- 1Department of Chemistry, University of Cape Town, Cape Town, South Africa
- 2Department of Computer Science, University of Cape Town, Cape Town, South Africa
Streptococcus pneumoniae is an encapsulated gram-negative bacterium and a significant human pathogen. The capsular polysaccharide (CPS) is essential for virulence and a target antigen for vaccines. Although widespread introduction of pneumococcal conjugate vaccines (PCVs) has significantly reduced disease, the prevalence of non-vaccine serotypes has increased. On the basis of the CPS, S. pneumoniae serogroup 10 comprises four main serotypes 10A, 10B, 10C, and 10F; as well as the recently identified 10D. As it is the most prevalent, serotype 10A CPS has been included as a vaccine antigen in the next generation PCVs. Here we use molecular modeling to provide conformational rationales for the complex cross-reactivity reported between serotypes 10A, 10B, 10C, and 10F anti-sera. Although the highly mobile phosphodiester linkages produce very flexible CPS, shorter segments are conformationally defined, with exposed
1 Introduction
S. pneumoniae is an encapsulated gram-negative bacterium and an important human pathogen responsible for significant disease and mortality, especially in children under five (Geno et al., 2015). The capsular polysaccharide (CPS) is essential for virulence and an important target antigen for vaccines (Moreau and Schulz, 2000; Geno et al., 2015). Although 100 different serotypes have been identified, most disease is caused by a subset of pathogenic serotypes (Ganaie et al., 2020). Vaccines against the CPS of S. pneumoniae have been developed either as a pneumococcal polysaccharide vaccine (PPV) or a pneumococcal conjugate vaccine (PCV)—polysaccharide conjugated to a carrier protein (Geno et al., 2015). Unlike polysaccharide vaccines, the glycoconjugate vaccines induce a T cell dependent immune response and are effective in young children; the age group highly susceptible to infections (Lindberg, 1999). This has led to the licensure of PCVs targeting, initially, seven (PCV7, serotypes 4, 6B, 9 V, 14, 18C, 19F, and 23F), then ten (PCV10, PCV7 plus serotypes 1, 5, and 7F), and 13 (PCV13, PCV10 plus serotypes 3, 6A, and 19A) serotypes.
Widespread introduction of PCVs has significantly reduced the burden of invasive pneumococcal disease (IPD) due to vaccine serotypes (Wahl et al., 2018). However, increased prevalence of non-vaccine serotypes (due to serotype replacement as well as different geographical and socio-economic vaccination programs and prevalence of serotypes) means that IPD is still a leading cause of lower respiratory infection morbidity and mortality (GBD 2016 Lower Respiratory Infections Collaborators, 2018; Balsells et al., 2017; Wasserman et al., 2021). This necessitated the development of the third generation of PCVs, with higher serotype valency and protection against the emerging serotypes (Klugman and Rodgers, 2021). A PCV15 vaccine (PCV13 plus serotypes 22F and 33F) and a PCV20 vaccine (PCV15 plus serotypes 8, 10A, 11A, 12F, and 15B) have been licensed and higher valency vaccines such PCV24 (PCV20 plus serotypes 2, 9N, 17F, and 20) and PCV30 are in development (Klugman and Rodgers, 2021; Janssens et al., 2022; Kobayashi, 2022).
The epidemiology of serogroup 10 varies quite significantly across different geographical regions, with Pn10A considered the most prevalent, followed by Pn10B, with Pn10C and Pn10F occasionally isolated but not considered a major burden of disease (Balsells et al., 2017; dos Santos et al., 2013; Jauneikaite et al., 2012; Messaoudi et al., 2016; Wouters et al., 2019). The prevalent serotype 10A is present in PPV23 and targeted for inclusion in the third generation PCVs: in PCV20 and PCV24 (Jones, 1995; Yang et al., 2011; McGuinness et al., 2021; Janssens et al., 2022).
The literature reports considerable cross-reactivity within S. pneumoniae serogroup 10: between serotypes 10A (Pn10A), 10B (Pn10B), 10C (Pn10C), and 10F (Pn10F), summarized in Figure 1 (Henrichsen, 1995; Yang et al., 2011). Specifically, serotype-specific rabbit antisera (
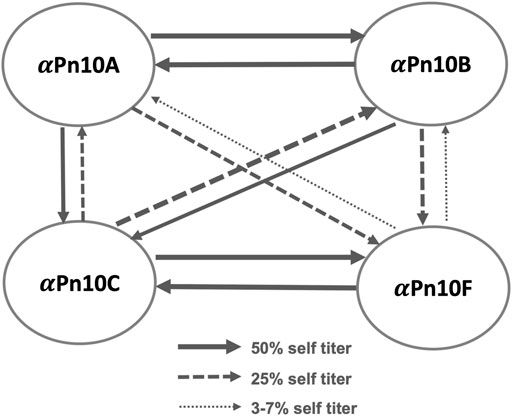
FIGURE 1. S. pneumoniae serogroup 10 rabbit antisera cross-reactivity trends showing the cross-reactivity of antisera (
The repeating unit (RU) structures of the four main capsular polysaccharides in serogroup 10 (Table 1; Figure 2) contain the sugars

TABLE 1. Line structures of S. pneumoniae repeating units for serogroup 10 CPSs, side groups in bold.
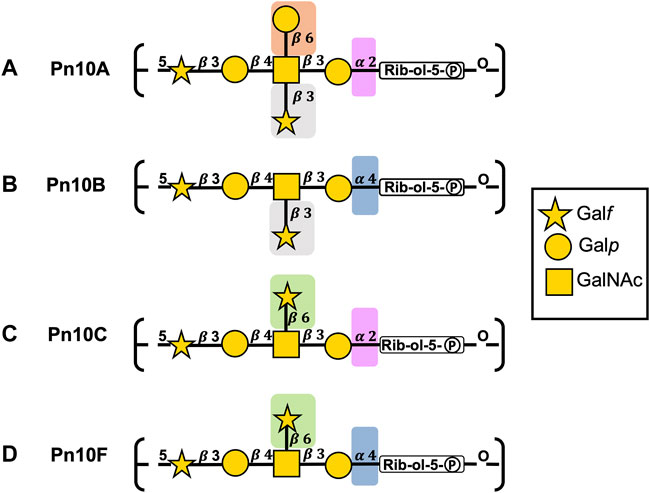
FIGURE 2. S. pneumoniae serogroup 10 CPS repeating unit structures for (A) Pn10A, (B) Pn10B, (C) Pn10C, and (D) Pn10F represented with the SNFG (Symbol Nomenclature for Glycans) system (Varki et al., 2015). Similarities and differences in repeating units are indicated by background color shading.
The backbones for Pn10A (Figure 2A) and Pn10C (Figure 2C) have a
All serogroup 10 serotypes contain the
Molecular modeling is a complementary technique that can be used to correlate the conformational features of carbohydrate antigens with data on serotype cross-protection produced by immunological studies (such as serological evaluations, animal trials, human trials, or epidemiological studies) (Zaccheus et al., 2012; Sarkar et al., 2013; Kang et al., 2014; Yang et al., 2017; Kuttel and Ravenscroft, 2018; Aytenfisu et al., 2019). Recently, we have explored the importance of the presentation of structural features or epitopes on bacterial CPS for serotype cross-protection (Richardson et al., 2021a; Kuttel, 2022). Here we apply our established molecular modeling methodology to the four primary S. pneumoniae serogroup 10 CPSs (Pn10A, Pn10B, Pn10C, and Pn10F) to establish their conformation and identify potential cross-protective epitopes. We aim to rationalize the observed cross-reactivity data and thus inform the design of the next generation of vaccines.
2 Materials and methods
We used our established systematic approach to the modeling of polysaccharides, as previously described (Hlozek et al., 2020; Richardson et al., 2021b), to build three repeating unit (3 RU) chains followed by 6 RU chains of Pn10A, Pn10B, Pn10C, and Pn10F in aqueous solution for initial molecular dynamics (MD) simulations.
Chain length is an important consideration when modeling CPSs, as a short chain may have insufficient molecular flexibility, while long chains are more computationally expensive to model. On the basis of our previous work, we consider a 6 RU chain to be representative of the behavior of the longer polysaccharide. Further, antibodies bind small fragments of the CPS between one and seven residues in length (Kabat, 1966) corresponding to 1 RU (or a fraction thereof) in the case of S. pneumoniae making a 6 RU model sufficient to explore antibody binding epitopes.
Following initial system equilibration, MD simulations were performed of 3 RU and 6 RU chains of each of the CPSs. Data analyses were performed on these production runs, as described below.
2.1 Molecular dynamics
The 3 RU and 6 RU chains were built using in-house CarbBuilder software (version 1.2.42) (Kuttel et al., 2011; Kuttel et al., 2016) and visualized with the Visual Molecular Dynamics (VMD) software (Humphrey et al., 1996). Starting structures for each molecule were built using low energy glycosidic linkages from potential mean force calculations, as per our previous work (Richardson et al., 2021b) and the psfgen tool was used by CarbBuilder to create protein structure (PSF) files for simulation with the CHARMM36 additive force field used to model the carbohydrates (Guvench et al., 2008; Guvench et al., 2009). The starting structures were subsequently minimized with the Nanoscale Molecular Dynamics (NAMD) program (version 2.13) for 10,000 steps at 300 K. Minimized structures were solvated using VMD’s built in solvation and ionization tools to add TIP3P (Jorgensen et al., 1983) cubic water boxes of 84 Å per side for 3 RU systems and 140 Å per side for 6 RU systems. Systems were then neutralized with one sodium (Na+) counter ion per repeating unit (three ions for the 3 RU, six for the 6 RU). Initial minimization and heating protocols comprised 5 K incremental temperature reassignments beginning at 10 K up to 300 K with 500 steps of NAMD minimization and 8,000 steps of MD at each temperature reassignment. Solvated and ionized structures (PDB and PSF files) for each 6 RU system are available as Supplementary Material.
Simulations of 3 RU were run using NAMD (version 2.13) (Phillips et al., 2005) with CUDA extensions for graphics processor acceleration (Stone et al., 2007); simulations of 6 RU were run using NAMD (version 3.0) with CUDA extensions for graphics processor acceleration and a GPU resident computational mode.
Periodic boundary conditions equivalent to the cubic box size were employed for the solvated simulation with wrapping on. Long range electrostatics were implemented with the Particle Mesh Ewald summation grid spacing set to 1 (Darden et al., 1993). Atoms were not held fixed, and the initial center of mass motion was off. The 1-3 pairs were excluded from non-bonded interactions, 1-4 interactions were not scaled, and the dielectric constant was set to 1. Smoothing functions were applied to both the electrostatics and van der Waals forces with switching and cut-off distances of 10 and 12 Å, respectively.
A Leap-Frog Verlet integrator was used to integrate the equations of motion over a step size of 1 fs. A distance of 15 Å was used as the cut-off for inclusion in the pair list for calculation of non-bonded forces. The short-range non-bonded interactions were calculated every 1 fs, full electrostatics calculations were performed every 2 fs, and atoms were reassigned every 10 fs (Van Gunsteren and Berendsen, 1988).
Simulations were sampled under isothermal-isobaric (nPT) ensemble. Langevin dynamics (Feller et al., 1995) were used to control the temperature with a damping coefficient of 5/ps. Nosé-Hoover Langevin piston dynamics were used as a barostat to maintain a target pressure of 1 atm (Nosé and Klein, 1983; Hoover, 1985). Variable system volume was used with a piston period of 100 fs and decay of 50 fs. Simulations of 3,000 ns were performed for the 6 RU systems (3 RU systems were run to 1,000 ns) comprising 200 ns of equilibration and 2,800 ns of production run as was required for convergence.
2.2 Convergence
We addressed convergence using block standard averaging (Grossfield and Zuckerman, 2009) applied to two metrics: end-to-end distance and radius of gyration (Supplementary Figure S1). Block standard averaging was implemented with in-house Python scripts.
For all simulations, the blocked standard error (BSE) reached plateaus for both metrics, indicating convergence. The simulation lengths were large multiples of the correlation times for end-to-end distance (Pn10A, 74 ns; Pn10B, 110 ns; Pn10C, 69 ns; Pn10F, 99 ns) and radius of gyration (Pn10A, 70 ns; Pn10B, 108 ns; Pn10C, 69 ns; Pn10F, 120 ns). Further, the numbers of independent samples were >>1 for both the end-to-end distance (Pn10A, 40; Pn10B, 27; Pn10C, 45; Pn10F, 30) and the radius of gyration (Pn10A, 43; Pn10B, 28; Pn10C, 44; Pn10F, 25). Our designated equilibration time of 200 ns is therefore greater than the correlation time.
2.3 Data analysis
Molecular conformations were visualized using VMD, with the PaperChain and Twister visualization algorithms used to highlight carbohydrate rings and chains (Cross et al., 2009) as required.
Trajectories were extracted at 25 ps intervals with analysis of performed on frames 250 ps apart. Metrics such as end-to-end distances were extracted from the simulation trajectories using Tcl scripting via VMD’s Tk console. Data analyses performed with in-house Python scripts and plots generated using Matplotlib (Hunter, 2007).
2.3.1 Chain flexibility
The end-to-end distance, r, was measured from C1 of
2.3.2 Conformational analysis
The most common chain conformations for each simulation were determined by clustering the production trajectory frames into families and calculating the relative occupancies of each family. Clusters comprising less than 6% of the production run (post equilibration) were discarded. Clustering was performed using the WMC PhysBio plug-in for VMD’s built-in measure cluster command (Gracia Luis, 2012). Prior to clustering, the molecules were aligned on the RU 4 backbone excluding the Rib-ol-5P residue and any hydrogens on the backbone. Clustering was then performed as an RMSD fit to the ring and linkage atoms of the central four repeating units of the chains, excluding the highly flexible terminal RU 1 and RU 6. Eight clusters were created with a cut-off of 8 Å.
Clustering analysis was also performed on a single repeating unit, RU 4, and on the
Similarly, for the
2.4 hIntL-1 binding
The protein data bank (PDB) file describing the structure of human intelectin-1 (hIntL-1) bound to allyl-
3 Results
We begin with a comparison of the flexibility of the serogroup 10 CPSs. This is followed by analysis of the CPS molecular conformation, exposed potential binding epitopes and, finally, an exploration of the potential for CPS binding to the hIntL-1 human innate immune protein.
3.1 Chain flexibility and conformation
The fluctuation in molecular end-to-end distance, r, over the length of the simulation is commonly used as a measure of chain extension and flexibility in carbohydrates. Here we define r to exclude the mobile terminal residues (Figure 3A). Times series plots of r for each CPS (Figures 3B–E, left column) reveal that all four molecules are highly flexible, fluctuating between a wide range of r values. Trajectory snapshots at 50 ns intervals (shown above the graphs) illustrate the considerable diversity in the molecular conformations over all the simulations. This flexibility is an expected consequence of a linear alditol (Rib-ol-5P) with a phosphodiester linkage in the CPS backbone: modeling of Haemophilus influenzae serotypes a and b CPS, which also contain this moiety, showed similarly flexible molecules (Richardson et al., 2021a).
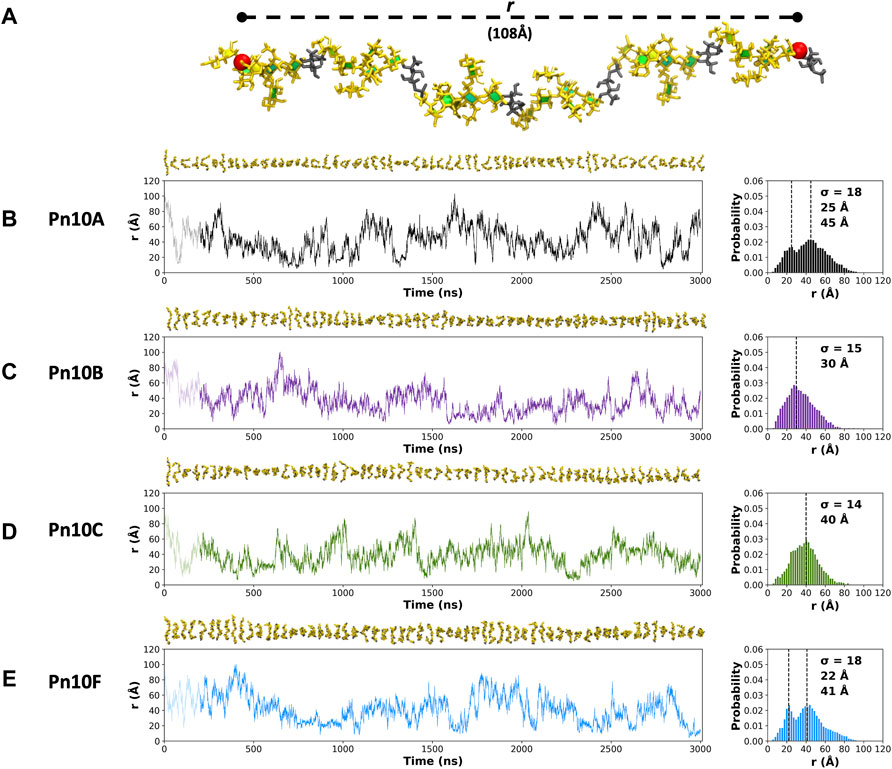
FIGURE 3. (A) The end-to-end distance, r, is indicated on the 6 RU Pn10A molecule. Time series graphs (left column) of r and corresponding histograms (right column) for the 3,000 ns simulation trajectories are shown for: (B) Pn10A, (C) Pn10B, (D) Pn10C, and (E) Pn10F. Conformational snapshots at 50 ns intervals are shown above the time series plots. The histograms are labeled with the standard deviations (
Comparison of the r distributions (Figures 3B–E, right column) reveals some broad differences between the serotypes: Pn10A and Pn10F have bimodal distributions, whereas Pn10B and Pn10C are closer to unimodal, narrower and somewhat shifted to smaller values of r. Interestingly, it is the most structurally dissimilar pairs of molecules—Pn10A and Pn10F (Figures 2B,E); Pn10B and Pn10C (Figures 2C,D)—that are most similar with respect to the r distributions. While all four molecules are flexible, these data suggest an order of flexibility of Pn10A ∼ Pn10F > Pn10B > Pn10C and, relatively speaking, a slightly increased incidence of extended conformations for Pn10A and Pn10F.
The extreme flexibility of the CPS backbone means that there are no dominant chain conformations for any of the four carbohydrates (Figure 4). For RU 2 to RU 5 of the CPS backbone (i.e., 20 backbone residues) we found very few significant conformational families that occupy more than 6% of the simulation. Further, those we identified accounted for relatively small portions of the simulation (less than 25%). This indicates that these flexible chains behave as random coils, in common with other flexible carbohydrates that we have modeled (Richardson et al., 2021a; Richardson et al., 2021b).
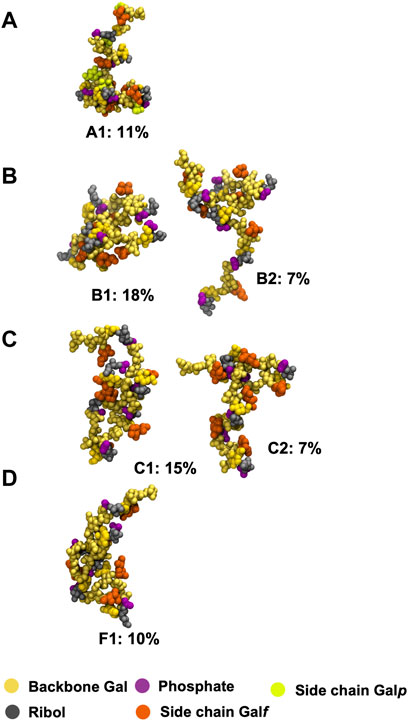
FIGURE 4. Main conformational families identified for the 6 RU S. pneumoniae serotype CPS, with the terminal repeat units (RU 1 and RU 6) excluded from analysis: (A) Pn10A, (B) Pn10B, (C) Pn10C, and (D) Pn10F. Backbone galactose residues are shown in yellow, ribitol residues shown in grey, phosphate in purple, side group galactofuranose residues in orange, and side group galactopyranose residues in light green. The primary clusters occurred regularly throughout the trajectory. The secondary cluster of Pn10B occurred regularly in the second half of the simulation along with the primary cluster; for Pn10C the secondary cluster occurred only for ∼400 ns around the 1,500 ns timestep.
We therefore conclude that none of these flexible CPS show evidence for a conformational epitope. However, most of the CPS chain flexibility originates from the backbone phosphodiester linkage to the Rib-ol-5P in the CPS backbone, with the other glycosidic linkages being relatively constrained (Supplementary Figure S2), potentially forming stable epitopes. Further, in all four molecules, the side chain
3.2 Epitopes
The principal conformations for the single central repeating unit (RU 4) in each of the CPS chains are shown in Figure 5. With the highly mobile phosphodiester linkage excluded, we find dominant conformations with high occupancies ranging from 30% to 80% for the primary cluster and 13%–24% for the secondary cluster; more than 50% of the simulation trajectory falls within these dominant clusters. For Pn10A (Figure 5A) the 6-linked
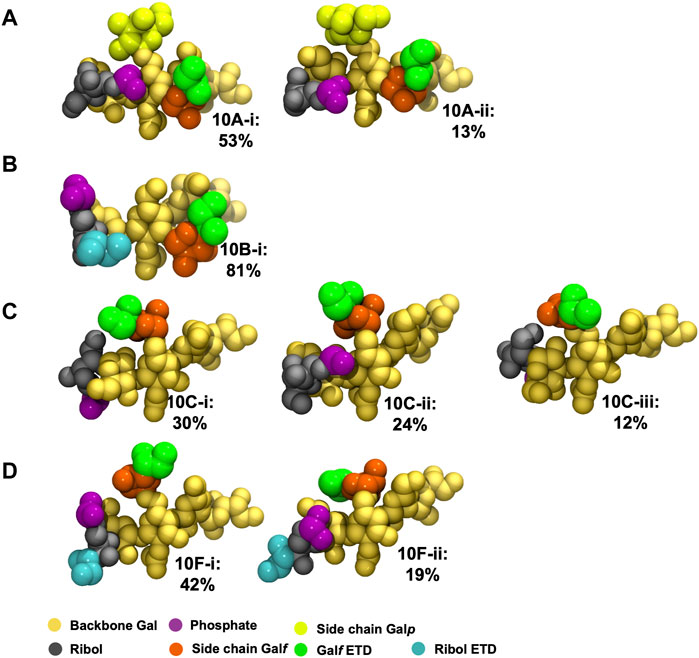
FIGURE 5. Main conformational families identified for RU 4 in the backbone of 6 RU S. pneumoniae serotype CPS: (A) Pn10A, (B) Pn10B, (C) Pn10C, and (D) Pn10F. Backbone galactose residues shown in yellow, Rib-ol-5P residues shown in grey, phosphate in purple, side group
To explore the range of conformations of the immunodominant
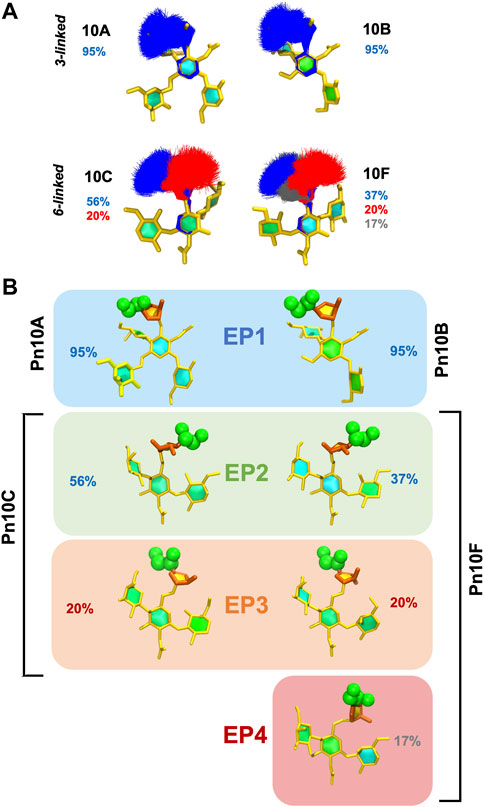
FIGURE 6. Conformational epitopes of the
These conformational epitopes identified for
3.3 hIntL-1 binding
The exocyclic 1,2-terminal diol on
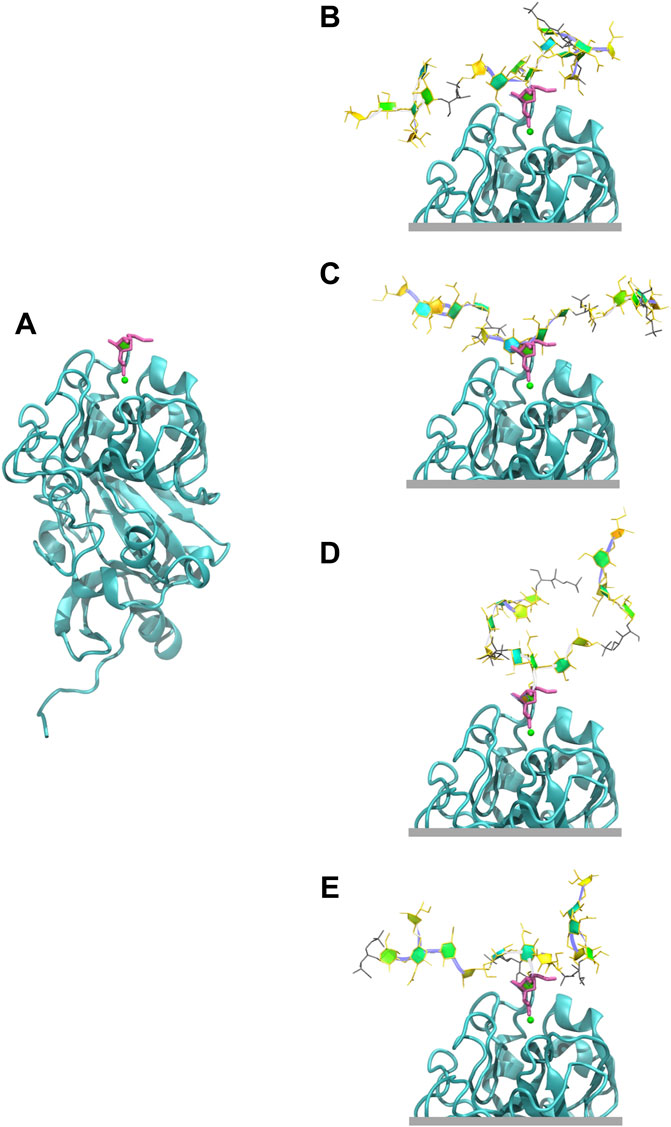
FIGURE 7. Human intelectin-1 (hIntL-1) binding. (A) Monomer of the crystal structure of hIntL-1 bound with allyl-beta-galactofuranose (PDB ID: 4WMY). Example conformations of the 3 RU CPSs with the
4 Discussion
Our simulations show that S. pneumoniae serogroup 10 have highly flexible CPSs, which is unsurprisingly due to the backbone linear Rib-ol-5P alditol. Highly flexible bacterial CPSs are common and we have previously suggested that this is a strategy for bacterial evasion of the host immune system: a flexible backbone with multiple conformations presents a “moving target” to the immune system (Kuttel and Ravenscroft, 2019). For serogroup 10, this mobility may be especially necessary given the presence of an immunodominant PO4 group (Zhang et al., 2021) and the presence of a non-mammalian galactofuranose sugar to which the human immune system has a natural defense. Between the highly mobile phosphodiester linkages to Rib-ol-5P, the segments of the CPS chain are relatively rigid and the
The more flexible 6-linked
Pn10C and Pn10F also share both the
The recently discovered serotype Pn10D has a different backbone (and was not modeled in this study), but also exposes the 6-linked
Finally, we showed that the hIntL-1 human innate immune protein has the potential to bind the EP1/EP2 epitope in serogroup 10, containing the exocyclic 1,2-terminal diol on
In conclusion, the common epitopes we have identified for serogroup 10 support a vaccine containing serotype 10A providing adequate cross-protection against serogroup 10 disease, as indicated by serological studies.
Data availability statement
The datasets presented in this study can be found in online repositories. The names of the repository/repositories and accession number(s) can be found below: https://www.rcsb.org/structure/4WMY.
Author contributions
Conceptualization, MMK and NR; methodology, MMK; software, MMK and NIR; validation, MMK and NIR; investigation, NIR; resources, MMK and NR; data curation, MMK; writing-original draft preparation, NIR; writing-review and editing, MMK and NR; supervision, MMK and NR; visualization, MMK and NIR; funding acquisition, MMK and NR.
Funding
This research was partially funded by the University of Cape Town (research scholarship for NIR).
Acknowledgments
Computations were performed using facilities provided by the University of Cape Town’s ICTS High Performance Computing team: http://hpc.uct.ac.za.
Conflict of interest
The authors declare that the research was conducted in the absence of any commercial or financial relationships that could be construed as a potential conflict of interest.
Publisher’s note
All claims expressed in this article are solely those of the authors and do not necessarily represent those of their affiliated organizations, or those of the publisher, the editors and the reviewers. Any product that may be evaluated in this article, or claim that may be made by its manufacturer, is not guaranteed or endorsed by the publisher.
Supplementary material
The Supplementary Material for this article can be found online at: https://www.frontiersin.org/articles/10.3389/fmolb.2022.961532/full#supplementary-material
References
Aytenfisu, A. H., Simon, R., and MacKerell, A. D. (2019). Impact of branching on the conformational heterogeneity of the lipopolysaccharide from Klebsiella pneumoniae: Implications for vaccine design. Carbohydr. Res. 475, 39–47. doi:10.1016/j.carres.2019.02.003
Balsells, E., Guillot, L., Nair, H., and Kyaw, M. H. (2017). Serotype distribution of Streptococcus pneumoniae causing invasive disease in children in the post-PCV era: A systematic review and meta-analysis. PloS One 12 (5), e0177113. doi:10.1371/journal.pone.0177113
Bush, C. A., Yang, J., Yu, B., and Cisar, J. O. (2014). Chemical structures of Streptococcus pneumoniae capsular polysaccharide type 39 (CPS39), CPS47F, and CPS34 characterized by nuclear magnetic resonance spectroscopy and their relation to CPS10A. J. Bacteriol. 196 (18), 3271–3278. doi:10.1128/JB.01731-14
Chen, L., Li, J., and Yang, G. (2020). A comparative review of intelectins. Scand. J. Immunol. 92 (1), e12882. doi:10.1111/sji.12882
Cross, S., Kuttel, M. M., Stone, J. E., and Gain, J. E. (2009). Visualisation of cyclic and multi-branched molecules with VMD. J. Mol. Graph. Model. 28 (2), 131–139. doi:10.1016/j.jmgm.2009.04.010
Darden, T., York, D., and Pedersen, L. (1993). Particle mesh Ewald: AnN log(N) method for Ewald sums in large systems. J. Chem. Phys. 98 (12), 10089–10092. doi:10.1063/1.464397
dos Santos, S. R., Passadore, L. F., Takagi, E. H., Fujii, C. M., Yoshioka, C. R., and Gilio, A. E. (2013). Serotype distribution of Streptococcus pneumoniae isolated from patients with invasive pneumococcal disease in Brazil before and after ten-pneumococcal conjugate vaccine implementation. Vaccine 31 (51), 6150–6154. doi:10.1016/j.vaccine.2013.05.042
Feller, S. E., Zhang, Y., Pastor, R. W., and Brooks, B. R. (1995). Constant pressure molecular dynamics simulation: The Langevin piston method. J. Chem. Phys. 103 (11), 4613–4621. doi:10.1063/1.470648
Ganaie, F., Saad, J. S., McGee, L., van Tonder, A. J., Bentley, S. D., Lo, S. W., et al. (2020). A new pneumococcal capsule type, 10D, is the 100th serotype and has a large cps fragment from an oral streptococcus. mBio 11 (3), 937. doi:10.1128/mBio.00937-20
GBD 2016 Lower Respiratory Infections Collaborators (2018). Estimates of the global, regional, and national morbidity, mortality, and aetiologies of lower respiratory infections in 195 countries, 1990–2016: A systematic analysis for the global burden of disease study 2016. Lancet.Infectious Dis. 18 (11), 1191. doi:10.1016/S1473-3099(18)30310-4
Geno, K. A., Gilbert, G. L., Song, J. Y., Skovsted, I. C., Klugman, K. P., Jones, C., et al. (2015). Pneumococcal capsules and their types: Past, present, and future. Clin. Microbiol. Rev. 28 (3), 871–899. doi:10.1128/CMR.00024-15
Gracia Luis (2012). WMC PhysBio clustering. Available at: https://github.com/luisico/clustering (Accessed June, 2020).
Grossfield, A., and Zuckerman, D. M. (2009). Quantifying uncertainty and sampling quality in biomolecular simulations. Annu. Rep. Comput. Chem. 5, 23–48. doi:10.1016/S1574-1400(09)00502-7
Guvench, O., Greene, S. N., Kamath, G., Brady, J. W., Venable, R. M., Pastor, R. W., et al. (2008). Additive empirical force field for hexopyranose monosaccharides. J. Comput. Chem. 29 (15), 2543–2564. doi:10.1002/jcc.21004
Guvench, O., Hatcher, E., Venable, R. M., Pastor, R. W., and MacKerell, A. D. (2009). CHARMM additive all-atom force field for glycosidic linkages between hexopyranoses. J. Chem. Theory Comput. 5 (9), 2353–2370. doi:10.1021/ct900242e
Henrichsen, J. (1995). Six newly recognized types of Streptococcus pneumoniae. J. Clin. Microbiol. 33 (10), 2759–2762. doi:10.1128/jcm.33.10.2759-2762.1995
Hlozek, J., Owen, S., Ravenscroft, N., and Kuttel, M. M. (2020). Molecular modeling of the Shigella flexneri serogroup 3 and 5 O-antigens and conformational relationships for a vaccine containing serotypes 2a and 3a. Vaccines 8 (4), 643. doi:10.3390/vaccines8040643
Hoover, W. G. (1985). Canonical dynamics: Equilibrium phase-space distributions. Phys. Rev. A 31 (3), 1695. doi:10.1103/PhysRevA.31.1695
Humphrey, W., Dalke, A., and Schulten, K. (1996). Vmd: Visual molecular dynamics. J. Mol. Graph. 14 (1), 33–38. doi:10.1016/0263-7855(96)00018-5
Hunter, J. D. (2007). Matplotlib: A 2D graphics environment. Comput. Sci. Eng. 9 (3), 90–95. doi:10.1109/MCSE.2007.55
Isabella, C. R. (2021). Carbohydrate and bacterial binding specificity of human intelectin-1. Available at: http://dspace.mit.edu/handle/1721.1/7582.
Janssens, E., Flamaing, J., Vandermeulen, C., Peetermans, W. E., Desmet, S., and De Munter, P. (2022). The 20-valent pneumococcal conjugate vaccine (PCV20): Expected added value. Acta Clin. Belg. Prepr., 1–9. doi:10.1080/17843286.2022.2039865
Jauneikaite, E., Jefferies, J. M., Hibberd, M. L., and Clarke, S. C. (2012). Prevalence of Streptococcus pneumoniae serotypes causing invasive and non-invasive disease in south east asia: A review. Vaccine 30 (24), 3503–3514. doi:10.1016/j.vaccine.2012.03.066
Jones, C. (1995). Full assignment of the NMR spectrum of the capsular polysaccharide from Streptococcus pneumoniae serotype 10A. Carbohydr. Res. 269 (1), 175–181. doi:10.1016/0008-6215(94)00340-L
Jorgensen, W. L., Chandrasekhar, J., Madura, J. D., Impey, R. W., and Klein, M. L. (1983). Comparison of simple potential functions for simulating liquid water. J. Chem. Phys. 79 (2), 926–935. doi:10.1063/1.445869
Kang, Y., Barbirz, S., Lipowsky, R., and Santer, M. (2014). Conformational diversity of O-antigen polysaccharides of the Gram-negative bacterium Shigella flexneri serotype Y. J. Phys. Chem. B 118 (9), 2523–2534. doi:10.1021/jp4111713
Kiessling, L. L. (2018). Chemistry-driven glycoscience. Bioorg. Med. Chem. 26 (19), 5229–5238. doi:10.1016/j.bmc.2018.09.024
Klugman, K. P., and Rodgers, G. L. (2021). Time for a third-generation pneumococcal conjugate vaccine. Lancet Infect. Dis. 21 (1), 14–16. doi:10.1016/S1473-3099(20)30513-2
Kobayashi, M. (2022). Use of 15-valent pneumococcal conjugate vaccine and 20-valent pneumococcal conjugate vaccine among US adults: Updated recommendations of the advisory committee on immunization practices—United States, 2022. Morb. Mortal. Wkly. Rep. 71, 109. doi:10.15585/mmwr.mm7104a1
Konradsen, H. B. (2005). Validation of serotyping of Streptococcus pneumoniae in Europe. Vaccine 23 (11), 1368–1373. doi:10.1016/j.vaccine.2004.09.011
Kuttel, M., Mao, Y., Widmalm, G., and Lundborg, M. (2011). “CarbBuilder: An adjustable tool for building 3D molecular structures of carbohydrates for molecular simulation,” in Presented at the 2011 IEEE Seventh International Conference on eScience, Stockholm, Sweden, December 2011 (IEEE), 395–402. doi:10.1109/eScience.2011.61
Kuttel, M. M. (2022). Comparative molecular modelling of capsular polysaccharide conformations in Streptococcus suis serotypes 1, 2, 1/2 and 14 identifies common epitopes for antibody binding. Front. Mol. Biosci. 9, 830854. doi:10.3389/fmolb.2022.830854
Kuttel, M. M., and Ravenscroft, N. (2018). “The role of molecular modeling in predicting carbohydrate antigen conformation and understanding vaccine immunogenicity,” in Carbohydrate-based vaccines: From concept to clinic. Editor A. Prasad Krishna (Washington, D.C: ACS Publications), 139–173. doi:10.1021/bk-2018-1290.ch007
Kuttel, M. M., and Ravenscroft, N. (2019). Conformation and cross-protection in group B Streptococcus serotype III and Streptococcus pneumoniae serotype 14: A molecular modeling study. Pharmaceuticals 12 (1), 28. doi:10.3390/ph12010028
Kuttel, M. M., Ståhle, J., and Widmalm, G. (2016). CarbBuilder: Software for building molecular models of complex oligo‐and polysaccharide structures. J. Comput. Chem. 37 (22), 2098–2105. doi:10.1002/jcc.24428
Kuttel, M. M., Timol, Z., and Ravenscroft, N. (2017). Cross-protection in Neisseria meningitidis serogroups Y and W polysaccharides: A comparative conformational analysis. Carbohydr. Res. 446-447 (29), 40–47. doi:10.1016/j.carres.2017.05.004
Lindberg, A. A. (1999). Glycoprotein conjugate vaccines. Vaccine 17 (S2), S28–S36. doi:10.1016/S0264-410X(99)00232-7
Marino, C., Rinflerch, A., and de Lederkremer, R. M. (2017). Galactofuranose antigens, a target for diagnosis of fungal infections in humans. Future Sci. OA 3 (3), FSO199. doi:10.4155/fsoa-2017-0030
McGuinness, D., Kaufhold, R. M., McHugh, P. M., Winters, M. A., Smith, W. J., Giovarelli, C., et al. (2021). Immunogenicity of PCV24, an expanded pneumococcal conjugate vaccine, in adult monkeys and protection in mice. Vaccine 39 (30), 4231–4237. doi:10.1016/j.vaccine.2021.04.067
Messaoudi, M., Milenkov, M., Albrich, W. C., van der Linden, , Mark, P. G., Bénet, T., et al. (2016). The relevance of a novel quantitative assay to detect up to 40 major Streptococcus pneumoniae serotypes directly in clinical nasopharyngeal and blood specimens. PLoS One 11 (3), e0151428. doi:10.1371/journal.pone.0151428
Moreau, M., and Schulz, D. (2000). Polysaccharide based vaccines for the prevention of pneumococcal infections. J. Carbohydr. Chem. 19 (4-5), 419–434. doi:10.1080/07328300008544091
Nahm, M. H., Yu, J., Vlach, J., and Bar-Peled, M. (2020). A common food glycan, pectin, shares an antigen with Streptococcus pneumoniae capsule. Msphere 5 (2), 74. doi:10.1128/mSphere.00074-20
Nosé, S., and Klein, M. L. (1983). Constant pressure molecular dynamics for molecular systems. Mol. Phys. 50 (5), 1055–1076. doi:10.1080/00268978300102851
Petersen, B. O., Meier, S., Paulsen, B. S., Redondo, A. R., and Skovsted, I. C. (2014). Determination of native capsular polysaccharide structures of Streptococcus pneumoniae serotypes 39, 42, and 47F and comparison to genetically or serologically related strains. Carbohydr. Res. 395, 38–46. doi:10.1016/j.carres.2014.06.018
Phillips, J. C., Braun, R., Wang, W., Gumbart, J., Tajkhorshid, E., Villa, E., et al. (2005). Scalable molecular dynamics with NAMD. J. Comput. Chem. 26 (16), 1781–1802. doi:10.1002/jcc.20289
Richardson, N. I., Kuttel, M. M., Michael, F. S., Cairns, C., Cox, A. D., and Ravenscroft, N. (2021a). Cross-reactivity of Haemophilus influenzae type a and b polysaccharides: Molecular modeling and conjugate immunogenicity studies. Glycoconj. J. 38, 735–746. doi:10.1007/s10719-021-10020-0
Richardson, N. I., Ravenscroft, N., Arato, V., Oldrini, D., Micoli, F., and Kuttel, M. M. (2021b). Conformational and immunogenicity studies of the Shigella flexneri serogroup 6 O-antigen: The effect of O-acetylation. Vaccines 9 (5), 432. doi:10.3390/vaccines9050432
Sarkar, A., Fontana, C., Imberty, A., Perez, S., and Widmalm, G. (2013). Conformational preferences of the O-antigen polysaccharides of Escherichia coli O5ac and O5ab using NMR spectroscopy and molecular modeling. Biomacromolecules 14 (7), 2215–2224. doi:10.1021/bm400354y
Spencer, B. L., Saad, J. S., Shenoy, A. T., Orihuela, C. J., and Nahm, M. H. (2017). Position of O-acetylation within the capsular repeat unit impacts the biological properties of pneumococcal serotypes 33A and 33F. Infect. Immun. 85 (7), 132. doi:10.1128/IAI.00132-17
Stone, J. E., Phillips, J. C., Freddolino, P. L., Hardy, D. J., Trabuco, L. G., and Schulten, K. (2007). Accelerating molecular modeling applications with graphics processors. J. Comput. Chem. 28 (16), 2618–2640. doi:10.1002/jcc.20829
Tefsen, B., Ram, A. F., van Die, I., and Routier, F. H. (2012). Galactofuranose in eukaryotes: Aspects of biosynthesis and functional impact. Glycobiology 22 (4), 456–469. doi:10.1093/glycob/cwr144
Van Gunsteren, W. F., and Berendsen, H. J. (1988). A leap-frog algorithm for stochastic dynamics. Mol. Simul. 1 (3), 173–185. doi:10.1080/08927028808080941
Varki, A., Cummings, R. D., Aebi, M., Packer, N. H., Seeberger, P. H., Esko, J. D., et al. (2015). Symbol nomenclature for graphical representations of glycans. Glycobiology 25 (12), 1323–1324. doi:10.1093/glycob/cwv091
Wahl, B., O'Brien, K. L., Greenbaum, A., Majumder, A., Liu, L., Chu, Y., et al. (2018). Burden of Streptococcus pneumoniae and Haemophilus influenzae type b disease in children in the era of conjugate vaccines: Global, regional, and national estimates for 2000–15. Lancet Glob. Health 6 (7), e744–e757. doi:10.1016/S2214-109X(18)30247-X
Wasserman, M. D., Perdrizet, J., Grant, L., Hayford, K., Singh, S., Saharia, P., et al. (2021). Clinical and economic burden of pneumococcal disease due to serotypes contained in current and investigational pneumococcal conjugate vaccines in children under five years of age. Infect. Dis. Ther. 10 (4), 2701–2720. doi:10.1007/s40121-021-00544-1
Wesener, D. A., Wangkanont, K., McBride, R., Song, X., Kraft, M. B., Hodges, H. L., et al. (2015). Recognition of microbial glycans by human intelectin-1. Nat. Struct. Mol. Biol. 22 (8), 603–610. doi:10.1038/nsmb.3053
Wouters, I., Desmet, S., Van Heirstraeten, L., Blaizot, S., Verhaegen, J., Van Damme, P., et al. (2019). Follow-up of serotype distribution and antimicrobial susceptibility of Streptococcus pneumoniae in child carriage after a PCV13-to-PCV10 vaccine switch in Belgium. Vaccine 37 (8), 1080–1086. doi:10.1016/j.vaccine.2018.12.068
Yang, J., Nahm, M. H., Bush, C. A., and Cisar, J. O. (2011). Comparative structural and molecular characterization of Streptococcus pneumoniae capsular polysaccharide serogroup 10. J. Biol. Chem. 286 (41), 35813–35822. doi:10.1074/jbc.M111.255422
Yang, J., Shelat, N. Y., Bush, C. A., and Cisar, J. O. (2010). Structure and molecular characterization of Streptococcus pneumoniae capsular polysaccharide 10F by carbohydrate engineering in Streptococcus oralis. J. Biol. Chem. 285 (31), 24217–24227. doi:10.1074/jbc.M110.123562
Yang, M., Simon, R., and MacKerell, A. D. (2017). Conformational preference of serogroup B Salmonella O polysaccharide in presence and absence of the monoclonal antibody Se155–4. J. Phys. Chem. B 121 (15), 3412–3423. doi:10.1021/acs.jpcb.6b08955
Yu, J., Lin, J., Benjamin, W. H., Waites, K. B., Lee, C., and Nahm, M. H. (2005). Rapid multiplex assay for serotyping pneumococci with monoclonal and polyclonal antibodies. J. Clin. Microbiol. 43 (1), 156–162. doi:10.1128/JCM.43.1.156-162.2005
Zaccheus, M., Pendrill, R., Jackson, T. A., Wang, A., Auzanneau, F., and Widmalm, G. (2012). Conformational dynamics of a central trisaccharide fragment of the LeaLex tumor associated antigen studied by NMR spectroscopy and molecular dynamics simulations. Eur. J. Org. Chem. 2012 (25), 4705–4715. doi:10.1002/ejoc.201200569
Zhang, S., Sella, M., Sianturi, J., Priegue, P., Shen, D., and Seeberger, P. H. (2021). Discovery of oligosaccharide antigens for semi‐synthetic glycoconjugate vaccine leads against Streptococcus suis serotypes 2, 3, 9 and 14. Angew. Chem. Int. Ed. 60 (26), 14679–14692. doi:10.1002/anie.202103990
Glossary
allyl
BSE block standard averaging
CPS capsular polysaccharide
CUDA compute unified device architecture
EP epitope
ETD exocyclic terminal-1,2-diol
GPU graphics processing unit
hIntL-1 human intelectin-1
IPD invasive pneumococcal disease
KDO 3-deoxy-D-manno-oct-2-ulopyranosonic acid
KO D-glycero-D-talo-oct-2-ulosonic acid
MD molecular dynamics
NAMD nanoscale molecular dynamics
nPT isothermal-isobaric
PCV pneumococcal conjugate vaccine
PDB Protein Data Bank
Pn10A S. pneumoniae serotype 10A
Pn10B S. pneumoniae serotype 10B
Pn10C S. pneumoniae serotype 10C
Pn10D S. pneumoniae serotype 10D
Pn10F S. pneumoniae serotype 10F
PPV pneumococcal polysaccharide vaccine
PSF protein structure file
r end-to-end distance
Rib-ol-5P D-ribitol-5-phosphate
RMSD root-mean-square deviation
RSCB Royal Society of Chemistry Biology
RU repeating unit
VMD visual molecular dynamics
Keywords: S. pneumoniae, serogroup 10, capsular polysaccharide, vaccine antigen, molecular modeling, cross-protection, carbohydrate epitopes
Citation: Richardson NI, Kuttel MM and Ravenscroft N (2022) Modeling of pneumococcal serogroup 10 capsular polysaccharide molecular conformations provides insight into epitopes and observed cross-reactivity. Front. Mol. Biosci. 9:961532. doi: 10.3389/fmolb.2022.961532
Received: 04 June 2022; Accepted: 04 July 2022;
Published: 08 August 2022.
Edited by:
Daron I. Freedberg, United States Food and Drug Administration, United StatesReviewed by:
Alexander MacKerell, University of Maryland, Baltimore, United StatesJeahoo Kwon, United States Food and Drug Administration, United States
Andreas Franz, The University of the Pacific, United States
Copyright © 2022 Richardson, Kuttel and Ravenscroft. This is an open-access article distributed under the terms of the Creative Commons Attribution License (CC BY). The use, distribution or reproduction in other forums is permitted, provided the original author(s) and the copyright owner(s) are credited and that the original publication in this journal is cited, in accordance with accepted academic practice. No use, distribution or reproduction is permitted which does not comply with these terms.
*Correspondence: Neil Ravenscroft, neil.ravenscroft@uct.ac.za