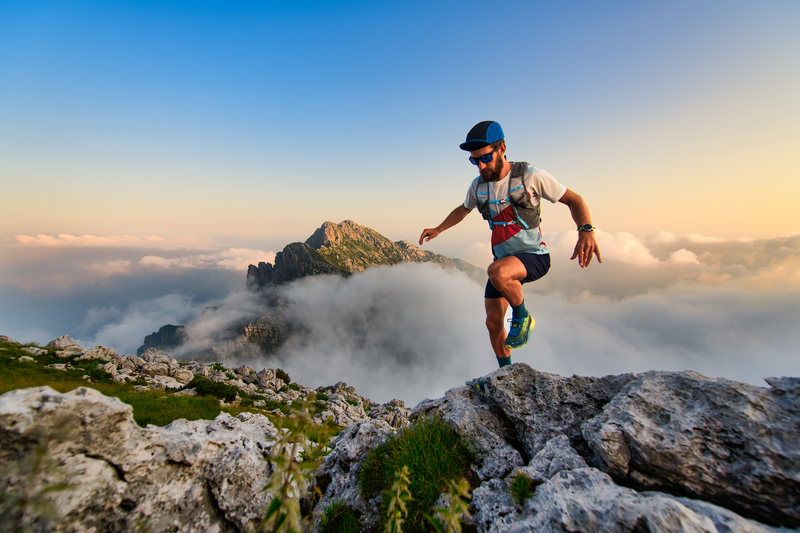
94% of researchers rate our articles as excellent or good
Learn more about the work of our research integrity team to safeguard the quality of each article we publish.
Find out more
ORIGINAL RESEARCH article
Front. Mol. Biosci. , 17 August 2022
Sec. RNA Networks and Biology
Volume 9 - 2022 | https://doi.org/10.3389/fmolb.2022.976878
This article is part of the Research Topic Volume II: The Role of Epithelial-Mesenchymal Transition (EMT)-Related Non-coding RNAs in Cancer View all 4 articles
Purpose: This study aimed to develop a prognostic indicator based on epithelial-mesenchymal transition (EMT)-related long noncoding RNAs (lncRNAs) and explore the function of EMT-related lncRNAs in malignant progression in lung adenocarcinoma (LUAD).
Materials and methods: A LUAD dataset was acquired from The Cancer Genome Atlas (TCGA) to identify prognostic EMT-related lncRNAs via differential expression analysis and univariate Cox regression analysis. Least Absolute Shrinkage and Selection Operator (LASSO) Cox regression analysis was utilized for variable selection and model construction. The EMT-related prognostic index (ERPI) was calculated according to the model and served as a classifier to divide LUAD individuals into high-ERPI and low-ERPI groups. A nomogram incorporating ERPI and clinicopathological variables was constructed. TCGA-LUAD, GSE50081, and GSE31210 were used to test the predictive capacity of the ERPI and nomogram. The characteristics of the tumor microenvironment (TME) were evaluated via the ESTIMATE, TIMER, and ssGSEA algorithms. Gene set variation analysis (GSVA) and ssGSEA were used to annotate the functions of the high-ERPI and low-ERPI groups. CCK8, transwell assay, wound-healing assay, and clone formation assay were conducted to clarify the biological functions of prognostic EMT-related lncRNAs.
Results: Ninety-seven differentially expressed EMT-related lncRNAs were identified, 15 of which were related to overall survival (OS). A prognostic signature was constructed based on 14 prognostic EMT-related lncRNAs to calculate the ERPI of each patient, and the predictive ability of ERPI was verified in TCGA, GSE50081, and GSE31210. The low-ERPI group survived longer and had a lower percentage of patients in advanced stage than the high-ERPI group. The nomogram had the highest predictive accuracy, followed by ERPI and stage. Patients with low ERPI had higher infiltration degree of immune cells and stronger immune responses than those with high ERPI. A series of in vitro experiments demonstrated that knockdown of LINC01138 dampened variability, proliferation, and motility of A549 and H460 cells.
Conclusion: Our study developed a prognostic classifier with robust prognostic performance and clarified the biological functions of LINC01138 in LUAD, aiding in making individual treatments for patients with LUAD and dissecting the mechanism of oncogenesis.
Lung cancer is not only the second most frequently diagnosed cancer but also the primary cause of cancer-related death worldwide, which accounts for 18% of cancer-related deaths (Sung et al., 2021). Although the survival of lung cancer has been extended in recent years due to advances in detection and treatment, only 22% of patients with lung cancer can survive 5 years or longer (Siegel et al., 2022). Lung cancer can be classified into diverse histological subtypes, and LUAD is the most common histological subtype, accounting for approximately 40% of lung cancer cases (Ganti et al., 2021).
EMT is known to play a fundamental role in embryogenesis, tissue regeneration, and malignant progression (Thiery et al., 2009; Pastushenko and Blanpain, 2019). EMT is characterized by the repressed expression of E-cadherin and increased expression of vimentin and can lead to changes in morphology, loss of cell polarity, reorganization of the cytoskeleton, and disassembly of cell-cell junctions. EMT is modulated by transcription factors, including the ZEB family, SNAIL, and TWIST1, which repress expression of epithelial markers and activate expression of mesenchymal markers. Interactions between TME and EMT are critical mechanisms in malignant progression. Constituents of TME can induce EMT by activating expression of EMT-related transcription factors or effector molecules, which in turn promote accumulation of immunosuppressive cells and expression of immunosuppressive molecules (Dongre and Weinberg, 2019). During the process of EMT, carcinoma cells enter a mesenchymal state and acquire malignant properties, including motility capacity, invasive behavior, metastasis, cancer stemness, and resistance to antitumor therapies (Pastushenko and Blanpain, 2019). A number of studies have underscored the significance of EMT in the development of lung cancer (Salazar et al., 2020; Yin et al., 2020; Deng et al., 2021).
Characterized as transcripts longer than 200 nucleotides, lncRNAs are important components of the transcriptome (Kopp and Mendell, 2018). Although lncRNAs are not translated into proteins, they regulate gene expression and control diverse cellular processes (Dangelmaier and Lal, 2020). Recent efforts have revealed the role of lncRNAs in tumorigenesis and malignant progression (Slack and Chinnaiyan, 2019; Wang et al., 2021a; Kim et al., 2021). Understanding the multiple functions of lncRNAs provides clues to dissect the mechanism of oncogenesis and aids in developing new strategies in cancer treatment. Many studies have indicated that lncRNAs can be potential prognostic predictors and therapeutic targets for patients with cancer (Wang et al., 2021a; Wu et al., 2021; Tang et al., 2022). The process of EMT can be regulated by lncRNAs such as H19, MALAT1, and MEG3 (Yang et al., 2020a; Hu et al., 2020; Ji et al., 2020; Wang et al., 2020; Ye et al., 2020; Liu et al., 2021), and EMT-related lncRNAs have been demonstrated to be prognostic indicators in cancer (Tuo et al., 2018; Xu et al., 2020; Wang et al., 2021b). However, the prognostic potential of EMT-related lncRNAs in LUAD still remains to be explored.
The objective of this study was to identify prognostic EMT-related lncRNAs in LUAD and develop a classifier that can predict outcomes for patients with LUAD. The LUAD dataset in TCGA was acquired to identify prognostic EMT-related lncRNAs and construct a prognostic model, and three LUAD cohorts were used to validate the prognostic value of the model. In vitro experiments were conducted to explore the role of LINC01138 in the development of lung cancer.
Three LUAD cohorts, including the TCGA-LUAD dataset, GSE50081 and GSE31210, with transcriptomic data and clinicopathological information were acquired from the TCGA (https://gdc-portal.nci.nih.gov/) database and Gene Expression Omnibus (GEO) database (https://www.ncbi.nlm.nih.gov/geo/) (Okayama et al., 2012; Yamauchi et al., 2012; Der et al., 2014). The TCGA-LUAD dataset consists of 535 LUAD samples and 59 peritumoural normal samples. After those followed up for less than 30 days or without complete clinicopathological information were removed, a total of 822 LUAD samples were used in our study, including 469 samples from the TCGA-LUAD dataset, 127 samples from GSE50081, and 226 samples from GSE31210. The clinicopathological parameters of the above datasets are provided in Supplementary Table S1. A total of 1594 EMT-related genes were obtained from the Gene Ontology website (http://geneontology.org/), Epithelial-Mesenchymal Transition Gene Database (http://dbemt.bioinfo-minzhao.org/download.cgi), Molecular Signatures Database (https://www.gsea-msigdb.org/), GeneCard database (https://www.genecards.org/), and OMIM database (https://omim.org/). Pearson correlation analysis was used to determine the correlation between the expression of lncRNAs and EMT-related genes, and lncRNAs that met the threshold of correlation coefficient |r| > 0.3 and p < 0.001 were considered EMT-related lncRNAs. All the EMT-related genes and EMT-related lncRNAs are listed in Supplementary Tables S2, S3, respectively.
The expression of all samples in the TCGA cohort was normalized, and the genes with a mean expression less than 5 in all samples were discarded. Differential expression analysis was carried out using the “edgeR” package to identify all the differentially expressed genes (DEGs) based on the threshold of false discovery rate (FDR) < 0.05 and |log2-fold change (FC)| > 1 (Robinson et al., 2010; McCarthy et al., 2012; Chen et al., 2016). Next, the genes from DEGs, EMT-related lncRNAs, GSE50081, and GSE31210 were intersected by the “VennDiagram” package to obtain differentially expressed EMT-related lncRNAs. The “ggplot2” and “pheatmap” packages were used to create volcano plot and heatmaps, respectively, to present the differential expression of the EMT-related lncRNAs.
The prognostic EMT-related lncRNAs were acquired from the differentially expressed EMT-related lncRNAs by univariate Cox regression analysis using the “survival” package. Lasso Cox regression analysis was executed using the “glmnet” package to screen prognostic EMT-related lncRNAs for model construction (Tibshirani, 1996; Friedman et al., 2010). A prognostic EMT-related model was constructed in the TCGA cohort based on candidate EMT-related lncRNAs and corresponding coefficients obtained in Lasso Cox regression analysis. Each patient with LUAD could obtain a score according to the prognostic model, which was named EMT-related prognostic index (ERPI). LUAD individuals in TCGA-LUAD, GSE50081, and GSE31210 were classified into high-ERPI and low-ERPI groups according to the median ERPI.
The predictive capacity of ERPI was validated in the TCGA cohort, GSE50081 and GSE31210. Principal component analysis (PCA) was utilized to confirm whether the high-ERPI and low-ERPI groups could be separated based on the prognostic EMT-related lncRNAs. Differences in the overall survival rate between the high-ERPI and low-ERPI groups were evaluated by Kaplan–Meier survival curves. The effect of the ERPI on survival was delineated via univariate and multivariate Cox regression analyses. The “survival” package was utilized to conduct the above survival analyses. (Therneau et al., 2000). The proportion of survivors in the high-ERPI and low-ERPI groups was calculated, and the ERPI between the surviving and nonsurviving patients was compared. The time-dependent receiver operating characteristic (ROC) curves were created using the “timeROC” package to show the predictive capacity of ERPI (Blanche et al., 2013). A nomogram based on the ERPI and clinicopathological variables was established to predict the survival probability of patients with LUAD. The C-index and area under the curve (AUC) were calculated to assess the predictive accuracy of the nomogram.
The distribution of clinicopathological subtypes between the high- and low-ERPI groups was compared using the chi-square test, which is presented in the form of a heatmap. Then, patients were divided into diverse subgroups according to the clinicopathological characteristics, and the ERPI between these subgroups was compared by using the Wilcoxon test.
A number of studies have reported interactions between EMT and the TME. Thus, we evaluated the relationship between TME characteristics and ERPI. The ESTIMATE algorithm was utilized to calculate the stromal score, immune score, and tumor purity, which represent the infiltration degree of stromal cells, immune cells, and tumor cells, respectively (Yoshihara et al., 2013). The infiltration degree of the major subtypes of immune cells in the TME was acquired via the TIMER and ssGSEA algorithms (Subramanian et al., 2005; Hänzelmann et al., 2013; Li et al., 2020). ssGSEA was also used to identify the related immune functions of the high- and low-ERPI groups. A study conducted a comprehensive analysis in 33 cancer types in the TCGA database to identify six immune subtypes associated with the immune environment and survival of patients with cancer (Thorsson et al., 2018). We assessed the link between the ERPI and the immune subtypes via the chi-square test, ggalluvial, and Kaplan–Meier survival curves.
GSVA was applied to identify the pathways and hallmarks that are associated with the ERPI by using the “GSVA” package (Hänzelmann et al., 2013). Enrichment scores were compared between the ERPI groups via the “Limma” package (Ritchie et al., 2015; Phipson et al., 2016). The pathways and hallmarks meeting the screening criterion of FDR <0.05 were considered to be differentially enriched between the two ERPI groups.
The lung cancer cell lines A549 and H460 were purchased from the China Center for Type Culture Collection and cultured in RPMI-1640 medium (HyClone, United States) with 10% FBS (Gibco, United States) at 37°C with 5% CO2.
The siRNA of LINC01138 was transfected into lung cancer cells using Lipofectamine 3,000 (Invitrogen, United States). The siRNA target sequence for LINC01138 was as follows: 5′-CCUCCUCUUCAGCCUACUU-3′.
Total cell RNA was extracted using an RNA extraction kit (TaKaRa, Japan) and reverse transcribed using Hi Script II QRT SuperMix (Vazyme, China). Next, the qRT-PCR was run with a Real-Time PCR System (7900HT, Applied Biosystems, United States). The primers used in this study were as follows: LINC01138, 5′-TATTTACGAAAGCTGAAAGCG-3' (forward) and 5′-CTGCATGGGATAGGAGAAAC-3' (reverse); GAPDH, 5′-GACAGTCAGCCGCATCTTCT-3' (forward) and 5′-GCGCCCAATACGACCAAATC-3' (reverse) (Zhang et al., 2018).
A total of 3,000 cells/well were seeded into 96-well plates overnight, and then the medium with 10% CCK8 (MedChem Express, United States) was incubated in each well for 1–2 h at different time points. OD values (wavelength of 450 nm) were detected by a microplate reader (BioTek, Winooski, VT, United States) to evaluate cell viability.
A total of 1,000 cells/well were seeded in 6-well plates. After approximately 2 weeks, cell clones were fixed with methanol, stained with 1% crystal violet and photographed.
Cells were seeded in 12-well plates, cultured for 12 h to 100% density, and then scratched with 10 μL pipette tips to create wounds. At 0 h and 48 h, images of wounds were captured, and the wound healing area was calculated using ImageJ software.
A total of 2 × 104 cells were seeded in the upper chambers with 200 μL serum-free medium, and 600 μL medium with 20% FBS was added to the lower chambers. Twenty-four hours later, the migrated cells were fixed, stained and photographed.
All analyses were completed using R software (version 4.1.0). Survival differences were compared by the log-rank test in Kaplan–Meier survival analysis. The Wilcoxon test was utilized to evaluate the difference in continuous variables between the two groups. Differences in the distribution of categorical variables between two groups were compared via the chi-square test. Pearson correlation analysis was used to evaluate the correlation between numeric variables. P values were two sided, and a p value < 0.05 was considered statistically significant.
Differential expression analysis in the TCGA cohort identified a total of 8507 differentially expressed genes (DEGs), among which 97 DEGs were EMT-related lncRNAs that could be found in the GEO datasets (Figure 1A). Ninety-seven differentially expressed EMT-related lncRNAs included 79 lncRNAs that were upregulated in LUAD and 18 lncRNAs that were downregulated in LUAD (Figures 1B,C). Fifteen differentially expressed EMT-related lncRNAs were found to be related to OS in univariate Cox regression analysis (Figure 1D), among which 14 lncRNAs (FENDRR, EP300-AS1, LINC00857, TMPO-AS1, LINC00460, LINC01138, PLAC4, SYNPR-AS1, LINC00996, MIR31HG, LINC01116, CASC15, ATP13A4-AS1, LINC01133) were selected by LASSO Cox regression analysis to construct a prognostic signature (Figure 1E). Expression of the 14 lncRNAs was displayed in Supplementary Figure S1. The prognostic model was presented as a formula, and the ERPI of all LUAD individuals was calculated based on the expression of 14 prognostic lncRNAs and the corresponding regression coefficients in LASSO Cox regression analysis (Supplementary Table S4).
FIGURE 1. Construction of a prognostic signature based on the expression of EMT-related lncRNAs. (A) Venn diagram of differentially expressed EMT-related lncRNAs in GEO and TCGA cohorts. (B) Volcano plot of differentially expressed EMT-related lncRNAs. (C) Heatmap of differentially expressed EMT-lncRNAs. (D) Forest plot of prognostic EMT-related lncRNAs. (E) Identification of key prognostic EMT-related lncRNAs via variable selection in LASSO Cox regression.
The predictive capacity of ERPI was validated in the TCGA-LUAD dataset which was used as the discovery cohort. The results of PCA demonstrated that the high-ERPI and low-ERPI groups could be separated based on the expression of 14 prognostic EMT-related lncRNAs (Figure 2A). The survival rate of the low-ERPI group was higher than that of the high-ERPI group (p < 0.001) (Figure 2B). The proportions of survivors in the high- and low-ERPI groups were 57% and 69%, respectively (Figure 2C). Compared to the survivors, the nonsurviving patients had an elevated ERPI (p < 0.001) (Figure 2D). Univariate (HR = 3.122, 95% confidence interval (CI): 2.446–3.985, p < 0.001) and multivariate Cox regression analyses (HR = 2.752, 95% CI: 2.150–3.523, p < 0.001) revealed that ERPI was an independent prognostic factor affecting survival adversely (Figures 2E,F). Time-dependent ROC curves demonstrated the high sensitivity and specificity of ERPI in predicting survival for patients with LUAD. The AUCs at 1, 3, and 5 years were 0.741, 0.684, and 0.647, respectively (Figure 2G).
FIGURE 2. Validation of the ERPI in the TCGA dataset. (A) Distribution of high- and low-ERPI groups in PCA based on the expression of 14 EMT-related lncRNAs in the prognostic model in TCGA. (B) Survival curves of the high- and low-ERPI groups in TCGA. (C) Proportion of dead and living patients in the high- and low-ERPI groups in TCGA. (D) ERPI of the dead and living patients. (E) Effect of ERPI and clinicopathological parameters on survival in univariate Cox regression analysis in TCGA. (F) Effect of ERPI and clinicopathological parameters on survival in multivariate Cox regression analysis in TCGA. (G) Time-dependent ROC curves of ERPI in TCGA.
Next, the results of survival analyses in the external cohorts GSE50081 and GSE31210 further confirmed the predictive power of ERPI. The expression of the prognostic EMT-related lncRNAs in the low-ERPI group was significantly different from that in the high-ERPI group in GSE50081 (Figure 3A) and GSE31210 (Figure 3B). The low-ERPI group survived longer than the high-ERPI group in both GSE50081 (p < 0.001) (Figure 3C) and GSE31210 (p < 0.001) (Figure 3D). In GSE50081, the proportion of survivors in the high- and low-ERPI groups was 37% and 67%, respectively (Figure 3E). In GSE31210, the proportion of survivors in the high- and low-ERPI groups was 77% and 94%, respectively (Figure 3G). The survivors had lower ERPI than the nonsurvivors in both GSE50081 (p = 0.0073) (Figure 3F) and GSE31210 (p < 0.001) (Figure 3H). ERPI is an indicator of unfavorable survival for patients with LUAD in GSE50081 (HR = 4.648, 95% CI: 1.892–11.418, p < 0.001) (Figure 4A) and GSE31210 (HR = 2.907, 95% CI: 1.770–4.774, p < 0.001) (Figure 4B). Additionally, the prognostic value of ERPI was not affected by clinical variables in GSE50081 (HR = 4.609, 95% CI: 1.766–12.024, p = 0.002) (Figure 4C) and GSE31210 (HR: 2.058, 95% CI: 1.173–3.610, p = 0.012) (Figure 4D). The AUCs at 1, 3, and 5 years in GSE50081 were 0.652, 0.711, and 0.682, respectively (Figure 4E). The AUCs at 1, 3, and 5 years in GSE31210 were 0.815, 0.736, and 0.709, respectively (Figure 4F).
FIGURE 3. Validation of ERPI in GSE50081 and GSE31210. (A) Distribution of high- and low-ERPI groups in PCA based on the expression of 14 EMT-related lncRNAs in the prognostic model in GSE50081. (B) Distribution of high- and low-ERPI groups in PCA based on the expression of 14 EMT-related lncRNAs in the prognostic model in GSE31210. (C) Survival curves of the high- and low-ERPI groups in GSE50081. (D) Survival curves of the high- and low-ERPI groups in GSE31210. (E) Proportion of dead and living patients in the high- and low-ERPI groups in GSE50081. (F) ERPI of the dead and living patients in GSE50081. (G) Proportion of dead and surviving patients in the high- and low-ERPI groups in GSE31210. (H) ERPI of the dead and surviving patients in GSE31210.
FIGURE 4. Validation of ERPI in GSE50081 and GSE31210. (A) Effect of ERPI and clinicopathological parameters on survival in univariate Cox regression analysis in GSE50081. (B) Effect of ERPI and clinicopathological parameters on survival in univariate Cox regression analysis in GSE31210. (C) Effect of ERPI and clinicopathological parameters on survival in multivariate Cox regression analysis in GSE50081. (D) Effect of ERPI and clinicopathological parameters on survival in multivariate Cox regression analysis in GSE31210. (E) Time-dependent ROC curves of ERPI in GSE50081. (F) Time-dependent ROC curves of ERPI in GSE31210.
Clinicopathological variables and ERPI are all prognostic factors that can predict survival, so we established a nomogram incorporating ERPI and clinicopathological variables to develop a tool for survival prediction with high predictive accuracy (Figures 5A,B). C-index and ROC curves demonstrated that the nomogram had the best prognostic performance and highest predictive accuracy, followed by ERPI and stage (Figures 5C–F).
FIGURE 5. Establishment and validation of the nomogram. (A) Nomogram incorporating ERPI and clinicopathological variables. (B) Calibration curves of the nomogram. (C) C-indexes of the nomogram, ERPI, and clinicopathological variables. (D) ROC curves of the nomogram, ERPI, and clinicopathological variables at 1 year. (E) ROC curves of the nomogram, ERPI, and clinicopathological variables at 3 years. (F) ROC curves of the nomogram, ERPI, and clinicopathological variables at 5 years.
Compared to the low-ERPI group, the high-ERPI group had an elevated proportion of male patients and patients with large primary tumors or lymph node metastasis (Figure 6A). Most of the patients in advanced stage were distributed in the high-ERPI group, indicating that ERPI was related to malignant progression. Meanwhile, when the patients were grouped according to the clinicopathological variables, increased ERPI was observed in male patients and patients in an advanced stage (Figures 6B–F). Although ERPI was correlated with large primary tumors and lymph node metastasis, no association was observed between ERPI and distant metastasis (Figures 6A,G).
FIGURE 6. Relationship between ERPI and clinicopathological variables. (A) Clinicopathological variables between the high- and low-ERPI groups. (B–G) ERPI between subgroups divided by clinicopathological variables.
Compared to the high-ERPI group, the low-ERPI group had a significantly higher proportion of stromal cells and immune cells and a lower proportion of tumor cells in the TME (Figures 7A–D). Immune cells are fundamental components of the TME and can be classified into diverse subtypes that have different roles in tumor progression and antitumor immunity. We analysed the relationship between immune cell subsets and the ERPI via the TIMER and ssGSEA algorithms. The low-ERPI group had not only higher abundance of CD8+ T cells, CD4+ T cells, B cells, and dendritic cells (DCs) but also higher infiltration degree of activated CD8+ T cells, B cells, and DCs than the high-ERPI group (Figures 7E,F). These results revealed that the infiltration and function of immune cells in the TME were suppressed in the high-ERPI group. Then, we analysed the immune subtypes identified in another study to further elucidate the relationship between ERPI and tumor immunity. C3 accounted for the majority of the low-ERPI group, whereas C1 and C2 were the most common immune subtypes in the high-ERPI group (Figures 8A,B). The survival rate of C3 was the highest among all the immune subtypes (Figure 8C).
FIGURE 7. TME characteristics in the high- and low-ERPI groups. (A–D) ESTIMATE score, tumor purity, stromal score, and immune score between the high- and low-ERPI groups. (E) Abundance of immune cell subsets acquired via TIMER between the high- and low-ERPI groups. (F) Abundance of immune cell subsets acquired via ssGSEA between the high- and low-ERPI groups.
FIGURE 8. Correlation between ERPI and immune subgroups. (A) Percentage of immune subgroups in the high- and low-ERPI groups. (B) Distribution of immune subgroups in the high- and low-ERPI groups. (C) Survival curves of the immune subgroups.
GSVA in hallmarks revealed that the high-ERPI group was associated with the activation of hallmarks such as oxidative phosphorylation, DNA repair, MYC targets, glycolysis, E2F targets, and mTORC1 signaling (Figure 9A). Regarding pathways, DNA replication, p53 signaling pathway, and mismatch repair were upregulated in the high-ERPI group, while the B-cell and T-cell receptor signaling pathways and pathways related to cell adhesion were enriched in the low-ERPI group (Figure 9B). The ssGSEA results indicated that immune processes, including the interferon response, parainflammation, chemokine receptor, T-cell co-inhibition, human leucocyte antigen, and antigen presenting cell co-inhibition, were activated in the low-ERPI group (Figure 9C). The ssGSEA results suggested that the low-ERPI group had a stronger immune response than the low-ERPI group.
FIGURE 9. Functional annotation of the ERPI groups. (A) Differences in hallmarks between the high- and low-ERPI groups in GSVA. (B) Differences in pathways between the high- and low-ERPI groups in GSVA. (C) Differences in immune processes between the high- and low-ERPI groups in ssGSEA.
Among these key signature lncRNAs, LINC01138 was reported to promote tumor progression in liver cancer, gastric cancer, glioma and kidney malignancy; however, whether LINC01138 promotes lung cancer progression remains unclear. Therefore, we investigated the effects of LINC01138 on malignant behaviors of lung cancer cells through transfection with its specific siRNA, and the interfering efficiency was assessed by RT-qPCR (Figure 10A). Then, we checked the effect of LINC01138 on the proliferation of lung cancer cells through CCK8 and clone formation assays. The results of the CCK8 assay showed that silencing LINC01138 dramatically decreased the viability of lung cancer cells (p < 0.001) (Figures 10B,C). In addition, the clone formation assay also indicated that silencing LINC01138 significantly suppressed clone formation of lung cancer cells (p < 0.01) (Figures 10D,E). These results suggested that silencing LINC01138 could inhibit lung cancer cells’ proliferation. Next, we further explored the role of LINC01138 in tumor metastasis through wound healing and transwell assays. The results showed that silencing LINC01138 markedly reduced wound healing ability of lung cancer cells (p < 0.01) (Figures 10F–H) as well as the migration capability (p < 0.01) (Figures 10I,J). The results demonstrated that silencing LINC01138 represses lung cancer metastasis in vitro. Altogether, the above results suggested that LINC01138 accelerates progression of lung cancer cells.
FIGURE 10. Knockdown of LINC01138 repressed viability, proliferation, and migration of A549 and H460 cells. (A) Expression of LINC01138 in A549 and H460 cells after its knockdown. (B,C) CCK-8 assay results showing viability of A549 and H460 cells after LINC01138 knockdown. (D,E) The proliferation of A549 and H460 cells evaluated using colony formation assay. (F–H) Migration capacity of A549 and H460 cells tested using wound-healing assay. (I,J) Migration capacity of A549 and H460 cells tested using Transwell assay. The scale bars represent 200 μm.
The 5-year survival rate of patients with lung cancer is 22%, which is as low as 6% in the case of distant metastasis (Siegel et al., 2022). Lung cancer consists of divergent histological subtypes, among which LUAD is the most frequently diagnosed subtype. Powerful tools for survival prediction are necessary to make precise clinical decisions and individual treatment strategies for patients with LUAD. EMT is a critical cellular program in malignant progression, and EMT-related lncRNAs have been proven to regulate the development of cancer. Nevertheless, few studies have explored the potential of EMT-related lncRNAs in survival prediction in LUAD.
To explore novel survival predictors, we analysed TCGA-LUAD data to identify prognostic EMT-related lncRNAs. A prognostic signature was constructed based on the expression of 14 EMT-related lncRNAs (FENDRR, EP300-AS1, LINC00857, TMPO-AS1, LINC00460, LINC01138, PLAC4, SYNPR-AS1, LINC00996, MIR31HG, LINC01116, CASC15, ATP13A4-AS1, and LINC01133). FENDRR, EP300-AS1, SYNPR-AS1, LINC00996, and ATP13A4-AS1 are tumor suppressor genes whose overexpression is associated with favorable outcome, whereas the other genes in the model are oncogenes. The results of the studies exploring the functions of prognostic EMT-related lncRNAs in cancers were consistent with our study. FENDRR was reported to be positively correlated with survival in prostate cancer and renal cell carcinoma (He et al., 2019; Liu et al., 2019). FENDRR has also been demonstrated to impair the invasion capacity of non-small cell lung cancer (NSCLC) and genitourinary system malignancies (Zhang et al., 2019a; Zhang et al., 2019b; Zhu et al., 2020). One study suggested that knockdown of LINC00857 suppressed the viability of bladder cancer cells and sensitized bladder cancer cells to cisplatin (Dudek et al., 2018). Silencing LINC00857 inhibited the malignant behaviors of colorectal cancer and pancreatic cancer (Chang et al., 2021; Li et al., 2021; Meng et al., 2021). Xia C et al. reported that LINC00857 enhanced EMT to promote the invasion capacity of hepatocellular carcinoma (Xia et al., 2018). LINC00460 acts as an oncogene in breast cancer and gastric cancer, and its high expression is correlated with unfavorable outcomes in patients with breast cancer (Zhang et al., 2019c; Zhu et al., 2019). A number of experimental studies revealed that LINC00460 promoted invasion and metastasis in various malignancies. LINC00460 was reported to promote the viability and migration of NSCLC (Zhao et al., 2019). Zhang J et al. observed that LINC00460 controls radiation sensitivity of colon cancer by modulating EMT (Zhang et al., 2020). Another study suggested that LINC00460 promoted EMT and metastatic potential of esophageal cancer (Cui et al., 2020). The role of LINC00460 as an oncogene was also reported in hepatocellular carcinoma, pancreatic cancer, kidney malignancy, and prostate cancer (Dong and Quan, 2019; Yang et al., 2020b; Cheng et al., 2021). Overexpression of MIR31HG was associated with malignant progression and high infiltration degree of immune cells in thyroid cancer (Chen et al., 2022). A meta-analysis suggested that overexpression of MIR31HG predicted poor survival and metastasis in respiratory system and digestive system tumors (Wei et al., 2022). MIR31HG acted as a predictor of survival and risk of recurrence for patients with colorectal cancer (Zhang et al., 2019d; Eide et al., 2019). Additionally, increased expression of MIR31HG has been demonstrated to promote tumor progression and reduce the efficacy of gefitinib in NSCLC (Wang et al., 2017; Ning et al., 2018; Dandan et al., 2019). MIR31HG also exhibited oncogenic properties in breast cancer and esophageal squamous cell carcinoma (Sun et al., 2018; Xin et al., 2021).
Studies have demonstrated that LINC01138 promotes malignant behavior in liver cancer, gastric cancer, glioma, and kidney malignancy (Li et al., 2018; Zhang et al., 2018; Dou et al., 2019; Xu et al., 2021). However, no mechanistic exploration has been made to clarify the role of LINC01138 in LUAD. In this study, we found that LINC01138 was upregulated in LUAD and that upregulation of LINC01138 was associated with poor survival by analyzing expression data in TCGA. Then, we conducted in vitro experiments to further clarify the exact role of LINC01138 in the progression of lung cancer. Silencing LINC01138 repressed viability, proliferation, and metastasis of A549 and H460 cells, indicating that LINC01138 was an oncogene in lung cancer. Our findings and other studies suggest that the oncogenic properties of LINC01138 may be universal across cancers.
Each patient could obtain a score named ERPI, which served as a classifier to distinguish patients with favorable prognosis from those with poor prognosis. ERPI is a reliable survival predictor for patients with LUAD, and the predictive capacity of ERPI is independent of clinicopathological factors. High ERPI is associated with advanced TNM stage, which also predicts poor survival for patients. Given that clinicopathological variables are also robust prognostic factors, we established a nomogram incorporating ERPI and clinicopathological variables to further improve the prognostic performance of these factors. The C-index and ROC curves demonstrated that the nomogram improved the prognostic performance of the ERPI and TNM stage. The nomogram can become a simple tool with high predictive accuracy for survival prediction in clinical practice. GSVA revealed the overactivation of mTORC1 signaling and MYC targets in the high-ERPI group. Dysregulation of mTOR signaling is associated with the initiation and progression of cancer, and overactivated mTORC1 signaling has been demonstrated to promote malignant behaviors of cancer cells (Kim et al., 2017). MYC is an oncogene supporting oncogenic processes and resistance to therapy (Massó-Vallés et al., 2020). MYC deregulation has been proven to accelerate oncogenesis and program stroma to induce immune suppression in lung cancer (Kortlever et al., 2017). These findings indicated that oncogenic processes are overactivated in the high-ERPI group.
Recruitment of immune cells and activation of immune processes are associated with not only tumor progression but also the efficacy of immune checkpoint inhibitors (ICIs), which are effective treatments for a subset of LUAD individuals (Quail and Joyce, 2013; Thommen et al., 2018; Helmink et al., 2020; Bagchi et al., 2021). Studies have suggested that EMT dampens the functions of immune cells, induces immune evasion, and promotes resistance to immunotherapy (Kudo-Saito et al., 2009; Akalay et al., 2013a; Akalay et al., 2013b). Mesenchymal carcinoma cells display increased resistance to immune attack and induce formation of immunosuppressive cells (Dongre et al., 2017). We analyzed the relationship between the ERPI and TME characteristics. The low-ERPI group had a higher percentage of immune cells and stromal cells and lower tumor purity than the high-ERPI group. ERPI was negatively related to the abundance of immune cells and activated immune cells, including CD8+ cells, B cells, and DCs. The abundance and function of these immune cell subsets are associated with survival outcome and response to ICIs. For example, single-cell sequencing analyses suggested that exhaustion of T cells correlated with survival outcome in LUAD individuals (Guo et al., 2018). One study identified a CD8+ T-cell subset whose high abundance was proven to be related to resistance to ICIs (Sanmamed et al., 2021). DCs are critical mediators of antigen presentation, promoting T-cell activity and immune control (Böttcher et al., 2018; Ahluwalia et al., 2021). One study suggested that DC deficiency led to dysfunction in immune surveillance (Hegde et al., 2020). DCs were demonstrated to be crucial targets of ICI treatment, dictating the efficacy of PD-L1 blockade (Mayoux et al., 2020). NSCLC individuals treated with atezolizumab with a high DC signature had improved OS compared with those with a low DC signature (Mayoux et al., 2020). High abundance of B cells was also reported to indicate favorable prognosis in patients with LUAD (Cui et al., 2021). Immune-related responses, including the IFN response and HLA, were enhanced in the low-ERPI group and are critical processes in antitumor immunity. Impaired HLA class I antigen processing reduces responsiveness to ICIs in lung cancer (Gettinger et al., 2017). Type I IFN responses can activate the immune system and repress tumor progression (Gozgit et al., 2021). IFN enhances the expression of immunomodulatory molecules to augment the activities of CD8+ T cells and NK cells (Edwards et al., 1985; Hervas-Stubbs et al., 2012; Zitvogel et al., 2015). IFN can also induce the formation of antitumorigenic cells and repress the accumulation of immunosuppressive cells (Duluc et al., 2009; Sisirak et al., 2012). High abundance of immune cells and highly activated immune processes may be one of the reasons why the low-ERPI group showed survival advantage over the high-ERPI group.
The present study has several limitations. The prognostic signature was constructed and tested retrospectively based on LUAD cohorts in public databases. A large and prospective LUAD cohort is necessary to validate the prognostic performance of ERPI and evaluate whether clinical application of ERPI can help patients acquire survival benefits. ICIs are effective treatments for a subset of patients with LUAD. Although the high- and low-ERPI groups had different characteristics of immune infiltration and immune response, the relationship between ERPI and efficacy of ICIs in LUAD was not explored due to lack of LUAD cohorts treated with ICIs. In addition, only the function of LINC01138 was clarified in lung cancer, whereas the mechanisms underlying other prognostic EMT-related lncRNAs have not been elucidated.
In conclusion, this study explored the prognostic EMT-related lncRNAs in LUAD to identify a prognostic signature that served as a classifier to predict outcome for patients with LUAD. We also constructed a nomogram that could be used as a simple tool for survival prediction. Additionally, our study clarified the biological function of LINC01138 in tumorigenesis, indicating that it could promote malignant behaviors in lung cancer. Our study lays foundation for stratification of LUAD individuals to achieve personalized treatment and contributes to understanding the role of EMT-related lncRNAs in LUAD.
The original contributions presented in the study are included in the article/Supplementary Material, further inquiries can be directed to the corresponding authors.
Conceptualization: LX, YH, and BL; design and methodology: LX, YH, QL, SW, ZF, ZT, and BL; data analysis: LX, YH, QL, SW, ZT, and LM; writing-original draft: LX and YH; writing-review and editing: ZT, XY, and BL; supervision: ZT, XY, and BL.
This work was supported by grants from the National Natural Science Foundation of China (grant numbers 81902619 and 82130092) and Wuhan Shuguang Project (grant number 2022020801020447).
We acknowledge the TCGA and GEO databases for providing their platforms and contributors for uploading their meaningful datasets.
The authors declare that the research was conducted in the absence of any commercial or financial relationships that could be construed as a potential conflict of interest.
All claims expressed in this article are solely those of the authors and do not necessarily represent those of their affiliated organizations, or those of the publisher, the editors and the reviewers. Any product that may be evaluated in this article, or claim that may be made by its manufacturer, is not guaranteed or endorsed by the publisher.
The Supplementary Material for this article can be found online at: https://www.frontiersin.org/articles/10.3389/fmolb.2022.976878/full#supplementary-material
EMT, epithelial-mesenchymal transition; lncRNAs, long noncoding RNAs; LUAD, lung adenocarcinoma; TCGA, The Cancer Genome Atlas; LASSO, Least Absolute Shrinkage and Selection Operator; ERPI, EMT-related prognostic index; TME, tumor microenvironment; ESTIMATE, Estimation of STromal and Immune cells in Malignant Tumours using Expression data; TIMER, Tumor Immune Estimation Resource; ssGSEA, single sample gene set enrichment analysis; GSVA, Gene set variation analysis; OS, overall survival; GEO, Gene Expression Omnibus; DEGs, differentially expressed genes; FDR, false discovery rate; FC, fold change; ROC, receiver operating characteristic; AUC, area under the curve; CI, confidence interval; DC, dendritic cell; NSCLC, non-small cell lung cancer; ICIs, immune checkpoint inhibitors.
Ahluwalia, P., Ahluwalia, M., Mondal, A. K., Sahajpal, N. S., Kota, V., Rojiani, M. V., et al. (2021). Natural killer cells and dendritic cells: Expanding clinical relevance in the non-small cell lung cancer (NSCLC) tumor microenvironment. Cancers (Basel) 13, 4037. doi:10.3390/cancers13164037
Akalay, I., Janji, B., Hasmim, M., Noman, M. Z., André, F., De Cremoux, P., et al. (2013). Epithelial-to-mesenchymal transition and autophagy induction in breast carcinoma promote escape from T-cell-mediated lysis. Cancer Res. 73, 2418–2427. doi:10.1158/0008-5472.Can-12-2432
Akalay, I., Janji, B., Hasmim, M., Noman, M. Z., Thiery, J. P., Mami-Chouaib, F., et al. (2013). EMT impairs breast carcinoma cell susceptibility to CTL-mediated lysis through autophagy induction. Autophagy 9, 1104–1106. doi:10.4161/auto.24728
Bagchi, S., Yuan, R., and Engleman, E. G. (2021). Immune checkpoint inhibitors for the treatment of cancer: Clinical impact and mechanisms of response and resistance. Annu. Rev. Pathol. 16, 223–249. doi:10.1146/annurev-pathol-042020-042741
Blanche, P., Dartigues, J. F., and Jacqmin-Gadda, H. (2013). Estimating and comparing time-dependent areas under receiver operating characteristic curves for censored event times with competing risks. Stat. Med. 32, 5381–5397. doi:10.1002/sim.5958
Böttcher, J. P., Bonavita, E., Chakravarty, P., Blees, H., Cabeza-Cabrerizo, M., Sammicheli, S., et al. (2018). NK cells stimulate recruitment of cDC1 into the tumor microenvironment promoting cancer immune control. Cell 172, 1022–1037. e1014. doi:10.1016/j.cell.2018.01.004
Chang, N., Cui, Y., Liang, X., Han, D., Zheng, X., Wu, A., et al. (2021). Long noncoding RNA LINC00857 promotes proliferation, migration, and invasion of colorectal cancer cell through miR-1306/vimentin Axis. Comput. Math. Methods Med. 2021, 5525763. doi:10.1155/2021/5525763
Chen, C., Qin, L., and Xiao, M. F. (2022). Long noncoding RNA LOC554202 predicts a poor prognosis and correlates with immune infiltration in thyroid cancer. Comput. Math. methods Med. 1, 1–12. doi:10.1155/2022/3585626
Chen, Y., Lun, A. T., and Smyth, G. K. (2016). From reads to genes to pathways: Differential expression analysis of RNA-seq experiments using rsubread and the edgeR quasi-likelihood pipeline. F1000Res. 5, 1438. doi:10.12688/f1000research.8987.2
Cheng, J., Lou, Y., and Jiang, K. (2021). Downregulation of long non-coding RNA LINC00460 inhibits the proliferation, migration and invasion, and promotes apoptosis of pancreatic cancer cells via modulation of the miR-320b/ARF1 axis. Bioengineered 12, 96–107. doi:10.1080/21655979.2020.1863035
Cui, C., Wang, J., Fagerberg, E., Chen, P. M., Connolly, K. A., Damo, M., et al. (2021). Neoantigen-driven B cell and CD4 T follicular helper cell collaboration promotes anti-tumor CD8 T cell responses. Cell 184, 6101–6118.e13. e6113. doi:10.1016/j.cell.2021.11.007
Cui, Y., Zhang, C., Lian, H., Xie, L., Xue, J., Yin, N., et al. (2020). LncRNA linc00460 sponges miR-1224-5p to promote esophageal cancer metastatic potential and epithelial-mesenchymal transition. Pathol. Res. Pract. 216, 153026. doi:10.1016/j.prp.2020.153026
Dandan, W., Jianliang, C., Haiyan, H., Hang, M., and Xuedong, L. (2019). Long noncoding RNA MIR31HG is activated by SP1 and promotes cell migration and invasion by sponging miR-214 in NSCLC. Gene 692, 223–230. doi:10.1016/j.gene.2018.12.077
Dangelmaier, E., and Lal, A. (2020). Adaptor proteins in long noncoding RNA biology. Biochim. Biophys. Acta. Gene Regul. Mech. 1863, 194370. doi:10.1016/j.bbagrm.2019.03.003
Deng, Q. D., Lei, X. P., Zhong, Y. H., Chen, M. S., Ke, Y. Y., Li, Z., et al. (2021). Triptolide suppresses the growth and metastasis of non-small cell lung cancer by inhibiting β-catenin-mediated epithelial-mesenchymal transition. Acta Pharmacol. Sin. 42, 1486–1497. doi:10.1038/s41401-021-00657-w
Der, S. D., Sykes, J., Pintilie, M., Zhu, C. Q., Strumpf, D., Liu, N., et al. (2014). Validation of a histology-independent prognostic gene signature for early-stage, non-small-cell lung cancer including stage IA patients. J. Thorac. Oncol. 9, 59–64. doi:10.1097/jto.0000000000000042
Dong, Y., and Quan, H. Y. (2019). Downregulated LINC00460 inhibits cell proliferation and promotes cell apoptosis in prostate cancer. Eur. Rev. Med. Pharmacol. Sci. 23, 6070–6078. doi:10.26355/eurrev_201907_18420
Dongre, A., Rashidian, M., Reinhardt, F., Bagnato, A., Keckesova, Z., Ploegh, H. L., et al. (2017). Epithelial-to-Mesenchymal transition contributes to immunosuppression in breast carcinomas. Cancer Res. 77, 3982–3989. doi:10.1158/0008-5472.Can-16-3292
Dongre, A., and Weinberg, R. A. (2019). New insights into the mechanisms of epithelial-mesenchymal transition and implications for cancer. Nat. Rev. Mol. Cell Biol. 20, 69–84. doi:10.1038/s41580-018-0080-4
Dou, G. X., Zhang, J. N., Wang, P., Wang, J. L., and Sun, G. B. (2019). Long Intergenic Non-Protein-Coding RNA 01138 Accelerates Tumor Growth and Invasion in Gastric Cancer by Regulating miR-1273e. Med. Sci. Monit. 25, 2141–2150. doi:10.12659/msm.914248
Dudek, A. M., van Kampen, J. G. M., Witjes, J. A., Kiemeney, L., and Verhaegh, G. W. (2018). LINC00857 expression predicts and mediates the response to platinum-based chemotherapy in muscle-invasive bladder cancer. Cancer Med. 7, 3342–3350. doi:10.1002/cam4.1570
Duluc, D., Corvaisier, M., Blanchard, S., Catala, L., Descamps, P., Gamelin, E., et al. (2009). Interferon-gamma reverses the immunosuppressive and protumoral properties and prevents the generation of human tumor-associated macrophages. Int. J. Cancer 125, 367–373. doi:10.1002/ijc.24401
Edwards, B. S., Merritt, J. A., Fuhlbrigge, R. C., and Borden, E. C. (1985). Low doses of interferon alpha result in more effective clinical natural killer cell activation. J. Clin. Invest. 75, 1908–1913. doi:10.1172/jci111905
Eide, P. W., Eilertsen, I. A., Sveen, A., and Lothe, R. A. (2019). Long noncoding RNA MIR31HG is a bona fide prognostic marker with colorectal cancer cell-intrinsic properties. Int. J. Cancer 144, 2843–2853. doi:10.1002/ijc.31998
Friedman, J., Hastie, T., and Tibshirani, R. (2010). Regularization paths for generalized linear models via coordinate descent. J. Stat. Softw. 33, 1–22. doi:10.18637/jss.v033.i01
Ganti, A. K., Klein, A. B., Cotarla, I., Seal, B., and Chou, E. (2021). Update of incidence, prevalence, survival, and initial treatment in patients with non-small cell lung cancer in the US. JAMA Oncol. 7, 1824–1832. doi:10.1001/jamaoncol.2021.4932
Gettinger, S., Choi, J., Hastings, K., Truini, A., Datar, I., Sowell, R., et al. (2017). Impaired HLA class I antigen processing and presentation as a mechanism of acquired resistance to immune checkpoint inhibitors in lung cancer. Cancer Discov. 7, 1420–1435. doi:10.1158/2159-8290.Cd-17-0593
Gozgit, J. M., Vasbinder, M. M., Abo, R. P., Kunii, K., Kuplast-Barr, K. G., Gui, B., et al. (2021). PARP7 negatively regulates the type I interferon response in cancer cells and its inhibition triggers antitumor immunity. Cancer Cell 39, 1214–1226. doi:10.1016/j.ccell.2021.06.018
Guo, X., Zhang, Y., Zheng, L., Zheng, C., Song, J., Zhang, Q., et al. (2018). Global characterization of T cells in non-small-cell lung cancer by single-cell sequencing. Nat. Med. 24, 978–985. doi:10.1038/s41591-018-0045-3
Hänzelmann, S., Castelo, R., and Guinney, J. (2013). GSVA: Gene set variation analysis for microarray and RNA-seq data. BMC Bioinforma. 14, 7. doi:10.1186/1471-2105-14-7
He, W., Zhong, G., Wang, P., Jiang, C., Jiang, N., Huang, J., et al. (2019). Downregulation of long noncoding RNA FENDRR predicts poor prognosis in renal cell carcinoma. Oncol. Lett. 17, 103–112. doi:10.3892/ol.2018.9624
Hegde, S., Krisnawan, V. E., Herzog, B. H., Zuo, C., Breden, M. A., Knolhoff, B. L., et al. (2020). Dendritic cell paucity leads to dysfunctional immune surveillance in pancreatic cancer. Cancer Cell 37, 289–307. e289. doi:10.1016/j.ccell.2020.02.008
Helmink, B. A., Reddy, S. M., Gao, J., Zhang, S., Basar, R., Thakur, R., et al. (2020). B cells and tertiary lymphoid structures promote immunotherapy response. Nature 577, 549–555. doi:10.1038/s41586-019-1922-8
Hervas-Stubbs, S., Mancheño, U., Riezu-Boj, J. I., Larraga, A., Ochoa, M. C., Alignani, D., et al. (2012). CD8 T cell priming in the presence of IFN-α renders CTLs with improved responsiveness to homeostatic cytokines and recall antigens: Important traits for adoptive T cell therapy. J. Immunol. 189, 3299–3310. doi:10.4049/jimmunol.1102495
Hu, X. T., Xing, W., Zhao, R. S., Tan, Y., Wu, X. F., Ao, L. Q., et al. (2020). HDAC2 inhibits EMT-mediated cancer metastasis by downregulating the long noncoding RNA H19 in colorectal cancer. J. Exp. Clin. Cancer Res. 39, 270. doi:10.1186/s13046-020-01783-9
Ji, Y., Feng, G., Hou, Y., Yu, Y., Wang, R., Yuan, H., et al. (2020). Long noncoding RNA MEG3 decreases the growth of head and neck squamous cell carcinoma by regulating the expression of miR-421 and E-cadherin. Cancer Med. 9, 3954–3963. doi:10.1002/cam4.3002
Kim, L. C., Cook, R. S., and Chen, J. (2017). mTORC1 and mTORC2 in cancer and the tumor microenvironment. Oncogene 36, 2191–2201. doi:10.1038/onc.2016.363
Kim, L. K., Park, S. A., Yang, Y., Kim, Y. T., Heo, T. H., Kim, H. J., et al. (2021). LncRNA SRA mediates cell migration, invasion, and progression of ovarian cancer via NOTCH signaling and epithelial-mesenchymal transition. Biosci. Rep. 41, BSR20210565. doi:10.1042/bsr20210565
Kopp, F., and Mendell, J. T. (2018). Functional classification and experimental dissection of long noncoding RNAs. Cell 172, 393–407. doi:10.1016/j.cell.2018.01.011
Kortlever, R. M., Sodir, N. M., Wilson, C. H., Burkhart, D. L., Pellegrinet, L., Brown Swigart, L., et al. (2017). Myc cooperates with ras by programming inflammation and immune suppression. Cell 171, 1301–1315. doi:10.1016/j.cell.2017.11.013
Kudo-Saito, C., Shirako, H., Takeuchi, T., and Kawakami, Y. (2009). Cancer metastasis is accelerated through immunosuppression during Snail-induced EMT of cancer cells. Cancer Cell 15, 195–206. doi:10.1016/j.ccr.2009.01.023
Li, T., Fu, J., Zeng, Z., Cohen, D., Li, J., Chen, Q., et al. (2020). TIMER2.0 for analysis of tumor-infiltrating immune cells. Nucleic Acids Res. 48, W509–W514. doi:10.1093/nar/gkaa407
Li, T., Zhao, H., Zhou, H., and Geng, T. (2021). LncRNA LINC00857 strengthens the malignancy behaviors of pancreatic adenocarcinoma cells by serving as a competing endogenous RNA for miR-340-5p to upregulate TGFA expression. PLoS One 16, e0247817. doi:10.1371/journal.pone.0247817
Li, Z., Zhang, J., Liu, X., Li, S., Wang, Q., Di, C., et al. (2018). The LINC01138 drives malignancies via activating arginine methyltransferase 5 in hepatocellular carcinoma. Nat. Commun. 9, 1572. doi:10.1038/s41467-018-04006-0
Liu, S. J., Dang, H. X., Lim, D. A., Feng, F. Y., and Maher, C. A. (2021). Long noncoding RNAs in cancer metastasis. Nat. Rev. Cancer 21, 446–460. doi:10.1038/s41568-021-00353-1
Liu, Y., Yang, B., Su, Y., Xiang, Q., and Li, Q. (2019). Downregulation of long noncoding RNA LINC00683 associated with unfavorable prognosis in prostate cancer based on TCGA. J. Cell. Biochem. 120, 14165–14174. doi:10.1002/jcb.28691
Massó-Vallés, D., Beaulieu, M. E., and Soucek, L. (2020). MYC, MYCL, and MYCN as therapeutic targets in lung cancer. Expert Opin. Ther. Targets 24, 101–114. doi:10.1080/14728222.2020.1723548
Mayoux, M., Roller, A., Pulko, V., Sammicheli, S., Chen, S., Sum, E., et al. (2020). Dendritic cells dictate responses to PD-L1 blockade cancer immunotherapy. Sci. Transl. Med. 12, eaav7431. doi:10.1126/scitranslmed.aav7431
McCarthy, D. J., Chen, Y., and Smyth, G. K. (2012). Differential expression analysis of multifactor RNA-Seq experiments with respect to biological variation. Nucleic Acids Res. 40, 4288–4297. doi:10.1093/nar/gks042
Meng, X., Deng, Y., He, S., Niu, L., and Zhu, H. (2021). m(6 A-mediated upregulation of LINC00857 promotes pancreatic cancer tumorigenesis by regulating the miR-150-5p/E2F3 Axis. Front. Oncol. 11, 629947. doi:10.3389/fonc.2021.629947
Ning, P., Wu, Z., Hu, A., Li, X., He, J., Gong, X., et al. (2018). Integrated genomic analyses of lung squamous cell carcinoma for identification of a possible competitive endogenous RNA network by means of TCGA datasets. PeerJ 6, e4254. doi:10.7717/peerj.4254
Okayama, H., Kohno, T., Ishii, Y., Shimada, Y., Shiraishi, K., Iwakawa, R., et al. (2012). Identification of genes upregulated in ALK-positive and EGFR/KRAS/ALK-negative lung adenocarcinomas. Cancer Res. 72, 100–111. doi:10.1158/0008-5472.Can-11-1403
Pastushenko, I., and Blanpain, C. (2019). EMT transition states during tumor progression and metastasis. Trends Cell Biol. 29, 212–226. doi:10.1016/j.tcb.2018.12.001
Phipson, B., Lee, S., Majewski, I. J., Alexander, W. S., and Smyth, G. K. (2016). Robust hyperparameter estimation protects against hypervariable genes and improves power to detect differential expression. Ann. Appl. Stat. 10, 946–963. doi:10.1214/16-aoas920
Quail, D. F., and Joyce, J. A. (2013). Microenvironmental regulation of tumor progression and metastasis. Nat. Med. 19, 1423–1437. doi:10.1038/nm.3394
Ritchie, M. E., Phipson, B., Wu, D., Hu, Y., Law, C. W., Shi, W., et al. (2015). Limma powers differential expression analyses for RNA-sequencing and microarray studies. Nucleic Acids Res. 43, e47. doi:10.1093/nar/gkv007
Robinson, M. D., McCarthy, D. J., and Smyth, G. K. (2010). edgeR: a Bioconductor package for differential expression analysis of digital gene expression data. Bioinforma. Oxf. Engl. 26, 139–140. doi:10.1093/bioinformatics/btp616
Salazar, Y., Zheng, X., Brunn, D., Raifer, H., Picard, F., Zhang, Y., et al. (2020). Microenvironmental Th9 and Th17 lymphocytes induce metastatic spreading in lung cancer. J. Clin. Invest. 130, 3560–3575. doi:10.1172/jci124037
Sanmamed, M. F., Nie, X., Desai, S. S., Villaroel-Espindola, F., Badri, T., Zhao, D., et al. (2021). A burned-out CD8(+) T-cell subset expands in the tumor microenvironment and curbs cancer immunotherapy. Cancer Discov. 11, 1700–1715. doi:10.1158/2159-8290.Cd-20-0962
Siegel, R. L., Miller, K. D., Fuchs, H. E., and Jemal, A. (2022). Cancer statistics, 2022. Ca. Cancer J. Clin. 2022 (72), 7–33. doi:10.3322/caac.21708
Sisirak, V., Faget, J., Gobert, M., Goutagny, N., Vey, N., Treilleux, I., et al. (2012). Impaired IFN-α production by plasmacytoid dendritic cells favors regulatory T-cell expansion that may contribute to breast cancer progression. Cancer Res. 72, 5188–5197. doi:10.1158/0008-5472.Can-11-3468
Slack, F. J., and Chinnaiyan, A. M. (2019). The role of non-coding RNAs in oncology. Cell 179, 1033–1055. doi:10.1016/j.cell.2019.10.017
Subramanian, A., Tamayo, P., Mootha, V. K., Mukherjee, S., Ebert, B. L., Gillette, M. A., et al. (2005). Gene set enrichment analysis: A knowledge-based approach for interpreting genome-wide expression profiles. Proc. Natl. Acad. Sci. U. S. A. 102, 15545–15550. doi:10.1073/pnas.0506580102
Sun, K., Zhao, X., Wan, J., Yang, L., Chu, J., Dong, S., et al. (2018). The diagnostic value of long non-coding RNA MIR31HG and its role in esophageal squamous cell carcinoma. Life Sci. 202, 124–130. doi:10.1016/j.lfs.2018.03.050
Sung, H., Ferlay, J., Siegel, R. L., Laversanne, M., Soerjomataram, I., Jemal, A., et al. (2021). Global cancer statistics 2020: GLOBOCAN estimates of incidence and mortality worldwide for 36 cancers in 185 countries. Ca. Cancer J. Clin. 71, 209–249. doi:10.3322/caac.21660
Tang, R., Wu, Z., Rong, Z., Xu, J., Wang, W., Zhang, B., et al. (2022). Ferroptosis-related lncRNA pairs to predict the clinical outcome and molecular characteristics of pancreatic ductal adenocarcinoma. Brief. Bioinform. 23, bbab388. doi:10.1093/bib/bbab388
Therneau, T. M., and Grambsch, P. M., SpringerLink (2000). Modeling survival data: Extending the Cox model. New York: Springer.
Thiery, J. P., Acloque, H., Huang, R. Y., and Nieto, M. A. (2009). Epithelial-mesenchymal transitions in development and disease. Cell 139, 871–890. doi:10.1016/j.cell.2009.11.007
Thommen, D. S., Koelzer, V. H., Herzig, P., Roller, A., Trefny, M., Dimeloe, S., et al. (2018). A transcriptionally and functionally distinct PD-1(+) CD8(+) T cell pool with predictive potential in non-small-cell lung cancer treated with PD-1 blockade. Nat. Med. 24, 994–1004. doi:10.1038/s41591-018-0057-z
Thorsson, V., Gibbs, D. L., Brown, S. D., Wolf, D., Bortone, D. S., Ou Yang, T. H., et al. (2018). The immune landscape of cancer. Immunity 48, 812–830. e814. doi:10.1016/j.immuni.2018.03.023
Tibshirani, R. (1996). Regression shrinkage and selection via the Lasso. J. R. Stat. Soc. Ser. B 58, 267–288. doi:10.1111/j.2517-6161.1996.tb02080.x
Tuo, Z., Zhang, J., and Xue, W. (2018). LncRNA TP73-AS1 predicts the prognosis of bladder cancer patients and functions as a suppressor for bladder cancer by EMT pathway. Biochem. Biophys. Res. Commun. 499, 875–881. doi:10.1016/j.bbrc.2018.04.010
Wang, B., Jiang, H., Wang, L., Chen, X., Wu, K., Zhang, S., et al. (2017). Increased MIR31HG lncRNA expression increases gefitinib resistance in non-small cell lung cancer cell lines through the EGFR/PI3K/AKT signaling pathway. Oncol. Lett. 13, 3494–3500. doi:10.3892/ol.2017.5878
Wang, L. F., Wu, L. P., and Wen, J. D. (2021). LncRNA AC079630.4 expression associated with the progression and prognosis in lung cancer. Aging 13, 18658–18668. doi:10.18632/aging.203310
Wang, P., Chen, Y., Zheng, Y., Fu, Y., and Ding, Z. (2021). Identification of epithelial-mesenchymal transition- (EMT-) related LncRNA for prognostic prediction and risk stratification in esophageal squamous cell carcinoma. Dis. Markers 2021, 5340240. doi:10.1155/2021/5340240
Wang, R., Lu, X., and Yu, R. (2020). lncRNA MALAT1 promotes EMT process and cisplatin resistance of oral squamous cell carcinoma via PI3K/AKT/m-TOR signal pathway. Onco. Targets. Ther. 13, 4049–4061. doi:10.2147/ott.S251518
Wei, Y., Zhai, Y., Liu, X., Jin, S., Zhang, L., Wang, C., et al. (2022). Long non-coding RNA MIR31HG as a prognostic predictor for malignant cancers: A meta- and bioinformatics analysis. J. Clin. Lab. Anal. 36, e24082. doi:10.1002/jcla.24082
Wu, H., Zhang, Z. Y., Zhang, Z., Xiao, X. Y., Gao, S. L., Lu, C., et al. (2021). Prediction of bladder cancer outcome by identifying and validating a mutation-derived genomic instability-associated long noncoding RNA (lncRNA) signature. Bioengineered 12, 1725–1738. doi:10.1080/21655979.2021.1924555
Xia, C., Zhang, X. Y., Liu, W., Ju, M., Ju, Y., Bu, Y. Z., et al. (2018). LINC00857 contributes to hepatocellular carcinoma malignancy via enhancing epithelial-mesenchymal transition. J. Cell. Biochem. 120, 7970–7977. doi:10.1002/jcb.28074
Xin, C., Bi, X., Xiao, C., and Dong, L. (2021). MIR31HG regulates the proliferation, migration and invasion of breast cancer by regulating the expression of POLDIP2. J. B.U.ON, official J. Balkan Union Oncol. 26, 459–465.
Xu, C., Yin, H., Jiang, X., and Sun, C. (2021). Silencing long noncoding RNA LINC01138 inhibits aerobic glycolysis to reduce glioma cell proliferation by regulating the microRNA-375/SP1 axis. Mol. Med. Rep. 24, 846. doi:10.3892/mmr.2021.12486
Xu, W., Zhou, G., Wang, H., Liu, Y., Chen, B., Chen, W., et al. (2020). Circulating lncRNA SNHG11 as a novel biomarker for early diagnosis and prognosis of colorectal cancer. Int. J. Cancer 146, 2901–2912. doi:10.1002/ijc.32747
Yamauchi, M., Yamaguchi, R., Nakata, A., Kohno, T., Nagasaki, M., Shimamura, T., et al. (2012). Epidermal growth factor receptor tyrosine kinase defines critical prognostic genes of stage I lung adenocarcinoma. PLoS One 7, e43923. doi:10.1371/journal.pone.0043923
Yang, J., Li, K., Chen, J., Hu, X., Wang, H., Zhu, X., et al. (2020). Long noncoding RNA LINC00460 promotes hepatocellular carcinoma progression via regulation of miR-342-3p/AGR2 Axis. Onco. Targets. Ther. 13, 1979–1991. doi:10.2147/ott.S239258
Yang, Z., Bian, E., Xu, Y., Ji, X., Tang, F., Ma, C., et al. (2020). Meg3 induces EMT and invasion of glioma cells via autophagy. Onco. Targets. Ther. 13, 989–1000. doi:10.2147/ott.S239648
Ye, W., Ma, J., Wang, F., Wu, T., He, M., Li, J., et al. (2020). LncRNA MALAT1 regulates miR-144-3p to facilitate epithelial-mesenchymal transition of lens epithelial cells via the ROS/NRF2/Notch1/Snail pathway. Oxidative Med. Cell. Longev. 2020, 8184314. doi:10.1155/2020/8184314
Yin, H., Wang, X., Zhang, X., Zeng, Y., Xu, Q., Wang, W., et al. (2020). UBE2T promotes radiation resistance in non-small cell lung cancer via inducing epithelial-mesenchymal transition and the ubiquitination-mediated FOXO1 degradation. Cancer Lett. 494, 121–131. doi:10.1016/j.canlet.2020.06.005
Yoshihara, K., Shahmoradgoli, M., Martínez, E., Vegesna, R., Kim, H., Torres-Garcia, W., et al. (2013). Inferring tumour purity and stromal and immune cell admixture from expression data. Nat. Commun. 4, 2612. doi:10.1038/ncomms3612
Zhang, G., Wang, Q., Zhang, X., Ding, Z., and Liu, R. (2019). LncRNA FENDRR suppresses the progression of NSCLC via regulating miR-761/TIMP2 axis. Biomed. Pharmacother. = Biomedecine Pharmacother. 118, 109309. doi:10.1016/j.biopha.2019.109309
Zhang, H., Wang, Z., Wu, J., Ma, R., and Feng, J. (2019). Long noncoding RNAs predict the survival of patients with colorectal cancer as revealed by constructing an endogenous RNA network using bioinformation analysis. Cancer Med. 8, 863–873. doi:10.1002/cam4.1813
Zhang, J., Ding, L., Sun, G., Ning, H., and Huang, R. (2020). Suppression of LINC00460 mediated the sensitization of HCT116 cells to ionizing radiation by inhibiting epithelial-mesenchymal transition. Toxicol. Res. 9, 107–116. doi:10.1093/toxres/tfaa010
Zhang, S., Xu, J., Wang, H., and Guo, H. (2019). Downregulation of long noncoding RNA LINC00460 expression suppresses tumor growth in vitro and in vivo in gastric cancer. Cancer Biomark. 24, 429–437. doi:10.3233/cbm-182177
Zhang, X., Wu, J., Wu, C., Chen, W., Lin, R., Zhou, Y., et al. (2018). The LINC01138 interacts with PRMT5 to promote SREBP1-mediated lipid desaturation and cell growth in clear cell renal cell carcinoma. Biochem. Biophys. Res. Commun. 507, 337–342. doi:10.1016/j.bbrc.2018.11.036
Zhang, Y. Q., Chen, X., Fu, C. L., Zhang, W., Zhang, D. L., Pang, C., et al. (2019). FENDRR reduces tumor invasiveness in prostate cancer PC-3 cells by targeting CSNK1E. Eur. Rev. Med. Pharmacol. Sci. 23, 7327–7337. doi:10.26355/eurrev_201909_18838
Zhao, H., Wang, Y., and Ren, X. (2019). Nicotine promotes the development of non-small cell lung cancer through activating LINC00460 and PI3K/Akt signaling. Biosci. Rep. 39, BSR20182443. doi:10.1042/bsr20182443
Zhu, Y., Yang, L., Chong, Q. Y., Yan, H., Zhang, W., Qian, W., et al. (2019). Long noncoding RNA Linc00460 promotes breast cancer progression by regulating the miR-489-5p/FGF7/AKT axis. Cancer Manag. Res. 11, 5983–6001. doi:10.2147/cmar.S207084
Zhu, Y., Zhang, X., Wang, L., Zhu, X., Xia, Z., Xu, L., et al. (2020). FENDRR suppresses cervical cancer proliferation and invasion by targeting miR-15a/b-5p and regulating TUBA1A expression. Cancer Cell Int. 20, 152. doi:10.1186/s12935-020-01223-w
Keywords: epithelial-mesenchymal transition, lncRNA, lung adenocarcinoma, prognosis, tumor microenvironment
Citation: Xiao L, Huang Y, Li Q, Wang S, Ma L, Fan Z, Tang Z, Yuan X and Liu B (2022) Identification of a prognostic classifier based on EMT-related lncRNAs and the function of LINC01138 in tumor progression for lung adenocarcinoma. Front. Mol. Biosci. 9:976878. doi: 10.3389/fmolb.2022.976878
Received: 23 June 2022; Accepted: 12 July 2022;
Published: 17 August 2022.
Edited by:
Rui Cao, Capital Medical University, ChinaCopyright © 2022 Xiao, Huang, Li, Wang, Ma, Fan, Tang, Yuan and Liu. This is an open-access article distributed under the terms of the Creative Commons Attribution License (CC BY). The use, distribution or reproduction in other forums is permitted, provided the original author(s) and the copyright owner(s) are credited and that the original publication in this journal is cited, in accordance with accepted academic practice. No use, distribution or reproduction is permitted which does not comply with these terms.
*Correspondence: Zhe Tang, enRhbmdAdGpoLnRqbXUuZWR1LmNu; Xianglin Yuan, eXVhbnhpYW5nbGluQGh1c3QuZWR1LmNu; Bo Liu, Ym9saXU4ODhAaG90bWFpbC5jb20=
†These authors have contributed equally to this work and share first authorship
‡These authors have contributed equally to this work
Disclaimer: All claims expressed in this article are solely those of the authors and do not necessarily represent those of their affiliated organizations, or those of the publisher, the editors and the reviewers. Any product that may be evaluated in this article or claim that may be made by its manufacturer is not guaranteed or endorsed by the publisher.
Research integrity at Frontiers
Learn more about the work of our research integrity team to safeguard the quality of each article we publish.