- 1Department of Ecological and Biological Sciences, University of Tuscia, Viterbo, Italy
- 2Department of Innovativative Biology, Agro-food, and Forestry, University of Tuscia, Viterbo, Italy
Coronavirus disease 2019 (COVID 19) is a systemic infection that exerts a significant impact on cell metabolism. In this study we performed metabolomic profiling of 41 in vitro cultures of peripheral blood mononuclear cells (PBMC), 17 of which displayed IgG memory for spike-S1 antigen 60–90 days after infection. By using mass spectrometry analysis, a significant up-regulation of S-adenosyl-Homocysteine, Sarcosine and Arginine was found in leukocytes showing IgG memory. These metabolites are known to be involved in physiological recovery from viral infections and immune activities, and our findings might represent a novel and easy measure that could be of help in understanding SARS-Cov-2 effects on leukocytes.
Introduction
The SARS-CoV-2 virus induces an unprecedented pandemic (COVID-19) characterized by a range of respiratory symptoms that may progress to acute respiratory distress syndrome (ARDS), and multi-organ dysfunction (Zhou et al., 2020). SARS-CoV-2 triggers both innate and specific immune responses, and once the virus gains access to the target cell, the host’s immune system mainly recognizes its surface epitopes including the spike-S1 protein (Shen et al., 2020). Among other effects, a recent study showed that COVID-19 leads to a reduced population of regulatory lymphocytes, inducing an increase in inflammatory responses, cytokine production, and proceeds toward tissue damage and systemic deficiency of organs (Shah et al., 2020). However, there is very limited understanding of the immune responses, especially adaptive immune responses to SARS-CoV-2 infection. To this regard Ni, Ling et al., characterized SARS-CoV-2-specific humoral and cellular immunity in recovered patients funding that both B and T cells participate in immune-mediated protection against viral infection (Ni et al., 2020). In addition, Turner JS et al., conducted a study among recovered individuals who experienced mild SARS-CoV-2 infection. The authors reported that mild infection induced a robust antigen-specific, long-lived humoral immune memory in humans (Turner et al., 2020). Although longer follow-up studies are needed, the first evidence confirms the protective effect of recovery from the previous infection. Hence, it is conceivable to speculate that at later stages after infection, patients that recovered from the pathology developed an immune response with memory and maintained antiviral defense, however the cellular physiological pathways underlying these mechanisms remain to be better understood. To this, a piece of knowledge on cellular processes that could be related to an infection can be achieved through metabolomics, an emerging tool applied to systems immunology platforms and already employed in COVID-19 studies (Diray-Arce et al., 2020). This preliminary study aimed to investigate possible relationships between leukocytes displaying an IgG antibody memory to SARS-CoV-2 (Zarletti et al., 2020) and their metabolic profile, to detect possible metabolites involved in the late stages of antiviral defenses.
Results
Metabolites were extracted from peripheral blood mononuclear cells (PBMC) cultures displaying an anti-spike-S1 IgG-memory (IgGm+; N = 17) and from PBMC without measurable IgG-memory (IgGm-; N = 24) measured by Cell-ELISA 60–90 days after SARS-Cov-2 screening. Supplementary Table S1 shows age/gender and Cell-ELISA levels for each subject. We use MetaboAnalyst 5.0 platforms to perform untargeted metabolomics and identify the most relevant metabolites altered in IgGm + subjects, and results indicate that the two different groups are well clustered, as shown in Figure 1A by the supervised Least-Squares Discriminant Analysis (PLS-DA), with red spots representing IgGm + samples and green spots IgGm-. R2 and Q2 values were thus calculated as measures of prediction accuracy (accuracy 0.82, R2 > 0.71, Q2 > 0.33; data not shown). Multivariate analysis suggests significant variations present in IgGm + subjects. The loading plot of metabolites in the analyzed samples projected into the PLS-DA model is shown in Figure 1B. The significantly-discriminated metabolites were identified using the Volcano plot analysis (Figure 1C). The univariate analysis identified a significant accumulation of specific metabolites most of which were expressed in IgGm + leukocytes. The metabolites that displayed a major alteration linked to physiological recovery from viral infections and immune activities, were arginine, sarcosine and s-adenosyl-homocysteine. The last two metabolites were involved in the methionine cycle (Figure 2).
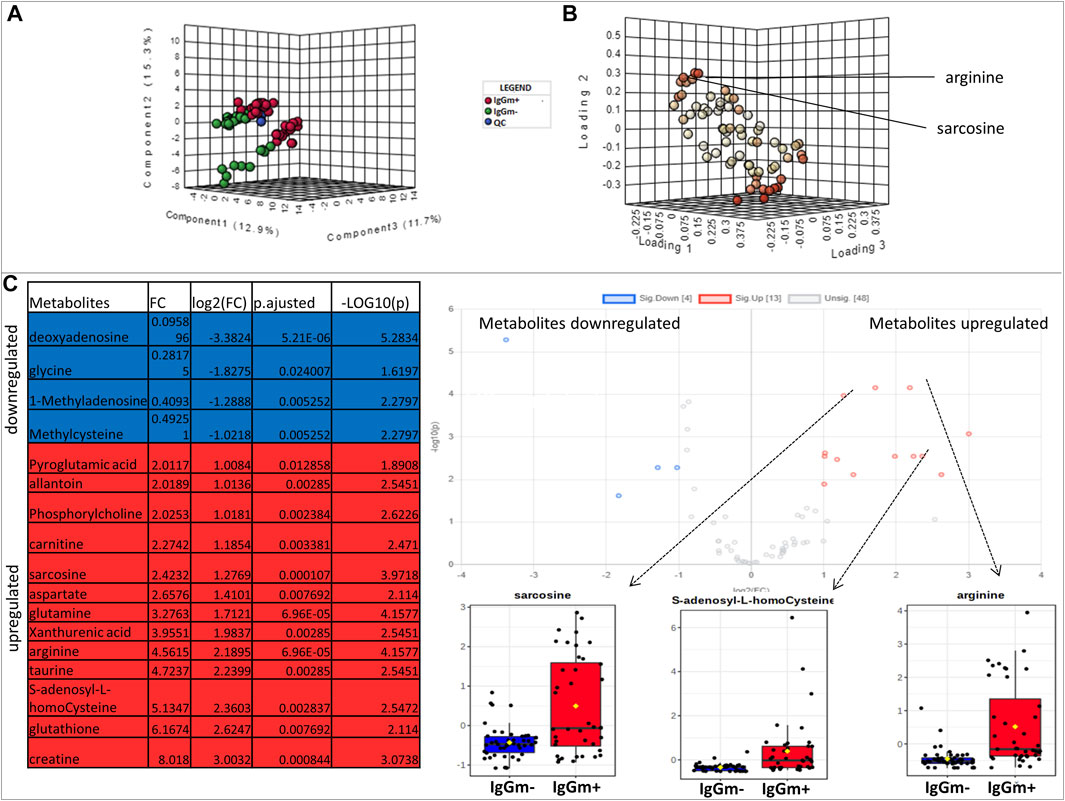
FIGURE 1. (A) Multivariate statistical analysis based on untargeted metabolite profile data derived from leukocytes of patients IgGm+ (Red), IgGm- (green) and QC (Blue) (B) shows the loading plot of the metabolites in the analyzed samples projected into the PLS-DA model. The loading plot is used to assess the features that have the greatest influence on each component and red spots represent metabolites that have strong contributions to the separation in the loading plot (C) Volcano plot showing the most significant metabolites found by univariate analysis. The table represents the results of volcano plots: for volcano plot analysis the conditions of fold change were ≥1.5 (x-axis) and the false discovery rate-adjusted p-value threshold was set at ≤ 0.05 (y-axis). The levels of metabolites were significantly different in the IgGm + compared to IgGm- The volcano plot summarizes both fold-change and t-test criteria for all metabolites. The blue-highlighted table shows metabolites up-regulated in IgGm-leukocytes, whereas in the red-highlighted metabolites are up-regulated in IgGm + leukocytes. The more significantly altered metabolites in IgGm + leukocytes are displayed as bar plots and were sarcosine, s-adenosyl-homocysteine, and arginine.
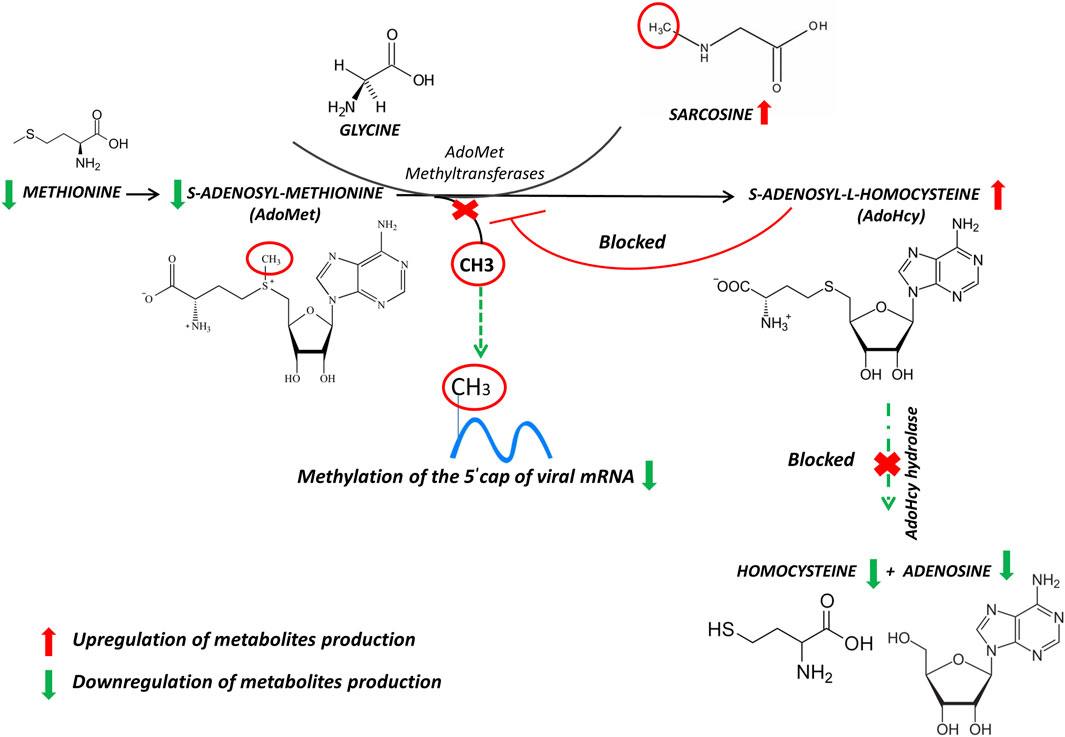
FIGURE 2. Reactions of methionine metabolism. S-adenosyl-methionine (SAM) donates its methyl group to acceptor molecules, sarcosine, generating S-adenosylhomocysteine (SAH) limiting methylation of the 5′ cap of viral messenger RNA. The arrows represent the trend of our results. Up-modulations are in red, whereas down-modulations are in green.
Discussion
The SARS-CoV-2 induces an antibody memory against the spike-S1 antigen that can be measured by in vitro assays in PBMC from 2 months onward after infection (Zarletti et al., 2020), and our work aimed to investigate metabolic features in these conditions. Through metabolomic analysis, the results showed that in vitro cultured and unstimulated PBMC display a significant up-regulation of metabolically-related metabolites sarcosine, s-adenosyl-L-homocysteine and arginine.
The S-adenosyl-methionine (AdoMet, also frequently abbreviated as SAM and SAMe) is widely known as a main biological methyl donor converted to s-adenosyl-L-homocysteine (AdoHcy), and sarcosine as an acceptor of the methyl group. The SAM to AdoHcy ratio depends also on the availability of methionine, which is converted to SAM by the enzyme methionine adenosyltransferase (MAT). AdoHcy cellular content is regulated by the enzyme AdoHcy hydrolase (AHCY), which reversibly cleaves this molecule into adenosine and homocysteine (Hcy). The thermodynamics of AHCY stimulates AdoHcy biosynthesis rather than hydrolysis, and in vivo, the reaction proceeds in the direction of hydrolysis only if the products, adenosine and Hcy, are rapidly removed. This is essential to prevent the accumulation of AdoHcy (Figure 2) which is a potent inhibitor of methylation reactions (Tehlivets et al., 2013).
The ratio of AdoMet to AdoHcy is frequently considered a metabolic gauge controlling in vivo methylation reactions, where a decrease in this ratio predicts reduced methylation capacity (Czarnecka et al., 2020). These methylations are required for the 5′-cap formation of viral mRNAs. The RNA cap has multiple roles in gene expression, including enhancement of RNA stability, splicing, nucleocytoplasmic transport, and translation initiation, necessary for viral RNA replication (Byszewska et al., 2014).
So since the methylation (capping) of viral RNA is necessary for its life cycle, a high SAM level and high methylation index are associated with the risk of lung injury in patients with COVID-19 (Kryukov et al., 2021). Metabolomic analyses revealed that SAM was significantly elevated in critical cases of COVID-19 and those with a fatal outcome as compared to control, mild and moderate cases of COVID-19 (Roberts et al., 2021; Perła-Kaján and Jakubowski, 2022).
In this respect, inhibition of the enzyme AdoHcy hydrolase can be used as a therapy against virus infection, because indirectly limits the bioavailability of SAM and methylation of the 5′ cap of viral messenger RNA as already noticed for both Ebola virus and African swine fever virus (Villalón et al., 1993; Bray et al., 2000). Moreover, it should be noted that RNA methylation in SARS Cov-2 prevents its degradation by host nucleases (Wilamowski et al., 2021) and thus might induce long-lasting effects in leukocyte metabolism. To our results, the strong increase of AdoHcy allowed us to postulate an inhibition of s-adenosyl-L-homocysteine hydrolase, and consequently, imbalance of SAM/AdoHcy ratio. Furthermore, the restricted level of methionine availability may inhibit or eliminate the replication as well as prevent proliferation of cells infected with SARS-CoV-2 (Hoffman and Han, 2020). Hence, It is also conceivable to conclude that blocking capping viral mRNAs could be an initial step to developing therapeutics that could help the antiviral therapies.
Interestingly, we also observed a strong up-regulation of arginine in IgGm + leukocytes, in addition to AdoHcy and sarcosine. Arginine is a substrate for nitric oxide (NO) production, which can induce antiviral activity against RNA viruses, such as SARS-CoV-2 (Tatum et al., 2020). The up-rugualted of the arginase 1 (Arg1), enzyme that metabolizes arginine to ornithine and urea, was associated with higher virus load in the PBMC of COVID-19 patients (Hemmat et al., 2020).
According to previous work (Fraser et al., 2020), the plasma of COVID 19 patients contains a lower amount of arginine and, as a consequence, its depletion could potentially delay and/or compromise intensive care unit recovery (Fraser et al., 2020). Concerning immune defenses, it should be noted that an increase in arginine concentration may play a role in the regulation of immune cell reactivity through the proliferation and differentiation of naive T cells to memory (Geiger et al., 2016). Arginine could preferentially enhance the proliferation of T lymphocyte subpopulations by increasing specific receptor expression and IL-2 production (Tate et al., 2008), and increasing T cell number/responses (Kim et al., 2018). Moreover, an arginine increase could also enhance affect lymphocyte proliferation and macrophage activities by providing a substrate for protein synthesis and/or precursors of polyamines or nitric oxide, being important in sustaining cellular proliferation and macrophage physiology (Geiger et al., 2016; Ji et al., 2019). Interestingly, patients with severe COVID-19 have an increased inflammatory response that depletes arginine, impairs T cell and endothelial cell function, and causes extensive pulmonary damage. Therefore, inhibition of arginase-1 and/or replenishment of arginine may be important in preventing/treating severe COVID-19 (Dean et al., 2021).
In conclusion, these preliminary results suggest that unstimulated PBMC analyzed up to 90 days after SARS-CoV-2 infection shows an increase in antiviral metabolites (sarcosine and S-adenosylhomocysteine), and a modulation of arginine metabolism, involved in innate and adaptive immunity. The analysis of these metabolites might represent a biomarker of effective and long-standing antiviral activation of PBMC. Although more studies are needed by increasing the number of samples and taking a longer time course, our work provides a basis for further analysis of protective immunity to SARS-CoV-2.
Materials and Methods
41 subjects (23 males and 18 females, Supplementary Table S1) undergoing COVID-19 serological analysis (Centro Polispecialistico Giovanni Paolo I, Viterbo, I) were enrolled in this study from October 2020 to March 2021. All participants provided informed written consent to participate in the research project, and the study was approved by the Regional Ethical Board in Ospedale L. Spallanzani, Roma, (number 169, approval 22/07/2020) and, following the Helsinki Declaration, written informed consent was obtained from all subjects. The comorbidities from donors have been excluded on the base of the declaration released by donors when enrolled in the protocol. No specific medical analyses have been done, with the exclusion of a Covid-19 screening from declared asymptomatic donors 3 days before bleeding.
Determination of in vitro IgG B cell memory for spike-S1 virus protein and of specific IgG was performed by Cell-ELISA in PBMC, from all donors, employing spike-S1 coated wells from a commercial source, experimental outputs were net absorbance values (A 450 nm) with background subtracted. A cutoff value for negative samples was arbitrarily established at an OD450 value of 0.07 as previously described without modifications (Zarletti et al., 2020). Parallel cultures of PBMC employed in Cell-ELISA were incubated without stimulants at 106/ml at 37°C for 48 h in 100 ul/well of RPMI medium containing 10% FCS and antibiotics, then centrifuged at 500 xg, and pelleted cells were immediately employed for metabolomic analysis. Metabolites were extracted and analyzed by LC-MS following the protocol published in (Ambrosini et al., 2020). Each sample was added to 1,000 μl of a chloroform/methanol/water (1:3:1 ratio) solvent mixture stored at −20°C. The tubes were mixed for 30 min and subsequently centrifuged at 1,000 × g for 1 min at 4°C, before being transferred to −20°C for 2–8 h. The solutions were then centrifuged for 15 min at 15,000×g and were dried to obtain visible pellets. Finally, the dried samples were re-suspended in 0.1 ml of water, 5% formic acid, and transferred to glass autosampler vials for LC/MS analysis. Twenty microliters of extracted supernatant samples were injected into an ultrahigh-performance liquid chromatography (UHPLC) system (Ultimate 3,000, Thermo) and run on a positive mode: samples were loaded onto a Reprosil C18 column (2.0 × 150 mm, 2.5 μm-DrMaisch, Germany) for metabolite separation. For positive ion mode (+) MS analyses, a 0–100% linear gradient of solvent A (ddH2O, 0.1% formic acid) to B (acetonitrile, 0.1%formic acid) was employed over 20 min, returning to 100% A in 2 min and holding solvent A for a 1-min post time hold. Acetonitrile, formic acid, and HPLC-grade water and standards (≥98% chemical purity) were purchased from Sigma Aldrich. Chromatographic separations were made at a column temperature of 30°C and a flow rate of 0.2 ml/min. The UHPLC system was coupled online with a Q Exactive mass spectrometer (Thermo) scanning in full MS mode (2 μ scans) at the resolution of 70,000 in the 67–1,000 m/z range, a target of 1,106 ions, and a maximum ion injection time (IT) of 35 ms with 3.8 kV spray voltage, 40 sheath gas, and 25 auxiliary gas. Calibration was performed before each analysis against positive or negative ion mode calibration mixes (Pierce, Thermo Fisher, Rockford, IL) to ensure the error of the intact mass within the sub-ppm range. 10 μl aliquots of all samples were pooled as a quality control (QC) sample which was run every 10 samples. Replicates were exported as. mzXML files and processed through MAVEN.5.2. Multivariate Partial Least-Squares Discriminant Analysis (PLS-DA) and Univariate (Volcano plot) statistical analyses were performed on the entire metabolomics data set using the MetaboAnalyst 5.0 software. Before the analysis, raw data were normalized by sum and autoscaling to increase the importance of low-abundance ions without significant amplification of noise. This type of plot displays the fold change differences and the statistical significance for each variable. The false discovery rate (FDR) was used for controlling multiple testing (p-value FDR cutoff 0.05).
Data Availability Statement
The original contributions presented in the study are included in the article/Supplementary Material, further inquiries can be directed to the corresponding authors.
Ethics Statement
The studies involving human participants were reviewed and approved by the (number 169, approval 22/07/2020. The patients/participants provided their written informed consent to participate in this study.
Author Contributions
Samples were collected by GZ, AT, and GS conceived and designed the experiment; GF and FG performed the experiments and contributed analysis tools; AT, GF, and FG wrote the paper with the input of GS, AT, GF, FG, GS, GZ, AT, and VD reviewed and edited the manuscript. All authors have read and agreed to the published version of the manuscript.
Funding
This work was supported by PRIN PROT 2017 XCXAFZ from University of Viterbo. and by Ministero dell’Istruzione, dell’Università e della Ricerca (MIUR), Rome, Italy.
Conflict of Interest
The authors declare that the research was conducted in the absence of any commercial or financial relationships that could be construed as a potential conflict of interest.
Publisher’s Note
All claims expressed in this article are solely those of the authors and do not necessarily represent those of their affiliated organizations, or those of the publisher, the editors and the reviewers. Any product that may be evaluated in this article, or claim that may be made by its manufacturer, is not guaranteed or endorsed by the publisher.
Supplementary Material
The Supplementary Material for this article can be found online at: https://www.frontiersin.org/articles/10.3389/fmolb.2022.894207/full#supplementary-material
Supplementary Table 1 | Cell-ELISA absorbance (A450 nm) net values, positive values are in bold with a cutoff value of 0.07. The Cell-ELISA protocol and cutoff value areas previously described (Zarletti et al., 2020). Materials were from a commercial kit (Dia. Pro Diagnostics Bioprobes SRL, Milano, Italy. Lot 0420/5AA, Ref: COV19G.CE.192). The negatives were asymptomatic donors screened for Covid-19 at 35 and 3 days before CellELISA.
References
Ambrosini, G., Pozza, E. D., Fanelli, G., Di Carlo, C., Vettori, A., Cannino, G., et al. (2020). Progressively De-differentiated Pancreatic Cancer Cells Shift from Glycolysis to Oxidative Metabolism and Gain a Quiescent Stem State. Cells 9 (7), 1572. doi:10.3390/cells9071572
Bray, M., Driscoll, J., and Huggins, J. W. (2000). Treatment of Lethal Ebola Virus Infection in Mice with a Single Dose of an S-Adenosyl-L-Homocysteine Hydrolase Inhibitor. Antivir. Res. 45 (2), 135–147. doi:10.1016/s0166-3542(00)00066-8
Byszewska, M., Śmietański, M., Purta, E., and Bujnicki, J. M. (2014). RNA Methyltransferases Involved in 5′ Cap Biosynthesis. RNA Biol. 11 (12), 1597–1607. doi:10.1080/15476286.2015.1004955
Czarnecka, A. M., Hilgier, W., and Zielińska, M. (2020). S-adenosylmethionine Deficiency and Brain Accumulation of S-Adenosylhomocysteine in Thioacetamide-Induced Acute Liver Failure. Nutrients 12 (7), 2135. doi:10.3390/nu12072135C
Dean, M. J., Ochoa, J. B., Sanchez-Pino, M. D., Zabaleta, J., Garai, J., Del Valle, L., et al. (2021). Severe COVID-19 Is Characterized by an Impaired Type I Interferon Response and Elevated Levels of Arginase Producing Granulocytic Myeloid Derived Suppressor Cells. Front. Immunol. 12, 695972. doi:10.3389/fimmu.2021.695972
Diray-Arce, J., Conti, M. G., Petrova, B., Kanarek, N., Angelidou, A., and Levy, O. (2020). Integrative Metabolomics to Identify Molecular Signatures of Responses to Vaccines and Infections. Metabolites 10 (12), 492. doi:10.3390/metabo10120492
Fraser, D. D., Slessarev, M., Martin, C. M., Daley, M., Patel, M. A., Miller, M. R., et al. (2020). Metabolomics Profiling of Critically Ill Coronavirus Disease 2019 Patients: Identification of Diagnostic and Prognostic Biomarkers. Crit. care Explor. 2 (10), e0272. doi:10.1097/CCE.0000000000000272
Geiger, R., Rieckmann, J. C., Wolf, T., Basso, C., Feng, Y., Fuhrer, T., et al. (2016). L-arginine Modulates T Cell Metabolism and Enhances Survival and Anti-tumor Activity. Cell 167 (3), 829–842. e13. doi:10.1016/j.cell.2016.09.031
Hemmat, N., Derakhshani, A., Baghi, H. B., Silvestris, N., Baradaran, B., and De Summa, S. (2020). Neutrophils, Crucial, or Harmful Immune Cells Involved in Coronavirus Infection: A Bioinformatics Study. Front. Genet. 11, 641. doi:10.3389/fgene.2020.00641
Hoffman, R. M., and Han, Q. (2020). Oral Methioninase for Covid-19 Methionine-Restriction Therapy. Vivo 34 (3 Suppl. l), 1593–1596. doi:10.21873/invivo.11948
Ji, L., Zhao, X., Zhang, B., Kang, L., Song, W., Zhao, B., et al. (2019). Slc6a8-Mediated Creatine Uptake and Accumulation Reprogram Macrophage Polarization via Regulating Cytokine Responses. Immunity 51 (2), 272–284. e7. doi:10.1016/j.immuni.2019.06.007
Kim, S.-H., Roszik, J., Grimm, E. A., and Ekmekcioglu, S. (2018). Impact of L-Arginine Metabolism on Immune Response and Anticancer Immunotherapy. Front. Oncol. 8, 67. doi:10.3389/fonc.2018.00067
Kryukov, E. V., Ivanov, A. V., Karpov, V. O., Aleksandrin, V. V., Dygai, A. M., Kruglova, M. P., et al. (2021). Plasma S-Adenosylmethionine Is Associated with Lung Injury in COVID-19. Dis. markers 2021, 1–10. doi:10.1155/2021/7686374
Ni, L., Ye, F., Cheng, M.-L., Feng, Y., Deng, Y.-Q., Zhao, H., et al. (2020). Detection of SARS-CoV-2-specific Humoral and Cellular Immunity in COVID-19 Convalescent Individuals. Immunity 52 (6), 971–977. e3. doi:10.1016/j.immuni.2020.04.023
Perła-Kaján, J., and Jakubowski, H. (2022). COVID-19 and One-Carbon Metabolism. Ijms 23 (8), 4181. 10 Apr. doi:10.3390/ijms23084181
Roberts, I., Muelas, M. W., Taylor, J. M., Davison, A. S., Xu, Y., Grixti, J. M., et al. (2021). Untargeted Metabolomics of COVID-19 Patient Serum Reveals Potential Prognostic Markers of Both Severity and Outcome. Metabolomics 18 (1), 6. doi:10.1007/s11306-021-01859-3
Shah, V. K., Firmal, P., Alam, A., Ganguly, D., and Chattopadhyay, S. (2020). Overview of Immune Response during SARS-CoV-2 Infection: Lessons from the Past. Front. Immunol. 11, 1949. doi:10.3389/fimmu.2020.01949
Shen, B., Yi, X., Sun, Y., Bi, X., Du, J., Zhang, C., et al. (2020). Proteomic and Metabolomic Characterization of COVID-19 Patient Sera. Cell 182 (1), 59–72. e15. doi:10.1016/j.cell.2020.05.032
Tate, D. J., Vonderhaar, D. J., Caldas, Y. A., Metoyer, T., Patterson, J. R., Aviles, D. H., et al. (2008). Effect of Arginase II on L-Arginine Depletion and Cell Growth in Murine Cell Lines of Renal Cell Carcinoma. J. Hematol. Oncol. 1, 14. doi:10.1186/1756-8722-1-14
Tatum, D., Taghavi, S., Houghton, A., Stover, J., Toraih, E., and Duchesne, J. (2020). Neutrophil-to-Lymphocyte Ratio and Outcomes in Louisiana COVID-19 Patients. Shock (Augusta, Ga.) 54 (5), 652–658. doi:10.1097/SHK.0000000000001585
Tehlivets, O., Malanovic, N., Visram, M., Pavkov-Keller, T., and Keller, W. (2013). S-adenosyl-L-homocysteine Hydrolase and Methylation Disorders: Yeast as a Model System. Biochimica Biophysica Acta (BBA) - Mol. Basis Dis. 1832 (1), 204–215. doi:10.1016/j.bbadis.2012.09.007
Turner, J. S., Kim, W., Kalaidina, E., Goss, C., Rauseo, A. M., Schmitz, A. J., et al. (2020). SARS-CoV-2 Infection Induces Long-Lived Bone Marrow Plasma Cells in Humans. Res. square. rs.3.rs-132821. doi:10.21203/rs.3.rs-132821/v1
Villalón, M. D., Gil-Fernández, C., and De Clercq, E. (1993). The Activity of Several S-Adenosylhomocysteine Hydrolase Inhibitors against African Swine Fever Virus Replication in Vero Cells. Antivir. Res. 20 (2), 131–144. doi:10.1016/0166-3542(93)90003-2
Wilamowski, M., Sherrell, D. A., Minasov, G., Kim, Y., Shuvalova, L., Lavens, A., et al. (2021). 2′-O Methylation of RNA Cap in SARS-CoV-2 Captured by Serial Crystallography. Proc. Natl. Acad. Sci. U.S.A. 118 (21), e2100170118. doi:10.1073/pnas.2100170118
Zarletti, G., Tiberi, M., De Molfetta, V., Bossù, M., Toppi, E., Bossù, P., et al. (2020). A Cell-Based ELISA to Improve the Serological Analysis of Anti-SARS-CoV-2 IgG. Viruses 12 (11), 1274. doi:10.3390/v12111274
Keywords: metabolomics, COVID-19, in vitro B-cell memory, cell-ELISA, mass spectrometry
Citation: Fanelli G, Gevi F, Zarletti G, Tiberi M, De Molfetta V, Scapigliati G and Timperio AM (2022) An Altered Metabolism in Leukocytes Showing in vitro igG Memory From SARS-CoV-2-Infected Patients. Front. Mol. Biosci. 9:894207. doi: 10.3389/fmolb.2022.894207
Received: 11 March 2022; Accepted: 12 May 2022;
Published: 30 June 2022.
Edited by:
Pasquale Pagliano, University of Salerno, ItalyReviewed by:
Miguel A. Ortega, University of Alcalá, SpainCarmen Gherasim, University of Michigan, United States
Copyright © 2022 Fanelli, Gevi, Zarletti, Tiberi, De Molfetta, Scapigliati and Timperio. This is an open-access article distributed under the terms of the Creative Commons Attribution License (CC BY). The use, distribution or reproduction in other forums is permitted, provided the original author(s) and the copyright owner(s) are credited and that the original publication in this journal is cited, in accordance with accepted academic practice. No use, distribution or reproduction is permitted which does not comply with these terms.
*Correspondence: G. Scapigliati, scapigliati@unitus.it; A. M. Timperio, timperio@unitus.it
†These authors have contributed equally to this work