- 1i3S—Instituto de Investigação e Inovação em Saúde, Universidade do Porto, Porto, Portugal
- 2Institute of Molecular Pathology and Immunology of the University of Porto (IPATIMUP), Porto, Portugal
- 3Doctoral Program on Cellular and Molecular Biotechnology Applied to Health Sciences, School of Medicine and Biomedical Sciences Abel Salazar (ICBAS), University of Porto, Porto, Portugal
- 4Faculty of Medicine, University of Porto, Porto, Portugal
- 5Department of General Surgery, Centro Hospitalar Universitário de São João, Porto, Portugal
- 6Institute of Biomedical Engineering (INEB), University of Porto, Porto, Portugal
- 7School of Medicine and Biomedical Sciences Abel Salazar (ICBAS), University of Porto, Porto, Portugal
- 8Department of Pathology, Centro Hospitalar Universitário de São João, Porto, Portugal
The extracellular matrix (ECM) plays an undisputable role in tissue homeostasis and its deregulation leads to altered mechanical and biochemical cues that impact cancer development and progression. Herein, we undertook a novel approach to address the role of gastric ECM in tumorigenesis, which remained largely unexplored. By combining decellularization techniques with a high-throughput quantitative proteomics approach, we have performed an extensive characterization of human gastric mucosa, uncovering its composition and distribution among tumor, normal adjacent and normal distant mucosa. Our results revealed a common ECM signature composed of 142 proteins and indicated that gastric carcinogenesis encompasses ECM remodeling through alterations in the abundance of 24 components, mainly basement membrane proteins. Indeed, we could only identify one de novo tumor-specific protein, the collagen alpha-1(X) chain (COL10A1). Functional analysis of the data demonstrated that gastric ECM remodeling favors tumor progression by activating ECM receptors and cellular processes involved in angiogenesis and cell-extrinsic metabolic regulation. By analyzing mRNA expression in an independent GC cohort available at the TGCA, we validated the expression profile of 12 differentially expressed ECM proteins. Importantly, the expression of COL1A2, LOX and LTBP2 significantly correlated with high tumor stage, with LOX and LTBP2 further impacting patient overall survival. These findings contribute for a better understanding of GC biology and highlight the role of core ECM components in gastric carcinogenesis and their clinical relevance as biomarkers of disease prognosis.
Introduction
Gastric cancer (GC) remains a major clinical burden as one of the most reported malignancies globally, despite a steady decrease in incidence in most developed countries (Arnold et al., 2020). Strikingly, GC ranks fourth on the list of cancer-related deaths (Sung et al., 2021), reflective of its indolent nature and scarce therapeutic strategies. Indeed, surgical resection is still the cornerstone of GC treatment, however with limited effectiveness since the majority of patients are diagnosed at advanced stages of disease (Takahashi et al., 2013). Improved detection of GC, particularly at an early stage, would no doubt be crucial to ameliorate both treatment strategies and prediction of patient prognosis. In this sense, the identification of GC biomarkers has been the subject of intense investigation.
The extracellular matrix (ECM) is a key component of the gastric tumor microenvironment long overlooked as a critical source of relevant molecules for carcinogenesis (Quail and Joyce, 2013). In fact, due to its influence in cellular behavior and fate, the ECM is now regarded as a key regulator of tumorigenesis (Bonnans et al., 2014; Moreira et al., 2020), and excessive ECM deposition is considered one of the hallmarks of cancer associated with poor patient prognosis (Erler et al., 2006; Levental et al., 2009). Accordingly, the ECM provides cancer cells with sustained proliferative signals, such as growth factors and chemokines, and shields them from growth suppressors, acting as a diffusion barrier for anti-cancer conventional drugs (Henke et al., 2020). Moreover, these biochemical and mechanical changes are paramount for cancer progression since they translate into disturbed cell-cell and cell-ECM adhesion, as well as up-regulation of ECM receptors, impacting downstream signaling pathways that promote resistance to cell death, angiogenesis, cancer cell invasion and metastasis (Pickup et al., 2014; Mohan et al., 2020; Figueiredo et al., 2021).
Although known to be involved in many oncogenic processes (Pickup et al., 2014), the ECM proteome and its regulators, or “matrisome”, remained largely unexplored due to technical constraints associated to ECM protein properties, namely its high insolubility. Notably, this past decade has witnessed extensive development of tools that allow high-throughput quantitative proteomic analysis of ECM-enriched samples (Naba et al., 2012; Naba et al., 2015). This has led to the identification of matrisome protein signatures that distinguish normal tissues and primary tumors in several cancer types, including colorectal cancer (Naba et al., 2014b), triple-negative breast cancer (Naba et al., 2017) and multiple myeloma (Glavey et al., 2017). However, and to the best of our knowledge, this is the first study focusing on the gastric matrisome and on the identification of a gastric tumor ECM signature.
Herein, we undertook a comprehensive characterization of gastric ECM components following a decellularization procedure that yielded ECM enriched samples of both normal and tumor tissues. Using high-throughput proteomics, we defined the gastric matrisome and pinpointed a set of differentially expressed proteins in the tumor ECM that impact patient prognosis.
These findings contribute for the identification of molecules that play functional roles in GC development and thus can be targeted or serve as diagnostic and prognostic biomarkers.
Materials and Methods
Patient Samples
Human samples were obtained from the Department of Pathology from Centro Hospitalar Universitário de São João (CHUSJ, Porto, Portugal), upon patients’ informed consent. The study was approved by CHUSJ Ethics Committee for Health (Reference 32/18), in agreement with the Helsinki declaration. Gastric antrum adenocarcinoma samples, normal distant and adjacent mucosa from nine patients were collected within 1 h of surgery. The distance between adjacent normal and cancer tissue boundary was about 1 cm, while that between distant normal tissue and cancer tissue was approximately 5 cm. Samples were rinsed in ice cold PBS without calcium and magnesium, and processed into smaller fragments. When not used immediately, samples were immersed in mounting medium for cryotomy (OCT compound, Thermo Fisher Scientific, Germany), frozen in 2-methylbutane cooled with liquid nitrogen, and stored at −80°C. Patient clinicopathological data is summarized in Supplementary Table S1.
Decellularization and ECM-Enrichment
Normal and tumor samples were cut into 4 × 4 mm fragments, weighed, placed in a 24-well plate, and decellularized as previously described (Pinto et al., 2017). Briefly, samples were incubated in a hypotonic buffer (10 mM Tris-HCl, 0.1% EDTA, pH 7.8) for 18 h, washed with PBS, and decellularized for 24 h in 0.1% SDS. Fragments were washed (10 mM Tris-HCl, pH 7.8) and incubated for 3 hours at 37°C with 50 U/ml DNase (Gibco, Thermo Fisher Scientific, Germany) in reaction buffer (10 mM Tris-HCl, 2.5 mM MgCl2, 100 nM CaCl2, pH 7.8). All steps were performed under constant agitation (165 rpm) in the presence of 10 mg/ml of Gentamicin (Gibco, Thermo Fisher Scientific, Germany). DNA content of native and decellularized tissues was quantified on a Qubit Fluorometer (Thermo Fisher Scientific, Germany) using the Qubit dsDNA High Sensitivity kit (Thermo Fisher Scientific, Germany), according to the manufacturer’s instructions. For nuclei staining with DAPI, one fragment from each sample was formalin-fixed and paraffin-embedded. To confirm decellularization efficiency, 3 μm-thick sections from FFPE samples were processed and stained with Hematoxylin and Eosin (H&E), as well as through Masson’s Trichrome (MT) protocol. Images were obtained on a Light microscope Olympus DP 25 (Olympus, United States).
Sample Preparation and Liquid Chromatography-Tandem Mass Spectrometry
Normal and tumor decellularized fragments (ca. 30 mg/sample) were prepared for mass spectrometry analysis following an adapted protocol (Naba et al., 2015). Samples were homogenized on ice with a tissue ruptor in an urea solution with reducing agent dithiothreitol (DTT), alkylated with iodoacetamide, and subsequently digested with PNGaseF and trypsin/Lys-C.
Protein identification and quantitation was performed by nanoscale liquid chromatography with tandem mass spectrometry (nano LC-MS/MS) using an Ultimate 3,000 liquid chromatography system coupled to a Q-Exactive Hybrid Quadrupole-Orbitrap mass spectrometer (Thermo Fisher Scientific, Germany), as previously described (Osório et al., 2021). Data was acquired using Xcalibur 4.0 and Tune 2.09 software (Thermo Fisher Scientific, Germany).
Data Analysis
Raw data was processed using Proteome Discoverer 2.4.0.305 software (Thermo Fisher Scientific, Germany) and submitted as query in the UniProt database for the reviewed Homo sapiens Proteome 2020_02 with 20,350 entries and the NIST human spectral library. A common protein contaminant list from MaxQuant was also considered. The MSPepSearch and Sequest HT search engines were used to identify tryptic peptides. The ion mass tolerance was 10 ppm for precursor ions and 0.02 Da for fragment ions in both software. Maximum number of missed cleavage sites allowed was set to two. Peptide confidence was set to high. The processing node Percolator was enabled with the following settings: maximum delta Cn 0.05, decoy database search target FDR 1%, and validation based on q value. Protein label free quantitation was performed with the Minora feature detector node at the processing step. Precursor ions quantification was performed considering unique plus razor peptides with the following parameters: precursor abundance based on intensity, and normalization based on total peptide amount. Concerning post-translational modifications, we have considered static modifications, such as Carbamidomethylation (cysteine), and dynamic modifications, including Oxidation (methionine) and N-terminal modifications (Acetyl, Met-loss and Met-loss + Acetyl). For protein identification, at least two unique peptides and an FDR level of confidence of “high” (p ≤ 0.01) were required. Single unique peptide identifications were included only when its amino acid sequence was exclusively related to the identified protein [sequences were queried using the UniProt Blast tool (Pundir et al., 2016)]. Matrisome annotations were identified using MatrisomeDB [http://matrisomedb.pepchem.org/; (Shao et al., 2019)]. Pairwise correlations of protein abundance for the same tissue across different patients were quantified using the Pearson’s correlation coefficient. The Overlap Coefficient was used to calculate the similarity between qualitative profiles obtained for each of the datasets. To define the matrisome composition of each tissue, we have only considered core ECM proteins and ECM-associated proteins that were present in at least a third of patient samples.
Identification of Differentially Expressed Proteins
For the identification of differentially expressed matrisome proteins among tumor and normal paired samples, we first performed a multiple two-tailed Student’s t test corrected for multiple comparisons using the Benjamini-Hochberg method. Next, we have calculated fold change (FC) values for each protein (protein expression in tumor samples normalized against protein expression in normal distant or normal adjacent tissues). For further analysis, we have selected proteins with a q value <0.05 and a FC ≥ 1.5 (log2 FC ≥ 0.585).
Functional and Pathway Enrichment Analysis
STRING v11.0 (Search Tool for the Retrieval of Interacting Genes/Proteins database) online database [https://string-db.org/; (Szklarczyk et al., 2019)] was used to identify functional systems or pathways overrepresented in our sets, when compared with the software’s default background (Whole genome, Homo sapiens). Three classification networks were considered, including gene ontology domains [“Biological Process”, “Molecular Function” and “Cellular Component” (Ashburner et al., 2000; Gene Ontology Consortium., 2021)], InterPro Protein Domains and Features (Mitchell et al., 2019; Blum et al., 2020), and KEGG pathways [Kyoto Encyclopedia of Genes and Genomes; (Kanehisa et al., 2016)]. Significance threshold was set to FDR adjusted p value < 0.01. Enrichment strength for each category, which refers to log10 values of the ratio between observed proteins from our network and the number of proteins expected to be annotated with the term in a random network of the same size, was calculated using the STRING software.
Analysis of mRNA-Seq Data From the TCGA
The mRNA-Seq data of 27 tumor and matched normal pairs from The Cancer Genome Atlas (TCGA) stomach adenocarcinoma cohort (STAD) were plotted and compared using the TNMplot platform [https://tnmplot.com/analysis/; (Bartha and Győrffy, 2021)]. Comparisons were performed using the Wilcoxon test with a statistical significance cutoff set at p < 0.05. Candidates presenting tumor/normal expression ratios similar to those identified in our proteomic data were selected, and their association with clinicopathological features and overall survival of GC patients was further analysed.
For this purpose, RNA-seq data and clinical metadata of 383 STAD cases were retrieved from the cBioportal platform [https://www.cbioportal.org/study/summary?id=stad_tcga_pan_can_atlas_2018; (Cerami et al., 2012; Gao et al., 2013)] and from the supplemental data of TCGA STAD Pan (Cancer Genome Atlas Research Network., 2014; Liu et al., 2018), respectively.
To evaluate the association of ECM genes with tumor progression, patients were divided into four groups according to the Tumor Node Metastasis (TNM) grading system (I, n = 51; II, n = 115; III, n = 162; and IV, n = 39). Association with tumor histological type was studied for the 238 patients with available data. Using the Laurén classification system, patients were grouped into two main categories: diffuse type (n = 77) and intestinal type (n = 161) GC. Gene expression was compared using One-way ANOVA followed by post-hoc Tukey’s Honest Significant Difference test to perform multiple comparisons between groups of samples, or unpaired t test. Significant differences were considered when p < 0.05.
Using Kaplan-Meier survival analysis, GC patients were divided into two groups (high and low), according to median expression of ECM genes. Overall survival of the two groups was compared with the log rank test (Mantel-Cox) and a p < 0.05 was considered statistically significant.
Statistical Analysis
To determine significant differences among independent groups, we have used parametric tests, such as Student’s t test or One-way ANOVA, followed by multiple comparison with post hoc correction (when applicable). A p value below 0.05 was considered statistically significant. To evaluate normality of the distribution, we applied the Kolmogorov-Smirnov test. Equality of group variances was verified using the Brown-Forsythe test. All analysis were carried out using GraphPad Prism (version 6.05) or IBM SPSS Statistics version 26.
Results
In this study, we aimed to uncover the role of ECM in gastric carcinogenesis and its potential as a biomarker for cancer monitoring and surveillance. For that purpose, we have characterized the gastric matrisome evaluating both distant and adjacent mucosa, as well as tumor samples from gastric cancer patients.
Tumor and Normal Gastric ECM Share Core Matrisome Components
To characterize the ECM composition of gastric mucosa and potential alterations that may be associated with carcinogenesis, we performed quantitative proteomic analysis of normal distant (ND), normal adjacent (NA) and tumor (T) decellularized ECM samples from nine GC patients (Supplementary Table S1).
To obtain an ECM-enriched preparation, we undertook a three-step decellularization protocol using a hypotonic buffer, an anionic surfactant and DNAse treatment. As depicted in Figure 1A, a change in the color of gastric tissues was indicative of removal of cellular components. Decellularization efficiency was evaluated through DNA quantification and DAPI staining. Specifically, we observed nuclei loss in decellularized tissues and total DNA decreased by over 99% in normal distant (855.6 ng/mg in native vs. 5.8 ng/mg in decellularized tissue), normal adjacent (760.9 ng/mg in native vs. 6.5 ng/mg in decellularized tissue) and tumor tissues (695.5 ng/mg in native vs. 6.8 ng/mg in decellularized tissue) (Figures 1B,C). Further, we performed Hematoxylin and Eosin (H&E), as well as Masson’s Trichrome (MT) staining, confirming loss of cellular components and maintenance of ECM structure (Supplementary Figure S1).
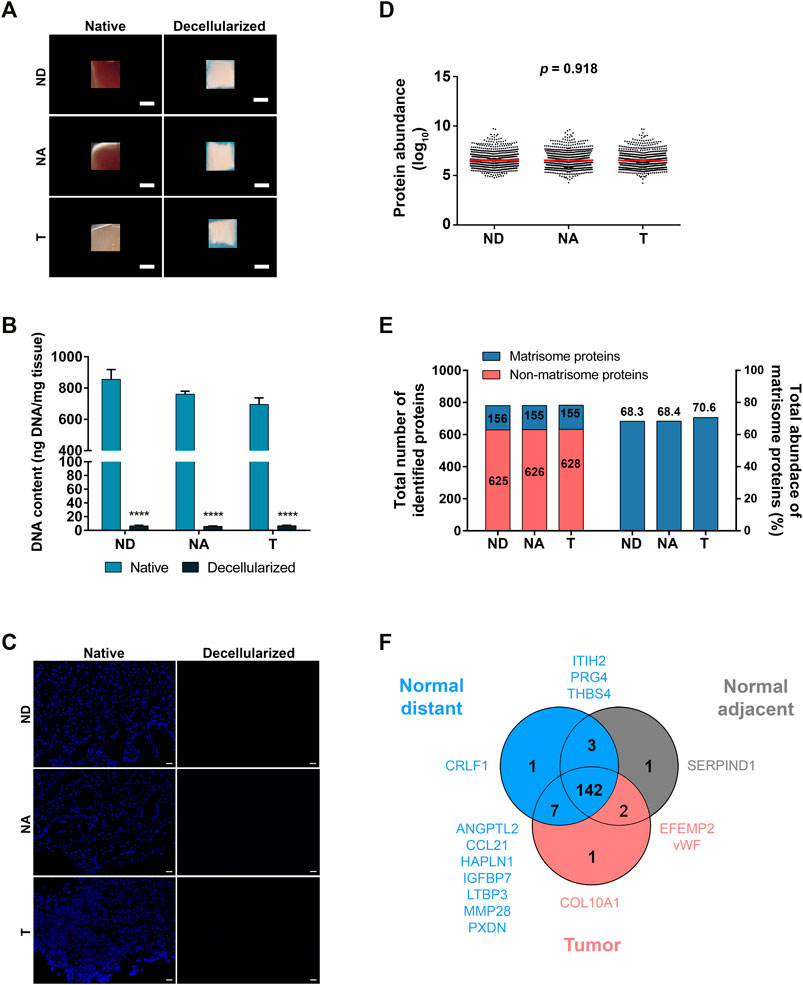
FIGURE 1. Characterization and proteomic analysis of decellularized gastric ECM. (A) Representative macroscopic images of normal distant (ND), normal adjacent (NA) and tumor (T) samples from human gastric mucosa before and after decellularization; scale bar = 1 mm. (B) DNA quantification of native and decellularized ECM samples (n = 3). Data are shown as mean ± SD, ****p < 0.0001. (C) Representative images of DAPI staining of native and decellularized samples; scale bar = 20 µm. (D) Log10 of normalized abundance distribution for all identified proteins in decellularized samples. (E) Total number of matrisome and non-matrisome proteins identified in decellularized ECM, with the corresponding abundance (%) of each set of matrisome proteins. (F) Venn diagram with the number of matrisome proteins identified in each tissue in at least 1/3 of the samples (n = 9). We have identified a gastric matrisome signature composed of 153 proteins (in blue) and a tumor-specific ECM signature with three proteins (in red). Proteins present in only one or two ECM sets are specified.
Subsequent analysis by LC-MS/MS revealed that there was no sample preparation bias given the similar normalized abundance distribution among tissue types (Figure 1D). We have identified a total of 781 proteins both in ND and in NA mucosa, and 783 proteins in T samples (Supplementary Material S1). Bioinformatic analysis and filtering through MatrisomeDB, a comprehensive database platform for ECM-derived protein identification, indicated that only 20% of these proteins were core matrisome (ECM glycoproteins, proteoglycans and collagens) or ECM-associated proteins (secreted factors, ECM regulators and ECM-affiliated proteins). Notwithstanding, they represent 68–70% of total protein abundance (Figure 1E). Analysis of matrisome proteins in biological replicates across the three tissues revealed they are highly correlated, with average Pearson correlation coefficients for protein abundance of 0.78, 0.83 and 0.88 for ND, NA and T samples, respectively, and an overlap coefficient of 0.94, which suggests both technical reproducibility and low inter-patient variability (Supplementary Figure S2; Supplementary Material S2).
To define each tissue’s matrisome, we considered only core matrisome and ECM-associated proteins that were present in at least a third of patient samples. Accordingly, the matrisome of normal distant mucosa was found to be composed of 153 proteins and that of normal adjacent tissue of 148 proteins, whereas the tumor matrisome comprised 152 proteins. When comparing matrisome proteins of the three tissues (Figure 1F and Supplementary Figure S3), we have identified a set of 142 common and 11 more proteins that were present in the normal distant mucosa alone or in combination with one of the other tissues. The glycoproteins EGF-containing fibulin-like extracellular matrix protein 2 (EFEMP2) and von Willebrand factor (vWF) were present in tumor and normal adjacent tissue but not in the normal distant mucosa matrisome and, surprisingly, there was only one tumor-specific protein, collagen alpha-1(X) chain (COL10A1).
Significant post-translational modifications were not identified, with the exception of Met-loss + Acetyl of LGALS4, which was enriched in normal distant when compared with normal adjacent mucosa.
Overall, these results revealed a similar protein composition between normal and tumor ECM, suggesting that ECM remodeling is mostly linked to alterations in the levels of its components, rather than to qualitative changes of the components themselves.
Tumor ECM Presents a Distinct Protein Expression Signature
Given that most ECM proteins were ubiquitously expressed in normal and tumor tissues, we next aimed to disclose molecular alterations at the protein expression level associated with GC. For that purpose, we focused on the established matrisome lists and determined differentially expressed proteins among the three tissues (Table 1 and Figure 2A).
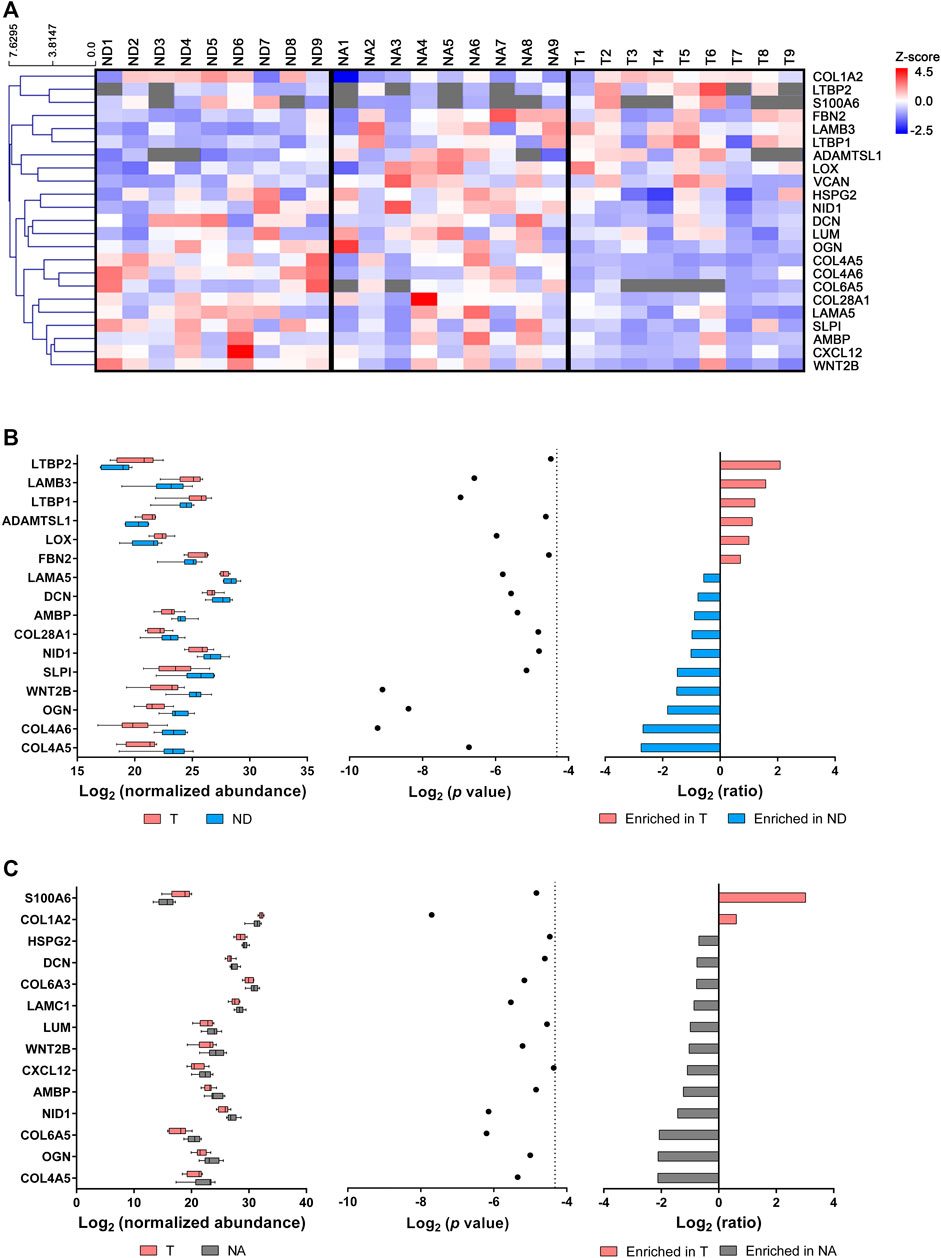
FIGURE 2. Differentially expressed proteins in gastric ECM. (A) Heatmap representation of hierarchical clustering (average linkage and Euclidean metric) of protein expression profiles indicated as log2 (normalized abundance). Grey rectangles represent expression below detection limit. Tumor (T) ECM (n = 9) yielded six upregulated and 10 downregulated proteins in comparison with normal distant (ND) mucosa (B), while two were upregulated and 12 were downregulated when compared with normal adjacent (NA) mucosa (C). Normalized abundance values were compared using a two-tailed, unpaired Student’s t-test corrected for multiple comparisons with the Benjamini-Hochberg method. Dotted line represents statistical significance threshold (p < 0.05).
When comparing tumor and normal distant mucosa, we identified 16 differentially expressed proteins, six of which were upregulated and 10 were downregulated in tumors (Figure 2B). Specifically, the upregulated components in tumor tissue were: glycoproteins latent-transforming growth factor beta-binding protein 1 (LTBP1) and latent-transforming growth factor beta-binding protein 2 (LTBP2), fibrillin-2 (FBN2), laminin subunit beta-3 (LAMB3), as well as ECM regulators lysyl oxidase (LOX) and ADAMTS-like protein 1 (ADAMTSL1). Regarding downregulated tumor ECM constituents, we identified the basement membrane components collagen alpha-5(IV) chain (COL4A5), collagen alpha-6(IV) chain (COL4A6), collagen alpha-1 (XXVIII) chain (COL28A1), laminin subunit alpha-5 (LAMA5), nidogen 1 (NID1), proteoglycans osteoglycin (OGN) and decorin (DCN), ECM regulators alpha-1-microgobulin/bikunin precursor (AMBP) and secretory leucocyte protease inhibitor (SLPI), along with secreted factor protein Wnt-2b (WNT2B).
The comparison between tumor tissue and normal adjacent mucosa yielded two upregulated and twelve downregulated proteins in tumors (Figure 2C). Collagen alpha-2 (I) chain (COL1A2) and secreted factor S100 calcium binding protein A6 (S100A6) were found upregulated in GC, whereas downregulated proteins included collagen alpha-5 (VI) chain (COL6A5) and collagen alpha-3 (VI) chain (COL6A3), heparan sulfate proteoglycan 2 (HSPG2), lumican (LUM), laminin subunit gamma-1 (LAMC1) and secreted factor stromal cell-derived factor 1 (CXCL12), along with COL4A5, WNT2B, NID1, OGN, AMBP and DCN, which had been already identified when comparing tumor with normal distant mucosa.
Interestingly, when comparing normal adjacent and distant tissues, we uncovered a set of differentially expressed proteins that may represent a pro-oncogenic signature. In fact, we were able to detect five upregulated proteins in the normal mucosa adjacent to the tumor, namely laminin subunit alpha-4 (LAMA4), elastin microfibril interfacer 1 (EMILIN1), LTBP1 and fibrinogen beta chain (FGB), as well as proteoglycan versican core protein (VCAN). In contrast, COL4A6 was the only protein found downregulated in this context (Supplementary Figure S4).
Taken together, these data suggest that there is a remodeling of the ECM during GC development, encompassing changes in the abundance of matrix components, particularly of basement membrane proteins, which can impact tumor cell behavior.
Functional Analysis Identifies Main Biological Traits Associated With Structural Remodeling of the ECM
In order to uncover the biological significance of the set of differentially expressed ECM proteins in the tumor tissues, when compared with normal distant and adjacent mucosa, these were submitted to functional and pathway enrichment analysis (Figure 3 and Supplementary Table S2).
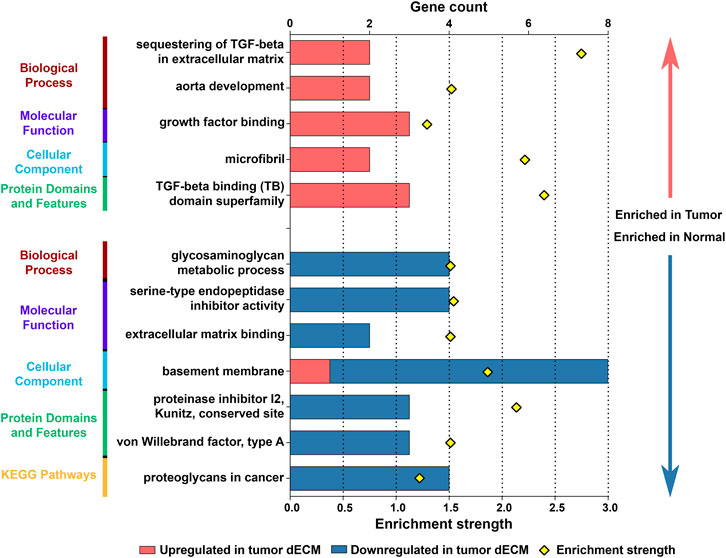
FIGURE 3. Functional and pathway enrichment analysis of differentially expressed proteins in tumor ECM. For GO term analysis, proteins were assigned to three main categories: Biological Process, Molecular Function and Cellular Component. The main Protein Domains and Features and KEGG Pathways were also identified. Graph depicts the most relevant results for each category enriched in tumor and normal ECM (additional data in Supplementary Table S2). Bars represent gene count and dots depict enrichment strength.
Through functional analysis, we found that biological processes related with sequestering of TGF-beta in the ECM (LTBP1 and FBN2; p = 1.10e-03) and aorta development (LOX and LTBP1; p = 2.99e-02), growth factor binding functions (LTBP1, LTBP2 and COL1A2; p = 4.40e-03), and microfibril components (LTBP1 and FBN2; p = 3.40e-04) were particularly enriched in upregulated proteins. Likewise, TGF-beta binding (TB) domain superfamily (LTBP1, LTBP2, FBN2; p = 4.76e-06) was over-represented in upregulated tumor ECM proteins. On the contrary, glycosaminoglycan metabolic processes, such as catabolism and biosynthesis (DCN, OGN, LUM and HSPG2; p ≤ 0.02), and molecular functions related to inhibition of enzymatic activity, such as serine-type endopeptidase inhibitor activity (AMBP, COL6A3, COL28A1, SLPI p = 2.2e-04), and extracellular matrix binding (DCN, NID1; p = 0.0151) were only correlated with downregulated proteins. Further, there was an over-representation of basement membrane components (e.g., NID1, LAMB3, HSPG2, COL4A5 and COL4A6-00; p = 3.64e-12), as well as proteinase inhibitors with Kunitz (AMBP, COL6A3, COL28A1; p = 1.56e-05) and von Willebrand Factor type A (COL6A3, COL6A5, COL28A1; p = 4.90e-04) domains in the downregulated class. Significantly enriched KEGG pathways, such as proteoglycans in cancer (DCN, LUM, WNT2B, HSPG2; p = 3.60e 04), were also mainly associated with downregulated proteins.
Collectively, the data suggest that gastric ECM remodeling favors tumor progression by activating ECM receptors and cellular processes that are involved in angiogenesis and cell-extrinsic metabolic regulation.
ECM Alterations Associate With Gastric Cancer Progression and Overall Survival
To validate the ECM changes identified in our proteomic survey, we used RNA-seq data from normal and paired tumor gastric carcinoma samples available at the TCGA-STAD cohort.
We verified that out of the 24 differentially expressed components in tumor ECM (Figures 2B,C), 12 presented a transcriptomic profile in line with our proteomic results. In particular, we noticed that COL1A2, LAMB3, LOX, LTBP2 and S100A6 were significantly upregulated in tumor specimens, when compared with normal tissue (Figure 4A). The opposite pattern was detected for COL4A5, COL4A6, COL6A5, COL28A1, CXCL12, DCN and OGN, whose expression was found decreased in GC samples (Figure 4B).
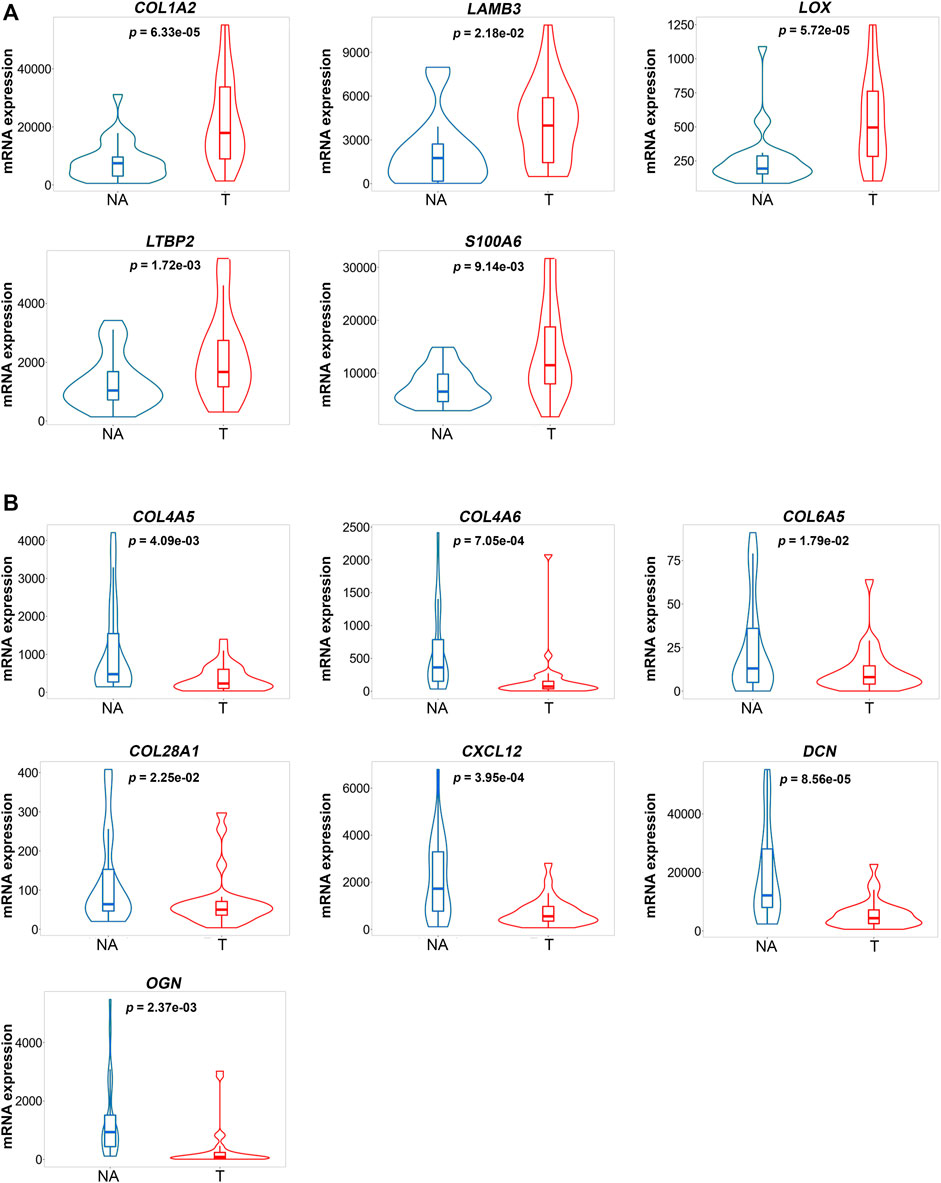
FIGURE 4. Gene expression violin plots of matrisome components differentially expressed in tumor ECM. Gastric cancer RNA-Seq expression from TCGA-STAD database comparing paired tumor (T) and normal adjacent (NA) tissue (n = 27). ECM genes significantly upregulated (A) or downregulated (B) in tumor samples. Plots were generated in the TNMplot platform [https://tnmplot.com/analysis/; (Bartha and Győrffy, 2021)]. Gene expression levels were normalized through DESeq2 (median-of-ratios method). Groups were compared using the Wilcoxon test and a p value below 0.05 was considered statistically significant.
We then evaluated the relationship of the validated candidates with available clinicopathological features (Supplementary Table S3). Remarkably, we observed that increased mRNA levels of COL1A2, LOX and LTBP2 were significantly associated with diffuse-type gastric carcinomas and with higher TNM stage (Figures 5A,B).
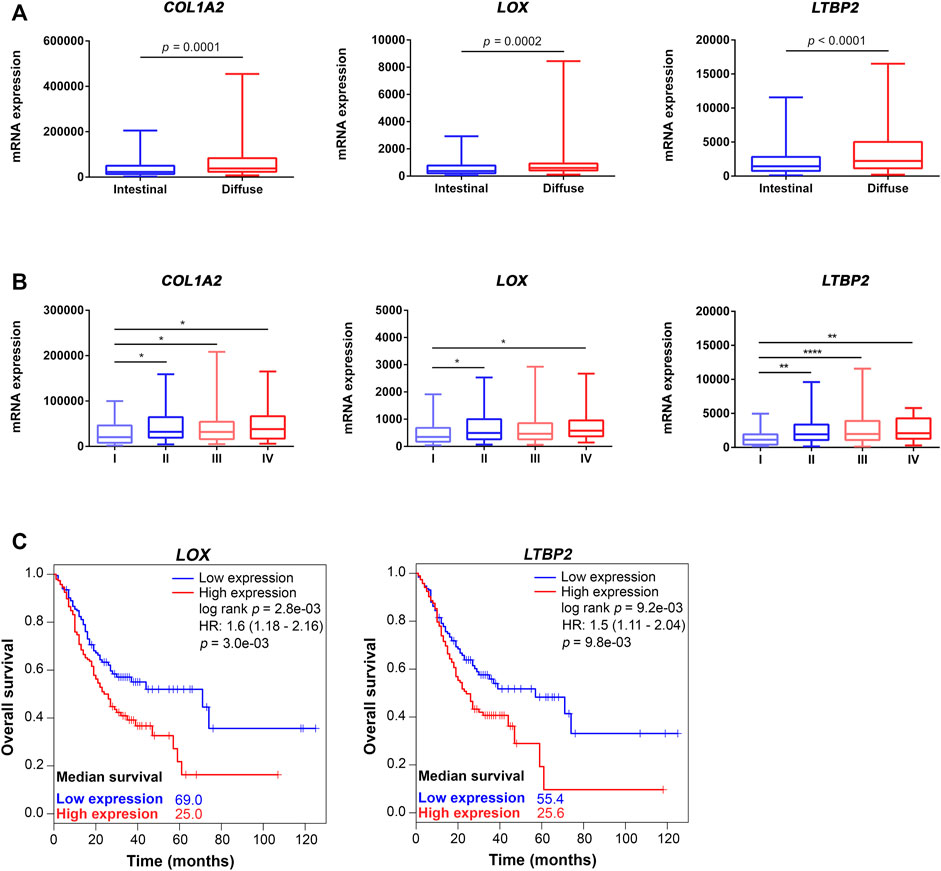
FIGURE 5. Association of differentially expressed ECM components with GC pathological features and overall survival. Increased expression of COL1A2, LOX and LTBP2 is significantly associated with diffuse type GC (A) and with high TNM stage (B). Gene expression levels were normalized through DESeq2 (median-of-ratios method). Expression values were compared using student’s t test or one-way ANOVA followed by Tukey’s HSD test. *p < 0.05, **p < 0.01 and ****p < 0.0001. (C) Gastric cancer patients with increased tumor expression of LOX and LTBP2 have worse overall survival when compared with those exhibiting low expression. Survival between groups was compared using the Mantel-Cox test and log rank p value below 0.05 was considered statistically significant. Median survival refers to overall survival probability S(t) = 0.5. Vertical lines on Kaplan-Meier curves represent censored subjects.
To uncover the impact of candidate genes in patient overall survival, cases were divided into High and Low expression groups according to median expression of each of the 12 genes individually. Using Kaplan-Meier curves, we observed that increased expression of LOX and LTBP2 was significantly associated with worst overall survival (log rank p < 0.05) (Figure 5C).
Consistent with what we observed for LOX and LTBP2, we found that COL10A1, which was the only tumor-specific protein, is strongly increased in tumor tissue (p = 5.93e-06), when compared with paired normal adjacent mucosa. Moreover, we observed an association between increased expression of COL10A1 and diffuse type gastric cancer, as well as with high TNM stage. Patients with tumors expressing high levels of COL10A1 also displayed lower overall survival, when compared with those harbouring tumors with low COL10A1 expression (Supplementary Figure S5).
These data highlight the role of core ECM components in gastric carcinogenesis and their clinical relevance as biomarkers of disease prognosis.
Discussion
Gastric cancer remains a worldwide clinical burden, with scarce therapeutic options for patients who are often diagnosed at advanced stages of disease (Takahashi et al., 2013). The ECM is acknowledged to impact several cancer hallmarks and, in fact, matrisome changes have been described for a number of solid cancers (Naba et al., 2014a; Naba et al., 2014b; Acerbi et al., 2015; Laklai et al., 2016). To date, however, no proteomics-based matrisome signatures have been described for GC.
Research on the roles of ECM in cancer has traditionally focused on single or multiple pre-established combinations of ECM components. In the last decade, advances in decellularization strategies have attempted to overcome this limitation and obtain reconstituted ECM in vitro (Hoshiba, 2019). Herein, we present a comprehensive analysis of the gastric matrisome and the identification of key constituents using tissue-derived decellularized ECM, which could represent useful biomarkers for GC management. To the best of our knowledge, this is the first study that characterizes molecular alterations of gastric tumor ECM, considering both adjacent tissue and distant mucosa. Indeed, most studies addressing tumor/matched normal pairs use normal tissue surrounding the lesion. Although histologically normal, evidence indicate that these adjacent tissues display alterations in gene expression related to fibrosis, wound healing, epithelial-to-mesenchymal transition, and ECM remodeling (Troester et al., 2009; Trujillo et al., 2011; Casbas-Hernandez et al., 2015). Therefore, to obtain a thorough characterization of gastric matrisome alterations that may impact tumor development, we have used paired distant mucosa as our main control.
Through a decellularization approach that combined the use of a hypotonic buffer, an anionic surfactant, and DNase, we have obtained ECM-enriched samples of gastric adenocarcinomas as well as paired normal adjacent and normal distant mucosa, which were then processed for high resolution mass spectrometry. For the definition of each tissue matrisome, we have considered only proteins present in at least 1/3 of patient samples. This resulted in the identification of a total of 157 ECM proteins, 93 of which were core matrisome proteins and 64 ECM-associated proteins. To define the gastric matrisome, we identified a common ECM signature among the three tissues composed of 142 proteins. These, along with 11 more proteins that were detected in the normal distant mucosa (alone, or in combination with one of the other tissues) constitute the gastric matrisome.
Interestingly, our proteomic analysis has uncovered three proteins that were present either in tumor ECM alone (COL10A1) or in tumor and adjacent mucosa (EFEMP2 and vWF) and were absent in most distant mucosa samples. Corroborating our results, Necula and colleagues reported increased levels of COL10A1 in the plasma of GC patients, which were associated with poor overall survival (Necula et al., 2020). With respect to EFEMP2, our results are consistent with previous reports of EFEMP2 mRNA overexpression associated with pathohistological alterations and overall survival in GC (Chen et al., 2018). Regarding vWF expression in GC, it was found upregulated both at the mRNA and protein levels, and increased vWF antigen levels correlated with disease severity (Yang et al., 2015). We propose that these three proteins hold great potential as clinical biomarkers for early detection of GC and could be more useful than currently used markers, such as CEA and pepsinogen, which lack sensitivity and specificity (Battaglin et al., 2018; Necula et al., 2019).
Given that most ECM proteins were ubiquitously expressed in normal and tumor tissues, we postulated that the major molecular alterations occurring in gastric mucosa during transformation could be related to differential expression of its components. To disclose these modifications, we have compared the matrisome of gastric adenocarcinoma to those of normal distant and adjacent mucosa, identifying 24 differentially expressed ECM proteins, of which eight were upregulated and 16 were downregulated in tumor tissues. Overexpressed in tumor matrix were proteins involved in ECM organization such as LOX, LAMB3, ADAMTSL1 and FBN2. Indeed, LOX has been reported to play a major role in ECM remodeling by establishing covalent crosslinks between collagen and elastic fibers that lead to ECM fibrosis and set the grounds for adherence and colonization of cancer cells (Zhao et al., 2019). LAMB3 has been shown to activate the PI3K/Akt signaling pathway, promoting cancer cell proliferation, migration and invasion in vitro (Zhang et al., 2019). ADAMTS-like proteins are a subgroup of the ADAMTS family that lack the catalytic domain (Porter et al., 2005) and have been described to play a role in microfibril formation through FBN1 (fibrillin-1) and FBN2 binding (Tsutsui et al., 2010). Fibrillin microfibrils not only award elastic properties to the matrix, but also bind LTBP proteins, controlling bioavailability of TGF-β superfamily molecules and, thus, regulating growth factor signaling (Chaudhry et al., 2007). Although there is little information concerning ADAMTSL1, we propose that alterations in both this component and FBN2 will impact cell-matrix interactions and influence cellular fate towards an oncogenic phenotype. Likewise, the increase of LTBPs is expected to enhance GC cells metastatic ability by promoting TGF-β-induced EMT (Chandramouli et al., 2011).
Among the downregulated proteins, we identified important basement membrane components, namely COL4A5, COL4A6, COL28A1, NID1, and LAMA5. The basement membrane is a specialized form of ECM that supports the epithelial layer and acts as a barrier that cancer cells need to breach to invade the surrounding stroma (Glentis et al., 2014). Baba and colleagues (Baba et al., 2007) have reported the gradual disappearance of type IV collagen alpha chains from the basement membrane which, along with changes in other structural components like laminins and nidogen, weaken this complex, favoring cancer cell invasion and progression. In addition, the proteoglycan decorin was found to be downregulated in the identified tumor matrisome, consistent with its role as a negative regulator of TGF-β signaling (Troup et al., 2003). In fact, our data suggest that during the gastric carcinogenic process, there is an increase in the levels of ECM components responsible for growth factor binding, particularly to TGF-β superfamily molecules, potentiating TGF-β-driven tumor growth and invasion, evasion of immune surveillance, cancer cell dissemination and future metastasis (Massagué, 2008). In contrast, downregulated proteins are mainly involved in cell adhesive properties, metabolism of glycosaminoglycans, and negative regulation of endopeptidase activity, in frame with the modulation of a pro-tumorigenic microenvironment (Wei et al., 2020; Zhang and Lin, 2021).
To corroborate our findings, we have analysed mRNA expression of identified candidates in an independent GC cohort available at the TGCA. Importantly, the expression profile of tumor and normal tissues was confirmed for 12 genes. Further, analysis of the association between those ECM components and GC clinicopathological features revealed that increased expression of COL1A2, LOX and LTBP2 significantly correlated with high tumor stage. Elevated COL1A2 levels were previously reported in patients with advanced disease stages (Yasui et al., 2004). Interestingly, co-expression of COL1A2 and LOX—whose expression in the TCGA-STAD database is highly correlated (Pearson’s correlation coefficient = 0.87, p-value = 6.21e-83)—promotes cancer drug-resistance by increasing collagen cross linking, consequently, stiffening the extracellular matrix and blocking drug diffusion (Di Paolo and Bocci, 2007; Sterzyńska et al., 2018). LTBP2 is known to regulate EMT markers and improve migratory and invasive capacities of GC cells through a mechanism independent of TGF-β signaling (Saharinen and Keski-Oja, 2000). Accordingly, we verified that high levels of LOX and LTBP2 were also associated with poorer patient outcome, when compared with those exhibiting low expression.
In conclusion, we have identified a set of ECM constituents that, to our knowledge, characterize for the first time the matrisome of gastric normal and tumor contexts. Of note, we found that quantitative changes of LOX, LTBP2 and COL1A2 contribute to disease progression and patient poor prognosis. These data provide evidence that ECM components should be explored as novel diagnostic and prognostic biomarkers in GC. Moreover, an essential topic within this research area will be to further advance the functional significance of the relevant ECM components. The understanding of ECM dynamics and the identification of its regulators will be key for the development of innovative therapeutic approaches.
Data Availability Statement
The mass spectrometry proteomics data have been deposited to the ProteomeXchange Consortium via the PRIDE (Perez-Riverol et al., 2019) partner repository (https://www.ebi.ac.uk/pride/archive/) with the dataset identifier PXD029782 and 10.6019/PXD029782.
Ethics Statement
The studies involving human participants were reviewed and approved by CHUSJ Ethics Committee for Health (Reference 32/18), in agreement with the Helsinki declaration.
Author Contributions
Study concept and design: AMM and RS. Data acquisition: AMM, RMF, HO, JB, JP, and FC. Data analysis and interpretation: AMM, RMF, PC, JF, HO, PP-d-Ó, and RS. Writing of the original draft: AMM and PC. Editing and critical review of the manuscript: RMF, JF, HO, JB, JP, PP-d-Ó, FC, and RS. All authors approved the final version of manuscript.
Funding
This work was financed by FEDER funds through the Operational Programme for Competitiveness Factors (COMPETE 2020), Programa Operacional de Competitividade e Internacionalizaçȧo (POCI) and Programa Operacional Regional do Norte (Norte 2020); by National Funds through the Portuguese Foundation for Science and Technology (FCT projects PTDC/BTM-SAL/30383/2017, PTDC/MED-GEN/30356/2017, NORTE-01-0145-FEDER-000029, and doctoral grant SFRH/BD/114687/2016-AMM. RMF is funded by the “FCT Scientific Employment Stimulus—Individual Call” program (CEECIND/01854/2017). We acknowledge the American Association of Patients with Hereditary Gastric Cancer “No Stomach for Cancer” for funding Seruca’s research, and the support from the Portuguese Mass Spectrometry Network, integrated in the National Roadmap of Research Infrastructures of Strategic Relevance (ROTEIRO/0028/2013; LISBOA-01-0145-FEDER-022125).
Conflict of Interest
The authors declare that the research was conducted in the absence of any commercial or financial relationships that could be construed as a potential conflict of interest.
Publisher’s Note
All claims expressed in this article are solely those of the authors and do not necessarily represent those of their affiliated organizations, or those of the publisher, the editors and the reviewers. Any product that may be evaluated in this article, or claim that may be made by its manufacturer, is not guaranteed or endorsed by the publisher.
Acknowledgments
The authors would like to thank all patients who have participated in this study. We thank Marta Diogo, Gilza Gonçalves and Cátia Silva from the Department of Pathology (CHSJ) for technical support.
Supplementary Material
The Supplementary Material for this article can be found online at: https://www.frontiersin.org/articles/10.3389/fmolb.2022.818552/full#supplementary-material
References
Acerbi, I., Cassereau, L., Dean, I., Shi, Q., Au, A., Park, C., et al. (2015). Human Breast Cancer Invasion and Aggression Correlates with ECM Stiffening and Immune Cell Infiltration. Integr. Biol. (Camb) 7 (10), 1120–1134. doi:10.1039/c5ib00040h
Arnold, M., Park, J. Y., Camargo, M. C., Lunet, N., Forman, D., and Soerjomataram, I. (2020). Is Gastric Cancer Becoming a Rare Disease? A Global Assessment of Predicted Incidence Trends to 2035. Gut 69 (5), 823–829. doi:10.1136/gutjnl-2019-320234
Ashburner, M., Ball, C. A., Blake, J. A., Botstein, D., Butler, H., Cherry, J. M., et al. (2000). Gene Ontology: Tool for the Unification of Biology. Nat. Genet. 25 (1), 25–29. doi:10.1038/75556
Baba, Y., Iyama, K.-i., Ikeda, K., Ishikawa, S., Hayashi, N., Miyanari, N., et al. (2007). Differential Expression of Basement Membrane Type IV Collagen α Chains in Gastric Intramucosal Neoplastic Lesions. J. Gastroenterol. 42 (11), 874–880. doi:10.1007/s00535-007-2112-2
Bartha, Á., and Győrffy, B. (2021). TNMplot.com: A Web Tool for the Comparison of Gene Expression in Normal, Tumor and Metastatic Tissues. Ijms 22 (5), 2622. doi:10.3390/ijms22052622
Battaglin, F., Naseem, M., Puccini, A., and Lenz, H.-J. (2018). Molecular Biomarkers in Gastro-Esophageal Cancer: Recent Developments, Current Trends and Future Directions. Cancer Cel Int 18, 99. doi:10.1186/s12935-018-0594-z
Blum, M., Chang, H.-Y., Chuguransky, S., Grego, T., Kandasaamy, S., Mitchell, A., et al. (2020). The InterPro Protein Families and Domains Database: 20 Years on. Nucleic Acids Res. 49 (D1), D344–D354. doi:10.1093/nar/gkaa977
Bonnans, C., Chou, J., and Werb, Z. (2014). Remodelling the Extracellular Matrix in Development and Disease. Nat. Rev. Mol. Cel Biol 15 (12), 786–801. doi:10.1038/nrm3904
Cancer Genome Atlas Research Network (2014). Comprehensive Molecular Characterization of Gastric Adenocarcinoma. Nature 513 (7517), 202–209. doi:10.1038/nature13480
Casbas-Hernandez, P., Sun, X., Roman-Perez, E., D'Arcy, M., Sandhu, R., Hishida, A., et al. (2015). Tumor Intrinsic Subtype Is Reflected in Cancer-Adjacent Tissue. Cancer Epidemiol. Biomarkers Prev. 24 (2), 406–414. doi:10.1158/1055-9965.epi-14-0934
Cerami, E., Gao, J., Dogrusoz, U., Gross, B. E., Sumer, S. O., Aksoy, B. A., et al. (2012). The cBio Cancer Genomics Portal: An Open Platform for Exploring Multidimensional Cancer Genomics Data: Figure 1. Cancer Discov. 2 (5), 401–404. doi:10.1158/2159-8290.cd-12-0095
Chandramouli, A., Simundza, J., Pinderhughes, A., and Cowin, P. (2011). Choreographing Metastasis to the Tune of LTBP. J. Mammary Gland Biol. Neoplasia 16 (2), 67–80. doi:10.1007/s10911-011-9215-3
Chaudhry, S. S., Cain, S. A., Morgan, A., Dallas, S. L., Shuttleworth, C. A., and Kielty, C. M. (2007). Fibrillin-1 Regulates the Bioavailability of TGFβ1. J. Cel Biol 176 (3), 355–367. doi:10.1083/jcb.200608167
Chen, J., Wang, X., Hu, B., He, Y., Qian, X., and Wang, W. (2018). Candidate Genes in Gastric Cancer Identified by Constructing a Weighted Gene Co-expression Network. PeerJ 6, e4692. doi:10.7717/peerj.4692
Di Paolo, A., and Bocci, G. (2007). Drug Distribution in Tumors: Mechanisms, Role in Drug Resistance, and Methods for Modification. Curr. Oncol. Rep. 9 (2), 109–114. doi:10.1007/s11912-007-0006-3
Erler, J. T., Bennewith, K. L., Nicolau, M., Dornhöfer, N., Kong, C., Le, Q.-T., et al. (2006). Lysyl Oxidase Is Essential for Hypoxia-Induced Metastasis. Nature 440 (7088), 1222–1226. doi:10.1038/nature04695
Figueiredo, J., Ferreira, R. M., Xu, H., Gonçalves, M., Barros-Carvalho, A., Cravo, J., et al. (2021). Integrin β1 Orchestrates the Abnormal Cell-Matrix Attachment and Invasive Behaviour of E-Cadherin Dysfunctional Cells. Gastric Cancer 25, 124–137. doi:10.1007/s10120-021-01239-9
Gao, J., Aksoy, B. A., Dogrusoz, U., Dresdner, G., Gross, B., Sumer, S. O., et al. (2013). Integrative Analysis of Complex Cancer Genomics and Clinical Profiles Using the cBioPortal. Sci. Signal. 6 (269), pl1. doi:10.1126/scisignal.2004088
Gene Ontology Consortium (2021). The Gene Ontology Resource: Enriching a GOld Mine. Nucleic Acids Res. 49 (D1), D325–d334. doi:10.1093/nar/gkaa1113
Glavey, S. V., Naba, A., Manier, S., Clauser, K., Tahri, S., Park, J., et al. (2017). Proteomic Characterization of Human Multiple Myeloma Bone Marrow Extracellular Matrix. Leukemia 31 (11), 2426–2434. doi:10.1038/leu.2017.102
Glentis, A., Gurchenkov, V., and Vignjevic, D. M. (2014). Assembly, Heterogeneity, and Breaching of the Basement Membranes. Cell Adhes. Migration 8 (3), 236–245. doi:10.4161/cam.28733
Henke, E., Nandigama, R., and Ergün, S. (2020). Extracellular Matrix in the Tumor Microenvironment and its Impact on Cancer Therapy. Front. Mol. Biosci. 6 (160). doi:10.3389/fmolb.2019.00160
Hoshiba, T. (2019). Decellularized Extracellular Matrix for Cancer Research. Materials 12 (8), 1311. doi:10.3390/ma12081311
Kanehisa, M., Sato, Y., Kawashima, M., Furumichi, M., and Tanabe, M. (2016). KEGG as a Reference Resource for Gene and Protein Annotation. Nucleic Acids Res. 44 (D1), D457–D462. doi:10.1093/nar/gkv1070
Laklai, H., Miroshnikova, Y. A., Pickup, M. W., Collisson, E. A., Kim, G. E., Barrett, A. S., et al. (2016). Genotype Tunes Pancreatic Ductal Adenocarcinoma Tissue Tension to Induce Matricellular Fibrosis and Tumor Progression. Nat. Med. 22 (5), 497–505. doi:10.1038/nm.4082
Levental, K. R., Yu, H., Kass, L., Lakins, J. N., Egeblad, M., Erler, J. T., et al. (2009). Matrix Crosslinking Forces Tumor Progression by Enhancing Integrin Signaling. Cell 139 (5), 891–906. doi:10.1016/j.cell.2009.10.027
Liu, Y., Sethi, N. S., Hinoue, T., Schneider, B. G., Cherniack, A. D., Sanchez-Vega, F., et al. (2018). Comparative Molecular Analysis of Gastrointestinal Adenocarcinomas. Cancer Cell 33 (4), 721–e8. doi:10.1016/j.ccell.2018.03.010
Mitchell, A. L., Attwood, T. K., Babbitt, P. C., Blum, M., Bork, P., Bridge, A., et al. (2019). InterPro in 2019: Improving Coverage, Classification and Access to Protein Sequence Annotations. Nucleic Acids Res. 47 (D1), D351–d360. doi:10.1093/nar/gky1100
Mohan, V., Das, A., and Sagi, I. (2020). Emerging Roles of ECM Remodeling Processes in Cancer. Semin. Cancer Biol. 62, 192–200. doi:10.1016/j.semcancer.2019.09.004
Moreira, A. M., Pereira, J., Melo, S., Fernandes, M. S., Carneiro, P., Seruca, R., et al. (2020). The Extracellular Matrix: An Accomplice in Gastric Cancer Development and Progression. Cells 9 (2), 394. doi:10.3390/cells9020394
Naba, A., Clauser, K. R., Hoersch, S., Liu, H., Carr, S. A., and Hynes, R. O. (2012). The Matrisome: In Silico Definition and In Vivo Characterization by Proteomics of normal and Tumor Extracellular Matrices. Mol. Cell Proteomics 11 (4), M111014647. doi:10.1074/mcp.M111.014647
Naba, A., Clauser, K. R., and Hynes, R. O. (2015). Enrichment of Extracellular Matrix Proteins from Tissues and Digestion into Peptides for Mass Spectrometry Analysis. JoVE 101, e53057. doi:10.3791/53057
Naba, A., Clauser, K. R., Lamar, J. M., Carr, S. A., and Hynes, R. O. (2014a). Extracellular Matrix Signatures of Human Mammary Carcinoma Identify Novel Metastasis Promoters. Elife 3, e01308. doi:10.7554/eLife.01308
Naba, A., Clauser, K. R., Whittaker, C. A., Carr, S. A., Tanabe, K. K., and Hynes, R. O. (2014b). Extracellular Matrix Signatures of Human Primary Metastatic colon Cancers and Their Metastases to Liver. BMC Cancer 14, 518. doi:10.1186/1471-2407-14-518
Naba, A., Pearce, O. M. T., Del Rosario, A., Ma, D., Ding, H., Rajeeve, V., et al. (2017). Characterization of the Extracellular Matrix of Normal and Diseased Tissues Using Proteomics. J. Proteome Res. 16 (8), 3083–3091. doi:10.1021/acs.jproteome.7b00191
Necula, L., Matei, L., Dragu, D., Neagu, A. I., Mambet, C., Nedeianu, S., et al. (2019). Recent Advances in Gastric Cancer Early Diagnosis. Wjg 25 (17), 2029–2044. doi:10.3748/wjg.v25.i17.2029
Necula, L., Matei, L., Dragu, D., Pitica, I., Neagu, A. I., Bleotu, C., et al. (2020). High Plasma Levels of COL10A1 Are Associated with Advanced Tumor Stage in Gastric Cancer Patients. Wjg 26 (22), 3024–3033. doi:10.3748/wjg.v26.i22.3024
Osório, H., Silva, C., Ferreira, M., Gullo, I., Máximo, V., Barros, R., et al. (2021). Proteomics Analysis of Gastric Cancer Patients with Diabetes Mellitus. Jcm 10 (3), 407. doi:10.3390/jcm10030407
Perez-Riverol, Y., Csordas, A., Bai, J., Bernal-Llinares, M., Hewapathirana, S., Kundu, D. J., Inuganti, A., Griss, J., Mayer, G., Eisenacher, M., Pérez, E., Uszkoreit, J., Pfeuffer, J., Sachsenberg, T., Yilmaz, S., Tiwary, S., Cox, J., Audain, E., Walzer, M., Jarnuczak, A. F., Ternent, T., Brazma, A., and Vizcaíno, J. A. (2019). The PRIDE database and related tools and resources in 2019: improving support for quantification data. Nucleic Acids Res. 2019 Jan 8; 47 (D1), D442–D450. doi:10.1093/nar/gky1106
Pickup, M. W., Mouw, J. K., and Weaver, V. M. (2014). The Extracellular Matrix Modulates the Hallmarks of Cancer. EMBO Rep. 15 (12), 1243–1253. doi:10.15252/embr.201439246
Pinto, M. L., Rios, E., Silva, A. C., Neves, S. C., Caires, H. R., Pinto, A. T., et al. (2017). Decellularized Human Colorectal Cancer Matrices Polarize Macrophages towards an Anti-inflammatory Phenotype Promoting Cancer Cell Invasion via CCL18. Biomaterials 124, 211–224. doi:10.1016/j.biomaterials.2017.02.004
Porter, S., Clark, I. M., Kevorkian, L., and Edwards, D. R. (2005). The ADAMTS Metalloproteinases. Biochem. J. 386 (Pt 1), 15–27. doi:10.1042/bj20040424
Pundir, S., Martin, M. J., and O'Donovan, C. (2016). UniProt Tools. Curr. Protoc. Bioinformatics 53, 121–2921. doi:10.1002/0471250953.bi0129s53
Quail, D. F., and Joyce, J. A. (2013). Microenvironmental Regulation of Tumor Progression and Metastasis. Nat. Med. 19 (11), 1423–1437. doi:10.1038/nm.3394
Saharinen, J., and Keski-Oja, J. (2000). Specific Sequence Motif of 8-Cys Repeats of TGF-β Binding Proteins, LTBPs, Creates a Hydrophobic Interaction Surface for Binding of Small Latent TGF-β. MBoC 11 (8), 2691–2704. doi:10.1091/mbc.11.8.2691
Shao, X., Taha, I. N., Clauser, K. R., Gao, Y., and Naba, A. (2019). MatrisomeDB: the ECM-Protein Knowledge Database. Nucleic Acids Res. 48 (D1), D1136–D1144. doi:10.1093/nar/gkz849
Sterzyńska, K., Klejewski, A., Wojtowicz, K., Świerczewska, M., Nowacka, M., Kaźmierczak, D., et al. (2018). Mutual Expression of ALDH1A1, LOX, and Collagens in Ovarian Cancer Cell Lines as Combined CSCs- and ECM-Related Models of Drug Resistance Development. Ijms 20 (1), 54. doi:10.3390/ijms20010054
Sung, H., Ferlay, J., Siegel, R. L., Laversanne, M., Soerjomataram, I., Jemal, A., et al. (2021). Global Cancer Statistics 2020: GLOBOCAN Estimates of Incidence and Mortality Worldwide for 36 Cancers in 185 Countries. CA A. Cancer J. Clin. 71 (3), 209–249. doi:10.3322/caac.21660
Szklarczyk, D., Gable, A. L., Lyon, D., Junge, A., Wyder, S., Huerta-Cepas, J., et al. (2019). STRING V11: Protein-Protein Association Networks with Increased Coverage, Supporting Functional Discovery in Genome-wide Experimental Datasets. Nucleic Acids Res. 47 (D1), D607–d613. doi:10.1093/nar/gky1131
Takahashi, T., Saikawa, Y., and Kitagawa, Y. (2013). Gastric Cancer: Current Status of Diagnosis and Treatment. Cancers 5 (1), 48–63. doi:10.3390/cancers5010048
Troester, M. A., Lee, M. H., Carter, M., Fan, C., Cowan, D. W., Perez, E. R., et al. (2009). Activation of Host Wound Responses in Breast Cancer Microenvironment. Clin. Cancer Res. 15 (22), 7020–7028. doi:10.1158/1078-0432.ccr-09-1126
Troup, S., Njue, C., Kliewer, E. V., Parisien, M., Roskelley, C., Chakravarti, S., et al. (2003). Reduced Expression of the Small Leucine-Rich Proteoglycans, Lumican, and Decorin Is Associated with Poor Outcome in Node-Negative Invasive Breast Cancer. Clin. Cancer Res. 9 (1), 207–214.
Trujillo, K. A., Heaphy, C. M., Mai, M., Vargas, K. M., Jones, A. C., Vo, P., et al. (2011). Markers of Fibrosis and Epithelial to Mesenchymal Transition Demonstrate Field Cancerization in Histologically normal Tissue Adjacent to Breast Tumors. Int. J. Cancer 129 (6), 1310–1321. doi:10.1002/ijc.25788
Tsutsui, K., Manabe, R.-i., Yamada, T., Nakano, I., Oguri, Y., Keene, D. R., et al. (2010). ADAMTSL-6 Is a Novel Extracellular Matrix Protein that Binds to Fibrillin-1 and Promotes Fibrillin-1 Fibril Formation. J. Biol. Chem. 285 (7), 4870–4882. doi:10.1074/jbc.M109.076919
Wei, J., Hu, M., Huang, K., Lin, S., and Du, H. (2020). Roles of Proteoglycans and Glycosaminoglycans in Cancer Development and Progression. Ijms 21 (17), 5983. doi:10.3390/ijms21175983
Yang, X., Sun, H.-j., Li, Z.-r., Zhang, H., Yang, W.-j., Ni, B., et al. (2015). Gastric cancer-associated enhancement of von Willebrand factor is regulated by vascular endothelial growth factor and related to disease severity. BMC Cancer 15, 80. doi:10.1186/s12885-015-1083-6
Yasui, W., Oue, N., Ito, R., Kuraoka, K., and Nakayama, H. (2004). Search for New Biomarkers of Gastric Cancer through Serial Analysis of Gene Expression and its Clinical Implications. Cancer Sci. 95 (5), 385–392. doi:10.1111/j.1349-7006.2004.tb03220.x
Zhang, H., Pan, Y.-z., Cheung, M., Cao, M., Yu, C., Chen, L., et al. (2019). LAMB3 Mediates Apoptotic, Proliferative, Invasive, and Metastatic Behaviors in Pancreatic Cancer by Regulating the PI3K/Akt Signaling Pathway. Cell Death Dis 10 (3), 230. doi:10.1038/s41419-019-1320-z
Zhang, W., and Lin, Y. (2021). The Mechanism of Asparagine Endopeptidase in the Progression of Malignant Tumors: A Review. Cells 10 (5), 1153. doi:10.3390/cells10051153
Keywords: extracellular matrix (ECM), gastric cancer, matrisome, biomarker, proteomics
Citation: Moreira AM, Ferreira RM, Carneiro P, Figueiredo J, Osório H, Barbosa J, Preto J, Pinto-do-Ó P, Carneiro F and Seruca R (2022) Proteomic Identification of a Gastric Tumor ECM Signature Associated With Cancer Progression. Front. Mol. Biosci. 9:818552. doi: 10.3389/fmolb.2022.818552
Received: 19 November 2021; Accepted: 18 January 2022;
Published: 01 March 2022.
Edited by:
Rohit Upadhyay, Tulane University, United StatesReviewed by:
Daniele Vergara, University of Salento, ItalyMeenakshi Umar, Tulane University, United States
Copyright © 2022 Moreira, Ferreira, Carneiro, Figueiredo, Osório, Barbosa, Preto, Pinto-do-Ó, Carneiro and Seruca. This is an open-access article distributed under the terms of the Creative Commons Attribution License (CC BY). The use, distribution or reproduction in other forums is permitted, provided the original author(s) and the copyright owner(s) are credited and that the original publication in this journal is cited, in accordance with accepted academic practice. No use, distribution or reproduction is permitted which does not comply with these terms.
*Correspondence: Raquel Seruca, cnNlcnVjYUBpcGF0aW11cC5wdA==