- 1Ottawa Research and Development Centre, Agriculture and Agri-Food Canada, Ottawa, ON, Canada
- 2Department of Chemistry and Biomolecular Sciences, University of Ottawa, Ottawa, ON, Canada
Alternaria section Alternaria is comprised of many species that infect a broad diversity of important crop plants and cause post-harvest spoilage. Alternaria section Alternaria species, such as A. alternata and A. arborescens, are prolific producers of secondary metabolites that act as virulence factors of disease and are mycotoxins that accumulate in infected tissues—metabolites that can vary in their spectrum of production between individuals from the same fungal species. Untargeted metabolomics profiling of secondary metabolite production using mass spectrometry is an effective means to detect phenotypic anomalies in secondary metabolism within a species. Secondary metabolite phenotypes from 36 Alternaria section Alternaria isolates were constructed to observe frequency of production patterns. A clear and unique mass feature pattern was observed for three of the strains that were linked with the production of the dehydrocurvularin family of toxins and associated detoxification products. Examination of corresponding genomes revealed the presence of the dehydrocurvularin biosynthesis gene cluster associated with a sub-telomeric accessory region. A comparison of sequence similarity and occurrences of the dehydrocurvularin biosynthetic gene cluster within Pleosporalean fungi is presented and discussed.
Introduction
In agriculture, many secondary metabolites produced by plant pathogenic fungi act as virulence factors and mycotoxins involved in the onset of the disease state and/or accumulate in harvested produce and grains, both of which directly impact crop yields and market suitability. The profile of secondary metabolites expressed during disease onset and throughout the pathogen life cycle within a crop can vary between individuals from the same fungal species. One reason for observed within-species variation in secondary metabolism is the presence of lineage-specific accessory chromosomes or subtelomeric accessory regions within the genome that encode for unique secondary metabolite biosynthetic gene clusters. Understanding trends in intraspecific secondary metabolite expression patterns of a pathogen population is therefore of interest to pathologists and regulatory agencies that monitor for the emergence of crop diseases and associated mycotoxins of concern.
Profiling small molecule production using mass spectrometry and untargeted metabolomics tools is an effective means to detect phenotypic anomalies in secondary metabolism within a species (Witte and Overy, 2022). ‘Consensus’ chemical phenotypes representing the breadth in secondary metabolism expressed by a given strain can be generated from extracts of single-spore isolates cultured under axenic conditions on multiple different types of media. Profiling culture extracts using ultra-performance liquid chromatography - high resolution mass spectrometry (UPLC-HRMS) coupled with untargeted metabolomics tools for data processing and analysis are used to designate mass features of interest from chemical phenotype modelling. A mass spectrometry technique called tandem MS involves applying energy to selected mass feature ions (precursor ions) generating molecular fragments (product ions)—when this event is carried out a single time it is designated as MS/MS (or MS2). Through MS2 experiments, molecular fragmentation patterns of mass features of interest are then compared to online MS2 spectral databases for putative metabolite annotation (Dührkop et al., 2015, 2019; Hoffmann et al., 2021). Molecular networking analysis can also be performed to detect deviations in the molecular structures of known secondary metabolite products, indicating if new metabolite analogs are present (Wandy et al., 2018; Nothias et al., 2020).
Combining genomics and untargeted metabolomics is a powerful way to screen populations of fungi for secondary metabolite production resulting from transcriptionally active biosynthetic gene clusters. Recent work in the field has revealed that fast-evolving “accessory” genomic regions, including small “accessory or conditionally dispensable” chromosomes, can often encode isolate-specific secondary metabolite biosynthetic gene clusters which diversify metabolite profiles within a population or species (for review see Witte et al., 2021b). Structural information of observed secondary metabolite products can be correlated with synthase/synthetase motifs from predicted secondary metabolite biosynthetic gene clusters in the genomes, and associations inferred. Recent studies employing this multi-omic approach have proven useful in the discovery of apicidin from Fusarium poae (Witte et al., 2021a) and to explain rapidly evolving ergopeptine patterns in Claviceps purpurea (Hicks et al., 2021).
Alternaria is a speciose, cosmopolitan fungal genus currently divided into 26 sub-generic sections, whose members can be found growing as saprobes, endophytes, pathogens, or something in between (Woudenberg et al., 2013; Woudenberg et al., 2014; Grum-Grzhimaylo et al., 2016). Of particular relevance to the agronomic sector is Alternaria section Alternaria, which contains many species that infect important crop plants and cause post-harvest spoilage. Commensurate with the broad diversity of plant hosts associated with Alternaria section Alternaria is the diversity of their associated secondary metabolites, which were the focus of intensive study for at least half a century (Lou et al., 2013). Chemotaxonomy has played an important role in understanding the pathogenic competency of A. alternata, the type species of section Alternaria. This widespread species is associated with plant disease in a remarkably diverse number of hosts (Rotem 1994), and certain strains have been deemed “formae speciales” or “pathotypes” based on their production of phytotoxins required to surmount specific host plant defenses (reviewed in Tsuge et al., 2013; Meena et al., 2017).
Isolates belonging to A. alternata, and the closely related A. arborescens species complex, are of particular notoriety because they are commonly detected both as plant pathogens and as food spoilage agents producing mycotoxins of concern for consumers. Toxicity data associated with chronic exposure for many Alternaria toxins is lacking, however, the genotoxins alternariol and alternariol monomethyl ether have been flagged for further study due to potential deleterious effects on human and animal health (EFSA scientific opinion 2011). Laboratory-based studies of exposure to other A. alternata associated toxins, such as tentoxin, tenuazonic acid, altertoxins, altenuene and AAL-toxins have either demonstrated acute toxicity or other harmful effects to animal or cellular models, including teratogenic and fetotoxic effects. Many Alternaria metabolites are therefore considered “emerging mycotoxins” of significant regulatory concern, whose effects on plants and plant consumers require further investigation, including in holistic or synergistic multi-toxin contexts.
In the current research study, the aforementioned untargeted metabolomics profiling techniques (Witte and Overy, 2022) were leveraged to characterize and compare UPLC-HRMS secondary metabolite profiles of representative Alternaria extracts derived from axenic cultures on differing media. Untargeted chemical phenotypes from 36 Alternaria isolates were constructed by converting preprocessed metabolomics data into simple binary matrices representing mass feature presence/absences per media tested which were in turn combined to observe frequency of production patterns. Binary matrices were used to simplify interpretation of secondary metabolite phenotypes by eliminating mass feature intensity variation which may arise from extract concentration variability or fungal competence levels (Witte and Overy, 2022). A clear and unique mass feature pattern was observed for three of the strains that were linked with the production of the dehydrocurvularin family of toxins and associated detoxification products. Examination of corresponding genomes revealed the presence of the dehydrocurvularin biosynthesis gene cluster (BGC) associated with a sub-telomeric accessory region. Comparison of the observed dehydrocurvularin BGC within Pleosporalean fungi is presented and discussed in terms of the potential of horizontal gene transfer between plant pathogens.
Materials and methods
Fungal strains
A total of 36 Alternaria strains identified to Alternaria section Alternaria were selected for study. For strains not previously identified to species, section-specific diagnostic markers ASA-10 and ASA-19, and the commonly used marker RNA polymerase II second largest subunit (rpb2), were sequenced for species determination at greater taxonomic resolution. Sequences were aligned with a representative set of strains and phylogenetic analyses were used to place strains within species or lineages (data not shown). Barcode DNA extraction, polymerase chain reaction (PCR), Sanger sequencing, representative strains, and phylogenetic analyses were as described in Dettman & Eggertson (2022).
Media and growth conditions
The four medium types used for axenic growth of fungal strains were CYS80, DRYES, MMK2, and ZM2 (media formulations are provided in the Supplementary Section 1.1 Media Formulations). For metabolomic profiling, all strains were 3-point inoculated onto plates of the four different media, with four plate replicates per medium. Inoculated plates were incubated for 14 days under conditions of 25°C and a light/dark cycle of 8/16 h. Additional media control plates (non-inoculated) were included.
Metabolite extraction
Nine agar plugs (5 mm diameter) were harvested from each fungal replicate into distinct scintillation vials and frozen at −20°C until they were able to undergo chemical extraction. Extractions were performed with 10 ml ethyl acetate (EtOAc) per vial; each vial was shaken on a rotary shaker for 3 h (at 160 RPM). Resulting solvent extracts were transferred to clean scintillation vials and evaporated until dry using a GeneVac (SP Scientific EZ-2 Elite) and stored at −20°C prior to UPLC-HRMS analysis. Details of chemicals/solvents and associated manufacturers are provided in (Supplementary Section 1.2 Chemical and Solvent List).
UPLC-HRMS analyses
Dried metabolite extracts were suspended in 1 ml of methanol (MeOH) and analyzed on a Thermo Ultimate 3000 UPLC coupled to a Thermo LTQ Orbitrap XL high resolution mass spectrometer and a Thermo Dionex Ulitmate 3,000 Diode array detector (190–800 nm). Chromatography was performed using a Phenomenex C18 Kinetex column (50 mm × 2.1 mm ID, 1.7 μm) with a flow rate of 0.35 ml/min over 10-min run, using a gradient of acetonitrile (ACN) (+0.1% formic acid) and water (H2O) (+0.1% formic acid): starting at 5% ACN and increased to 95% ACN by 4.5 min; held at 95% ACN until 8 min; returned to 5% ACN by 9 min; and then held at 5% ACN to 10 min to equilibrate the column to starting conditions. The HRMS was operated in electro-spray ionization mode (ESI+), monitoring a range of 100–2,000 m/z). The ESI used a capillary temp of 320°C, a sheath gas flow of 40, an auxiliary gas flow of 5, a sweep gas flow of 2, a source voltage of 4kV, a capillary voltage of 35 V, and a tube lens of 100 V.
MSn fragmentation was performed in high resolution on select ions in subsequent experiments using CID at 35 NCE. MassWorks™ software (v5.0.0, Cerno Bioscience) was used to improve spectral accuracy and confirm the molecular formulas of annotated ions. The sCLIPS searches were performed in dynamic analysis mode with elements C, H, N, and O allowances set at minimum 1 and maximum 100. Charge was specified as 1 and mass tolerance set to 5 ppm.
NanoLC-HRMS/MS analyses
Chromatographic separation of metabolites was performed on a Proxeon EASY nLC II System (Thermo Fisher Scientific) equipped with a Thermo Scientific™ Acclaim™ PepMap™ RSLC C18 column (15 cm × 75 μm ID, 3 μm, 100 Å) employing a 60 min, H2O/ACN (0.1% formic acid) gradient at a flow rate of 0.25 μL/min. Compounds were separated using a linear gradient from 10 to 100% ACN for 45 min, followed by washing 5 min at 100% ACN, then using a gradient from 100 to 10% ACN for 5 min and washing for 5 min at 100% H2O. Eluted compounds were analyzed on a Thermo Q-Exactive Plus HRMS using positive electrospray ionization (ESI) at an ion source temperature of 250°C and an ionspray (Thermo Scientific™ EASY spray) voltage of 2.1 kV. The FTMS scan type was full MS/data dependent (dd)-MS2 with the following parameters: a resolution of 70,000, an auto gain control target under 3.06, a maximum isolation time of 100 ms, and an m/z range of 100–1,500. The parameters of the dd-MS2 scan were as follows: a resolution of 17,500, an auto gain control target under 1.05, a maximum isolation time of 100 ms, a loop count of top 10 peaks, an isolation window of m/z 2, a normalized collision energy of 35 and dynamic exclusion duration of 10 s.
Metabolomics: Data preprocessing, curation, and visualization
UPLC-HRMS raw data files were converted to centroid format using Proteowizard MSConvert (opensource software: https://proteowizard.sourceforge.io/) to convert the datafiles into centroided. mzML format prior to preprocessing using MZmine 2.51 (Pluskal et al., 2010). Raw data files included MeOH blanks run after every sixth sample, and all were carefully examined to determine a minimum noise level threshold for data analysis. Mass feature detection was performed using the centroid mass detection algorithm using a noise level cut off of 9.0 × 104. ADAP chromatogram building was performed using a minimum groups size of 5 scans, a group intensity threshold of 1.0 × 105, a minimum highest intensity set to 1.0 × 107, and the m/z tolerance set to 10 ppm. Chromatogram deconvolution was performed using the local minimum module, with a chromatographic threshold set to 35%, a search minimum retention time range 0.05 min, a minimum relative height at 15.0%, a minimum absolute height at 5.0 × 106, and a minimum ratio of peak top/edge at 1.2, with a peak duration max of 2.00 min. Monotonic shape isotope grouping was performed using a m/z tolerance of 8.0 ppm, a retention time tolerance of 0.08 min, and a maximum charge of 1. Mass feature peak alignment was performed using the join aligner with the m/z tolerance set to 10.0 ppm (with a weight value set to 2) and the retention time tolerance set to 0.1 min (with a weight value set to 1). Finally, the multithreaded peakfinder gap-filling algorithm was applied to the dataset with an intensity tolerance of 70%, the m/z tolerance parameter specified as 10.0 ppm and the retention time tolerance set to 0.08 min. The data was then normalized to the total ion current prior to export. Selection of the utilized parameters was informed by inspection of the raw spectra, and accounting for the variance in the caffeine standard (included in the extraction solvent) and reserpine calibration standard which was run prior to each batch of samples.
A data matrix containing mass feature m/z, retention time, and normalized height was exported from MZmine and data curation, binary conversion and mass feature frequency phenotyping was performed in the R environment using in-house scripts according to Witte and Overy (2022). Mass features associated with media components and UPLC-HRMS system contaminants were removed. Data reduction was performed using Pearson correlation analysis over a sliding elution time window by grouping mass features if their intensities correlated across samples (correlation coefficients threshold set to 0.8 with a maximum p-value of 0.05) and if they eluted from the column within 0.02 min of each other. Mass feature peak height binary conversion was then performed and sample replication was compressed into a single mass feature value using a filtering step, where mass features were assigned a ‘0’ if they occurred in less than 3 of the 4 replicates (ie., <0.75). The data matrix was further reduced by summing mass feature values across the 4 media tested to form a ‘pseudo-binary’ matrix of detection frequencies for each feature. For the generation of the chemical phenotype heatmap, row and column clustering was calculated using “ward.D2” clustering of Euclidean distances. The heatmap was generated using the “Complexheatmap” R package (Gu et al., 2016).
MS2 molecular network analysis
One replicate extract of Alternaria arborescens strain DET2035 grown on CYS80 media was selected as a representative sample and submitted to obtain increased chromatographic resolution of metabolite components and untargeted HRMS/MS analysis for use with molecular ion network modelling. Data preprocessing was performed using MZMine2 pre-release version 2.37.1corr17.7 utilizing the Ion Identity Networking (IIN) module (Schmid et al., 2020). MS1 and MS2 mass detection noise levels were set to 1.0 × 105 and 1.0 × 102 respectively, all m/z tolerances were set to 10 ppm, minimum highest intensity for chromatograph building and deconvolution was set to 1.0 × 107, and maximum peak duration range was set to 8 min. Ion identities (adducts or in-source fragments) were determined by first grouping features using the Metacorrelate module with the following parameters: RT tolerance set at 0.08 min, minimum height at 1.0 × 105, noise level at 1.0 × 104, and with correlation grouping parameters set to 5 minimum data points, 2 minimum data points on edge and Pearson correlation minimum feature shape correlation at 85% (minimum total correlation not checked). The IIN module in MZmine was then used to annotate adducts and simple fragment ions within correlation groupings on the assumption that correlated groupings represent fragments and adducts from the same parent ion. The parameters for this module were as follows: the m/z tolerance was set to 10 ppm, with a minimum height of 1.0 × 107, and a simple annotation library was used containing only [M+H]+ [M+Na]+, [M+NH4]+, and [M-H2O]+ as potential adducts and modifications, with a maximum charge of 1 and with a maximum of 2 molecules/cluster. Features were filtered from the data if they lacked annotation and/or MS2 scans.
Preprocessed features were networked using feature-based molecular network analysis as part of the GNPS FBMN online workflow (version release_28.2) (Nothias et al., 2020). MS2 spectra (nodes) were linked to form clusters in the network if they had at least 6 matched fragment ions and a cosine score greater than 0.75, or if they had annotated ion identities within correlation groupings. Default settings were used for the FBMN workflow with the following exceptions: Network TopK was set to 20, and Maximum Connected Component Size was set to 0 (unlimited). The resulting analysis was imported and visualized in Cytoscape v 3.9.1. Nodes were coloured based on ion identity. To predict the presence of shared mass motifs in the MS2 scans, the dataset was analyzed using MS2LDA (release_31) through the GNPS web interface (Wandy et al., 2018). The resulting mass motifs were imported into cytoscape and merged with FBMN data to visualize motif presence patterns. The network was then reduced by collapsing nodes linked as adducts/fragments either via the IIN module or by manual annotation of adducts and fragments which the IIN module had missed (by mathematical relationships and extract ion chromatogram peak shape comparison in Thermo Xcalibur).
Mass feature annotation
UPLC-HRMS/MS and nanoLC-HRMS/MS MS2 scans were compared to freely available libraries of experimentally derived MS2 data via the GNPS online interface (version 28.2). Matches were made if they had cosine similarity scores over 0.7, and at least 6 matched fragment ions. All libraries available via GNPS were selected by default, including the Massbank of North America and the GNPS natural products library. In addition to the above, CSI Finger-ID version 5.5.5 was utilized to interpret MS2 scans of the features of interest for in silico-based chemical formula and structural prediction (Dührkop et al., 2015, 2019; Hoffmann et al., 2021). CSI Finger-ID performs high-resolution isotope pattern analysis in combination with fragmentation tree analysis to assist in structure elucidation, comparing MS2 data to chemical structures harvested from online databases (such as PubChem, ChemSpider, KEGG, MetaCyc, MINE, CHEBI, UNPD, etc.). Predictions resulting from in silico analyses were interpreted with reference to a previously-compiled database of 300+ secondary metabolites identified and associated with Alternaria species in scientific literature, to filter out suggestions inappropriate to the taxa under study.
For features not annotated by either experimental or in silico derived comparisons listed above, identities were predicted through comparison of the pseudomolecular ion masses to the above-mentioned literature-based Alternaria secondary metabolite database, and MS2 spectra/mass motifs were manually examined to support the resulting annotations.
Whole-genome sequencing and assembly
Strains were grown on potato dextrose agar (PDA) plates at 25°C for 14 days. Genomic DNA extraction and short-read sequencing were performed as described in Dettman & Eggertson (2021). In brief, genomic DNA samples were sheared to approximately 350 bp fragment lengths using a Covaris M220 sonicator (Covaris, Massachusetts), PCR-free libraries were constructed with a NxSeq AmpFREE Low DNA Library Kit (Lucigen, Wisconsin) and sequenced with paired-end reads (2 × 150bp) on a Illumina NextSeq500 instrument (Illumina, California) using a NextSeq High Output Reagent Kit. For long-read sequencing with a MinION device (Oxford Nanopore Technologies, UK), libraries were prepared from genomic DNA using kit SQK-LSK109. Libraries were run for 48 h on a FLO-MIN106 flow cell following the manufacturer’s recommended protocols, with a resulting estimated read N50 of 13.8 Kb. Basecalling to fastq format was performed with GUPPY (4.2.2, https://nanoporetech.com/community) under default settings.
Genome assembly from short-read data was performed as described in Dettman & Eggertson (2021). In brief, raw reads were clipped of adapters and quality trimmed with TRIMMOMATIC (0.38, Bolger et al., 2014) and assembled with SPADES (3.12, Bankevich et al., 2012). Contigs were removed if they were less than 500 bp in length, or if BLASTn searches suggested they were bacterial or mitochondrial in nature. To estimate the completeness of genomes, the BUSCO (benchmarking universal single-copy orthologs; 4.0.6, Simão et al., 2015; Seppey et al., 2019) metric was determined for nucleotide-level assemblies using the ascomycota_odb10 ortholog dataset as reference.
Genome assembly from long-read data was performed with CANU (2.1.1; Koren et al., 2017) with genomeSize = 34 m, correctedErrorRate = 0.154, and minOverlapLength = 1,000 (all other settings were default). The assembly was polished by signal-level analyses using NANOPOLISH (0.13.2; https://github.com/jts/nanopolish) (Loman et al., 2015). Four successive rounds of error-correction were then performed with short-read data using PILON (v1.23; Walker et al., 2014). Chromosome 8 was identified via whole chromosome alignments against the A. solani NL03003 genome assembly, performed with progressiveMAUVE (2.4; Darling et al., 2004).
Gene prediction and annotation
Gene prediction and annotation were performed using FUNANNOTATE (1.8.7; https://github.com/nextgenusfs/funannotate; Palmer and Stajich., 2020) with methods described in Dettman and Eggertson, 2021. A modification made here was that evidence from SNAP and CodingQuarry were excluded during the EvidenceModeler step. Genome-wide gene predictions were run through antiSMASH (6.0, Blin et al., 2021) to identify secondary metabolite gene clusters. Repetitive and transposable elements were identified using the TransposableELMT wrapper script (1.0.0; https://github.com/PlantDr430/TransposableELMT) described in Wyka et al., 2021.
Search for dehydrocurvularin BGC homologs in Pleosporalean fungi
Searches of NCBI databases included full gene and coding sequence queries against the “nt” database using BLASTn, and protein sequence queries against the “nr” database using BLASTp. Additional searches against “refseq_genomes”, “refseq_representative_genomes”, and “wgs” databases did not expand the taxonomic diversity of hit subjects so the results are not presented. Next, genomes of Pleosporalean fungi that were publicly available from sequence repositories (e.g. NCBI, JGI Mycocosm) were downloaded (accessed 8 December 2021) and a custom, local BLAST database was created. The local Pleosporalean database was queried with BLASTn searches with a relaxed threshold of 70% minimum similarity to allow detection of potentially highly divergent genes. The dehydrocurvularin BGC was considered present in a genome if both of the polyketide synthase genes had full length hits.
Results
Taxonomy of Alternaria strains
Each of the Alternaria strains used in this study were micro-morphologically examined and found to produce branched chains of transverse and longitudinally septate, melanized conidia with tapering beaks. These micro-morphological observations, along with characteristics of culture growth, were consistent with taxonomic descriptions of species belonging to the small-spored Alternaria section Alternaria. Twelve strains were previously identified to species/lineage in Dettman & Eggertson (2021), Dettman & Eggertson (2022). For the remaining 24 strains, we sequenced three informative molecular markers (ASA-10, ASA-19, and rpb2) and assigned strains to species/lineage with phylogenetic analyses (data not shown) using the approach described in Dettman & Eggertson (2022). Overall, the majority of the 36 strains belonged to A. alternata (n = 23) or the A. arborescens species complex (n = 11), with one A. longipes strain, and one strain that could not be placed in a known lineage. An example of species determination via phylogenetic analysis for 15 representative strains is provided in Supplementary Figure S3).
Untargeted metabolomics profiling and detection of dehydrocurvularin-like mass features
Trends in the occurrence and frequency of secondary metabolite production between Alternaria strains were visualized using a consensus metabolomic phenotype heatmap (Figure 1). The phenotype heatmap was derived from UPLC-HRMS profiling of culture extracts from four media conditions and following data reduction steps, consisted of 52 representative mass features. Mass features with m/z matching the exact mass (within 5 ppm) of monoisotopic adducts of known Alternaria mycotoxins altenuene, altenusin, alternariol, alternariol monomethyl ether, altersetin, tricycloalternarene 2b, tenuazonic acid, 3-aipta (an analog of tenuazonic acid), tentoxin and dihydrotentoxin were annotated by analysis of MS2 fragmentation patterns using in silico and MS2-database derived comparisons. There were numerous mass features which shared detection frequencies and similar predicted chemical formulas to tricycloalternarene 2b, indicating they are likely related terpenoid compounds, most likely in the bi- or tri-cycloalternarene family, and were annotated as “TCA-associated”. Additionally, there were a number of unannotated mass features which did not share m/z with known Alternaria secondary metabolites. Among the unannotated signals, three isomeric mass features were detected from extracts of all isolates profiled, and were predicted to share a chemical formula of C12H10O5 (8 unsaturations predicted). We tentatively annotated these three signals as “unidentified hexaketide”.
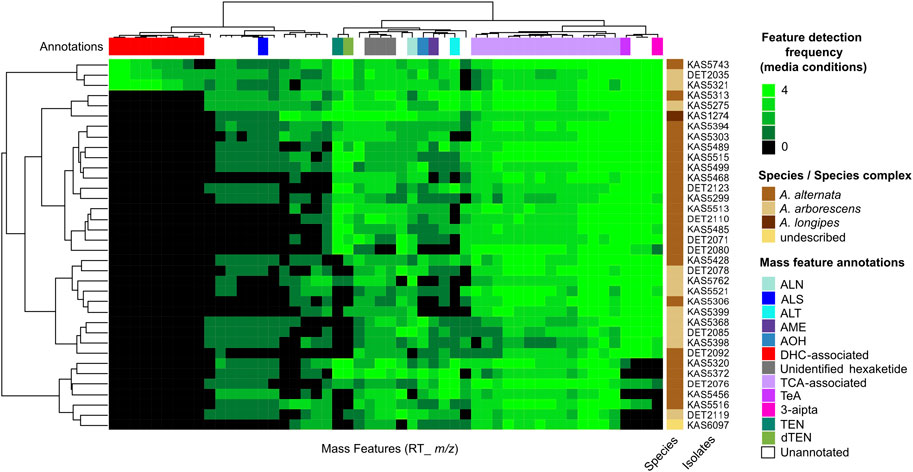
FIGURE 1. Untargeted metabolomics analysis of 36 Alternaria isolates, mainly from A. alternata and A. arborescens. Columns represent mass features, with heatmap values representing detection frequencies across four media conditions. Hierarchical cluster analysis was used to group isolates by the similarity of their mass feature detection frequencies (row dendrogram), and mass features by the similarity of their frequencies between isolates (column dendrogram). Annotations in the top colour bar are interpretations based on representative mass feature annotations: ALN, altersetin; ALS, altenusin; ALT, altenuene; AME, alternariol monomethyl ether; AOH, alternariol; DHC-associated, dehydrocurvularin and associated mass features annotated in this study; TCA, tricycloalternarene-associated mass features; TeA, tenuazonic acid-associated mass features; 3-aipta, 3-acetyl-5-isopropyltetramic acid; TEN, tentoxin; dTEN, dihydrotentoxin.
Detection patterns of the annotated mass features showed interesting patterns of variance between strains and between the secondary metabolites themselves: some were detected in all or nearly all 36 strains profiled, including altersetin (n = 34), alternariol (n = 32), alternariol monomethyl ether (n = 31), tenuazonic acid (n = 32), 3-aipta (n = 30) and the TCA-associated mass features (n = 36). Others were less frequently detected but were still produced by a majority of isolates, including tentoxin (n = 23), dihydrotentoxin (n = 26), altenuene (n = 26) and altenusin (n = 24). Hierarchical clustering of mass feature detection frequency patterns between isolates did not correlate with species or species complex identifications.
Interestingly, analysis of the chemical phenotypes indicated isolates DET2035, KAS5321 and KAS5743 produced a group of mass features which were not detected from any of the other isolates (Figure 1, red colour bar). This group of features included exact m/z matches (<5 ppm) to known masses from adducts of the ‘dehydrocurvularin’ family of molecules, namely curvularin, dehydrocurvularin, hydroxycurvularin, methoxycurvularin, sumalarin C, and cyclothiocurvularin (Table 1). Confirmation of dehydrocurvularin production was obtained through purification of the molecule from a scaled-up fermentation of DET2035 on solid CYS80 media, followed by comparison of 1D and 2D NMR and HRMS/MS experimental results to literature data (Hassan, 2007; Aly et al., 2010). A full accounting of the detailed purification and structural elucidation of dehydrocurvularin is provided in the Supplementary Section 4.0–4.10. A MS2 mass motif associated with the fragmentation of the dehydrocurvularin [M+H]+ ion was derived from MS/MS experimental data using both the MS2LDA mass motif analysis tool and by manual examination of MS2 spectra (Table 1—red font). Application of the derived dehydrocurvularin MS2 mass motif was essential for the association and interpretation of various other dehydrocurvularin-associated mass features.

TABLE 1. Relevant observed [M+H]+, associated MS2 fragment ions m/z and UV maxima from dehydrocurvularin-associated metabolites annotated in this study by UPLC-HRMS/MS-DAD.
A set of mass features containing the dehydrocurvularin MS2 mass motif and predicted to contain a sulphur atom (based upon isotopic ratios) were associated with the ‘sumalarins’, which is consistent with the hypothesis that structural differences in sumalarin analogs are located on the C11 thioether-linked functional group. The sumalarin C MS2 spectrum differed slightly from the dehydrocurvularin MS2 motif as it contained a fragment ion with a m/z of 203, and the 205 m/z ion was reduced to very low levels (rationalization of the sumalarin C annotation based on MS/MS experiments is provided in Supplementary Section 5.0–5.4. Curvularin fragmentation was also generally consistent with dehydrocurvularin MS2 motif, however some fragment ions were 2 mass units greater, consistent with an alkane bond between C10 and C11 as found in curvularin. Similarly, 11-hydroxycurvularin and 11-methoxycurvularin-associated mass fragment features had minor variations on the dehydrocurvularin MS2 motif. Our MS2 fragmentation motif findings agree with literature precedents of fragment ions with a m/z of 273, 195, 167, and 123 reported from dehydrocurvularin (Hassan, 2007; Jiang et al., 2008). Additionally, the observed UV maxima from dehydrocurvularin, 11-hydroxycurvularin, 11-methoxycurvularin and sumalarin C are consistent with reported UV absorbance spectra from the literature (Kobayashi et al., 1988; Lai et al., 1989; Meng et al., 2013).
Feature-based molecular network analysis of A. arborescens curvularins
Next, we processed an extract of a dehydrocurvularin-producing strain (DET2035) using a nanoLC-HRMS/MS in order to increase our chromatographic resolution and build an extensive library of MS2 scans from mass features potentially representing additional dehydrocurvularin-related molecules. Using the feature-based molecular networking workflow as part of the GNPS online suite of tools for MS2 analysis, we built a molecular network of dehydrocurvularin-associated mass features (Figure 2). Features matching dehydrocurvularin, curvularin, and sumalarin C were annotated by comparison to experimentally derived MS2 data from the GNPS and Massbank spectral libraries as part of the GNPS workflow. Additionally, mass features matching 11-hydroxycurvularin, 11-methoxycurvularin, cyclothiocurvularin, cyclosulfoxicurvularin and sumalarin A were annotated via in silico analysis of MS2 fragmentation patterns using SIRIUS/CSI-FingerID (relevant GNPS and CSI-FingerID annotation scores are provided in Supplementary Section 6.1, 6.2. Lastly, some mass features had no match to predicted structural fragmentation patterns, usually because the structures were not present in online libraries. Mass features associated with this category included dehydrocurvularin-S-cysteine, dehydrocurvularin-S-cysteinylglycine, dehydrocurvularin-S-(N)-acetyl-cysteine, cyclosulfoxicurvularin and sulfoxicurvularin, which were tentatively annotated using predicted molecular formula based on m/z and isotopic ratios as well as by comparison to dehydrocurvularin-associated MS2 fragmentation patterns.
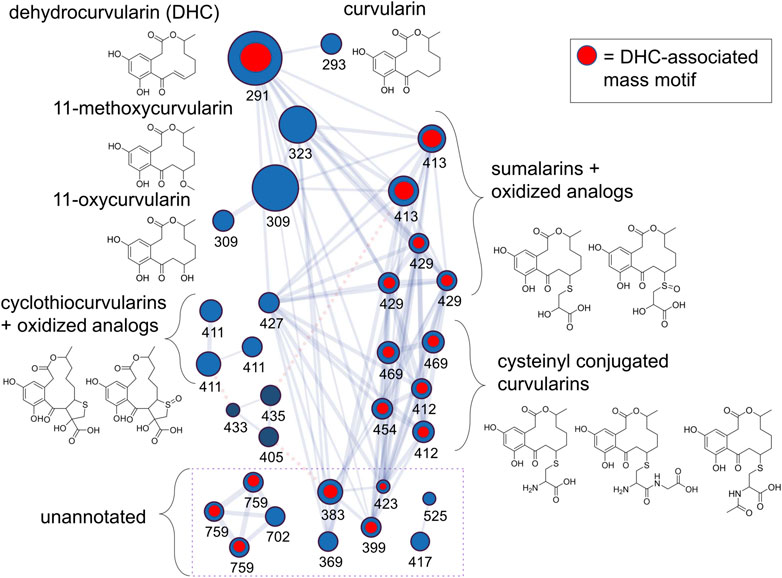
FIGURE 2. Dehydrocurvularins, sumalarins and related molecules annotated by analysis of MS2 fragmentation data from an extract of isolate DET2035. Nodes (circles) represent mass features, where size is proportional to the ion precursor intensity, and are labeled by their nominal mass (see Table 2 for exact masses and annotation details). Nodes are linked with a blue line if their MS2 spectra share a minimum of 6 fragment ions, and a cosine score >0.75. Nodes linked with a dotted red line represent putative adducts of the same molecule (linked via the ion identity networking module of MZMine2, see methods), and were not collapsed in the network in order to show connectivity between the cyclothiocurvularins and the other signals in the cluster. Blue nodes represent [M+H]+ adducts and dark blue nodes represent [M+Na]+ adducts. Structures drawn are hypothetical annotations based on exact mass and MS2-analysis. The network is derived from the GNPS feature based molecular network analysis workflow incorporating a mix of manually annotated ion identities, and MZMine2-derived ion identities.
The dehydrocurvularin MS2 motif was also detected in a number of mass features which were predicted to have nitrogen in their chemical formulas (Table 2), suggesting the production of dehydrocurvularin analogs with cysteine or modified cysteine-like functional groups at the C11 position (Figure 2). Additionally, at least eight mass features were detected at low intensities which either grouped with the molecular network and/or had MS2 fragmentation patterns matching the dehydrocurvularin MS2 motif. These eight features ranged in m/z from 369.13735 to 759.27629, and had unique peak shapes/retention times in our chromatography, suggesting the masses in the 700 + Da region were not a result of dimer adducts formed during ionization. We did not structurally elucidate these features and note that they are present only at relatively low intensities. Additionally, chlorinated forms of dehydrocurvularin, as have been reported from Alternaria and Cochliobolus isolates (Ghisalberti and Rowland 1993; Bashyal et al., 2017), were not observed in any extract in this study.
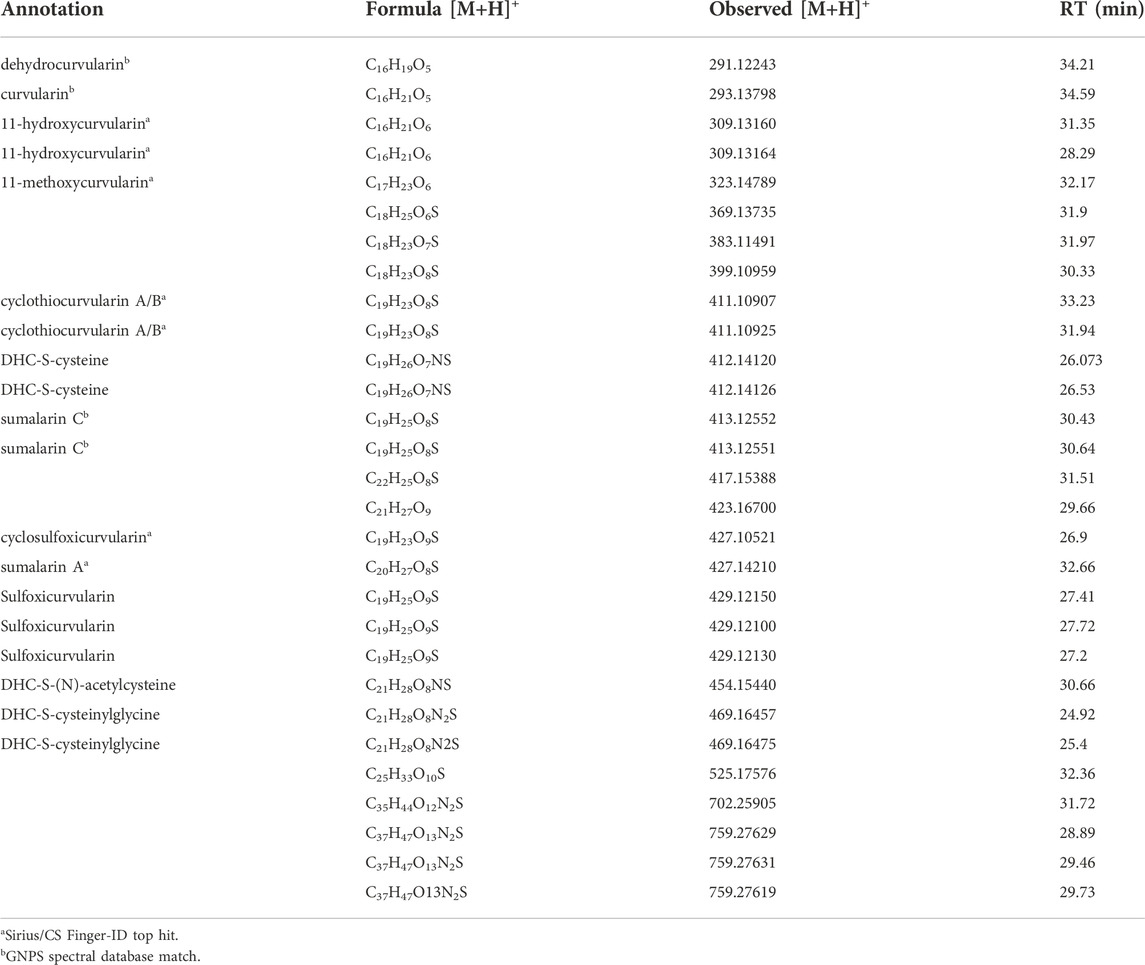
TABLE 2. Isolate-specific mass features linked to dehydrocurvularin (DHC) detection in this study. All mass features are [M+H]+ adducts, with observed m/z detected within 5 ppm accuracy to the exact mass of the predicted chemical formula. Retention times are from the nanoLC-HRMS based analysis of an extract from DET2035.
Whole-genome sequencing of dehydrocurvularin-producing strains
Illumina short-read sequence data were generated from genomic DNA extracted from the three dehydrocurvularin producing Alternaria strains (DET2035, KAS5321, and KAS5743). An average of 28.2 million reads were generated per sample and whole-genome assemblies were produced. NCBI accession numbers for the newly generated assemblies are as follows: DET2035 = JAERPI000000000; KAS5321 = JAERNY000000000; KAS5743 = JAERND000000000. The resulting assemblies had an average sequence coverage of 125X and quality statistics were similar to previous Alternaria assemblies (Dettman & Eggertson 2021). The genome sizes ranged from 33.0 to 34.1 Mb, with a mean N50 of 897.8 Kb, and BUSCO analyses indicated the assemblies were over 99% complete (for whole-genome assembly statistics refer to Supplementary Section 7).
Dehydrocurvularin BGC prediction and comparison to characterized BGCs
Analysis of ab initio gene prediction, annotation, and secondary metabolite gene cluster identification with antiSMASH revealed that each of the three genomes had seven to eight type I polyketide synthase (PKS) containing BGCs. Only a single PKS BGC in each genome contained a highly reducing PKS—non reducing PKS pair (that is fairly rare in nature), and based on similarity to known dehydrocurvularin BGCs for Aspergillus terreus and Alternaria cinerariae (Xu et al., 2013; Cochrane et al., 2015), each showed evidence for involvement in dehydrocurvularin production. The predicted dehydrocurvularin BGCs ranged in size from 40.6 to 41.5 Kb in length and included between nine and ten genes. Only six of these genes were shared consistently by all three of the dehydrocurvularin BGCs (Figure 3).
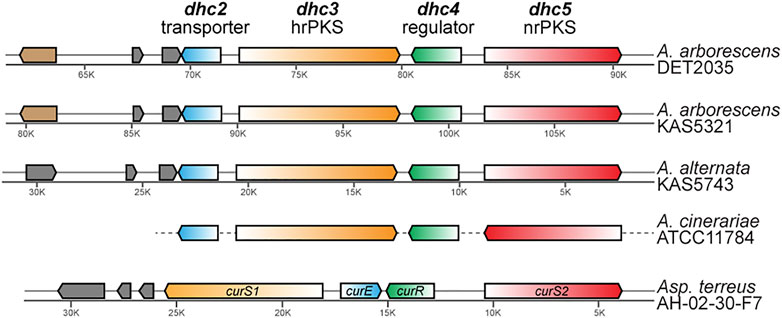
FIGURE 3. Comparison of homologous gene arrangement in the dehydrocurvularin BGC from the newly characterized Alternaria strains and previously characterized taxa (A. cinerariae and Asp. terreus). Genes with homologous sequence and predicted protein function are shown in the same color. The gene (light brown) that is unique to A. arborescens encodes for a glutamine-dependent NAD (+) synthetase. Genes shown in grey are not necessarily homologous and encode for hypothetical proteins that currently lack any functional predictions. Gene arrangements in A. cinerariae are as inferred by Cochrane et al. (2015).
Sequence comparison between the dehydrocurvularin BGC genes revealed clear homologous relationships for the four core genes identified here (Table 3). Functional annotation of conserved domains provided putative roles for four of the shared genes, all of which had plausible functions in dehydrocurvularin biosynthesis: 1) an efflux transporter in the major facilitator superfamily (MFS); 2) a highly reducing type I polyketide synthase (hrPKS); 3) a transcription factor with a Zn2-C6 fungal-type DNA-binding domain (regulator); and 4) a non-reducing type I polyketide synthase (nrPKS). Sequences of the new Alternaria dehydrocurvularin BGC genes were 94.4–95.5% similar to the A. cinerariae sequences. For consistency, we adopted the gene naming convention applied to A. cinerariae (dhc2, dhc3, dhc4, and dhc5) for the newly characterized biosynthetic genes in A. arborescens and A. alternata.
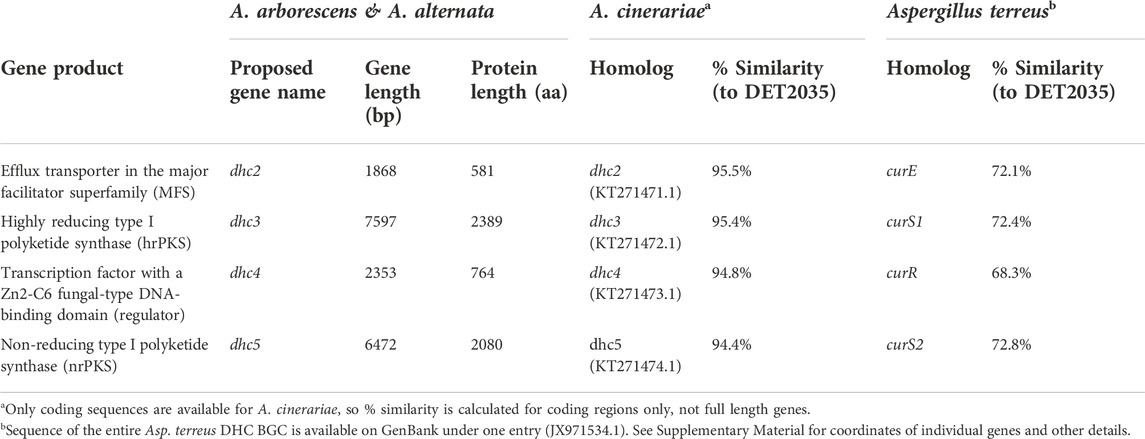
TABLE 3. Description and sequence homology comparison of four core genes involved in dehydrocurvularin (DHC) biosynthesis as observed from A. arboresecens and A. alternata strains and compared with published sequences from A. cinerariae and Asp. terreus.
The physical arrangement of the four core dhc genes within the BGC were quite similar between Alternaria spp. and the well-characterized Asp. terreus, with the main difference being the placement and transcriptional direction of dhc2 (Figure 3). Although the full length sequence of the A. cinerariae dehydrocurvularin BGC is not available, the gene arrangements inferred by Cochrane et al. (2015) are shown for comparison. When considering the entire BGC, the gene content of the two A. arborescens strains were more similar to each other than to that of the A. alternata strain.
Dehydrocurvularin BGC in other fungi
To explore the distribution of the dehydrocurvularin BGC in other fungal taxa, we searched the full NCBI nt database using each of the four core A. arborescens DET2035 dhc gene sequences as queries. BLASTn hits with greater than 80% sequence similarity were returned for only four taxa, all within the order Pleosporales: A. atra, A. cinerariae, A. solani, and Pyrenophora tritici-repentis (see Supplementary Section 8). Compared to BLASTn hits within Pleosporales (mean sequence similarity = 94.0%), the next closest matched sequences outside of Pleosporales had a much lower sequence similarity (mean = 74.8%) and were always from a species of Aspergillus. BLASTn searches using the known A. cinerariae dhc coding sequences returned similar results. For these reasons, we focused our attention on the dehydrocurvularin BGC in Pleosporales.
To expand the scope of our search, we gathered a panel of whole-genome assemblies of 281 Pleosporalean fungi from various public sources (for genome list see Supplementary Section 9). We maximized the phylogenetic diversity of taxa that were available at the time, with a total of 28 families, 65 genera, and 125 species being represented (including our three new Alternaria genomes). A local, custom database was created and BLASTn searches with A. arborescens DET2035 dhc gene sequences as queries revealed that only 25 of the 284 genomes (8.8%) contained the dehydrocurvularin BGC. Despite the wide diversity of taxa in our Pleosporalean genome database, the distribution of the dehydrocurvularin BGC was restricted to just one family, Pleosporaceae, and three genera within it: Alternaria, Pyrenophora, and Stemphylium (Table 4).
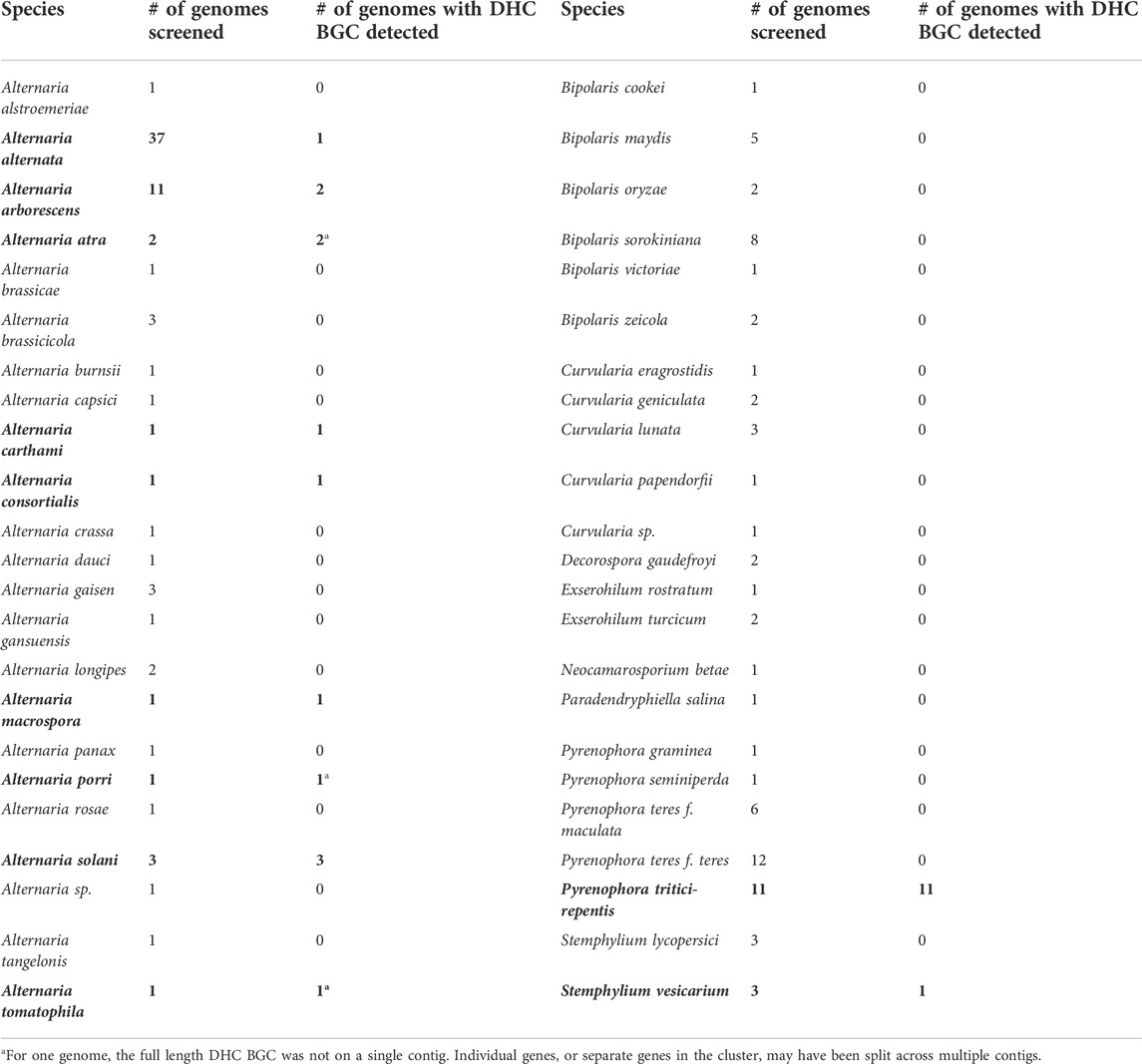
TABLE 4. BLASTn screening results for the dehydrocurvularin (DHC) BGC for the Pleosporaceae. Species with homologous BGCs are in bold text.
The dehydrocurvularin BGC was detected in only nine of the 23 screened species of Alternaria (A. alternata, A. arborescens, A. atra, A. carthami, A. consortialis, A. macrospora, A. porri, A. solani, and A. tomatophila), totalling ten species when the known BGC from A. cinerariae is included. In three dehydrocurvularin-positive Alternaria genomes, the BGC was split across multiple contigs (see Supplementary Section 10). In these cases, the cluster was typically close to the edge of a fairly short contig, suggesting that BGC contig splitting was caused by incomplete assemblies rather than the genes being located in non-adjacent regions of the genome. Our largest sample sizes were for A. alternata (n = 37) and A. arborescens (n = 11), however, all of the previously published genomes for A. alternata and A. arborescens lacked the dehydrocurvularin BGC. The only strains from these species that possessed the dehydrocurvularin BGC were the three strains that were denoted from our untargeted metabolomics screening (that were newly characterized and sequenced here). Our metabolic screening of strains, therefore, was essential for identifying the extremely rare curvularin producers in A. alternata and A. arborescens.
In contrast to the genus Alternaria, the pattern of dehydrocurvularin BGC distribution in the genus Pyrenophora was very consistent within the genus. The dehydrocurvularin BGC was found in only one of the screened species, Pyrenophora tritici-repentis, but it was detected in 100% of the 11 available genomes of that species. In Stemphylium, the dehydrocurvularin BGC was found in only one out of the six available genomes for the genus (S. vesicarium); only two species, S. lycopersici (n = 3) and S. vesicarium (n = 3) were represented in the database.
Genomic location of dehydrocurvularin BGC in Alternaria
The chromosomal genomic location of previously characterized dehydrocurvularin BGCs in A. cinerariae and Asp. terreus cannot be determined because chromosomal length genome sequences were not available for the studied strains (Xu et al., 2013; Cochrane et al., 2015). When comparing the positional coordinates of the dehydrocurvularin BGCs in positive genomes of the A. alternata and A. arborescens strains studied here, we found that the BGC was typically near a contig edge. The dehydrocurvularin BGC boundary was, on average, within the first or last 10.0% (SE 1.5%) of the contig sequence. Restricting the analysis to genomes with chromosome-level assemblies from long-read data revealed the BGC is located in subtelomeric regions of the chromosome. In the only available Alternaria chromosome-level assembly (A. solani NL03003), the dehydrocurvularin BGC is located in the first 1.7% of the 2.51 Mb chromosome 8 (Figure 4).
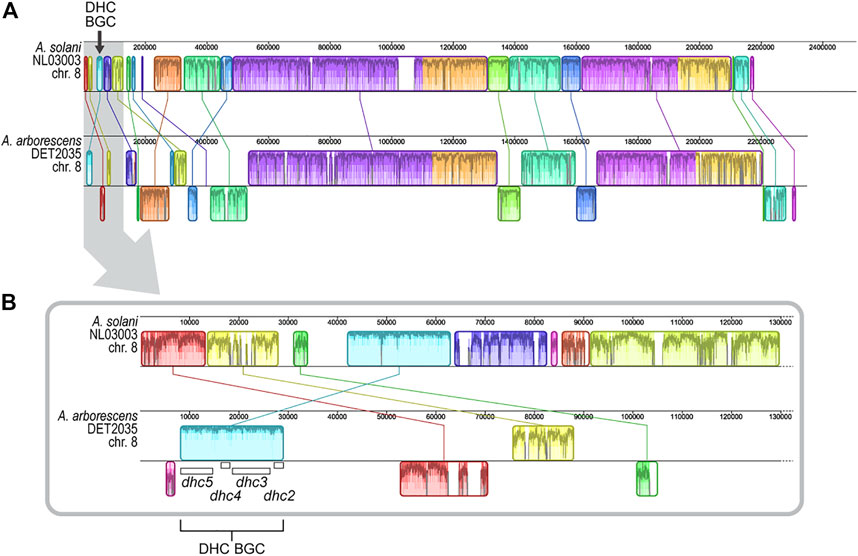
FIGURE 4. Alignment of genome regions from A. arborescens DET2035 and A. solani NL03003. Locally collinear blocks (LCBs) are shown in matching colors and linked by lines. LCBs shown below the horizontal centre line in A. arborescens DET2035 have inverse orientation in comparison to A. solani NL03003. Regions outside LCBs lack detectable homology between the genomes. Within each LCB, the height of the similarity plot corresponds to the average level of sequence conservation in that region, and sections in white are unique to that specific genome. (A) Alignment of full-length chromosome 8. (B) Alignment of the first 130 Kb of chromosome 8 (area highlighted in grey in panel A). The location of the four dhc genes are shown.
We generated 22.43 Gb of long-read sequence data (Oxford Nanopore) for A. arborescens DET2035 and produced a telomere-to-telomere assembly of chromosome 8 (NCBI accession number CP104802). Similar to A. solani, the dehydrocurvularin BGC in A. arborescens DET2035 is located 8.3 kb from the chromosome 8 telomeric edge. Full chromosome alignment of A. solani NL03003 and A. arborescens DET2035 (Figure 4) showed that the chromosomal structure was fairly well conserved, with large locally colinear blocks of sequence in the central regions. As expected, the subtelomeric regions appear more variable, with smaller conserved blocks and evidence of rearrangements, inversions, and non-homologous regions.
Next, we examined the genomic regions adjacent to the dehydrocurvularin BGC for evidence of transposable elements. Interestingly, fragments of a Gypsy transposon were immediately adjacent to the dehydrocurvularin BGC (telomere-proximal), with one of the long terminal repeats occurring only 318 nucleotides from the end of the dhc5 gene. On the other side of the dehydrocurvularin BGC (telomere-distal), fragments of a hAT transposon and a DDE transposase were found within 7.8–15.4 Kb of the dhc2 gene.
Discussion
To date, dehydrocurvularins have been isolated from a number of phytopathogenic fungal species, namely Alternaria, Aspergillus, Cochliobolus/Curvularia, Nectria, and Penicillium spp. (Musgrave, 1956; Hyeon et al., 1976; Robeson et al., 1985; Ghisalberti & Rowland, 1993; He et al., 2004; Zhan et al., 2004; Gutiérrez et al., 2005) and the geographical distribution of these instances spans most of the world (with production observed in isolates from Chile, Japan, Europe, Egypt, North America, and Korea) (Musgrave, 1956; Munro et al., 1967; Kusano et al., 2003; Gutiérrez et al., 2005; Aly et al., 2010; Jeon et al., 2010; Bashyal et al., 2017). Alternaria spp. that are reported to produce dehydrocurvularins include A. cinerariae, A. zinniae, A. macrospora, A. tomatophila, and an Alternaria sp. isolate from the leaves of Acaia mangium (Hyeon et al., 1976; Robeson & Strobel, 1981; Robeson et al., 1985; Vurro et al., 1998; Jeon et al., 2010). Additionally, two chlorinated dehydrocurvularin analogs were previously isolated from an Alternaria sp. isolated from the leaf tissue of Astragalus lentinginosus (Bashyal et al., 2017). The findings of the present study represent the first isolation of dehydrocurvularin from verified A. alternata or A. arborescens isolates. Additionally, this represents the first report of sumalarins A and C in any Alternaria species.
Dehydrocurvularin exhibits broad, non-specific phytotoxicity against rice (Oryza sativa), lettuce (Lactuca sativa), millet (Panicum miliaceum), common Zinnia (Zinnia elegans), Canadian thistle (Cirsium arvense), crabgrass (Digitaria sanguinalis), Noogoora burr (Xanthium occidentale) and a number of other agronomic weedy plant species (Robeson & Strobel, 1981; Vurro et al., 1998; Kusano et al., 2003; Gutiérrez et al., 2005; Jiang et al., 2008). Mechanistic phytotoxicity assays are lacking beyond one study which linked dehydrocurvularin exposure to disruption of mitosis in garlic root tip cells (Jiang et al., 2008). However, symptoms of dehydrocurvularin exposure have been linked to reductions in seed germination, root growth, epicotyl growth, cotyledon growth, and the appearance of necrotic lesions and/or chlorosis in leaf tissue assays (see above citations). Among the dehydrocurvularin analogs tested, dehydrocurvularin is consistently associated with the most pronounced phytotoxic response. In addition to phytotoxicity, dehydrocurvularin exhibits antifungal activity through suppression of sporulation and spore germination (Hyeon et al., 1976), modest antibacterial activity against several Gram-positive and Gram-negative bacteria (such as Bacillus subtilis, Staphylococcus aureus, and Escherichia coli; Caputo and Viola, 1977), and significant nematicidal activity against Meloidogyne graminicola (Xiang et al., 2020). Dehydrocurvularin also disrupted mitosis in sea urchin embryos (Kobayashi et al., 1988). Given the broad toxicity profile of this molecule, it is reasonable to conclude that dehydrocurvularin is not a host-specific toxin and will likely have a toxic effect upon the producing organism when produced at high enough levels (such as during in vitro cultivation). It is currently unknown if the dehydrocurvularins will impart increased disease virulence of the producing organism or whether production will provide a fitness advantage in some other context.
The untargeted metabolomics profiling experiments showed various dehydrocurvularin-associated molecules were produced in high abundance by our A. alternata and A. arborescens strains, including dehydrocurvularin, 11-hydroxycurvularin, 11-methoxycurvularin and sumalarin C. While curvularin was detected, it was not as a major product of DET2035. Various low intensity dehydrocurvularin-associated mass features were also detected, many of which were predicted to contain a sulfur atom and were annotated as cyclothiocurvularins, sulfoxicurvularins and nitrogen-containing curvularins. This large family of dehydrocurvularin-associated molecules likely arises from spontaneous addition of various nucleophiles to the enone group of dehydrocurvularin followed in some cases by further chemistry.
11-hydroxycurvularin and 11-methoxycurvularin are presumably formed by spontaneous addition of water or methanol to dehydrocurvularin, likely during handling of the fungal extracts as has been previously proposed (He et al., 2004). This spontaneous Michael addition is supported by the observation of duplicate sets of isomeric mass features at different retention times for each due to the non-stereo controlled formation of the α- and β-stereoisomers. Similarly, the sumalarins, originally isolated from Penicillium sumatrense MA-92 are proposed to arise from Michael addition of the cysteine metabolite 3-mercaptolactate, and may play a role in detoxification of dehydrocurvularin by the producing fungus during in vitro cultivation (Meng et al., 2013). The related cyclothiocurvularins have been isolated from Penicillium sp. isolate DRF2 (de Castro et al., 2016). The cyclothiocurvularins have unambiguously been shown to form from in vitro treatment of dehydrocurvularin with cysteine derived mercaptopyruvate. de Castro et al. (2016) also observed formation of the α- and β-stereoisomers from attack on the enone in vitro, which is consistent with our data showing duplicate isomeric mass features for cyclothiocurvularins. These data and prior precedent are consistent with spontaneous non-enzyme catalyzed Michael addition generating these metabolites.
Thiol-dehydrocurvularin Michael adducts can undergo additional processing. For example these thioethers are known to undergo oxidation to generate sulfoxides. Cyclothiocurvularin is readily oxidized to the sulfoxide cyclosulfoxicurvularin, which has been isolated along with cyclothiocurvularin (de Castro et al., 2016). Similarly we observed a mass feature consistent with this sulfoxide (Table 2). In addition we detected mass features consistent with oxidized sumalarin C (sulfoxicurvularin, Table 2). The possibility that sulfoxidized analogs are produced during sample handling and analysis cannot be ruled out at this time, and we note that these forms were detected only at low intensities in the fungal extracts.
We detect a number of mass features that were annotated as cysteinylglycine-, cysteine-, and N-acetylcysteine- Michael adducts of dehydrocurvularin. The detection of these nitrogen-containing dehydrocurvularins has not been described to date. We propose that these are a by-product of glutathione addition to dehydrocurvularin. Although we did not detect any m/z matching dehydrocurvularin-S-gluthathione in this experiment, cysteine, cysteinylglycine, and N-acetylcysteine conjugates are known to be generated from the glutathione adducts in fungi including Sclerotinia sclerotiorum (Chen et al., 2020).
Conjugation with thiol nucleophiles likely represents a detoxification pathway for dehydrocurvularin. Electrophilic compounds, like dehydrocurvularin, can be detoxified via the mercapturate conjugation pathway. For example, deoxynivalenol, an electrophilic Fusarium graminearum mycotoxin, is converted into deoxynivalenol-S-glutathione, deoxynivalenol-S-cysteine and deoxynivalenol-S-cysteinylglycine for detoxification by wheat tissue (Kluger et al., 2013). Similarly, the Brassicales plants defensive compounds containing electrophilic isothiocyanates are detoxified by pathogenic S. sclerotium isolates via the mercapturate conjugation pathway, resulting in detection of -cysteine, -cysteinylglycine, and -N-acetylcysteine conjugates (Chen et al., 2020). Our data thus suggests that a mercapturate conjugation detoxification pathway is also activated in the case of dehydrocurvularin-producing Alternaria strains.
Conjugation of dehydrocurvularin with mercaptolactate or mercaptopyruvate to form sumalarin C or cyclothiocurvularins, respectively, could represent an additional detoxification route. This has been proposed for cyclothiocurvularins because both cyclothiocurvularin and sulfoxicyclothiocurvularin have significantly reduced toxicity towards cancer cell lines in comparison to dehydrocurvularin (de Castro et al., 2016). Interestingly, sumalarin C was found to be comparably toxic towards cancer cell lines relative to dehydrocurvularin (Meng et al., 2013). An alternative explanation for the reported cytotoxicity of sumalarin C could be that the Michael addition of mercaptolactate to dehydrocurvularin is reversible in biological contexts, as has been found for the aculeatusquinone C conjugate of dehydrocurvularin (Shang et al., 2016). Similarly, 11-methoxycurvularin has been reported to be highly unstable and easily dehydrated to give dehydrocurvularin during separation on preparative TLC plates and on HPLC columns (Lai et al., 1991). The presence of numerous, potentially reversible conjugates of dehydrocurvularin raises the intriguing possibility that conjugated forms of dehydrocurvularin could act as “masked” or modified toxins which are metabolized or spontaneously revert to toxic dehydrocurvularin forms in host cells. This hypothesis must be tempered however by the observation that thiol-adducts are typically formed irreversibly by Michael addition to enones under biological relevant conditions (Jackson et al., 2017). Regardless, the multitude of proposed “detoxification” products detected in this experiment and previously reported in the literature (de Castro et al., 2016) raises the question of self-toxicity for dehydrocurvularin-producing isolates, and offers a potential rationale for selective pressures favoring loss of the dehydrocurvularin cluster in certain contexts.
The sporadic distribution of the dehydrocurvularin BGC across a wide diversity of taxa demonstrated how rare dehydrocurvularin production may be in the order Pleosporales. We discovered the dehydrocurvularin BGC in only one of 28 families (3.6%), and in only three of 65 genera (4.6%; Alternaria, Pyrenophora, and Stemphylium). Within genera, the prevalence of the dehydrocurvularin BGC was slightly higher; one of five Pyrenophora species (20%), and ten of the 24 Alternaria species (41.7%), had evidence of the dehydrocurvularin BGC in their genomes. Although this is the most comprehensive survey possible at the time, we fully acknowledge that discovery is limited to the breadth of the database, and that some dehydrocurvularin-positive taxa are likely to be missing. More intensive sampling at all taxonomic levels is required before we can propose predictive guidelines for which taxa may possess the dehydrocurvularin BGC. Interestingly, the dehydrocurvularin BGC was not detected in any of the eight examined genomes from the genus Curvularia, the namesake of curvularin.
The order and orientation of the four core genes within the dehydrocurvularin BGC were well conserved within the Alternaria, Pyrenophora, and Stemphylium genomes. The location of the dehydrocurvularin BGC in the greater genomic context was much more difficult to determine with certainty. Based on the positional coordinates of complete BGCs, the cluster was typically located near the terminal ends of a contig, and in some cases, the contig was so short that the dehydrocurvularin BGC comprised nearly the entire contig. This pattern suggests the dehydrocurvularin BGC is flanked by genomic regions that are difficult to assemble with short-read sequence data, such as regions with repetitive elements such as transposable elements or telomeres. To overcome this problem, we generated long-read sequence data and created a telomere-to-telomere assembly of the dehydrocurvularin BGC-containing chromosome from A. arborescens DET2035. Comparative analyses with the only other dehydrocurvularin-positive Alternaria strain with a chromosome-level assembly (A. solani NL03003) confirmed the dehydrocurvularin BGC was located in a subtelomeric region of chromosome 8 in both strains (Figure 4).
Subtelomeric regions of fungal chromosomes are commonly repeat-rich and gene-poor, with elevated rates of recombination and mutation, resulting in overall elevated rates of genomic evolution at chromosome ends (Brown et al., 2010; Rahnama et al., 2020). As a consequence, the high rate of gene content gain/loss within lineages can lead to high levels of gene content diversity and divergence between lineages in subtelomeric regions, thereby providing the raw material for adaptive evolution. Our preliminary comparative analysis was consistent with this prediction, in that subtelomeric regions did have smaller conserved blocks and more rearrangements, inversions, and non-homologous regions. We note that our sample size is only two chromosomes, and that additional full chromosome assemblies are needed to properly assess relative rates of subtelomeric variation in Alternaria.
Due to the sporadic occurrence of the dehydrocurvularin BGC as observed within A. arborescens and A. alternata, questions arise with regards to the origin of the dehydrocurvularin BGC within the genus. For example, is the pattern of the dehydrocurvularin BGC distribution in Pleosporalean fungi more consistent with horizontal gene transfer or vertical gain/loss during lineage evolution? In cases like Pyrenophora tritici-repentis, where all examined genomes have the dehydrocurvularin BGC, the most logical explanation is a single gain-of-function event that occurred shortly after the species diverged from the other Pyrenophora species. In contrast, the disjunct distribution observed in Alternaria and Stemphylium suggests both options are possible. These three genera are known to commonly infect the same agricultural hosts (e.g. wheat), creating opportunity for close physical contact. An intriguing observation made from the telomeric synteny comparison in this study (Figure 4) was that the dhc four-gene collinearity block appears to exhibit consistently less divergence compared to all other nearby collinear blocks. This observation contradicts our understanding of the high rate of mutation associated with subtelomeric regions, and may suggest either a recent horizontal transfer of the dehydrocurvularin BGC has occurred, or that this BGC region is under strong negative selective pressure. However, the mechanism by which genetic information would be transferred between genera is not clear, so at this point, vertical gain/loss during lineage evolution is the preferred hypothesis. More examples of dehydrocurvularin BGC-positive Alternaria and Stemphylium strains need to be discovered and chromosomal-level genome assemblies acquired, to facilitate more detailed analyses of the phylogenetic relationships among strains and of the chromosomal organization of the BGC themselves. Only then will the evolutionary history of these dehydrocurvularin BGCs be revealed.
Extensive whole genome-based screening for unique secondary metabolite biosynthetic gene clusters within species is, at the moment, cost prohibitive; although costs for whole-genome sequencing are on the decline. Untargeted metabolomics screening offers a more affordable option to screen larger numbers of strains. As a proof of concept for the genus Alternaria, the utility of untargeted metabolomics profiling has proven to be successful at detecting unique metabolite production within an intra-specific population—metabolite production that can be linked to biosynthetic gene clusters from informed selection-based whole-genome screening. A caveat of untargeted metabolomics screens of fungal populations is that not all secondary metabolite biosynthetic gene clusters are expressed under a single given in vitro cultivation condition. Utilizing multiple media conditions is therefore necessary to stimulate the maximum breadth of secondary metabolite diversity from the screening population as minor variations in environmental and/or nutritional conditions have the potential to impact the diversity of secondary metabolism in fungi (Bode et al., 2002; Bills et al., 2008). However, increasing the number of culture conditions quickly becomes a limiting factor, especially when sufficient replication is required for statistical analyses. In the case of this current study, an initial pilot study was first performed using eight different media and a subset of the strains (data not shown)—in the end four growth media were selected as they covered the breadth of secondary metabolite production as observed from the initial eight.
The untargeted metabolomics screening results generated in this study can be utilized for further informed strain selection for targeted whole-genome sequencing, in particular to investigate production trends for other Alternaria secondary metabolites. Interesting patterns of production frequency variance were observed between strains and between the secondary metabolites themselves; some were detected in most, but not all of the 36 strains profiled (i.e. altersetin, alternariol, alternariol monomethyl ether, and tenuazonic acid) while others were limited to subsets of the population and production frequency was limited to only a few media (i.e. tentoxin, dihydrotentoxin, altenuene and altenusin). This observed variability in secondary metabolite production is consistent with previous targeted secondary metabolite screening of A. alternata and A. arborescens populations (Patriarca et al., 2019). However from our screening results, it is unclear if the non-producing strains lack the associated BGC in the genome; or if the BGC resides in a subtelomeric region of the chromosome and due to transposable element insertion has been pseudogenized and rendered non-functional; or if the growth conditions provided were insufficient to trigger expression of the BGC. Metabolomics guided strain selection for whole-genome sequencing (both short read and long read), chromosomal level assembly, and BGC comparison between producing and non-producing strains will provide the necessary insight to answer these questions. Understanding the underlying mechanism to account for the variability in production of alternariol, alternariol methyl ether, tenuazonic acid, and tentoxin, is of particular interest as these mycotoxins contaminate agricultural products and are of concern for regulatory and advisory agencies such as the European Food Safety Authority (EFSA, 2011; Solfizzo, 2017). The biosynthetic gene clusters for each of these mycotoxins of concern are currently known (Saha et al., 2012; Li et al., 2016; Sun et al., 2022). Additional research efforts focused towards BGC comparison as outlined above are currently underway, along with an expanded strain screening of Alternaria section Alternaria to explore the occurrence of unique secondary metabolite production.
Data availability statement
The datasets presented in this study can be found in online repositories. The names of the repository/repositories and accession number(s) can be found in the article/Supplementary Material.
Author contributions
JD and DO designed the project. NV and QE prepared and extracted fungal culture plates. AS and SS acquired UPLC-HRMS/DAD data and provided chemistry technical support. TW, NV, and DO performed metabolomics analyses and mass feature annotations from UPLC-HRMS data. TW performed molecular networking and nanoLC-HRMS data analysis. NV performed scaled up cultivation of DET2035 and purified dehydrocurvularin. NV and CB performed NMR confirmation of dehydrocurvularin. SS provided HRMS/MS confirmation of sumalarin C. QE extracted DNA and performed Sanger sequencing. JD performed whole genome assembly, genomic database creation and queries, phylogenetic and comparative genomics analyses. NK performed annotation of whole genome assemblies. NV and JD performed dehydrocurvularin biosynthetic gene cluster comparisons. CB, JD, and DO supervised the project. CB supervised NV. JD supervised QE and NK. DO supervised TW, NV, SS, and AS. JD and DO provided funding support. TW, NV, JD and DO wrote the manuscript. TW, SS, CB, JD, and DO edited the manuscript.
Funding
Funding for this research was provided by the Science and Technology Branch of Agriculture and Agri-Food Canada (Project J-002272 “Fungal and Bacterial Biosystematics—bridging taxonomy and ‘omics’ technology in agricultural research and regulation”) to JD and DO. CB is supported by NSERC (RGPIN 2019-06859).
Acknowledgments
We thank Kasia Dadej, and the Molecular Technologies Laboratory (MTL) at the Ottawa Research and Development Centre of Agriculture and Agri-Food Canada for sequencing support.
Conflict of interest
The authors declare that the research was conducted in the absence of any commercial or financial relationships that could be construed as a potential conflict of interest.
Publisher’s note
All claims expressed in this article are solely those of the authors and do not necessarily represent those of their affiliated organizations, or those of the publisher, the editors and the reviewers. Any product that may be evaluated in this article, or claim that may be made by its manufacturer, is not guaranteed or endorsed by the publisher.
Supplementary material
The Supplementary Material for this article can be found online at: https://www.frontiersin.org/articles/10.3389/fmolb.2022.1038299/full#supplementary-material
References
Aly, A. H., Debbab, A., Edrada-Ebel, R. A., Müller, W. E. G., Kubbutat, M. H. G., Wray, V., et al. (2010). Protein kinase inhibitors and other cytotoxic metabolites from the fungal endophyte Stemphylium botryosum isolated from Chenopodium album. Mycosphere 1, 153–162.
Bankevich, A., Nurk, S., Antipov, D., Gurevich, A. A., Dvorkin, M., Kulikov, A. S., et al. (2012). SPAdes: A new genome assembly algorithm and its applications to single-cell sequencing. J. Comput. Biol. 19, 455–477. doi:10.1089/cmb.2012.0021
Bashyal, B. P., Wijeratne, E. M. K., Tillotson, J., Arnold, A. E., Chapman, E., and Gunatilaka, A. A. L. (2017). Chlorinated dehydrocurvularins and alterperylenepoxide A from Alternaria sp. AST0039, a fungal endophyte of Astragalus lentiginosus. J. Nat. Prod. 80, 427–433. doi:10.1021/acs.jnatprod.6b00960
Bills, G. F., Platas, G., Fillola, A., Jiménez, M. R., Collado, J., Vicente, F., et al. (2008). Enhancement of antibiotic and secondary metabolite detection from filamentous fungi by growth on nutritional arrays. J. Appl. Microbiol. 104, 1644–1658. doi:10.1111/j.1365-2672.2008.03735.x
Blin, K., Shaw, S., Kloosterman, A. M., Charlop-Powers, Z., van Wezel, G. P., Medema, M. H., et al. (2021). antiSMASH 6.0: improving cluster detection and comparison capabilities. Nucleic Acids Res. 49, W29–W35. doi:10.1093/NAR/GKAB335
Bode, H. B., Bethe, B., Höfs, R., and Zeeck, A. (2002). Big effects from small changes: Possible ways to explore nature's chemical diversity. ChemBioChem 3, 619–627. doi:10.1002/1439-7633(20020703)3:7<619::AID-CBIC619>3.0.CO;2-9
Bolger, A. M., Lohse, M., and Usadel, B. (2014). Trimmomatic: A flexible trimmer for Illumina sequence data. Bioinformatics 30, 2114–2120. doi:10.1093/bioinformatics/btu170
Brown, C. A., Murray, A. W., and Verstrepen, K. J. (2010). Rapid expansion and functional divergence of subtelomeric gene families in yeasts. Curr. Biol. 20, 895–903. doi:10.1016/j.cub.2010.04.027
Caputo, O., and Viola, F. (1977). Isolation of α, β–dehydrocurvularin from Aspergillus aureofulgens. Planta Med. 31, 31–32. doi:10.1055/s-0028-1097485
Chen, J., Ullah, C., Reichelt, M., Beran, F., Yang, Z.-L., Gershenzon, J., et al. (2020). The phytopathogenic fungus Sclerotinia sclerotiorum detoxifies plant glucosinolate hydrolysis products via an isothiocyanate hydrolase. Nat. Commun. 11, 3090. doi:10.1038/s41467-020-16921-2
Cochrane, R. V. K., Gao, Z., Lambkin, G. R., Xu, W., Winter, J. M., Marcus, S. L., et al. (2015). Comparison of 10, 11-dehydrocurvularin polyketide synthases from Alternaria cinerariae and Aspergillus terreus highlights key structural motifs. ChemBioChem 16, 2479–2483. doi:10.1002/cbic.201500428
Darling, A. C. E., Mau, B., Blattner, F. R., and Perna, N. T. (2004). Mauve: Multiple alignment of conserved genomic sequence with rearrangements. Genome Res. 14, 1394–1403. doi:10.1101/gr.2289704
De Castro, M. V., Ióca, L. P., Williams, D. E., Costa, B. Z., Mizuno, C. M., Santos, M. F. C., et al. (2016). Condensation of macrocyclic polyketides produced by Penicillium sp. DRF2 with mercaptopyruvate represents a new fungal detoxification pathway. J. Nat. Prod. 79, 1668–1678. doi:10.1021/ACS.JNATPROD.6B00295
Dettman, J. R., and Eggertson, Q. (2022). New molecular markers for distinguishing the main phylogenetic lineages within Alternaria section Alternaria. Can. J. Plant Pathology 0, 754–766. doi:10.1080/07060661.2022.2061605
Dettman, J. R., and Eggertson, Q. (2021). Phylogenomic analyses of Alternaria section Alternaria: A high-resolution, genome-wide study of lineage sorting and gene tree discordance. Mycologia 113, 1218–1232. doi:10.1080/00275514.2021.1950456
Dührkop, K., Fleischauer, M., Ludwig, M., Aksenov, A. A., Melnik, A. V., Meusel, M., et al. (2019). Sirius 4: A rapid tool for turning tandem mass spectra into metabolite structure information. Nat. Methods 16, 299–302. doi:10.1038/s41592-019-0344-8
Dührkop, K., Shen, H., Meusel, M., Rousu, J., and Böcker, S. (2015). Searching molecular structure databases with tandem mass spectra using CSI:FingerID. Proc. Natl. Acad. Sci. U. S. A. 112, 12580–12585. doi:10.1073/pnas.1509788112
EFSA (2011). Scientific Opinion on the risks for animal and public health related to the presence of Alternaria toxins in feed and food. EFSA J. 9, 2407. doi:10.2903/j.efsa.2011.2407
Ghisalberti, E. L., and Rowland, C. Y. (1993). 6-chlorodehydrocurvularin, a new metabolite from Cochliobolus spicifer. J. Nat. Prod. 56, 2175–2177. doi:10.1021/np50102a022
Grum-Grzhimaylo, A. A., Georgieva, M. L., Bondarenko, S. A., Debets, A. J. M., and Bilanenko, E. N. (2016). On the diversity of fungi from soda soils. Fungal Divers. 76, 27–74. doi:10.1007/s13225-015-0320-2
Gu, Z., Eils, R., and Schlesner, M. (2016). Complex heatmaps reveal patterns and correlations in multidimensional genomic data. Bioinformatics 32, 2847–2849. doi:10.1093/bioinformatics/btw313
Gutiérrez, M., Theoduloz, C., Rodríguez, J., Lolas, M., and Schmeda-Hirschmann, G. (2005). Bioactive metabolites from the fungus Nectria galligena, the main apple canker agent in Chile. J. Agric. Food Chem. 53, 7701–7708. doi:10.1021/jf051021l
Hassan, A. E. H. A. (2007). Novel natural products from endophytic fungi of Egyptian medicinal plants - chemical and biological characterization. Düsseldorf, Germany: Dusseldorf University. [Doctoral dissertation]. [Dusseldorf].
He, J., Wijeratne, E. M. K., Bashyal, B. P., Zhan, J., Seliga, C. J., Liu, M. X., et al. (2004). Cytotoxic and other metabolites of Aspergillus inhabiting the rhizosphere of Sonoran Desert plants. J. Nat. Prod. 67, 1985–1991. doi:10.1021/np040139d
Hicks, C., Witte, T. E., Sproule, A., Lee, T., Shoukouhi, P., Popovic, Z., et al. (2021). Evolution of the ergot alkaloid biosynthetic gene cluster results in divergent mycotoxin profiles in Claviceps purpurea sclerotia. Toxins 13, 861. doi:10.3390/toxins13120861
Hoffmann, M. A., Nothias, L.-F., Ludwig, M., Fleischauer, M., Gentry, E. C., Witting, M., et al. (2021). Assigning confidence to structural annotations from mass spectra with COSMIC. bioRxiv. [Preprint]. doi:10.1101/2021.03.18.435634
Hyeon, S.-B., Ozaki, A., Suzuki, A., and Tamura, S. (1976). Isolation of α β -dehydrocurvularin and β -hydroxycurvularin from Alternaria tomato as sporulation-suppressing factors. Agric. Biol. Chem. 40, 1663–1664. doi:10.1080/00021369.1976.10862283
Jackson, P. A., Widen, J. C., Harki, D. A., and Brummond, K. M. (2017). Covalent modifiers: A chemical perspective on the reactivity of α, β unsaturated carbonyls with thiols via hetero-michael addition reactions. J. Med. Chem. 60, 839–885. doi:10.1021/acs.jmedchem.6b00788
Jeon, Y.-T., Ryu, K.-H., Kang, M.-K., Park, S.-H., Yun, H., Qt, P., et al. (2010). Alternariol monomethyl ether and α, β-dehydrocurvularin from endophytic fungi Alternaria spp. inhibit appressorium formation of Magnaporthe grisea. J. Korean Soc. Appl. Biol. Chem. 53, 39–42. doi:10.3839/jksabc.2010.007
Jiang, S.-J., Qiang, S., Zhu, Y.-Z., and Dong, Y.-F. (2008). Isolation and phytotoxicity of a metabolite from Curvularia eragrostidis and characterisation of its modes of action. Ann. Appl. Biol. 152, 103–111. doi:10.1111/j.1744-7348.2007.00202.x
Kluger, B., Bueschl, C., Lemmens, M., Berthiller, F., Häubl, G., Jaunecker, G., et al. (2013). Stable isotopic labelling-assisted untargeted metabolic profiling reveals novel conjugates of the mycotoxin deoxynivalenol in wheat. Anal. Bioanal. Chem. 405, 5031–5036. doi:10.1007/s00216-012-6483-8
Kobayashi, A., Hino, T., Yata, S., Itoh, T. J., Sato, H., and Kawazu, K. (1988). Unique spindle poisons, curvularin and its derivatives, isolated from Penicillium species. Agric. Biol. Chem. 52, 3119–3123. doi:10.1080/00021369.1988.10869190
Koren, S., Walenz, B. P., Berlin, K., Miller, J. R., Bergman, N. H., and Phillippy, A. M. (2017). Canu: Scalable and accurate long-read assembly via adaptive k-mer weighting and repeat separation. Genome Res. 27, 722–736. doi:10.1101/gr.215087.116
Kusano, M., Nakagami, K., Fujioka, S., Kawano, T., Shimada, A., and Kimura, Y. (2003). βγ-dehydrocurvularin and related compounds as nematicides of Pratylenchus penetrans from the fungus Aspergillus sp. Biosci. Biotechnol. Biochem. 67, 1413–1416. doi:10.1271/bbb.67.1413
Lai, S., Shizuri, Y., Yamamura, S., Kawai, K., and Furukawa, H. (1991). New curvularin-type metabolites from the hybrid strain ME 0005 derived from Penicillium citreo-viride B. IFO 4692 and 6200. Bull. Chem. Soc. Jpn. 64, 1048–1050. doi:10.1246/bcsj.64.1048
Lai, S., Shizuri, Y., Yamamura, S., Kawai, K., Terada, Y., and Furukawa, H. (1989). Novel curvularin-type metabolites of a hybrid strain ME 0005 derived from Penicillium citreo-viride B. IFO 6200 and 4692. Tetrahedron Lett. 30, 2241–2244. doi:10.1016/S0040-4039(00)99658-8
Li, Y.-H., Han, W.-J., Gui, X.-W., Wei, T., Tang, S.-Y., and Jin, J.-M. (2016). Putative nonribosomal peptide synthetase and cytochrome P450 genes responsible for tentoxin biosynthesis in Alternaria alternata ZJ33. Toxins 8, 234. doi:10.3390/toxins8080234
Loman, N. J., Quick, J., and Simpson, J. T. (2015). A complete bacterial genome assembled de novo using only nanopore sequencing data. Nat. Methods 12, 733–735. doi:10.1038/nmeth.3444
Lou, J., Fu, L., Peng, Y., and Zhou, L. (2013). Metabolites from Alternaria fungi and their bioactivities. Molecules 18, 5891–5935. doi:10.3390/molecules18055891
Meena, M., Gupta, S. K., Swapnil, P., Zehra, A., Dubey, M. K., and Upadhyay, R. S. (2017). Alternaria toxins: Potential virulence factors and genes related to pathogenesis. Front. Microbiol. 8, 1451. doi:10.3389/fmicb.2017.01451
Meng, L.-H., Li, X.-M., Lv, C.-T., Li, C.-S., Xu, G.-M., Huang, C.-G., et al. (2013). Sulfur-containing cytotoxic curvularin macrolides from Penicillium sumatrense ma-92, a fungus obtained from the rhizosphere of the mangrove Lumnitzera racemosa. J. Nat. Prod. 76, 2145–2149. doi:10.1021/np400614f
Munro, H. D., Musgrave, O. C., and Templeton, R. (1967). Curvularin. Part V. The compound C16H18O5, αβ-dehydrocurvularin. J. Chem. Soc. C 0, 947–948. doi:10.1039/J39670000947
Musgrave, O. C. (1956). Curvularin. Part I. Isolation and partial characterisation of a metabolic product from a new species of Curvularia. J. Chem. Soc. 828, 4301–4305. doi:10.1039/JR9560004301
Nothias, L. F., Petras, D., Schmid, R., Dührkop, K., Rainer, J., Sarvepalli, A., et al. (2020). Feature-based molecular networking in the GNPS analysis environment. Nat. Methods 17, 905–908. doi:10.1038/s41592-020-0933-6
Palmer, J. M., and Stajich, J. (2020). Funannotate v1.8.1: Eukaryotic genome annotation. Zenodo. doi:10.5281/ZENODO.4054262
Patriarca, A., da Cruz Cabral, L., Pavicich, M. A., Nielsen, K. F., and Andersen, B. (2019). Secondary metabolite profiles of small-spored Alternaria support the new phylogenetic organization of the genus. Int. J. Food Microbiol. 291, 135–143. doi:10.1016/j.ijfoodmicro.2018.11.022
Pluskal, T., Castillo, S., Villar-Briones, A., and Orešič, M. (2010). MZmine 2: Modular framework for processing, visualizing, and analyzing mass spectrometry-based molecular profile data. BMC Bioinforma. 11, 395. doi:10.1186/1471-2105-11-395
Rahnama, M., Novikova, O., Starnes, J. H., Zhang, S., Chen, L., and Farman, M. L. (2020). Transposon-mediated telomere destabilization: A driver of genome evolution in the blast fungus. Nucleic Acids Res. 48, 7197–7217. doi:10.1093/nar/gkaa287
Robeson, D. J., Strobel, G. A., and Strange, R. N. (1985). The identification of a major phytotoxic component from Alternaria macrospora as αβ-dehydrocurvularin. J. Nat. Prod. 48, 139–141. doi:10.1021/np50037a028
Robeson, D. J., and Strobel, G. A. (1981). αβ-dehydrocurvularin and curvularin from Alternaria cinerariae. Z. Naturforsch. C 36, 1081–1083. doi:10.1515/znc-1981-11-1231
Rotem, J. (1994). The genus Alternaria: Biology, epidemiology, and pathogenicity. St. Paul, MN, USA: American Phytopathological Society Press.
Saha, D., Fetzner, R., Burkhardt, B., Podlech, J., Metzler, M., Dang, H., et al. (2012). Identification of a polyketide synthase required for alternariol (AOH) and alternariol-9-methyl ether (AME) formation in Alternaria alternata. PLoS ONE 7, e40564. doi:10.1371/journal.pone.0040564
Schmid, R., Petras, D., Nothias, L. F., Wang, M., Aron, A. T., Jagels, A., et al. (2020). Ion identity molecular networking for mass spectrometry-based metabolomics in the GNPS environment. Nat. Commun. 21, 3832. doi:10.1038/s41467-021-23953-9
Seppey, M., Manni, M., and Zdobnov, E. M. (2019). “BUSCO: Assessing genome assembly and annotation completeness,” in Methods mol. Biol. (New Jersey, United States: Humana Press), 227–245. doi:10.1007/978-1-4939-9173-0_14
Shang, Z., Khalil, Z., Li, L., Salim, A. A., Quezada, M., Kalansuriya, P., et al. (2016). Roseopurpurins: Chemical diversity enhanced by convergent biosynthesis and forward and reverse michael additions. Org. Lett. 18, 4340–4343. doi:10.1021/acs.orglett.6b02099
Simão, F. A., Waterhouse, R. M., Ioannidis, P., Kriventseva, E. V., and Zdobnov, E. M. (2015). BUSCO: Assessing genome assembly and annotation completeness with single-copy orthologs. Bioinformatics 31, 3210–3212. doi:10.1093/bioinformatics/btv351
Solfizzo, M., A, B., and M, P. (2017). Recent advances on Alternaria mycotoxins. Curr. Opin. Food Sci. 17, 57–61. doi:10.1016/j.cofs.2017.09.012
Sun, F., Cao, X., Yu, D., Hu, D., Yan, Z., Fan, Y., et al. (2022). AaTAS1 and AaMFS1 genes for biosynthesis or efflux transport of tenuazonic acid and pathogenicity of Alternaria alternata. Mol. Plant. Microbe. Interact. 35, 416–427. doi:10.1094/MPMI-12-21-0300-R
Tsuge, T., Harimoto, Y., Akimitsu, K., Ohtani, K., Kodama, M., Akagi, Y., et al. (2013). Host-selective toxins produced by the plant pathogenic fungus Alternaria alternata. FEMS Microbiol. Rev. 37, 44–66. doi:10.1111/j.1574-6976.2012.00350.x
Vurro, M., Evidente, A., Andolfi, A., Chiara Zonno, M., Giordano, F., and Motta, A. (1998). Brefeldin A and α, β-dehydrocurvularin, two phytotoxins from Alternaria zinniae, a biocontrol agent of Xanthium occidentale. Plant Sci. 138, 67–79. doi:10.1016/S0168-9452(98)00131-9
Walker, B. J., Abeel, T., Shea, T., Priest, M., Abouelliel, A., Sakthikumar, S., et al. (2014). Pilon: An integrated tool for comprehensive microbial variant detection and genome assembly improvement. PLoS ONE 9, e112963. doi:10.1371/journal.pone.0112963
Wandy, J., Zhu, Y., Van Der Hooft, J. J. J., Daly, R., Barrett, M. P., and Rogers, S. (2018). Ms2lda.org: Web-based topic modelling for substructure discovery in mass spectrometry. Bioinformatics 34, 317–318. doi:10.1093/bioinformatics/btx582
Witte, T. E., Harris, L. J., Nguyen, H. D. T., Hermans, A., Johnston, A., Sproule, A., et al. (2021a). Apicidin biosynthesis is linked to accessory chromosomes in Fusarium poae isolates. BMC Genomics 22, 591–618. doi:10.1186/s12864-021-07617-y
Witte, T. E., and Overy, D. P. (2022). “Untargeted metabolomic profiling of fungal species populations,” in Proteomics in systems biology: Methods and protocols. Editor J. Geddes-McAlister (Berlin, Germany: Springer Nature), 2456.
Witte, T. E., Villeneuve, N., Boddy, C. N., and Overy, D. P. (2021b). Accessory chromosome-acquired secondary metabolism in plant pathogenic fungi: The evolution of biotrophs into host-specific pathogens. Front. Microbiol. 12, 664276. doi:10.3389/fmicb.2021.664276
Woudenberg, J. H. C., Groenewald, J. Z., Binder, M., and Crous, P. W. (2013). Alternaria redefined. Stud. Mycol. 75, 171–212. doi:10.3114/sim0015
Woudenberg, J. H. C., Truter, M., Groenewald, J. Z., and Crous, P. W. (2014). Large-spored Alternaria pathogens in section Porri disentangled. Stud. Mycol. 79, 1–47. doi:10.1016/j.simyco.2014.07.003
Wyka, S. A., Mondo, S. J., Liu, M., Dettman, J., Nalam, V., and Broders, K. D. (2021). Whole-genome comparisons of ergot fungi reveals the divergence and evolution of species within the genus Claviceps are the result of varying mechanisms driving genome evolution and host range expansion. Genome Biol. Evol. 13, evaa267. doi:10.1093/gbe/evaa267
Xiang, C., Liu, Y., Liu, S.-M., Huang, Y.-F., Kong, L.-A., Peng, H., et al. (2020). αβ-Dehydrocurvularin isolated from the fungus Aspergillus welwitschiae effectively inhibited the behaviour and development of the root-knot nematode Meloidogyne graminicola in rice roots. BMC Microbiol. 20, 48. doi:10.1186/s12866-020-01738-2
Xu, Y., Espinosa-Artiles, P., Schubert, V., Xu, Y., Zhang, W., Lin, M., et al. (2013). Characterization of the biosynthetic genes for 10, 11-dehydrocurvularin, a heat shock response-modulating anticancer fungal polyketide from Aspergillus terreus. Appl. Environ. Microbiol. 79, 2038–2047. doi:10.1128/AEM.03334-12
Keywords: Alternaria, fungal plant pathogen, dehydrocurvularin, untargeted metabolomics, mass spectrometry, secondary metabolites, biosynthetic gene cluster, accessory region
Citation: Witte TE, Villenueve N, Shields SW, Sproule A, Eggertson Q, Kim NE, Boddy CN, Dettman JR and Overy DP (2022) Untargeted metabolomics screening reveals unique secondary metabolite production from Alternaria section Alternaria. Front. Mol. Biosci. 9:1038299. doi: 10.3389/fmolb.2022.1038299
Received: 06 September 2022; Accepted: 20 October 2022;
Published: 24 November 2022.
Edited by:
Young Hae Choi, Leiden University, NetherlandsReviewed by:
Rachel Basques Caligiorne, Grupo Santa Casa BH, BrazilRabih Darwiche, Laronde Inc., United States
Copyright © 2022 Christopher N. Boddy, and His Majesty the King in Right of Canada, as represented by the Minister of Agriculture and Agri-Food Canada for the contribution of Thomas E. Witte, Nicolas Villeneuve, Sam W. Shields, Amanda Sproule, Quinn Eggertson, Natalie E. Kim, Jeremy R. Dettman and David P. Overy. This is an open-access article distributed under the terms of the Creative Commons Attribution License (CC BY). The use, distribution or reproduction in other forums is permitted, provided the original author(s) and the copyright owner(s) are credited and that the original publication in this journal is cited, in accordance with accepted academic practice. No use, distribution or reproduction is permitted which does not comply with these terms.
*Correspondence: Jeremy R. Dettman, jeremy.dettman@agr.gc.ca; David P. Overy, david.overy@agr.gc.ca
†These authors have contributed equally to this work and share first authorship