotic Linear Motif (ELM) (Dinkel et al., 2011; Kumar et al., 2021) is the first database focused on collecting, storing and providing experimentally confirmed short linear motif (SLiM) information and is an important repository for studying protein-protein interactions of SLiM. ELM is available at http://elm.eu.org.
MobiDB (Piovesan et al., 2020) is a database of IDPs commonly used by predictors, forming four-level annotations of ' CDHP ' (curated, derived, homology, prediction) by connecting other databases and applying various tools. The latest version is MobiDB 4.1, which contains more than 200 million proteins. MobiDB is available at http://mobidb.bio.unipd.it/.
The Structural Classification of Proteins (SCOP) (Andreeva et al., 2019) is a database for storing protein domains. Due to the differences in the evolutionary properties and structural similarity of proteins. Protein domains are divided into various categories. There are six classification levels in SCOP which IUPR is related to IDP. SCOP is available at http://scop.mrc-lmb.cam.ac.uk.
There are some relevant and commonly used databases such as Database of Disordered Protein Prediction (D2P2) and Mutual Folding Induced by Binding (MFIB). D2P2 (Oates et al., 2012) is a database for disorder and SCOP domain prediction and annotation of over 10 million protein sequences by multiple predictors. D2P2 is more commonly used for the study of protein disorder and structural relationships. D2P2 is available at http://d2p2.pro.
MFIB (Fichó et al., 2017) is the database consisting only of complexes formed by intrinsically disordered proteins, and with far more data than other similar datasets, it is an important repository for studying disordered protein interactions. MFIB is available at http://mfib.enzim.ttk.mta.hu/.
2.2 Features
The second critical part of constructing predictors is to obtain representative features of disordered protein sequences. Features integrated by the predictor determine the accuracy of distinguishing between IDP protein sites and general amino acid residues. In IDP-PPIS prediction, the most widely used features include amino acid compositions, predicted structural features, disorder scores, physicochemical properties, evolutionary information, and other features. We summarize these features and tools to generate these features in Table 1.
2.2.1 Amino acid composition
Amino acid composition (AAC) is the proportion of a particular amino acid in the whole protein sequence. The amino acid compositions are distinct among IDR, MoRF, flanking regions and other protein regions. It is reported that there are more Ile, Leu, Phe, Tyr, Lys, Arg and Asp enriched and less Ala and Gly comparing with the normal regions. Meanwhile, the flanking region includes more Ala, Gly, Glu, Ser and Thr which turns to promote disorder (Fang et al., 2013; Wang et al., 2017).
AAC could be calculated by the formula (Wang et al., 2017):
2.2.2 Predicted structural features
The rapid structural transformation of disordered proteins during binding (Uversky and Dunker, 2010) suggests that the predicted structural characteristics of disordered proteins can improve the accuracy of binding site prediction. Commonly used predicted structural features are: secondary structure, structural motif, solvent accessible surface area, contact number, hemispheric exposure, backbone angle, and B-factor.
Molecular recognition feature (MoRF) and short linear motif (SLim) are involved in protein-protein interaction as a secondary structure element (Oldfield et al., 2005; Davey et al., 2012b), including α-helix, β-sheet, curl and other forms. Therefore, using the secondary structure (SS) of protein as a feature can better identify the binding sites. Structural motifs (Efimov, 2017) are folding units formed by the close contact of two or more adjacent secondary structural elements in three-dimensional space, which are often used as a structural feature to predict binding sites.
The biophysical properties of disordered protein change during protein interaction which could be used for IDP-PPIS prediction. For instance, protein-protein interactions are mainly achieved through surface contact, and when the protein state is transformed, the Accessible surface area (ASA) of contact area changes as well, which helps us to identify the binding site (Disfani et al., 2012; Heffernan et al., 2015). Contact numbers (CN) (Yuan, 2005; Heffernan et al., 2015) are also an indicator for measuring protein solvent exposure, which can effectively identify protein contact changes in folding state. Hemisphere exposure (HSE) (Hamelryck, 2005) is a two-dimensional measure for assessing protein solvent exposure that is superior to ASA and CN in terms of computational speed and detection stability. The participation of disordered proteins in protein-protein interactions leads to a shift in the protein backbone (Hanson et al., 2019). The functional realization of disordered proteins is based on their flexible state transitions, and B-factor (temperature factor) is a feature that assesses protein flexibility and helps predict disordered protein binding sites (Disfani et al., 2012; Uversky, 2018).
2.2.3 Disorder scores
Disorder propensity is an important feature for predicting disordered protein binding sites (Dosztányi et al., 2005), and could be predicted by many predictors such as IUPred (Dosztanyi et al., 2005), ESpritz (Walsh et al., 2011), VSL2 (Peng et al., 2006), DISOPRED2 (Ward et al., 2004), DISOclust (McGuffin, 2008), MFDp (Mizianty et al., 2010). IUPred (Dosztanyi et al., 2005) calculates total interacting amino acid pair energy to predict disordered protein regions based on amino acid composition. ESpritz (Walsh et al., 2011) predicts three types of disorder regions (X-ray disorder, DisProt disorder, NMR mobility) based on sequence information using bidirectional recurrent neural networks (BRNN). VSL2 (Peng et al., 2006) predictor consists of VSL2-M1 and VSL2-M2, which solves the problem of heterogeneity in amino acid composition and sequence properties when predicting disordered proteins. DISOPRED2 (Ward et al., 2004) predicts disordered regions using support vector machines based on PSI-BLAST profiles. DISOclust (McGuffin, 2008) predicts disordered proteins by identifying conserved errors in fold recognition models. MFDp (Mizianty et al., 2010) applies three support vector machines to predict different types of disordered regions using multifaceted information.
2.2.4 Physicochemical properties
The structure and function of proteins are affected by the physicochemical properties of amino acids. Disordered proteins are more hydrophobic than ordered proteins, and disordered binding sites can be predicted by an increase in the hydrophobicity (Mészáros et al., 2007). MoRF and linear motifs have more net charge compared to surrounding protein fragments (Fuxreiter et al., 2007). Therefore, the feature of amino acid physicochemical properties is considered in predictors of disordered protein binding sites.
The physicochemical properties of amino acids can be obtained from the AAindex database (Kawashima et al., 2007). The physicochemical and biochemical indexes of amino acids and amino acid pairs in the AAindex database are derived from published literature, which includes three parts amino acid index, substitution matrix and contact potential. In addition, if a protein sequence has similar average amino acid index with MoRF sequence, it suggests that the protein sequence is MoRF (Malhis and Gsponer, 2015). The common physicochemical properties of amino acids include hydrophobicity, polarity, polarizability, charge, aliphatic, aromatics, etc (Fang et al., 2013; Wang et al., 2017).
2.2.5 Evolutionary information
Some studies show that the evolution speed of disordered regions is often faster than other parts of proteins (Brown et al., 2002). Davey et al. (2012a) found that SLiM is more conserved than the surrounding residues and that might be due to protein interactions are highly related to protein functions. Protein evolutionary information is also widely used for protein folding recognition (Lyons et al., 2015), and disordered proteins show protein folding changes during binding. Therefore, many predictors applied protein evolutionary information to identify IDP-PPIS.
Protein evolutionary information is often obtained through position-specific scoring matrix (PSSM), hidden Markov model (HMM) profiles and Information entropy. To improve prediction performance, certain studies use modified PSSM to enhance the sequence conservation signal, such as MFSPSSMpred (Fang et al., 2013) by masking, filtering and smoothing to retain only highly locally conserved information (Fang et al., 2018), obtained highly locally conserved features by amplification.
It is shown that using bigram to extract evolutionary features in natural language processing can reduce redundant features (Sharma et al., 2013), and also extract local evolutionary features for the identification of protein folding process (Lyons et al., 2015). Bigram is also an important feature for identifying IDP-PPIS.
Protein conservation is closely related to its structure and function, and the average Shannon entropy is used as a characteristic of general conservatism in protein. Some studies have used relative entropy to improve the prediction of protein functional sites (Wang and Samudrala, 2006), and Shannon entropy was also applied by (Hanson et al., 2016), to predict IDP-PPIS.
2.2.6 Other features
The length and location of IDRs correlate with general protein functional classes. Lobley et al. (2007) identified short disordered fragments involved in protein interactions in the mid to N-terminal region of GTPase regulatory proteins. FFPred (Minneci et al., 2013) used this feature to predict the biological functions of unknown proteins. Therefore, applying the length and location features of IDRs can improve the prediction accuracy of IDP binding sites.
Compared with the ordered protein, the sequence complexity of the disordered protein is lower (Romero et al., 2000), so the sequence complexity may be an important feature to identify the binding site of IDP. SEG algorithm (Peng and Kurgan, 2015) is mainly used to calculate the complexity of protein sequence.
2.3 Algorithms
Algorithm is essential to take the features as input and predict the IDP-PPIS, which is the core element for each predictor. We summarize main information about 30 predictors for IDP-PPIS prediction in Table 2. In this paper, these predictors are classified into three categories according to algorithms: scoring function based, machine-learning based and consensus, and we selectively describe the most representative predictors.
2.3.1 Scoring function based
The scoring function method is widely used in the evaluation of protein interaction (Liu and Wang, 2015). Its principle is to obtain the final prediction results by scoring the protein binding ability with various functions (Liu and Wang, 2015). This paper mainly introduces retro-MoRFs based on sequence alignment and ANCHOR series predictors based on paired energy estimation method to predict IDP-PPIS.
2.3.1.1 Sequence alignment
Sequence alignment is a commonly used tool to predict the structural and functional properties of proteins (Edgar and Batzoglou, 2006). Retro-MoRFs (Xue et al., 2010b) predictor used the software package PONDR-RIBS to make sequence alignment by CLUSTALW method (Thompson et al., 1994), and then successfully predicted α-MoRF in RNase E, p53 and SRC-3 by combining PONDR-FIT (Xue et al., 2010a)and PONDR-VLXT (Romero et al., 2000) out-of-order prediction. The predictor innovatively used reverse sequence alignment to identify retro-MoRF.
2.3.1.2 Energy estimation
ANCHOR (Dosztanyi et al., 2009; Mészáros et al., 2009) is a benchmark method in IDP-PPIS prediction. Compared with other predictors, ANCHOR did not include the features of the secondary structure and its combining partners, but still had good predictive performance. The accuracy of ANCHOR reaches 0.67. The principle of the predictor is to predict IDP-PPIS based on the transition of disordered proteins from energy-deficient state to energy-sufficient state during binding. Based on this principle, ANCHOR identified the fragments which were located in the disordered region which could not form enough favorable intra-chain interactions to fold on their own, and might gain stable energy by interacting with globular proteins partners.
The algorithm of the ANCHOR can be expressed as:
On the basis of ANCHOR (Dosztanyi et al., 2009), ANCHOR2 (Mészáros et al., 2018) increased the energy used to estimate the interaction between spherical protein and local disordered sequence environment. In other words, IDP-PPPS must be exposed to disordered environments and finish the binding process on the surface. ANCHOR2 performs well with AUC up to 0.901.
The new function is defined as follows:
Here,
2.3.2 Machine-learning based
From Table 2, we can observe that more than two third of the predictors are based on machine learning. These types of methods usually integrate multiple features derived from the protein sequence information into the model, and use a variety of machine learning algorithms to train the predictors and predict the IDP-PPIS. The prediction results include the binding site propensity score and binary classification prediction. Our collection of machine learning-based predictors mainly uses support vector machines (SVM) (Chang and Lin, 2011) and various types of neural networks (Cheng et al., 2007). In addition, other algorithms such as Logistic regression (Li et al., 1999), gradient boosting trees (Wong and Gsponer, 2019b), conditional random fields (Wong and Gsponer, 2019b) and random forests (RF) (Basu et al., 2017) are also used.
2.3.2.1 Support vector machine
Support vector machine (SVM) (Fan et al., 2008; Chang and Lin, 2011) is a supervised machine learning method, which has been widely used to solve classification and regression problems. Support vector machines usually use kernel functions to solve linear (such as linear kernel) and nonlinear problems (such as Sigmoid function and radial basis function (RBF)). The commonly used SVM algorithms in these predictors are LIBSVM and LIBLINEAR. The data were processed by SVM and a probability value was obtained. When the probability value was greater than 0.5, the amino acid residue was considered as a protein binding site.
MoRFpred (Disfani et al., 2012) is a predictor for identifying different types of MoRF based on protein sequence derived information, using linear kernel support vector machine (SVM) and annotation generated by sequence alignment. MoRFpred chose SVM model with parameter
Due to the slow running speed of MoRFpred for large-scale prediction (Yan et al., 2016), developed a fMoRFpred predictor using SVM method to identify MoRF in 2016. fMoRFpred used the data set of MoRFpred, but selected a larger number of feature sets such as predicted disordered region and secondary structure, and the features of a smaller sliding window to improve the performances. Meanwhile, fMoRFpred also used only high-throughput disordered predictors and secondary structure to speed up the computing. The SVM model used by fMoRFpred chose the default parameter
MFSPSSMpred (Fang et al., 2013) improved the position-specific scoring matrix (PSSM) encoding scheme and extracted protein sequence information from PSSM, and finally applied an SVM model with kernel radial basis function (RBF) to predict MoRF. MFSPSSMpred outperforms other predictors in their paper with the highest AUC of 0.758.
In 2016 (Fang et al., 2016), developed a PSSMpred predictor for predicting the SLiM region. PSSMpred also only used the evolutionary information obtained from the position-specific scoring matrix (PSSM), and applied the SVM model with the kernel of radial basis function (RBF). Its performance is also better than that of other predictors, and obtained the AUC of 0.758.
DISOPRED3 (Jones and Cozzetto, 2014) used amino acid composition, PSSM obtained by PSI-BLAST and the length and location of IDR to apply an SVM classifier with an RBF kernel to predict protein binding sites. DISOPRED3 performs well with an MCC of 0.126.
MoRFCHiBi (Malhis and Gsponer, 2015) trained SVMS and SVMT models of Sigmoid and Radial Basis Function (RBF) Gaussian kernels using the physicochemical properties of amino acids. MoRFCHiBi predicts MoRF by integrating the results of both models with the help of Bayesian rules. It employed SVMS to predict MoRF propensity based on component comparison information and SVMT to predict MoRF propensity based on similarity information. MoRFCHiBi performs better than other predictors with the highest AUC of 0.770 but slower than ANCHOR.
MoRFCHiBiLight (Malhis et al., 2016) used Bayes rules to combine MoRFCHiBi with ESpritz’s (Walsh et al., 2011) disordered prediction results to obtain the final MoRF propensity score. MoRFCHiBiLight performs better than MoRFCHiBi with a maximum AUC of 0.868.
MoRFCHiBiWeb (Malhis et al., 2015; Malhis et al., 2016) predicts MoRF using Bayes rules combining the MoRFCHiBi and MoRFDC predictors (Malhis et al., 2015). MoRFDC used Bayes rules to integrate disorder scores and the conservativeness scores obtained by PSI-BLAST. The AUC of MoRFCHiBiWeb is up to 0.894.
Predict-MoRFs (Sharma et al., 2016) is the first predictor to predict MoRFs using protein sequence evolution information obtained from HMM profiles, and applied SVM with both radial basis function (RBF) and sigmoid kernels to calculate amino acid residue propensity scores. Predict-MoRFs outperformed other predictors with an AUC of 0.702. Since Predict-MoRFs uses the HHblits method (Remmert et al., 2011) to extract the information of the HMM, it runs faster than MoRFpred.
Later in 2018 (Sharma et al., 2018a), improved the Predict-MoRFs and named as MoRFPred-plus. MoRFPred-plus combines HMM profiles and the feature of physicochemical properties of amino acids by applying SVM models with two kernels, radial basis function (RBF) and sigmoid, to obtain final prediction results. The prediction results are better than other predictors, with a maximum AUC of 0.821.
2.3.2.2 Logistic regression
Logistic regression (Li et al., 1999) is a probabilistic classification algorithm, which has the advantages of short running time and high prediction performance, and is widely used in binary classification problems such as predicting protein disorder and ordered protein-protein interaction (Lin et al., 2004). DisoRDPbind (Peng and Kurgan, 2015) used this method to predict IDP-PPIS.
DisoRDPbind (Peng and Kurgan, 2015) input the features extracted from the protein sequences into a logistic regression model to obtain a propensity score for protein residues involved in disordered RNA, DNA, and protein binding, which was then combined with sequence comparison annotations to obtain a final propensity score. Regression coefficients for the input features in the logistic regression model are determined by the ridge. DisoRDPbind performs well with the AUC from 0.62 to 0.72.
2.3.2.3 Gradient boosted trees and conditional random field models
IDRBind (Wong and Gsponer, 2019a) predicted the binding sites of disordered proteins by combining gradient ascending tree and conditional random field model. IDRBind first used XGBoost from R packet (Chen and Guestrin, 2016) to train two classifiers to identify core and edge interface residues by gradient boosting tree method, and then integrated the predicted scores of the two classifiers by conditional random field (CRF) to form the final classification label. IDRBind performs well with an MCC of 0.31.
Gradient boosting tree performs well in solving classification problems, which can be implemented by R package XGBoost (Chen and Guestrin, 2016). Conditional random field (CRF) can be established by EDTSurf and Instant Meshes, which is a different indirect probabilistic graph model, including scoring components and adjacent components. The score component was composed of feature variables (i.e., the results from the gradient boosting tree), factors describing the compatibility of feature variables and label variables, and category deviation related factors. Adjacent components consist of pairwise factors that contain information from adjacent residues.
2.3.2.4 Random forest
The random forest (RF) model (Basu et al., 2017) is widely used in classification problems. The principle is that each decision tree in a random forest makes a judgment on the example to classify it as a positive or negative result, and the result with the higher number of votes is determined as the final result. The random forest model can be obtained through the Python package scikit-learn. Random Forest Model is applied by flDPnn (Hu et al., 2021) to predict IDP-PPIS.
FlDPnn (Hu et al., 2021) used multiple machine learning models to extract predicted feature sets about disorder and disorder function, then applied the disorder feature set to train deep feedforward neural networks to better predict disorder, and finally used a random forest model to combine the disorder function features extracted from the machine learning models and the disorder features obtained from the deep feedforward neural networks to predict the protein binding sites of IDP. flDPnn performs better than other predictors with an AUC of 0.79.
2.3.2.5 Neural network
Neural network is widely used in the study of protein-protein interaction. In this paper, we introduce five types of neural network models: Feedforward neural network (Zell, 1994), Bidirectional recurrent neural network (BRNN) (Mooney et al., 2012), Two-hidden layer neural network (Faraggi et al., 2009), Long Short-Term Memory (LSTM) (Hochreiter and Schmidhuber, 1997), and multi-task deep neural network (Zhang et al., 2021).
Feedforward neural networks (Zell, 1994; Sazli, 2006) are artificial neural networks in which information is transmitted unidirectionally from the input layer to the output layer via a hidden layer. They are classified into single-layer and multi-layer feedforward neural networks according to the presence or absence of hidden layers and are trained using a back propagation algorithm. Alpha-MoRFpred (Cheng et al., 2007) applied the feedforward neural network model to predict IDP-PPIS.
Alpha-MoRFpred (Cheng et al., 2007) used conditional probability method to select a representative feature set, and then constructed a feedforward neural network with a hidden layer, which was trained by the supervised learning algorithm in the neural network toolbox of Matlab, and finally predicted the α-MoRF involved in the combination. The sensitivity, specificity and accuracy of alpha-MoRFpred were close to 0.9.
Bidirectional recurrent neural network (BRNN) was applied to predict IDP-PPIS by SLiMPred (Mooney et al., 2012) and PepBindPred (Khan et al., 2013). BRNN (Schuster and Paliwal, 1997) was to obtain sequence information from the opposite direction to the output layer, that is, to predict disordered protein binding sites using the context information of protein sequences. Due to its recursive nature, BRNN had fewer free parameters.
The architecture of BRNN (Mooney et al., 2012; Khan et al., 2013):
where
SLiMPred (Mooney et al., 2012) applied BRNN to predict SLiMs using information on predicted secondary structure, structural motifs, solvent accessibility, and disorder prediction. The AUC was 0.69. Khan et al. (2013) developed another predictor for SLiMs, PepBindPred, which applied BRNN to predict SLiMs using information on sequence, predicted secondary structure, disorder scores, and Vina score. Adding Vina score improves the predictor performance. PepBindPred performed well with the AUC of 0.75.
SPINE-D (Faraggi et al., 2009; Zhang et al., 2012; Zhang et al., 2013) used predicted torsional angle fluctuations, predicted secondary structure and solvent accessibility as input features to train the neural network to predict IDP-PPIS. The neural network consists of a neural network with two hidden layers and a filtering layer, using a hyperbolic activation function and guided learning techniques. Each hidden layer contains 51 hidden neurons and a bias, and the filter layer contains 11 hidden neurons. SPINE-D performs well with the MCC of 0.15.
Two-hidden layer neural network architecture used by SPINE-D (Faraggi et al., 2009):
Calculation formula of output result of the hidden layers:
Where
The training process of a neural network is to compare the output results,
Error,
where
Both SPOT-Disorder (Hanson et al., 2016) and SPOT-Disorder2 (Hanson et al., 2019) applied Long Short-Term Memory (LSTM) networks (Hochreiter and Schmidhuber, 1997) to predict IDP-PPIS. LSTM networks are a modified recurrent neural network (CNN) capable of solving long time series problems, including single and bidirectional LSTM. The hidden layer contains one or more neurons capable of storing long term memory, and each neuron determines the input, output or forget constant error conveyor (CEC) through a gate function. Long short-term memory (LSTM) networks have been widely used to solve text classification problems (Singh et al., 2022).
SPOT-Disorder (Hanson et al., 2016) used deep bidirectional long-term and short-term memory cyclic neural network to improve prediction performance. The neural network includes bidirectional cyclic neural network (BRNN) composed of three hidden layers. In the first layer, there is cyclic feedforward layer with correction linear unit (ReLU) activation function. The second and third layers are composed of LSTM. The circulation layer contained 200 neurons and bias in each direction, and each neuron in each direction in the LSTM layer contained 200 memory blocks. The model is trained using back-propagation (BPTT) algorithm. Finally, the probability distribution is obtained using the softmax function. The MCC value is 0.309.
The neural network structure of SPOT-Disorder2 (Hanson et al., 2019) consists of IncReSeNet, LSTM and fully connected (FC) layers. IncReSeNet contains three parts: an initial path, a Squeeze-and-Excitation network and a residual connection, each consisting of a residual connection and two convolutional paths with three and one convolutional operations, respectively. SPOT-Disorder2 used similar features as SPOT-Disorder and applied hidden Markov model (HMM) features from HHblits. The MCC value is 0.155.
Based on the information derived from protein sequences, DeepDISOBind (Zhang et al., 2021) applied multi-task deep neural network to accurately predict the binding regions of disordered protein with DNA, RNA and protein. DeepDISOBind included shared layer, nucleic acid binding layer, protein binding layer, DNA binding layer and RNA binding layer. Various sequence feature would be input into a shared layer, which is compose of four different kernel convolutional neural network (CNN) and feedforward neural network (FNN) modules. The shared layer is connected to the protein-binding layer and the nucleic acid-binding layer. The nucleic acid-binding layer is connected to the DNA-binding layer and the RNA-binding layer. The output layer consists of three neurons using sigmoid transfer function, and finally the interaction propensity of disordered protein with RNA, DNA and protein is obtained. For IDP-PPIS prediction, DeepDISOBind outperformed other predictors with an AUC of 0.771.
2.3.3 Consensus
Consensus-based predictor (Fan and Kurgan, 2013) refers to the combination of multiple predictors by using different methods in a weighted manner. The purpose of using consensus predictor is to improve prediction accuracy.
OPAL (Sharma et al., 2018b) is a consensus predictor that combined two predictors, PROMIS (Sharma et al., 2018b) and MoRFCHiBi, to obtain the MoRF propensity score using the simple average method. The MoRFCHiBi predictor is described in detail above. The average method is the sum of the scores of all SVM models divided by the number of models used. The OPAL predictor performs well with an AUC of 0.795–0.870.
Sharma et al. (2018c) also developed the OPAL + predictor in 2018, which is an enhanced version of OPAL. OPAL + combined four independent SVM models with radial basis function (RBF) kernel for different length amino acid residues with MoRFpred-plus and MoRFCHiBi to obtain the final MoRF propensity score by average method. OPAL + performed better than other predictors with AUC of 0.820–0.876.
Another consensus-based predictor was also constructed by Sharma et al. (2019). Using the structural information obtained from the protein sequence, two independent SVM models with radial basis function (RBF) as the kernel were used to predict the MoRF located in the terminal and in the middle, respectively. Combined with the prediction scores of the two models, the final MoRF propensity score was obtained with AUCs 0.729–0.864. Then, the predictor was combined with MoRFpred-plus, PROMIS and MoRFCHiBi to form a consensus predictor that can obtain sequence information from different aspects and combine different algorithms. The final MoRF propensity score was obtained by averaging the scores of each predictor. The consensus predictor performs better than other predictors with AUC of 0.797–0.881.
DEPICTER (Barik et al., 2020) designed consensus predictors for predicting protein disorder and protein-binding IDR, respectively, and further improved the protein-binding IDR prediction performance by the disorder consensus predictor. The consensus predictor for IDR-PPIS combined three common predictors DisoRDPbind, ANCHOR2, and fMoRFpred. DEPICTER selected 54 features and applied four machine learning methods, Logistic Regression, Parsimonious Bayes, Random Forest, and Extreme Gradient Boosting Tree, to develop the consensus predictor. The prediction results obtained by DisoRDPbind, ANCHOR2 and fMoRFpred were transformed into feature vectors to be input to the consensus predictor to obtain new disordered proteins combining predicted propensity real values and dichotomous propensity. DEPICTER selected the best performing consensus predictor relying on extreme gradient boosting tree construction for testing, which outperformed the independent predictor with an AUC of 0.87.
The most recent consensus predictor HybridPBRpred (Zhang et al., 2020) combined the predictions of DisoRDPbind trained on disordered annotated data and the predictor SCRIBER trained on structured data to predict different types of protein binding residues. HybridPBRpred first normalized the scores of each predictor to [-1, 1], and for binary prediction, the prediction is protein binding residue score >0 otherwise score <0. Then, the final score was obtained in the following way: if at least one predictor predicts the residue to be a protein-binding residue, the higher score is chosen as the final score; if both predictors predict a non-protein-binding residue, the average of the two scores is used as the final score. The consensus predictor HybridPBRpred has improved IDP-PPIS prediction compared to non-consensus predictors with an AUC of 0.795.
3 Discussion
Disorder proteins lack stable structures but have many important functions through protein-protein interactions. There are a large number of studies have focused on identifying the protein binding sites of disordered proteins which will provide better functional annotations of disorder proteins. We scanned the literature since the publication of alpha-MoRFpred in 2007 and found that there has been a sharp increase in protein-binding IDR predictors, with 6–10 new predictors published every 3 years. These predictors continuously improve the prediction performance by applying different algorithms, screening more representative features or combining multiple models, and so on. The AUC is increased from 0.6 to 0.9. However, there might be still some limitations for current methods which can be improved from several directions.
We found that the existing predictors mainly curated the datasets from two large databases Disprot and PDB, especially there are many predictors on MoRF using the high-quality dataset from Disfani et al. Since this dataset contains a large number of immune-related proteins (Fang et al., 2013), the trained predictors might suffer from bias problems and neglect some potential binding sites.
In addition, most of the existing predictors are trained only for disordered protein data, but HybridPBRpred expands the benchmark dataset by combining predictors trained from structured protein datasets, allowing the predictors to improve the prediction performance in terms of protein binding sites for disordered proteins. There might be some common properties shared by different types of protein interfaces (Hou et al., 2017; Hou et al., 2019). Therefore, constructing benchmark datasets by expanding database sources such as from D2P2, MFIB, etc., and using more comprehensive datasets that include not only disordered protein data but also ordered protein data, could improve the performance of predictors in the future.
The existing predictors mainly focus on short binding regions such as MoRF (Fang et al., 2018) and SLiMs (Mooney et al., 2012), but there are still non-MoRF and non-SLiMs binding regions in disordered proteins. Therefore, predicting long disordered binding regions is an important hotspot in future development. In recent years, more and more predictors have improved the prediction performance by combining various features or algorithms. However, this approach easily causes high-dimensional feature space and the algorithm complexity and also reduce the computing efficiency (Malhis et al., 2016).
Salt bridges involved in disordered proteins have not yet been considered as a feature in all the predictors summarized. When disordered proteins participate in protein-protein interactions, ionic bonds are formed between oppositely charged amino acid side-chains, i.e., salt bridges. Studies (Basu and Biswas, 2018; Roy et al., 2022) have found that the formation of salt bridges contributes to the generation of local rigid structure of IDP, and triggers the disordered to ordered transition of IDP. For example, α -synuclein binds with tubulin to form an inter-chain salt bridge and mediate the transformation of protein conformation (Basu and Biswas, 2018). The arginines of FLCSSpike and the anions of Furin form dynamically interchangeable and durable salt bridge networks at the Spike-Furin binding interface, which triggers the transition from disorder to order (Roy et al., 2022). Therefore, we believe that the performance of the protein-binding sites predictor can be further improved by adding the feature of salt bridges in disordered proteins.
Since disordered proteins lack stable tertiary structures, most existing predictors are developed based on sequences, but disordered proteins still retain structural conformational properties such as secondary structure features, and it has been pointed out that transient secondary structure pre-structured motifs (PreSMos) (Kim and Han, 2021) exist in intrinsic disordered proteins and are involved in the development of various diseases by binding to corresponding targets. Furthermore, with the rapid development of structure prediction technology in the protein field, disorder predictors constructed based on AlphaFold2 (Wilson et al., 2022) structures were found to have potentials to identify disordered regions. Alphafold2 and RoseTTAFold have been successfully used to predict the structure of protein complexes (Bryant et al., 2022). The PLDDT value of Alphafold2 is commonly used to identify the structure of protein complex and the disordered regions. Tsaban et al. (2022) found that Alphafold2 recognized SLiM in the peptide-protein complex and also correctly characterized the conformational change upon protein binding. Akdel et al. (2021) proved that Alphafold2 was able to successfully predict the structure of complexes involving disordered proteins. Therefore, the construction of structure-based predictors may further enhance the protein binding site prediction performance of disordered proteins. In addition, inspired by HybridPBRpred, we can also improve the existing structure-based predictors to develop more comprehensive protein binding site predictors.
4 Conclusion
In this paper, we collected 30 predictors related to IDP-PPIS published up to January 2022, and described them in terms of three key aspects of the predictor construction process: databases, features, and algorithms. By summarizing the advantages and disadvantages of the existing predictors, we believe that the development of more comprehensive protein-binding site predictors by expanding the data sources, applying the features related to structural changes and binding to ordered protein-binding site predictors may further improve the performance of IDP-PPIS in the near future. In addition, since disordered proteins are involved in a variety of important physiological and biochemical processes using protein-protein interactions in various organisms, we also hope our review will help researchers to gain new ideas for solving various disease problems mediated by disordered proteins.
Author contributions
Conceptualization, RC, XL, and YY; methodology XL, RC, and XS; writing—original draft preparation, RC, XL, and YY; writing—review and editing, CW, RC, XL, and XS; supervision, project administration CW, DQ; funding acquisition, CW and DQ. All authors have read and agreed to the published version of the manuscript.
Funding
CW was supported by the Young Scholars Program of Shandong University (21,320,082,164,070). DQ was supported by Shandong Province Key R&D Program (2021SFGC0504).
Acknowledgments
Thanks to all those who have helped this article.
Conflict of interest
The authors declare that the research was conducted in the absence of any commercial or financial relationships that could be construed as a potential conflict of interest.
Publisher’s note
All claims expressed in this article are solely those of the authors and do not necessarily represent those of their affiliated organizations, or those of the publisher, the editors and the reviewers. Any product that may be evaluated in this article, or claim that may be made by its manufacturer, is not guaranteed or endorsed by the publisher.
References
Akdel, M., Pires, D. E. V., Porta Pardo, E., Jänes, J., Zalevsky, A. O., Mészáros, B., et al. (2021). A structural biology community assessment of AlphaFold 2 applications. bioRxiv. doi:10.1101/2021.09.26.461876
Altschul, S. (1997). Gapped BLAST and PSI-blast: A new generation of protein database search programs. Nucleic Acids Res. 25 (17), 3389–3402. doi:10.1093/nar/25.17.3389
Andreeva, A., Kulesha, E., Gough, J., and Murzin, A. G. (2019). The SCOP database in 2020: Expanded classification of representative family and superfamily domains of known protein structures. Nucleic Acids Res. 48 (D1), D376–D382. doi:10.1093/nar/gkz1064
Apweiler, R., Martin, M. J., O'Donovan, C., Magrane, M., Alam-Faruque, Y., Antunes, R., et al. (2010). The universal protein resource (UniProt) in 2010. Nucleic Acids Res. 38, D142–D148. doi:10.1093/nar/gkp846
Barik, A., Katuwawala, A., Hanson, J., Paliwal, K., Zhou, Y., and Kurgan, L. (2020). Depicter: Intrinsic disorder and disorder function prediction server. J. Mol. Biol. 432 (11), 3379–3387. doi:10.1016/j.jmb.2019.12.030
Basu, S., and Biswas, P. (2018). Salt-bridge dynamics in intrinsically disordered proteins: A trade-off between electrostatic interactions and structural flexibility. Biochimica Biophysica Acta (BBA) - Proteins Proteomics 1866 (5-6), 624–641. doi:10.1016/j.bbapap.2018.03.002
Basu, S., Söderquist, F., and Wallner, B. (2017). Proteus: A random forest classifier to predict disorder-to-order transitioning binding regions in intrinsically disordered proteins. J. Comput. Aided Mol. Des. 31 (5), 453–466. doi:10.1007/s10822-017-0020-y
Bateman, A., Martin, M-J., Orchard, S., Magrane, M., Agivetova, R., Ahmad, S., et al. (2021). UniProt: The universal protein knowledgebase in 2021. Nucleic Acids Res. 49 (D1), D480–D489. doi:10.1093/nar/gkaa1100
Brown, C. J., Takayama, S., Campen, A. M., Vise, P., Marshall, T. W., Oldfield, C. J., et al. (2002). Evolutionary rate heterogeneity in proteins with long disordered regions. J. Mol. Evol. 55 (1), 104–110. doi:10.1007/s00239-001-2309-6
Bryant, P., Pozzati, G., and Elofsson, A. (2022). Improved prediction of protein-protein interactions using AlphaFold2. Nat. Commun. 13 (1). doi:10.1038/s41467-022-28865-w
Burley, S. K., Berman, H. M., Bhikadiya, C., Bi, C., Chen, L., Costanzo, L. D., et al. (2018). Protein Data Bank: The single global archive for 3D macromolecular structure data. Nucleic Acids Res. 47 (D1), D520–D528. doi:10.1093/nar/gky949
Chandra, S., Chattopadhyay, G., and Varadarajan, R. (2021). Rapid identification of secondary structure and binding site residues in an intrinsically disordered protein segment. Front. Genet. 12. doi:10.3389/fgene.2021.755292
Chandra, S., Manjunath, K., Ashok, A., and Varadarajan, R. (2022) Inferring bound structure and residue specific contributions to binding energetics in the Intrinsically Disordered Protein, CcdA. bioRxiv. DOI: doi:doi:10.1101/2022.04.08.487678
Chang, C-C., and Lin, C-J. (2011). Libsvm. ACM Trans. Intell. Syst. Technol. 2 (3), 1–27. doi:10.1145/1961189.1961199
Chen, T., and Guestrin, C. (2016). “XGBoost,” in Proceedings of the 22nd ACM SIGKDD international conference on knowledge discovery and data mining, (ACM). doi:10.1145/2939672.2939785
Cheng, Y., Oldfield, C. J., Meng, J., Romero, P., Uversky, V. N., and Dunker, A. K. (2007). Mining α-helix-forming molecular recognition features with cross species sequence alignments. Biochemistry 46 (47), 13468–13477. doi:10.1021/bi7012273
Davey, N. E., Cowan, J. L., Shields, D. C., Gibson, T. J., Coldwell, M. J., and Edwards, R. J. (2012a). SLiMPrints: Conservation-based discovery of functional motif fingerprints in intrinsically disordered protein regions. Nucleic Acids Res. 40 (21), 10628–10641. doi:10.1093/nar/gks854
Davey, N. E., Van Roey, K., Weatheritt, R. J., Toedt, G., Uyar, B., Altenberg, B., et al. (2012b). Attributes of short linear motifs. Mol. Biosyst. 8 (1), 268–281. doi:10.1039/c1mb05231d
DeForte, S., and Uversky, V. N. (2016). Resolving the ambiguity: Making sense of intrinsic disorder when PDB structures disagree. Protein Sci. 25 (3), 676–688. doi:10.1002/pro.2864
Dinkel, H., Michael, S., Weatheritt, R. J., Davey, N. E., Van Roey, K., Altenberg, B., et al. (2011). ELM--the database of eukaryotic linear motifs. Nucleic Acids Res. 40 (D1), D242–D251. doi:10.1093/nar/gkr1064
Disfani, F. M., Hsu, W-L., Mizianty, M. J., Oldfield, C. J., Xue, B., Dunker, A. K., et al. (2012). MoRFpred, a computational tool for sequence-based prediction and characterization of short disorder-to-order transitioning binding regions in proteins. BIOINFORMATICS 28 (12), I75–I83. doi:10.1093/bioinformatics/bts209
Dogan, J., Mu, X., Engström, Å., and Jemth, P. (2013). The transition state structure for coupled binding and folding of disordered protein domains. Sci. Rep. 3 (1). doi:10.1038/srep02076
Dosztanyi, Z., Csizmok, V., Tompa, P., and Simon, I. (2005). IUPred: Web server for the prediction of intrinsically unstructured regions of proteins based on estimated energy content. Bioinformatics 21 (16), 3433–3434. doi:10.1093/bioinformatics/bti541
Dosztányi, Z., Csizmók, V., Tompa, P., and Simon, I. (2005). The pairwise energy content estimated from amino acid composition discriminates between folded and intrinsically unstructured proteins. J. Mol. Biol. 347 (4), 827–839. doi:10.1016/j.jmb.2005.01.071
Dosztanyi, Z., Meszaros, B., and Simon, I. (2009). Anchor: Web server for predicting protein binding regions in disordered proteins. Bioinformatics 25 (20), 2745–2746. doi:10.1093/bioinformatics/btp518
Dubreuil, B., Matalon, O., and Levy, E. D. (2019). Protein abundance biases the amino acid composition of disordered regions to minimize non-functional interactions. J. Mol. Biol. 431 (24), 4978–4992. doi:10.1016/j.jmb.2019.08.008
Dyson, H. J., and Wright, P. E. (2002). Coupling of folding and binding for unstructured proteins. Curr. Opin. Struct. Biol. 12 (1), 54–60. doi:10.1016/S0959-440X(02)00289-0
Edgar, R. C., and Batzoglou, S. (2006). Multiple sequence alignment. Curr. Opin. Struct. Biol. 16 (3), 368–373. doi:10.1016/j.sbi.2006.04.004
Efimov, A. V. (2017). Structural motifs in which β-strands are clipped together with the П-like module. Proteins 85 (10), 1925–1930. doi:10.1002/prot.25346
Fan, R. E., Chang, K. W., Hsieh, C. J., Wang, X. R., and Lin, C. J. (2008). Liblinear: A library for large linear classification. J. Mach. Learn. Res. 9, 1871–1874.
Fan, X., and Kurgan, L. (2013). Accurate prediction of disorder in protein chains with a comprehensive and empirically designed consensus. J. Biomol. Struct. Dyn. 32 (3), 448–464. doi:10.1080/07391102.2013.775969
Fang, C., Moriwaki, Y., Zhu, D., and Shimizu, K. (2018). “Identifying MoRFs in disordered proteins using enlarged conserved features,” in Proceedings of the 2018 6th international conference on bioinformatics and computational Biology (ACM). doi:10.1145/3194480.3198908
Fang, C., Noguchi, T., Tominaga, D., and Yamana, H. (2013). MFSPSSMpred: Identifying short disorder-to-order binding regions in disordered proteins based on contextual local evolutionary conservation. BMC Bioinforma. 14 (1). doi:10.1186/1471-2105-14-300
Fang, C., Noguchi, T., Yamana, H., and Sun, F. (2016). “Identifying protein short linear motifs by position-specific scoring matrix,” in Advances in swarm intelligence. Editors Y. Tan, Y. Shi, and L. Li (Cham: Springer International Publishing), 206–214. doi:10.1007/978-3-319-41009-8_22
Faraggi, E., Xue, B., and Zhou, Y. (2009). Improving the prediction accuracy of residue solvent accessibility and real-value backbone torsion angles of proteins by guided-learning through a two-layer neural network. Proteins 74 (4), 847–856. doi:10.1002/prot.22193
Fichó, E., Reményi, I., Simon, I., and Mészáros, B. (2017). Mfib: A repository of protein complexes with mutual folding induced by binding. Bioinformatics 33 (22), 3682–3684. doi:10.1093/bioinformatics/btx486
Fukuchi, S., Amemiya, T., Sakamoto, S., Nobe, Y., Hosoda, K., Kado, Y., et al. (2013). IDEAL in 2014 illustrates interaction networks composed of intrinsically disordered proteins and their binding partners. Nucl. Acids Res. 42 (D1), D320–D325. doi:10.1093/nar/gkt1010
Fukuchi, S., Sakamoto, S., Nobe, Y., Murakami, S. D., Amemiya, T., Hosoda, K., et al. (2011). Ideal: Intrinsically disordered proteins with extensive annotations and literature. Nucleic Acids Res. 40 (D1), D507–D511. doi:10.1093/nar/gkr884
Fuxreiter, M., Tompa, P., and Simon, I. (2007). Local structural disorder imparts plasticity on linear motifs. Bioinformatics 23 (8), 950–956. doi:10.1093/bioinformatics/btm035
Garnier, J., Gibrat, J-F., and Robson, B. (1996). [32] GOR method for predicting protein secondary structure from amino acid sequence. Methods Enzym., 540–553. Elsevier. doi:10.1016/s0076-6879(96)66034-0
Giri, R., Morrone, A., Toto, A., Brunori, M., and Gianni, S. (2013). Structure of the transition state for the binding of c-Myb and KIX highlights an unexpected order for a disordered system. Proc. Natl. Acad. Sci. U.S.A. 110 (37), 14942–14947. doi:10.1073/pnas.1307337110
Hamelryck, T. (2005). An amino acid has two sides: A new 2D measure provides a different view of solvent exposure. Proteins 59 (1), 38–48. doi:10.1002/prot.20379
Hanson, J., Paliwal, K., Litfin, T., Yang, Y., and Zhou, Y. (2018). Improving prediction of protein secondary structure, backbone angles, solvent accessibility and contact numbers by using predicted contact maps and an ensemble of recurrent and residual convolutional neural networks. Bioinformatics 35 (14), 2403–2410. doi:10.1093/bioinformatics/bty1006
Hanson, J., Paliwal, K. K., Litfin, T., and Zhou, Y. (2019). SPOT-Disorder2: Improved protein intrinsic disorder prediction by ensembled deep learning. Genomics, Proteomics Bioinforma. 17 (6), 645–656. doi:10.1016/j.gpb.2019.01.004
Hanson, J., Yang, Y., Paliwal, K., and Zhou, Y. (2016). Improving protein disorder prediction by deep bidirectional long short-term memory recurrent neural networks. Bioinformatics. doi:10.1093/bioinformatics/btw678
Heffernan, R., Paliwal, K., Lyons, J., Dehzangi, A., Sharma, A., Wang, J., et al. (2015). Improving prediction of secondary structure, local backbone angles and solvent accessible surface area of proteins by iterative deep learning. Sci. Rep. 5 (1). doi:10.1038/srep11476
Hochreiter, S., and Schmidhuber, J. (1997). Long short-term memory. Neural Comput. 9 (8), 1735–1780. doi:10.1162/neco.1997.9.8.1735
Hou, Q., De Geest, P. F. G., Griffioen, C. J., Abeln, S., Heringa, J., and Feenstra, K. A. (2019). SeRenDIP: SEquential REmasteriNg to DerIve profiles for fast and accurate predictions of PPI interface positions. Bioinformatics 35 (22), 4794–4796. doi:10.1093/bioinformatics/btz428
Hou, Q., De Geest, P. F. G., Vranken, W. F., Heringa, J., and Feenstra, K. A. (2017). Seeing the trees through the forest: Sequence-based homo- and heteromeric protein-protein interaction sites prediction using random forest. Bioinformatics 33 (10), btx005–1487. doi:10.1093/bioinformatics/btx005
Hu, G., Katuwawala, A., Wang, K., Wu, Z., Ghadermarzi, S., Gao, J., et al. (2021). flDPnn: Accurate intrinsic disorder prediction with putative propensities of disorder functions. Nat. Commun. 12 (1). doi:10.1038/s41467-021-24773-7
Jensen, M. R., Communie, G., Ribeiro, E. A., Martinez, N., Desfosses, A., Salmon, L., et al. (2011). Intrinsic disorder in measles virus nucleocapsids. Proc. Natl. Acad. Sci. U.S.A. 108 (24), 9839–9844. doi:10.1073/pnas.1103270108
Jones, D. T., and Cozzetto, D. (2014). DISOPRED3: Precise disordered region predictions with annotated protein-binding activity. Bioinformatics 31 (6), 857–863. doi:10.1093/bioinformatics/btu744
Jones, D. T. (1999). Protein secondary structure prediction based on position-specific scoring matrices 1 1Edited by G. Von Heijne. J. Mol. Biol. 292 (2), 195–202. doi:10.1006/jmbi.1999.3091
Katuwawala, A., Ghadermarzi, S., and Kurgan, L. (2019a). Computational prediction of functions of intrinsically disordered regions. Prog. Mol. Biol. Transl. Sci., 341–369. doi:10.1016/bs.pmbts.2019.04.006
Katuwawala, A., Peng, Z., Yang, J., and Kurgan, L. (2019b). Computational prediction of MoRFs, short disorder-to-order transitioning protein binding regions. Comput. Struct. Biotechnol. J. 17, 454–462. doi:10.1016/j.csbj.2019.03.013
Kawashima, S., Pokarowski, P., Pokarowska, M., Kolinski, A., Katayama, T., and Kanehisa, M. (2007). AAindex: Amino acid index database, progress report 2008. Nucleic Acids Res. 36, D202–D205. doi:10.1093/nar/gkm998
Khan, W., Duffy, F., Pollastri, G., Shields, D. C., and Mooney, C. (2013). Predicting binding within disordered protein regions to structurally characterised peptide-binding domains. PLOS ONE 8 (9), e72838. doi:10.1371/journal.pone.0072838
Kim, D-H., and Han, K-H. (2021). Target-binding behavior of IDPs via pre-structured motifs. Prog. Mol. Biol. Transl. Sci. 183, 187–247. doi:10.1016/bs.pmbts.2021.07.031
Kumar, M., Michael, S., Alvarado-Valverde, J., Mészáros, B., Sámano-Sánchez, H., Zeke, A., et al. (2021). The eukaryotic linear motif resource: 2022 release. Nucleic Acids Res. 50 (D1), D497–D508. doi:10.1093/nar/gkab975
Kussie, P. H., Gorina, S., Marechal, V., Elenbaas, B., Moreau, J., Levine, A. J., et al. (1996). Structure of the MDM2 oncoprotein bound to the p53 tumor suppressor transactivation domain. Science 274 (5289), 948–953. doi:10.1126/science.274.5289.948
Li, R., Romero, P., MDunker, A. K., Rani, M., and Obradovic, Z. (1999). Predicting protein disorder for N-, C-, and internal regions. Genome Inf. Ser. Workshop Genome Inf. 10, 30–40.,
Lin, N., Wu, B., Jansen, R., Gerstein, M., and Zhao, H. (2004). BMC Bioinforma. 5 (1), 154. doi:10.1186/1471-2105-5-154
Lindström, I., Andersson, E., and Dogan, J. (2018). The transition state structure for binding between TAZ1 of CBP and the disordered Hif-1α CAD. Sci. Rep. 8 (1). doi:10.1038/s41598-018-26213-x
Liu, J., and Wang, R. (2015). Classification of current scoring functions. J. Chem. Inf. Model. 55 (3), 475–482. doi:10.1021/ci500731a
Lobley, A., Swindells, M. B., Orengo, C. A., and Jones, D. T. (2007). Inferring function using patterns of native disorder in proteins. PLoS Comput. Biol. 3 (8), e162. doi:10.1371/journal.pcbi.0030162
Lyons, J., Dehzangi, A., Heffernan, R., Yang, Y., Zhou, Y., Sharma, A., et al. (2015). Advancing the accuracy of protein fold recognition by utilizing profiles from hidden Markov models. IEEE Trans.on Nanobioscience 14 (7), 761–772. doi:10.1109/TNB.2015.2457906
Malhis, N., and Gsponer, J. (2015). Computational identification of MoRFs in protein sequences. Bioinformatics 31 (11), 1738–1744. doi:10.1093/bioinformatics/btv060
Malhis, N., Jacobson, M., and Gsponer, J. (2016). MoRFchibi SYSTEM: Software tools for the identification of MoRFs in protein sequences. Nucleic Acids Res. 44 (W1), W488–W493. doi:10.1093/nar/gkw409
Malhis, N., Wong, E. T. C., Nassar, R., and Gsponer, J. (2015). Computational identification of MoRFs in protein sequences using hierarchical application of Bayes rule. PLOS ONE 10 (10), e0141603. doi:10.1371/journal.pone.0141603
McGuffin, L. J. (2008). Intrinsic disorder prediction from the analysis of multiple protein fold recognition models. Bioinformatics 24 (16), 1798–1804. doi:10.1093/bioinformatics/btn326
Mészáros, B., Erdős, G., and Dosztányi, Z. (2018). IUPred2A: Context-dependent prediction of protein disorder as a function of redox state and protein binding. Nucleic Acids Res. 46 (W1), W329–W337. doi:10.1093/nar/gky384
Mészáros, B., Simon, I., and Dosztányi, Z. (2009). Prediction of protein binding regions in disordered proteins. PLoS Comput. Biol. 5 (5), e1000376. doi:10.1371/journal.pcbi.1000376
Mészáros, B., Tompa, P., Simon, I., and Dosztányi, Z. (2007). Molecular principles of the interactions of disordered proteins. J. Mol. Biol. 372 (2), 549–561. doi:10.1016/j.jmb.2007.07.004
Minneci, F., Piovesan, D., Cozzetto, D., and Jones, D. T. (2013). FFPred 2.0: Improved homology-independent prediction of gene ontology terms for eukaryotic protein sequences. PLOS ONE 8 (5), e63754. doi:10.1371/journal.pone.0063754
Mizianty, M. J., Stach, W., Chen, K., Kedarisetti, K. D., Disfani, F. M., and Kurgan, L. (2010). Improved sequence-based prediction of disordered regions with multilayer fusion of multiple information sources. Bioinformatics 26 (18), i489–i496. doi:10.1093/bioinformatics/btq373
Mohan, A., Oldfield, C. J., Radivojac, P., Vacic, V., Cortese, M. S., Dunker, A. K., et al. (2006). Analysis of molecular recognition features (MoRFs). J. Mol. Biol. 362 (5), 1043–1059. doi:10.1016/j.jmb.2006.07.087
Mollica, L., Bessa, L. M., Hanoulle, X., Jensen, M. R., Blackledge, M., and Schneider, R. (2016). Binding mechanisms of intrinsically disordered proteins: Theory, simulation, and experiment. Front. Mol. Biosci. 3. doi:10.3389/fmolb.2016.00052
Monzon, A. M., Necci, M., Quaglia, F., Walsh, I., Zanotti, G., Piovesan, D., et al. (2020). Experimentally determined long intrinsically disordered protein regions are now abundant in the protein Data Bank. Ijms 21 (12), 4496. doi:10.3390/ijms21124496
Mooney, C., Pollastri, G., Shields, D. C., and Haslam, N. J. (2012). Prediction of short linear protein binding regions. J. Mol. Biol. 415 (1), 193–204. doi:10.1016/j.jmb.2011.10.025
Mooney, C., Vullo, A., and Pollastri, G. (2006). Protein structural motif prediction in multidimensional ø-ψ space leads to improved secondary structure prediction. J. Comput. Biol. 13 (8), 1489–1502. doi:10.1089/cmb.2006.13.1489
Oates, M. E., Romero, P., Ishida, T., Ghalwash, M., Mizianty, M. J., Xue, B., et al. (2012). D2P2: Database of disordered protein predictions. Nucleic Acids Res. 41 (D1), D508–D516. doi:10.1093/nar/gks1226
Oldfield, C. J., Cheng, Y., Cortese, M. S., Romero, P., Uversky, V. N., and Dunker, A. K. (2005). Coupled folding and binding with α-helix-forming molecular recognition elements. Biochemistry 44 (37), 12454–12470. doi:10.1021/bi050736e
Oldfield, C. J., Uversky, V. N., and Kurgan, L. (2018). Predicting functions of disordered proteins with MoRFpred. Methods Mol. Biol., 337–352. doi:10.1007/978-1-4939-8736-8_19
Peng, K., Radivojac, P., Vucetic, S., Dunker, A. K., and Obradovic, Z. (2006). Length-dependent prediction of protein intrinsic disorder. BMC Bioinforma. 7 (1). doi:10.1186/1471-2105-7-208
Peng, Z., and Kurgan, L. (2015). High-throughput prediction of RNA, DNA and protein binding regions mediated by intrinsic disorder. Nucleic Acids Res. 43 (18), e121. doi:10.1093/nar/gkv585
Peng, Z., Wang, C., Uversky, V. N., and Kurgan, L. (2016). Prediction of disordered RNA, DNA, and protein binding regions using DisoRDPbind. Methods Mol. Biol., 187–203. doi:10.1007/978-1-4939-6406-2_14
Piovesan, D., Necci, M., Escobedo, N., Monzon, A. M., Hatos, A., Mičetić, I., et al. (2020). MobiDB: Intrinsically disordered proteins in 2021. Nucleic Acids Res. 49 (D1), D361–D367. doi:10.1093/nar/gkaa1058
Pollastri, G., and McLysaght, A. (2004). Porter: A new, accurate server for protein secondary structure prediction. Bioinformatics 21 (8), 1719–1720. doi:10.1093/bioinformatics/bti203
Quaglia, F., Mészáros, B., Salladini, E., Hatos, A., Pancsa, R., Chemes, L. B., et al. (2021). DisProt in 2022: Improved quality and accessibility of protein intrinsic disorder annotation. Nucleic Acids Res. 50 (D1), D480–D487. doi:10.1093/nar/gkab1082
Radhakrishnan, I., Pérez-Alvarado, G. C., Parker, D., Dyson, H. J., Montminy, M. R., and Wright, P. E. (1997). Solution structure of the kix domain of CBP bound to the transactivation domain of CREB: A model for activator:coactivator interactions. Cell. 91 (6), 741–752. doi:10.1016/S0092-8674(00)80463-8
Remmert, M., Biegert, A., Hauser, A., and Söding, J. (2011). HHblits: Lightning-fast iterative protein sequence searching by HMM-HMM alignment. Nat. Methods 9 (2), 173–175. doi:10.1038/nmeth.1818
Romero, P., Obradovic, Z., Li, X., Garner, E. C., Brown, C. J., and Dunker, A. K. (2000). Sequence complexity of disordered protein. Proteins 42 (1), 38–48. doi:10.1002/1097-0134(20010101)42:1<38::aid-prot50>3.0.co;2-3
Roy, S., Ghosh, P., Bandyopadhyay, A., and Basu, S. (2022). Capturing a crucial ‘disorder-to-order transition’ at the heart of the coronavirus molecular pathology—triggered by highly persistent, interchangeable salt-bridges. Vaccines 10 (2), 301. doi:10.3390/vaccines10020301
Sazli, M. (2006). A brief review of feed-forward neural networks. Commun. Fac. Sci. Univ. Ankara 50, 11–17. doi:10.1501/0003168
Schad, E., Fichó, E., Pancsa, R., Simon, I., Dosztányi, Z., and Mészáros, B. (2017). Dibs: A repository of disordered binding sites mediating interactions with ordered proteins. BIOINFORMATICS 34 (3), 535–537. doi:10.1093/bioinformatics/btx640
Schlessinger, A., Yachdav, G., and Rost, B. (2006). PROFbval: Predict flexible and rigid residues in proteins. Bioinformatics 22 (7), 891–893. doi:10.1093/bioinformatics/btl032
Schuster, M., and Paliwal, K. K. (1997). Bidirectional recurrent neural networks. IEEE Trans. Signal Process. 45 (11), 2673–2681. doi:10.1109/78.650093
Sharma, A., Lyons, J., Dehzangi, A., and Paliwal, K. K. (2013). A feature extraction technique using bi-gram probabilities of position specific scoring matrix for protein fold recognition. J. Theor. Biol. 320, 41–46. doi:10.1016/j.jtbi.2012.12.008
Sharma, R., Bayarjargal, M., Tsunoda, T., Patil, A., and Sharma, A. (2018a). MoRFPred-plus: Computational identification of MoRFs in protein sequences using physicochemical properties and HMM profiles. J. Theor. Biol. 437, 9–16. doi:10.1016/j.jtbi.2017.10.015
Sharma, R., Kumar, S., Tsunoda, T., Patil, A., and Sharma, A. (2016). Predicting MoRFs in protein sequences using HMM profiles. BMC Bioinforma. 17 (S19). doi:10.1186/s12859-016-1375-0
Sharma, R., Raicar, G., Tsunoda, T., Patil, A., and Sharma, A. (2018b). Opal: Prediction of MoRF regions in intrinsically disordered protein sequences. Bioinformatics 34 (11), 1850–1858. doi:10.1093/bioinformatics/bty032
Sharma, R., Sharma, A., Patil, A., and Tsunoda, T. (2019). Discovering MoRFs by trisecting intrinsically disordered protein sequence into terminals and middle regions. BMC Bioinforma. 19 (S13). doi:10.1186/s12859-018-2396-7
Sharma, R., Sharma, A., Raicar, G., Tsunoda, T., and Patil, A. (2018c). OPAL+: Length‐Specific MoRF prediction in intrinsically disordered protein sequences. PROTEOMICS 19 (6), 1800058. doi:10.1002/pmic.201800058
Sickmeier, M., Hamilton, J. A., LeGall, T., Vacic, V., Cortese, M. S., Tantos, A., et al. (2007). DisProt: The database of disordered proteins. Nucleic Acids Res. 35, D786–D793. doi:10.1093/nar/gkl893
Singh, A., Dargar, S. K., Gupta, A., Kumar, A., Srivastava, A. K., Srivastava, M., et al. (2022). Evolving long short-term memory network-based text classification. Comput. Intell. Neurosci. 2022, 1–11. doi:10.1155/2022/4725639
Thompson, J. D., Higgins, D. G., and Gibson, T. J. (1994). Clustal W: Improving the sensitivity of progressive multiple sequence alignment through sequence weighting, position-specific gap penalties and weight matrix choice. Nucl. Acids Res. 22 (22), 4673–4680. doi:10.1093/nar/22.22.4673
Tsaban, T., Varga, J. K., Avraham, O., Ben-Aharon, Z., Khramushin, A., and Schueler-Furman, O. (2022). Harnessing protein folding neural networks for peptide-protein docking. Nat. Commun. 13 (1). doi:10.1038/s41467-021-27838-9
Uversky, V. N., and Dunker, A. K. (2010). Understanding protein non-folding. Biochimica Biophysica Acta (BBA) - Proteins Proteomics 1804 (6), 1231–1264. doi:10.1016/j.bbapap.2010.01.017
Uversky, V. N. (2018). Functions of short lifetime biological structures at large: The case of intrinsically disordered proteins. Briefings Funct. Genomics. doi:10.1093/bfgp/ely02310.1093/bfgp/ely023
Uversky, V. N., Oldfield, C. J., and Dunker, A. K. (2008). Intrinsically disordered proteins in human diseases: Introducing the D2 concept. Annu. Rev. Biophys. 37 (1), 215–246. doi:10.1146/annurev.biophys.37.032807.125924
Uversky, V. N., Oldfield, C. J., and Dunker, A. K. (2005). Showing your ID: Intrinsic disorder as an ID for recognition, regulation and cell signaling. J. Mol. Recognit. 18 (5), 343–384. doi:10.1002/jmr.747
Uversky, V. N. (2013). Unusual biophysics of intrinsically disordered proteins. Biochimica Biophysica Acta (BBA) - Proteins Proteomics 1834 (5), 932–951. doi:10.1016/j.bbapap.2012.12.008
Walsh, I., Martin, A. J. M., Di Domenico, T., and Tosatto, S. C. E. (2011). ESpritz: Accurate and fast prediction of protein disorder. Bioinformatics 28 (4), 503–509. doi:10.1093/bioinformatics/btr682
Wang, K., and Samudrala, R. (2006). Incorporating background frequency improves entropy-based residue conservation measures. BMC Bioinforma. 7 (1). doi:10.1186/1471-2105-7-385
Wang, Y., Guo, Y., Pu, X., and Li, M. (2017). A sequence-based computational method for prediction of MoRFs. RSC Adv. 7 (31), 18937–18945. doi:10.1039/c6ra27161h
Ward, J. J., Sodhi, J. S., McGuffin, L. J., Buxton, B. F., and Jones, D. T. (2004). Prediction and functional analysis of native disorder in proteins from the three kingdoms of life. J. Mol. Biol. 337 (3), 635–645. doi:10.1016/j.jmb.2004.02.002
Weatheritt, R. J., and Gibson, T. J. (2012). Linear motifs: Lost in (pre)translation. Trends Biochem. Sci. 37 (8), 333–341. doi:10.1016/j.tibs.2012.05.001
Wilson, C. J., Choy, W-Y., and Karttunen, M. (2022). AlphaFold2: A role for disordered protein/region prediction? Ijms 23 (9), 4591. doi:10.3390/ijms23094591
Wong, E. T. C., and Gsponer, J. (2019a). Predicting protein-protein interfaces that bind intrinsically disordered protein regions. J. Mol. Biol. 431 (17), 3157–3178. doi:10.1016/j.jmb.2019.06.010
Wong, E. T. C., and Gsponer, J. (2019b). Predicting protein-protein interfaces that bind intrinsically disordered protein regions. J. Mol. Biol. 431 (17), 3157–3178. doi:10.1016/j.jmb.2019.06.010
Xu, D., and Zhang, Y. (2009). Generating triangulated macromolecular surfaces by euclidean distance transform. PLOS ONE 4 (12), e8140. doi:10.1371/journal.pone.0008140
Xue, B., Dunbrack, R. L., Williams, R. W., Dunker, A. K., and Uversky, V. N. (2010a). PONDR-FIT: A meta-predictor of intrinsically disordered amino acids. Biochimica Biophysica Acta (BBA) - Proteins Proteomics 1804 (4), 996–1010. doi:10.1016/j.bbapap.2010.01.011
Xue, B., Dunker, A. K., and Uversky, V. N. (2010b). Retro-MoRFs: Identifying protein binding sites by normal and reverse alignment and intrinsic disorder prediction. Ijms 11 (10), 3725–3747. doi:10.3390/ijms11103725
Yan, J., Dunker, A. K., Uversky, V. N., and Kurgan, L. (2016). Molecular recognition features (MoRFs) in three domains of life. Mol. Biosyst. 12 (3), 697–710. doi:10.1039/c5mb00640f
Yuan, Z. (2005). Better prediction of protein contact number using a support vector regression analysis of amino acid sequence. BMC Bioinforma. 6 (1). doi:10.1186/1471-2105-6-248
Zhang, F., Zhao, B., Shi, W., Li, M., and Kurgan, L. (2021). DeepDISOBind: Accurate prediction of RNA-, DNA- and protein-binding intrinsically disordered residues with deep multi-task learning. Briefings Bioinforma. 23 (1). doi:10.1093/bib/bbab521
Zhang, J., Ghadermarzi, S., and Kurgan, L. (2020). Prediction of protein-binding residues: Dichotomy of sequence-based methods developed using structured complexes versus disordered proteins. BIOINFORMATICS 36 (18), 4729–4738. doi:10.1093/bioinformatics/btaa573
Zhang, T., Faraggi, E., Li, Z., and Zhou, Y. (2013). Intrinsically semi-disordered state and its role in induced folding and protein aggregation. Cell. Biochem. Biophys. 67 (3), 1193–1205. doi:10.1007/s12013-013-9638-0
Zhang, T., Faraggi, E., Xue, B., Dunker, A. K., Uversky, V. N., and Zhou, Y. (2012). SPINE-D: Accurate prediction of short and long disordered regions by a single neural-network based method. J. Biomol. Struct. Dyn. 29 (4), 799–813. doi:10.1080/073911012010525022
Keywords: intrinsically disordered protein (IDP), protein interaction sites prediction, machine learning, ML, protein functions, protein sequence
Citation: Chen R, Li X, Yang Y, Song X, Wang C and Qiao D (2022) Prediction of protein-protein interaction sites in intrinsically disordered proteins. Front. Mol. Biosci. 9:985022. doi: 10.3389/fmolb.2022.985022
Received: 03 July 2022; Accepted: 27 July 2022;
Published: 30 September 2022.
Edited by:
Sankar Basu, University of Calcutta, IndiaReviewed by:
Hitesh Kumar Waghwani, Affinivax Inc., United StatesGopinath Chattopadhyay, Indian Institute of Science, India
Copyright © 2022 Chen, Li, Yang, Song, Wang and Qiao. This is an open-access article distributed under the terms of the Creative Commons Attribution License (CC BY). The use, distribution or reproduction in other forums is permitted, provided the original author(s) and the copyright owner(s) are credited and that the original publication in this journal is cited, in accordance with accepted academic practice. No use, distribution or reproduction is permitted which does not comply with these terms.
*Correspondence: Cheng Wang, chengwang@sdu.edu.cn; Dongdong Qiao, qiaochina74@126.com
†These authors have contributed equally to this work