Corrigendum: Comparing Antibody Interfaces to Inform Rational Design of New Antibody Formats
- 1Department of General, Inorganic and Theoretical Chemistry, Center for Molecular Biosciences Innsbruck (CMBI), University of Innsbruck, Innsbruck, Austria
- 2Roche Pharma Research and Early Development, Large Molecule Research, Roche Innovation Center Munich, Penzberg, Germany
As the current biotherapeutic market is dominated by antibodies, the design of different antibody formats, like bispecific antibodies and other new formats, represent a key component in advancing antibody therapy. When designing new formats, a targeted modulation of pairing preferences is key. Several existing approaches are successful, but expanding the repertoire of design possibilities would be desirable. Cognate immunoglobulin G antibodies depend on homodimerization of the fragment crystallizable regions of two identical heavy chains. By modifying the dimeric interface of the third constant domain (CH3-CH3), with different mutations on each domain, the engineered Fc fragments form rather heterodimers than homodimers. The first constant domain (CH1-CL) shares a very similar fold and interdomain orientation with the CH3-CH3 dimer. Thus, numerous well-established design efforts for CH3-CH3 interfaces, have also been applied to CH1-CL dimers to reduce the number of mispairings in the Fabs. Given the high structural similarity of the CH3-CH3 and CH1-CL domains we want to identify additional opportunities in comparing the differences and overlapping interaction profiles. Our vision is to facilitate a toolkit that allows for the interchangeable usage of different design tools from crosslinking the knowledge between these two interface types. As a starting point, here, we use classical molecular dynamics simulations to identify differences of the CH3-CH3 and CH1-CL interfaces and already find unexpected features of these interfaces shedding new light on possible design variations. Apart from identifying clear differences between the similar CH3-CH3 and CH1-CL dimers, we structurally characterize the effects of point-mutations in the CH3-CH3 interface on the respective dynamics and interface interaction patterns. Thus, this study has broad implications in the field of antibody engineering as it provides a structural and mechanistical understanding of antibody interfaces and thereby presents a crucial aspect for the design of bispecific antibodies.
Introduction
Antibodies play a central role in the adaptive immune system, as they can recognize and neutralize foreign antigens (Chiu et al., 2019). In the last years, antibodies emerged as a new class of pharmaceuticals (Kaplon et al., 2020; Kaplon and Reichert, 2021), with over one hundred antibody-based drugs being marketed or pending approval.
Structurally, antibodies consist of two heavy and two light chains and have a unique modular anatomy facilitating their engineering and design (Davies and Chacko, 1993). The immunoglobulin heavy and light chains are composed of various discrete protein domains. Especially interesting is that these domains all have a similar folded structure, which is known as the immunoglobulin fold (Chiu et al., 2019). However, even though they share a similar fold, there are distinct structural differences between these domains (Figure 1). In general, antibodies can be divided into a crystallizable fragment (Fc) and two identical antigen-binding fragments (Fabs). The Fab can further be subdivided into constant (CH1-CL) and variable (VH-VL) domains (Davies and Chacko, 1993; Röthlisberger et al., 2005). The variable domains of the heavy and the light chain (VH and VL) shape the antigen binding site and are responsible for antigen binding and recognition (Colman and Dixon, 1988; Addis et al., 2014; Fernández-Quintero et al., 2020c). The variable and the constant domains in the Fab are linked via a so-called switch region (Stanfield et al., 2006). The CH1-CL heterodimer plays an essential role for antibody assembly and secretion in the cell (Adachi et al., 2003). Comparison of the VH-VL and the CH1-CL heterodimers revealed that the CH1-CL heterodimer is more stable than the VH–VL heterodimer (Röthlisberger et al., 2005). The individual CH1 domain is not stable in folded form and requires interactions with either the chaperone BiP or the CL domain for folded state stability (Vanhove et al., 2001; Feige et al., 2014). The crystallizable fragment is composed of a CH2-CH2 and a CH3-CH3 homodimer (Teplyakov et al., 2013). The CH2-CH2 domain has no direct protein interactions in the interface as the interface is formed by glycans (Teplyakov et al., 2013). Thus, the CH2-CH2 domain differs from all other domains and consequently will not be discussed in this manuscript. The CH3 domains bind tightly with each other by hydrophobic interactions at the center, surrounded by salt bridges and thereby forming the foundation for the heavy chain dimer association (Teplyakov et al., 2013). Mutations in the CH3-CH3 interface have been shown to strongly influence the stability and the association of the two domains (Rose et al., 2013).
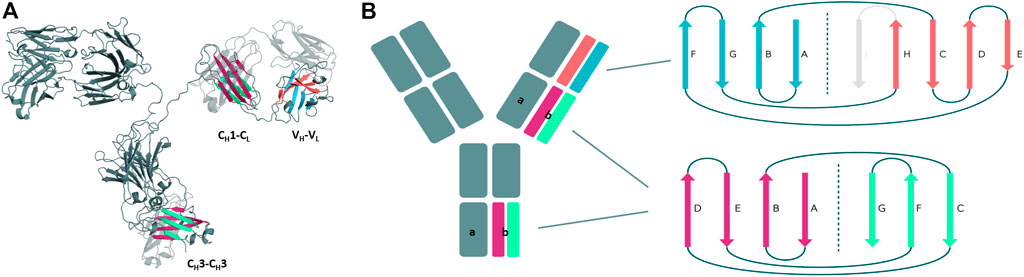
FIGURE 1. Structural and schematic representation of the modular anatomy of an antibody focusing on the discrete protein domains, which are characterized by the immunoglobulin fold. (A) Crystal structure of a whole IgG2 antibody (PDB: 1IGT) highlighting the different interface classes and their respective domain architecture. (B) Schematic depiction of the tertiary structure features, i.e., number and organization of the β-strands, for each of the individual antibody domains sharing the immunoglobulin fold. The CH3 and CL/CH1 domains do not only share a similar structure and topology but also contain the same number and arrangement of β-strands. The variable domains on the other hand differ in their number of β-strands and their architecture and are therefore color-coded differently.
The concept of having an antibody with two different antigen binding sites was established more than 50 years ago by Nisonoff and co-workers and evolved alongside numerous advances and technical innovations in the field of antibody engineering, leading to more than 100 bispecific antibody (bsAb) formats known up to now (Nisonoff and Rivers, 1961; Fudenberg et al., 1964). BsAb formats expand the functionality of traditional antibodies by their ability to target effector cells to kill tumor cells, to enhance tissue specificity or to combine the antigen binding of two antibodies in a single molecule to simultaneously target two signaling pathways (Brinkmann and Kontermann, 2017; Sedykh et al., 2018). BsAbs can be assembled from different heavy and light chains. To suppress random assembly of different chains, resulting in various non-desired molecules, engineering efforts are required (Bönisch et al., 2017). A major breakthrough in the development of bsAb formats was the invention of the knobs-into-holes (KiH) technology for CH3-CH3 interfaces (Ridgway et al., 1996; Elliott et al., 2014). Precisely, advances like the KiH technology for CH3-CH3 interfaces represented a novel and effective design strategy for engineering heavy chain homodimers towards heterodimers, to reduce the risk of random assembly of different chains (Ridgway et al., 1996; Elliott et al., 2014; Kuglstatter et al., 2017). Thus, the idea of modifying the interfaces has motivated numerous studies to find variations of this approach by following a number of different strategies, such as alterations of the charge polarity in the interfaces compared to the homodimer, e.g., inverted charge interactions (DE-KK and DD-KK variants) (Ha et al., 2016; Moore et al., 2019). More recently, also KiH mutations in combination with charge inversions have been introduced into both Fab interfaces, CH1-CL and VH-VL, enforcing the correct pairings of light chains with the corresponding heavy chains (Bönisch et al., 2017; Dillon et al., 2017; Regula et al., 2018).
In this study, we use classical molecular dynamics simulations to provide a systematic and extensive comparison of different antibody interfaces, which are in the spotlight of antibody engineering as they offer numerous design opportunities for bispecific antibody formats (Brinkmann and Kontermann, 2017; Sedykh et al., 2018). As CH1-CL dimers are inherently heterodimers, we compare them with the homo-and-heterodimeric CH3-CH3 domains. We aim to identify different and overlapping interaction profiles of either the CH3-CH3 or CH1-CL interfaces with the intention to crosslink the knowledge covering the two interfaces (Jost Lopez et al., 2020). Apart from that, we compare the interface flexibilities of CH3-CH3 or CH1-CL domains and provide key determinants that contribute to the stability and their tendency to heterodimerize.
The investigated CH3-CH3 and CH1-CL dimers and their respective PDB accession codes are summarized in Supplementary Table S1, covering a variety of different design strategies to enforce the formation of heterodimers. The Fabs to study the CH1-CL dimers were chosen based on their availability of experimentally determined structure and stability data and their light chain isotypes. We also included in our dataset three antibody Fabs with mutations in the CH1-CL, which facilitate selective Fab assembly in combination with previously described KiH mutations for preferential heavy chain heterodimerization.
Results
Structural Architecture of the Investigated Antibody Interfaces
First, we introduce and structurally characterize different antibody interfaces and their respective architectures (Figure 1). All investigated antibody interfaces (23 Fab fragments and 23 CH3-CH3 domains), summarized in Supplementary Table S1, have been simulated for 1 µs with classical molecular dynamics simulations (extracting 10,000 frames) in explicit solvation to better understand and capture the variability of these interfaces. Figure 1 shows the comparison of the dimeric antibody interfaces in the antigen-binding fragment (VH-VL and CH1-CL domains) and in the third constant domain (CH3-CH3 domain). All of the presented interfaces share the same immunoglobulin fold, which is characterised by hydrogen bond interactions between the different β-strands. Additionally, we find that the CH1-CL and CH3-CH3 domains have actually the same number of β-strands, i.e., a 3-stranded sheet packed against a 4-stranded sheet. Also, the relative orientation of the two monomers with respect to each other (approximately 90° observed in X-ray structures) is nearly identical between the CH3-CH3 and CH1-CL dimers. Thus, the CH3-CH3 and CH1-CL dimers share a very similar structure and fold. However, we observe structural differences in the overall architecture between the CH1-CL/CH3-CH3 and the VH-VL domains, as the VH-VL domains differ in their number of strands (9 β-strands arranged in two sheets of 4 and 5 strands), and in their relative orientation between the VH and VL monomers with respect to each other (approximately 50° observed in X-ray structures).
Relative Interdomain Orientations of CH1-CL and CH3-CH3 Domains
Apart from understanding the structural architecture, the dimeric interfaces are strongly influenced by the relative interdomain orientation and their respective dynamics. To calculate the interface movements, we used the well-established ABangle tool (Dunbar et al., 2013) and a recently presented python tool, called OCD tool (Hoerschinger et al., 2021), which both allow to calculate the interface orientations of different immunoglobulin like domains by defining five angles (one torsion angle (HL/AB)/four tilt angles (LC1, LC2, HC1, HC2/AC1, AC2, BC1, BC2) and one distance (dC). For the CH1-CL domains we added the prefix c to the angle names (cHL, cLC1, cLC2, cHC1, cHC2, dC), as the CH1-CL dimer forms the constant domain of the Fab fragment and to be able to distinguish them from the variable fragment (Fv) nomenclature. The detailed definition of these angles is presented in the methods section. Figure 2A shows a superimposition of the two dimers (CH1-CL and CH3-CH3), highlighting the high structural similarity of the β-strands, while the loops on the other hand differ between the two dimers (Cα-RMSD 1.8Å). Figure 2B depicts the interdomain angle distributions of the relative interdomain orientations for all investigated CH1-CL and CH3-CH3 simulations and shows significant overlaps in the interface angle (cHL/AB) distributions. However, the CH1-CL shows a higher variability in the interface angle, which is reflected in broader angle distributions, compared to the CH3-CH3 dimer. Apart from the higher flexibility in the interdomain angle, we also find shifted CH1-CL distributions towards lower cHL-Angle values. The torsion angle (cHL) of all CH1-CL domains ranges from 65°–110°, while the torsion angle (AB angle) of all CH3-CH3 ranges from 85–125° (cHL angle, AB angle). The biggest difference in the relative interdomain orientations can be observed for the Fv torsion angles (HL angle), which range from 35°–80° (Supplementary Figure S1).
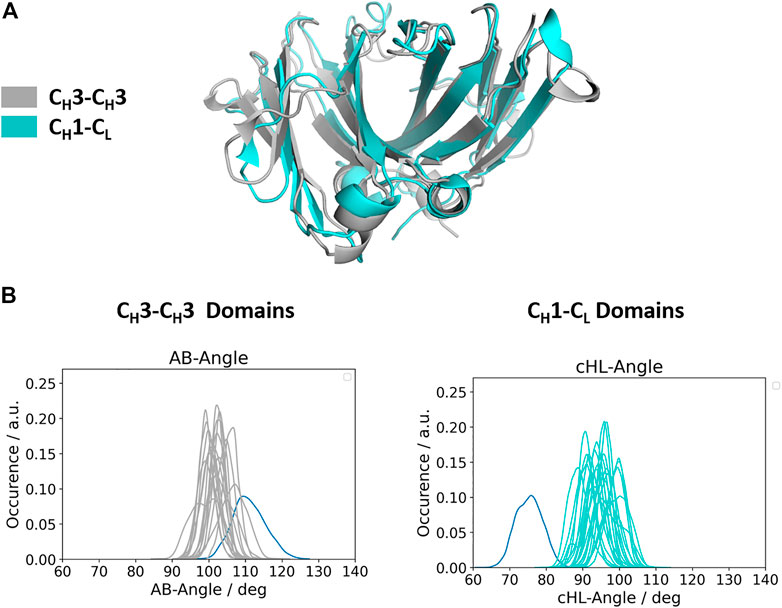
FIGURE 2. Comparison of the structurally highly similar CH3-CH3 and CH1-CL domains. (A) Structural overlay of a CH3-CH3 (grey, PDB: 3AVE) and a CH1-CL (cyan, PDB: 5I19) dimer illustrating their identical scaffold, despite having diverging loop structures. (B) Distributions of interdomain angles for all CH3-CH3 and CH1-CL domains, respectively. These angles haven been calculated with the recently published OCD tool and show that the CH3-CH3 interfaces cover narrower angle ranges, while the CH1-CL, due to their higher number of sequence variations, reveal a larger spread. The highlighted distributions shown in blue correspond to the CH3-CH3 heterodimeric DE-KK variant (PDB: 5NSC) and to the λ-light chain antibody (PDB: 1NL0).
Structural Characterization of the CH1-CL and CH3-CH3 Interfaces
To structurally characterize interactions in the CH1-CL and CH3-CH3 interfaces, we use the GetContacts tool (Stanford University, adate), which calculates the interface contacts in a time-resolved way and depicts them with so-called flareplots (https://getcontacts.github.io/). To better visualize the comparison between the two interfaces we grouped the residues belonging to the same loops and β-strands to obtain coarse grained flareplots. This coarse-grained representation of the CH1-CL and CH3-CH3 interfaces also allows having a better overview about the regions of these interfaces that actually form key interactions, which contribute to their structural integrity and to their stability. The β-strands are labelled with single letters, while the loops are tagged with a two-letter combination of the respective β-strands before and after the loop. To ease the comparison between CH1-CL and CH3-CH3 we refer both to the CL domain and one of the CH3 domains (the domain A) as “a” and to the CH1 domain and to the other CH3 domain (the domain B) as “b.” The thickness of the lines in the flareplots corresponds to the occurrence of a contact (ratio) over the whole simulation time (10,000 frames/simulation). A representative CH1-CL and CH3-CH3 structure color-coded and labeled according to the flareplots (right) is depicted in Figure 3. The coarse-grained flareplots presented in Figure 3 show all interdomain contact patterns for both the CH1-CL and CH3-CH3 interface. While the CH1-CL and CH3-CH3 domains share common interaction patterns, we also investigated the type of interactions contributing to the formation of the respective interface. The flareplots shown in Supplementary Figure S2, Figures 4, 5 are just exemplary plots. The barplots on the right quantitatively summarize and compare the contacts observed for all investigated CH3-CH3 and CH1-CL domains. Supplementary Figure S2 illustrates representative coarse-grained flareplots showing the interdomain hydrogen bond interactions of both the CH1-CL and CH3-CH3 domains. While we find overlaps in the hydrogen bond interaction patterns for the CH3-CH3 and CH1-CL interfaces (Supplementary Figures S2A,B), they differ substantially in number and occurrence of interdomain hydrogen bonds between CH1-CL and CH3-CH3 domains, i.e., the CH3-CH3 domains form significantly more hydrogen bonds between the a_E – b_DE, a_DE – b_E, a_A – b_AB, a_B – b_E, a_B – b_B and a_G – b_AB loops/strands (Supplementary Figure S2).
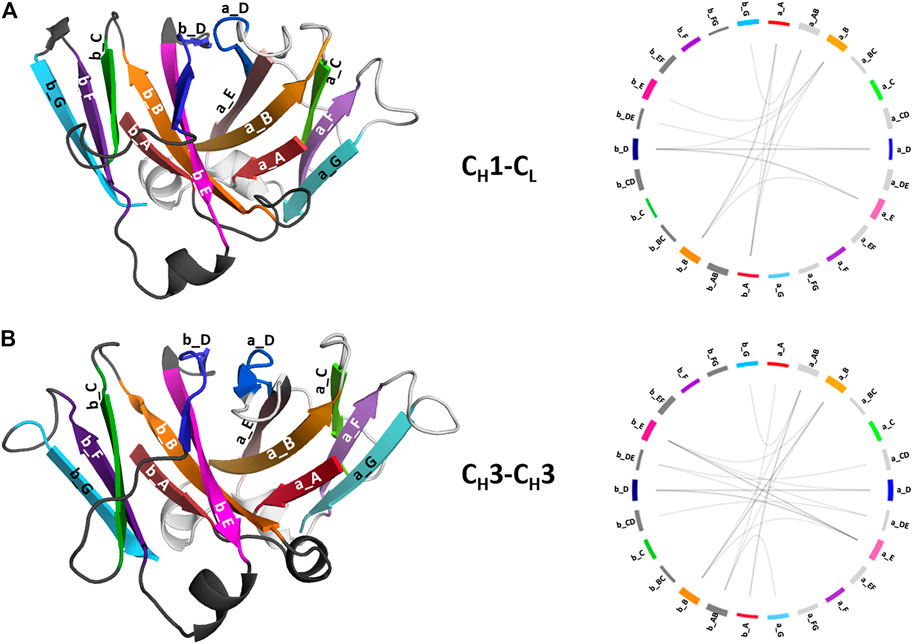
FIGURE 3. Structural representation of the CH3-CH3 and CH1-CL domains including a coarse-grained contact analysis of the interactions contributing to the formation and stabilization of the domain interfaces. (A) Structure of CH1-CL heterodimer (PDB: 5I17) color-coded and labeled according to the coarse-grained flareplots on the right showing the interdomain interactions present in the X-ray structure. We coarse grained residues belonging to the same loops or β-strands. (B) Structure of CH3-CH3 (PDB: 5DJ0) dimer color-coded and labeled according to the coarse-grained flareplots on the right, which illustrate the interdomain contacts present in the X-ray structure.
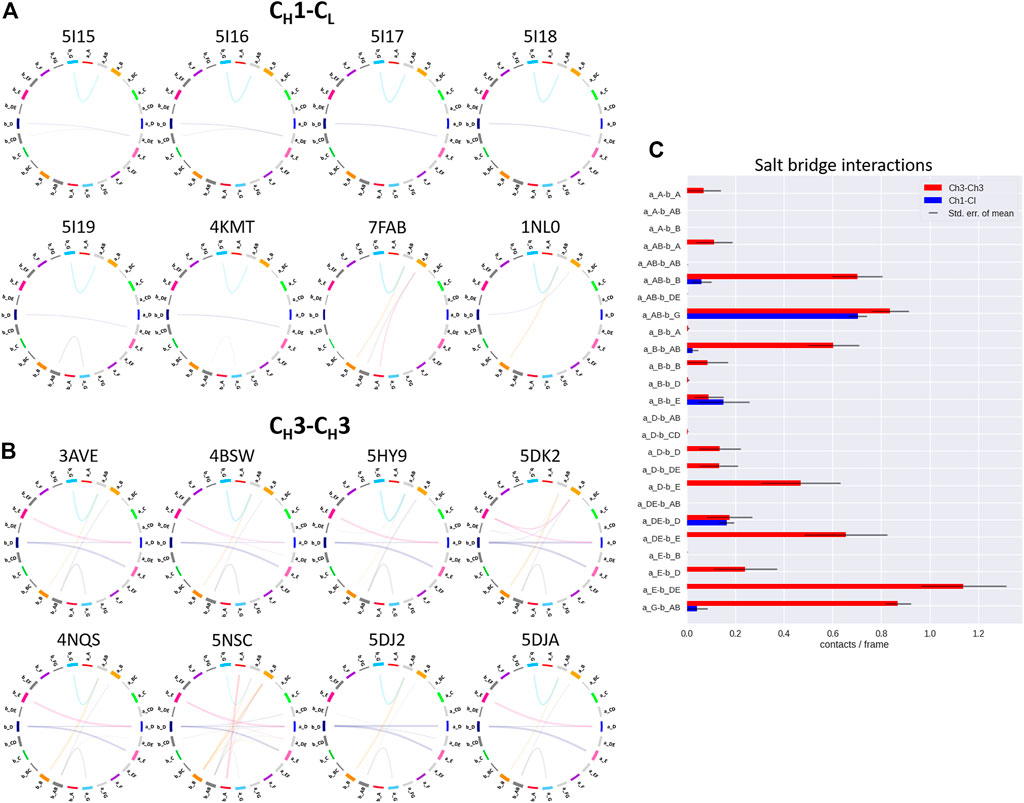
FIGURE 4. Exemplary coarse-grained flareplots showing the salt bridge interactions formed between the different interdomain β-strands and loops of both (A) CH1-CL and (B) CH3-CH3 domains. (C) Bar plots quantitatively depicting differences in per strand/loop salt bridge interactions. We compare the two interface classes, i.e., CH1-CL (blue) and CH3-CH3 (red). Thus, we show averages and standard errors of the mean of all investigated antibodies within the respective class.
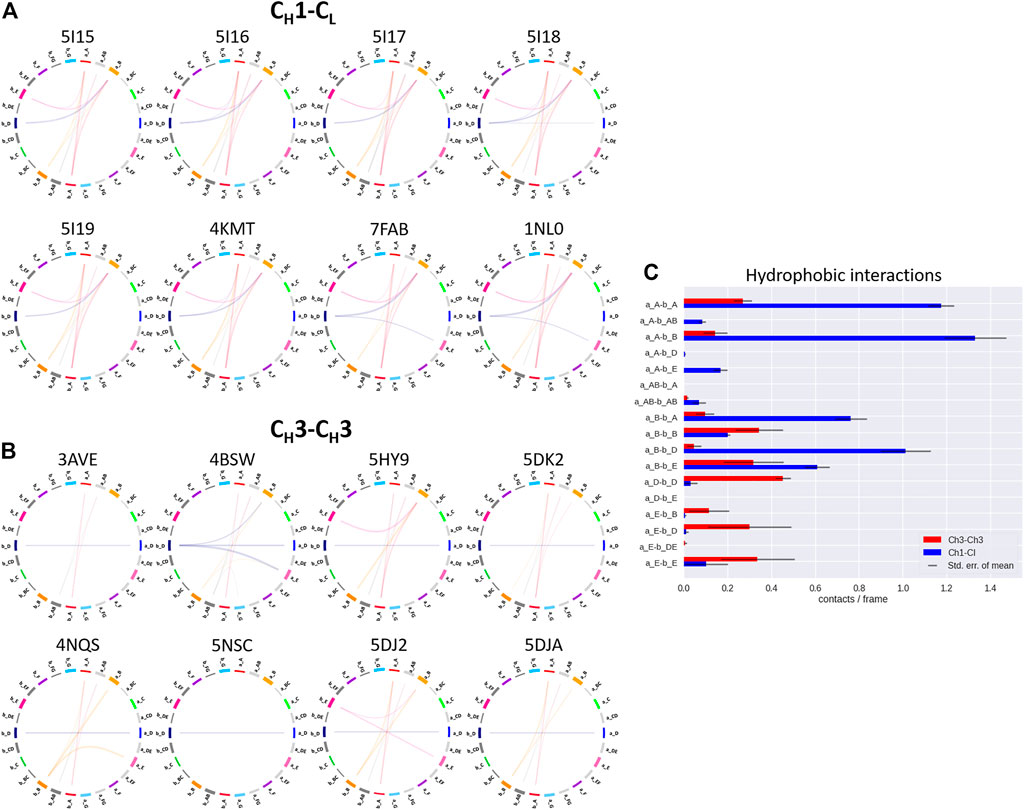
FIGURE 5. Exemplary coarse-grained flareplots showing the hydrophobic interactions formed between the different interdomain β-strands and loops of both (A) CH1-CL and (B) CH3-CH3 domains. (C) Bar plots quantitatively depicting differences in per strand/loop hydrophobic interactions. We compare the two interface classes, i.e., CH1-CL (blue) and CH3-CH3 (red). Thus, we show averages and standard errors of the mean of all investigated antibodies within the respective class.
In line with these observations, we find that the CH3-CH3 interfaces are strongly stabilized by salt bridges (Figure 4), while the CH1-CL interfaces reveal substantially more hydrophobic interactions (Figure 5). Long-lasting salt bridge interactions (>60% of the simulation time) in the CH3-CH3 interfaces are formed by the a_E – b_DE, a_DE – b_E, a_D – b_E, a_B – b_AB, a_AB – b_B and a_G – b_AB loops/strands. Salt bridges between the a_AB – b_G and a_DE – b_D loops/strands are present in both CH1-CL and CH3-CH3 domains (Figure 4). While the CH3-CH3 domains are characterized by a substantially higher number of charged interactions, the CH1-CL domains are stabilized by hydrophobic interactions between the a_B – b_D, a_B – b_A, a_A – b_A, a_B – b_E and a_A – b_B strands. Even though the CH3-CH3 interface is strongly stabilized by salt bridge interactions, the hydrophobic interactions between the a_E – b_B, a_D – b_D, a_E – b_E and a_E – b_D strands (Figure 5C) are characteristic for the CH3-CH3 domains, compared to the CH1-CL domains.
Moreover, we find interdomain van der Waals interaction patterns that are present in both the CH1-CL and CH3-CH3 domains, e.g., interactions between a_D – b_D strands (Supplementary Figure S3). However, also substantial differences between CH1-CL and CH3-CH3 domains can be identified for the interdomain van der Waals interactions, such as the interactions between the a_E – b_E strands and the a_A – b_AB strand/loop, which are dominantly present in CH3-CH3 domains and the a_E – b_D and a_A – b_B strands, which can be found more in CH1-CL domains. Figure 6 illustrates contact maps depicting differences in the number and duration of hydrogen bond, salt bridge and hydrophobic interactions for all investigated antibody fragments. The color bar is normalized according to the most frequent contacts in either of the two interface classes.
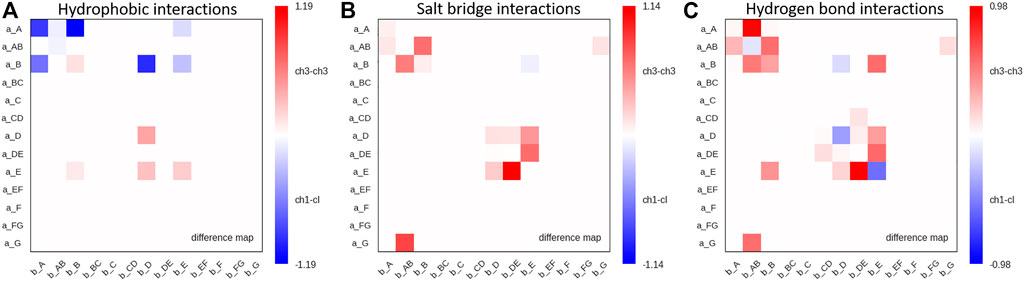
FIGURE 6. Maps depicting the differences in hydrophobic interactions, salt bridges and hydrogen bonds between CH3-CH3 and CH1-CL domains. (A) Difference in hydrophobic interactions between all investigated CH3-CH3 and CH1-CL domains (Supplementary Table S1) based on the previously defined coarse graining of the residues belonging to the same loops or β-strands. We normalized the colorbar according to the most frequent contact in either of the two interface classes. (B) Difference in salt bridge interactions between all investigated CH3-CH3 and CH1-CL interfaces, showing the substantially higher number of salt bridge interactions dominating the CH3-CH3 interface. (C) Hydrogen bond difference maps for all investigated CH3-CH3 and CH1-CL interfaces.
Thus, Figure 6 summarizes the findings shown in Figures 4, 5 and Supplementary Figure S2, as it clearly displays the substantially higher number of hydrogen bond and salt bridge interactions for the CH3-CH3 domains, while the CH1-CL interface is dominated by hydrophobic interactions. To quantify this difference even more, we calculated the electrostatic interface interaction energies for all investigated CH1-CL and CH3-CH3 dimers (Supplementary Table S2). The strong difference in the type of interactions between the CH1-CL and CH3-CH3 are even more pronounced in the electrostatic interface interaction energies, where we find significantly higher electrostatic interaction energies for the CH3-CH3 dimer, compared to the CH1-CL domains.
Supplementary Figure S4 shows the comparison of three CH3-CH3 domains (Dengl et al., 2020) with three engineered CH1-CL interfaces (Dillon et al., 2017), which were designed following similar heterodimerization strategies. The goal of redesigning the CH1/CL interface was to reduce mispairings by having a stably paired CH1-CL interface due to mutations that create incompatibilities towards the binding of wildtype CH1 or CL domains (Dillon et al., 2017). Apart from inserting KiH mutations, the interface was redesigned by introducing charge mutations, which co-determine orthogonal heavy and light chain pairing preferences. The first two presented CH1-CL domains (Supplementary Figures S4A,B) have newly introduced charge pairs and are therefore described as KE (CH1 S183K interacts with CL V133E) and EK (CH1 S183E interacts with CL V133K) variants (PDB accession codes: 5TDN and 5TDO, respectively). The third CH1-CL interface (Supplementary Figure S4C) contains mutations at the edge of the interface at position CL F116A and CH1 S181M, which introduce more flexibility. Additionally, KiH modifications are introduced at position CH1 F170S and CL S176F (PDB accession code: 5TDP). Supplementary Figures S4A,B shows strong hydrogen bond networks for the KE and EK variants, especially between the a_E – b_E and a_B – b_E strands. Additionally, also strong salt bridge interactions can be observed for both the KE and EK variants between a_B – b_E strands, which cannot be observed in the third variant (Supplementary Figure S4C). Differences can also be observed in the hydrophobic contacts between the three engineered CH1-CL variants. While hydrophobic contacts between a_A – b_A and a_A – b_B are present in all three variants, the third variant has long-lasting contacts between the a_E – b_E strands (Supplementary Figure S4C). Additionally, the two charge optimized CH1-CL domains make strong hydrophobic interactions between the a_B – b_D and a_B – b_E strands (Supplementary Figures S4A,B). Comparing CH1-CL variants with CH3-CH3 domains, we find that the EK and KE CH1-CL variants (Supplementary Figures S4A,B) are able to form salt bridges between the a_B – b_E strands, which we only identified in CH3-CH3 domains before and not in other investigated CH1-CL domains. The hydrophobic interactions of the KiH designed CH1-CL domain (Supplementary Figure S4C) also show CH3-CH3 specific interactions between a_E – b_E strands, while the EK and KE variants show hydrophobic interaction patterns which are present in both CH3-CH3 and CH1-CL domains. Panels d–f in Supplementary Figure S4 illustrate the interdomain interactions of three engineered CH3-CH3 variants, which are part of bispecific antibody matrices generated by Format Chain Exchange (FORCE), which enables the screening of the combinatorial format spaces (Dengl et al., 2020). These variants were originally designed by further modifying the 5HY9 KiH structure, which already differs from the 4NQS KiH structure by an additional intermolecular disulfide bridge.
In Figure 7 we show three exemplary CH1-CL and three exemplary CH3-CH3 interfaces color-coded according to the number of interdomain salt bridge interactions. To facilitate the visualization of interface interactions, we flip the CH3 domain A and the CL domain. In line with the results presented in Figure 6, we find that the CH3-CH3 interface is dominated by salt bridge interactions, while the CH1-CL interface reveals a substantially lower number of ionic interactions, precisely the CH1-CL reveals one characteristic salt bridge between loop a_AB and β-strand b_G.
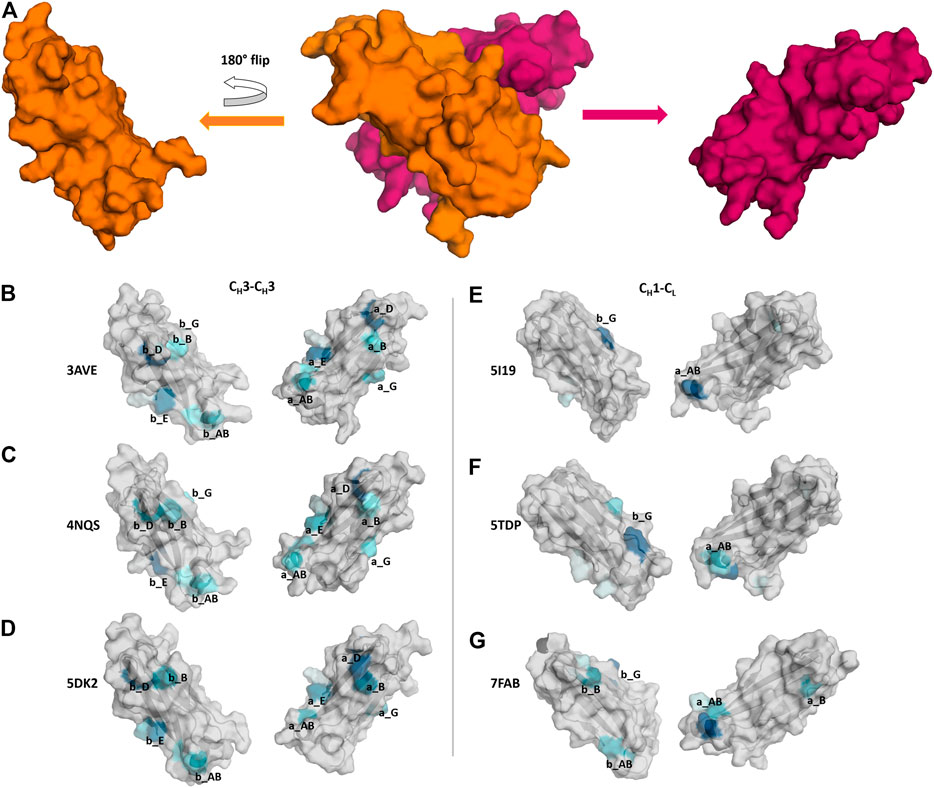
FIGURE 7. Comparison of CH3-CH3 and CH1-CL interface interaction patterns by analyzing their salt bridge interdomain interactions. (A) Stepwise illustration of the workflow to obtain the “open-book” representation (PDB: 3AVE). (B–G) Each individual domain is gradually colored based on the number and duration of interdomain interactions. The color-gradient (grey to blue) corresponds to the number of interdomain salt bridges each residue is forming (the higher the number of contacts, the darker are the shades of blue).
Structural CH3-CH3 Interface Characterization
Apart from identifying differences in interface interaction patterns between the structurally highly similar CH1-CL and CH3-CH3 interfaces, we provide in Figure 8 an overview of the main interactions stabilizing the homo-and-heterodimeric CH3-CH3 interfaces (wildtype, KiH and charge inversion). Already from the panels in Figure 8 the unique and well-defined organization of the CH3-CH3 interface becomes apparent. Together with the hydrophobic core interactions (shown in green), various salt bridge interactions located at the N-terminal and C-terminal charge cluster (highlighted in pink) contribute to the stabilization of the dimeric interface. To characterize interactions and to identify residues that are critical for the interface formation, we analysed the investigated CH3-CH3 homo-and-heterodimer simulations in-detail. We find that the interactions in the core of the interface are particularly important for stabilization and formation of the dimer. One of these crucial interactions is the stacking interaction between residues Y407-Y407, which are present in all frames of the simulation in the variants with both interaction partners present (highlighted in Figure 8). We observe that especially mutations at the centre of the interface have a strong influence on the hydrophobic and salt bridge interaction network of the whole interface. One example would be the DE-KK variant (PDB accession code: 5NSC) (De Nardis et al., 2017), which introduces two ion pair interactions into the hydrophobic core by substituting L351D and L368E in one domain and L351K and T366K in the other. Even though these introduced residues strongly interact with each other, the mutations result in a change of the overall interdomain interaction patterns, which also differ from all other engineered variants. Particularly interesting is, that this DE-KK variant has the highest variability in the interdomain orientations (dC, AB, AC1, AC2, BC1, BC2) compared to all other investigated variants (Figure 2B). It also shows a slightly higher distance (dc) between the two domains and bigger variations in the tilt and bend angles, allowing also water molecules to interact with the N-terminal and C-terminal charge clusters. We also find similar results for the DD-KK variant (PDB accession code: 5DK2). The main difference between the DE-KK and the DD-KK variant is the location of the mutations. While the DE-KK disrupts the hydrophobic core interactions at the centre of the interface, the DD-KK variant introduces substitutions in the N-terminal charge cluster and C-terminal charge cluster. Introducing charge reversions in the charge clusters in this example results in an imbalance of positive and negative charges in the respective domains and, i.e., five negative charges in domain A, six positive charges in domain B. In particular the E356K mutation additionally results in a loss of a critical salt bridge interaction situated at the N-terminal charge cluster, which consequently shifts the interdomain tilt angles AC1 and BC1 and thereby increases the conformational variability in the interface. In line with these findings, we observe an increase in flexibility of the core interface residues for the KiH (PDB: 4NQS) and the charge inversion variants (PDB: 5DK2, 5NSC), which is reflected in higher root-mean-square-fluctuation (RMSF) values, compared to the homodimer (Supplementary Figure S7).
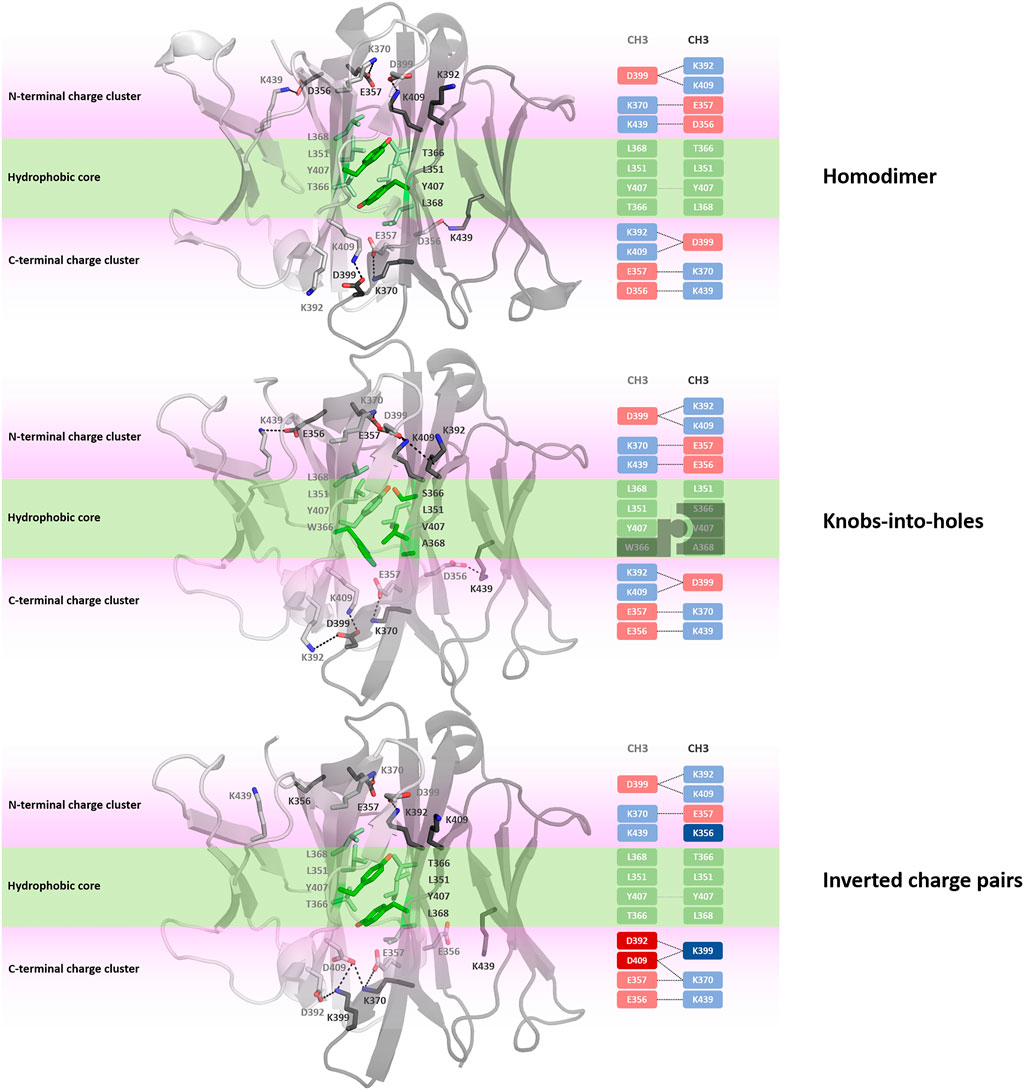
FIGURE 8. Interface interaction analysis of the homodimer and two heterodimers following different design strategies, i.e., knobs-into-holes (PDB: 4NQS) and charge inversion (PDB: 5DK2). The CH3-CH3 dimers consist of a hydrophobic core at the centre of the CH3-CH3 interface (illustrated in palegreen) and two highly charged regions (N-terminal charge cluster and C-terminal charge cluster), shown in light pink, that stabilize the interface.
Discussion
The idea of modifying antibody interfaces to reduce the risk of random assembly of different chains has motivated numerous studies to find variations of the proposed KiH approach, e.g., introducing charge pairs. Inverted charge interactions, instead of steric KiH interactions, were used for example for the design of the CH3-CH3 heterodimer DD-KK (K409D, K392D-D399K, E356K) variant (PDB accession code: 5DK2) (Ha et al., 2016). Also, the combination of the KiH interactions with the introduction of charge mutations have been presented in the CH3-CH3 heterodimer EW-RVT (K360E, K409W – Q347R, K399V, F405T) variant (PDB accession code: 4X98) (Choi et al., 2015).
One of the most frequent interactions situated in the centre of the homodimeric CH3-CH3 interface is the Y407-Y407 pi-stacking contact, residing in the central part of the E strands (Figure 8). (Dall’Acqua et al., 1998) Mutational studies confirmed the importance of these residues for the formation of the homodimeric interface. The salt bridge interactions at the N-terminal charge cluster and the C-terminal charge cluster (Figure 8) determine the characteristic interaction profile of the CH3-CH3 interface and substantially stabilize the dimer. The hydrophobic core in the homodimeric CH3-CH3 interface is formed by contacts between residues F405, L368, L351, Y407 and T366. These hydrophobic interactions are often modified following the KiH strategy (Ridgway et al., 1996; Elliott et al., 2014; Kuglstatter et al., 2017). The KiH variant (PDB accession codes: 4NQS, 5HY9, 5DI8) contains a knob in one CH3 domain (domain A) by mutating residue T366 to the bulkier amino acid tryptophane (Figure 8). Three other residues on the other CH3 domain (domain B) are also exchanged to smaller residues (T366S, Y407A, L368V) to ensure hydrophobic and steric complementarity. The orientation and position of the introduced tryptophane residue, also called “knob,” dominates the shape complementary between the two domains.
For the CH3-CH3 interfaces investigated in this study, we provide a sequence alignment showing the respective mutations including a classification of the underlying engineering strategies. To connect the sequence variations to our coarse-grained flareplots, we included our color-coded strand/loop definition in the alignment (Supplementary Figures S5, S6).
In our simulations of all different CH3-CH3 homo-and-heterodimers, we find that if both tyrosine residues are present, the pi-stacking interaction occurs in all frames of the simulation and contributes to stabilizing the interface. Additionally, Y407 forms a stabilizing and conserved hydrogen bond with T366, located in strand B, which occurs on average in 65% of the simulation time. Thus, as these Y407 residues form critical interactions, stabilizing the centre of the CH3-CH3 interface, mutating one of these residues can already prevent homodimerization (Ridgway et al., 1996; Von Kreudenstein et al., 2013). Additionally, we observe that introducing charge mutations/inversions at the hydrophobic core, can strongly influence the interface interaction network as shown for the DE-KK variant and result in a different interface formation, which can be accompanied by a decrease in stability. We find that this decrease in stability for the KiH (PDB: 4NQS) and the charge inversion variants (PDB: 5DK2, 5NSC), can result in a higher flexibility of the core interface residues, which is reflected in higher RMSF values (Supplementary Figure S7).
To compare the interaction patterns of the structurally highly similar CH1-CL and CH3-CH3 interfaces, we calculate coarse-grained interdomain interaction maps, which are visualized as flareplots and quantified as barplots. When comparing different CH3-CH3 interfaces we find a highly conserved salt bridge between two glutamate residues (E356/E357) located in the a_AB loop with the lysine (K439) located in the b_G strand. These interactions can also be found in the CH1-CL interfaces containing a λ light chain (PDB accession codes: 7FAB, 1NL0). Another critical conserved interdomain interaction among CH1-CL domains can be found between the a_DE loop and the b_D strand, which is unique for kappa light chain antibodies. Especially for the salt bridges and hydrophobic interactions the patterns between κ and λ light chains differ the most (Figures 4A, 5A). Apart from the conserved contacts among all CH1-CL interfaces, salt bridges are formed between the a_AB loops and b_B strands for the λ light chain antibodies. Interestingly, these salt bridges between a_AB loops and b_B strands are actually present in all considered CH3-CH3 domains (Figure 4B). Furthermore, an additional hydrophobic interaction can be found for the λ light chain antibodies between the a_E - b_D strands, which again can also be found in the CH3-CH3 interface (Figure 5C). Astonishingly, we observe in Figure 6 that the CH3 dimer is not only primarily stabilized by hydrophobic interactions but actually dominated by strong electrostatic interactions. Our observation, that the CH3-CH3 domains have a substantially higher number of salt bridges and hydrogen bonds, can also be explained by very frequently occurring interactions between residues D399-K409, D399-K392, E356-K439 and E357-K370, which surround the hydrophobic core. The high number of salt bridge interactions in the CH3-CH3 interface are also reflected in the electrostatic interaction energies, which are substantially higher compared to the CH1-CL domains (Supplementary Table S2). However, there are high fluctuations in the electrostatic energies of the individual CH3-CH3 interfaces, which result from repairing salt bridge interactions between different residues. The CH1-CL interface on the other hand is formed by mainly hydrophobic contacts.
The difference in electrostatic interaction energy is also reflected in the findings presented in Figure 7, which show a comparison of three CH3-CH3 and three CH1-CL interfaces, illustrated as an “open-book” representation. The surfaces of the individual domains are color-coded according to the number of interdomain salt bridge interactions. We find substantial differences in the interface interaction patterns between the two interface classes. In particular, the CH1-CL interface is dominated by one salt bridge between the a_AB loop and the b_G strand (Figure 7E). Figure 7F shows an engineered and mutated CH1-CL interface, which contains mutations at the edge of the interface, which have been discussed to introduce more flexibility and indeed, we find more frequent switches in interdomain salt bridge interactions, which suggests a higher flexibility at the edge of the interface. In Figure 7G we depict interdomain salt bridge interactions of a CH1-CL interface containing a λ light chain. In agreement with the results in Figure 4, we find more salt bridge interactions in CH1-CL interface for λ light chain CH1-CL domains and thus observe similar interaction patterns compared to the CH3-CH3 interfaces.
Apart from a detailed characterization of the CH3-CH3 and CH1-CL interfaces, we also investigated the relative interdomain orientations during the simulations. In line with previous studies, we find that for the CH1-CL, as well as the CH3-CH3 domains, the majority of interdomain movements are surprisingly fast and can be captured in the low nanosecond timescale (Fernández-Quintero et al., 2020a; Fernández-Quintero et al., 2020b). Additionally, we observe for the investigated CH1-CL domains (both λ and κ) left shifted cHL angle distributions towards lower cHL angles with a broader spread angle in the angle ranges, compared to the CH3-CH3 domains. For one λ light chain antibody (PDB accession code: 1NL0) we even observe a substantially shifted angle distribution towards lower cHL angle ranges. This higher variability in these cHL angle distributions is not surprising considering the higher number of sequence variations that occur in CH1-CL domains, while the CH3-CH3 domains contain solely point mutations.
Conclusion
In conclusion, we present a systematic characterization and a structural comparison of different CH1-CL and CH3-CH3 domains. By using molecular dynamics simulations, we find substantial differences in interaction patterns of the structurally highly similar CH1-CL and CH3-CH3 interfaces. While CH1-CL interfaces are dominated by hydrophobic interactions, we find that the CH3-CH3 interfaces are stabilized by numerous salt bridge interactions surrounding the hydrophobic core. Furthermore, we provide quantitative contact maps comparing CH1-CL and CH3-CH3 domains and highlighting which strands are key determinants for their structural integrity. Apart from the comparison, we also mechanistically discuss different CH3-CH3 interface engineering strategies, which provide an extensive understanding of the CH3-CH3 interfaces and thereby advance the design of bispecific antibodies.
Methods
Dataset
The investigated CH1-CL and CH3-CH3 X-ray structures were chosen to have a representative set of antibodies covering various challenges in antibody engineering and design, as they differ in light chain types and follow different design strategies to reduce the risk of mispairings (Supplementary Table S1). (Ha et al., 2016; Teplyakov et al., 2016; Dillon et al., 2017; Dengl et al., 2020) 23 crystal structures of heterodimeric CH3 IgG1 mutants, as well as the corresponding wildtype were obtained from the PDB. The 23 mutants have been designed following different strategies: knobs-into-holes strategy, complementary electrostatic interactions, format chain exchange platform or by using Multistate Design (MSD), which is a computational sequence optimization tool.
Apart from the 23 CH3-CH3 domains, we also simulated 23 Fab crystal structures.
16 germline Fab crystal structures are from the same library (Teplyakov et al., 2016). We chose this dataset as it allows to systematically investigate the influence of different heavy and light chain pairings. The phage library is composed of 4 heavy chain germlines IGHV1-69 (H1-69), IGHV3-23 (H3-23), IGHV5-51 (H5-51) and IGHV3-53 (H5-53) and 4 light chain germlines (all κ) IGKV1-39 (L1-39), IGKV3-11 (L3-11), IGKV3-20 (L3-20) and IGKV4-1 (L4-1). These genes were selected based on the frequency of their use, their cognate canonical structures, which can recognize proteins and peptides and their ability to be expressed in bacteria. Additionally, we included three Fab fragments which were part of a study redesigning the Fab interfaces. Furthermore, we also investigated two λ light chain antibodies and two recently published DutaFab structures, which are characterized by their high stability and their ability to recognize two different antigens (Beckmann et al., 2021). Dual targeting (Duta) Fab molecules contain two independent and spatially separated binding sites within the CDR loops (H-side paratope and L-side paratope) that simultaneously allow to bind two target molecules at the same Fv.
MD Simulation Protocol
All X-ray structures were prepared in MOE (Molecular Operating Environment, Montreal, QC, Canada: 2019) (Chemical Computing Group, 2020) using the Protonate 3D (Labute, 2009) tool. With the tleap tool of the Amber Tools20 package, we explicitly bonded all existing disulphide bridges (Supplementary Figure S8) and placed the Fab and CH3-CH3 structures into cubic water boxes of TIP3P(Jorgensen et al., 1983) water molecules with a minimum wall distance to the protein of 10 Å (El Hage et al., 2018; Gapsys and de Groot, 2019). Parameters for all antibody simulations were derived from the AMBER force field 14SB (Cornell et al., 1995; Maier et al., 2015). To neutralize the charges, we used uniform background charges (Darden et al., 1993; Salomon-Ferrer et al., 2013; Hub et al., 2014). Each system was carefully equilibrated using a multistep equilibration protocol (Wallnoefer et al., 2010; Wallnoefer et al., 2011).
Molecular dynamics simulations were performed using pmemd.cuda in an NpT ensemble to be as close to the experimental conditions as possible and to obtain the correct density distributions of both protein and water. Bonds involving hydrogen atoms were restrained by applying the SHAKE algorithm (Miyamoto and Kollman, 1992), allowing a timestep of 2.0 fs. Atmospheric pressure of the system was preserved by weak coupling to an external bath using the Berendsen algorithm (Berendsen et al., 1984). The Langevin thermostat was used to maintain the temperature at 300K during simulations (Adelman and Doll, 1976). The parameter file used to perform all MD simulations is provided at the end of the Supporting Information.
Contacts
To calculate contacts of both CH1-CL and CH3-CH3 interfaces we used the GetContacts software (Stanford University, adate). This tool can compute interactions within one protein structure, but also between different protein interfaces and allows to monitor the evolution of contacts during the simulation. The development of the contacts during a simulation can be visualized in so-called flareplots. For all available simulations (Supplementary Table S1) we calculated all different types of contacts, including hydrogen bonds (sidechain/sidechain, sidechain/backbone, backbone/backbone), salt bridges, hydrophobic and Van der Waals interactions. The contacts are determined based on the default geometrical criteria provided by GetContacts. To recognize interface patterns and to describe the dissociation mechanisms of both the CH1-CL and CH3-CH3 domains, we coarse grained residues belonging to the same loops or β-strands. The secondary structure assignment has been performed with STRIDE (Frishman and Argos, 1995; Heinig and Frishman, 2004). To quantitively identify systematic differences in the interface interactions of the two interface classes, we evaluated the frequency of different interaction types. Thus, we counted contacts (for each type of interaction) of certain structural elements, e.g., salt bridges between the strand a_A and the loop b_AB. Furthermore, we calculated mean contact frequencies (contact per frame) in the simulations and averaged these frequencies within the interface classes and compared the results. In addition, we quantified the standard error of the mean of these contact frequencies within these classes. This comparison enabled us to find contacts, which, e.g., exist in all the CH1-CL interfaces, but not in CH3-CH3 interfaces, or vice versa. Apart from visualizing and quantifying the contacts of both CH1-CL and CH3-CH3 interfaces, we also calculated the linear interaction energies (LIE) by using the LIE tool implemented in cpptraj (Roe and Cheatham, 2013). We calculated the electrostatic interaction energies for all frames of each simulation (10,000 frames/simulation) and provided the simulation-averages of these interaction energies in Supplementary Table S2.
Interdomain Orientation Calculations
While computational tools to fully characterize the Fv region of antibodies and TCRs are already available, no such tools were published for other immunoglobulin domain interfaces, such as the CH3-CH3 and the CH1-CL interface (Dunbar et al., 2013). The OCD approach (Hoerschinger et al., 2021) creates a suitable coordinate system for the characterization of these interfaces for any user-provided reference structure. This allows a straight-forward analysis without the significant demands on previous structural knowledge. Using this tool, a reference coordinate system is created based on user-defined reference structures consisting of an atomic structure and two domain selections over these atoms. To this end, the reference structure for each domain is generated by considering a center axis linking the two centers of mass of the different domains, and the first principal axis P of inertia of each domain corresponding to the lowest eigenvalue of the inertia tensor. Each individual domain is aligned to the world coordinate system by aligning this principal axis to the z unit vector and the center axis as close as possible to the x unit vector, yielding a reference structure for each domain. To map the coordinate system onto a sample structure, the references are aligned to the sample and the alignment transformations are applied to the xyz unit vectors. The transformed z vectors (A1/B1) and y vectors (A2/B2) as well as the center axis are then used to calculate six orientational measures: Two tilt angles for each vector towards the center axis (AC1, AC2, BC1, BC2), the length of the center axis (dC) and a torsion angle (AB) between the two intersecting planes composed of A1, the centre axis and B1. To better visualize the relative interdomain orientations we performed the Gaussian kernel density estimation (KDE) on the HL angles, to obtain the probability density distributions. To calculate the KDE we used the recently published implementation of KDE in C++ (Kraml et al., 2021). We used 10,000 frames of each MD simulation (1µs) to calculate and plot the relative interdomain orientations.
Relative VH and VL Orientations Using ABangle
ABangle is a computational tool (Dunbar et al., 2013; Bujotzek et al., 2015; Bujotzek et al., 2016; Fernández-Quintero et al., 2020b) to characterize the relative orientations between the antibody variable domains (VH and VL) using six measurements (five angles and a distance). A plane is projected on each of the two variable domains. Between these two planes, a distance vector C is defined. The six measures are then two tilt angles between each plane (HC1, HC2, LC1, LC2) and a torsion angle (HL) between the two planes along the distance vector C (dC). The ABangle script can calculate these measures for an arbitrary Fv region by aligning the consensus structures to the found core set positions and fitting the planes and distance vector from this alignment. This online available tool was combined with an in‐house python script to reduce computational effort and to visualize our simulation data over time. The in‐house script makes use of ANARCI(Dunbar and Deane, 2016) for fast local annotation of the Fv region and pytraj from the AmberTools package (Case et al., 2020) for rapid trajectory processing.
Data Availability Statement
The original contributions presented in the study are included in the article/Supplementary Material, further inquiries can be directed to the corresponding author.
Author Contributions
MF and PQ performed research and wrote the manuscript. FW, CS, NP, KK, JL and VH analysed data. AB, GG, HK and KL advised and supervised the research. All authors contributed to writing the manuscript.
Funding
This work was supported by Austrian Science Fund (P30565, P30737, P34518 and DOC 30). Furthermore, this project has received funding from the European Union’s Horizon 2020 research and innovation program under grant agreement No 764958. The computational results presented have been achieved in part using the Vienna Scientific Cluster (VSC). European Union’s Horizon 2020 research and innovation programme under the Marie Skłodowska-Curie grant agreement No. 847476 to NP.
Conflict of Interest
Authors AB, GG and HK were employed by the company Roche.
The remaining authors declare that the research was conducted in the absence of any commercial or financial relationships that could be construed as a potential conflict of interest.
Publisher’s Note
All claims expressed in this article are solely those of the authors and do not necessarily represent those of their affiliated organizations, or those of the publisher, the editors, and the reviewers. Any product that may be evaluated in this article, or claim that may be made by its manufacturer, is not guaranteed or endorsed by the publisher.
Acknowledgments
We acknowledge PRACE for awarding us access to Piz Daint at CSCS, Switzerland.
Supplementary Material
The Supplementary Material for this article can be found online at: https://www.frontiersin.org/articles/10.3389/fmolb.2022.812750/full#supplementary-material
References
Adachi, M., Kurihara, Y., Nojima, H., Takeda-Shitaka, M., Kamiya, K., and Umeyama, H. (2003). Interaction between the Antigen and Antibody Is Controlled by the Constant Domains: normal Mode Dynamics of the HEL-HyHEL-10 Complex. Protein Sci. 12, 2125–2131. doi:10.1110/ps.03100803
Addis, P. W., Hall, C. J., Bruton, S., Veverka, V., Wilkinson, I. C., Muskett, F. W., et al. (2014). Conformational Heterogeneity in Antibody-Protein Antigen Recognition: Implications for High Affinity Protein Complex Formation. J. Biol. Chem. 289, 7200–7210. doi:10.1074/jbc.m113.492215
Adelman, S. A., and Doll, J. D. (1976). Generalized Langevin Equation Approach for Atom/solid-Surface Scattering: General Formulation for Classical Scattering off Harmonic Solids. J. Chem. Phys. 64, 2375–2388. doi:10.1063/1.432526
Beckmann, R., Jensen, K., Fenn, S., Speck, J., Krause, K., Meier, A., et al. (2021). DutaFabs Are Engineered Therapeutic Fab Fragments that Can Bind Two Targets Simultaneously. Nat. Commun. 12, 708. doi:10.1038/s41467-021-20949-3
Berendsen, H. J. C., van Postma, J. P. M., van Gunsteren, W. F., DiNola, A., and Haak, J. R. (1984). Molecular-Dynamics with Coupling to an External Bath. J. Chem. Phys. 81, 3684. doi:10.1063/1.448118
Bönisch, M., Sellmann, C., Maresch, D., Halbig, C., Becker, S., Toleikis, L., et al. (2017). Novel CH1:CL Interfaces that Enhance Correct Light Chain Pairing in Heterodimeric Bispecific Antibodies. Protein Eng. Des. Selection 30, 685–696. doi:10.1093/protein/gzx044
Brinkmann, U., and Kontermann, R. E. (2017). The Making of Bispecific Antibodies. mAbs 9, 182–212. doi:10.1080/19420862.2016.1268307
Bujotzek, A., Dunbar, J., Lipsmeier, F., Schäfer, W., Antes, I., Deane, C. M., et al. (2015). Prediction of VH-VL Domain Orientation for Antibody Variable Domain Modeling. Proteins 83, 681–695. doi:10.1002/prot.24756
Bujotzek, A., Lipsmeier, F., Harris, S. F., Benz, J., Kuglstatter, A., and Georges, G. (2016). VH-VL Orientation Prediction for Antibody Humanization Candidate Selection: A Case Study. mAbs 8, 288–305. doi:10.1080/19420862.2015.1117720
Case, D. A., Belfon, K., Ben-Shalom, I. Y., Brozell, S. R., Cerutti, D. S., Cheatham, T. E., et al. (2020). AMBER 2020. San Francisco: University of California.
Chemical Computing Group (2020). Molecular Operating Environment (MOE). 1010 Sherbrooke St. West, Suite #910. Montreal, QC, Canada: Chemical Computing Group, H3A. 2R7.
Chiu, M. L., Goulet, D. R., Teplyakov, A., and Gilliland, G. L. (2019). Antibody Structure and Function: The Basis for Engineering Therapeutics. Antibodies 8, 55. doi:10.3390/antib8040055
Choi, H.-J., Seok, S.-H., Kim, Y.-J., Seo, M.-D., and Kim, Y.-S. (2015). Crystal Structures of Immunoglobulin Fc Heterodimers Reveal the Molecular Basis for Heterodimer Formation. Mol. Immunol. 65, 377–383. doi:10.1016/j.molimm.2015.02.017
Colman, P. M. (1988). “Structure of Antibody-Antigen Complexes: Implications for Immune Recognition,” in Advances in Immunology. Editor F. J. Dixon (Cambridge, UK: Academic Press). doi:10.1016/s0065-2776(08)60364-8
Cornell, W. D., Cieplak, P., Bayly, C. I., Gould, I. R., Merz, K. M., Ferguson, D. M., et al. (1995). A Second Generation Force Field for the Simulation of Proteins, Nucleic Acids, and Organic Molecules. J. Am. Chem. Soc. 117, 5179–5197. doi:10.1021/ja00124a002
Dall’Acqua, W., Simon, A. L., Mulkerrin, M. G., and Carter, P. (1998). Contribution of Domain Interface Residues to the Stability of Antibody CH3 Domain Homodimers. Biochemistry 37, 9266–9273.
Darden, T., York, D., and Pedersen, L. (1993). Particle Mesh Ewald: AnN⋅Log(N) Method for Ewald Sums in Large Systems. J. Chem. Phys. 98, 10089–10092. doi:10.1063/1.464397
Davies, D. R., and Chacko, S. (1993). Antibody Structure. Acc. Chem. Res. 26, 421–427. doi:10.1021/ar00032a005
De Nardis, C., Hendriks, L. J. A., Poirier, E., Arvinte, T., Gros, P., Bakker, A. B. H., et al. (2017). A New Approach for Generating Bispecific Antibodies Based on a Common Light Chain Format and the Stable Architecture of Human Immunoglobulin G1. J. Biol. Chem. 292, 14706–14717. doi:10.1074/jbc.m117.793497
Dengl, S., Mayer, K., Bormann, F., Duerr, H., Hoffmann, E., Nussbaum, B., et al. (2020). Format Chain Exchange (FORCE) for High-Throughput Generation of Bispecific Antibodies in Combinatorial Binder-Format Matrices. Nat. Commun. 11, 4974. doi:10.1038/s41467-020-18477-7
Dillon, M., Yin, Y., Zhou, J., McCarty, L., Ellerman, D., Slaga, D., et al. (2017). Efficient Production of Bispecific IgG of Different Isotypes and Species of Origin in Single Mammalian Cells. mAbs 9, 213–230. doi:10.1080/19420862.2016.1267089
Dunbar, J., and Deane, C. M. (2016). ANARCI: Antigen Receptor Numbering and Receptor Classification. Bioinformatics 32, 298–300. doi:10.1093/bioinformatics/btv552
Dunbar, J., Fuchs, A., Shi, J., and Deane, C. M. (2013). ABangle: Characterising the VH-VL Orientation in Antibodies. Protein Eng. Des. Selection 26, 611–620. doi:10.1093/protein/gzt020
El Hage, K., Hédin, F., Gupta, P. K., Meuwly, M., and Karplus, M. (2018). Valid Molecular Dynamics Simulations of Human Hemoglobin Require a Surprisingly Large Box Size. eLife 7, e35560. doi:10.7554/eLife.35560
Elliott, J. M., Ultsch, M., Lee, J., Tong, R., Takeda, K., Spiess, C., et al. (2014). Antiparallel Conformation of Knob and Hole Aglycosylated Half-Antibody Homodimers Is Mediated by a CH2-CH3 Hydrophobic Interaction. J. Mol. Biol. 426, 1947–1957. doi:10.1016/j.jmb.2014.02.015
Feige, M. J., Grawert, M. A., Marcinowski, M., Hennig, J., Behnke, J., Auslander, D., et al. (2014). The Structural Analysis of Shark IgNAR Antibodies Reveals Evolutionary Principles of Immunoglobulins. Proc. Natl. Acad. Sci. 111, 8155–8160. doi:10.1073/pnas.1321502111
Fernández-Quintero, M. L., Hoerschinger, V. J., Lamp, L. M., Bujotzek, A., Georges, G., and Liedl, K. R. (2020). VH-VL Interdomain Dynamics Observed by Computer Simulations and NMR. Proteins: Struct. Funct. Bioinformatics 88, 830–839. doi:10.1002/prot.25872
Fernández-Quintero, M. L., Kroell, K. B., Heiss, M. C., Loeffler, J. R., Quoika, P. K., Waibl, F., Bujotzek, A., et al. (2020). Surprisingly Fast Interface and Elbow Angle Dynamics of Antigen-Binding Fragments. Front. Mol. Biosciences 7, 339. doi:10.3389/fmolb.2020.609088
Fernández-Quintero, M. L., Loeffler, J. R., Waibl, F., Kamenik, A. S., Hofer, F., and Liedl, K. R. (2020c). Conformational Selection of Allergen-Antibody Complexes—Surface Plasticity of Paratopes and Epitopes. Protein Eng. Des. Selection 32, 513–523. doi:10.1093/protein/gzaa014
Frishman, D., and Argos, P. (1995). Knowledge-based Protein Secondary Structure Assignment. Proteins 23, 566–579. doi:10.1002/prot.340230412
Fudenberg, H. H., Drews, G., and Nisonoff, A. (1964). Serologic Demonstration of Dual Specificity of Rabbit Bivalent Hybrid Antibody. J. Exp. Med. 119, 151–166. doi:10.1084/jem.119.1.151
Gapsys, V., and de Groot, B. L. (2019). Comment on 'Valid Molecular Dynamics Simulations of Human Hemoglobin Require a Surprisingly Large Box Size'. Elife 8, 563064. doi:10.7554/eLife.44718
Ha, J.-H., Kim, J.-E., and Kim, Y.-S. (2016). Immunoglobulin Fc Heterodimer Platform Technology: From Design to Applications in Therapeutic Antibodies and Proteins. Front. Immunol. 7, 394. doi:10.3389/fimmu.2016.00394
Heinig, M., and Frishman, D. (2004). STRIDE: a Web Server for Secondary Structure Assignment from Known Atomic Coordinates of Proteins. Nucleic Acids Res. 32, W500–W502. doi:10.1093/nar/gkh429
Hoerschinger, V. J., Fernández-Quintero, M. L., Waibl, F., Kraml, J., Bujotzek, A., Georges, G., et al. (2021). OCD.py - Characterizing Immunoglobulin Inter-domain Orientations. bioRxiv 2021 03 (15), 435379. doi:10.1101/2021.03.15.435379
Hub, J. S., de Groot, B. L., Grubmüller, H., and Groenhof, G. (2014). Quantifying Artifacts in Ewald Simulations of Inhomogeneous Systems with a Net Charge. J. Chem. Theor. Comput. 10, 381–390. doi:10.1021/ct400626b
Jorgensen, W. L., Chandrasekhar, J., Madura, J. D., Impey, R. W., and Klein, M. L. (1983). Comparison of Simple Potential Functions for Simulating Liquid Water. J. Chem. Phys. 79, 926–935. doi:10.1063/1.445869
Jost Lopez, A., Quoika, P. K., Linke, M., Hummer, G., and Köfinger, J. (2020). Quantifying Protein-Protein Interactions in Molecular Simulations. J. Phys. Chem. B 124, 4673–4685. doi:10.1021/acs.jpcb.9b11802
Kaplon, H., Muralidharan, M., Schneider, Z., and Reichert, J. M. (2020). Antibodies to Watch in 2020. mAbs 12, 1703531. doi:10.1080/19420862.2019.1703531
Kaplon, H., and Reichert, J. M. (2021). Antibodies to Watch in 2021. mAbs 13, 1860476. doi:10.1080/19420862.2020.1860476
Kraml, J., Hofer, F., Quoika, P. K., Kamenik, A. S., and Liedl, K. R. (2021). X-entropy: A Parallelized Kernel Density Estimator with Automated Bandwidth Selection to Calculate Entropy. J. Chem. Inf. Model. 61, 1533–1538. doi:10.1021/acs.jcim.0c01375
Kuglstatter, A., Stihle, M., Neumann, C., Müller, C., Schaefer, W., Klein, C., et al. Roche Pharmaceutical Research and Early Development (2017). Structural Differences between Glycosylated, Disulfide-Linked Heterodimeric Knob-Into-Hole Fc Fragment and its Homodimeric Knob–Knob and Hole–Hole Side Products. Protein Eng. Des. Selection 30, 649–656. doi:10.1093/protein/gzx041
Labute, P. (2009). Protonate3D: Assignment of Ionization States and Hydrogen Coordinates to Macromolecular Structures. Proteins 75, 187–205. doi:10.1002/prot.22234
Maier, J. A., Martinez, C., Kasavajhala, K., Wickstrom, L., Hauser, K. E., and Simmerling, C. (2015). ff14SB: Improving the Accuracy of Protein Side Chain and Backbone Parameters from ff99SB. J. Chem. Theor. Comput. 11, 3696–3713. doi:10.1021/acs.jctc.5b00255
Miyamoto, S., and Kollman, P. A. (1992). Settle: An Analytical Version of the SHAKE and RATTLE Algorithm for Rigid Water Models. J. Comput. Chem. 13, 952–962. doi:10.1002/jcc.540130805
Moore, G. L., Bernett, M. J., Rashid, R., Pong, E. W., Pong, D.-H. T., Jacinto, J., et al. (2019). A Robust Heterodimeric Fc Platform Engineered for Efficient Development of Bispecific Antibodies of Multiple Formats. Methods 154, 38–50. doi:10.1016/j.ymeth.2018.10.006
Nisonoff, A., and Rivers, M. M. (1961). Recombination of a Mixture of Univalent Antibody Fragments of Different Specificity. Arch. Biochem. Biophys. 93, 460–462. doi:10.1016/0003-9861(61)90296-x
Regula, J. T., Imhof-Jung, S., Mølhøj, M., Benz, J., Ehler, A., Bujotzek, A., et al. (2018). Variable Heavy-Variable Light Domain and Fab-Arm CrossMabs with Charged Residue Exchanges to Enforce Correct Light Chain Assembly. Protein Eng. Des. Selection 31, 289–299. doi:10.1093/protein/gzy021
Ridgway, J. B. B., Presta, L. G., and Carter, P. (1996). 'Knobs-into-holes' Engineering of Antibody CH3 Domains for Heavy Chain Heterodimerization. Protein Eng. Des. Sel 9, 617–621. doi:10.1093/protein/9.7.617
Roe, D. R., and Cheatham, T. E. (2013). PTRAJ and CPPTRAJ: Software for Processing and Analysis of Molecular Dynamics Trajectory Data. J. Chem. Theor. Comput. 9, 3084–3095. doi:10.1021/ct400341p
Rose, R. J., van Berkel, P. H. C., van den BremerLabrijn, E. T. J., Labrijn, A. F., Vink, T., Schuurman, J., et al. (2013). Mutation of Y407 in the CH3 Domain Dramatically Alters Glycosylation and Structure of Human IgG. MAbs 5, 219–228. doi:10.4161/mabs.23532
Röthlisberger, D., Honegger, A., and Plückthun, A. (2005). Domain Interactions in the Fab Fragment: A Comparative Evaluation of the Single-Chain Fv and Fab Format Engineered with Variable Domains of Different Stability. J. Mol. Biol. 347, 773–789. doi:10.1016/j.jmb.2005.01.053
Salomon-Ferrer, R., Götz, A. W., Poole, D., Le Grand, S., and Walker, R. C. (2013). Routine Microsecond Molecular Dynamics Simulations with AMBER on GPUs. 2. Explicit Solvent Particle Mesh Ewald. J. Chem. Theor. Comput. 9, 3878–3888. doi:10.1021/ct400314y
Sedykh, S., Prinz, V., Buneva, V., and Nevinsky, G. (2018). Bispecific Antibodies: Design, Therapy, Perspectives. Dddt 12, 195–208. doi:10.2147/dddt.s151282
Stanfield, R. L., Zemla, A., Wilson, I. A., and Rupp, B. (2006). Antibody Elbow Angles Are Influenced by Their Light Chain Class. J. Mol. Biol. 357, 1566–1574. doi:10.1016/j.jmb.2006.01.023
Stanford University (adate). GetContacts. Available at: https://getcontacts.github.io/
Teplyakov, A., Obmolova, G., Malia, T. J., Luo, J., Muzammil, S., Sweet, R., et al. (2016). Structural Diversity in a Human Antibody Germline Library. mAbs 8, 1045–1063. doi:10.1080/19420862.2016.1190060
Teplyakov, A., Zhao, Y., Malia, T. J., Obmolova, G., and Gilliland, G. L. (2013). IgG2 Fc Structure and the Dynamic Features of the IgG CH2-CH3 Interface. Mol. Immunol. 56, 131–139. doi:10.1016/j.molimm.2013.03.018
Vanhove, M., Usherwood, Y.-K., and Hendershot, L. M. (2001). Unassembled Ig Heavy Chains Do Not Cycle from BiP In Vivo but Require Light Chains to Trigger Their Release. Immunity 15, 105–114. doi:10.1016/s1074-7613(01)00163-7
Von Kreudenstein, T. S., Escobar-Carbrera, E., Lario, P. I., D’Angelo, I., Brault, K., Kelly, J. F., et al. (2013). Improving Biophysical Properties of a Bispecific Antibody Scaffold to Aid Developability. mAbs 5, 646–654. doi:10.4161/mabs.25632
Wallnoefer, H. G., Handschuh, S., Liedl, K. R., and Fox, T. (2010). Stabilizing of a Globular Protein by a Highly Complex Water Network: A Molecular Dynamics Simulation Study on Factor Xa. J. Phys. Chem. B 114, 7405–7412. doi:10.1021/jp101654g
Keywords: antibodies, structure, interface characterization, interface dynamics, antibody design, bispecific antibody formats
Citation: Fernández-Quintero ML, Quoika PK, Wedl FS, Seidler CA, Kroell KB, Loeffler JR, Pomarici ND, Hoerschinger VJ, Bujotzek A, Georges G, Kettenberger H and Liedl KR (2022) Comparing Antibody Interfaces to Inform Rational Design of New Antibody Formats. Front. Mol. Biosci. 9:812750. doi: 10.3389/fmolb.2022.812750
Received: 10 November 2021; Accepted: 10 January 2022;
Published: 26 January 2022.
Edited by:
Maya Topf, Leibniz Institute of Experimental Biology and UKE, GermanyReviewed by:
Didier Devaurs, University of Edinburgh, United KingdomStéphane Téletchéa, Université de Nantes, France
Copyright © 2022 Fernández-Quintero, Quoika, Wedl, Seidler, Kroell, Loeffler, Pomarici, Hoerschinger, Bujotzek, Georges, Kettenberger and Liedl. This is an open-access article distributed under the terms of the Creative Commons Attribution License (CC BY). The use, distribution or reproduction in other forums is permitted, provided the original author(s) and the copyright owner(s) are credited and that the original publication in this journal is cited, in accordance with accepted academic practice. No use, distribution or reproduction is permitted which does not comply with these terms.
*Correspondence: Klaus R. Liedl, Klaus.Liedl@uibk.ac.at
†These authors have contributed equally to this work