- 1Department of Chemistry, Iowa State University, Ames, IA, United States
- 2Department of Physics, Northeastern University, Boston, MA, United States
The interior of the eukaryotic cell nucleus has a crowded and heterogeneous environment packed with chromatin polymers, regulatory proteins, and RNA molecules. Chromatin polymer, assisted by epigenetic modifications, protein and RNA binders, forms multi-scale compartments which help regulate genes in response to cellular signals. Furthermore, chromatin compartments are dynamic and tend to evolve in size and composition in ways that are not fully understood. The latest super-resolution imaging experiments have revealed a much more dynamic and stochastic nature of chromatin compartments than was appreciated before. An emerging mechanism explaining chromatin compartmentalization dynamics is the phase separation of protein and nucleic acids into membraneless liquid condensates. Consequently, concepts and ideas from soft matter and polymer systems have been rapidly entering the lexicon of cell biology. In this respect, the role of computational models is crucial for establishing a rigorous and quantitative foundation for the new concepts and disentangling the complex interplay of forces that contribute to the emergent patterns of chromatin dynamics and organization. Several multi-scale models have emerged to address various aspects of chromatin dynamics, ranging from equilibrium polymer simulations, hybrid non-equilibrium simulations coupling protein binding and chromatin folding, and mesoscopic field-theoretic models. Here, we review these emerging theoretical paradigms and computational models with a particular focus on chromatin’s phase separation and liquid-like properties as a basis for nuclear organization and dynamics.
1 Introduction
The eukaryotic nucleus is a membrane bound-organelle with a crowded, heterogeneous, and dynamically changing biomolecular composition. A large fraction of the nucleus is occupied by chromatin, a tight association of genomic DNA with histone proteins. The 1D sequence of chromatin polymer is decorated with epigenetic marks, which add an extra layer of information on the top of the DNA sequence. The 3D conformations of chromatin in the nucleus evolve over cellular life because of the passive diffusion and binding of biomolecules in the nucleoplasm and the active processes acting on conformations and epigenetic states of chromatin. Despite the molecular stochasticity and dynamism present in the nucleus, the chromatin organization and dynamics are not random but highly correlated with transcriptional activities and phenotypic states of cells.
A fundamental question in genome biophysics is to understand the link between three layers of information encoded by chromatin and its environment; 1D epigenetic patterning, 3D architectures, and transcriptional processes. Historically, different experimental and computational techniques have been invented for interrogating different scales of chromatin, ranging from single nucleosomal units to folding of chromosomes and mesoscale chromatin organization in the nucleus. Recent advances in single-cell imaging techniques, computational modeling, and machine learning methods have brought experiment and theoretical approaches much closer, calling for more integrative analysis and interpretation of chromatin behavior by bridging spatial and temporal scales.
A testament to this is the founding of the 4D nucleome initiative Dekker et al. (2017) which aims to map 3D architectural models across time and space, thereby linking gene expression machinery and other biological functions to detailed chromatin conformational dynamics. Over the last decade, a great deal has been learned about the static 3D organization of chromatin thanks to the chromosome conformation capture techniques, especially the Hi-C Lieberman-Aiden et al. (2009a); van Berkum et al. (2010). There are many excellent reviews summarizing the current state of knowledge on structural aspects of 3D chromatin folding Jerkovic and Cavalli (2021); Ghosh and Meyer (2021); Grigoryev and Schubert (2019); Cremer et al. (2020); Itoh et al. (2021a); therefore, we only briefly list the significant findings that have direct implications for discussing chromatin dynamics.
Chromatin is self-organized in a hierarchical manner (Figure 1). At the nuclear scale, the chromosomes are organized into individual territories (CTs), which have nonrandom positioning relative to the nuclear center Cremer and Cremer (2001). Within the chromosomal territories, chromatin is partitioned into two spatial compartments, which, on the basis of epigenetic tracks and Hi-C maps, are quantitatively classified into A and B types. The compartmentalized A/B chromatin states strongly overlap with euchromatin and heterochromatin compartments, which are physically distinct states of chromatin differing in density, transcription activity, and histone modifications Lieberman-Aiden et al. (2009b); Dekker et al. (2013); Rao et al. (2014). Specifically, the euchromatin compartment is defined as the less dense genomic regions that are enriched by transcriptionally active genes and histone marks, while the heterochromatin compartment is defined as the dense genomic regions associated with transcriptionally inactive genes and repressive histone modifications. The spatial partition of the A and B compartments is cell type-specific with a strong dependence on the cell cycle and line Dixon et al. (2015); Su et al. (2020); Feodorova et al. (2020). At the intermediate scale, these 2 A/B compartments themselves have substructures referred to as topologically associated domains (TADs), which in turn are organized into smaller nano-domains and loops Dekker et al. (2013); Rao et al. (2014).
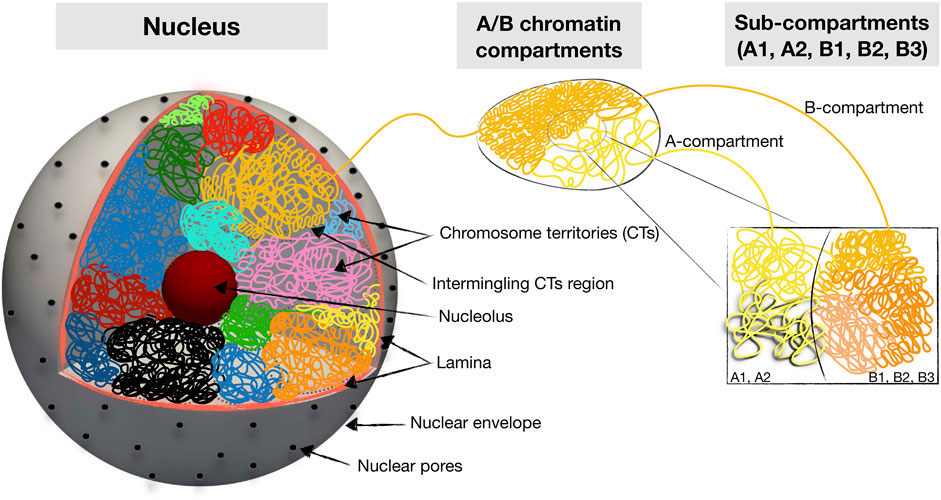
FIGURE 1. Schematic summary of hierarchical 3D folding of chromatin into compartments and domains. Shown are various keywords relevant for describing nuclear chromatin architecture along with length scales relevant for modeling and imaging studies.
To date, the majority of Hi-C data has been collected at the cell population level. The recently single-cell Hi-C and super-resolution imaging reveal a much more stochastic and dynamical behavior of chromatin throughout cell cycle and differentiation. This picture of chromatin is consistent with active multiphase liquid condensate Shaban et al. (2018); Shaban and Seeber (2020); Barth et al. (2020); Miron et al. (2020). Further support for multiphase condensate ideas comes from experiments on proteins and nucleic acids liquid-liquid phase separation (LLPS) Brangwynne et al. (2009); Brangwynne et al., 2011; Nott et al. (2015); Molliex et al. (2015); Pak et al. (2016). These findings imply that the liquid behavior strongly impacts chromatin dynamics and multivalent interactions with nuclear proteins and RNA peng and Weber (2019); Maeshima et al. (2020); Sanulli and Narlikar (2020); Choi et al. (2020); Smith et al. (2020). The notion of liquid chromatin states Maeshima et al. (2020) is further supported by the observations of the viscoelastic response of chromosomal loci Zidovska et al. (2013); Lucas et al. (2014); Di Pierro et al. (2018); Strickfaden et al. (2020), coherent motions of chromatin domains Zidovska et al. (2013); Saintillan et al. (2018), the coalescence and Ostwald ripening of chromatin droplets Caragine et al. (2018); Lee et al. (2021), the existence of epigenetic zonations and chains of interlinked
To dissect the complexity of nuclear chromatin organization and dynamics requires an inter-disciplinary approach combining experimental techniques to theoretical, mathematical, and physical modeling. Various computational models have been developed for addressing questions relevant to a particular chromatin scale. The models range from explicit all-atom simulations of nucleosomes to coarse-grained polymer models of chromosomes and to continuous mechanical models of the cell’s nucleus. Herein we will focus specifically on the models that embrace the liquid dynamical perspective of chromatin and make concrete predictions about the observed phenomena. These models’ continuous development and refinement will be essential for understanding the roles and impact of phase separation on emergent chromatin organization and dynamics.
2 Polymer Models of Phase Separation and Dynamics of Chromatin Compartments
Fluorescence in situ hybridization (FISH) techniques have established that interphase chromosomes occupy distinct nuclear regions known as chromosome territories within which chromosomes can be seen as polymers with nonrandom 3D folds Cremer and Cremer (2001). Therefore it is natural to appeal to the ideas of polymer physics for interpreting the data on chromatin folding and dynamics. Furthermore, computer simulations with polymeric models enable one to make concrete predictions on experimentally accessible quantities: frequency of contacts between two chromosomal loci, diffusion coefficients, spatial and temporal correlation functions, the impact of looping and chain entanglement on 3D architectures, etc. Before delving into the predictions made by various polymer models and simulations, it is instructive to review the basic assumptions that underlie polymer simulations of chromatin.
The two most common polymer models used for folding chromosomes are derived either via a forward or inverse approaches Tiana and Giorgetti (2019); Fiorillo et al. (2020); Brackey et al. (2020); Lin et al. (2021); Di Stefano et al. (2021a); Yildirim et al. (2021); Chiang et al. (2022). In the forward approach (also known as mechanistic approach), one first designs, an energy function that describes chromosomal loci interactions mainly based on accumulated evidence from experiments while relying on the physical intuition from polymer physics. In the forward approach, the input parameters of the designed potential energy function used in the polymer models are varied for testing a specific hypothesis in order to reproduce experimental data or for generating new ideas which guide the experiments. The flexibility and freedom of the forward approach allow navigating the parameter space and discovering insights that are not readily seen in the available data.
The forward or mechanistic approach has been widely used to investigate various biological questions related to the physical properties of DNA; the microphase separation of DNA into the nucleus, formation of protein-mediated chromatin loops, and genome organization Barbieri et al. (2012); Nuebler et al. (2018); Tiana and Giorgetti (2019); Brackey et al. (2020). In the mechanistic approach, most models used for simulating the whole-genome organization derived from the polymer model in which the usual potential energy function is completed by additional terms for chromatin constraints to describe the inter and intrachromosomal interactions and whose input parameters are derived from the experimental Hi-C maps Barbieri et al. (2012); Nuebler et al. (2018); Tiana and Giorgetti (2019); Brackey et al. (2020).
The inverse approach starts once again from an energy function motivated by a combination of polymer physics and experimental information. The parameters of the model in the inverse approach, however, are trained on a combination of Hi-C, 1D Chip-seq/RNA-seq and/or fluorescence in situ hybridization (FISH) data once and then used for making predictions on a new batch of data such as different cell lines or cell cycle stages. The accumulation of high precision Hi-C and FISH data has greatly expanded the reach of polymer models, which now play a crucial role in visualizing chromosome folding and dynamics Di Pierro et al. (2017); Tiana and Giorgetti (2019); Lin et al. (2021) (Figure 2).
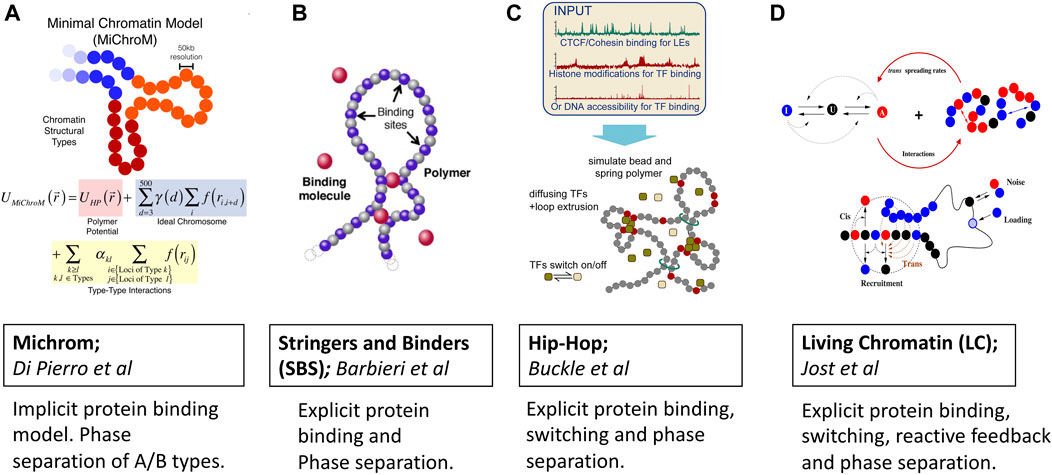
FIGURE 2. Predictive polymer models of 3D chromatin folding based on protein binding, loop extrusion and phase separation ideas. Images are adopted from original papers with copyright agreement. From left to right; (A) Michrom Di Pierro et al. (2018), (B) Stringers and Binders Barbieri et al. (2012), (C) Hip-Hop Buckle et al. (2018) and (D) Living Chromatin Jost and Vaillant (2018).
A fundamental question regarding the polymeric models which are trained against reproducing contact maps is whether they will be able to recapitulate the dynamic features of chromosomes? The dynamical information contained in FISH and other imaging approaches could, in principle, be used to complement and enhance information in Hi-C. However, the relationship between Hi-C and FISH is far from trivial to reconcile. For instance, Fudenberg et al. Fudenberg and Imakaev (2017) find that transforming ensemble average contact frequencies into spatial distances generates inconsistent models of chromosomal organization. This inconsistency stems from a mixture of conformational sub-populations of chromosomes, each characterized by its own statistics. This global heterogeneity is an intrinsic characteristic of all chromosomes and is the primary reason for the discrepancy between contact frequency and distance distributions obtained by Hi-C and FISH. Therefore, it is clear that for a deeper understanding of chromatin conformations and dynamics, one must at least be able to reconcile and integrate structural information from conformational capture techniques with dynamical information from imaging experiments. To this end, Shi et al. Shi and Thirumalai (2019) have deployed Generalized Rouse Chromosome Model for reconciling the Hi-C and FISH data. Recently, Onami’s group has proposed a simulation tool using a streamlined polymer network model called Phi-C to bridge the information gap between Hi-C and imaging experiments Shinkai et al. (2020).
The growing amount of data provided by Hi-C and imaging technologies coupled with the increasing resolution has bolstered the development of computational models that are becoming increasingly more quantitative in their predictions of complex structural aspects and underlying mechanisms behind chromatin folding and dynamics. Initially, models were as homo-polymeric chains, but over time as the realization of the importance of protein binding, bridging, and phase separation emerged, hetero-polymer models became more common. A beautiful illustration of a predictive reach of co-polymeric models is provided by a recent study by Falk et al. Falk et al. (2019) which parametrized A/B copolymer model of chromosomes against the Hi-C maps of rods cell and have shown that a judicious interplay of A-A and B-B self-interactions, A-B cross interactions and the strength of the heterochromatin attachment to the nuclear envelope dictate global nature of chromatin compartmentalization underlying conventional and inverted nuclear architectures. In a recent study of nucleoli formation in the presence of the chromatin network, Zhang’s group has used a diploid human genome model parameterized with chromosome conformation capture (Hi-C) data. They have shown the important role played by the viscoelastic chromatin network to stabilize the multi-droplet state for nucleoli [Qi and Zhang (2021)].
Below we review a class of models that incorporate protein binding and have shown de novo predictive capabilities regarding 3D folding and dynamics of chromatin domains.
MiChroM model developed by Di Pierro et al. Di Pierro et al., 2016; Di Pierro et al., 2017; Di Pierro et al., 2018) captures protein binding induced phase separation implicitly by learning energy landscapes of chromatin folding from Hi-C and Chip-seq data once (Figure 2A). The potential energy function is trained by the application of a MaxEnt approach which generates the least-biased choice of parameters Lin et al. (2021). In addition to the usual potential of the homopolymer model, the energy function accounts for the interactions between chromatin types, the interactions between loop anchors, and a translational invariant compaction contribution Di Pierro et al. (2016). The combination of MaxEnt and machine learning has made the model stand out due to its simplicity and predictive power. There is now a dedicated server that, given the Chip-seq input, generates input files for molecular dynamics simulations Contessoto et al. (2020). Thanks to the transferable nature of the model, the subdiffusive dynamics of chromatin loci were naturally and qualitatively explained within this framework of epigenetically decorated chromatin A/B copolymer. The subdiffusive exponent originates from chromatin’s effective energy landscape shaped by thermodynamics-driven phase separation of A/B epigenetic types and non-equilibrium motorized cross-linking. Viscoelasticity and coherent motions of chromatin domains over multiple seconds were similarly seen as a consequence of the micro-phase separation of chromatin types.
The chromatin-binding proteins play an important role in the large-scale chromatin organization because they regulate the formation of compartments and mediate interactions between distal genomic regions via phase separation.
For instance, the Heterochromatin Protein-1 (HP1a), which is known for binding to epigenetically distinct nucleosomes, is now believed to play a crucial role in the heterochromatin formation Razin and Ulianov (2020); Llorens-Giralt et al. (2021); Eissenberg and Elgin (2014). Furthermore HP1 has been shown to undergo phase-separation both in vitro and in vivo Zenk et al. (2021); Larson et al. (2017). While the ability of HP1a in driving the formation of heterochromatin is clearly demonstrated for embryonic cells Zenk et al. (2021), for the differentiated cells HP1a appears to have different regulatory roles. We note that a detailed mechanistic picture of heterochromatin formation still remains full of puzzles calling for more detailed mechanistic modeling of chromatin Erdel (2020); Misteli (2020); Itoh et al. (2021b); Bhat et al. (2021). Besides HP1a the RNA molecules which bind to proteins and phase separate have also been found to have active involvement in the euchromatin domain formation through the microphase separation mechanism (Hilbert et al. (2021). In sum, there is now growing evidence that protein-induced microphase separation regulates the formation of chromatin compartments. Still, it remains unclear just how important are the various biophysical properties and interactions of phase separating proteins and RNAs in driving the formation of chromatin compartments. The strings and binders (SBS) model class of classic polymer models (Figure 2B) developed by the group of Nicodemi Nicodemi and Prisco (2009); Barbieri et al. (2012) was among the first to consider protein binding explicitly. In the SBS model, the chromatin is considered as a self-avoiding polymer that hosts several binding sites that interact with diffusing protein binders. The binding is controlled through a binding energy term which sets the overall binding energy scales and the affinities of binders. Specifically, within the SBS framework, a local microphase separation of cognate binding sites along 1D chromatin sequence was treated as the foundation for producing 3D architecture. This protein-induced microphase separation has been confirmed to be one of the major driving forces behind the formation of A/B compartments observed in Hi-C experiments. Since its inception, SBS has undergone a series of refinements which enabled making several remarkable predictions and explanations of chromatin folding specificity and variability in different organisms Bianco et al. (2020); Esposito et al. (2021). For instance, a tissue-specific α-globin genomic region was successfully recapitulated by the SBS model Chiariello et al. (2020). Recently, the variability of the TADs across single cells was explained in terms of the thermodynamic degeneracy of conformations predicted by polymer phase separation Conte et al. (2020).
Similar to Stringers and Binders, the Hip-Hop model proposed by Buckle et al. Buckle et al. (2018) combines explicit protein diffusion and binding with loop extrusion for predicting the 3D folding of genomic loci at a population and single-cell levels (Figure 2C). With a minimal a priori knowledge of epigenetic marks, the Hip-Hop model has been shown to recapitulate complex genomic loci in 3D and enable predictions of chromatin folding paths.
Living Chromatin model proposed by Haddad et al. (2017); Jost et al. (2017); Jost and Vaillant (2018); Tortora et al. (2020) considers epigenetic marks to the 3D folding of chromatin fiber (Figure 2D). Within this model, the formation of heterochromatin/euchromatin compartments in the Drosophila originates from the microphase separation of A/B types, which gives rise to a dynamical and stochastic organization chromatin. Interestingly, the model predicts weaker local self-interaction for euchromatin than for heterochromatin which favors more long-range and complex patterns of self-association. Authors calibrate the copolymer chromatin model from the MSD measurement to have a reliable description of chromosome folding kinetics. The living chromatin model is a combination of the copolymer chromatin and the epigenome regulation models Jost et al., 2012; Jost et al., 2017; Jost and Vaillant (2018). Each monomer can be in one of the three states: A, U, and I; the inter-conversion dynamics between these states result from random or recruited inter-conversions. The chain is modeled by a semi-flexible self-avoiding bead-spring model with specific short-range attractions between monomers of the same epigenomic states (A or I). Recruited conversions are achieved either by recruitment in cis 1D nearest neighbors or in the 3D neighborhood. There is also a noisy conversion between I and U and A and U and the possibility of external loading at some specific recruitment sites.
3 Mesoscale Models of Phase Separation and Dynamics of Chromatin Compartments
Its chain dynamics do not completely describe chromatin polymer because it is subject to a number of external physical constraints, mechanical and biochemical forces in the nucleus Aboelnour and Bonev (2021); Jerkovic and Cavalli (2021); Di Stefano et al. (2021b); Bhat et al. (2021). In particular, chromatin physically interacts with actin filaments, nuclear lamins, and ATP-powered transcriptional machinery such as RNA polymerase Zidovska, 2020a; Zidovska, 2020b. Recent studies have highlighted the important role played by actin and microtubule cytoskeletons in inducing nuclear membrane fluctuations and thereby facilitating chromatin mobility Almonacid et al. (2019). The role of ATP-powered polymerizes has also been shown to generate coherent motions and directed flows inside nuclei Saintillan et al. (2018). In the backdrop of all the internal and external forces acting on chromatin, the biomolecular phase separation appears as a ubiquitous mechanism for transmitting the forces whether directly or through biochemical feedback mechanisms Laghmach and Potoyan (2020). For instance, the transcriptional condensate formation and recruitment of RNA polymerase, formation of heterochromatin domains, and exertion of ATP-powered forces that locally restructure chromatin fiber are all processes that happen over scales where phase separation of chromatin domains is relevant and likely plays a major organizational role.
How to describe and disentangle the motions of chromatin in the cell nucleus that result from the collective action of forces spanning vastly different scales and operate in a highly heterogeneous and out of equilibrium environment of the nucleus? This is certainly a very challenging problem and is most likely to develop several innovative approaches that could combine innovative computational models and integrate them with data from imaging experiments. A key challenge in modeling chromatin dynamics at the scale of the full nucleus is finding the appropriate resolution for capturing processes taking place over spatial and temporal scales of interest.
A promising approach is to employ field-theoretical and continuum-based models that coarse grain over chromatin’s particular nature and resolve it as a viscoelastic complex fluid mixture occupying the nucleus. Some of the earliest field-theoretical approaches for studying chromatin dynamics were provided by Bruinsma et al. Bruinsma et al. (2014). In this study, a theoretical framework based on the linear response theory was applied to a binary viscoelastic fluid to analyze studies of ATP-driven chromatin dynamics. A toy model demonstrated the relationship between chromatin density and velocity correlation with the viscoelastic moduli of the chromatin solution.
Recently, phase-field models have been finding increased application for studying cell biology ranging from mechanics and motility of individual cells to multicellular systems Nonomura (2012); Najem and Grant (2016); Akiyama et al. (2018); Jiang et al. (2019); Moure and Gomez (2021). The first phase-field model for multicellular systems was proposed by Nonomura Nonomura (2012). Modeling nuclear interior and dynamics of chromatin compartments is another natural application for phase-field models since the problem can be described in various moving chromatin interfaces that are segregated from one another. The chromatin state in the phase-field models can be resolved with an arbitrary number of chromatin types corresponding to heterochromatin/euchromatin or facultative/constitutive heterochromatin forms. The primary driving forces for emergent nuclear architecture and dynamics are derived from the microphase separation of heterochromatin sub-types, the surface tension of chromatin droplets, and differential affinity for chromatin-lamina interactions. Voltage and surface constraints are imposed on chromatin types to capture chromosomal and nuclear boundaries. Given the dense, active, and heterogeneous nature of nuclear chromatin, it is worth highlighting the advantage of field-theoretic description, which manages to avoid the notorious glassy states encountered in the particle-based polymer simulations, thereby facilitating the study of long-timescale chromatin dynamics and patterning at the scale of whole nucleus Shi et al. (2018); Kang et al. (2015).
Mesoscale liquid model of nucleus (MELON) developed by Laghmach et al. Laghmach et al. (2020); Laghmach et al., 2021) describes the state of the nucleus as a mixture of incompressible multiphase fluids (Figure 3A). In this description, the emergent chromatin patterns are seen as an interplay of phase separation, chromosomes’ territorial affinity, and surface tension of heterochromatin-euchromatin droplets. The significance of the surface tension of heterochromatin within the nucleus and its interaction with heterochromatin determines the nuclear morphology reminiscent of senescent, inverted, and conventional architectures. The model also has provided insights into how remodeling nuclear volume has dynamical implications for chromatin compartments where the kinetic barrier for phase separation is lowered thanks to the proximity of heterochromatic centers in adjacent chromosomal territories. This mesoscale perspective offers new avenues for integrating imaging experiments and reconciling dynamic phenomena observed in in-situ nucleus imaging. Using the MELON model with a ternary liquid-like chromatin state has looked at dynamics of chromatin compartment during interphase. Addressed dynamics of chromatin compartment formed by phase separation of constitutive and facultative heterochromatin, which lead to gel-like elongated channels and spheroidal droplets centered chromosome territory Laghmach et al. (2021).
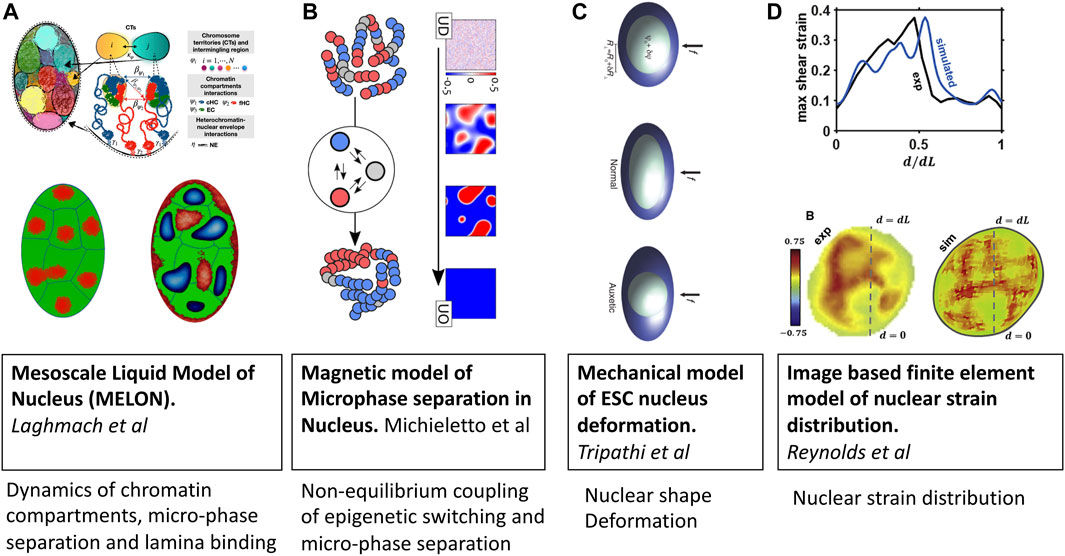
FIGURE 3. Mesoscale models of eukaryotic nucleus. Images are adopted from original papers with copyright agreement. From left to right; (A) Mesoscale liquid model of nucleus Laghmach et al. (2020), Laghmach et al., 2021, (B) Magnetic model of chromatin phase separation by Michieletto et al (2019), (C) Mechanical model of stem cell nucleus deformation Tripathi and Menon (2019), and (D) Image based finite element model of nucleus Reynolds et al. (2021).
Similar to the design of the living chromatin model discussed in the previous section, the group of D. Marenduzzo Michieletto et al., 2016; Michieletto et al., 2019; Coli et al. (2019) has also developed a series of models with explicit coupling between 1D epigenetic dynamics and 3D folding of chromatin (Figure 3B). The key difference from other explicit protein models is that epigenetic changes are modeled via spin-Hamiltonian dynamics instead of biochemical reactions. The application of magnetic models of chromatin has shown the importance of non-equilibrium processes in regulating chromatin domain patterning, which arrest microphase separation of euchromatin-heterochromatin in lifelike morphologies instead of falling into equilibrium dominated by either of chromatin types Michieletto et al. (2019).
An emerging area where mesoscale models of chromatin will be instrumental is cell mechanotransduction and mechanosensing. To understand the relationship between local DNA density and mechanical stress fields generated in the nucleus, Reynolds et al.Reynolds et al. (2021) have set up a mesoscale three-dimensional finite-element model of a cell nucleus from image stacks collected by confocal microscopy (Figure 3C). Simulations suggest that the mechanical behavior of the nucleus is highly heterogeneous, with a non-linear relationship between local chromatin packing and shear moduli. They also find that disruption of the nuclear envelope associated with lamin A/C depletion significantly increases nuclear strain in regions of low DNA concentration.
The coupling between chromatin compaction states and nuclear shape has been studied by Tripathy and Menon Tripathi and Menon (2019) using a mesoscopic mechanical model of the nucleus that resolves nuclear shape variables and applied force accessing in AFM experiments (Figure 3D). Armed with this mesoscale mechanical model of the nucleus, the authors explain the measurements of the deformability of cell nuclei in the transition state between embryonic stem-cell state and the differentiated state of mouse stem cells which have a negative Poisson’s ratio. A key insight from the study is that fluctuations in chromatin compaction are coupled to fluctuations in the relatively soft nucleus’s dimensions that characterize stem cells.
Another notable mesoscopic mechanical model of the nucleus has been developed by Marko and colleagues Banigan et al. (2017); Stephens et al. (2019) to understand force-extension regimes when stretching the nucleus and imaging the lamina of isolated cell nuclei. The model can explain the two linear tension-strain regimes, corresponding to a weak, linear elastic response to small tensions and a stiff linear response to nuclear deformation, which deforms sufficiently to align the inter-subunit bonds with the tension axis. Also, the model predicts the buckling transition between the two regimes.
In conclusion, we would also like to mention mesoscopic models that go beyond a lifetime of a single nucleus in order to probe the dynamics of the establishment of heterochromatin compartments and mechanisms of propagation of “epigenetic memory” through cell divisions Ng et al. (2018); Feinberg and Irizarry (2010); Himeoka and Kaneko (2020); Nickels et al. (2021). Mathematical models formulated as kinetic monte carlo Dodd et al. (2007); Nguyen et al. (2021) or agent-based Sneppen and Ringrose (2019) dynamical simulations have been widely used to study the stochastic dynamical interplay of local and global feedback mechanisms of histone methylation and acetylation along the 1D genomic sequence. While often lacking in spatially resolved details compared to polymeric and phase-field models, these stochastic kinetics studies have nevertheless offered the first glimpse into systems-level regulation of heterochromatin formation, maintenance, and regulation.
A recent study employing experiment and mathematical modeling Nickels et al. (2021) has looked at the fission yeast cell, which contains nucleation center of heterochromatin CenH. By incrementally varying the meting region size, the mathematical model aided by the experiments has come across a remarkable finding that heterochromatin propagation occurs in an all-or-none fashion, where the entire domains collapse abruptly. This stochastic burst-like mode of heterochromatin domain fashion goes against the linear propagation mechanism and necessitates the inclusion of distance-dependent kinetic processes. Future studies would be imperative to include spatially resolved and global feedback mechanisms that act on histone modifications. Since the mathematical models of epigenetic processes are rather extensive, we refer the readers to excellent books and reviewers which cover the accumulated knowledge and latest insights from mathematical modeling of emergent trans-generational epigenetic memory and establishment of chromatin domains from first principles stochastic kinetics of histone marking and erasure Sneppen (2014); Ringrose (2017); Menon et al. (2021); Lövkvist and Howard (2021).
4 Conclusion
Given the complex, crowded, and out of equilibrium nature of the nuclear environment, it is challenging to distill the driving forces of chromatin patterning and its dynamical evolution. High-resolution imaging studies have shown a much more stochastic, heterogeneous, and dynamical chromatin nature than expected. At the same time, ideas revolving around protein and RNA-induced phase separation have given us with mechanistic clues to rationalize the emergent nuclear architectures, and dynamical observables probed in imaging experiments. In this respect, mechanistic models incorporating physical intuition and empirical data prove crucial for interpreting and guiding future experiments.
Going forward we see a number of conceptual and methodological bottlenecks overcoming of which will take our understanding of chromatin organization and dynamics to the next level. (I) On the conceptual side, it is becoming evident that there are different chromatin compartments that have heterogeneous properties and different mechanistic paths of formation. Therefore grouping them under one umbrella and attempting to explain behavior through the lens of phase separation alone is not realistic. Hence, more systematic differentiation and classification schemes need to be developed in part by imaging different cell lines and updating the vocabulary of theoretical models of intracellular phase separation. (II) On the methodological side, as polymer models of chromosome 3D folding are becoming more established and predictive thanks to Hi-C experiments, we need to break new ground in the direction of mesoscale modeling techniques for characterizing chromatin patterning dynamics seen in high-resolution imaging experiments.
In summary, promising future directions are emerging where an integrative approach combining novel experimental biophysical imaging methods of nuclear chromatin, mechanistic mesoscale models together with the development of machine learning and analytic data methods will be instrumental in the quest for a deeper understanding of the nature of chromatin patterning and its functional implications.
Author Contributions
RL and DP developed the outline of the review. RL MD, and DP wrote the review.
Funding
This work has been funded by the National Institute Of General Medical Sciences of the National Institutes of Health under Award Number R35GM138243. The authors also acknowledge financial support from Iowa State University.
Conflict of Interest
The authors declare that the research was conducted in the absence of any commercial or financial relationships that could be construed as a potential conflict of interest.
Publisher’s Note
All claims expressed in this article are solely those of the authors and do not necessarily represent those of their affiliated organizations, or those of the publisher, the editors and the reviewers. Any product that may be evaluated in this article, orclaim that may be made by its manufacturer, is not guaranteed or endorsed by the publisher.
References
Aboelnour, E., and Bonev, B. (2021). Decoding the Organization, Dynamics, and Function of the 4D Genome. Dev. Cel 56, 1562–1573. doi:10.1016/j.devcel.2021.04.023
Akiyama, M., Nonomura, M., Tero, A., and Kobayashi, R. (2018). Numerical Study on Spindle Positioning Using Phase Field Method. Phys. Biol. 16, 016005. doi:10.1088/1478-3975/aaee45
Almonacid, M., Al Jord, A., El-Hayek, S., Othmani, A., Coulpier, F., Lemoine, S., et al. (2019). Active Fluctuations of the Nuclear Envelope Shape the Transcriptional Dynamics in Oocytes. Dev. Cel. 51, 145–157. doi:10.1016/j.devcel.2019.09.010
Banigan, E. J., Stephens, A. D., and Marko, J. F. (2017). Mechanics and Buckling of Biopolymeric Shells and Cell Nuclei. Biophysical J. 113, 1654–1663. doi:10.1016/j.bpj.2017.08.034
Barbieri, M., Chotalia, M., Fraser, J., Lavitas, L.-M., Dostie, J., Pombo, A., et al. (2012). Complexity of Chromatin Folding Is Captured by the Strings and Binders Switch Model. Proc. Natl. Acad. Sci. 109, 16173–16178. doi:10.1073/pnas.1204799109
Barth, R., Bystricky, K., and Shaban, H. A. (2020). Coupling Chromatin Structure and Dynamics by Live Super-resolution Imaging. Sci. Adv. 6, eaaz2196. doi:10.1126/sciadv.aaz2196
Bhat, P., Honson, D., and Guttman, M. (2021). Nuclear Compartmentalization as a Mechanism of Quantitative Control of Gene Expression. Nat. Rev. Mol. Cel Biol. 22, 1–18. doi:10.1038/s41580-021-00387-1
Bianco, S., Chiariello, A. M., Conte, M., Esposito, A., Fiorillo, L., Musella, F., et al. (2020). Computational Approaches from Polymer Physics to Investigate Chromatin Folding. Curr. Opin. Cel. Biol. 64, 10–17. doi:10.1016/j.ceb.2020.01.002
Brackey, C. A., Marenduzzo, D., and Gilbert, N. (2020). Mechanistic Modeling of Chromatin Folding to Understand Function. Nat. Methods 17, 767–775. doi:10.1038/s41592-020-0852-6
Brangwynne, C. P., Eckmann, C. R., Courson, D. S., Rybarska, A., Hoege, C., Gharakhani, J., et al. (2009). Germline P Granules Are Liquid Droplets that Localize by Controlled Dissolution/condensation. Science 324, 1729–1732. doi:10.1126/science.1172046
Brangwynne, C. P., Mitchison, T. J., and Hyman, A. A. (2011). Active Liquid-like Behavior of Nucleoli Determines Their Size and Shape in xenopus Laevis Oocytes. Proc. Natl. Acad. Sci. 108, 4334–4339. doi:10.1073/pnas.1017150108
Bruinsma, R., Grosberg, A. Y., Rabin, Y., and Zidovska, A. (2014). Chromatin Hydrodynamics. Biophysical J. 106, 1871–1881. doi:10.1016/j.bpj.2014.03.038
Buckle, A., Brackley, C. A., Boyle, S., Marenduzzo, D., and Gilbert, N. (2018). Polymer Simulations of Heteromorphic Chromatin Predict the 3D Folding of Complex Genomic Loci. Mol. Cel 72, 786–797.e11. doi:10.1016/j.molcel.2018.09.016
Caragine, C. M., Haley, S. C., and Zidovska, A. (2018). Surface Fluctuations and Coalescence of Nucleolar Droplets in the Human Cell Nucleus. Phys. Rev. Lett. 121, 148101. doi:10.1103/physrevlett.121.148101
Chiang, M., Forte, G., Gilbert, N., Marenduzzo, D., and Brackley, C. A. (2022). “Predictive Polymer Models for 3d Chromosome Organization,” in Hi-C Data Analysis (Springer), 267–291. doi:10.1007/978-1-0716-1390-0_14
Chiariello, A. M., Bianco, S., Oudelaar, A. M., Esposito, A., Annunziatella, C., Fiorillo, L., et al. (2020). A Dynamic Folded Hairpin Conformation Is Associated with α-Globin Activation in Erythroid Cells. Cel Rep. 30, 2125–2135. doi:10.1016/j.celrep.2020.01.044
Choi, J.-M., Holehouse, A. S., and Pappu, R. V. (2020). Physical Principles Underlying the Complex Biology of Intracellular Phase Transitions. Annu. Rev. Biophys. 49, 107–133. doi:10.1146/annurev-biophys-121219-081629
Chu, F.-Y., Haley, S. C., and Zidovska, A. (2017). On the Origin of Shape Fluctuations of the Cell Nucleus. Proc. Natl. Acad. Sci. USA 114, 10338–10343. doi:10.1073/pnas.1702226114
Colì, D., Orlandini, E., Michieletto, D., and Marenduzzo, D. (2019). Magnetic Polymer Models for Epigenetics-Driven Chromosome Folding. Phys. Rev. E 100, 052410. doi:10.1103/PhysRevE.100.052410
Conte, M., Fiorillo, L., Bianco, S., Chiariello, A. M., Esposito, A., and Nicodemi, M. (2020). Polymer Physics Indicates Chromatin Folding Variability across Single-Cells Results from State Degeneracy in Phase Separation. Nat. Commun. 11, 3289–3313. doi:10.1038/s41467-020-17141-4
Contessoto, V. G., Cheng, R. R., Hajitaheri, A., Dodero-Rojas, E., Mello, M. F., Lieberman-Aiden, E., et al. (2020). The Nucleome Data Bank: Web-Based Resources to Simulate and Analyze the Three-Dimensional Genome. Nucleic Acids Res. 49, D172–D182. doi:10.1093/nar/gkaa818
Cremer, T., and Cremer, C. (2001). Chromosome Territories, Nuclear Architecture and Gene Regulation in Mammalian Cells. Nat. Rev. Genet. 2, 292–301. doi:10.1038/35066075
Cremer, T., Cremer, M., Hübner, B., Silahtaroglu, A., Hendzel, M., Lanctôt, C., et al. (2020). The Interchromatin Compartment Participates in the Structural and Functional Organization of the Cell Nucleus. BioEssays 42, 1900132. doi:10.1002/bies.201900132
Dekker, J., Belmont, A. S., Belmont, A. S., Guttman, M., Leshyk, V. O., Lis, J. T., et al. (2017). The 4d Nucleome Project. Nature 549, 219–226. doi:10.1038/nature23884
Dekker, J., Marti-Renom, M. A., and Mirny, L. A. (2013). Exploring the Three-Dimensional Organization of Genomes: Interpreting Chromatin Interaction Data. Nat. Rev. Genet. 14, 390–403. doi:10.1038/nrg3454
Di Pierro, M., Cheng, R. R., Lieberman Aiden, E., Wolynes, P. G., and Onuchic, J. N. (2017). De Novo prediction of Human Chromosome Structures: Epigenetic Marking Patterns Encode Genome Architecture. Proc. Natl. Acad. Sci. U. S. A. 114, 12126–12131. doi:10.1073/pnas.1714980114
Di Pierro, M., Potoyan, D. A., Wolynes, P. G., and Onuchic, J. N. (2018). Anomalous Diffusion, Spatial Coherence, and Viscoelasticity from the Energy Landscape of Human Chromosomes. Proc. Natl. Acad. Sci. U. S. A. 115 (30), 7753–7758. doi:10.1073/pnas.1806297115
Di Pierro, M., Zhang, B., Aiden, E. L., Wolynes, P. G., and Onuchic, J. N. (2016). Transferable Model for Chromosome Architecture. Proc. Natl. Acad. Sci. USA 113, 12168–12173. doi:10.1073/pnas.1613607113
Di Stefano, M., Paulsen, J., Jost, D., and Marti-Renom, M. A. (2021a). 4d Nucleome Modeling. Curr. Opin. Genet. Dev. 67, 25–32. doi:10.1016/j.gde.2020.10.004
Di Stefano, M., Paulsen, J., Jost, D., and Marti-Renom, M. A. (2021b). 4D Nucleome Modeling. Curr. Opin. Genet. Dev. 67, 25–32. doi:10.1016/j.gde.2020.10.004
Dixon, J. R., Jung, I., Selvaraj, S., Shen, Y., Antosiewicz-Bourget, J. E., Lee, A. Y., et al. (2015). Chromatin Architecture Reorganization during Stem Cell Differentiation. Nature 518, 331–336. doi:10.1038/nature14222
Dodd, I. B., Micheelsen, M. A., Sneppen, K., and Thon, G. (2007). Theoretical Analysis of Epigenetic Cell Memory by Nucleosome Modification. Cell 129, 813–822. doi:10.1016/j.cell.2007.02.053
Eissenberg, J. C., and Elgin, S. C. R. (2014). HP1a: a Structural Chromosomal Protein Regulating Transcription. Trends Genet. 30, 103–110. doi:10.1016/j.tig.2014.01.002
Erdel, F. (2020). Biophysical Mechanisms of Chromatin Patterning. Curr. Opin. Genet. Dev. 61, 62–68. doi:10.1016/j.gde.2020.03.006
Esposito, A., Bianco, S., Fiorillo, L., Conte, M., Abraham, A., Musella, F., et al. (2021). Polymer Models Are a Versatile Tool to Study Chromatin 3d Organization. Biochem. Soc. Trans. 49 (4), 1675–1684. doi:10.1042/bst20201004
Falk, M., Feodorova, Y., Naumova, N., Imakaev, M., Lajoie, B. R., Leonhardt, H., et al. (2019). Heterochromatin Drives Compartmentalization of Inverted and Conventional Nuclei. Nature 570, 395–399. doi:10.1038/s41586-019-1275-3
Feinberg, A. P., and Irizarry, R. A. (2010). Stochastic Epigenetic Variation as a Driving Force of Development, Evolutionary Adaptation, and Disease. Proc. Natl. Acad. Sci. 107, 1757–1764. doi:10.1073/pnas.0906183107
Feodorova, Y., Falk, M., Mirny, L. A., and Solovei, I. (2020). Viewing Nuclear Architecture through the Eyes of Nocturnal Mammals. Trends Cel Biol. 30, 276–289. doi:10.1016/j.tcb.2019.12.008
Fiorillo, L., Bianco, S., Esposito, A., Conte, M., Sciarretta, R., Musella, F., et al. (2020). A Modern challenge of Polymer Physics: Novel Ways to Study, Interpret, and Reconstruct Chromatin Structure. Wiley Interdiscip. Rev. Comput. Mol. Sci. 10, e1454. doi:10.1002/wcms.1454
Fudenberg, G., and Imakaev, M. (2017). Fish-ing for Captured Contacts: towards Reconciling Fish and 3c. Nat. Methods 14, 673–678. doi:10.1038/nmeth.4329
Ghosh, R. P., and Meyer, B. J. (2021). Spatial Organization of Chromatin: Emergence of Chromatin Structure during Development. Annu. Rev. Cel Dev. Biol. 37, 199–232. null. doi:10.1146/annurev-cellbio-032321-035734
Gibson, B. A., Doolittle, L. K., Schneider, M. W. G., Jensen, L. E., Gamarra, N., Henry, L., et al. (2019). Organization of Chromatin by Intrinsic and Regulated Phase Separation. Cell 179, 470–484.e21. doi:10.1016/j.cell.2019.08.037
Grigoryev, S. A., and Schubert, M. (2019). Unraveling the Multiplex Folding of Nucleosome Chains in Higher Order Chromatin. Essays Biochem. 63, 109–121. doi:10.1042/EBC20180066
Haddad, N., Jost, D., and Vaillant, C. (2017). Perspectives: Using Polymer Modeling to Understand the Formation and Function of Nuclear Compartments. Chromosome Res. 25, 35–50. doi:10.1007/s10577-016-9548-2
Hilbert, L., Sato, Y., Kuznetsova, K., Bianucci, T., Kimura, H., Jülicher, F., et al. (2021). Transcription Organizes Euchromatin via Microphase Separation. Nat. Commun. 12, 1360. doi:10.1038/s41467-021-21589-3
Hildebrand, E. M., and Dekker, J. (2020). Mechanisms and Functions of Chromosome Compartmentalization. Trends Biochem. Sci. 45, 385–396. doi:10.1016/j.tibs.2020.01.002
Himeoka, Y., and Kaneko, K. (2020). Epigenetic Ratchet: Spontaneous Adaptation via Stochastic Gene Expression. Sci. Rep. 10, 459–467. doi:10.1038/s41598-019-57372-0
Itoh, Y., Woods, E. J., Minami, K., Maeshima, K., and Collepardo-Guevara, R. (2021a). Liquid-like Chromatin in the Cell: What Can We Learn from Imaging and Computational Modeling? Curr. Opin. Struct. Biol. 71, 123–135. doi:10.1016/j.sbi.2021.06.004
Itoh, Y., Woods, E. J., Minami, K., Maeshima, K., and Collepardo-Guevara, R. (2021b). Liquid-like Chromatin in the Cell: What Can We Learn from Imaging and Computational Modeling? Curr. Opin. Struct. Biol. 71, 123–135. doi:10.1016/j.sbi.2021.06.004
Jerkovic, I., and Cavalli, G. (2021). Understanding 3d Genome Organization by Multidisciplinary Methods. Nat. Rev. Mol. Cel Biol. 22, 1–18. doi:10.1038/s41580-021-00362-w
Jiang, J., Garikipati, K., and Rudraraju, S. (2019). A Diffuse Interface Framework for Modeling the Evolution of Multi-Cell Aggregates as a Soft Packing Problem Driven by the Growth and Division of Cells. Bull. Math. Biol. 81, 3282–3300. doi:10.1007/s11538-019-00577-1
Jost, D., and Vaillant, C. (2018). Epigenomics in 3d: Importance of Long-Range Spreading and Specific Interactions in Epigenomic Maintenance. Nucleic Acids Res. 46, 2252–2264. doi:10.1093/nar/gky009
Jost, D., Vaillant, C., and Meister, P. (2017). Coupling 1D Modifications and 3D Nuclear Organization: Data, Models and Function. Curr. Opin. Cel Biol. 44, 20–27. doi:10.1016/j.ceb.2016.12.001
Jost, K. L., Bertulat, B., and Cardoso, M. C. (2012). Heterochromatin and Gene Positioning: inside, outside, Any Side? Chromosoma 121, 555–563. doi:10.1007/s00412-012-0389-2
Kang, H., Yoon, Y. G., Thirumalai, D., and Hyeon, C. (2015). Confinement-Induced Glassy Dynamics in a Model for Chromosome Organization. Phys. Rev. Lett. 115, 198102–198105. doi:10.1103/PhysRevLett.115.198102
Laghmach, R., Di Pierro, M., and Potoyan, D. A. (2020). Mesoscale Liquid Model of Chromatin Recapitulates Nuclear Order of Eukaryotes. Biophysical J. 118, 2130–2140. doi:10.1016/j.bpj.2019.09.013
Laghmach, R., Di Pierro, M., and Potoyan, D. A. (2021). The Interplay of Chromatin Phase Separation and Lamina Interactions in Nuclear Organization. Biophysical J. 120, 5005–5017. doi:10.1016/j.bpj.2021.10.012
Laghmach, R., and Potoyan, D. A. (2020). Liquid-liquid Phase Separation Driven Compartmentalization of Reactive Nucleoplasm. Phys. Biol. 18, 015001. doi:10.1088/1478-3975/abc5ad
Larson, A. G., Elnatan, D., Keenen, M. M., Trnka, M. J., Johnston, J. B., Burlingame, A. L., et al. (2017). Liquid Droplet Formation by HP1α Suggests a Role for Phase Separation in Heterochromatin. Nature 547, 236–240. doi:10.1038/nature22822
Lee, D. S. W., Wingreen, N. S., and Brangwynne, C. P. (2021). Chromatin Mechanics Dictates Subdiffusion and Coarsening Dynamics of Embedded Condensates. Nat. Phys. 17 (4), 531–538. doi:10.1038/s41567-020-01125-8
Lieberman-Aiden, E., van Berkum, N. L., Williams, L., Imakaev, M., Ragoczy, T., Telling, A., et al. (2009a). Comprehensive Mapping of Long-Range Interactions Reveals Folding Principles of the Human Genome. Science 326, 289–293. doi:10.1126/science.1181369
Lieberman-Aiden, E., Van Berkum, N. L., Williams, L., Imakaev, M., Ragoczy, T., Telling, A., et al. (2009b). Comprehensive Mapping of Long-Range Interactions Reveals Folding Principles of the Human Genome. science 326, 289–293. doi:10.1126/science.1181369
Lin, X., Qi, Y., Latham, A. P., and Zhang, B. (2021). Multiscale Modeling of Genome Organization with Maximum Entropy Optimization. J. Chem. Phys. 155, 010901. doi:10.1063/5.0044150
Llorens-Giralt, P., Camilleri-Robles, C., Corominas, M., and Climent-Cantó, P. (2021). Chromatin Organization and Function in Drosophila. Cells 10, 2362. doi:10.3390/cells10092362
Lövkvist, C., and Howard, M. (2021). Using Computational Modelling to Reveal Mechanisms of Epigenetic Polycomb Control. Biochem. Soc. Trans. 49, 71–77. doi:10.1042/bst20190955
Lucas, J. S., Zhang, Y., Dudko, O. K., and Murre, C. (2014). 3D Trajectories Adopted by Coding and Regulatory DNA Elements: First-Passage Times for Genomic Interactions. Cell 158, 339–352. doi:10.1016/j.cell.2014.05.036
Maeshima, K., Tamura, S., Hansen, J. C., and Itoh, Y. (2020). Fluid-like Chromatin: Toward Understanding the Real Chromatin Organization Present in the Cell. Curr. Opin. Cel Biol. 64, 77–89. doi:10.1016/j.ceb.2020.02.016
Menon, G., Schulten, A., Dean, C., and Howard, M. (2021). Digital Paradigm for Polycomb Epigenetic Switching and Memory. Curr. Opin. Plant Biol. 61, 102012. doi:10.1016/j.pbi.2021.102012
Michieletto, D., Colì, D., Marenduzzo, D., and Orlandini, E. (2019). Nonequilibrium Theory of Epigenomic Microphase Separation in the Cell Nucleus. Phys. Rev. Lett. 123, 228101. doi:10.1103/physrevlett.123.228101
Michieletto, D., Orlandini, E., and Marenduzzo, D. (2016). Polymer Model with Epigenetic Recoloring Reveals a Pathway for the De Novo Establishment and 3d Organization of Chromatin Domains. Phys. Rev. X 6, 041047. doi:10.1103/physrevx.6.041047
Miron, E., Oldenkamp, R., Brown, J. M., Pinto, D. M. S., Xu, C. S., Faria, A. R., et al. (2020). Chromatin Arranges in Chains of Mesoscale Domains with Nanoscale Functional Topography Independent of Cohesin. Sci. Adv. 6, eaba8811. doi:10.1126/sciadv.aba8811
Misteli, T. (2020). The Self-Organizing Genome: Principles of Genome Architecture and Function. Cell 183, 28–45. doi:10.1016/j.cell.2020.09.014
Molliex, A., Temirov, J., Lee, J., Coughlin, M., Kanagaraj, A. P., Kim, H. J., et al. (2015). Phase Separation by Low Complexity Domains Promotes Stress Granule Assembly and Drives Pathological Fibrillization. Cell 163, 123–133. doi:10.1016/j.cell.2015.09.015
Moure, A., and Gomez, H. (2021). Phase-Field Modeling of Individual and Collective Cell Migration. Arch. Computat Methods Eng. 28, 311–344. doi:10.1007/s11831-019-09377-1
Najem, S., and Grant, M. (2016). Phase-field Model for Collective Cell Migration. Phys. Rev. E 93, 052405. doi:10.1103/PhysRevE.93.052405
Ng, K. K., Yui, M. A., Mehta, A., Siu, S., Irwin, B., Pease, S., et al. (2018). A Stochastic Epigenetic Switch Controls the Dynamics of T-Cell Lineage Commitment. Elife 7, e37851. doi:10.7554/eLife.37851
Nguyen, P., Pease, N. A., and Kueh, H. Y. (2021). Scalable Control of Developmental Timetables by Epigenetic Switching Networks. J. R. Soc. Interf. 18, 20210109. doi:10.1098/rsif.2021.0109
Nickels, J. F., Edwards, A. K., Charlton, S. J., Mortensen, A. M., Hougaard, S. C. L., Trusina, A., et al. (2021). Establishment of Heterochromatin in Domain-size-dependent Bursts. Proc. Natl. Acad. Sci. 118, e2022887118. doi:10.1073/pnas.2022887118
Nicodemi, M., and Prisco, A. (2009). Thermodynamic Pathways to Genome Spatial Organization in the Cell Nucleus. Biophysical J. 96, 2168–2177. doi:10.1016/j.bpj.2008.12.3919
Nonomura, M. (2012). Study on Multicellular Systems Using a Phase Field Model. PLoS One 7, e33501. doi:10.1371/journal.pone.0033501
Nott, T. J., Petsalaki, E., Farber, P., Jervis, D., Fussner, E., Plochowietz, A., et al. (2015). Phase Transition of a Disordered Nuage Protein Generates Environmentally Responsive Membraneless Organelles. Mol. Cel 57, 936–947. doi:10.1016/j.molcel.2015.01.013
Nuebler, J., Fudenberg, G., Imakaev, M., Abdennur, N., and Mirny, L. A. (2018). Chromatin Organization by an Interplay of Loop Extrusion and Compartmental Segregation. Proc. Natl. Acad. Sci. USA 115, E6697–E6706. doi:10.1073/pnas.1717730115
Pak, C. W., Kosno, M., Holehouse, A. S., Padrick, S. B., Mittal, A., Ali, R., et al. (2016). Sequence Determinants of Intracellular Phase Separation by Complex Coacervation of a Disordered Protein. Mol. Cel 63, 72–85. doi:10.1016/j.molcel.2016.05.042
Peng, A, and Weber, S. C. (2019). Evidence for and against Liquid-Liquid Phase Separation in the Nucleus. Noncoding RNA 5, 50. doi:10.3390/ncrna5040050
Qi, Y., and Zhang, B. (2021). Chromatin Network Retards Nucleoli Coalescence. Nat. Commun. 12, 6824. doi:10.1038/s41467-021-27123-9
Rao, S. S. P., Huntley, M. H., Durand, N. C., Stamenova, E. K., Bochkov, I. D., Robinson, J. T., et al. (2014). A 3D Map of the Human Genome at Kilobase Resolution Reveals Principles of Chromatin Looping. Cell 159, 1665–1680. doi:10.1016/j.cell.2014.11.021
Razin, S. V., and Ulianov, S. V. (2020). enDivide and Rule: Phase Separation in Eukaryotic Genome Functioning. Cells 9, 2480. doi:10.3390/cells9112480
Reynolds, N., McEvoy, E., Ghosh, S., Panadero Pérez, J. A., Neu, C. P., and McGarry, P. (2021). Image-derived Modeling of Nucleus Strain Amplification Associated with Chromatin Heterogeneity. Biophysical J. 120, 1323–1332. doi:10.1016/j.bpj.2021.01.040
Saintillan, D., Shelley, M. J., and Zidovska, A. (2018). Extensile Motor Activity Drives Coherent Motions in a Model of Interphase Chromatin. Proc. Natl. Acad. Sci. USA 115, 11442–11447. doi:10.1073/pnas.1807073115
Sanulli, S., and Narlikar, G. J. (2020). Liquid-like Interactions in Heterochromatin: Implications for Mechanism and Regulation. Curr. Opin. Cel Biol. 64, 90–96. doi:10.1016/j.ceb.2020.03.004
Shaban, H. A., Barth, R., and Bystricky, K. (2018). Formation of Correlated Chromatin Domains at Nanoscale Dynamic Resolution during Transcription. Nucleic Acids Res. 46, e77. doi:10.1093/nar/gky269
Shaban, H. A., and Seeber, A. (2020). Monitoring the Spatio-Temporal Organization and Dynamics of the Genome. Nucleic Acids Res. 48, 3423–3434. doi:10.1093/nar/gkaa135
Shi, G., and Thirumalai, D. (2019). Conformational Heterogeneity in Human Interphase Chromosome Organization Reconciles the Fish and Hi-C Paradox. Nat. Commun. 10, 3894–3910. doi:10.1038/s41467-019-11897-0
Shi, G., Liu, L., Hyeon, C., and Thirumalai, D. (2018). Interphase Human Chromosome Exhibits Out of Equilibrium Glassy Dynamics. Nat. Commun. 9, 3161. doi:10.1038/s41467-018-05606-6
Shinkai, S., Nakagawa, M., Sugawara, T., Togashi, Y., Ochiai, H., Nakato, R., et al. (2020). PHi-C: Deciphering Hi-C Data into Polymer Dynamics. NAR Genom Bioinform 2, lqaa020. doi:10.1093/nargab/lqaa020
Smith, K. P., Hall, L. L., and Lawrence, J. B. (2020). Nuclear Hubs Built on RNAs and Clustered Organization of the Genome. Curr. Opin. Cel Biol. 64, 67–76. doi:10.1016/j.ceb.2020.02.015
Sneppen, K., and Ringrose, L. (2019). Theoretical Analysis of Polycomb-Trithorax Systems Predicts that Poised Chromatin Is Bistable and Not Bivalent. Nat. Commun. 10, 2133–2218. doi:10.1038/s41467-019-10130-2
Stephens, A. D., Banigan, E. J., and Marko, J. F. (2019). Chromatin’s Physical Properties Shape the Nucleus and its Functions. Curr. Opin. Cel Biol. 58, 76–84. doi:10.1016/j.ceb.2019.02.006
Strickfaden, H., Tolsma, T. O., Sharma, A., Underhill, D. A., Hansen, J. C., and Hendzel, M. J. (2020). Condensed Chromatin Behaves like a Solid on the Mesoscale In Vitro and in Living Cells. Cell 183 (7), 1772–1784.e13. doi:10.1016/j.cell.2020.11.027
Su, J.-H., Zheng, P., Kinrot, S. S., Bintu, B., and Zhuang, X. (2020). Genome-scale Imaging of the 3d Organization and Transcriptional Activity of Chromatin. Cell 182, 1641–1659.e26. doi:10.1016/j.cell.2020.07.032
Tiana, G., and Giorgetti, L. (2019). Modeling the 3D Conformation of Genomes. Boca Raton, FL: CRC Press.
Tortora, M. M., Salari, H., and Jost, D. (2020). Chromosome Dynamics during Interphase: a Biophysical Perspective. Curr. Opin. Genet. Dev. 61, 37–43. doi:10.1016/j.gde.2020.03.001
Tripathi, K., and Menon, G. I. (2019). Chromatin Compaction, Auxeticity, and the Epigenetic Landscape of Stem Cells. Phys. Rev. X 9, 041020. doi:10.1103/physrevx.9.041020
van Berkum, N. L., Lieberman-Aiden, E., Williams, L., Imakaev, M., Gnirke, A., Mirny, L. A., et al. (2010). Hi-c: A Method to Study the Three-Dimensional Architecture of Genomes. JoVE 6, e1869. doi:10.3791/1869
Yildirim, A., Boninsegna, L., Zhan, Y., and Alber, F. (2021). Uncovering the Principles of Genome Folding by 3d Chromatin Modeling. Cold Spring Harb Perspect. Biol. 16, a039693. doi:10.1101/cshperspect.a039693
Zenk, F., Zhan, Y., Kos, P., Löser, E., Atinbayeva, N., Schächtle, M., et al. (2021). HP1 Drives De Novo 3D Genome Reorganization in Early Drosophila Embryos. Nature 593, 289–293. doi:10.1038/s41586-021-03460-z
Zidovska, A. (2020a). The Rich Inner Life of the Cell Nucleus: Dynamic Organization, Active Flows, and Emergent Rheology. Biophys. Rev. 12, 1093–1106. doi:10.1007/s12551-020-00761-x
Zidovska, A. (2020b). The Self-Stirred Genome: Large-Scale Chromatin Dynamics, its Biophysical Origins and Implications. Curr. Opin. Genet. Dev. 61, 83–90. doi:10.1016/j.gde.2020.03.008
Keywords: chromatin, liquid-liquid phase separation, heterochromatin, euchromatin, nuclear organization, lamin, imaging, mesoscale
Citation: Laghmach R, Di Pierro M and Potoyan D (2022) A Liquid State Perspective on Dynamics of Chromatin Compartments. Front. Mol. Biosci. 8:781981. doi: 10.3389/fmolb.2021.781981
Received: 23 September 2021; Accepted: 22 December 2021;
Published: 13 January 2022.
Edited by:
Winston Zhao, National University of Singapore, SingaporeReviewed by:
Andrey Cherstvy, University of Potsdam, GermanySrivastav Ranganathan, Harvard University, United States
Copyright © 2022 Laghmach, Di Pierro and Potoyan. This is an open-access article distributed under the terms of the Creative Commons Attribution License (CC BY). The use, distribution or reproduction in other forums is permitted, provided the original author(s) and the copyright owner(s) are credited and that the original publication in this journal is cited, in accordance with accepted academic practice. No use, distribution or reproduction is permitted which does not comply with these terms.
*Correspondence: Davit Potoyan, potoyan@iastate.edu