- 1Department of Central Laboratory, Cancer Hospital of China Medical University, Liaoning Cancer Hospital and Institute, Shenyang, China
- 2Department of Biobank, Cancer Hospital of China Medical University, Liaoning Cancer Hospital and Institute, Shenyang, China
- 3Department of Medical Imaging, Cancer Hospital of China Medical University, Liaoning Cancer Hospital and Institute, Shenyang, China
- 4Department of Neurosurgery, Cancer Hospital of China Medical University, Liaoning Cancer Hospital and Institute, Shenyang, China
Accumulating evidence has implicated members of the procollagen-lysine, 2-oxoglutarate 5-dioxygenase (PLOD) gene family, PLOD1, PLOD2, and PLOD3, in cancer progression and metastasis. However, their expression, prognostic value, and mechanisms underlying their roles in lung adenocarcinoma (LUAD) have not yet been reported. We downloaded PLOD data for LUAD and normal tissues from The Cancer Genome Atlas (TCGA). PLOD1-3 protein expression was evaluated using the Clinical Proteomics Tumor Analysis Consortium and Human Protein Atlas. Survival analysis was performed using the Kaplan–Meier method. A protein–protein interaction network was constructed using STRING software. The “ClusterProfiler” package was used for functional-enrichment analysis. The relationship between PLOD mRNA expression and immune infiltration was analyzed using the Tumor Immunity Assessment Resource and Tumor Immune System Interaction Database. The expression of PLODs in LUAD tissues was significantly upregulated compared with that in adjacent normal tissues. PLOD mRNA overexpression is associated with lymph node metastasis and high TNM staging. Receiver operating characteristic curve analysis showed that when the cut-off level was 6.073, the accuracy, sensitivity, and specificity of PLOD1 in distinguishing LUAD from adjacent controls were 84.4, 79.7, and 82.6%, respectively. The accuracy, sensitivity, and specificity of PLOD2 in distinguishing LUAD from adjacent controls were 81.0, 98.3, and 68.0%, respectively, at a cut-off value of 4.360. The accuracy, sensitivity, and specificity of PLOD3 in distinguishing LUAD from adjacent controls were 69.0, 86.4, and 52.0%, respectively, with a cut-off value of 5.499. Kaplan–Meier survival analysis demonstrated that LUAD patients with high PLODs had a worse prognosis than those with low PLODs. Correlation analysis showed that PLOD mRNA expression was related to immune infiltration and tumor purity. Upregulation of PLOD expression was significantly associated with poor survival and immune cell infiltration in LUAD. Our research shows that PLOD family members have potential as novel biomarkers for poor prognosis and as potential immunotherapy targets for LUAD.
Introduction
Lung cancer is one of the most malignant tumors that threaten human lives and health. Statistics on the pathological classification of lung cancer show that the most important type is non-small cell lung cancer (NSCLC), accounting for approximately 80% of all lung cancers. Lung adenocarcinoma (LUAD) is a common clinical type of NSCLC (Shergalis et al., 2020; Li et al., 2021). Although some progress has been made in surgical resection, chemotherapy, and radiotherapy, the 5-year survival rate of patients with LUAD remains low. The main reasons for this may be immunosuppression, cell proliferation, distant metastases, and drug resistance (Chen et al., 2021). Therefore, there is an urgent need to identify new, early diagnostic biomarkers and therapeutic targets for LUAD.
The procollagen-lysine, 2-ketoglutarate 5-dioxygenase (PLOD) gene family includes three members, PLOD1, PLOD2, and PLOD3, located on chromosomes 1p, 3q, and 7q, respectively. Multiple studies have shown that PLOD genes play essential roles in the development and progression of cancer (Guo et al., 2021a). Other studies have shown that, compared with normal kidney tissue, the expression of PLOD genes is increased in hepatocellular carcinoma (HCC) and positively correlated with the prognosis of HCC patients (Yang et al., 2020). In addition, bioinformatics analysis has shown that compared with adjacent normal tissues, PLOD gene family members are expressed at higher levels in LUAD tissues. However, the expression and prognostic effects of PLOD family members in LUAD require further elucidation.
To the best of our knowledge, the prognostic value of PLODs in LUAD and their correlation with immune infiltration are still not fully understood. To test this hypothesis, we evaluated TCGA database data and performed other bioinformatics analyses of the prognostic role of the PLOD family in LUAD. We further investigated the correlation between PLOD and immune cell infiltration.
Materials and Methods
Datasets Obtained From the Cancer Genome Atlas (TGCA) Datasets
TCGA lung adenocarcinoma (TCGA disease code: LUAD) cohorts were downloaded from TCGA official website (https://portal.gdc.cancer.gov/) (Tomczak et al., 2015). The 33 enrolled cancer types contained at least five samples in the normal group. At last, the RNA-Seq gene expression data with workflow type of fragments per kilobase per million (FPKM) were subsequently transformed to transcripts per million (TPM) and log2 conversion for further study. Since all the data are available from TCGA, no approval by an ethics committee is required.
RNA Sequencing (RNA-Seq) Data of PLODs in LUAD
Expression of PLODs in the RNA-Seq expression data in LUAD were also obtained from TCGA. This included 59 normal lung samples (normal-adjacent tissue to LUAD) and 535 LUAD tissue data. Specimen characteristics and associated clinical information including age, gender, smoker condition, tumor stage, and location are described. The mRNA expression data were summarized with means ± SD.
Clinical Proteomic Tumor Analysis Consortium (CPTAC)
The protein expression profile was downloaded from the CPTAC resource (https://cptac-data-portal.georgetown.edu) and the proteome of each tumor sample characterized (Edwards et al., 2015). UALCAN (Chandrashekar et al., 2017) is a user-friendly online web resource. The R package “Seurat” was applied for data standardization. Then, the plots were drawn with the given cell classification using R package “ggplot2”. In this study, a throughput analysis of PLOD protein expression was presented.
Biopsy Immunohistochemical Micrographs From the Human Protein Atlas (HPA)
The HPA (https://www.proteinatlas.org/) provides a map showing the distribution and relative abundance of human genes on protein level in normal human tissues and LUAD tissues (Uhlen et al., 2015; Uhlen et al., 2017). The HPA was used to assess tissue protein expression profiles.
Definition of Protein–Protein Interaction (PPI) Modules
Genes form PPI networks at any level that fulfill biological roles, and perturbing protein networks is an ordinary characteristic of disease-associated mutations. Construction of a PPI network was studied by STRING tool (https://www.string-db.org/) (an online database for the retrieval of interacting genes) (Szklarczyk et al., 2011). Gene ontology (GO) enrichment was performed for the categories gene ontology biological process, molecular function, cellular compartment, and Kyoto Encyclopedia of Genes and Genomes (KEGG) (https://www.kegg.jp/) (Yu et al., 2012).
Quantification of Immune Infiltration Based on Key Genes
Immune infiltrate estimation was explored to comprehensively analyze the correlation between PLOD genes and tumor-infiltrating immune components by the Tumor Immune Estimation Resource (TIMER) platform (http://timer.cistrome.org/) (Li et al., 2017). The infiltrating immune cells including B cells, CD4+ T cells, CD8+ T cells, neutrophils, macrophages, and dendritic cells.
Tumor-Immune System Interaction Database (TISIDB) Analysis
The integrated repository portal for tumor-immune system interactions with PLODs genes was assessed in TISIDB (Ru et al., 2019). Based on the gene expression profile, the relative abundance of tumor-infiltrating lymphocytes (TILs) was inferred by Gene Set Variation Analysis (GSVA). Spearman’s correlation test was conducted to explore the relation between numerical variables.
PrognoScan Database
PrognoScan database (http://dna00.bio.kyutech.ac.jp/PrognoScan/index.html) (Mizuno et al., 2009) provides a powerful online platform for evaluating potential tumor markers and therapeutic targets, as well as a tool for assessing the biological association between gene expression and prognosis. The correlation between PLOD gene expression and survival in LUAD was analyzed by the PrognoScan database (jacob-00182-CANDF, jacob-00182-MSK, jacob-00182-UM GSE31210, HARVARD-LC, MICHIGAN-LC, and jacob-00182-CANDF).
Prognostic Value of PLODs
The Gene Expression Profiling Interactive Analysis (GEPIA) contains high-throughput RNA sequencing data (TCGA and GTEx databases). Prognostic value, including OS and RFS, based on PLOD expression was generated by GEPIA database (http://gepia2.cancer-pku.cn/) (Tang et al., 2017).
Statistical Analyses
Data were performed with R (V 3.5.3) and graphs were generated using the ggplot2 package in R. Statistical significance was measured by paired t-test and Mann–Whitney U test. The “pROC” package (Robin et al., 2011) was performed to calculate the cut-off value. Kaplan–Meier curves were determined by using log-rank tests.
Results
Pan-Cancer Analyses
The mRNA expression levels of PLODs were analyzed using TCGA database. Our analysis revealed that 14 of 33 cancer types exhibited the highest mRNA expression of PLOD1 among the TCGA cohorts. Compared with normal tissues, PLOD2 was significantly upregulated in 11 cancer types. In addition, PLOD3 expression was markedly increased in 17 cancer types. These data imply that PLODs are often overexpressed across different cancer types, especially LUAD (Figure 1). The detailed data are presented in Supplementary Tables 1–3.
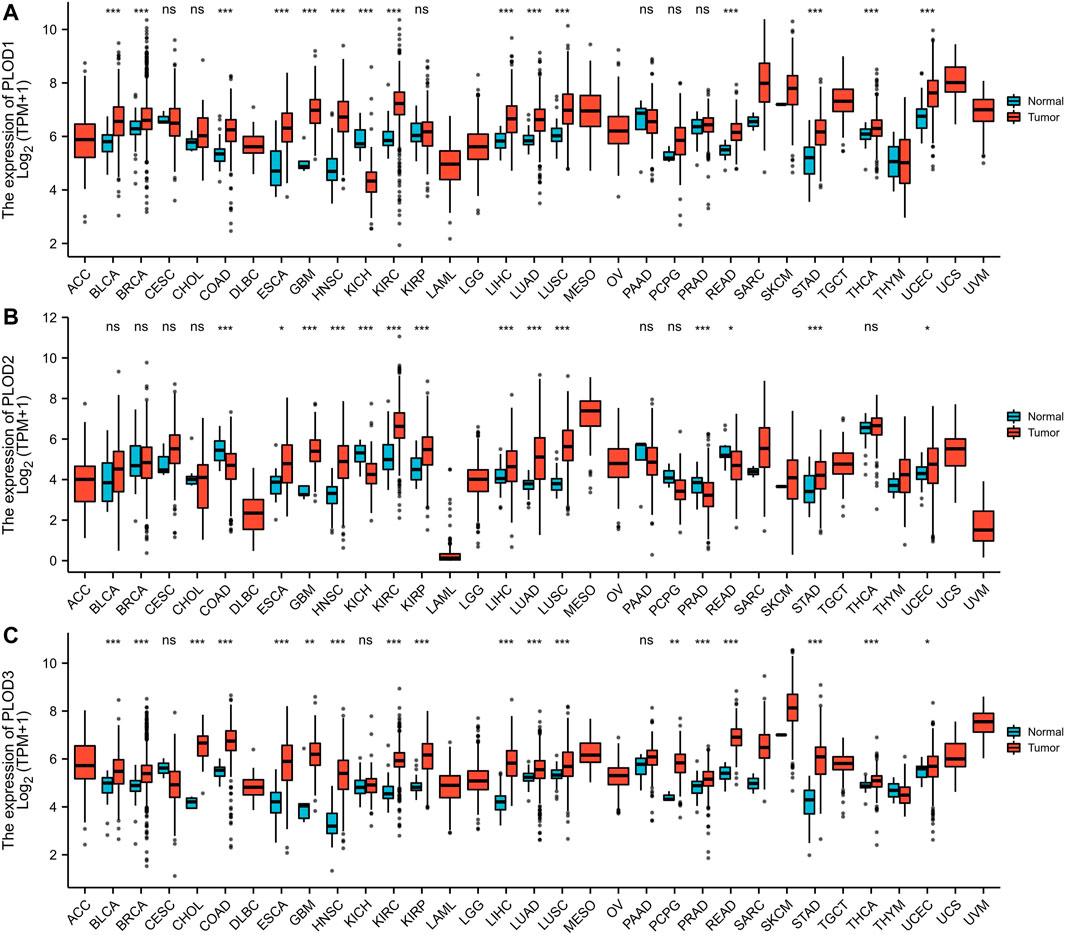
FIGURE 1. Pan-cancer analyses. (A) mRNA expression of PLOD1 upregulated in 14 of 33 cancer types; (B) mRNA expression of PLOD2 upregulated in 11 of 33 cancer types; (C) mRNA expression of PLOD3 upregulated in 17 of 33 cancer types. *p < 0.05, *p < 0.01, ***p < 0.001. ns, no significance; ACC, adrenocortical carcinoma; BLCA, bladder urothelial carcinoma; BRCA, breast invasive carcinoma; CESC, cervical squamous cell carcinoma and endocervical adenocarcinoma; CHOL, cholangiocarcinoma; COAD, colon adenocarcinoma; DLBC, lymphoid neoplasm diffuse large B-cell lymphoma; ESCA, esophageal carcinoma; GBM, glioblastoma multiforme; HNSC, head and neck squamous cell carcinoma; KICH, kidney chromophobe; KIRC, kidney renal clear cell carcinoma; KIRP, kidney renal papillary cell carcinoma; LAML, acute myeloid leukemia; LGG, brain lower grade glioma; LIHC, liver hepatocellular carcinoma; LUAD, lung adenocarcinoma; LUSC, lung squamous cell carcinoma; MESO, mesothelioma; OV, ovarian serous cystadenocarcinoma; PRAD, prostate adenocarcinoma; PCPG, pheochromocytoma and paraganglioma; READ, rectum adenocarcinoma; SARC, sarcoma; SKCM, skin cutaneous melanoma; STAD, stomach adenocarcinoma; THCA, thyroid carcinoma; THYM, thymoma; UCEC, uterine corpus endometrial carcinoma; UCS, uterine carcinosarcoma; UVM, uveal melanoma.
mRNA Expression
Expression of PLODs in normal lung (59 cases) and LUAD (535 cases) tissues from TCGA database were analyzed. Expression levels of PLOD mRNAs in LUAD tumor tissues were higher than those in adjacent normal tissues (Figure 2A). In subsequent analyses, the Mann–Whitney U test for unpaired data showed a significant difference between the expression levels of PLOD mRNAs in LUAD tissues and adjacent normal tissues (Figure 2B).
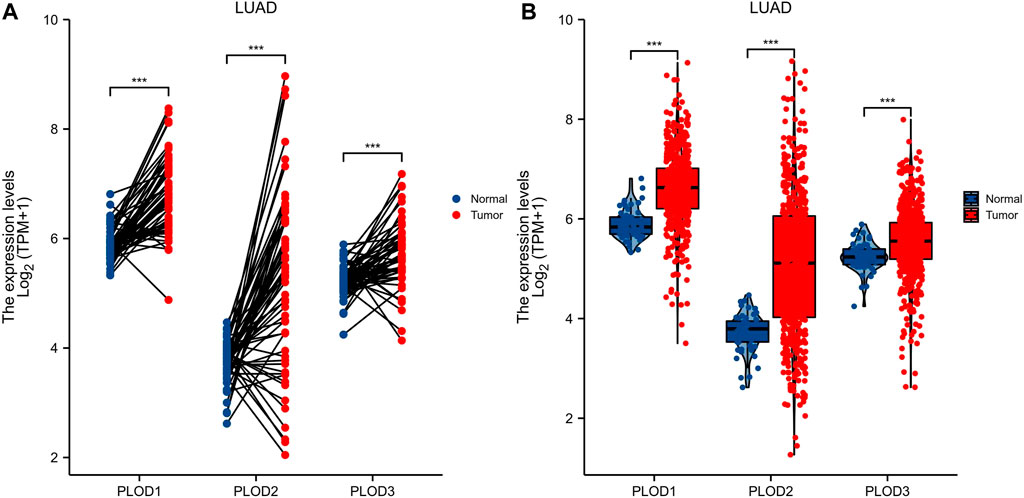
FIGURE 2. PLOD mRNA expression. Through TCGA database data screening and analysis, the expression of PLOD family genes in normal tissues and LUAD tissue samples was determined. (A) mRNA expression levels of PLOD1, PLOD2, and PLOD3 in LUAD tissues and matched adjacent normal samples. (B) mRNA expression levels of PLOD family genes in 535 lung adenocarcinoma samples and 59 normal samples. The results showed that, compared with normal lung tissue, the expression of PLOD1, PLOD2, and PLOD3 mRNA in LUAD increased significantly.
Protein Pathological Expression in Lung Tissue
To determine PLOD protein expression, we mined these data using CPTAC with UALCAN. We found that the protein expression of PLODs in LUAD was significantly higher (Figure 3A). Transcript data from the HPA and the corresponding staining patterns of PLODs were further supported by TCGA datasets. As shown in Figure 3B, PLOD1 is located in human cell vesicles and the nucleoplasm, PLOD2 is located in the nucleoli and cytosol, and PLOD3 is secreted by cells. In line with previous data, both mRNA and protein were overexpressed in LUAD tissues.
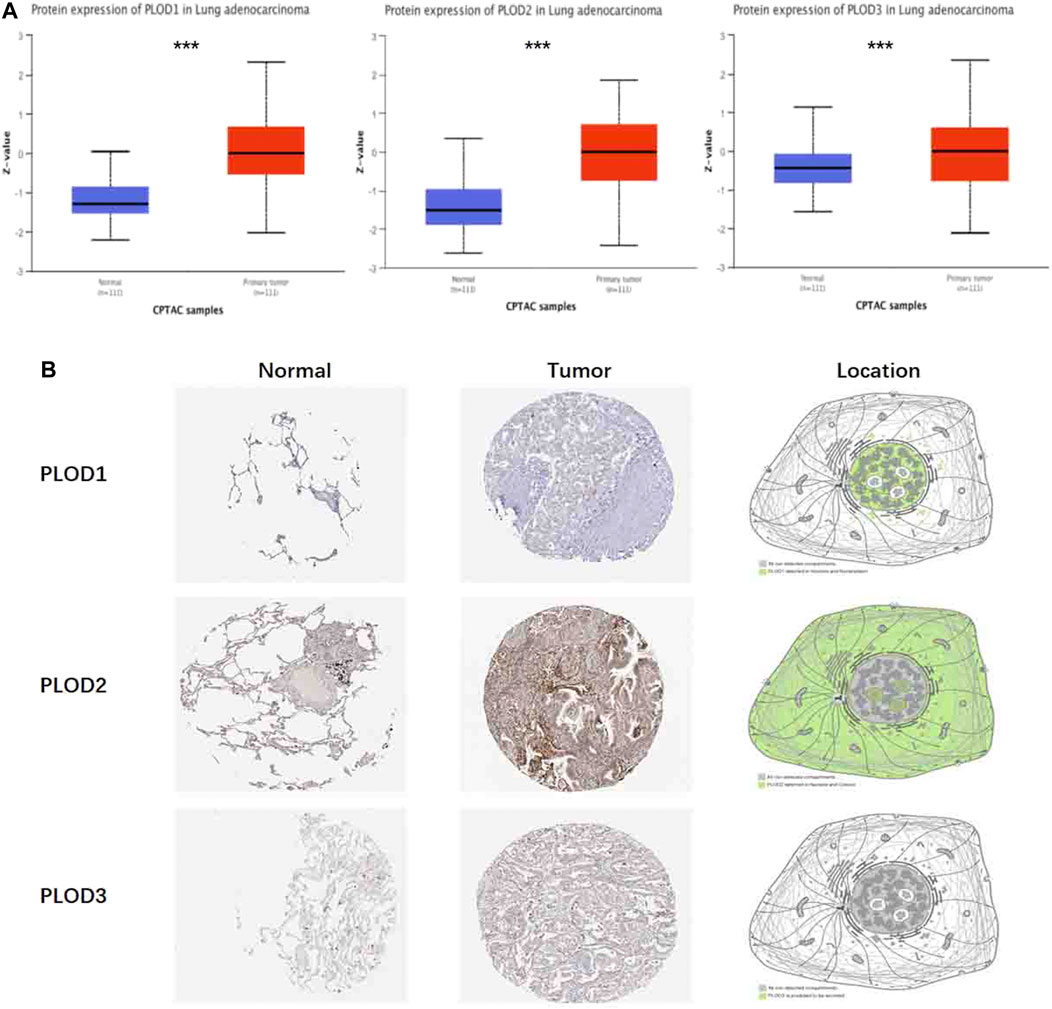
FIGURE 3. Protein pathological expression in lung tissue. To facilitate access to the protein expression of PLOD1, PLOD2, and PLOD3 in LUAD, CPTAC data were integrated with the UALCAN data portal (A), and the differential expression and location of PLOD1-3 were observed in the HPA (***p < 0.001) (B). PLOD1: normal tissue, https://www.proteinatlas.org/ENSG00000083444-PLOD1/tissue/lung; tumor tissue, https://www.proteinatlas.org/ENSG00000083444-PLOD1/pathology/lung + cancer. PLOD2: normal tissue, https://www.proteinatlas.org/ENSG00000152952-PLOD2/tissue/lung; tumor tissue, https://www.proteinatlas.org/ENSG00000152952-PLOD2/pathology. PLOD3: normal tissue, https://www.proteinatlas.org/ENSG00000106397-PLOD3/tissue/lung; tumor tissue, https://www.proteinatlas.org/ENSG00000106397-PLOD3/pathology/lung + cancer.
Relationships Between PLOD Expression Data and LUAD Clinicopathological Characteristics
We applied the Mann–Whitney U test and logistic regression analysis. As shown in Table 1, Table 2, Table 3, and Figure 4A-L, PLOD expression was correlated with clinical stage, T stage, OS, histopathological grade, and smoking history. However, PLOD expression and other clinicopathological characteristics, including age, smoking status, M stage, sex, and anatomical location (right and left; periphery and center), were not statistically significant. In summary, these results indicate that PLOD1 and PLOD2 may serve as biomarkers for poor prognosis of LUAD.
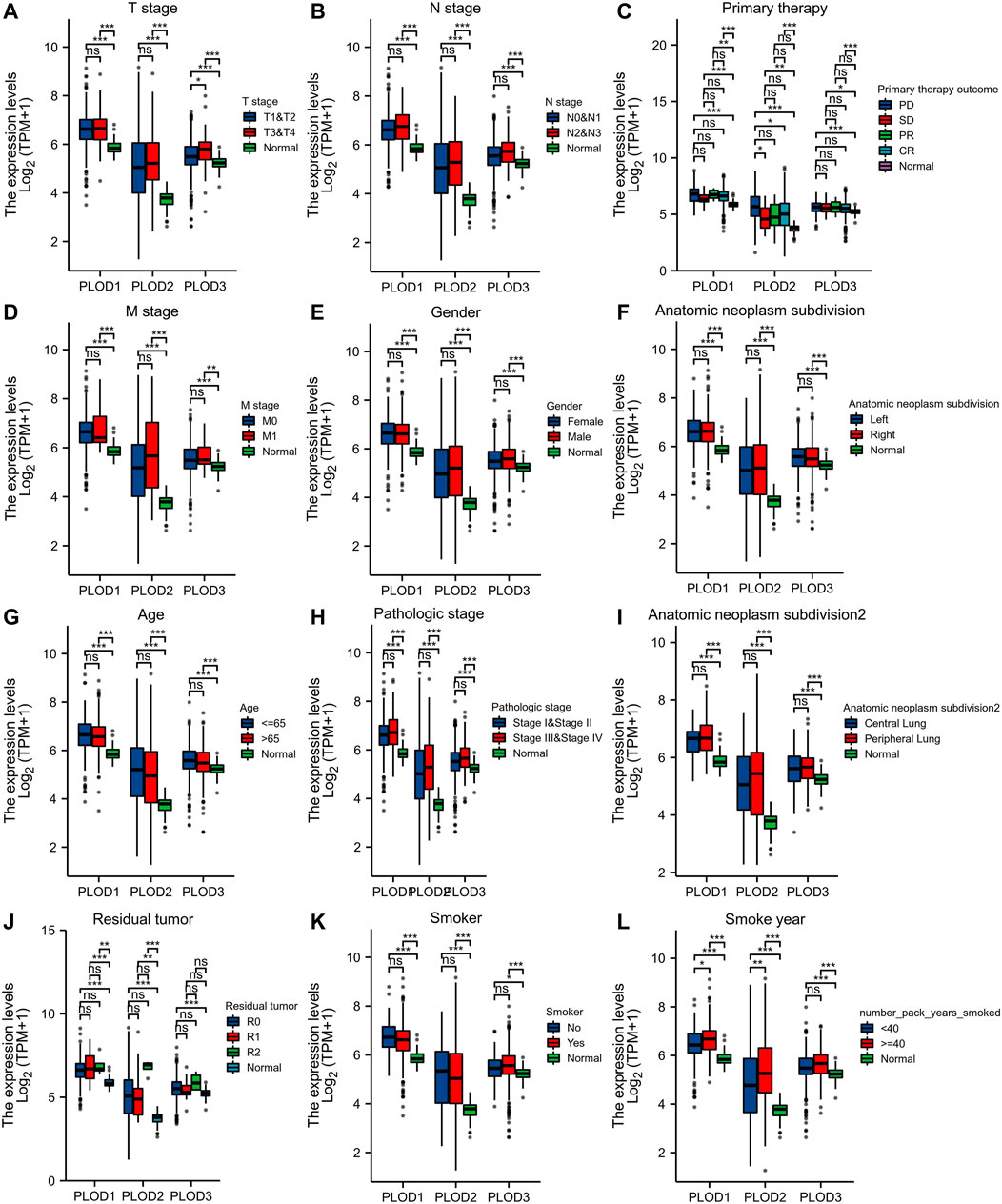
FIGURE 4. Evaluate relationship between mRNA expression level and various clinicopathological characteristics. The association of mRNA with clinical pathological characteristics including T stage (A), lymph node metastases (B), primary therapy outcome (C), M stage (D), sex (E), anatomic neoplasm subdivision (F), age (G), pathologic stage (H), anatomic neoplasm subdivision 2 (I), residual tumor (J), smoker (K), and smoking status (L) are demonstrated in this plot. ns, no significance, *p < 0.05, **p < 0.01, ***p < 0.001.
Potential Biomarkers for Distinguishing LUAD Samples From Normal Samples
ROC curve analysis showed (Figure 5A) significant predictive potential for PLOD1 (AUC = 0.844, CI = 0.806–0.881) and PLOD2 (AUC = 0.810, CI = 0.776–0.844). However, the predictive potential for PLOD3 was lower (AUC = 0.690, CI = 0.641–0.740). DeLong’s test showed that PLOD1 was superior to PLOD2 in predicting tumor and normal outcomes, but the test was not statistically significant (p = 0.148); PLOD1 was significantly superior to PLOD3 (p ≤ 0.01). The diagnostic efficiency of PLOD2 was significantly better than that of PLOD3 (p ≤ 0.01). Kaplan–Meier curves for the three PLOD family members showed that the OS of LUAD patients with high PLOD2 mRNA expression was shorter than that of patients with low PLOD2, and the difference was statistically significant.
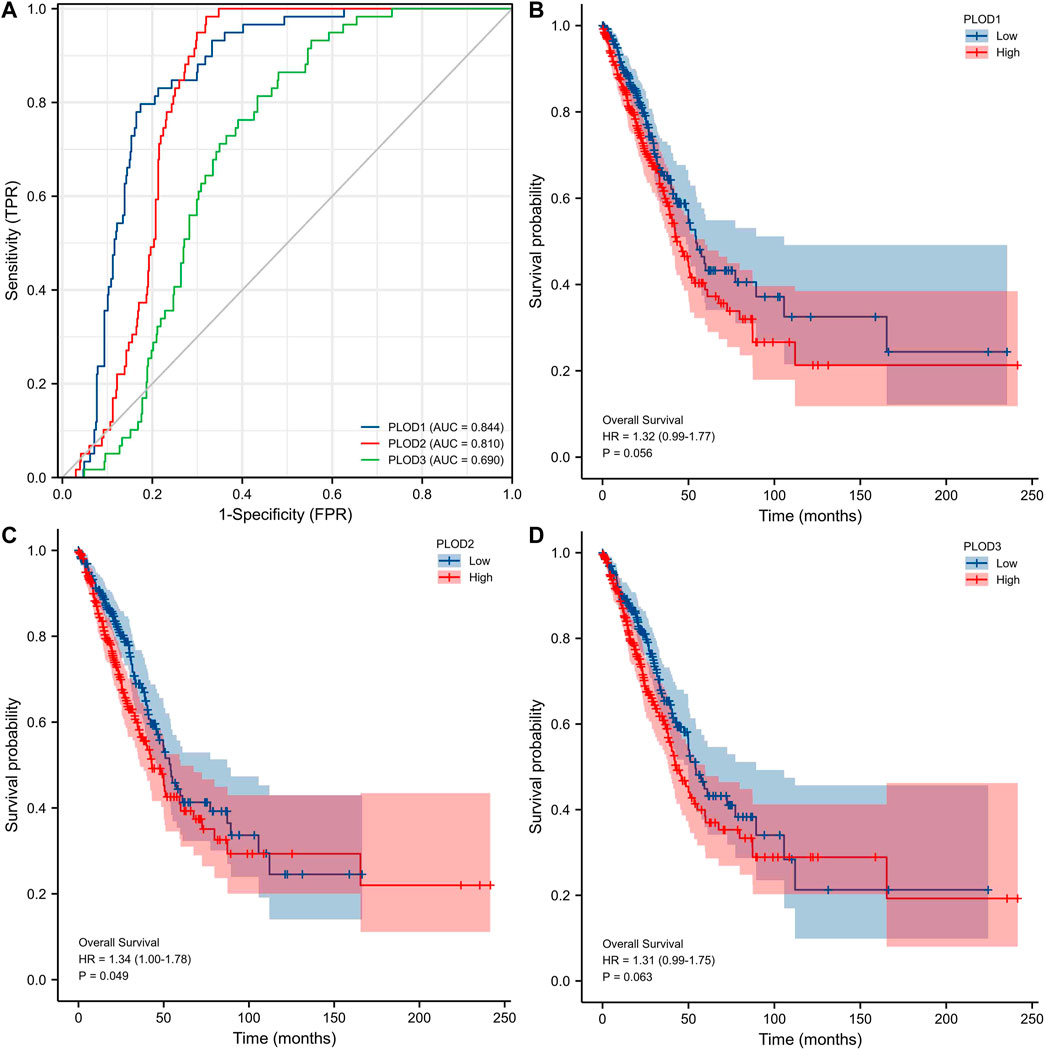
FIGURE 5. Prospective biomarkers for distinguishing LUAD from normal samples. The survival ROC package in the R language was used to generate the ROC curve. (A) ROC curve showing that PLOD1 and PLOD2 can distinguish lung adenocarcinoma tissue from healthy controls. (B–D) The Kaplan–Meier curve of the PLOD gene family showed that the OS of LUAD patients with high PLOD2 mRNA expression was shorter than that of patients with low PLOD2, and the difference was statistically significant.
PPI Network Analysis and Biological Function Annotation
Using the STRING database, Figure 6A, Figure 7A, and Figure 8A show networks of 10 co-expressed genes related to PLODs in LUAD patients. For PLOD1, the network included COL1A1, COL1A2, COL2A1, COL3A1, COL4A1, COL4A2, COL5A1, COL5A2, COLGALT1, and PLOD2. For PLOD2, the network included COL12A1, COL1A1, COL1A2, COL3A1, COL4A1, COL4A2, COL5A1, COL5A2, COLGALT1, and PLOD1. For PLOD3, the network included COL1A1, COL1A2, COL3A1, COL4A1, COL4A2, COL4A5, COL5A3, COL7A1, COLGALT1, and COLGALT2). As shown in Figure 6, Figure 7, and Figure 8B, the biological processes for PLODs were all related to collagen fibril, extracellular matrix, and extracellular structure organization. The functional annotations for PLOD1 were protein digestion and absorption, ECM–receptor interaction, and the AGE-RAGE signaling pathway in diabetic complications. The functional annotations of PLOD2 and PLOD3 were protein digestion and absorption, amoebiasis, and AGE-RAGE signaling pathways in diabetic complications. Correlation analyses between the expression of PLODs and TCGA LUAD co-expressed genes are shown in Figures 6C–L, Figures 7C–L, and Figures 8C–L.
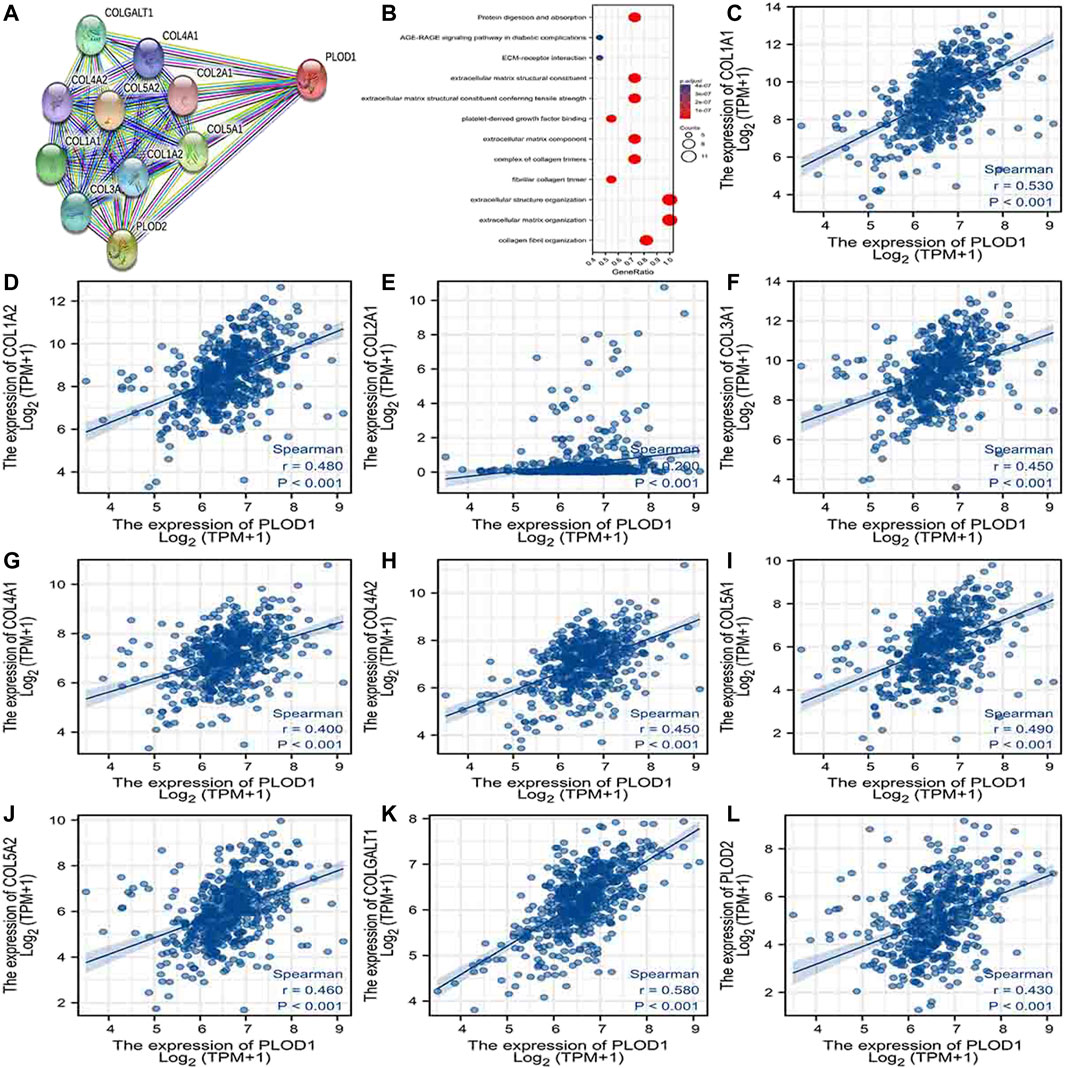
FIGURE 6. PLOD1 PPI network and biological function annotation. (A) Network of PLOD1 and its co-expressed genes. (B) Functional enrichment analysis of 10 network genes. PLOD1 is associated with collagen fibril organization, extracellular matrix organization, and extracellular structural organization. These genes are involved in protein digestion and absorption, ECM–receptor interactions, and the AGE-RAGE signaling pathway in diabetic complications. (C–L) Correlations between the expression of PLOD1 and co-expressed genes in LUAD. COL1A1, collagen alpha-1(I) chain; COL1A2, collagen alpha-2(I) chain; COL2A1, collagen alpha-1(II) chain; COL3A1, collagen alpha-1(III) chain; COL4A1, collagen alpha-1(IV) chain; COL4A2, collagen alpha-2(IV) chain; COL5A1, collagen alpha-1(V) chain; COL5A2, collagen alpha-2(V) chain; COLGALT1, procollagen galactosyltransferase 1; PLOD2: procollagen-lysine,2-oxoglutarate 5-dioxygenase 2.
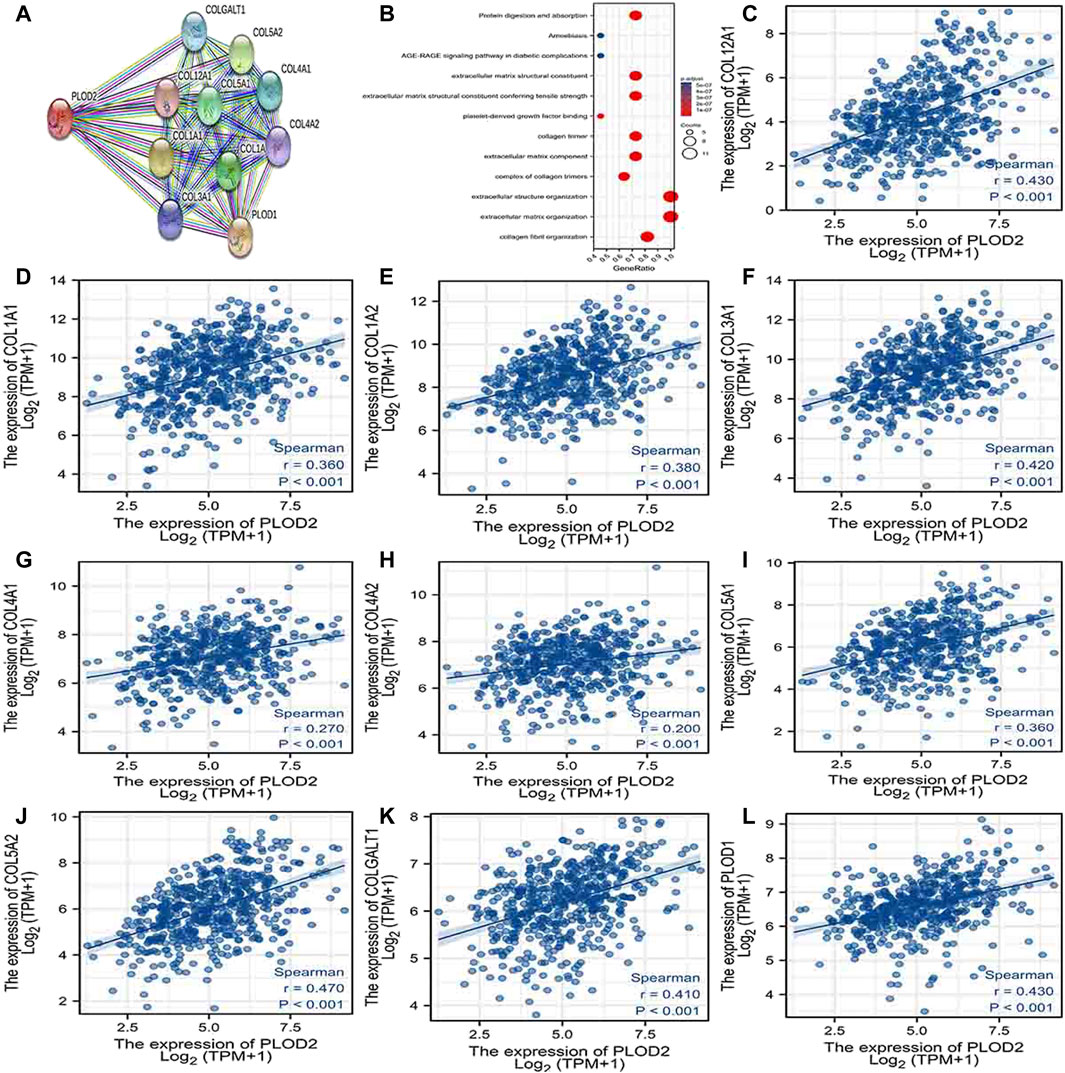
FIGURE 7. PLOD2 PPI network and biological function annotation. (A) Network of PLOD2 and its co-expressed genes. (B) Functional enrichment analysis of 10 network genes. PLOD2 was associated with collagen fibril organization, extracellular matrix organization, and extracellular structure organization. These genes are involved in protein digestion and absorption, amoebiasis, and AGE-RAGE signaling in diabetes. (C–L) Correlations between the expression of PLOD1 and co-expressed genes in lung adenocarcinoma. COL12A1, collagen alpha-1 (XII) chain; COL1A1, collagen alpha-1(I) chain; COL1A2, collagen alpha-2(I) chain; COL3A1, collagen alpha-1(III) chain; COL4A1, collagen alpha-1(IV) chain; COL4A2, collagen alpha-2(IV) chain; COL5A1, collagen alpha-1(V) chain; COL5A2, collagen alpha-2(V) chain; COLGALT1, procollagen galactosyltransferase 1; PLOD1: procollagen-lysine,2-oxoglutarate 5-dioxygenase 1.
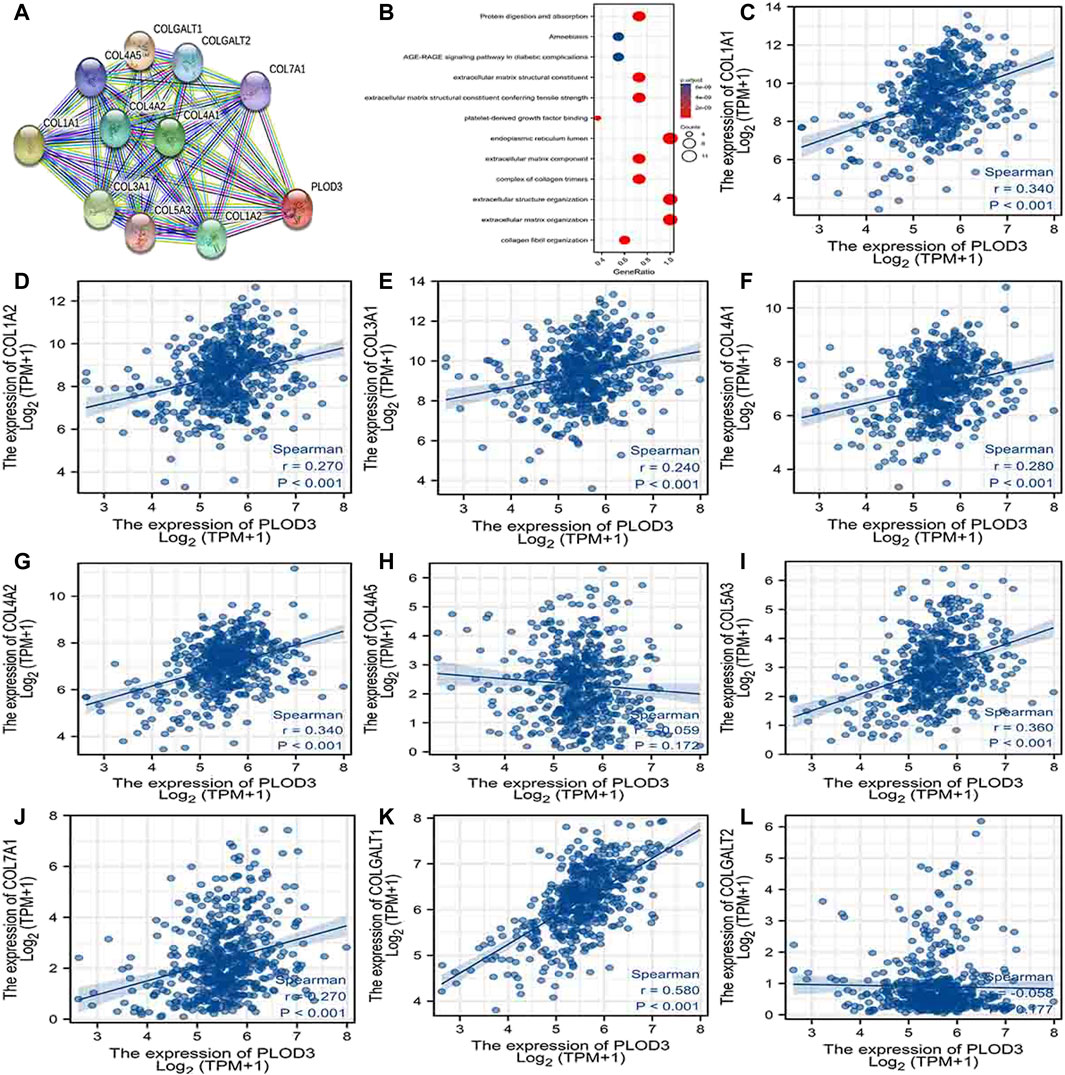
FIGURE 8. PLOD3 PPI network and biological function annotation. (A) Network of PLOD3 and its co-expressed genes. (B) Functional enrichment analysis of 10 network genes. PLOD3 was associated with collagen fibril organization, extracellular matrix organization, and extracellular structure organization. These genes were involved in protein digestion and absorption, amoebiasis, and AGE-RAGE signaling in diabetes. (C–L) Correlations between the expression of PLOD3 and co-expressed genes in lung adenocarcinoma. COL1A1, collagen alpha-1(I) chain; COL1A2, collagen alpha-2(I) chain; COL3A1, collagen alpha-1(III) chain; COL4A1, collagen alpha-1(IV) chain; COL4A2, collagen alpha-2(IV) chain; COL4A5, collagen alpha-5(IV) chain; COL5A3, collagen alpha-3(V) chain; COL7A1, collagen alpha-1(VII) chain; COLGALT1, procollagen galactosyltransferase 1; COLGALT2, procollagen galactosyltransferase 2.
Associations With Target Genes and Immune Infiltration
We used TCGA to analyze PLODs and overall immune components in tumor immunosuppressive networks (Figures 9A, 10, and 11A). We next expanded our analysis by interrogating TIMER (Figure 9B–L, Figure 10B–L, and Figure 11B–L). We found that in the LUAD patient cohort, high PLOD expression levels were negatively correlated with TFH cells, CD8 T cells, Tcm cells, and B-cell immune infiltration. In contrast, high PLOD1-3 expression was positively correlated with Tregs, macrophages, and cancer-associated fibroblasts.
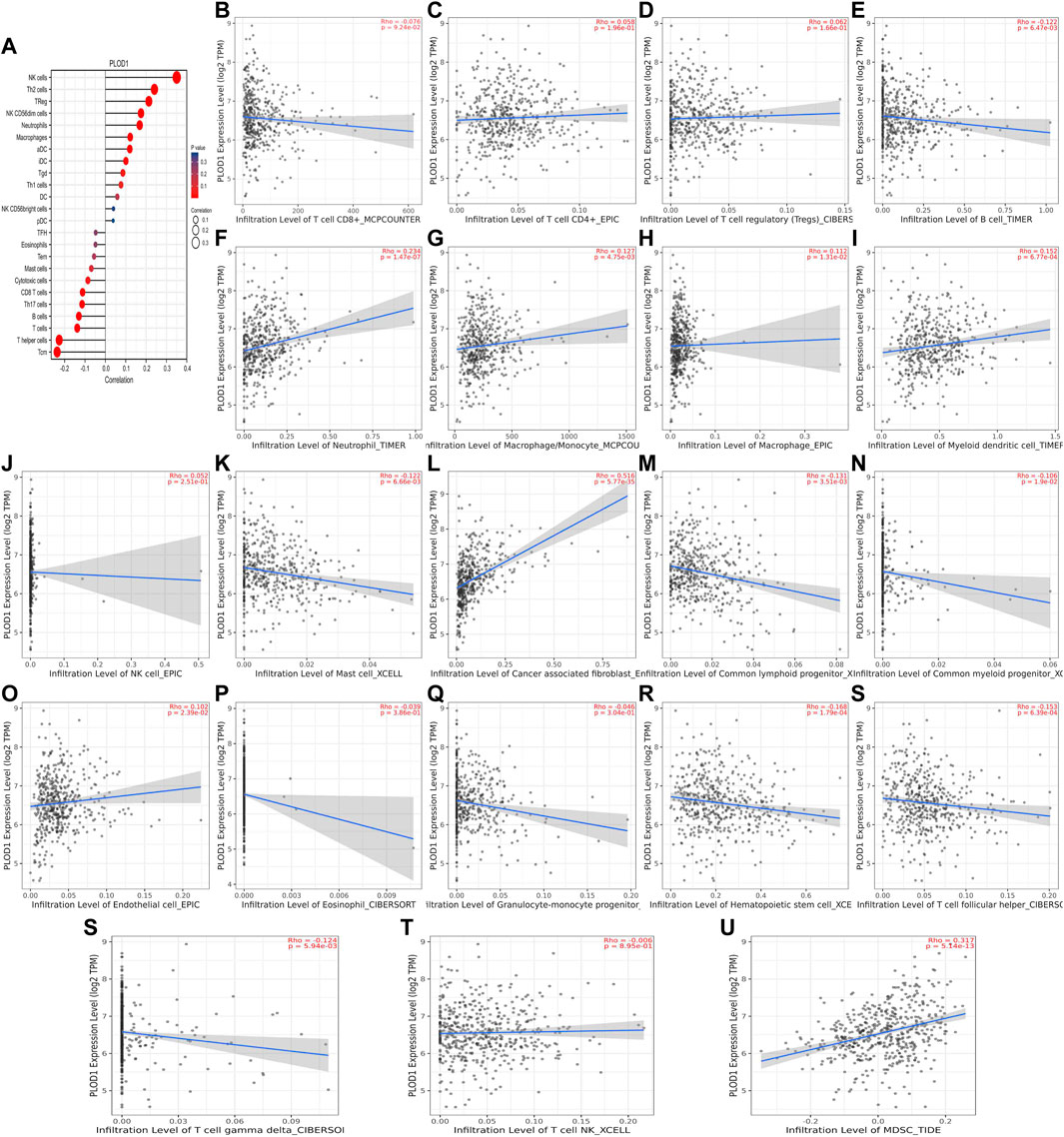
FIGURE 9. Association between PLOD1 and immune infiltration. (A) Summary plot of the relationship between PLOD1 expression and immune infiltration. (B–U) PLOD1 expression is negatively related to tumor purity and has correlations with TFH, CD8+ T, TH, cytotoxic, mast, and B cells; on the contrary, it is positively correlated with Tregs, macrophages, and cancer-associated fibroblasts.
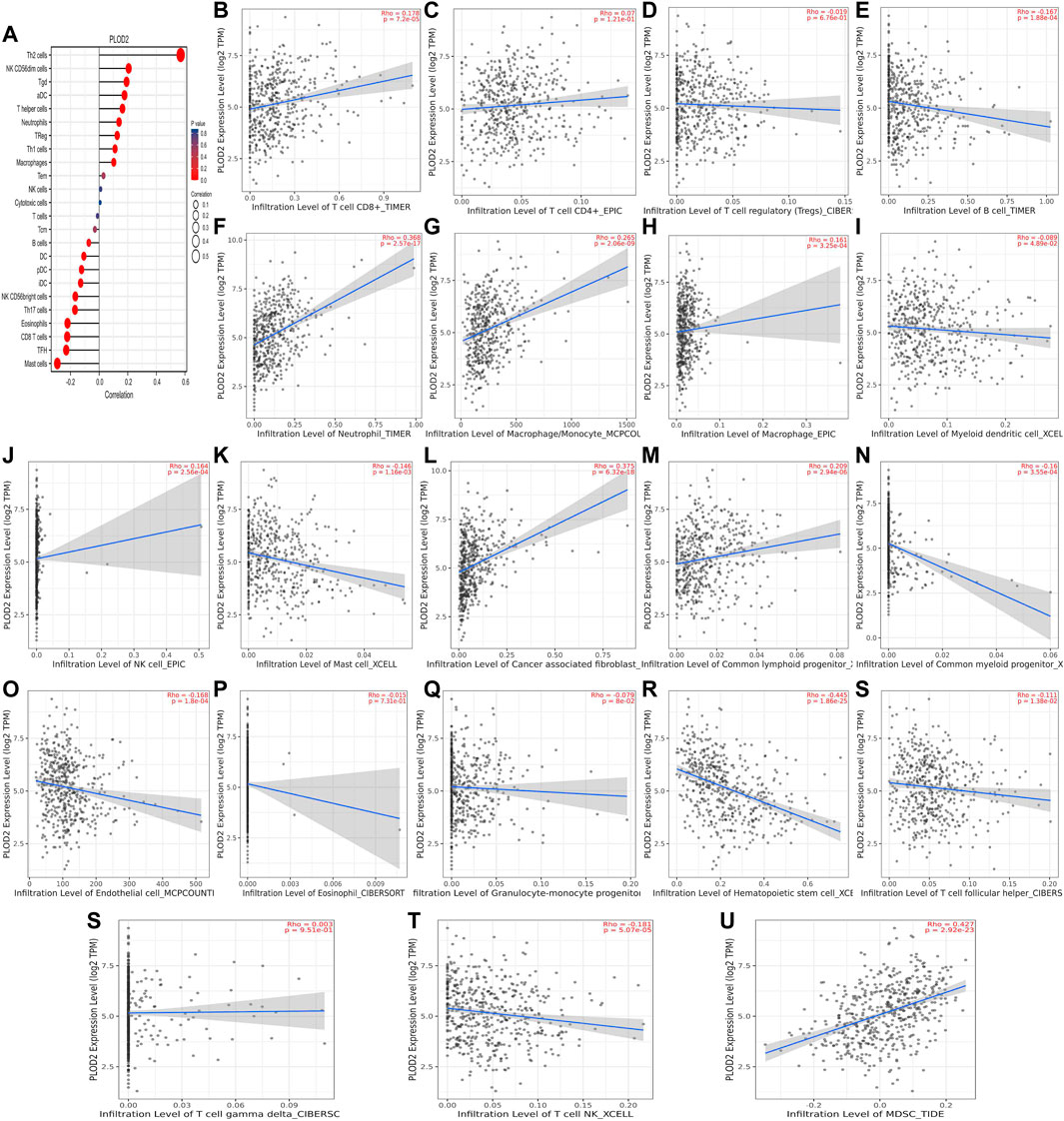
FIGURE 10. Association between PLOD2 and immune infiltration. (A) Summary plot of the relationship between PLOD2 expression and immune infiltration. (B–U) PLOD2 expression is negatively related to tumor purity and has correlations with TFH, CD8+ T, dendritic, CD56+ NK, mast, and B cells; on the contrary, it is positively correlated with Tregs, macrophages, and cancer-associated fibroblasts.
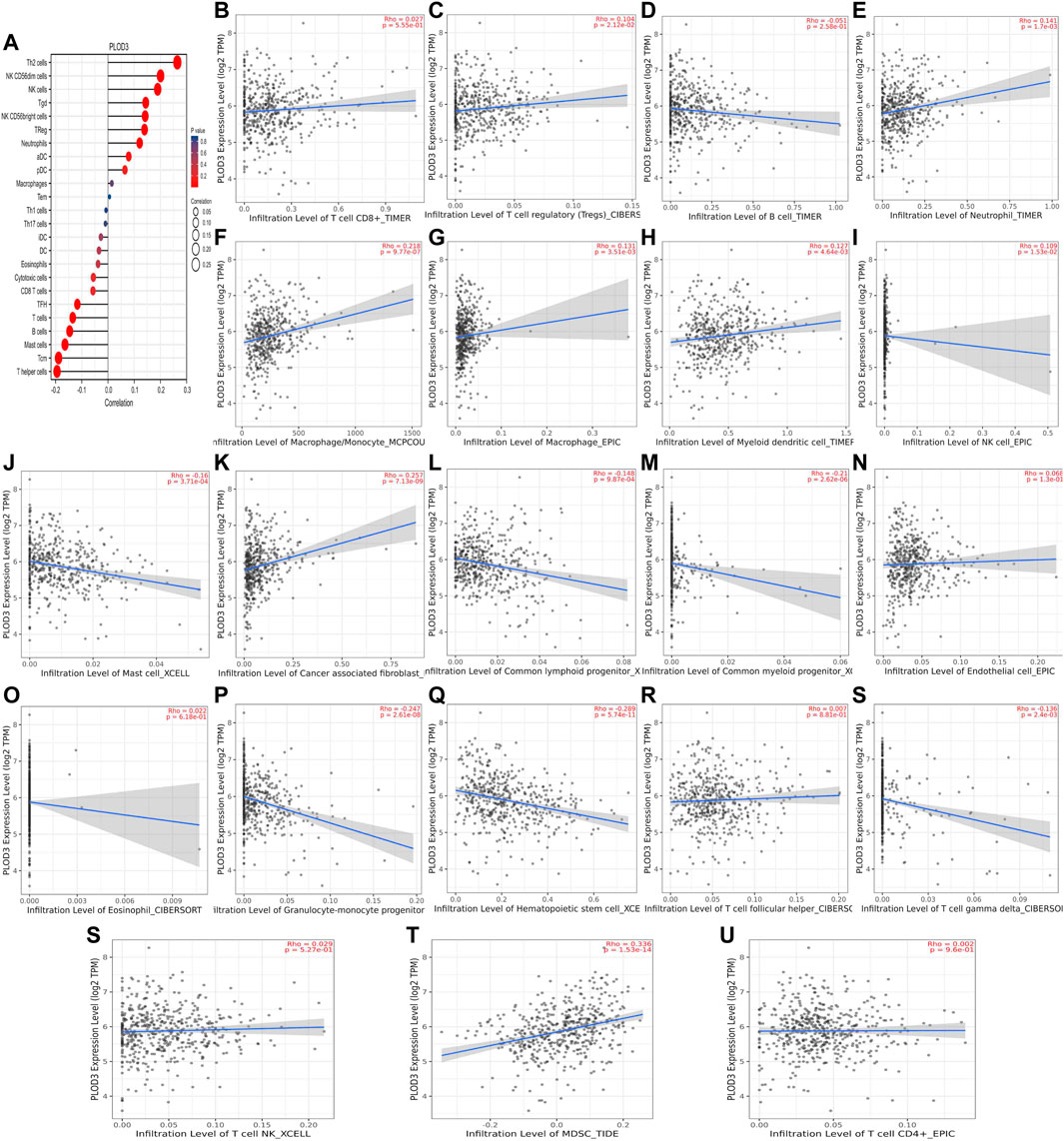
FIGURE 11. Association between PLOD3 and immune infiltration. (A) Summary plot of the relationship between PLOD2 expression and immune infiltration. (B–U) PLOD3 expression is negatively related to tumor purity and has correlations with TFH, CD8+ T, TH, dendritic, cytotoxic, and mast and B cells; on the contrary, it is positively correlated with Tregs, macrophages, and cancer-associated fibroblast cells.
Correlation of PLOD Expression With TILs
Using the TISIDB database, we found that the ratios of TIL subpopulations were highly correlated with PLOD expression in pan-cancer (Figure 12A, Figure 13A, and Figure 14A); representative pictures are shown in Figures 12B–L, Figures 13B–L, and Figures 14B–L. In general, the TISIDB results were similar to those obtained using TIMER.
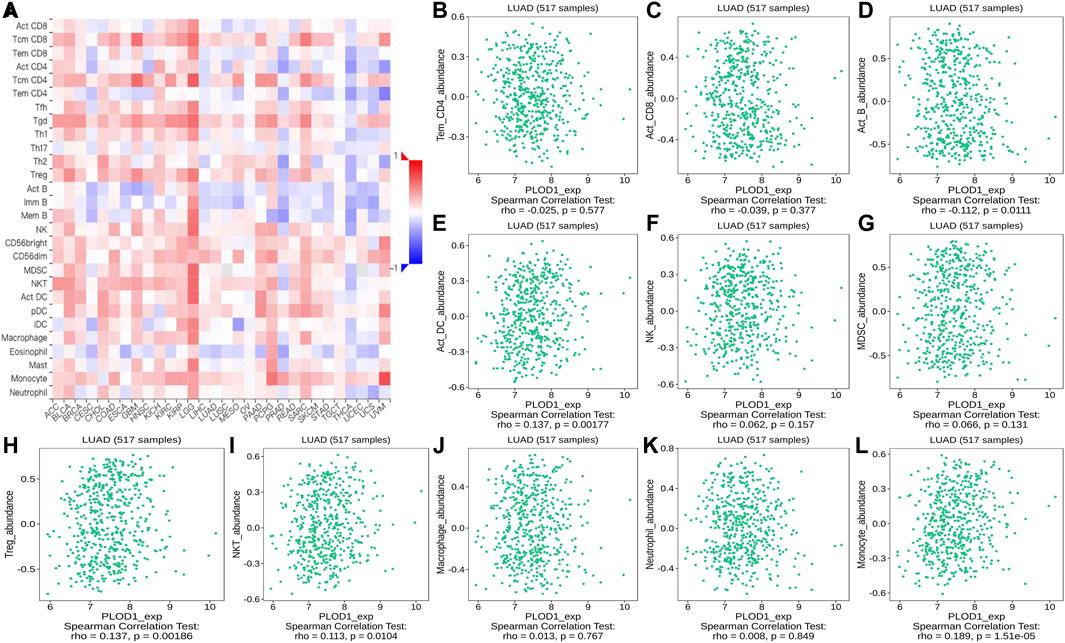
FIGURE 12. Correlation of PLOD1 expression with TILs. (A) Heatmap of PLOD1 expression in pan-cancer from the TISIDB database. (B–L) PLOD1 expression level is significantly correlated with infiltrating levels of activated CD8+ T cells, activated CD4+ T cells, activated B cells, Treg cells, NKT cells, and macrophages.
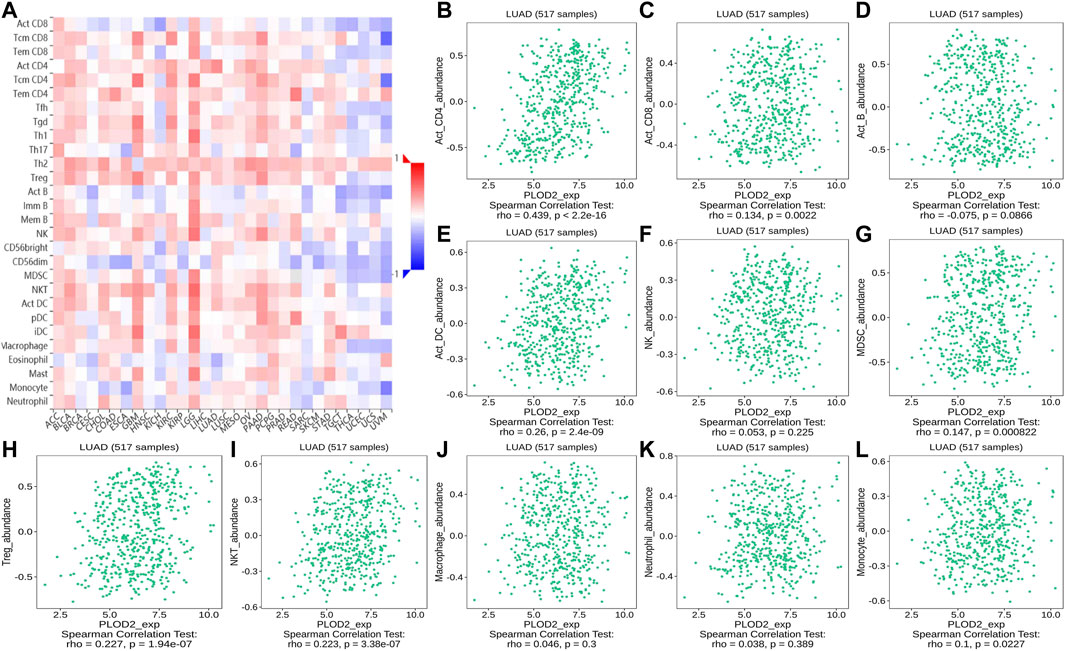
FIGURE 13. Correlation of PLOD2 expression with TILs. (A) Heatmap of PLOD2 expression in pan-cancer from the TISIDB database. (B–L) PLOD2 expression level is significantly correlated with infiltrating levels of activated CD8+ T cells, activated CD4+ T cells, activated DCs, activated CD8+ T cells, MDSCs, Treg cells, and NKT cells.
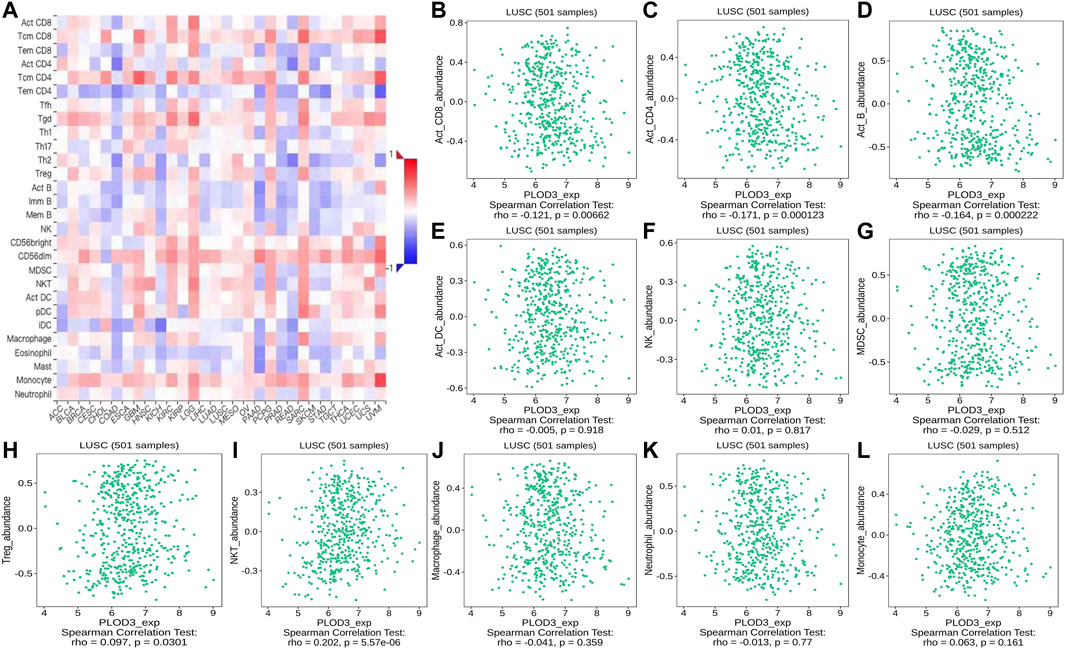
FIGURE 14. Correlation of PLOD3 expression with TILs. (A) Heatmap of PLOD3 expression in pan-cancer from the TISIDB database. (B–L) PLOD3 expression level is significantly correlated with infiltrating levels of activated CD8+ T cells, activated CD4+ T cells, activated B cells, Treg cells, and NKT cells.
Discussion
Lung adenocarcinoma has high mortality, ranking first and second in cancer-type incidence in men and women in China, respectively. It is also the leading cause of cancer-related deaths worldwide. Owing to the combined effects of tobacco, aging, and air pollution, the incidence of lung cancer is rapidly increasing. The most common subtype of lung cancer is LUAD. Since it is typically diagnosed late in its progression, the 5-year OS is only 15%. Therefore, it is important to identify differentially expressed molecules to use as markers for its diagnosis (Li et al., 2016; Tang et al., 2020).
In the present study, PLODs were found to be overexpressed in LUAD tissues; therefore, they may be independent prognostic biomarkers. The level of PLOD mRNA expression was positively correlated with tumor progression. ROC analysis demonstrated that PLODs may be a promising diagnostic indicator for distinguishing LUAD from normal tissues. From Kaplan–Meier curves, we confirmed that high expression of PLODs was associated with a shorter survival. Thus, PLODs represent attractive potential prognostic biomarkers for LUAD. Furthermore, PLODs may play specific roles in immune evasion.
Lysine hydroxylase (LH) is encoded by three lysine hydroxylase genes (procollagen-lysine, 2-oxoglutarate 5-dioxygenase PLOD1, PLOD2, and PLOD3) and is a key enzyme that mediates collagen association. PLOD family genes belong to the 2-ketoglutarate-dependent dioxygenase family (Zhao et al., 2021). They form homodimers in the presence of Fe2+ and 2-ketoglutaric acid (2-OG) to hydroxylate single-chain procollagen lysines. Collagen that undergoes lysine hydroxylation is secreted by the cell. It can be crosslinked to form stable hydroxylysine pyridine chains (Eisinger-Mathason et al., 2013). When PLOD1 and PLOD2 are significantly overexpressed, hydroxylysine pyridine chains are generated (Heikkinen et al., 1994). This leads to excessive deposition of collagen fibers, which destroys the ECM structure. Eventually, overexpression of PLODs promotes tumor progression and metastasis.
PLOD1 catalyzes hydroxylation of lysyl residues on collagen type V. It was originally used as a biochemical and histochemical marker for the assessment of bone mineral density (Shin et al., 2020). With the development of genome and transcriptome sequencing, PLOD1 mutations have been found to be associated with a variety of cancers (Xu et al., 2019). Wang et al. reported that PLOD1 is a potential therapeutic target for the treatment of mesenchymal gliomas (GBM). PLOD1 also enhances tumor vitality, activity, and migration, and promotes the transformation of GBM into malignant mesenchymal subtypes. They also found that PLOD1 expression is closely related to NF-κB signaling (Wang Z. et al., 2021). Furthermore, high expression level of PLOD1 was significantly correlated with the degree of response in hepatocellular carcinoma (HCC) patients and was positively correlated with immune infiltration. Further study of PLOD1 may provide new insights into diagnostic and prognostic biomarkers in HCC patients (Yang et al., 2020).
PLOD2 encodes lysine hydroxylase 2 (LH2). It is primarily located in the rough endoplasmic reticulum in multiple human tissues. PLOD2 specifically hydroxylates the lysine of the procollagen terminal peptide, which is important for the covalent crosslinking of collagen and tissue stiffness. Therefore, PLOD2 is a hydroxylase that mainly affects covalent crosslinking of collagen. After being secreted from the cell, the lysine residues of the PLOD2-modified collagen terminal peptide can be crosslinked to form a stable hydroxyl lysine pyridine chain (Noda et al., 2012; Cheriyamundath et al., 2021). In contrast, the crosslinks formed by collagen that has not undergone hydroxylation are less stable and prone to degradation. Therefore, the extent of lysine hydroxylation in the single-chain terminal peptide region of procollagen—that is, the expression and activity of LH2—determines the strength and arrangement of collagen fibers in the ECM (Gilkes et al., 2013; Du et al., 2017). In a variety of tumors, fibrous collagen is considered to provide a channel for cancer cell migration and is mainly modified by PLOD2. For example, PLOD2 can increase the invasiveness and migration of gastric cancer (GC) cells and promote their resistance to 5-fluorouracil by upregulating BCRP and inhibiting apoptosis (Wang et al., 2020).
PLOD3, also known as LH3, has a molecular weight of 85 kDa and catalyzes the hydroxylation of lysine residues in collagen. Studies have shown that PLOD3 has galactose hydroxylysine glucosyltransferase activity, which adds glucose groups to the galactose hydroxylysine residues of collagen (Shao et al., 2020). As previously reported, PLOD3 has a remarkable effect on the synthesis and modification of collagen. As modification of collagen and the extracellular matrix is related to tumor invasion and angiogenesis (Taler et al., 2020), PLOD3 may be involved in tumor development. Guo et al. found that PLOD3 is associated with ovarian cancer tumor progression and demonstrated that the interaction of COLGALT2 and PLOD3 can enhance the invasiveness of ovarian cancer (Guo et al., 2021b).
At present, PLODs, as novel oncogenes, have not been studied in LUAD in detail. Through data exploration, we discovered that PLODs were overexpressed in the tumor tissues of LUAD patients. The key findings were confirmed using the PrognoScan, GEPIA, HPA, and CPTAC databases. These results demonstrate that high PLOD expression may contribute to advanced disease, poor differentiation, or poor prognosis. Despite the fact that there was little statistical difference between groups, PLOD overexpression may be associated with tumor progression.
The more advanced T and N classifications were associated with higher PLOD expression, even though there was no statistically significant intergroup difference, possibly due to insufficient sample content. By analyzing GO, KEGG, and PPI network enrichment, we found that PLOD1 expression was positively associated with protein digestion and absorption, ECM–receptor interaction, and the AGE-RAGE signaling pathway in diabetic complications. The ECM–receptor interaction pathway mainly destroys the extracellular matrix, one of the necessary conditions for tumor invasion and metastasis. These data provide another explanation for the cancer-promoting mechanism of PLOD1. Functional annotations of PLOD2 and PLOD3 indicated that they are involved in protein digestion and absorption, amoebiasis, and AGE-RAGE signaling pathways in diabetic complications (Ruiz et al., 2021). Advanced glycation end products (AGEs) result from reducing sugars and substances, ingredients, or non-enzymatic reactions. AGEs interact with their receptor RAGE to activate multiple signal transduction pathways, as well as to disturb redox balance and regulate multiple cell death pathways (Waghela et al., 2021). Thus, PLOD genes play a special role in the progression of LUAD.
The interactions between the immune system and cancer are complex (Symmans, 2021). Several recent studies have revealed that systematic evaluation of immune infiltration is important for predicting clinical outcomes and developing immunotherapies (Nebot-Bral et al., 2017). Immune regulatory cells inhibit the antitumor response; they recognize and eliminate tumor cells that have undergone mutations, thereby inhibiting tumor growth. In addition, the immune system–mediated inflammatory response can promote tumor growth (Wang H. et al., 2021; Hoteit et al., 2021). The alteration of T cell and NK cell compartments found in stage I LUAD lesions suggests that the differential distribution of immune cells is important in the development of immunotherapy strategies for lung cancer (Lavin et al., 2017). The multiple roles of cancer-related T cells in lung cancer have been extensively studied. The characteristic genes of CD8+ depletion and pre-depletion T cells, as well as activated tumor Tregs, can be used as clinical biomarkers for LUAD patients (Guo et al., 2018). Studies have pointed out that the expression levels of PLOD1-3 in hepatocellular carcinoma are positively correlated with the activity of infiltrating immune cells, including macrophages, neutrophils, CD4+ T cells, and dendritic cells (Yang et al., 2020). We investigated the relationship between PLODs and infiltrating immune cells in LUAD. We found that PLOD1 was negatively correlated with the abundance of CD8+ T cells, cytotoxic cells, and Th cells. It was concluded that the expression of PLOD1 mainly reduces the infiltration of CD8+ T cells, cytotoxic cells, and Th cells. PLOD2 expression was negatively associated with the abundance of CD8+ T cells, B cells, and DCs. PLOD3 expression was negatively correlated with the abundance of CD8+ T cells, B cells, DCs, and cytotoxic cells. In contrast, our research has found that the expression of PLODs is positively correlated with the expression of immunosuppressive cells, such as Tregs and MDSCs. Based on our immunological analysis, we hypothesize that PLODs play an important role in immune escape in patients with LUAD.
In summary, we first proposed that PLOD expression is upregulated in LUAD. Our findings underscore the importance of PLODs in relation to disease stage, degree of differentiation, tumor size, and lymph-node metastasis. Therefore, PLODs can be considered an early diagnosis and independent prognostic biomarker for patients with LUAD. Compared with traditional experimental analysis, analyses using tumor databases have the advantages of larger sample sizes and higher reliability, which also provide a preliminary foundation for further research. Our data provide new potential indicators for the clinical prediction of patient survival and fundamental support for the progress of immunotherapy targets. This study provides a new strategy for individualized immunotherapy of LUAD, which will benefit more patients. In future studies, we will further verify the value of PLOD in LUAD.
Data Availability Statement
The datasets presented in this study can be found in online repositories. The names of the repository/repositories and accession number(s) can be found in the article/Supplementary Material.
Ethics Statement
Written informed consent was not obtained from the individual(s), nor the minor(s)’ legal guardian/next of kin, for the publication of any potentially identifiable images or data included in this article.
Author Contributions
HP, TY, and YM conducted research studies. JS and GZ performed data analysis using the tool. YM wrote the article. YM wrote the article. All authors helped with the review of the article.
Funding
This research work supported by “the Fundamental Research Funds for the Central Universities”, Natural Science Foundation (20180550488 and 2020-ZLLH-38 to YM) of Liaoning Province, Young and middle-aged technological innovation talents in Shenyang of YM (RC200491), and Excellent Talent Fund of Liaoning Province Cancer Hospital of YM.
Conflict of Interest
The authors declare that the research was conducted in the absence of any commercial or financial relationships that could be construed as a potential conflict of interest.
Publisher’s Note
All claims expressed in this article are solely those of the authors and do not necessarily represent those of their affiliated organizations, or those of the publisher, the editors, and the reviewers. Any product that may be evaluated in this article, or claim that may be made by its manufacturer, is not guaranteed or endorsed by the publisher.
Supplementary Material
The Supplementary Material for this article can be found online at: https://www.frontiersin.org/articles/10.3389/fmolb.2021.770729/full#supplementary-material
References
Chandrashekar, D. S., Bashel, B., Balasubramanya, S. A. H., Creighton, C. J., Ponce-Rodriguez, I., Chakravarthi, B. V. S. K., et al. (2017). UALCAN: A Portal for Facilitating Tumor Subgroup Gene Expression and Survival Analyses. Neoplasia 19 (8), 649–658. doi:10.1016/j.neo.2017.05.002
Chen, Z., Huang, Y., Hu, Z., Zhao, M., Li, M., Bi, G., et al. (2021). Landscape and Dynamics of Single Tumor and Immune Cells in Early and Advanced‐stage Lung Adenocarcinoma. Clin. Translational Med. 11 (3), e350. doi:10.1002/ctm2.350
Cheriyamundath, S., Kumar, A., Gavert, N., Brabletz, T., and Ben-Ze’ev, A. (2021). The Collagen-Modifying Enzyme PLOD2 Is Induced and Required during L1-Mediated Colon Cancer Progression. Ijms 22 (7), 3552. doi:10.3390/ijms22073552
Du, H., Pang, M., Hou, X., Yuan, S., and Sun, L. (2017). PLOD2 in Cancer Research. Biomed. Pharmacother. 90, 670–676. doi:10.1016/j.biopha.2017.04.023
Edwards, N. J., Oberti, M., Thangudu, R. R., Cai, S., McGarvey, P. B., Jacob, S., et al. (2015). The CPTAC Data Portal: A Resource for Cancer Proteomics Research. J. Proteome Res. 14 (6), 2707–2713. doi:10.1021/pr501254j
Eisinger-Mathason, T. S. K., Zhang, M., Qiu, Q., Skuli, N., Nakazawa, M. S., Karakasheva, T., et al. (2013). Hypoxia-dependent Modification of Collagen Networks Promotes Sarcoma Metastasis. Cancer Discov. 3 (10), 1190–1205. doi:10.1158/2159-8290.CD-13-0118
Gilkes, D. M., Bajpai, S., Wong, C. C., Chaturvedi, P., Hubbi, M. E., Wirtz, D., et al. (2013). Procollagen Lysyl Hydroxylase 2 Is Essential for Hypoxia-Induced Breast Cancer Metastasis. Mol. Cancer Res. 11 (5), 456–466. doi:10.1158/1541-7786.MCR-12-0629
Guo, T., Gu, C., Li, B., and Xu, C. (2021a). PLODs Are Overexpressed in Ovarian Cancer and Are Associated with gap Junctions via Connexin 43. Lab. Invest. 101 (5), 564–569. doi:10.1038/s41374-021-00533-5
Guo, T., Li, B., Kang, Y., Gu, C., Fang, F., Chen, X., et al. (2021b). COLGALT2 Is Overexpressed in Ovarian Cancer and Interacts with PLOD3. Clin. Translational Med. 11 (3), e370. doi:10.1002/ctm2.370
Guo, X., Zhang, Y., Zheng, L., Zheng, C., Song, J., Zhang, Q., et al. (2018). Global Characterization of T Cells in Non-small-cell Lung Cancer by Single-Cell Sequencing. Nat. Med. 24 (7), 978–985. doi:10.1038/s41591-018-0045-3
Heikkinen, J., Hautala, T., Kivirikko, K. I., and Myllylä, R. (1994). Structure and Expression of the Human Lysyl Hydroxylase Gene (PLOD): Introns 9 and 16 Contain Alu Sequences at the Sites of Recombination in Ehlers-Danlos Syndrome Type VI Patients. Genomics 24 (3), 464–471. doi:10.1006/geno.1994.1654
Hoteit, M., Oneissi, Z., Reda, R., Wakim, F., Zaidan, A., Farran, M., et al. (2021). Cancer Immunotherapy: A Comprehensive Appraisal of its Modes of Application (Review). Oncol. Lett. 22 (3), 655. doi:10.3892/ol.2021.12916
Lavin, Y., Kobayashi, S., Leader, A., Amir, E.-a. D., Elefant, N., Bigenwald, C., et al. (2017). Innate Immune Landscape in Early Lung Adenocarcinoma by Paired Single-Cell Analyses. Cell 169 (4), 750–765. e717. doi:10.1016/j.cell.2017.04.014
Li, S., Choi, Y.-L., Gong, Z., Liu, X., Lira, M., Kan, Z., et al. (2016). Comprehensive Characterization of Oncogenic Drivers in Asian Lung Adenocarcinoma. J. Thorac. Oncol. 11 (12), 2129–2140. doi:10.1016/j.jtho.2016.08.142
Li, T., Fan, J., Wang, B., Traugh, N., Chen, Q., Liu, J. S., et al. (2017). TIMER: A Web Server for Comprehensive Analysis of Tumor-Infiltrating Immune Cells. Cancer Res. 77 (21), e108–e110. doi:10.1158/0008-5472.CAN-17-0307
Li, Y., Gu, J., Xu, F., Zhu, Q., Chen, Y., Ge, D., et al. (2021). Molecular Characterization, Biological Function, Tumor Microenvironment Association and Clinical Significance of m6A Regulators in Lung Adenocarcinoma. Brief Bioinform 22 (4). doi:10.1093/bib/bbaa225
Mizuno, H., Kitada, K., Nakai, K., and Sarai, A. (2009). PrognoScan: a New Database for Meta-Analysis of the Prognostic Value of Genes. BMC Med. Genomics 2, 18. doi:10.1186/1755-8794-2-18
Nebot-Bral, L., Brandao, D., Verlingue, L., Rouleau, E., Caron, O., Despras, E., et al. (2017). Hypermutated Tumours in the Era of Immunotherapy: The Paradigm of Personalised Medicine. Eur. J. Cancer 84, 290–303. doi:10.1016/j.ejca.2017.07.026
Noda, T., Yamamoto, H., Takemasa, I., Yamada, D., Uemura, M., Wada, H., et al. (2012). PLOD2 Induced under Hypoxia Is a Novel Prognostic Factor for Hepatocellular Carcinoma after Curative Resection. Liver Int. 32 (1), 110–118. doi:10.1111/j.1478-3231.2011.02619.x
Robin, X., Turck, N., Hainard, A., Tiberti, N., Lisacek, F., Sanchez, J.-C., et al. (2011). pROC: an Open-Source Package for R and S+ to Analyze and Compare ROC Curves. BMC Bioinformatics 12, 77. doi:10.1186/1471-2105-12-77
Ru, B., Wong, C. N., Tong, Y., Zhong, J. Y., Zhong, S. S. W., Wu, W. C., et al. (2019). TISIDB: an Integrated Repository portal for Tumor-Immune System Interactions. Bioinformatics 35 (20), 4200–4202. doi:10.1093/bioinformatics/btz210
Ruiz, H. H., Nguyen, A., Wang, C., He, L., Li, H., Hallowell, P., et al. (2021). AGE/RAGE/DIAPH1 axis Is Associated with Immunometabolic Markers and Risk of Insulin Resistance in Subcutaneous but Not Omental Adipose Tissue in Human Obesity. Int. J. Obes. 45, 2083–2094. doi:10.1038/s41366-021-00878-3
Shao, S., Fang, H., Duan, L., Ye, X., Rao, S., Han, J., et al. (2020). Lysyl Hydroxylase 3 Increases Collagen Deposition and Promotes Pulmonary Fibrosis by Activating TGFβ1/Smad3 and Wnt/β-Catenin Pathways. aoms 16 (2), 436–445. doi:10.5114/aoms.2018.81357
Shergalis, A. G., Hu, S., Bankhead, A., and Neamati, N. (2020). Role of the ERO1-PDI Interaction in Oxidative Protein Folding and Disease. Pharmacol. Ther. 210, 107525. doi:10.1016/j.pharmthera.2020.107525
Shin, Y.-L., Park, Y. N., and Jang, M.-A. (2020). Rare Cases of PLOD1-Related Kyphoscoliotic Ehlers-Danlos Syndrome in a Korean Family Identified by Next Generation Sequencing. J. Korean Med. Sci. 35 (10), e96. doi:10.3346/jkms.2020.35.e96
Symmans, W. F. (2021). Interpreting the Complex Landscape of Immune-Tumor Interface. Clin. Cancer Res. 27, 5446–5448. doi:10.1158/1078-0432.CCR-21-2208
Szklarczyk, D., Franceschini, A., Kuhn, M., Simonovic, M., Roth, A., Minguez, P., et al. (2011). The STRING Database in 2011: Functional Interaction Networks of Proteins, Globally Integrated and Scored. Nucleic Acids Res. 39 (Database issue), D561–D568. doi:10.1093/nar/gkq973
Taler, K., Weiss, O., Rotem-Bamberger, S., Rubinstein, A. M., Seritrakul, P., Gross, J. M., et al. (2020). Lysyl Hydroxylase 3 Is Required for normal Lens Capsule Formation and Maintenance of Lens Epithelium Integrity and Fate. Developmental Biol. 458 (2), 177–188. doi:10.1016/j.ydbio.2019.10.020
Tang, Q., Li, W., Zheng, X., Ren, L., Liu, J., Li, S., et al. (2020). MELK Is an Oncogenic Kinase Essential for Metastasis, Mitotic Progression, and Programmed Death in Lung Carcinoma. Sig Transduct Target. Ther. 5 (1), 279. doi:10.1038/s41392-020-00288-3
Tang, Z., Li, C., Kang, B., Gao, G., Li, C., and Zhang, Z. (2017). GEPIA: a Web Server for Cancer and normal Gene Expression Profiling and Interactive Analyses. Nucleic Acids Res. 45 (W1), W98–W102. doi:10.1093/nar/gkx247
Tomczak, K., Czerwińska, P., and Wiznerowicz, M. (2015). Review the Cancer Genome Atlas (TCGA): an Immeasurable Source of Knowledge. wo 1A (1A), 68–77. doi:10.5114/wo.2014.47136
Uhlén, M., Fagerberg, L., Hallström, B. M., Lindskog, C., Oksvold, P., Mardinoglu, A., et al. (2015). Proteomics. Tissue-Based Map of the Human Proteome. Science 347 (6220), 1260419. doi:10.1126/science.1260419
Uhlen, M., Zhang, C., Lee, S., Sjöstedt, E., Fagerberg, L., Bidkhori, G., et al. (2017). A Pathology Atlas of the Human Cancer Transcriptome. Science 357 (6352), eaan2507. doi:10.1126/science.aan2507
Waghela, B. N., Vaidya, F. U., Ranjan, K., Chhipa, A. S., Tiwari, B. S., and Pathak, C. (2021). AGE-RAGE Synergy Influences Programmed Cell Death Signaling to Promote Cancer. Mol. Cel Biochem 476 (2), 585–598. doi:10.1007/s11010-020-03928-y
Wang, H., Liu, Y., Zhu, X., Chen, C., Fu, Z., Wang, M., et al. (2021a). Multistage Cooperative Nanodrug Combined with PD‐L1 for Enhancing Antitumor Chemoimmunotherapy. Adv. Healthc. Mater. 10, 2101199. doi:10.1002/adhm.202101199
Wang, X., Guo, J., Dai, M., Wang, T., Yang, T., Xiao, X., et al. (2020). PLOD2 Increases Resistance of Gastric Cancer Cells to 5-fluorouracil by Upregulating BCRP and Inhibiting Apoptosis. J. Cancer 11 (12), 3467–3475. doi:10.7150/jca.41828
Wang, Z., Shi, Y., Ying, C., Jiang, Y., and Hu, J. (2021b). Hypoxia-induced PLOD1 Overexpression Contributes to the Malignant Phenotype of Glioblastoma via NF-κB Signaling. Oncogene 40 (8), 1458–1475. doi:10.1038/s41388-020-01635-y
Xu, W.-H., Xu, Y., Wang, J., Tian, X., Wu, J., Wan, F.-N., et al. (2019). Procollagen-lysine, 2-oxoglutarate 5-dioxygenases 1, 2, and 3 Are Potential Prognostic Indicators in Patients with clear Cell Renal Cell Carcinoma. Aging 11 (16), 6503–6521. doi:10.18632/aging.102206
Yang, B., Zhao, Y., Wang, L., Zhao, Y., Wei, L., Chen, D., et al. (2020). Identification of PLOD Family Genes as Novel Prognostic Biomarkers for Hepatocellular Carcinoma. Front. Oncol. 10, 1695. doi:10.3389/fonc.2020.01695
Yu, G., Wang, L.-G., Han, Y., and He, Q.-Y. (2012). clusterProfiler: an R Package for Comparing Biological Themes Among Gene Clusters. OMICS: A J. Integr. Biol. 16 (5), 284–287. doi:10.1089/omi.2011.0118
Keywords: PLOD family member, LUAD, diagnosis, prognosis, immune infiltration
Citation: Meng Y, Sun J, Zhang G, Yu T and Piao H (2022) Clinical Prognostic Value of the PLOD Gene Family in Lung Adenocarcinoma. Front. Mol. Biosci. 8:770729. doi: 10.3389/fmolb.2021.770729
Received: 04 September 2021; Accepted: 30 December 2021;
Published: 21 February 2022.
Edited by:
Hossain Shekhar, University of Dhaka, BangladeshReviewed by:
Rajesh Sinha, University of Alabama at Birmingham, United StatesJie Wang, Nanjing Medical University, China
Copyright © 2022 Meng, Sun, Zhang, Yu and Piao. This is an open-access article distributed under the terms of the Creative Commons Attribution License (CC BY). The use, distribution or reproduction in other forums is permitted, provided the original author(s) and the copyright owner(s) are credited and that the original publication in this journal is cited, in accordance with accepted academic practice. No use, distribution or reproduction is permitted which does not comply with these terms.
*Correspondence: Tao Yu, dGFveXVsbnN6bHl5QHNpbmEuY29t; Haozhe Piao, aGFvemhlcGlhb2xuemx5eUBzaW5hLmNvbQ==