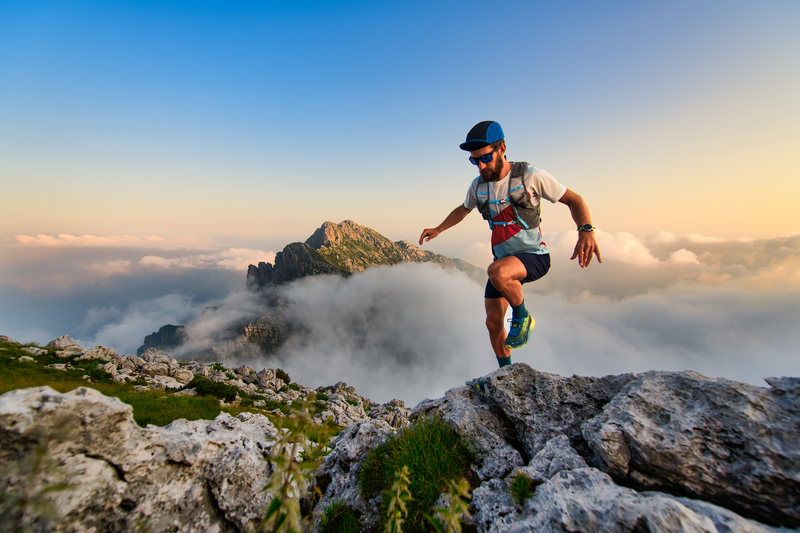
94% of researchers rate our articles as excellent or good
Learn more about the work of our research integrity team to safeguard the quality of each article we publish.
Find out more
ORIGINAL RESEARCH article
Front. Mol. Biosci. , 03 June 2022
Sec. Molecular Diagnostics and Therapeutics
Volume 9 - 2022 | https://doi.org/10.3389/fmolb.2022.812815
This article is part of the Research Topic Modelling Esophageal Adenocarcinoma View all 8 articles
Objectives: Potassium Calcium-Activated Channel Subfamily N Member 4 (KCNN4) is a member of the KCNN family. Studies have revealed that KCNN4 is implicated in various physiological processes as well as promotes the malignant phenotypes of cancer cells. However, little is known about its associations with survival outcomes across varying cancer types.
Methods: Herein, we systematically explored the prognostic value of KCNN4 in the pan-cancer dataset retrieved from multiple databases. Next, we performed correlation analysis of KCNN4 expression with tumor mutational burden (TMB) and microsatellite instability (MSI), and immune checkpoint genes (ICGs) to assess its potential as a predictor of immunotherapy efficacy. Afterwards, patients were divided into increased-risk group and decreased-risk group based on the contrasting survival outcomes in various cancer types. Furthermore, the underlying mechanisms of the distinctive effects were analyzed using ESTIMATE, CIBERSORT algorithms, and Gene Set Enrichment Analysis (GSEA) analysis.
Results: KCNN4 expression levels were aberrant in transcriptomic and proteomic levels between cancer and normal control tissues in pan-cancer datasets, further survival analysis elucidated that KCNN4 expression was correlated to multiple survival data, and clinical annotations. Besides, KCNN4 expression was correlated to TMB and MSI levels in 14 types and 12 types of pan-cancers, respectively. Meanwhile, different types of cancer have specific tumor-infiltrating immune cell (TICs) profiles.
Conclusions: Our results revealed that KCNN4 could be an essential biomarker for remodeling components in the tumor microenvironment (TME), and a robust indicator for predicting prognosis as well as immunotherapy response in pan-cancer patients.
Cancer is a serious disease which threatens human health and lowers life expectancy. According to statistics reported by the World Health Organization (WHO) in 2019, cancer was ranked as the first or second predominant cause of human death among people younger than 70 years in 112 out of 183 countries worldwide (Sung et al., 2021). In 2020, there were about 19,292,789 new cancer cases worldwide, with approximately 9,958,133 deaths. Notably, lung cancer is still the leading cause of cancer-related deaths, followed by colorectal cancer, liver cancer, stomach cancer, and female breast cancer (Sung et al., 2021). The discovery of immunotherapy introduced a new era into cancer treatment, and recent emergence of immune checkpoint inhibitors (ICIs) has revolutionized clinical cancer therapy (Billan et al., 2020). ICIs can block immune checkpoints pathway, thereby activating tumor-specific T cell immune responses and eliminating the cancer cells targeted by the immune system (Chen and Mellman, 2013). However, although clinical application of ICIs in recent years has presented encouraging efficacy in management of various tumors (Necchi et al., 2020), the treatment responses vary widely among different cancer types, along with individual differences in efficacy (Kelderman et al., 2014). Moreover, the adverse effects and the costs associated with the drugs pose significant challenges (Michot et al., 2016; Abdin et al., 2018). Therefore, this calls for identification of biomarkers to predict the prognosis and immunotherapy efficacy, with overarching goal of improving cancer treatment.
Potassium Calcium-Activated Channel Subfamily N Member 4 (KCNN4) is a member of the KCNN family, which encodes intermediate conductance potassium channel activated by intracytoplasmic calcium ions (Lin et al., 2020). Studies have revealed that KCNN4 is implicated in various physiological processes such as cell proliferation (Biasiotta et al., 2016), immune regulation (Chandy et al., 2004), and apoptosis (Simms et al., 2010). Notably, KCNN4 expression was upregulated in a variety of tumor tissues, including thyroid cancer (Wen et al., 2020), breast cancer (Zhang et al., 2016), and hepatocellular carcinoma (Du et al., 2019). In addition, aberrant KCNN4 expression was found to promote malignant phenotypes of cancer cells. Mo et al. (2021) reported that KCNN4 could induce progression and metastasis of pancreatic ductal adenocarcinoma (PDAC) through mesenchymal to epithelial transition factor (MET)-mediated AKT axis, whereas knockdown of KCNN4 resulted in apoptosis and cell cycle arrest in PDAC. These findings suggest its potential as a therapeutic target. Similarly, Lin et al. (2020) revealed that breast cancer progression and gemcitabine resistance could be a consequence of KCNN4 overexpression, suggesting that it could serve as a therapeutic strategy to overcome chemoresistance and improve the prognosis of breast cancer. However, it is worth noting that most studies on KCNN4 have been restricted to a single cancer type. Comparisons between diverse findings have been hampered by the sample sizes, methods for model construction, and the different experimental approaches. Therefore, there is an urgent need to extensively characterize the landscape of KCNN4 in pan-cancer datasets, which will provide novel insights for developing effective therapies for management of cancers.
Herein, we systematically dissected the prognostic value of KCNN4 in the pan-cancer dataset by combining multiple databases, including The Cancer Genome Atlas (TCGA), Tumor Immune Estimation Resource (TIMER), UALCAN, Clinical Proteomic Tumor Analysis Consortium (CPTAC), and cBioPortal databases. Correlation analysis of KCNN4 expression with tumor mutational burden (TMB), microsatellite instability (MSI), and immune checkpoint genes (ICGs) was then performed to explore its potential as a predictor of immunotherapy efficacy. Next, patients were divided into increased-risk, and decreased-risk groups based upon the contrasting survival outcomes in various cancer types. Furthermore, we elucidated the underlying mechanisms of the distinctive effects using ESTIMATE, CIBERSORT algorithms, and Gene Set Enrichment Analysis (GSEA). In summary, our findings revealed that KCNN4 is an essential identity for remodeling components in the tumor microenvironment (TME), and a robust biomarker for predicting prognosis and immunotherapy response in pan-cancer patients. Analytical workflow of the research is described in Figure 1.
RNA sequencing profiles, somatic mutations data, and associated clinical annotations for 33 types of human pan-cancer cohorts were downloaded from TCGA database using University of California Santa Cruz Xena (UCSC Xena; https://xena.ucsc.edu/) browser. The pan-cancer subtypes included the following: adrenocortical carcinoma (ACC), bladder urothelial carcinoma (BLCA), breast invasive carcinoma (BRCA), cervical squamous cell carcinoma and endocervical adenocarcinoma (CESC), cholangiocarcinoma (CHOL), colon adenocarcinoma (COAD), lymphoid neoplasm diffuse large B-cell lymphoma (DLBC), esophageal carcinoma (ESCA), glioblastoma multiforme (GBM), head and neck squamous cell carcinoma (HNSC), kidney chromophobe (KICH), kidney renal clear cell carcinoma (KIRC), kidney renal papillary cell carcinoma (KIRP), acute myeloid leukemia (LAML), brain lower grade glioma (LGG), lung squamous cell carcinoma (LUSC), liver hepatocellular carcinoma (LIHC), lung adenocarcinoma (LUAD), mesothelioma (MESO), ovarian serous cystadenocarcinoma (OV), prostate adenocarcinoma (PRAD), pancreatic adenocarcinoma (PAAD), pheochromocytoma and paraganglioma (PCPG), rectum adenocarcinoma (READ), stomach adenocarcinoma (STAD), sarcoma (SARC), skin cutaneous melanoma (SKCM), testicular germ cell tumors (TGCT), thyroid carcinoma (THCA), thymoma (THYM), uterine corpus endometrial carcinoma (UCEC), uveal melanoma (UVM), and uterine carcinosarcoma (UCS). The fragments per kilobase million (FPKM) values of RNA sequencing data downloaded from UCSC Xena were normalized as transcripts per kilobase million (TPMs) for the following analyses (Wagner et al., 2012; Abrams et al., 2019; Guo et al., 2022).
Differential expression analysis of KCNN4 between tumor and normal control tissues at the transcriptomic level was analyzed using TIMER database, which is an interactive web portal containing RNA sequencing profiles from TCGA database. Expression levels of KCNN4 were shown as log2 TPM value. In addition, comparison of protein expression patterns between tumor and normal control tissues were performed based on the UALCAN database, comprising proteomics data derived from the CPTAC database.
Kaplan-Meier curve analysis was performed to analyze correlation between KCNN4 expression and survival indicators, including overall survival (OS), disease-specific survival (DSS), and progression-free interval (PFI) of 33 types of pan-cancer patients. Subsequently, univariate Cox analysis was used to analyze the associations between KCNN4 expression and the above-mentioned survival indicators. Notably, “survival” (Therneau, 2015), “survminer” (Kassambara et al., 2018), and “forestplot” (Max Gordon and Lumley, 2020) R packages were used in the above analyses.
Somatic mutation data and clinical annotations of pan-cancer patients were downloaded from the TCGA database. For the clinical annotations such as age, gender, and tumor stage, we converted the clinical annotations into categorical variables, and compared the differences in KCNN4 expression levels between the two groups of patients with the Wilcoxon test via “limma” packages (Ritchie et al., 2015), and visualized by “ggpubr” (Kassambara, 2020) R package. Given the distribution pattern of the values and the variances between samples, Spearman’s correlation was used to analyze the correlation of KCNN4 expression with MSI and TMB was analyzed, and visualized as radar maps using “fmsb” (Nakazawa, 2018) R package.
To determine the proportion of immune components in the TME of pan-cancer samples, we used the ESTIMATE algorithm (Yoshihara et al., 2013) to calculate immune and stromal scores using “estimate” (Kosuke et al., 2021) and “limma” (Ritchie et al., 2015) R packages. Next, correlation analysis between KCNN4 expression and immune and stromal scores were performed. Moreover, relative tumor-infiltrating immune cells (TICs) levels were calculated via the CIBERSORT algorithm (Chen et al., 2018) and only tumor samples with p < 0.05 were retained for subsequent analysis. Similarly, correlation analysis between KCNN4 expression and relative TICs levels were carried out using Spearman’s correlation analysis, and visualized using “ggplot2” (Wickham, 2016), “ggpubr” (Kassambara, 2020), and “ggExtra” (Attali and Baker, 2019) R packages.
Co-expression analysis between KCNN4 and immune-related genes was performed through “limma” (Ritchie et al., 2015) R package, further processing the data via “reshape2” (Wickham, 2007), and visualized by “RColorBrewer” (Erich, 2014) package. GSEA analysis was then carried out to decipher the mechanisms of KCNN4 in pan-cancers, and the top five enrichment terms of each tumor types were shown using the “clusterProfiler” (Yu et al., 2012) R package.
Transcriptomic expression patterns of KCNN4 between cancer and normal control tissues were compared through non-parametric Mann-Whitney test according to TIMER database. Comparisons of protein expression patterns and methylation levels of KCNN4 between tumor and normal control tissues were performed using Student’s t test based on the UALCAN database. Moreover, Kaplan-Meier curve analysis and univariate Cox regression analysis were performed to determine the association between KCNN4 expression and survival information of pan-cancer patients. p < 0.05 was considered statistically significant.
To evaluate the expression patterns of KCNN4, we performed differential analysis of KCNN4 expression between pan-cancer and normal control tissues at transcriptomic level based on TIMER database, and at proteomic level based on the UALCAN database. Results obtained in the TIMER database denoted that KCNN4 was significantly upregulated in various types of cancer tissues compared to normal control, including BLCA, CHOL, COAD, KIRC, KIRP, LIHC, LUAD, LUSC, READ, STAD, THCA, and UCEC. Conversely, the expression level of KCNN4 was diminished in BRCA and KICH tissues (Figure 2). In addition, KCNN4 protein expression was augmented in COAD and LUAD tissues compared to normal tissues, which was consistent with the transcriptomic profiles (Supplementary Figure S1). Notwithstanding, KCNN4 protein expression was upregulated in BRCA tissues, which was in contrast to the transcriptomic expression levels. Collectively, the results revealed the distinctively different KCNN4 expression patterns between cancer and normal samples in pan-cancer datasets.
FIGURE 2. Expression patterns of KCNN4 in pan-cancer datasets based on TIMER database. ∗p < 0.05, ∗∗p < 0.01, ∗∗∗p < 0.001.
Next, we investigated genetic alteration of KCNN4 based on cBioPortal database. Results indicated that KCNN4 was altered in 168 out of 10,508 patients, accounting for 1.6% of the total participants in the pan-cancer atlas studies. Amplification was the most predominant type of genetic alteration, followed by mutations and deep deletion. UCEC had the highest alteration frequency among the pan-cancer types (Figure 3). Subsequently, the methylation levels of KCNN4 between pan-cancer and normal control tissues were analyzed, indicating significant downregulation of methylation levels in tumor tissues compared to normal tissues in BLCA, BRCA, CESC, COAD, ESCA, HNSC, KIRC, KIRP, LIHC, LUAD, LUSC, PAAD, PCPG, READ, THCA, and UCEC, whereas PRAD presented higher levels of methylation in cancer tissues compared to normal control tissues (Supplementary Figure S2).
We then analyzed the correlation between KCNN4 expression level and the survival indicators of pan-cancer patients, including OS, DSS, and PFI. The Kaplan–Meier curve analysis demonstrated that high expression of KCNN4 could exert unfavorable influences in the survival indicators (Figures 4A–C), including KIRP (DSS: p = 0.021; PFI: p = 0.029), LGG (OS: p < 0.001; DSS: p < 0.001; PFI: p = 0.002), MESO (OS: p = 0.044; PFI: p = 0.006), PAAD (OS: p = 0.001; DSS: p = 0.008; PFI: p = 0.003), GBM (OS: p = 0.004; DSS: p = 0.003; PFI: p = 0.003), LUSC (PFI: p = 0.023), and KIRC (OS: p < 0.001; DSS: p < 0.001; PFI: p < 0.001). Interestingly, high expression of KCNN4 played a protective role in six types of pan-cancers (Figures 4A–C), including SKCM (OS: p = 0.006; DSS: p = 0.024), THYM (OS: p = 0.01), BLCA (DSS: p = 0.038; PFI: p < 0.001); PRAD (PFI = 0.032), BRCA (OS: p = 0.042), and CHOL (PFI = 0.002).
FIGURE 4. Correlation between KCNN4 expression level and survival indicators as determined through Kaplan–Meier curve analysis. (A) Correlation between KCNN4 expression level and OS as determined through Kaplan–Meier curve analysis. (B) Correlation between KCNN4 expression level and DSS as determined by Kaplan–Meier curve analysis. (C) Correlation between KCNN4 expression level and PFI as determined by Kaplan–Meier curve analysis.
Next, we performed univariate Cox analysis on the described survival indicators (Figures 5A–C). Results showed that KCNN4 could act as an adverse prognostic factor in GBM (OS: HR = 1.033, p < 0.001; DSS: HR = 1.036, p < 0.001; PFI: HR = 1.032, p = 0.002), KICH (PFI: HR = 1.128, p = 0.009), KIRC (OS: HR = 1.072, p < 0.001; DSS: HR = 1.094, p < 0.001; PFI: HR = 1.063, p < 0.001), LGG (OS: HR = 1.113, p < 0.001; DSS: HR = 1.113, p < 0.001; PFI: HR = 1.120, p < 0.001), LUAD (OS: HR = 1.003, p = 0.043), LUSC (DSS: HR = 1.006, p = 0.013; PFI: HR = 1.006, p = 0.001), MESO (OS: HR = 1.005, p = 0.049), ACC (OS: HR = 1.015, p = 0.018), PRAD (PFI: HR = 1.002, p = 0.014), and PAAD (OS: HR = 1.002, p = 0.018; PFI: HR = 1.002, p = 0.014). According to the univariate Cox analysis results, KCNN4 was also a positive prognostic factor in the following pan-cancer types (Figures 5A–C), containing BLCA (DSS: HR = 0.997, p = 0.049; PFI: HR = 0.997, p = 0.038), PRAD (PFI: HR = 0.997, p = 0.041), THYM (OS: HR = 0.847, p = 0.006), SKCM (OS: HR = 0.997, p < 0.001; DSS: HR = 0.997, p < 0.001), and UCEC (DSS: HR = 0.991, p = 0.014; PFI: HR = 0.995, p = 0.013).
FIGURE 5. Correlation between KCNN4 expression level and survival indicators as determined through univariate Cox analysis. (A) Univariate Cox analysis of the correlation between KCNN4 expression level and OS. (B) Univariate Cox analysis of the correlation between KCNN4 expression level and DSS. (C) Univariate Cox analysis of the correlation between KCNN4 expression level and PFI.
Altogether, these results suggest that KCNN4 could be a potential biomarker for predicting the prognosis of cancer. In addition, KCNN4 may play a completely varying physiological role in various cancer types, thereby resulting in varying survival outcomes in patients.
The correlation between clinical annotations and KCNN4 expression was subsequently analyzed, with results confirming that KCNN4 expression was correlated with multiple clinical indicators. As shown in Figure 6A, patients younger than or equal to 65 years old had higher KCNN4 expression levels than patients older than 65 years old in SKCM, UCEC, PRAD, and BRCA cancers. In contrast, higher expression levels of KCNN4 were observed in patients older than 65 years in LGG and SARC cancers. Interestingly, KCNN4 expression also presented sex-specific differences in BRCA and LUAD (Figure 6B). Importantly, the expression levels of KCNN4 were significantly higher in stage III-IV patients than in stage I-II patients in THCA and KIRC cancers, suggesting that KCNN4 may reflect the clinical progression of these two tumors (Figure 6C).
FIGURE 6. Correlation between KCNN4 expression level and clinical annotations. (A) Correlation between KCNN4 expression level and ages in SKCM, UCEC, PRAD, BRCA, LGG, and SARC. (B) Correlation between KCNN4 expression level and gender in BRCA, and LUAD. (C) Correlation between KCNN4 expression level and tumor stages in KIRC, and THCA.
We further explored the correlation of KCNN4 expression with TMB, MSI, and ICGs to determine the potential of KCNN4 as a biomarker for reflecting the efficacy of immunotherapy. TMB has been shown to be directly correlated with immunotherapy response in a variety of tumors (Yarchoan et al., 2017; Chan et al., 2019). We discovered that KCNN4 expression was correlated with TMB levels in 14 pan-cancer subtypes (Figure 7A). Specifically, KCNN4 expression levels were positively associated with TMB levels in BRCA, DLBC, ESCA, LGG, PAAD, SKCM, and STAD. In contrast, KCNN4 expression was negatively correlated with TMB levels in CHOL, COAD, LIHC, LUSC, PRAD, THYM, and TGCT.
FIGURE 7. Correlation of KCNN4 expression with TMB, MSI, and ICGs. (A) Correlation between KCNN4 expression and TMB. (B) Correlation between KCNN4 expression and MSI. (C) Correlation of KCNN4 expression and ICGs.
Similarly, MSI is a recognized indicator that has a predictive value for the activity of immunotherapy (Dudley et al., 2016; Petrelli et al., 2020). Herein, we analyzed associations between the expression of KCNN4 and MSI levels in pan-cancer datasets (Figure 7B). Results revealed that KCNN4 expression was positively linked with MSI levels in BRCA, STAD, SARC, and THCA, and negatively linked with MSI levels in CHOL, COAD, LIHC, PCPG, PRAD, TGCT, THYM, and UCS.
Given that ICGs are a feasible indicator associated with efficacy of immunotherapy (Larkin et al., 2015; Chen and Mellman, 2017), we further performed correlation analysis between ICGs and KCNN4 expression in pan-cancer subtypes (Figure 7C). Notably, KCNN4 expression was significantly correlated with multiple ICGs, indicating positive correlation with most of the ICGs in LGG, TGCT, and THCA. From another point of view, KCNN4 expression was negatively correlated with a large portion of ICGs in BRCA and COAD. Besides, the results provided by Tumor Immune Dysfunction and Exclusion (TIDE) database (Jiang et al., 2018) indicated that KCNN4 has the moderate ability to predict clinical response of varying cancer cohorts treated with immunotherapy (Supplementary Figure S3), with 11 of the 21 immunotherapy-treated cohorts having an area under the receiver operating characteristic curve (AUC) greater than 0.5. Specifically, melanoma, and HNSC cohorts exhibited the strongest predictive likeliness of positive treatment responses for immunotherapy, with AUCs of 0.7229 and 0.65, respectively.
Overall, these results suggest that KCNN4 expression is correlated with TMB, MSI, and ICGs in multiple pan-cancer datasets, combined with evidence from TIDE, convincingly indicating that it could be a robust and reliable biomarker for predicting the responses of cancer cells to immunotherapy.
Studies have reported that immune and stromal components of the TME could affect cancer cell proliferation and response to therapy (Nakasone et al., 2012; Baghban et al., 2020). We aimed at unravelling the underlying mechanism of the contrary prognosis caused by KCNN4 via analyzing the components of TME in pan-cancer types. Therefore, the pan-cancers were divided into increased-risk group (four pan-cancer types) and decreased-risk group (two pan-cancer types) in according to the association between KCNN4 expression with survival outcome of pan-cancer patients obtained from Kaplan–Meier curve and univariate Cox analysis (Table 1). The ESTIMATE algorithm was then used to calculate the immune score and stromal score of the subjects in these six pan-cancer types, followed by analysis of their correlation with KCNN4.
TABLE 1. Univariate Cox analysis of the correlation between KCNN4 expression level and survival indicators.
Notably, p < 0.05 was used as the cut-off value for correlation analysis. Results manifested that the immune scores of four pan-cancer types in the increased-risk group, including LGG, PAAD, GBM, and KIRC, were correlated with KCNN4 expression levels (Figure 8A). In addition, the immune scores of SKCM in the decreased-risk group was correlated with KCNN4 expression levels (Figure 8B). Unexpectedly, the immune score was positively correlated with KCNN4 expression in most of the tumor types in the two groups, and PAAD was the only pan-cancer type which was negatively correlated with KCNN4 expression. Similarly, the stromal score was positively correlated with KCNN4 expression in most pan-cancer types in both groups, with the exception of PAAD. Therefore, we hypothesized that KCNN4 could reprogram immune and stromal components of TME, thereby affecting the survival outcome of patients.
FIGURE 8. Correlation between KCNN4 expression and different components of TME. (A) Correlation between KCNN4 expression and different components of the increased-risk group. (B) Correlation between KCNN4 expression and various components of the decreased-risk group.
To explore the association of each TIC subtypes with KCNN4 expression, the CIBERSORT algorithm was used to calculate the relative levels of TIC subtypes in patients in both groups, with p < 0.05 as the cut-off value. Interestingly, it was found that the immune components in the KIRC TME had the most profound effect of KCNN4, with a significant association between the levels of multiple TIC subtypes and KCNN4 expression (Figures 9A–K), and our results denoted that KCNN4 expression level was negatively linked with macrophage M2 in GBM (Figure 9L). In addition, KCNN4 expression levels were significantly positively correlated with macrophages (M0, M1, and M2), naïve B cells, and CD8 T cells in LGG, and negatively correlated with monocytes, activated NK cells, eosinophils, and activated mast cells. Furthermore, KCNN4 expression levels were positively linked with memory B cells, and macrophages M0, while negatively correlated with activated memory CD4 T cells and naïve B cells in PAAD. Significant associations also presented between immune components and KCNN4 expression in the decreased-risk group (Figure 10A). In summary, the results demonstrated that KCNN4 may exert its influence on tumor biological behavior by affecting various components in the TME, especially immune components. Thus, we performed a correlation analysis between KCNN4 and various immune-related genes (Figure 10B). Results revealed close interaction between KCNN4 and several immune-related genes, suggesting that KCNN4 may interfere with the balance between anti- and pro-tumor effects in the TME by influencing the expression of immune-related genes, which in turn mediates tumor progression and metastasis.
FIGURE 9. Association of TIC subtype with KCNN4 expression in increased-risk group. (A–K) Association of each TIC subtype with KCNN4 expression in KIRC. (L) Association of each TIC subtype with KCNN4 expression in GBM. (M–U) Association of each TIC subtype with KCNN4 expression in LGG. (V–Y) Association of each TIC subtype with KCNN4 expression in PAAD.
FIGURE 10. Association of TIC subtype with KCNN4 expression in decreased-risk group and the association of KCNN4 expression with immune-related genes. (A–E) Association of each TIC subtype with KCNN4 expression in BLCA. (F–M) Association of each TIC subtype with KCNN4 expression in SKCM. (N) Correlation between KCNN4 expression and immune-related genes.
To further elucidate the potential molecular mechanism of KCNN4 in pan-cancers in the two groups, GSEA analysis was further performed on the six pan-cancer types (Figure 11). The results showed that KCNN4 expression was correlated with multiple immune-related ontology (GO) terms in the increased-risk group (Figure 11A), such as regulation of immune effector process in PAAD, chemokine activity in GBM, and leukocyte migration in LGG. Similarly, the expression of KCNN4 was involved in the positive regulation of leukocytes cell-cell adhesion in SKCM in the decreased-risk group, indicating that immune regulation may be one of the vital mechanisms through which KCNN4 plays a biological role.
FIGURE 11. GSEA analysis of top functional terms associated with KCNN4 expression. (A) GO functional terms of KCNN4 in various cancer types in the increased-risk group and decreased-risk group. (B) KEGG pathway analysis of KCNN4 in various cancer types in the increased-risk group and decreased-risk group.
Moreover, Kyoto Encyclopedia of Genes and Genomes (KEGG) analysis indicated that KCNN4 was mainly enriched in primary immunodeficiency of KIRC, intestine immune network for IgA production of PAAD, and cytokine-cytokine receptor interaction shared by GBM, and LGG (Figure 11B). Overall, these results demonstrated that KCNN4 was consistently enriched in cytokine, regulation of immune system, and inflammatory response related pathways, which confirms that KCNN4 acts as an essential identity for remodeling of the TME in various cancers.
The global burden of cancer incidence and cancer-related mortality has been progressively increasing (Bray et al., 2021). Such epidemiologic transition is an indication of both the accelerated aging of the population and the increased exposure to cancer-related risk factors (Gomes et al., 2020). The clinical use of new classes of anticancer drugs (e.g., targeted agents and ICIs) has led to improved survival rates in many cancer patients. Nevertheless, the heavy financial burden has negative implications on both the society and individuals (Howard et al., 2015; Savage et al., 2017). Per capita spending was reported to be four times higher for patients who received chemotherapy compared to those who did not (Nardi et al., 2016). Meanwhile, the exorbitant expenditure is not always bound up with clinical benefits in patients (Carrera et al., 2018). Therefore, implementing the concept of precision medicine, and identifying clinical biomarkers for predicting cancer prognosis and therapeutic response will provide valuable insights for cancer management and prevention.
Herein, we performed a comprehensive and systematic study of KCNN4 using pan-cancer datasets, confirming that its aberrant expression and significant downregulation of methylation levels in cancer tissues compared to non-cancerous tissues. Interestingly, the expression of KCNN4 in BRCA tissues was contradictory at the transcriptomic and proteomic levels, which may be attributed to the limited control sample sizes in proteomics or the involvement of post-transcriptional regulation. Besides, KCNN4 was significantly tied in with survival indicators and clinical annotations, including tumor stages in multiple cancer types, which suggests its potential role as a prognostic biomarker for cancers. Summarily, the role of KCNN4 in tumorigenesis has been supported by in vitro, and in vivo experiments, which is often recognized as an oncogene involving in the regulation of multiple cancer-related signaling pathways, thus mediating tumor progression, and development. Li et al. (2020) previously reported that KCNN4 could promote progression and invasion of hepatocellular carcinoma cells through mitogen-activated protein kinase (MAPK)/extracellular signal regulated kinase (ERK) pathway. Similarly, the association of KCNN4 with PI3K (Lin et al., 2020), endoplasmic reticulum stress (Yu et al., 2017), and TGF-β1 (Huang et al., 2013) signaling pathway have also been demonstrated. Note worthily, it has been well documented that KCNN4 is implicated in the evolution of resistance to anti-cancer therapies, as evidenced by Mohr et al. (2019) and Lin et al. (2020), indicating that KCNN4 could confer chemoresistance, and radioresistance in breast cancer, respectively.
The clinical development of immunotherapy has seen an unprecedented rise since the first ICIs were available. During the past several years, researchers have made great strides in identifying a robust biomarker for predicting efficacy of immunotherapy treatment (Gong et al., 2018). Studies have shown that MSI, defined as the collection of microsatellites mutations normally constituted by repeat length alterations and caused by deficient mismatch repair system (Vilar and Gruber, 2010), plays an essential role as a predictive biomarker for immunotherapy (Luchini et al., 2019). Pembrolizumab is a selective monoclonal antibody against PD-1, showing encouraging efficacy in multiple cancer types (Le et al., 2017). Results obtained from the clinical trials revealed that administration of pembrolizumab had moderate disease control rates and better survival outcomes in microsatellite instability-high (MSI-H) progressive metastatic colorectal cancer (Le et al., 2015). Similarly, high tumor mutational burden (TMB-H) was intimately linked to better objective response rates (ORR) and longer survival times after administration of immunotherapy (Yarchoan et al., 2017; Hellmann et al., 2018). Moreover, a recent study reported that TMB-H is a predictive marker for better OS in advanced gastric cancer patients who received toripalimab as a single agent (Wang et al., 2019). It was also correlated with clinical efficacy of pembrolizumab in the treatment of non–small cell lung cancer, manifesting as better ORR and PFI referred to as low tumor mutational burden (TMB-L) (Hellmann et al., 2018). Together with TMB and MSI, ICGs have been documented to be reliable biomarkers for predicting efficacy of immunotherapy. Expression of PD-L1 and PD-1 were the earliest recognized candidate biomarkers used to predict the efficacy of immunotherapy (Topalian et al., 2012; Taube et al., 2014; Doroshow et al., 2021), and were approved by the FDA for pre-treatment evaluation in patients with melanoma (Kaushik et al., 2022), bladder cancer (Roviello et al., 2021), gastric cancer (Huynh et al., 2021), and cervical cancer (Odiase et al., 2021). Moreover, transcriptomic and methylation levels of CTLA4 can be used for the same purpose in melanoma patients (Goltz et al., 2018; Mo et al., 2018), thereby providing critical references for selection of cancer patients likely to respond to ICIs. Similarly, other ICGs, including lymphocyte activation gene-3 (LAG-3), T cell immunoglobulin, and ITIM domain (TIGIT), have presented promising results in clinical trials (Andrews et al., 2017; Qin et al., 2019). Whereas, although great breakthroughs have been made in this field in the past years, each of the above-mentioned indicators has its limitations (Balar and Weber, 2017), which hampers selection of the most appropriate biomarker by oncologists. This study explored the possibility of using KCNN4 as a predictor of immunotherapy efficacy. It is noteworthy that KCNN4 expression was significantly correlated with TMB levels in 14 tumor types, with MSI levels in 12 tumor types, and with expression levels of multiple ICGs in several tumor types. Surprisingly, the expression levels of KCNN4 showed a consistently significant correlation with the above indicators in BRCA, COAD, LIHC, and TGCT. The results suggest that KCNN4 may have a higher accuracy in reflecting the response rate of immunotherapy for these cancer types. To sum up, these findings provide novel insights to break the current bottleneck of biomarker development in immunotherapy.
The TME is an intricate ecosystem made up of multiple immune cells and stromal cells. Notably, tumor progression and invasion result from the dynamic crosstalk between varying components in the TME (Wu and Dai, 2017), which is the predominant culprit for the development of drug resistance in anti-tumor therapies (Hinshaw and Shevde, 2019). Herein, results showed that cancer cells could reprogram the immune and stromal components of the TME, which indicates that they play a decisive role in tumor metastasis. It should be noted that the immune components release growth factors to promote the invasion of cancer cells (Goswami et al., 2005), whereas the stromal components secret extracellular matrix and cytokines (Räsänen and Vaheri, 2010), thereby contributing to therapeutic resistance (Paraiso and Smalley, 2013). Future studies should explore the dynamic changes of varying components in the TME, which will aid in identifying the immune status of the TME and elucidating the underlying mechanisms of tumorigenesis in certain tumor types. Herein, we found significant correlations between KCNN4 and immune scores as well as stromal scores in four tumor types in the increased-risk group, whereas KCNN4 expression was associated with immune and stromal scores of the SKCM in the decreased-risk group. Collectively, the above results suggest that KCNN4 may participate in remodeling the TME, which is consistent with results reported in a previous study (Brown et al., 2018; Chimote et al., 2018; Chen et al., 2021). Interestingly, we discovered that KCNN4 expression was correlated with various TIC subtypes and macrophages are the most frequently occurring cell types in different cancers. Xu et al. (2014) reported that the expression levels of KCNN4 could be upregulated in colorectal cancer cells co-cultured with tumor-associated macrophages (TAMs), which confirms that KCNN4 could be involved in inducing secretion of interleukins by TAMs and enhancing the malignant phenotypes of cancer cells. Overall, our findings demonstrated that KCNN4 may modulate the immune status of the TME via varying TIC subtypes and exerting influences in the survival outcomes of cancer patients, thereby unravelling the underlying mechanism of KCNN4 in the TME. Although there are certain pivotal conclusions obtained in our study, it has some limitations. First, it should be noted that the pan-cancer cohorts in this study only originated from TCGA database, and additional datasets are still imperative to further confirm our conclusions. Second, we concentrate on bioinformatics analysis, and in vivo and in vitro experiments should be performed to elucidate the mechanism by which KCNN4 affects TME. Third, as presented, the effect size produced by KCNN4 is relatively limited, suggesting that it may exert synergistic effects with other essential genes in TME to influence tumorigenesis, but the underlying mechanisms have not been fully elucidated.
In summary, the results obtained in this study suggest that KCNN4 is a biomarker that can predict the prognosis of several cancer types. In addition, KCNN4 is associated with TMB, MSI, and varying components in TME, which confirms its potential for predicting the efficacy of immunotherapy treatment and immune status in TME. The findings of this study will provide insights for developing and expanding novel targets for further cancer management and prevention.
The datasets presented in this study can be found in online repositories. The names of the repository/repositories and accession number(s) can be found in the article/Supplementary Material.
ZM and SC designed and supervised the study. SC analyzed the data, XS interpreted the results and wrote the initial manuscript draft. SC and XS revised manuscript draft.
The authors declare that the research was conducted in the absence of any commercial or financial relationships that could be construed as a potential conflict of interest.
All claims expressed in this article are solely those of the authors and do not necessarily represent those of their affiliated organizations, or those of the publisher, the editors and the reviewers. Any product that may be evaluated in this article, or claim that may be made by its manufacturer, is not guaranteed or endorsed by the publisher.
The authors would like to thank Home for Researchers (www.home-for-researchers.com), for editing the manuscript.
The Supplementary Material for this article can be found online at: https://www.frontiersin.org/articles/10.3389/fmolb.2022.812815/full#supplementary-material
Supplementary Figure S1 | Analysis of KCNN4 expression patterns at the proteomic level between pan-cancer and non-cancerous samples using CPTAC datasets from UALCAN database. *p < 0.05, **p < 0.01, ***p < 0.001.
Supplementary Figure S2 | DNA methylation level of KCNN4 in pan-cancer datasets obtained from the UALCAN database. (A–Q) Analysis of DNA methylation level of KCNN4 between pan-cancer and non-cancerous samples. *p < 0.05, **p < 0.01, ***p < 0.001.
Supplementary Figure S3 | Biomarker analysis of KCNN4 compared to other canonical biomarker signatures in immunotherapy-treated cancer cohorts based on the TIDE database.
Abdin, S., Zaher, D., Arafa, E.-S., and Omar, H. (2018). Tackling Cancer Resistance by Immunotherapy: Updated Clinical Impact and Safety of PD-1/pd-L1 Inhibitors. Cancers 10 (2), 32. doi:10.3390/cancers10020032
Abrams, Z. B., Johnson, T. S., Huang, K., Payne, P. R. O., and Coombes, K. (2019). A Protocol to Evaluate RNA Sequencing Normalization Methods. BMC Bioinforma. 20 (Suppl. 24), 679. doi:10.1186/s12859-019-3247-x
Andrews, L. P., Marciscano, A. E., Drake, C. G., and Vignali, D. A. A. (2017). LAG3 (CD223) as a Cancer Immunotherapy Target. Immunol. Rev. 276 (1), 80–96. doi:10.1111/imr.12519
Attali, D., and Baker, C. (2019). ggExtra: Add Marginal Histograms to ‘ggplot2’, and More ‘ggplot2’enhancements. R package version.
Baghban, R., Roshangar, L., Jahanban-Esfahlan, R., Seidi, K., Ebrahimi-Kalan, A., Jaymand, M., et al. (2020). Tumor Microenvironment Complexity and Therapeutic Implications at a Glance. Cell Commun. Signal 18 (1), 59. doi:10.1186/s12964-020-0530-4
Balar, A. V., and Weber, J. S. (2017). PD-1 and PD-L1 Antibodies in Cancer: Current Status and Future Directions. Cancer Immunol. Immunother. 66 (5), 551–564. doi:10.1007/s00262-017-1954-6
Biasiotta, A., D’Arcangelo, D., Passarelli, F., Nicodemi, E. M., and Facchiano, A. (2016). Ion Channels Expression and Function Are Strongly Modified in Solid Tumors and Vascular Malformations. J. Transl. Med. 14 (1), 285. doi:10.1186/s12967-016-1038-y
Billan, S., Kaidar-Person, O., and Gil, Z. (2020). Treatment after Progression in the Era of Immunotherapy. Lancet Oncol. 21 (10), e463–e476. doi:10.1016/s1470-2045(20)30328-4
Bray, F., Laversanne, M., Weiderpass, E., and Soerjomataram, I. (2021). The Ever‐increasing Importance of Cancer as a Leading Cause of Premature Death Worldwide. Cancer 127 (16), 3029–3030. doi:10.1002/cncr.33587
Brown, B. M., Pressley, B., and Wulff, H. (2018). KCa3.1 Channel Modulators as Potential Therapeutic Compounds for Glioblastoma. Curr. Neuropharmacol. 16 (5), 618–626. doi:10.2174/1570159x15666170630164226
Carrera, P. M., Kantarjian, H. M., and Blinder, V. S. (2018). The Financial Burden and Distress of Patients with Cancer: Understanding and Stepping-Up Action on the Financial Toxicity of Cancer Treatment. CA Cancer J. Clin. 68 (2), 153–165. doi:10.3322/caac.21443
Chan, T. A., Yarchoan, M., Jaffee, E., Swanton, C., Quezada, S. A., Stenzinger, A., et al. (2019). Development of Tumor Mutation Burden as an Immunotherapy Biomarker: Utility for the Oncology Clinic. Ann. Oncol. 30 (1), 44–56. doi:10.1093/annonc/mdy495
Chen, B., Khodadoust, M. S., Liu, C. L., Newman, A. M., and Alizadeh, A. A. (2018). Profiling Tumor Infiltrating Immune Cells with CIBERSORT. Methods Mol. Biol. 1711, 243–259. doi:10.1007/978-1-4939-7493-1_12
Chen, D. S., and Mellman, I. (2017). Elements of Cancer Immunity and the Cancer-Immune Set Point. Nature 541 (7637), 321–330. doi:10.1038/nature21349
Chen, D. S., and Mellman, I. (2013). Oncology Meets Immunology: the Cancer-Immunity Cycle. Immunity 39 (1), 1–10. doi:10.1016/j.immuni.2013.07.012
Chen, S., Wang, C., Su, X., Dai, X., Li, S., and Mo, Z. (2021). KCNN4 Is a Potential Prognostic Marker and Critical Factor Affecting the Immune Status of the Tumor Microenvironment in Kidney Renal Clear Cell Carcinoma. Transl. Androl. Urol. 10 (6), 2454–2470. doi:10.21037/tau-21-332
Chimote, A. A., Balajthy, A., Arnold, M. J., Newton, H. S., Hajdu, P., Qualtieri, J., et al. (2018). A Defect in KCa3.1 Channel Activity Limits the Ability of CD8+ T Cells from Cancer Patients to Infiltrate an Adenosine-Rich Microenvironment. Sci. Signal. 11 (527), eaaq1616. doi:10.1126/scisignal.aaq1616
Doroshow, D. B., Bhalla, S., Beasley, M. B., Sholl, L. M., Kerr, K. M., Gnjatic, S., et al. (2021). PD-L1 as a Biomarker of Response to Immune-Checkpoint Inhibitors. Nat. Rev. Clin. Oncol. 18 (6), 345–362. doi:10.1038/s41571-021-00473-5
Du, Y., Song, W., Chen, J., Chen, H., Xuan, Z., Zhao, L., et al. (2019). The Potassium Channel KCa3.1 Promotes Cell Proliferation by Activating SKP2 and Metastasis through the EMT Pathway in Hepatocellular Carcinoma. Int. J. Cancer 145 (2), 503–516. doi:10.1002/ijc.32121
Dudley, J. C., Lin, M.-T., Le, D. T., and Eshleman, J. R. (2016). Microsatellite Instability as a Biomarker for PD-1 Blockade. Clin. Cancer Res. 22 (4), 813–820. doi:10.1158/1078-0432.Ccr-15-1678
George Chandy, K., Wulff, H., Beeton, C., Pennington, M., Gutman, G. A., and Cahalan, M. D. (2004). K+ Channels as Targets for Specific Immunomodulation. Trends Pharmacol. Sci. 25 (5), 280–289. doi:10.1016/j.tips.2004.03.010
Goltz, D., Gevensleben, H., Vogt, T. J., Dietrich, J., Golletz, C., Bootz, F., et al. (2018). CTLA4 Methylation Predicts Response to Anti-PD-1 and Anti-CTLA-4 Immunotherapy in Melanoma Patients. JCI Insight 3 (13), e96793. doi:10.1172/jci.insight.96793
Gomes, A. P., Ilter, D., Low, V., Endress, J. E., Fernández-García, J., Rosenzweig, A., et al. (2020). Age-induced Accumulation of Methylmalonic Acid Promotes Tumour Progression. Nature 585 (7824), 283–287. doi:10.1038/s41586-020-2630-0
Gong, J., Chehrazi-Raffle, A., Reddi, S., and Salgia, R. (2018). Development of PD-1 and PD-L1 Inhibitors as a Form of Cancer Immunotherapy: a Comprehensive Review of Registration Trials and Future Considerations. J. Immunother. cancer 6 (1), 8. doi:10.1186/s40425-018-0316-z
Goswami, S., Sahai, E., Wyckoff, J. B., Cammer, M., Cox, D., Pixley, F. J., et al. (2005). Macrophages Promote the Invasion of Breast Carcinoma Cells via a Colony-Stimulating Factor-1/epidermal Growth Factor Paracrine Loop. Cancer Res. 65 (12), 5278–5283. doi:10.1158/0008-5472.Can-04-1853
Guo, Y., Yang, J., Ren, K., Tian, X., Gao, H., Tian, X., et al. (2022). The Heterogeneity of Immune Cell Infiltration Landscape and its Immunotherapeutic Implications in Hepatocellular Carcinoma. Front. Immunol. 13, 861525. doi:10.3389/fimmu.2022.861525
Hellmann, M. D., Ciuleanu, T.-E., Pluzanski, A., Lee, J. S., Otterson, G. A., Audigier-Valette, C., et al. (2018). Nivolumab Plus Ipilimumab in Lung Cancer with a High Tumor Mutational Burden. N. Engl. J. Med. 378 (22), 2093–2104. doi:10.1056/NEJMoa1801946
Hinshaw, D. C., and Shevde, L. A. (2019). The Tumor Microenvironment Innately Modulates Cancer Progression. Cancer Res. 79 (18), 4557–4566. doi:10.1158/0008-5472.Can-18-3962
Howard, D. H., Bach, P. B., Berndt, E. R., and Conti, R. M. (2015). Pricing in the Market for Anticancer Drugs. J. Econ. Perspect. 29 (1), 139–162. doi:10.1257/jep.29.1.139
Huang, C., Shen, S., Ma, Q., Chen, J., Gill, A., Pollock, C. A., et al. (2013). Blockade of KCa3.1 Ameliorates Renal Fibrosis through the TGF-β1/Smad Pathway in Diabetic Mice. Diabetes 62 (8), 2923–2934. doi:10.2337/db13-0135
Huynh, J., Patel, K., Gong, J., Cho, M., Malla, M., Parikh, A., et al. (2021). Immunotherapy in Gastroesophageal Cancers: Current Evidence and Ongoing Trials. Curr. Treat. Options Oncol. 22 (11), 100. doi:10.1007/s11864-021-00893-6
Jiang, P., Gu, S., Pan, D., Fu, J., Sahu, A., Hu, X., et al. (2018). Signatures of T Cell Dysfunction and Exclusion Predict Cancer Immunotherapy Response. Nat. Med. 24 (10), 1550–1558. doi:10.1038/s41591-018-0136-1
Kassambara, A., Kosinski, M., Biecek, P., and Fabian, S. (2018). Survminer: Drawing Survival Curves Using ‘ggplot2’. R package version 0.4. 3". Google Scholar).
Kaushik, I., Ramachandran, S., Zabel, C., Gaikwad, S., and Srivastava, S. K. (2022). The Evolutionary Legacy of Immune Checkpoint Inhibitors. Seminars Cancer Biol. doi:10.1016/j.semcancer.2022.03.020
Kelderman, S., Schumacher, T. N. M., and Haanen, J. B. A. G. (2014). Acquired and Intrinsic Resistance in Cancer Immunotherapy. Mol. Oncol. 8 (6), 1132–1139. doi:10.1016/j.molonc.2014.07.011
Kosuke, Y., Hoon, K., and Roel, G. (2021). V. Estimate: Estimate of Stromal and Immune Cells in Malignant Tumor Tissues from Expression Data. R package version 1.0. 13/r21; 2016.
Larkin, J., Chiarion-Sileni, V., Gonzalez, R., Grob, J. J., Cowey, C. L., Lao, C. D., et al. (2015). Combined Nivolumab and Ipilimumab or Monotherapy in Untreated Melanoma. N. Engl. J. Med. 373 (1), 23–34. doi:10.1056/NEJMoa1504030
Le, D. T., Durham, J. N., Smith, K. N., Wang, H., Bartlett, B. R., Aulakh, L. K., et al. (2017). Mismatch Repair Deficiency Predicts Response of Solid Tumors to PD-1 Blockade. Science 357 (6349), 409–413. doi:10.1126/science.aan6733
Le, D. T., Uram, J. N., Wang, H., Bartlett, B. R., Kemberling, H., Eyring, A. D., et al. (2015). PD-1 Blockade in Tumors with Mismatch-Repair Deficiency. N. Engl. J. Med. 372 (26), 2509–2520. doi:10.1056/NEJMoa1500596
Li, Q.-T., Feng, Y.-M., Ke, Z.-H., Qiu, M.-J., He, X.-X., Wang, M.-M., et al. (2020). KCNN4 Promotes Invasion and Metastasis through the MAPK/ERK Pathway in Hepatocellular Carcinoma. J. Investig. Med. 68 (1), 68–74. doi:10.1136/jim-2019-001073
Lin, P., Li, J., Ye, F., Fu, W., Hu, X., Shao, Z., et al. (2020). KCNN4 Induces Multiple Chemoresistance in Breast Cancer by Regulating BCL2A1. Am. J. Cancer Res. 10 (10), 3302–3315.
Luchini, C., Bibeau, F., Ligtenberg, M. J. L., Singh, N., Nottegar, A., Bosse, T., et al. (2019). ESMO Recommendations on Microsatellite Instability Testing for Immunotherapy in Cancer, and its Relationship with PD-1/pd-L1 Expression and Tumour Mutational Burden: a Systematic Review-Based Approach. Ann. Oncol. 30 (8), 1232–1243. doi:10.1093/annonc/mdz116
Max Gordon Lumley, T. (2020). Forestplot: Advanced Forest Plot Using ‘grid’Graphics. R Package Version 1.10. 1.
Michot, J. M., Bigenwald, C., Champiat, S., Collins, M., Carbonnel, F., Postel-Vinay, S., et al. (2016). Immune-related Adverse Events with Immune Checkpoint Blockade: a Comprehensive Review. Eur. J. Cancer 54, 139–148. doi:10.1016/j.ejca.2015.11.016
Mo, X., Zhang, C.-f., Xu, P., Ding, M., Ma, Z.-j., Sun, Q., et al. (2021). KCNN4-mediated Ca2+/MET/AKT axis Is Promising for Targeted Therapy of Pancreatic Ductal Adenocarcinoma. Acta Pharmacol. Sin. 43, 735–746. doi:10.1038/s41401-021-00688-3
Mo, X., Zhang, H., Preston, S., Martin, K., Zhou, B., Vadalia, N., et al. (2018). Interferon-γ Signaling in Melanocytes and Melanoma Cells Regulates Expression of CTLA-4. Cancer Res. 78 (2), 436–450. doi:10.1158/0008-5472.Can-17-1615
Mohr, C. J., Gross, D., Sezgin, E. C., Steudel, F. A., Ruth, P., Huber, S. M., et al. (2019). KCa3.1 Channels Confer Radioresistance to Breast Cancer Cells. Cancers 11 (9), 1285. doi:10.3390/cancers11091285
Nakasone, E. S., Askautrud, H. A., Kees, T., Park, J.-H., Plaks, V., Ewald, A. J., et al. (2012). Imaging Tumor-Stroma Interactions during Chemotherapy Reveals Contributions of the Microenvironment to Resistance. Cancer Cell 21 (4), 488–503. doi:10.1016/j.ccr.2012.02.017
Nakazawa, M. (2018). Fmsb: Functions for Medical Statistics Book with Some Demographic Data. R package version 0.6 3(3).
Nardi, E. A., Wolfson, J. A., Rosen, S. T., Diasio, R. B., Gerson, S. L., Parker, B. A., et al. (2016). Value, Access, and Cost of Cancer Care Delivery at Academic Cancer Centers. J. Natl. Compr. Canc Netw. 14 (7), 837–847. doi:10.6004/jnccn.2016.0088
Necchi, A., Raggi, D., Gallina, A., Madison, R., Colecchia, M., Lucianò, R., et al. (2020). Updated Results of PURE-01 with Preliminary Activity of Neoadjuvant Pembrolizumab in Patients with Muscle-Invasive Bladder Carcinoma with Variant Histologies. Eur. Urol. 77 (4), 439–446. doi:10.1016/j.eururo.2019.10.026
Odiase, O., Noah-Vermillion, L., Simone, B. A., and Aridgides, P. D. (2021). The Incorporation of Immunotherapy and Targeted Therapy into Chemoradiation for Cervical Cancer: A Focused Review. Front. Oncol. 11, 663749. doi:10.3389/fonc.2021.663749
Paraiso, K. H. T., and Smalley, K. S. M. (2013). Fibroblast-mediated Drug Resistance in Cancer. Biochem. Pharmacol. 85 (8), 1033–1041. doi:10.1016/j.bcp.2013.01.018
Petrelli, F., Ghidini, M., Ghidini, A., and Tomasello, G. (2020). Outcomes Following Immune Checkpoint Inhibitor Treatment of Patients with Microsatellite Instability-High Cancers. JAMA Oncol. 6 (7), 1068–1071. doi:10.1001/jamaoncol.2020.1046
Qin, S., Xu, L., Yi, M., Yu, S., Wu, K., and Luo, S. (2019). Novel Immune Checkpoint Targets: Moving beyond PD-1 and CTLA-4. Mol. Cancer 18 (1), 155. doi:10.1186/s12943-019-1091-2
Räsänen, K., and Vaheri, A. (2010). Activation of Fibroblasts in Cancer Stroma. Exp. Cell Res. 316 (17), 2713–2722. doi:10.1016/j.yexcr.2010.04.032
Ritchie, M. E., Phipson, B., Wu, D., Hu, Y., Law, C. W., Shi, W., et al. (2015). Limma Powers Differential Expression Analyses for RNA-Sequencing and Microarray Studies. Nucleic acids Res. 43 (7), e47. doi:10.1093/nar/gkv007
Roviello, G., Catalano, M., Santi, R., Palmieri, V. E., Vannini, G., Galli, I. C., et al. (2021). Immune Checkpoint Inhibitors in Urothelial Bladder Cancer: State of the Art and Future Perspectives. Cancers 13 (17), 4411. doi:10.3390/cancers13174411
Savage, P., Mahmoud, S., Patel, Y., and Kantarjian, H. (2017). Cancer Drugs: An International Comparison of Postlicensing Price Inflation. J. Oncol. Pract. 13 (6), e538–e542. doi:10.1200/jop.2016.014431
Simms, L. A., Doecke, J. D., Roberts, R. L., Fowler, E. V., Zhao, Z. Z., McGuckin, M. A., et al. (2010). KCNN4 Gene Variant Is Associated with Ileal Crohn's Disease in the Australian and New Zealand Population. Am. J. Gastroenterol. 105 (10), 2209–2217. doi:10.1038/ajg.2010.161
Sung, H., Ferlay, J., Siegel, R. L., Laversanne, M., Soerjomataram, I., Jemal, A., et al. (2021). Global Cancer Statistics 2020: GLOBOCAN Estimates of Incidence and Mortality Worldwide for 36 Cancers in 185 Countries. CA A Cancer J. Clin. 71 (3), 209–249. doi:10.3322/caac.21660
Taube, J. M., Klein, A., Brahmer, J. R., Xu, H., Pan, X., Kim, J. H., et al. (2014). Association of PD-1, PD-1 Ligands, and Other Features of the Tumor Immune Microenvironment with Response to Anti-PD-1 Therapy. Clin. Cancer Res. 20 (19), 5064–5074. doi:10.1158/1078-0432.Ccr-13-3271
Topalian, S. L., Hodi, F. S., Brahmer, J. R., Gettinger, S. N., Smith, D. C., McDermott, D. F., et al. (2012). Safety, Activity, and Immune Correlates of Anti-PD-1 Antibody in Cancer. N. Engl. J. Med. 366 (26), 2443–2454. doi:10.1056/NEJMoa1200690
Vilar, E., and Gruber, S. B. (2010). Microsatellite Instability in Colorectal Cancer-The Stable Evidence. Nat. Rev. Clin. Oncol. 7 (3), 153–162. doi:10.1038/nrclinonc.2009.237
Wagner, G. P., Kin, K., and Lynch, V. J. (2012). Measurement of mRNA Abundance Using RNA-Seq Data: RPKM Measure Is Inconsistent Among Samples. Theory Biosci. 131 (4), 281–285. doi:10.1007/s12064-012-0162-3
Wang, F., Wei, X. L., Wang, F. H., Xu, N., Shen, L., Dai, G. H., et al. (2019). Safety, Efficacy and Tumor Mutational Burden as a Biomarker of Overall Survival Benefit in Chemo-Refractory Gastric Cancer Treated with Toripalimab, a PD-1 Antibody in Phase Ib/II Clinical Trial NCT02915432. Ann. Oncol. 30 (9), 1479–1486. doi:10.1093/annonc/mdz197
Wen, J., Lin, B., Lin, L., Chen, Y., and Wang, O. (2020). KCNN4 Is a Diagnostic and Prognostic Biomarker that Promotes Papillary Thyroid Cancer Progression. Aging 12 (16), 16437–16456. doi:10.18632/aging.103710
Wickham, H. (2007). Reshaping Data with the Reshape Package. J. Stat. Softw. 21, 1–20. doi:10.18637/jss.v021.i12
Wu, T., and Dai, Y. (2017). Tumor Microenvironment and Therapeutic Response. Cancer Lett. 387, 61–68. doi:10.1016/j.canlet.2016.01.043
Xu, H., Lai, W., Zhang, Y., Liu, L., Luo, X., Zeng, Y., et al. (2014). Tumor-associated Macrophage-Derived IL-6 and IL-8 Enhance Invasive Activity of LoVo Cells Induced by PRL-3 in a KCNN4 Channel-dependent Manner. BMC Cancer 14, 330. doi:10.1186/1471-2407-14-330
Yarchoan, M., Hopkins, A., and Jaffee, E. M. (2017). Tumor Mutational Burden and Response Rate to PD-1 Inhibition. N. Engl. J. Med. 377 (25), 2500–2501. doi:10.1056/NEJMc1713444
Yoshihara, K., Shahmoradgoli, M., Martínez, E., Vegesna, R., Kim, H., Torres-Garcia, W., et al. (2013). Inferring Tumour Purity and Stromal and Immune Cell Admixture from Expression Data. Nat. Commun. 4 (1), 2612. doi:10.1038/ncomms3612
Yu, G., Wang, L.-G., Han, Y., and He, Q.-Y. (2012). clusterProfiler: an R Package for Comparing Biological Themes Among Gene Clusters. Omics a J. Integr. Biol. 16 (5), 284–287. doi:10.1089/omi.2011.0118
Yu, Z., Yi, M., Wei, T., Gao, X., and Chen, H. (2017). KCa3.1 Inhibition Switches the Astrocyte Phenotype during Astrogliosis Associated with Ischemic Stroke via Endoplasmic Reticulum Stress and MAPK Signaling Pathways. Front. Cell. Neurosci. 11, 319. doi:10.3389/fncel.2017.00319
Keywords: KCNN4, pan-cancer, tumor microenvironment, biomarker, tumor-infiltrating immune cells
Citation: Chen S, Su X and Mo Z (2022) KCNN4 is a Potential Biomarker for Predicting Cancer Prognosis and an Essential Molecule that Remodels Various Components in the Tumor Microenvironment: A Pan-Cancer Study. Front. Mol. Biosci. 9:812815. doi: 10.3389/fmolb.2022.812815
Received: 10 November 2021; Accepted: 02 May 2022;
Published: 03 June 2022.
Edited by:
Patrick P. Boor, Erasmus Medical Center, NetherlandsReviewed by:
Robert Ancuceanu, Carol Davila University of Medicine and Pharmacy, RomaniaCopyright © 2022 Chen, Su and Mo. This is an open-access article distributed under the terms of the Creative Commons Attribution License (CC BY). The use, distribution or reproduction in other forums is permitted, provided the original author(s) and the copyright owner(s) are credited and that the original publication in this journal is cited, in accordance with accepted academic practice. No use, distribution or reproduction is permitted which does not comply with these terms.
*Correspondence: Zengnan Mo, bW96ZW5nbmFuQGd4bXUuZWR1LmNu
†These authors have contributed equally to this work and share first authorship
Disclaimer: All claims expressed in this article are solely those of the authors and do not necessarily represent those of their affiliated organizations, or those of the publisher, the editors and the reviewers. Any product that may be evaluated in this article or claim that may be made by its manufacturer is not guaranteed or endorsed by the publisher.
Research integrity at Frontiers
Learn more about the work of our research integrity team to safeguard the quality of each article we publish.