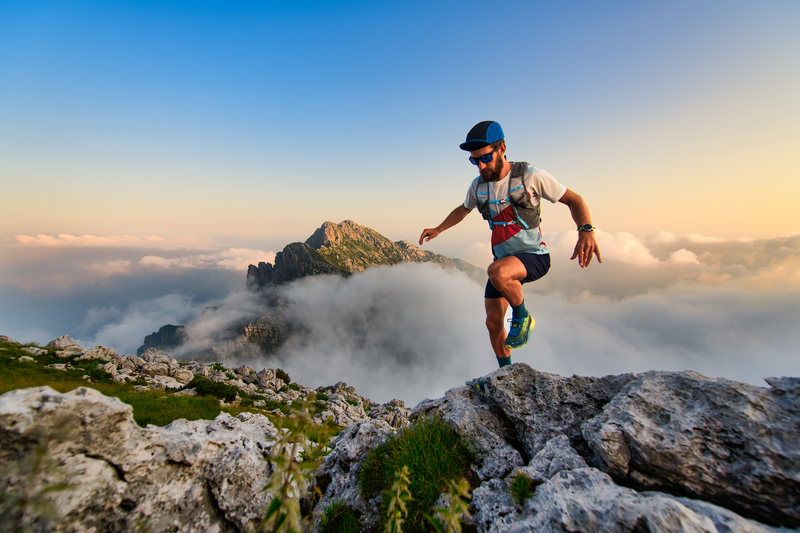
94% of researchers rate our articles as excellent or good
Learn more about the work of our research integrity team to safeguard the quality of each article we publish.
Find out more
ORIGINAL RESEARCH article
Front. Mol. Biosci. , 15 July 2022
Sec. Molecular Diagnostics and Therapeutics
Volume 9 - 2022 | https://doi.org/10.3389/fmolb.2022.916689
This article is part of the Research Topic Machine Learning and Big Data Analysis in Multi-Omics Medical Bioinformatics View all 7 articles
Background: Ferroptosis, a form of non-apoptotic cell death, has aroused worldwide interest in cancer researchers. However, the current study about the correlation between ferroptosis-related genes (FRGs) and endometrial cancer (EC) remains limited.
Methods: First, the transcriptome profiling and clinical data of EC patients were downloaded from The Cancer Genome Atlas (TCGA) and Clinical Proteomic Tumor Analysis Consortium (CPTAC) program as the training group and testing group, respectively. FRGs were acquired through literature mining. Then, we used R 4.1.1 software to screen the differently expressed FRGs from TCGA, which was also connected with the prognosis of EC patients. Subsequently, the risk score of each tumor sample was identified by LASSO regression analysis, and we classified these samples into the high- and low-risk groups in the light of the median risk score. Receiver operating characteristic (ROC) curve analysis and Kaplan-Meier analysis were performed to assess the accuracy of this signature. Significantly, the data from CPTAC was used to validate the prediction model externally. Furthermore, we evaluated the immune microenvironment in this model via single-sample gene set enrichment analysis (ssGSEA).
Results: Among the 150 FRGs, 6 differentially expressed genes (DEGs) based on TCGA had a relationship with the prognosis of EC patients, namely, TP53, AIFM2, ATG7, TLR4, PANX1 and MDM2. The survival curve indicated a higher survival probability in the low-risk group. Moreover, the FRGs-based signature acted well in the prediction of overall survival (OS). The results of external verification confirmed the prediction model we established. Finally, ssGSEA revealed significant differences in the abundance of 16 immune cells infiltration and the activity of 13 immune functions between different risk groups.
Conclusion: We identified a novel ferroptosis-related gene signature which could concisely predict the prognosis and immunotherapy in EC patients.
As endometrial cancer (EC) is the most common malignancies of the female genitals in developed countries, it is estimated that there were 417,367 new cases and 97,370 uterine corpus cancer-related deaths in 2020 (Sung et al., 2021). And the incidence continues to increase at an annual rate of 1% (Lortet-Tieulent et al., 2018). Although a large number of patients are diagnosed in the early stage, some of the early patients will suffer from recurrence. In addition, it is difficult to effectively identify these high-risk groups and apply individualized adjuvant therapies. Thus, there is an urgent need to improve the accuracy of the prognostic evaluation system for EC.
Ferroptosis, an iron-dependent non-apoptotic cell death, is caused by membrane damage mediated by excess lipid peroxidation (Stockwell et al., 2017). It is reported that ferroptosis is a vital process in varieties of cancers including EC (Mou et al., 2019; Zhang Y. Y. et al., 2021). The activation of ferroptosis can significantly enhance the cytotoxic effect of gemcitabine on pancreatic cancer (Ye et al., 2021). CircRHOT1 is involved in the malignant progression of breast cancer by attenuating ferroptosis (Zhang H. et al., 2021). In vivo experiments, the energy metabolism of HepG2 cells can be blocked by inducing ferroptosis (Cui et al., 2022). What’s more, ferroptosis shows enormous potential to induce cancer cells death in the treatment of gastrointestinal tumors and lung cancer (Li and Liu, 2021; Sun et al., 2021; Zhao et al., 2021). A recent study indicates that PTPN18 participated in the regulation of ferroptosis in EC cells by targeting the p-p38/Gpx4/XCT axis, thus inhibiting the proliferation of EC cells (Wang et al., 2021a). Therefore, ferroptosis seems to be a promising target for cancer therapy. There have been several reports on the correlation between ferroptosis-related genes (FRGs) and clinical prognosis in EC (Liu et al., 2021; Weijiao et al., 2021). Wang et al. identify a 13-FRGs prognostic signature for EC. Liu et al. establish a prognostic model based on 6-FRGs. In addition, Wei et al. construct a 6-FRGs contained prediction signature. However, genes in these models are widely divergent, and the relationship between ferroptosis and the prognosis of EC is still controversial. Therefore, the search for new biomarkers of ferroptosis is of great significance not only to clarify the specific mechanism of ferroptosis in EC more precisely, but also to improve the prognosis prediction for patients with EC.
In this study, we purpose to recognize the role of FRGs in EC, which can help to improve the prognosis evaluation system of EC and build a bridge between ferroptosis and immune microenvironment, expecting to provide theoretical reference for immunotherapy of EC.
We acquired the quantification of gene expression and clinical data of cases from ‘TCGA-UCEC’ project in The Cancer Genome Atlas (TCGA) (https://portal.gdc.cancer.gov/projects/TCGA-UCEC), including 35 normal samples and 552 tumor samples. Then the gene expression of 99 tumor samples and the survival outcomes of corresponding patients were extracted on the basis of the Clinical Proteomic Tumor Analysis Consortium (CPTAC) database (https://proteomics.cancer.gov/programs/cptac) (Edwards et al., 2015). In addition, the clinical data must consist of survival time, vital status, stage, grade and age of patients. In this study, the tumor samples from TCGA and CPTAC represented the training group and the testing group, respectively. Finally, a total of 150 FRGs were identified based on FerrDb database (Zhou and Bao, 2020) as well as previous literature (Supplementary Table S1) (Stockwell et al., 2017; Bersuker et al., 2019; Doll et al., 2019; Hassannia et al., 2019).
First, differentially expressed FRGs between tumor and normal samples from TCGA were sifted by the “limma” package in R 4.1.1 software following the threshold of false discovery rate (FDR) < 0.05. Then, we used the “survival” package to do univariate Cox analysis of overall survival (OS), and identified potential genes with prognostic difference (p < 0.05) in the training group. And the intersection of the two gene sets as mentioned above were the target FRGs with prognostic values, with which we queried the STRING website (https://cn.string-db.org/) (Szklarczyk et al., 2021) to construct functional protein association networks. Finally, we used the “glmnet” package in R to fit the least absolute shrinkage and selection operator (LASSO) regression model and classified the risk of patients in the training group and the testing group.
To examine the accuracy of the signature, we used “survival” package to draw Kaplan-Meier curves for the comparison of OS between high- and low-risk groups firstly, then used “timeROC” and “pheatmap” package to conduct receiver operating characteristic (ROC) curve analysis. t-Distributed Stochastic Neighbor Embedding (t-SNE) as well as Principal component analysis (PCA) was employed to reveal the dimensionality reduction of target FRGs data. Finally, the prognosis-related factors were recognized by univariate and multivariate Cox analyses from clinical information.
Additionally, we used the Human Protein Atlas (HPA) database (https://www.proteinatlas.org/) to test the core FRGs in other cancers prognosis.
According to the filter criteria of FDR <0.05 as well as |log fold change (log FC)|>1, we filtered out differential FRGs between two risk cohorts using the “limma” package. Afterwards, Gene Ontology (GO) and Kyoto Encyclopedia of Genes and Genomes (KEGG) analyses were performed via “clusterProfiler”, “org.Hs.eg.db”, “enrichplot” and “ggplot2” packages in R.
We implemented Single sample gene set enrichment analysis (ssGSEA) to visualize the activity of immune functions and the infiltration levels of immune cells in the risk prediction model in terms of the ssGSEA scores through “limma”, “ggpubr” and “reshape2” R packages. Prior to that, we involved the 16 immune cells and 13 immune functions in the calculation of ssGSEA scores.
We performed drug sensitivity prediction with the “pRRophetic” package so as to investigate the therapeutic benefit of EC patients from chemotherapy.
Through wilcoxTest, 101 differentially expressed FRGS were obtained between 552 tumor samples and 35 normal samples, and 13 FRGs might significantly influence the prognosis of EC patients via univariate Cox analysis (Figure 1A). Picking the intersection of the two gene sets, we got 6 candidate FRGs (Supplementary Table S2), namely, TP53, AIFM2, ATG7, TLR4, PANX1 and MDM2 (Figure 1B). In addition, the univariate Cox analysis results revealed that TP53, AIFM2, ATG7, TLR4 and MDM2 were favorable genes, while PANX1 was the unfavorable gene (Figure 1C). In order to visualize the differences of the gene expression in the signature between normal and tumor samples, the heatmap is generated (Figure 1D). Specifically, the expression of TP53, AIFM2, PANX1, ATG7, MDM2 was up-regulated in tumor samples, while TLR4 was down-regulated (Supplementary Table S3). The details of the 6 ferroptosis-related genes were provided in Supplementary Table S4 (Hou et al., 2016; Bersuker et al., 2019; Chen et al., 2019; Doll et al., 2019; Su et al., 2019; Dai et al., 2020; Venkatesh et al., 2020; Zhang et al., 2020; Lei et al., 2021; Zhang Y. et al., 2021; Zhu et al., 2021).
FIGURE 1. The screening of FRGs related to prognosis. (A) The forest plot of 13 prognosis-related FRGs; (B) The Venn plot of intersection FRGs. (C) The forest plot of intersection FRGs; (D) The heatmap of intersection FRGs; (E) The PPI network of the 6 FRGs; (F) The correlation network of the 6 FRGs. Red line: the positive correlation; blue line: the negative correlation.
The PPI results based on the STRING database was disclosed in Figure 1E. And as shown in the correlation network, we observed a positive correlation of coexpression among ATG7, TLR4, MDM2, AIFM2 and TP53, and a negative correlation between PANX1 and AIFM2 (Figure 1F). In brief, both of the two networks confirmed the close relationship among the six candidate genes.
Table 1 showed the coef value of each gene, and the risk score of each sample = (gene1 expression * gene1 coef) + (gene2 expression * gene2 coef) + … + (gene6 expression * gene6 coef). With this method, we determined the risk scores of each sample in two databases, and patients from CPTAC were categorized into two groups with different risks in accordance with the median value in the TCGA database (Figures 2A–D). It was clear that high-risk patients showed a worse survival probability than those with low-risk scores in the Kaplan-Meier curves (Figures 2E,F). And the area under the curve (AUC) of the ROC curve were 0.669, 0.708 and 0.681 at 1, 2 and 3 years, respectively, in the training group (Figure 2G). Similarly, the 1-, 2- and 3-year AUC were 0.654, 0.725 and 0.750 in the testing group (Figure 2H). The results of PCA and t-NSE indicated that the EC patients could be well distinguished according to our risk prediction model (Figures 2I–2L). Taken together, our FRGs signature performed well in predicting prognosis in both training and testing groups.
FIGURE 2. Construction and validation of the prognostic signature. (A) The distribution and median value of the risk scores of patients from the training cohort; (B) The distribution and median value of the risk scores of patients from the testing cohort; (C) Survival status distribution in the training cohort; (D) Survival status distribution in the testing cohort. (E) The Kaplan-Meier curves for the OS of patients in the training group; (F) the Kaplan-Meier curves for the OS of patients in the testing group; (G) the ROC curves in the training group; (H) the ROC curves in the testing group. (I) PCA plot of the training cohort; (J) PCA plot of the testing cohort; (K) t-SNE analysis result of the training cohort; (L) t-SNE analysis result of the testing cohort. (M,N) Independent prognostic factors in the univariate (M) and multivariate Cox regression (N).
The test results of the core FRGs in other cancers suggested that these genes were also significantly associated with multiple cancers survival, including prostate cancer, urothelial cancer, renal cancer, colorectal cancer, testis cancer and cervical cancer (Supplementary Figure S1). Except that the high expression of TLR4 was associated with the poor prognosis of testis cancer, the effects of other genes on the survival of patients with different tumors were all consistent with that of our study. To be a bit more specific, TP53, AIFM2, ATG7 and MDM2 were favorable genes for tumor patients survival, while PANX1 represented a favorable factor affecting the prognosis of patients with renal cancer.
Age, grade, FIGO stage and risk score were brought into the univariate and multivariate Cox regression analyses. As illustrated in Figure 2M, univariate Cox analysis implied that all age, grade, FIGO stage and risk score were significantly correlated with survival. Furthermore, the multivariate Cox analysis results demonstrated that both FIGO stage [hazard ratio (HR): 3.100; 95% confidence interval (CI): 1.975-4.866] and risk score (HR: 1.671; 95% CI: 1.112-2.510) were independent prognostic factors in OS of EC patients (Figure 2N).
Differential genes between two groups were used for GO and KEGG analysis (Figures 3A–D). It was observed from the biological processes category that these genes were mainly involved in cilium movement, axoneme assembly and cilium organization. And the cellular compounds results demonstrated significant enrichment of these genes in motile cilium, axoneme and ciliary plasm. For the category of molecular functions, genes had a marked relationship with G protein-coupled receptor binding and microtubule motor activity.
FIGURE 3. GO and KEGG enrichment analysis results. (A,C) GO and KEGG pathway enrichment analysis in the training cohort; (B,D) GO and KEGG pathway enrichment analysis in the testing cohort; (E,F) GO and KEGG pathway enrichment analysis of the core genes in this signature.
It was noteworthy that we found many cancer and immune-related pathways-related pathways, such as ‘Proteoglycans in cancer’, ‘Hepatocellular carcinoma’, ‘Breast cancer’, ‘Gastric cancer’, ‘Endometrial cancer’, ‘Platinum drug resistance’, ‘p53 signaling pathway’, ‘Cellular senescence’, ‘Cell cycle’, ‘NF-kappa B signaling pathway’ and ‘Human T-cell leukemia virus 1 infection’ from the results of KEGG analysis. In addition, these genes were also involved in ferroptosis-associated pathways, including ‘Arachidonic acid metabolism’, ‘PI3K-Akt signaling pathway’ and ‘Glycine, serine and threonine metabolism’.
Correspondingly, we carried out enrichment analysis for the 6 FRGs to gain more insight into the function of core genes in this signature (Figures 3E,F). GO term showed these genes mainly took part in the biological processes of ‘cellular response to oxidative stress’, ‘cellular response to chemical stress’ and ‘response to oxidative stress’. Remarkably, we noticed that these genes were signaficantly enriched in ‘ferroptosis pathway’ and some cancer-related pathways, suggestting the strong possibility of genes to affect tumor initiation and progression in a ferroptosis manner.
Considering ferroptosis has been documented to play an indispensable part in anti-tumor immunity, we performed ssGSEA to quantify the differences in immune status in our risk model (Figure 4). It was apparent from the ssGSEA results that there was a significant difference in the infiltration of immune cells between the two different risk states on the basis of the immune scores. CD8+ T cells, dendritic cells (DCs), interstitial DCs, neutrophils, T helper cells, Th1, Th2 cells and tumor-infiltrating lymphocytes (TILs) had higher infiltrating scores in the low-risk group. In other words, high abundance of these immune cells may be connected to a relatively good prognosis in EC patients. On the contrary, activated DCs displayed a higher infiltration in high-risk patients. In addition, we can notice that the enrichment of immune functions like antigen-presenting cell (APC) coinhibition, type I interferons (IFN) response and parainflammation were more significant in patients with high-risk scores, while type II IFN response, chemokine receptor (CCR), cytolytic activity, check point, human leukocyte antigen, T cell costimulation and T cell coinhibition were more significantly enriched in low-risk patients.
FIGURE 4. Immune status between high- and low-risk group. (A) Scores of 16 immune cells; (B) Scores of 13 immune-related functions; *p < 0.05; **p < 0.01; ***p < 0.001.
Furthermore, the 6 FRGs were imported into the TIMER database (https://cistrome.shinyapps.io/timer/) (Li et al., 2017) separately to explore the correlation between single FRG and immune infiltration in TCGA (Figure 5). MDM2 was negatively correlated with CD4+T cells (p = 0.0309), but correlated positively with CD8+T cells, macrophages and DC (p < 0.05). AIFM2 had a positively correlation with CD4+T cells (p = 0.00894), and ATG7 positively correlated with B cells, CD8+T cells, macrophages, neutrophils and DC (p < 0.05). In addition, there should be a significantly positive correlation between TLR4 and such immune cells, including B cells, neutrophils, macrophages, CD8+T cells and DC (p < 0.001). PANX1 expression level was positively correlated with neutrophils, CD8+T cells and DC (p < 0.01), and TP53 correlated negatively with B cells and macrophages (p < 0.05).
FIGURE 5. The correlations between the expression of 6 FRGs and immune cells from the TIMER database. (A) TP53 and immune cells; (B) AIFM2 and immune cells; (C) ATG7 and immune cells; (D) TLR4 and immune cells; (E) PANX1 and immune cells; (F) MDM2 and immune cells.
There was a significant difference in the estimated half-maximal inhibitory concentration (IC50) between two risk cohorts (Supplementary Presentation S1). Crucially, compared with the low-risk patients, IC50 values of docetaxel, paclitaxel, sorafenib and rapamycin were lower in the high-risk group (Figure 6), which indicated that the patients with high-risk scores had a more sensitive response to chemotherapy commonly applicated in EC treatment.
FIGURE 6. The correlation between different risk groups and drug sensitivity in EC patients. (A) Docetaxel; (B) Paclitaxel; (C) Sorafenib; (D) Rapamycin.
In order to realize the landscape expansion in EC therapeutic, gigantic efforts have been devoted to unveiling the role of ferroptosis in inducing EC cell death over recent years (Wang et al., 2021a; Zhang M. et al., 2021; Zhang Y. Y. et al., 2021). Moreover, previous studies have attempted to explore the feasibility of ferroptosis in prognosis prediction of EC patients (Liu et al., 2021; Wang et al., 2021b; Weijiao et al., 2021). Compared with them, we have incorporated more updated FRGs in this research. Meanwhile, we directly identified DEGs between the whole EC samples and normal samples, rather than, as in other similar studies, only half of the EC samples were involved in the process due to the existence of internal verification. Both of them make our research more comprehensive and scientific. Futhermore, the conclusions of this study have been verified by an external database rigorously, which is precisely lacking in other studies. In the present study, we first constructed a prognostic model that integrated 6 FRGs in TCGA, namely, TP53, AIFM2, ATG7, TLR4, PANX1 and MDM2. EC patients were categorized into high- and low-risk groups in this signature according to the expression levels of the FRGs and the corresponding coef value. It was found that low-risk cases had a significant survival advantage, which was verified by data from the CPTAC database. Finally, we discovered significant differences between high- and low-risk samples in the immune infiltration according to the prognostic signature. It provides brilliant ideas for improving the prognosis evaluation system and immunotherapy in EC patients.
As taught in the traditional sense, P53, the tumor suppressor, is involved in controlling cell division and survival under a variety of stresses, including cell cycle apoptosis and autophagy. In the past few years, It has been confirmed in both animal models and cell cultures that p53 stands for a fresh ferroptosis regulator (Jiang et al., 2015; Ou et al., 2016; Xie et al., 2017; Tarangelo et al., 2018). Unfortunately, the bidirectional control of p53 in the network of ferroptosis remains unknown and needs to be identified according to the context. In combination, our results indicated a favorable effect of P53 on prognosis in EC patients. AIFM2, a DNA-binding oxidoreductase protein derived from the mitochondria, is characterized as an endogenous ferroptosis suppressor. Remarkably, it has been reported that AIFM2 protects cancer cells from erastin-, sorafenib-, and RSL3-induced ferroptosis via an approach independent of ubiquinol (Bersuker et al., 2019; Doll et al., 2019). Similarly, several studies have shown that AIFM2 represents an unfavorable prognostic factor in prostate cancer, acute myeloid leukemia and uveal melanoma (Luo and Ma, 2021; Lv et al., 2021; Song et al., 2021). However, a recent study has indicated that AIFM2 act as a favorable factor for OS of gastric cancer patients, which is consistent with the findings of our study (Shao et al., 2021). Therefore, it is interesting to explore the effect of AIFM2 on the prognosis of different types of tumors. ATG7, known as a vital autophagy-related gene, exerts a major impact on the formation of the autophagosome. ATG5-ATG7-NCOA4 pathway is critical for the regulation of iron metabolism and knockout of ATG7 can lead to a limited elastin-induced ferroptosis in pancreatic cancer cell lines (Hou et al., 2016). It is an embodiment of the interplay between ferroptosis and autophagy. Likewise, ATG7 predicts a good prognosis in EC patients in our research. Gene TLR4 encodes a transmembrane protein TLR4, responsible for regulating the innate immune response (Moresco et al., 2011). In vitro and in vivo experiments have confirmed that the activation of TLR4 contributes to neuronal ferroptosis through TLR4-p38 MAPK signaling pathway (Zhu et al., 2021). In addition, TLR4/TRIF/type I IFN signaling pathway participates in the recruitment of neutrophils to the injured myocardium in the process of ferroptosis (Li et al., 2019). Notably, TLR4 agonists have aroused widespread concern as a brilliant immunotherapeutic for the treatment of cancer (Shetab Boushehri and Lamprecht, 2018). Recently, protective effects of TLR4 have been identified on the outcome for lung adenocarcinoma (Ren et al., 2021; Zhang A. et al., 2021), which are in good agreement with our signature in EC. More significantly, TLR4 expression is severely attenuated in EC tissues, while its expression levels positively correlate with the infiltration of multiple immune cell types significantly, including B cell (cor = 0.26, p = 7.29e−06), CD8+ T cell (cor = 0.456, p = 2.95e−16), macrophages (cor = 0.312, p = 5.19e−08) and dendritic cells (cor = 0.445, p = 1.22e−15). Hopefully, these findings may thus offer exciting research prospects for immunotherapy of EC. Serving as an E3 ligase, MDM2 has been considered a negative regulator of p53 which can mediate it ubiquitination (Karni-Schmidt et al., 2016). Nevertheless, previous research has also revealed that MDM2 facilitates ferroptosis in cells with or without p53 by altering PPARα activity (Venkatesh et al., 2020). Although MDM2 downregulation in EC tissues may inhibit the progression of EC via suppressing the migration, invasion and anti-apoptosis of EC cells (Liu et al., 2019). The effect of MDM2 expression on the prognosis of EC is still controversial. But it is undeniable that there is a strong positive correlation between the expression of MDM2 and CD8+ T cells in the tumor microenvironment.
As a damage-associated molecular pattern (DAMP) molecule, PANX1 represents a key player in modulating ATP release. And PANX1 knockout protects against renal ischemia/reperfusion injury through attenuating MAPK/ERK activation in a ferroptotic pathway. Moreover, it is reported that PANX1 promotes tumorigenesis in breast cancer through EMT pathway.
Damage-related molecules are released along with the process of ferroptosis, which are perceived by immune cells to amplify the inflammatory response. With the development of modern medicine, people are increasingly conscious of the crucial role of the immune microenvironment in cancer treatment (López-Janeiro et al., 2021). In this article, we performed ssGSEA to evaluate the immune status in different risk groups. Undoubtedly, in contrast to the previous model (Weijiao et al., 2021), low-risk patients were present with more abundant immune cells infiltration and immune functions enrichment in ours. As the most important anti-tumor effector cells, activated CD8+ T cells eliminate tumor cells through recognition by their expressed T cell receptors of tumor-associated antigens on major histocompatibility complex I (MHC I) (Gajewski et al., 2013). The high infiltration of CD8+ T cells is associated with favorable survival outcomes in EC (Qin et al., 2021). DCs bridge the gap between the innate and the adaptive immune systems (Hinshaw and Shevde, 2019). And numerous strategies in the fight against cancer have been developed, which exactly target DCs (Wculek et al., 2020). Th1 and Th2, T helper cells subclasses, are involved in molecular crosstalk among different immune signaling pathways, whose relevance in tumor immunotherapy is under investigation. In particular, Th1 cells can kill tumor cells directly by activating death receptors on the surface of them, enhance CD8+ T cells priming and expansion via cytokines release and promote natural killer cells and type I macrophages to recruit to tumors (Knutson and Disis, 2005; LaCasse et al., 2011; Kim and Cantor, 2014). In addition, increased Th1 cells have been reported to be associated with protracted disease-free survival in colorectal cancer patients (Tosolini et al., 2011).
The decline of human leukocyte antigen (HLA) function will cause the loss of HLA-I expression on the surface of tumor cells, thereby leading to deficiencies in the antigen presentation pathway and helping tumors escape from immunotherapy (2018; Hazini et al., 2021). It is clear that the HLA function was significantly insufficient in high-risk patients. Parainflammation is a low-grade inflammatory response between primary dynamic balance and chronic inflammation. Animal experiments have suggested that parainflammation attenuation can prevent tumor growth, which may be related to its inability to recruit immune cells (Lasry et al., 2017). The antitumoral immune cytolytic activity of the patients is calculated from the geometric mean of perforin 1 (PRF1) and granzyme A (GZMA) genes expression (Rooney et al., 2015; Narayanan et al., 2018). While the overexpression of these two effector molecules is upon the activation of CD8+T cells and high scores of checkpoint in the low-risk group (Johnson et al., 2003). This also validates the emphasis that neoadjuvant therapy combined with multiple immune checkpoints expands the clinical benefits of tumor patients (Zaravinos et al., 2019). To add to that, coupled with the poor enrichment of APC costimulation, type II IFN response and T cell costimulation, the immune response to EC cells is suppressed in the high-risk group.
Furthermore, the response to chemotherapy in high-risk patients was significantly stronger than that in the low-risk group, which may provide more rational choices in the treatment for patients at a high risk.
In the current study, we identified a prognostic signature consisting of 6 FRGs with novel forecast value in the OS of EC. Significantly, we verified the results based on an external database for the first time. The ssGSEA results pointed out a tight link between ferroptosis and the tumor immune microenvironment, which is expected to provide a new prospect for the immunotherapy of EC in future work.
The datasets presented in this study can be found in online repositories. The names of the repository/repositories and accession number(s) can be found below: https://portal.gdc.cancer.gov/, TCGA-UCEC; https://proteomics.cancer.gov/programs/cptac, 3.
Ethical review and approval was not required for the study on human participants in accordance with the local legislation and institutional requirements. Written informed consent for participation was not required for this study in accordance with the national legislation and the institutional requirements.
SL and QZ drafted the work, selected search engines and search terms, analyzed the database and approved the final draft. WL prepared the figures and tables and approved the final draft. XH analyzed the data, revised the work critically for important content and approved the final draft.
This work were supported by the S&T Program of Hebei (21377706D) and the innovation ability of Hebei Obstetrics and Gynaecology Clinical Medicine Research Centre (20577705D).
The authors declare that the research was conducted in the absence of any commercial or financial relationships that could be construed as a potential conflict of interest.
All claims expressed in this article are solely those of the authors and do not necessarily represent those of their affiliated organizations, or those of the publisher, the editors and the reviewers. Any product that may be evaluated in this article, or claim that may be made by its manufacturer, is not guaranteed or endorsed by the publisher.
We would like to express our deepest gratitude to all of those who helped us in this paper.
The Supplementary Material for this article can be found online at: https://www.frontiersin.org/articles/10.3389/fmolb.2022.916689/full#supplementary-material
EC, endometrial cancer; FRGs, ferroptosis-related genes; TCGA, The Cancer Genome Atlas; CPTAC, Clinical Proteomic Tumor Analysis Consortium; FDR, false discovery rate; OS, overall survival; LASSO, least absolute shrinkage and selection operator; ROC, receiver operating characteristic; t-SNE, t-Distributed Stochastic Neighbor Embedding; PCA, Principal component analysis; FC, fold change; GO, Gene Ontology; KEGG, Kyoto Encyclopedia of Genes and Genomes; ssGSEA, Single sample gene set enrichment analysis; PPI, protein-protein interactions; AUC, area under the curve; HR, hazard ratio; CI, confidence interval; DCs, dendritic cells; TILs, tumor-infiltrating lymphocytes; APC, antigen-presenting cell; IFN, interferons.
Author Anonymous, (2018). HLA Loss Facilitates Immune Escape. Cancer Discov. 8 (1), 8. doi:10.1158/2159-8290.CD-NB2017-166
Bersuker, K., Hendricks, J. M., Li, Z., Magtanong, L., Ford, B., Tang, P. H., et al. (2019). The CoQ Oxidoreductase FSP1 Acts Parallel to GPX4 to Inhibit Ferroptosis. Nature 575 (7784), 688–692. doi:10.1038/s41586-019-1705-2
Chen, X., Xu, S., Zhao, C., and Liu, B. (2019). Role of TLR4/NADPH Oxidase 4 Pathway in Promoting Cell Death through Autophagy and Ferroptosis during Heart Failure. Biochem. Biophysical Res. Commun. 516, 37–43. doi:10.1016/j.bbrc.2019.06.015
Cui, Z., Wang, H., Li, S., Qin, T., Shi, H., Ma, J., et al. (2022). Dihydroartemisinin Enhances the Inhibitory Effect of Sorafenib on HepG2 Cells by Inducing Ferroptosis and Inhibiting Energy Metabolism. J. Pharmacol. Sci. 148 (1), 73–85. doi:10.1016/j.jphs.2021.09.008
Dai, E., Zhang, W., Cong, D., Kang, R., Wang, J., and Tang, D. (2020). AIFM2 Blocks Ferroptosis Independent of Ubiquinol Metabolism. Biochem. Biophysical Res. Commun. 523, 966–971. doi:10.1016/j.bbrc.2020.01.066
Doll, S., Freitas, F. P., Shah, R., Aldrovandi, M., da Silva, M. C., Ingold, I., et al. (2019). FSP1 Is a Glutathione-independent Ferroptosis Suppressor. Nature 575 (7784), 693–698. doi:10.1038/s41586-019-1707-0
Edwards, N. J., Oberti, M., Thangudu, R. R., Cai, S., McGarvey, P. B., Jacob, S., et al. (2015). The CPTAC Data Portal: A Resource for Cancer Proteomics Research. J. Proteome Res. 14 (6), 2707–2713. doi:10.1021/pr501254j
Gajewski, T. F., Schreiber, H., and Fu, Y.-X. (2013). Innate and Adaptive Immune Cells in the Tumor Microenvironment. Nat. Immunol. 14 (10), 1014–1022. doi:10.1038/ni.2703
Hassannia, B., Vandenabeele, P., and Vanden Berghe, T. (2019). Targeting Ferroptosis to Iron Out Cancer. Cancer Cell. 35 (6), 830–849. doi:10.1016/j.ccell.2019.04.002
Hazini, A., Fisher, K., and Seymour, L. (2021). Deregulation of HLA-I in Cancer and its Central Importance for Immunotherapy. J. Immunother. Cancer 9 (8). doi:10.1136/jitc-2021-002899
Hinshaw, D. C., and Shevde, L. A. (2019). The Tumor Microenvironment Innately Modulates Cancer Progression. Cancer Res. 79 (18), 4557–4566. doi:10.1158/0008-5472.can-18-3962
Hou, W., Xie, Y., Song, X., Sun, X., Lotze, M. T., Zeh, H. J., et al. (2016). Autophagy Promotes Ferroptosis by Degradation of Ferritin. Autophagy 12 (8), 1425–1428. doi:10.1080/15548627.2016.1187366
Jiang, L., Kon, N., Li, T., Wang, S.-J., Su, T., Hibshoosh, H., et al. (2015). Ferroptosis as a P53-Mediated Activity during Tumour Suppression. Nature 520 (7545), 57–62. doi:10.1038/nature14344
Johnson, B. J., Costelloe, E. O., Fitzpatrick, D. R., Haanen, J. B. A. G., Schumacher, T. N. M., Brown, L. E., et al. (2003). Single-cell Perforin and Granzyme Expression Reveals the Anatomical Localization of Effector CD8 + T Cells in Influenza Virus-Infected Mice. Proc. Natl. Acad. Sci. U.S.A. 100 (5), 2657–2662. doi:10.1073/pnas.0538056100
Karni-Schmidt, O., Lokshin, M., and Prives, C. (2016). The Roles of MDM2 and MDMX in Cancer. Annu. Rev. Pathol. Mech. Dis. 11, 617–644. doi:10.1146/annurev-pathol-012414-040349
Kim, H.-J., and Cantor, H. (2014). CD4 T-Cell Subsets and Tumor Immunity: the Helpful and the Not-so-helpful. Cancer Immunol. Res. 2 (2), 91–98. doi:10.1158/2326-6066.cir-13-0216
Knutson, K. L., and Disis, M. L. (2005). Tumor Antigen-specific T Helper Cells in Cancer Immunity and Immunotherapy. Cancer Immunol. Immunother. 54 (8), 721–728. doi:10.1007/s00262-004-0653-2
LaCasse, C. J., Janikashvili, N., Larmonier, C. B., Alizadeh, D., Hanke, N., Kartchner, J., et al. (2011). Th-1 Lymphocytes Induce Dendritic Cell Tumor Killing Activity by an IFN-γ-dependent Mechanism. J. Immunol. 187 (12), 6310–6317. doi:10.4049/jimmunol.1101812
Lasry, A., Aran, D., Butte, A. J., and Ben-Neriah, Y. (2017). Cancer Cell-Autonomous Parainflammation Mimics Immune Cell Infiltration. Cancer Res. 77 (14), 3740–3744. doi:10.1158/0008-5472.can-16-3383
Lei, G., Zhang, Y., Hong, T., Zhang, X., Liu, X., Mao, C., et al. (2021). Ferroptosis as a Mechanism to Mediate P53 Function in Tumor Radiosensitivity. Oncogene 40, 3533–3547. doi:10.1038/s41388-021-01790-w
Li, H., and Liu, L. (2022). Zinc Moderates Circular RNA CircFOXP1 Expression in Order to Regulate Ferroptosis during Lung Adenocarcinoma. Chem. Biol. Interact. 352, 109760. doi:10.1016/j.cbi.2021.109760
Li, T., Fan, J., Wang, B., Traugh, N., Chen, Q., Liu, J. S., et al. (2017). TIMER: A Web Server for Comprehensive Analysis of Tumor-Infiltrating Immune Cells. Cancer Res. 77 (21), e108–e110. doi:10.1158/0008-5472.CAN-17-0307
Li, W., Feng, G., Gauthier, J. M., Lokshina, I., Higashikubo, R., Evans, S., et al. (2019). Ferroptotic Cell Death and TLR4/Trif Signaling Initiate Neutrophil Recruitment after Heart Transplantation. J. Clin. Invest. 129 (6), 2293–2304. doi:10.1172/jci126428
Liu, J., Wang, Y., Meng, H., Yin, Y., Zhu, H., and Ni, T. (2021). Identification of the Prognostic Signature Associated with Tumor Immune Microenvironment of Uterine Corpus Endometrial Carcinoma Based on Ferroptosis-Related Genes. Front. Cell. Dev. Biol. 9, 735013. doi:10.3389/fcell.2021.735013
Liu, L., Yang, L., Chang, H., Chen, Y. N., Zhang, F., Feng, S., et al. (2019). CP31398 Attenuates Endometrial Cancer Cell Invasion, Metastasis and Resistance to Apoptosis by Downregulating MDM2 Expression. Int. J. Oncol. 54 (3), 942–954. doi:10.3892/ijo.2019.4681
Lortet-Tieulent, J., Ferlay, J., Bray, F., and Jemal, A. (2018). International Patterns and Trends in Endometrial Cancer Incidence, 1978-2013. J. Natl. Cancer Inst. 110 (4), 354–361. doi:10.1093/jnci/djx214
Luo, H., and Ma, C. (2021). A Novel Ferroptosis-Associated Gene Signature to Predict Prognosis in Patients with Uveal Melanoma. Diagn. (Basel). 11 (2), 20219. doi:10.3390/diagnostics11020219
Lv, Z., Wang, J., Wang, X., Mo, M., Tang, G., Xu, H., et al. (2021). Identifying a Ferroptosis-Related Gene Signature for Predicting Biochemical Recurrence of Prostate Cancer. Front. Cell. Dev. Biol. 9, 666025. doi:10.3389/fcell.2021.666025
Moresco, E. M. Y., LaVine, D., and Beutler, B. (2011). Toll-like Receptors. Curr. Biol. 21 (13), R488–R493. doi:10.1016/j.cub.2011.05.039
Mou, Y., Wang, J., Wu, J., He, D., Zhang, C., Duan, C., et al. (2019). Ferroptosis, a New Form of Cell Death: Opportunities and Challenges in Cancer. J. Hematol. Oncol. 12 (1), 34. doi:10.1186/s13045-019-0720-y
Narayanan, S., Kawaguchi, T., Yan, L., Peng, X., Qi, Q., and Takabe, K. (2018). Cytolytic Activity Score to Assess Anticancer Immunity in Colorectal Cancer. Ann. Surg. Oncol. 25 (8), 2323–2331. doi:10.1245/s10434-018-6506-6
Ou, Y., Wang, S. J., Li, D., Chu, B., and Gu, W. (2016). Activation of SAT1 Engages Polyamine Metabolism with P53-Mediated Ferroptotic Responses. Proc. Natl. Acad. Sci. U. S. A. 113 (44), E6806–E6812. doi:10.1073/pnas.1607152113
Qin, M., Hamanishi, J., Ukita, M., Yamanoi, K., Takamatsu, S., Abiko, K., et al. (2021). Tertiary Lymphoid Structures Are Associated with Favorable Survival Outcomes in Patients with Endometrial Cancer. Cancer Immunol Immunother 71, 7431. doi:10.1007/s00262-021-03093-1
Ren, Z., Hu, M., Wang, Z., Ge, J., Zhou, X., Zhang, G., et al. (2021). Ferroptosis-Related Genes in Lung Adenocarcinoma: Prognostic Signature and Immune, Drug Resistance, Mutation Analysis. Front. Genet. 12, 672904. doi:10.3389/fgene.2021.672904
Rooney, M. S., Shukla, S. A., Wu, C. J., Getz, G., and Hacohen, N. (2015). Molecular and Genetic Properties of Tumors Associated with Local Immune Cytolytic Activity. Cell. 160 (1-2), 48–61. doi:10.1016/j.cell.2014.12.033
López-Janeiro, A., Ruz-Caracuel, I., Ramón-Patino, J. L., De Los Ríos, V., Villalba Esparza, M., Berjón, A., et al. (2021). Proteomic Analysis of Low-Grade, Early-Stage Endometrial Carcinoma Reveals New Dysregulated Pathways Associated with Cell Death and Cell Signaling. Cancers (Basel). 13 (4), 794. doi:10.3390/cancers13040794
Shao, Y., Jia, H., Li, S., Huang, L., Aikemu, B., Yang, G., et al. (2021). Comprehensive Analysis of Ferroptosis-Related Markers for the Clinical and Biological Value in Gastric Cancer. Oxid. Med. Cell. Longev. 2021, 7007933. doi:10.1155/2021/7007933
Shetab Boushehri, M. A., and Lamprecht, A. (2018). TLR4-Based Immunotherapeutics in Cancer: A Review of the Achievements and Shortcomings. Mol. Pharm. 15 (11), 4777–4800. doi:10.1021/acs.molpharmaceut.8b00691
Song, Y., Tian, S., Zhang, P., Zhang, N., Shen, Y., and Deng, J. (2021). Construction and Validation of a Novel Ferroptosis-Related Prognostic Model for Acute Myeloid Leukemia. Front. Genet. 12, 708699. doi:10.3389/fgene.2021.708699
Stockwell, B. R., Friedmann Angeli, J. P., Bayir, H., Bush, A. I., Conrad, M., Dixon, S. J., et al. (2017). Ferroptosis: A Regulated Cell Death Nexus Linking Metabolism, Redox Biology, and Disease. Cell. 171 (2), 273–285. doi:10.1016/j.cell.2017.09.021
Su, L., Jiang, X., Yang, C., Zhang, J., Chen, B., Li, Y., et al. (2019). Pannexin 1 Mediates Ferroptosis that Contributes to Renal Ischemia/reperfusion Injury. J. Biol. Chem. 294, 19395–19404. doi:10.1074/jbc.RA119.010949
Sun, J., Cheng, X., Pan, S., Wang, L., Dou, W., Liu, J., et al. (2021). Dichloroacetate Attenuates the Stemness of Colorectal Cancer Cells via Trigerring Ferroptosis through Sequestering Iron in Lysosomes. Environ. Toxicol. 36 (4), 520–529. doi:10.1002/tox.23057
Sung, H., Ferlay, J., Siegel, R. L., Laversanne, M., Soerjomataram, I., Jemal, A., et al. (2021). Global Cancer Statistics 2020: GLOBOCAN Estimates of Incidence and Mortality Worldwide for 36 Cancers in 185 Countries. CA A Cancer J. Clin. 71 (3), 209–249. doi:10.3322/caac.21660
Szklarczyk, D., Gable, A. L., Nastou, K. C., Lyon, D., Kirsch, R., Pyysalo, S., et al. (2021). The STRING Database in 2021: Customizable Protein-Protein Networks, and Functional Characterization of User-Uploaded Gene/measurement Sets. Nucleic Acids Res. 49 (D1), D605–D612. doi:10.1093/nar/gkaa1074
Tarangelo, A., Magtanong, L., Bieging-Rolett, K. T., Li, Y., Ye, J., Attardi, L. D., et al. (2018). p53 Suppresses Metabolic Stress-Induced Ferroptosis in Cancer Cells. Cell. Rep. 22 (3), 569–575. doi:10.1016/j.celrep.2017.12.077
Tosolini, M., Kirilovsky, A., Mlecnik, B., Fredriksen, T., Mauger, S., Bindea, G., et al. (2011). Clinical Impact of Different Classes of Infiltrating T Cytotoxic and Helper Cells (Th1, Th2, Treg, Th17) in Patients with Colorectal Cancer. Cancer Res. 71 (4), 1263–1271. doi:10.1158/0008-5472.can-10-2907
Venkatesh, D., O'Brien, N. A., Zandkarimi, F., Tong, D. R., Stokes, M. E., Dunn, D. E., et al. (2020). MDM2 and MDMX Promote Ferroptosis by PPARα-Mediated Lipid Remodeling. Genes Dev. 34 (7-8), 526–543. doi:10.1101/gad.334219.119
Wang, H., Wu, Y., Chen, S., Hou, M., Yang, Y., and Xie, M. (2021b). Construction and Validation of a Ferroptosis-Related Prognostic Model for Endometrial Cancer. Front. Genet. 12, 729046. doi:10.3389/fgene.2021.729046
Wang, H., Peng, S., Cai, J., and Bao, S. (2021a). Silencing of PTPN18 Induced Ferroptosis in Endometrial Cancer Cells through P-P38-Mediated GPX4/xCT Down-Regulation. Cmar Vol. 13, 1757–1765. doi:10.2147/cmar.s278728
Wculek, S. K., Cueto, F. J., Mujal, A. M., Melero, I., Krummel, M. F., and Sancho, D. (2020). Dendritic Cells in Cancer Immunology and Immunotherapy. Nat. Rev. Immunol. 20 (1), 7–24. doi:10.1038/s41577-019-0210-z
Weijiao, Y., Fuchun, L., Mengjie, C., Xiaoqing, Q., Hao, L., Yuan, L., et al. (2021). Immune Infiltration and a Ferroptosis-Associated Gene Signature for Predicting the Prognosis of Patients with Endometrial Cancer. Aging 13 (12), 16713–16732. doi:10.18632/aging.203190
Xie, Y., Zhu, S., Song, X., Sun, X., Fan, Y., Liu, J., et al. (2017). The Tumor Suppressor P53 Limits Ferroptosis by Blocking DPP4 Activity. Cell. Rep. 20 (7), 1692–1704. doi:10.1016/j.celrep.2017.07.055
Ye, Z., Zhuo, Q., Hu, Q., Xu, X., Mengqi liu, fnm, Zhang, Z., et al. (2021). FBW7-NRA41-SCD1 axis Synchronously Regulates Apoptosis and Ferroptosis in Pancreatic Cancer Cells. Redox Biol. 38, 101807. doi:10.1016/j.redox.2020.101807
Zaravinos, A., Roufas, C., Nagara, M., de Lucas Moreno, B., Oblovatskaya, M., Efstathiades, C., et al. (2019). Cytolytic Activity Correlates with the Mutational Burden and Deregulated Expression of Immune Checkpoints in Colorectal Cancer. J. Exp. Clin. Cancer Res. 38 (1), 364. doi:10.1186/s13046-019-1372-z
Zhang, Z., Guo, M., Shen, M., Kong, D., Zhang, F., Shao, J., et al. (2020). The BRD7-P53-Slc25a28 axis Regulates Ferroptosis in Hepatic Stellate Cells. Redox Biol. 36, 101619. doi:10.1016/j.redox.2020.101619
Zhang, A, A., Yang, J., Ma, C., Li, F., and Luo, H. (2021). Development and Validation of a Robust Ferroptosis-Related Prognostic Signature in Lung Adenocarcinoma. Front. Cell. Dev. Biol. 9, 616271. doi:10.3389/fcell.2021.616271
Zhang, H, H., Ge, Z., Wang, Z., Gao, Y., Wang, Y., and Qu, X. (2021). Circular RNA RHOT1 Promotes Progression and Inhibits Ferroptosis via mir-106a-5p/STAT3 axis in Breast Cancer. Aging 13 (6), 8115–8126. doi:10.18632/aging.202608
Zhang, M, M., Zhang, T., Song, C., Qu, J., Gu, Y., Liu, S., et al. (2021). Guizhi Fuling Capsule Ameliorates Endometrial Hyperplasia through Promoting P62-Keap1-NRF2-Mediated Ferroptosis. J. Ethnopharmacol. 274, 114064. doi:10.1016/j.jep.2021.114064
Zhang, Y, Y., Xia, M., Zhou, Z., Hu, X., Wang, J., Zhang, M., et al. (2021). p53 Promoted Ferroptosis in Ovarian Cancer Cells Treated with Human Serum Incubated-Superparamagnetic Iron Oxides. Int. J. Nano. Vol. 16, 283–296. doi:10.2147/IJN.S282489
Zhang, Y. Y, Y.-Y., Ni, Z.-J., Elam, E., Zhang, F., Thakur, K., Wang, S., et al. (2021). Juglone, a Novel Activator of Ferroptosis, Induces Cell Death in Endometrial Carcinoma Ishikawa Cells. Food Funct. 12 (11), 4947–4959. doi:10.1039/d1fo00790d
Zhao, L., Peng, Y., He, S., Li, R., Wang, Z., Huang, J., et al. (2021). Apatinib Induced Ferroptosis by Lipid Peroxidation in Gastric Cancer. Gastric Cancer 24 (3), 642–654. doi:10.1007/s10120-021-01159-8
Zhou, N., and Bao, J. (2020). FerrDb: a Manually Curated Resource for Regulators and Markers of Ferroptosis and Ferroptosis-Disease Associations, Database (Oxford) 2020, 2020. doi:10.1093/database/baaa021
Keywords: ferroptosis, endometrial cancer, prognosis, signature, immune microenvironment
Citation: Liu S, Zhang Q, Liu W and Huang X (2022) Prediction of Prognosis in Patients With Endometrial Carcinoma and Immune Microenvironment Estimation Based on Ferroptosis-Related Genes. Front. Mol. Biosci. 9:916689. doi: 10.3389/fmolb.2022.916689
Received: 09 April 2022; Accepted: 20 June 2022;
Published: 15 July 2022.
Edited by:
Stella Liberman-Aronov, Ariel University, IsraelReviewed by:
Li-Xing Yang, National Cheng Kung University, TaiwanCopyright © 2022 Liu, Zhang, Liu and Huang. This is an open-access article distributed under the terms of the Creative Commons Attribution License (CC BY). The use, distribution or reproduction in other forums is permitted, provided the original author(s) and the copyright owner(s) are credited and that the original publication in this journal is cited, in accordance with accepted academic practice. No use, distribution or reproduction is permitted which does not comply with these terms.
*Correspondence: Xianghua Huang, eGlhbmdodWFodWFuZzAzMTFAMTYzLmNvbQ==
†These authors have contributed equally to this work
Disclaimer: All claims expressed in this article are solely those of the authors and do not necessarily represent those of their affiliated organizations, or those of the publisher, the editors and the reviewers. Any product that may be evaluated in this article or claim that may be made by its manufacturer is not guaranteed or endorsed by the publisher.
Research integrity at Frontiers
Learn more about the work of our research integrity team to safeguard the quality of each article we publish.