- Key Laboratory of Molecular Biology for Infectious Diseases (Ministry of Education), Department of Infectious Diseases, Institute for Viral Hepatitis, The Second Affiliated Hospital of Chongqing Medical University, Chongqing, China
Accumulating evidence suggests that tenofovir disoproxil fumarate (TDF) can attenuate liver fibrosis directly, the mechanism of which, however, has not been fully elucidated, and there is a paucity of data concerning whether TDF can also mitigate other chronic liver diseases (CLDs). We aimed to identify the molecular targets and potential mechanism of TDF itself in ameliorating CLDs. RNA-sequencing was performed on mouse liver tissues treated with TDF or normal saline. Then the differentially expressed genes (DEGs) were screened, and enrichment analyses of the function and signaling pathways of DEGs were performed with Database for Annotation, Visualization, and Integrated Discovery (DAVID) and Metascape. Next, protein-protein interaction (PPI) networks were constructed and module analyses were utilized to identify significant genes. Subsequently, the DisGeNET platform was used to identify the potential target genes of TDF in mitigating these diseases. Finally, prediction of the transcription factors (TFs) and microRNAs (miRNAs) of the target genes was done to conjecture the underlying mechanism by which TDF relieved CLDs. As a result, a total of 854 DEGs were identified, and the DEGs were involved mainly in “immunity,” “inflammation,” and “metabolism” processes. In addition, 50 significant genes were obtained via PPI construction and module analyses. Furthermore, by means of DisGeNET, 19 genes (Adra2a, Cxcl1, Itgam, Cxcl2, Ccr1, Ccl5, Cxcl5, Fabp5, Sell, Lilr4b, Ccr2, Tlr2, Lilrb4a, Tnf, Itgb2, Lgals3, Cxcr4, Sucnr1, and Mme) were identified to be associated with nine CLDs. Finally, 34 miRNAs (especially mmu-miR-155-5p) and 12 TFs (especially Nfkb1) were predicted to be upstream of the nine target genes (Cxcl1, Cxcl2, Ccl5, Ccr2, Sell, Tlr2, Tnf, Cxcr4, and Mme) of TDF in ameliorating CLDs. In conclusion, our study suggests that TDF have the potential to ameliorate CLDs independently of its antiviral activity by affecting the expression of genes involved in hepatic immune, inflammatory, and metabolic processes via mmu-miR-155-5p-NF-κB signaling. These findings provided prima facie evidence for using TDF in CHB patients with concurrent CLDs.
Introduction
Tenofovir disoproxil fumarate (TDF), an orally administered ester prodrug of tenofovir, is widely used for effective treatment of hepatitis B virus (HBV) infection (Perry and Simpson, 2009). The REVEAL-HBV study group reported an increased serum level of HBV DNA at baseline to be a strong and independent risk predictor of chronic liver diseases (CLDs) development (Chen, 2006; Iloeje et al., 2006). Numerous studies have shown that TDF can achieve sustained suppression of HBV in the long-term management of chronic hepatitis B (CHB) patients regardless of hepatitis B e antigen’s status and ethnicity (Heathcote et al., 2011; Gordon et al., 2013; Marcellin et al., 2019). Meanwhile, long-term studies have demonstrated sustained suppression of HBV replication with TDF to be associated with regression and a reduced risk of CLDs in CHB patients (Marcellin et al., 2013; Liu et al., 2019). Those effects were considered to be due mainly to reduced hepatic damage caused by HBV infection, but the direct non-antiviral effects of TDF might also be involved.
Two abstracts demonstrated that TDF could regress liver fibrosis directly by blocking proliferation (Signal Transduction and Cell Function, 2013) and inducing apoptosis of activated hepatic stellate cells (Abstracts, 2020). Recently, a basic study showed that TDF could attenuate liver fibrosis by upregulating expression of hepatitis C virus’s non-structural protein 5A transactivated protein 9 (NS5ATP9), thereby inhibiting TGFβ1/Smad3 and NF-κB/NLRP3 signaling pathways (Zhao et al., 2020). However, the mechanism by which TDF mitigates liver fibrosis has not been elucidated fully. Furthermore, there are no data suggesting whether TDF can also alleviate other CLDs independently of its antiviral activity.
We wished to explore the potential mechanism and molecular targets of TDF in improving CLDs. Hence, we undertook RNA-sequencing (RNA-seq) on the liver tissues of wild-type mice treated with TDF and employed an integrated bioinformatic analysis.
Materials and Methods
TDF
TDF was kindly gifted by Guangshengtang Co., Ltd (Fujian, China). The purity of TDF was 99.5%.
Animals and TDF Treatment
The study protocol was approved by the Animal Protection Organization and Ethics Committee of Chongqing Medical University (Chongqing, China). Female C57BL/6J mice (8 weeks) from the Animal Center of Chongqing Medical University were housed in a room with a 12-h light and dark cycle at 22°C with free access to mouse chow and water. After 1 week of acclimatization, mice were divided randomly into two groups. Mice in the TDF group were administered with TDF solution (455 mg of TDF powder + 0.5 g of sodium carboxymethyl cellulose +100 ml of normal saline were mixed thoroughly with a homogenizer until the solution was transparent) at 45.5 mg/kg/day by oral gavage for 4 months. Dose determination of TDF was performed via conversion of human equivalent doses to murine doses based on the body surface area (Reagan‐Shaw et al., 2008; Ng et al., 2015). Mice in the control group received an equivalent volume of vehicle (100 ml of normal saline + 0.5 g of sodium carboxymethyl cellulose were mixed thoroughly with a homogenizer until the solution was transparent) for 4 months. Each mouse was weighed once a week. At study termination, mice were killed after 12 h of fasting. Blood samples were taken by excising the eyeballs. The liver was collected and weighed for subsequent experiments. A flowchart of this study is shown in Figure 1.
Biochemical Parameters
Serum was collected after centrifugation at 1,000 rpm for 15 min. Serum ALT levels were measured in the Clinical Laboratory, The Second Affiliated Hospital, Chongqing Medical University.
Hematoxylin and Eosin Staining
Liver tissues of mice were fixed in 4% paraformaldehyde, embedded in paraffin, sliced, and stained with hematoxylin and eosin (H&E).
Isolation and Sequencing of RNA
According to manufacturer instructions, TRIzol® (Invitrogen, United States) was used to extract total RNA from the liver. Then a bioanalyzer (2,200 series; Agilent Technologies, United States) was used to evaluate the concentration, purity, and quality of RNA. Then RNA was sequenced using the DNBseq platform in BGI (Shenzhen, China).
Differential Gene Expression
Low-quality reads, adaptor reads, and reads with > 10% unknown bases (poly-N) were removed from raw data to obtain high-quality “clean” reads for subsequent analyses. The Q20 (percentage of bases with a quality value ≥ 20) and Q30 content of clean data were also calculated. Clean reads were mapped to the Mus musculus reference genome (GRCm38.p6) using HISAT2 (http://daehwankimlab.github.io/hisat2/) (Kim et al., 2015). Expression was calculated by RSEM (Li and Dewey, 2011) and represented in fragments per kilobase per million (FPKM) reads. Differential gene expression was identified using the “DEGseq” package with R (R Institute for Statistical Computing, Vienna, Austria) (Wang et al., 2010). The absolute value of fold change (FC) ≥ 2 and adjusted p-value (Q-value) ≤ 0.001 were adopted as criteria for determining the significance of differential expression of a particular gene.
Functional Enrichment Analyses of DEGs via DAVID and Metascape
The Gene Ontology (GO) database and Kyoto Encyclopedia of Genes and Genomes (KEGG) database were employed to identical enrichment of function and signaling pathways, respectively, based on Database for Annotation, Visualization, and Integrated Discovery (DAVID, https://david.ncifcrf.gov/) (Huang et al., 2009). Following the instructions of the DAVID manual, first, we clicked on the “Start Analysis” on the Internet website. Second, we entered the DEGs list, selected identifiers as “entrez gene ID,” selected list types as “gene lists,” and submitted lists. Third, we chose to limit annotations and background by M. musculus. Finally, the enrichment results of GO and KEGG databases were presented. p < 0.05 and gene count ≥ 2 were considered significant.
Furthermore, additional analyses of enrichment of function signaling pathways were done using Metascape (https://metascape.org/gp/index.html#/main/step1/) (Zhou et al., 2019). First, we pasted the gene list as “entrez gene ID.” Second, we chose to input the species as M. musculus. Third, we clicked on “Express Analysis.” Finally, analyses of enrichment of function and signaling pathways were carried out with the following ontology sources: Biological Process (BP) within the GO database, KEGG Pathway, Reactome Gene Sets, CORUM, TRRUST, PaGenBase, WikiPathways, and PANTHER Pathway. Terms with p < 0.01, minimum count of 3, and enrichment factor >1.5 (the enrichment factor is the ratio between the observed counts and the counts expected by chance) were collected and grouped into clusters based on their membership similarities.
Construction of Protein-Protein Interaction (PPI) Networks, Significant Modules, and a “Hub Gene” Network
First, Metascape was utilized to construct a PPI network and identify the significant modules. Besides, the Search Tool for the Retrieval of Interacting Genes (STRING, https://string-db.org/), a user-friendly online system that provides predicted and experimental interactions of proteins (Szklarczyk et al., 2019), was used to establish a PPI network of DEGs with a confidence score ≥ 0.7 for significant differences. Then the PPI network was visualized using Cytoscape 3.6.1 (www.cytoscape.org) (Shannon, 2003). Molecular Complex Detection (MCODE) 1.5.1 (a plugin of Cytoscape) (Bader and Hogue, 2003) was used to screen and identify the most significant modules in the PPI network. MCODEs were extracted when the node score cutoff was 0.2 and K-core was 2. cytoHubba (a plugin of Cytoscape) was employed to calculate the properties of the network topology for nodes to identify hub genes with a degree ≥ 10. The “degree” indicates the number of edges connected with a specific node. Nodes with a high degree are identified as hub genes (i.e., may contribute to vital biological behaviors).
Identification and Analyses of Significant Genes
A Venn diagram was delineated to identify significant union genes among “Metascape_MCODE,” “Cytoscape_MCODE,” and “Cytoscape_cytoHubba” by Bioinformatics (www.bioinformatics.com.cn/), an online platform for the analyses and visualization of data. Summaries for the basic information of the significant genes were obtained via Mouse Genome Informatics (www.informatics.jax.org/). A hierarchical clustering heatmap of significant genes was plotted by using OriginPro 2021 based on gene expression, and classified by the biological function of genes. Correlation analyses among significant genes were achieved through Pearson’s correlation test.
Identification of Potential Target Genes of TDF for Ameliorating CLDs
DisGeNET 7.0 (www.disgenet.org/) is a discovery platform containing one of the largest publicly available collections of genes and variants associated to human diseases (Piñero et al., 2019). The current version of DisGeNET contains 1,134,942 gene–disease associations (GDAs), between 21,671 genes and 30,170 diseases, disorders, traits, and clinical or abnormal human phenotypes. The relationships between the significant genes and nine common CLDs were analyzed via this tool. First, we entered the name of the diseases in the “Search” box. Subsequently, the summary of the GDA score and evidence for GDAs were presented, and the results were downloaded. Finally, the target genes associated with CLDs were identified from the results.
Prediction of Transcription Factors (TFs) and MicroRNAs (miRNAs) and Construction of TF-miRNA Co-regulatory Networks of Target Genes
To further explore how TDF improves CLDs, Transcriptional Regulatory Relationships Unraveled by Sentence-based Text mining (TRRUST 2, https://www.grnpedia.org/trrust/), a database containing 6552 TF–target interactions for 828 mouse TFs (Han et al., 2018), was employed to predict the TFs of target genes. First, we selected the species as “mouse,” and submitted the list of target genes in the bottom panel of the search area titled “Find key regulators for query genes.” Then the results were downloaded. Meanwhile, prediction of miRNAs of the target genes and TFs identified from TRRUST was done using DIANA-TarBase v8 (www.microrna.gr/tarbase/), a database that curates experimentally verified miRNA targets manually and contains 665,843 unique miRNA–target pairs (Karagkouni et al., 2018). We defined the species as M. musculus, entered the genes and TFs one-by-one, and tabulated the results. Ultimately, pairwise-related genes, TFs, and miRNAs were screened, and Cytoscape was utilized to visualize the TF–miRNA co-regulatory networks of the target genes.
Results
TDF had No Significant Effect on Liver Function of Normal Mice
TDF was administered to mice by oral gavage for 4 months to simulate the long-term use of TDF in humans. Before intervention, there was no significant difference in the body weight (BW) of mice (Supplementary Figure S1A) between the control group and TDF group. At study termination, no mice died from TDF administration. Besides, no significant differences were found between the TDF group (n = 9) and control group (n = 11) in BW (Supplementary Figure S1B), liver weight (LW) (Supplementary Figure S1C), liver index (LW/BW) (Supplementary Figure S1D), ALT level (Supplementary Figure S1E), and liver histology (Supplementary Figure S1F). Taken together, these results indicated that the TDF dose we employed was non-toxic, and had no significant effect on liver function of normal mice.
RNA-Seq and Read Mapping
To explore the transcriptional changes in the liver induced by TDF administration, RNA-seq was done using the liver tissues of the mice: 639.99 million raw reads (Supplementary Table S1) were generated. After removing adaptors and low-quality reads, we obtained 616.74 million clean reads, with a high quality of Q30 ≥ 93.36%. Then the trimmed clean reads were mapped onto the M. musculus reference genome, and 76.32–81.35% of clean reads were mapped uniquely to the genome (Supplementary Table S1B). The uniquely mapped reads were used in all subsequent analyses.
A Total of 854 Annotated Genes Were Identified to be DEGs Induced by TDF in Mouse Livers
A total of 17,199 mRNAs were annotated (Supplementary Table S2). DEGseq was employed to screen for DEGs. A total of 1,341 annotated genes were identified to be differentially expressed when considering exclusively a stringent threshold of Q-value ≤ 0.001 and an absolute value of FC ≥ 2, which is presented as a volcano plot (Figure 2A). After removing DEGs that caused differences between groups due to abnormal expression within the group, we obtained 854 DEGs eventually (217 downregulated DEGs and 637 upregulated DEGs) (Figure 2B and Supplementary Table S3). Hence, TDF could affect gene expression in mouse livers directly. To obtain a global view of these 854 DEGs, hierarchical clustering (Figure 2C) was done with normalized FPKM values, and indicated that our samples were of “good” quality with gene expression of similar proportion in each group.
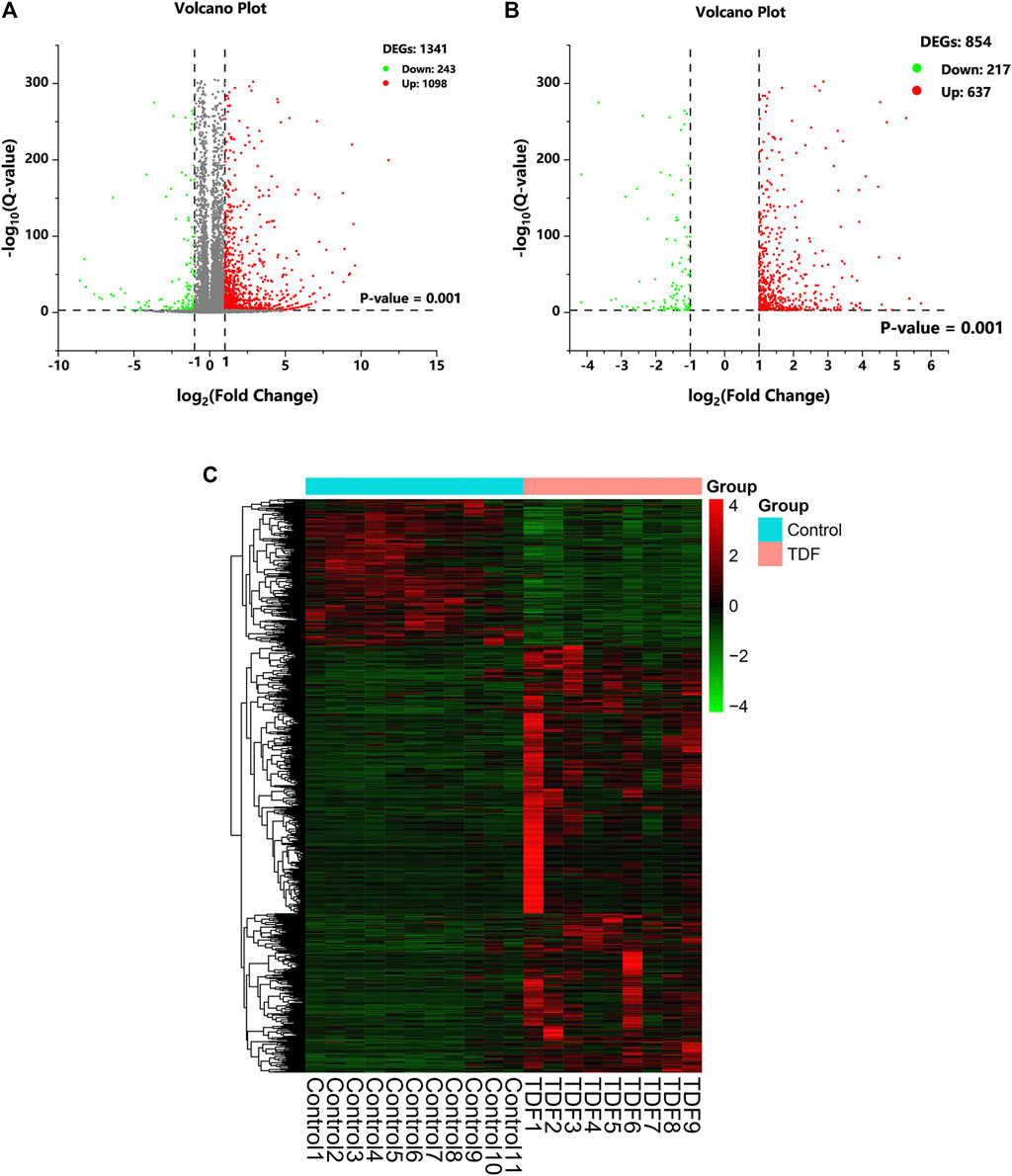
FIGURE 2. Differentially expressed genes (DEGs) in response to TDF. (A, B) The volcano plot of DEGs. The cutoff values fold change ≥ 2 and Q-value ≤ 0.001 were utilized to identify DEGs. Downregulated DEGs are marked in green, upregulated DEGs are marked in red, and unchanged genes are marked in gray. (C) Hierarchical clustering of DEGs.
Functional Enrichment Analyses Indicated That the 854 DEGs Induced by TDF Were Involved Mainly in “Immunity,” “Inflammation,” and “Metabolism” Processes
First, enrichment analyses using the GO database and KEGG database were undertaken using DAVID. We discovered that 774 out of 854 profiled DEGs were assigned to 394 GO terms: 264 for biological process (BP), 32 for cellular component (CC), and 98 for molecular function (MF). Variations in DEGs related to BP were involved mainly in “immune system process,” “inflammatory response,” “lipid metabolic process,” and “glucose metabolic process” (Figure 3A). With regard to CC, DEGs were significantly enriched in the “extracellular region,” “extracellular space,” “organelle membrane,” and “cell surface” (Figure 3B). Variations in DEGs associated with MF were significantly enriched in “small molecule binding,” “insulin-activated receptor activity,” “iron ion binding,” and “chemokine activity” (Figure 3C). The KEGG database indicated that 356 out of 854 profiled DEGs were assigned to 46 signaling pathways. The significant pathways relevant to DEGs were immune, inflammatory pathways (“cytokine-cytokine receptor interaction,” “NOD-like receptor signaling pathway,” “chemokine signaling pathway,” and “TNF signaling pathway”), and metabolic pathways (“retinol metabolism,” “steroid hormone biosynthesis,” “arachidonic acid metabolism,” and “glutathione metabolism”) (Figure 3D). The data of GO and KEGG classifications are shown in Supplementary Table S4.
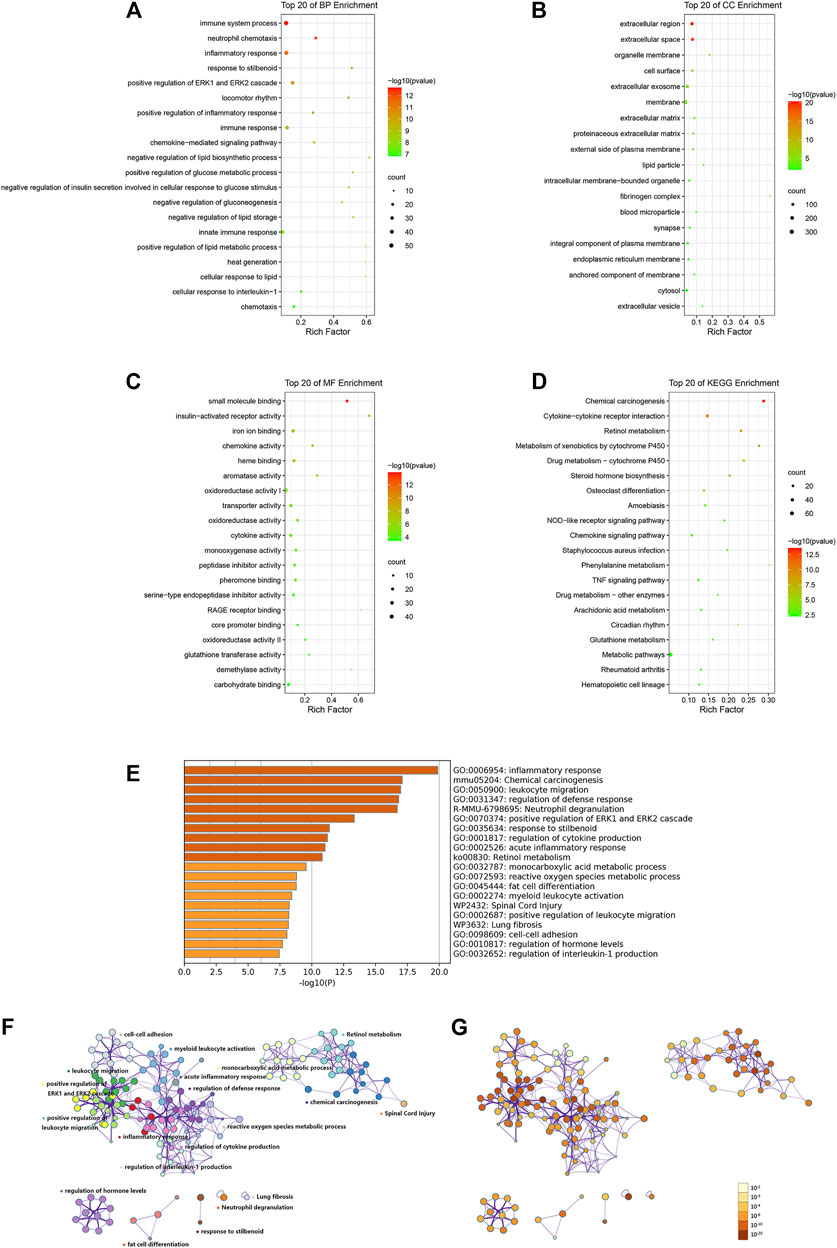
FIGURE 3. Functional enrichment analyses of DEGs via DAVID (A–D) and Metascape (E–G). Variations in DEGs associated with (A) biological process, (B) cellular component, (C) molecular function, and (D) KEGG analysis. Rich factor is the ratio of the DEG number to the total gene number in a certain pathway. The color and size of the dots represent the range of the p-value and the number of DEGs mapped to the indicated pathways, respectively. (E) Bar graph of enriched clusters across inputted DEGs lists, colored by p-values. Network of enriched terms: (F) colored by cluster ID, where nodes that share the same cluster ID are typically close to each other, (G) colored by p-value, where terms containing more genes tend to have a more significant p-value. The top 20 significant enriched pathways are shown.
To gain further insight into the functions of DEGs, analyses of enrichment of signaling pathways and function were carried out via Metascape. The results (in accordance with the results using DAVID) indicated that the DEGs induced by TDF were significantly enriched in inflammatory processes (“leukocyte migration,” “neutrophil degranulation,” “acute inflammatory response,” “positive regulation of leukocyte migration,” and “regulation of interleukin-1 production”) and metabolic processes (“retinol metabolism” and “monocarboxylic acid metabolism”) (p < 0.05, Figures 3E–G).
PPI Construction and Module Analyses Identified 50 Genes as Significant Genes, and These Genes Were Involved Mainly in “Immunity,” “Inflammation,” and “Metabolic” Processes
First, a PPI network of DEGs was constructed through Metascape (Figure 4A). Fourteen MCODE modules were identified from the PPI network. Notably, MCODE1 with the highest score consisted of 42 genes: Sirpb1c, Gm9733, Sirpb1b, Gm5150, Sirpb1a, Fcgr4, Ticam2, Sucnr1, Siglece, P2ry13, Cd177, Aldh3b1, Cxcl13, Lair1, Atp11a, Tlr2, Sstr2, Sell, Cxcl5, Cxcl2, Ccl6, Ccl5, Ccl4, Pld1, Pirb, Mtnr1a, Clec4d, Mme, Lgals3, Fabp5, Itgb2, Itgam, Cxcl1, Fpr1, Fpr2, Fcer1g, Ccr2, Ccr1, Cxcr4, C5ar1, Adra2a, and Adam8 (Figure 4B).
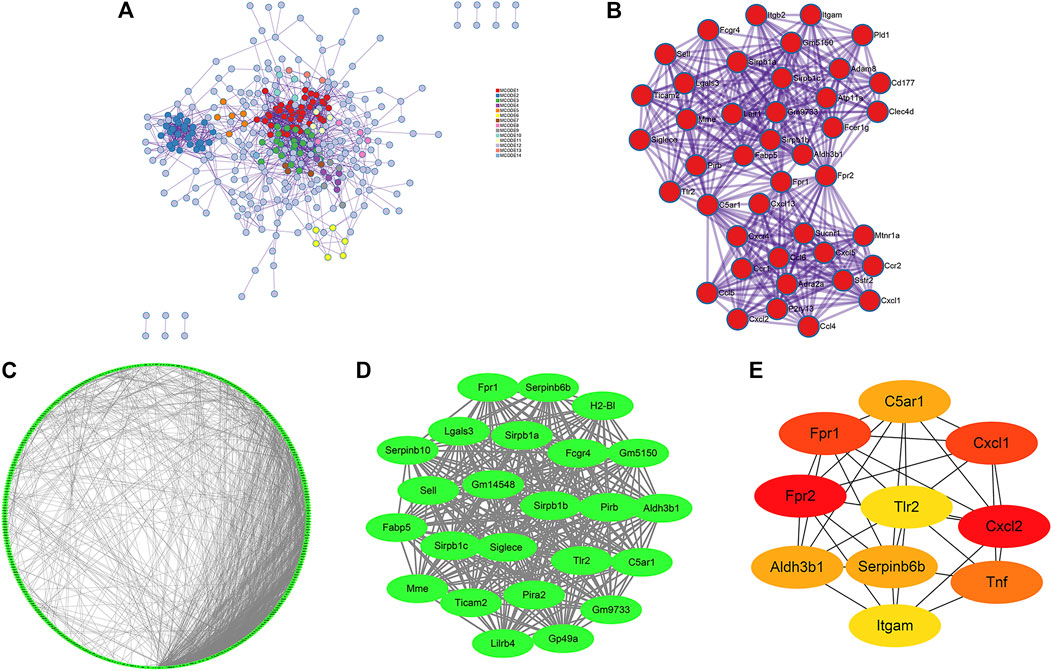
FIGURE 4. PPI network, significant modules and hub gene networks constructed through Metascape (A, B), STRING (C), and Cytoscape (D, E). (A) A PPI network of DEGs including 14 MCODE modules was established via Metascape. (B) The Metascape_MCODE1 consists of 42 genes. (C) A PPI network of DEGs consisting of 1937 edges and 443 nodes was established via STRING with a confidence score of ≥ 0.7 for significant differences. (D) The Cytoscape_MCODE1 consists of 24 genes. (E) Ten genes were identified as hub genes with Cytoscape_cytoHubba.
Simultaneously, construction of the PPI network was also established by STRING with a confidence score of ≥ 0.7 for significant differences. There were 1937 edges and 443 nodes in the PPI network (PPI enrichment p-value <0.001) (Figure 4C). Thirty MCODE modules were identified from the PPI network by the Cytoscape_MCODE. In particular, MCODE1 with the highest score comprised 24 genes: Serpinb6b, Sirpb1c, Pirb, Aldh3b1, Sell, Lilrb4, C5ar1, Fabp5, Pira2, Mme, Tlr2, Siglece, Lgals3, Sirpb1b, H2-Bl, Fcgr4, Sirpb1a, Gp49a, Ticam2, Gm5150, Gm9733, Fpr1, Gm14548, and Serpinb10 (Figure 4D). With degree ≥ 10 considered as the standard of judgment, 10 genes were identified as hub genes with Cytoscape_cytoHubba: Fpr2, Cxcl2, Fpr1, Cxcl1, Tnf, C5ar1, Serpinb6b, Aldh3b1, Tlr2, and Itgam (Figure 4E).
Finally, a VENN diagram was delineated and showed 50 significant union genes among “Metascape_MCODE1,” “Cytoscape_MCODE1,” and “Cytoscape_cytoHubba,” including four common genes: Aldh3b1, Tlr2, Fpr1, and C5ar1 (Figure 5A). The basic information of the 50 significant genes is summarized in Supplementary Table S5. Hierarchical clustering indicated that the significant genes could largely differentiate the TDF group from the control group (Figure 5B). Moreover, we found that these 50 genes were involved mainly in “immunity” (44%, 22/50), “inflammation” (34%, 17/50), and “metabolic” processes (10%, 5/50), and six genes (12%, 6/50) exhibited other or undefined functions (Figure 5B). The Pearson correlation analysis showed a positive correlation among most genes except for Sucnr1, H2-Bl, Mme, and Cxcl5 (Figure 5C).
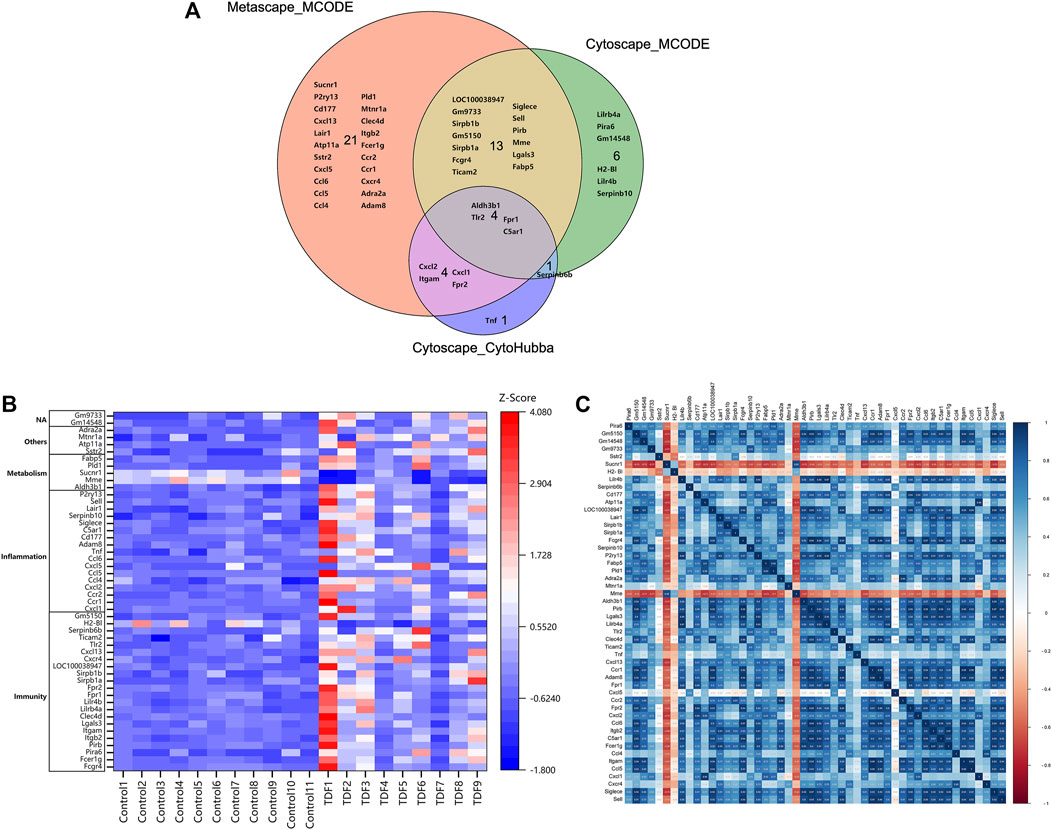
FIGURE 5. Identification of significant genes. (A) A VENN diagram of the 50 significant union genes among “Metascape_MCODE1,” “Cytoscape_MCODE1,” and “Cytoscape_cytoHubba.” (B) Hierarchical clustering heatmap and biological functional classification of the 50 significant genes. (C) Pearson correlation analysis of the 50 significant genes.
Nineteen Genes Were Identified to be the Potential Targets of TDF for Alleviating Nine CLDs Directly
The DisGeNET platform was employed to identify the potential targets of TDF for alleviating CLDs. The nine most common CLDs including non-alcoholic fatty liver disease (NAFLD), cholestasis, primary biliary cholangitis (PBC), primary sclerosing cholangitis (PSC), autoimmune hepatitis (AIH), non-alcoholic steatohepatitis (NASH), liver fibrosis (LF), cirrhosis, and hepatocellular carcinoma (HCC) were analyzed. The gene–disease association score (GDAs) between the potential target genes and these CLDs are portrayed in Figure 6A. Nineteen genes were associated with these nine CLDs. The number of genes involved in “inflammatory,” “immune,” and “metabolic” processes was eight (42%), seven (37%), and three (16%), respectively. From the vertical perspective, 10 genes were related to NAFLD: Tnf, Ccr2, Tlr2, Lgals3, Ccl5, Cxcl, Sell, Lilrb4a, Lilr4b, and Fabp5. The four genes relevant to cholestasis were Tnf, Ccr2, Cxcl2, and Mme. Five genes (Tnf, Tlr2, Lgals3, Ccl5, and Itgb2) were connected with PBC. In addition, Tnf and Ccr2 were associated with PSC. Tnf along with Tlr2 were related to AIH. Moreover, seven genes were involved in NASH: Tnf, Ccr2, Tlr2, Lgals3, Cxcl5, Cxcr4, and Adra2a. Eight genes (Tnf, Tlr2, Lgals3, Ccl5, Cxcl2, Cxcr4, Ccr1, and Sucnr1) were relevant to LF. Besides, there were nine genes associated with cirrhosis: Tnf, Ccr2, Tlr2, Lgals3, Ccl5, Cxcl5, Adra2a, Itgam, and Cxcl1. Tnf together with Ccr2 were related to HCC. In addition, according to the GDA score, the gene most associated with PBC was chemokine C-C motif ligand 5 (Ccl5), and the gene most closely related to the other eight liver diseases was Tnf. From the lateral perspective, we found that tumor necrosis factor (Tnf) was related to all of the nine CLDs, with cirrhosis (GDA score: 0.4) and cholestasis (GDA score: 0.34) showing the closest associations. Chemokine C-C motif receptor 2 (Ccr2) and toll-like receptor 2 (Tlr2) were associated with six CLDs, and galectin 3 (Lgals3) was related to five CLDs. The log2FC of genes indicated that 19 genes consisted of 17 upregulated DEGs and two downregulated DEGs, and the most significantly altered genes were adrenergic receptor alpha 2a (Adra2a), chemokine C-X-C motif ligand 1 (Cxcl1), and integrin alpha M (Itgam), with log2FC values of 2.84, 2.83, and 2.76, respectively (Figure 6B). The basic information of these genes can be found in Supplementary Table S5.
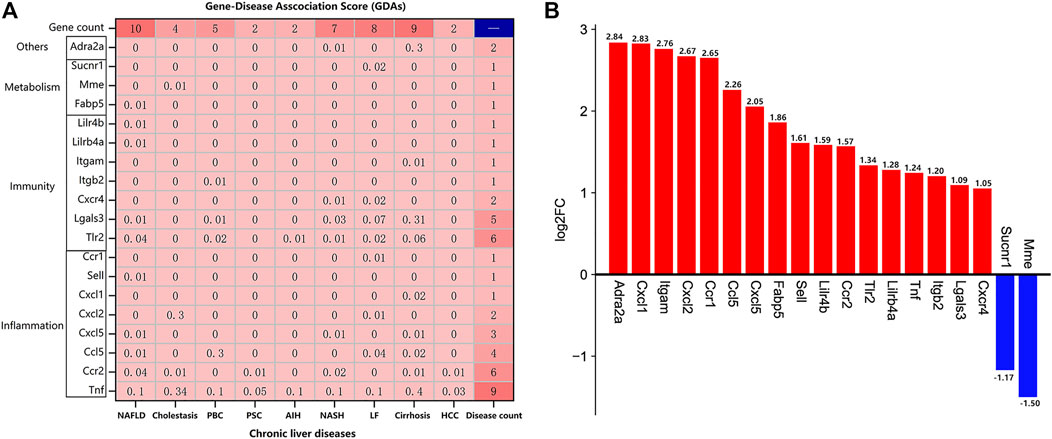
FIGURE 6. Identification of potential target genes of TDF for direct alleviation of chronic liver diseases (CLDs). (A) The gene–disease association (GDAs) score between the 19 potential target genes and nine CLDs. Gene count: the number of genes associated with a certain CLD; disease count: the number of CLDs associated with the corresponding gene. (B) The log2FC of the 19 potential target genes using RNA-seq data.
A Total of 34 microRNAs (miRNAs) and 12 Transcription Factors (TFs) Were Predicted to be Upstream of the Nine Potential Target Genes
First, TF–gene analyses were undertaken with TRRUST, and 13 upstream TFs targeting 12 target genes (seven genes had no results) were identified (Table 1). Quite specifically, NF-κB family (Nfkb1, Rela, and Rel) were the most significantly enriched TFs and targeted seven genes (Ccl5, Cxcl1, Cxcl2, Itgam, Tlr2, Tnf, and Cxcr4). Additionally, the RNA-seq data showed that TDF could also affect expression of Nfkb1 (log2FC = 0.22, Q-value <0.001), Rela (log2FC = 0.33, Q-value <0.001), and Rel (log2FC = 0.23, Q-value = 0.29). Overall, these results indicated that NF-κB might be the most critical TF for the genes targeted by TDF to relieve CLDs.
Subsequently, DIANA-TarBase v8 was used to predict the upstream miRNAs of the 19 target genes, and 80 unique miRNAs were identified (Supplementary Table S6A). The most pivotal miRNAs are shown in Table 2. These results suggested that mmu-miR-155-5p was the most important miRNA and regulated 15 target genes.
Meanwhile, TF–miRNA interactions were predicted for the 13 identified TFs by using DIANA-TarBase, and 156 unique miRNAs were identified (Table S6B). Table 3 shows the most pivotal miRNAs. The results indicated that mmu-miR-155-5p and mmu-miR-124-3p, interacting with eight TFs, might be the most important miRNAs.
Finally, nine genes, 12 TFs, and 34 miRNAs that were pairwise-related were screened out to construct the TF–miRNA co-regulatory networks of the target genes, which consisted of 247 edges and 55 nodes (Figure 7A). These results indicated that mmu-miR-155-5p (involved in 15 edges) might be the most important miRNA. The most important TF might be Nfkb1 (19 edges). Their target genes were Tnf, Ccl5, Tlr2, Cxcl1, and Cxcl2 (Figure 7B).
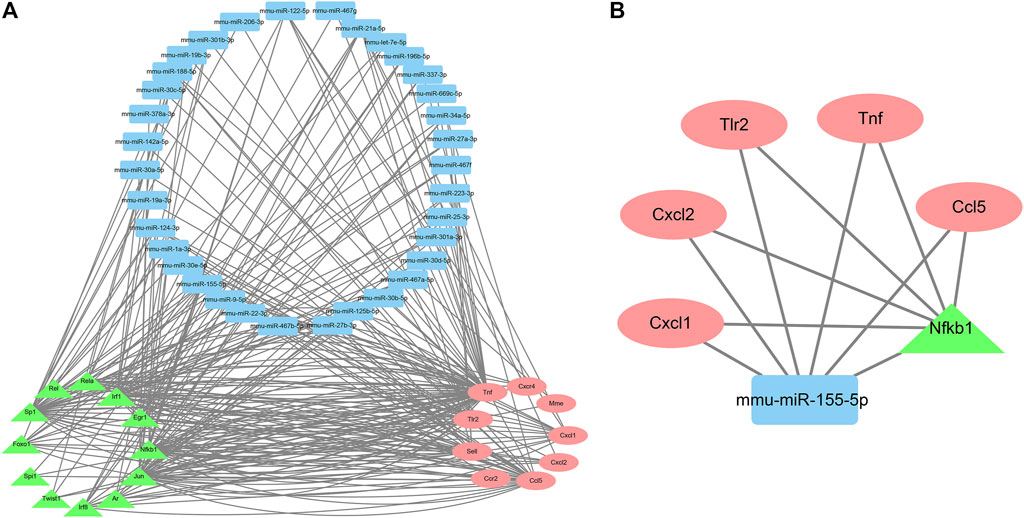
FIGURE 7. The TF-miRNA co-regulatory networks of the target genes. A. Co-regulatory networks consisting of nine genes, 12 TFs, and 34 miRNAs with pairwise relationships. B. Co-regulatory networks consisting of mmu-miR-155-5p and Nfkb1.
Taken together, these results suggested that co-regulatory networks consisting of mmu-miR-155-5p and Nfkb1 might be (at least in part) the underlying mechanisms by which TDF improved CLDs.
Discussion
TDF has been recommended as a first-line oral antiviral agents by the European Association for the Study of the Liver (Lampertico et al., 2017) and American Association for the Study of Liver Diseases (Terrault et al., 2018) for treatment of chronic hepatitis B (CHB) patients due to its high efficacy and genetic barrier. Evidence suggests that CHB patients predispose towards other hepatic comorbidities (Liu et al., 2018; Oh et al., 2020), in addition to viral factors, in which immune, inflammatory, and metabolic disorders also have pivotal roles (Seto et al., 2018). Meanwhile, these chronic liver diseases (CLDs) can in turn affect the disease progression (Yu et al., 2017; Choi et al., 2020; Guo et al., 2020; Bockmann et al., 2021) and the efficacy of antiviral strategies in CHB patients (Jin et al., 2012; Kim et al., 2019). Consequently, we hypothesized that TDF can inhibit the replication of HBV (a major cause of CLDs) and also attenuate CLDs directly by regulating the hepatic immune, inflammatory, and metabolic status of the host. If this is true, then TDF could be a promising antiviral drug for CHB patients with other hepatic comorbidities.
In the current study, RNA-seq of murine livers from the TDF group and control group was carried out and 854 DEGs were identified (Figure 2). Analyses of functional enrichment showed that the DEGs were involved mainly in “immunity,” “inflammation,” and “metabolism” processes (Figure 3). Subsequently, 50 genes were screened out as significant genes by PPI construction and module analyses, and participated mainly in “immunity” (44%, 22/50), “inflammation” (34%, 17/50), and “metabolic” processes (10%, 5/50) (Figures 4, 5). Finally, 19 out of the 50 significant genes were identified to be potential target genes of TDF in alleviating nine CLDs directly, and were enriched in a greater proportion of “immunity” (37%, 7/19), “inflammation” (42%, 8/19), and “metabolic” processes (16%, 3/19) (Figure 6). Compelling studies have described immunity, inflammation, and metabolism to be closely related in the pathogenesis and progression of CLDs (Marra and Tacke, 2014; Heymann and Tacke, 2016; Tang et al., 2020; Ahmed et al., 2021). In addition, treatment strategies that synergistically affect hepatic immune, inflammatory, and metabolic states can regress CLDs (Hegade et al., 2016; Chen et al., 2019). Given that TDF could affect hepatic immune, inflammatory, and metabolic processes directly, one can speculate that TDF had the potential to alleviate CLDs independently of its antiviral activity.
Furthermore, it is well known that transcription factors (TFs) and microRNA (miRNAs) can jointly regulate target gene expression and contribute to multiple biological processes and different diseases. Notably, the mmu-miR-155-5p-NF-κB signaling pathway may have critical roles in CLDs by regulating expression of the genes involved in immune, inflammatory, and metabolic processes (Wang et al., 2009; Bala et al., 2011; Yuan et al., 2016; Qian et al., 2019; Ali et al., 2021). In this study, we predicted the upstream TFs and miRNAs of the 19 target genes. Then we screened nine genes, 12 TFs, and 34 miRNAs with pairwise relationships to construct the TF–miRNA co-regulatory networks of the target genes (Figure 7). mmu-miR-155-5p and Nfkb1 were identified to be the most important upstream moieties of these target genes. Hence, TDF might be able to attenuate CLDs by affecting hepatic immune, inflammatory, and metabolic processes by mmu-miR-155-5p-NF-κB signaling.
Our study had three main limitations. First, the enrichment analyses (using GO and KEGG databases) used in our study are based on the theory of over-representation analysis (ORA). This method considers only the DEGs list regardless of the expression and change in trends of DEGs, which may cause bias to some extent. However, considering that our study was qualitative, ORA is sufficient. Of course, the gene set enrichment analysis (GSEA) would be recommended for further studies. Second, only the direct effects of TDF on the liver were studied; other nucleoside/nucleotide analogs were not investigated. Hence, whether the direct ameliorative potential of TDF upon CLDs was unique to TDF or shared by all nucleoside/nucleotide analogs is not known. Future studies on ETV are needed to confirm this question. Third, the conclusions of our study are mainly from observations in immunocompetent mice with normal liver function, so inevitably the results will be a little overstated. Despite its descriptive nature, this study provides preliminary evidence that TDF affect the expression of genes associated with CLDs. Of course, we must verify the target genes and miRNAs by building corresponding disease models through in vitro and in vivo experiments. If we want to further translate our results to humans, chimeric mice with humanized liver is recommended to help us clarify the mechanism by which TDF improves a specific liver disease.
In conclusion, we report, for the first time, the hepatic transcriptional changes induced by TDF in healthy mice. Our findings indicate that TDF could ameliorate CLDs independently of its antiviral activity by influencing expression of the genes involved in hepatic immune, inflammatory, and metabolic processes via mmu-miR-155-5p-NF-κB signaling.
Data Availability Statement
The datasets presented in this study can be found in online repositories. The names of the repository/repositories and accession number(s) can be found below: https://www.ncbi.nlm.nih.gov/ PRJNA763152.
Ethics Statement
The animal study was reviewed and approved by the Animal Protection Organization and Ethics Committee of Chongqing Medical University for humanistic care.
Author Contributions
YD designed the study, undertook the data processing, and drafted the original manuscript. ZC carried out the experiments. HL, WS, and YZ provided experimental guidance and technical support. MP modified the original draft of the manuscript. PH revised the manuscript and supervised the entire process. All authors contributed to this study and approved the submitted version of the manuscript.
Funding
This work was supported by grants from the National Science and Technology Major Project of China (2017ZX10202203008, 2017ZX10202203007).
Conflict of Interest
The authors declare that the research was conducted in the absence of any commercial or financial relationships that could be construed as a potential conflict of interest.
Publisher’s Note
All claims expressed in this article are solely those of the authors and do not necessarily represent those of their affiliated organizations, or those of the publisher, the editors, and the reviewers. Any product that may be evaluated in this article, or claim that may be made by its manufacturer, is not guaranteed or endorsed by the publisher.
Supplementary Material
The Supplementary Material for this article can be found online at: https://www.frontiersin.org/articles/10.3389/fmolb.2021.763150/full#supplementary-material
References
Ahmed, O., Robinson, M. W., and O’Farrelly, C. (2021). Inflammatory Processes in the Liver: Divergent Roles in Homeostasis and Pathology. Cell Mol Immunol 18, 1375–1386. doi:10.1038/s41423-021-00639-2
Ali, A. M., El-Tawil, O. S., Al-Mokaddem, A. K., and Abd El-Rahman, S. S. (2021). Promoted Inhibition of TLR4/miR-155/NFkB P65 Signaling by Cannabinoid Receptor 2 Agonist (AM1241), Aborts Inflammation and Progress of Hepatic Fibrosis Induced by Thioacetamide. Chemico-Biological Interactions 336, 109398. doi:10.1016/j.cbi.2021.109398
Bader, G. D., and Hogue, C. W. (2003). An Automated Method for Finding Molecular Complexes in Large Protein Interaction Networks. BMC Bioinformatics 4, 2. doi:10.1186/1471-2105-4-2
Bala, S., Marcos, M., Kodys, K., Csak, T., Catalano, D., Mandrekar, P., et al. (2011). Up-regulation of MicroRNA-155 in Macrophages Contributes to Increased Tumor Necrosis Factor α (TNFα) Production via Increased mRNA Half-Life in Alcoholic Liver Disease. J. Biol. Chem. 286, 1436–1444. doi:10.1074/jbc.M110.145870
Bockmann, J.-H., Kohsar, M., Murray, J. M., Hamed, V., Dandri, M., Lüth, S., et al. (2021). High Rates of Liver Cirrhosis and Hepatocellular Carcinoma in Chronic Hepatitis B Patients with Metabolic and Cardiovascular Comorbidities. Microorganisms 9, 968. doi:10.3390/microorganisms9050968
Chen, C.-J. (2006). Risk of Hepatocellular Carcinoma across a Biological Gradient of Serum Hepatitis B Virus DNA Level. JAMA 295, 65. doi:10.1001/jama.295.1.65
Chen, J., Hu, Y., Chen, L., Liu, W., Mu, Y., and Liu, P. (2019). The Effect and Mechanisms of Fuzheng Huayu Formula against Chronic Liver Diseases. Biomed. Pharmacother. 114, 108846. doi:10.1016/j.biopha.2019.108846
Choi, H. S. J., Brouwer, W. P., Zanjir, W. M. R., Man, R. A., Feld, J. J., Hansen, B. E., et al. (2020). Nonalcoholic Steatohepatitis Is Associated with Liver‐Related Outcomes and All‐Cause Mortality in Chronic Hepatitis B. Hepatology 71, 539–548. doi:10.1002/hep.30857
Gordon, S. C., Krastev, Z., Horban, A., Petersen, J., Sperl, J., Dinh, P., et al. (2013). Efficacy of Tenofovir Disoproxil Fumarate at 240 Weeks in Patients with Chronic Hepatitis B with High Baseline Viral Load. Hepatology 58, 505–513. doi:10.1002/hep.26277
Guo, H., Liao, M., Jin, J., Zeng, J., Li, S., Schroeder, D. R., et al. (2020). How Intrahepatic Cholestasis Affects Liver Stiffness in Patients with Chronic Hepatitis B: A Study of 1197 Patients with Liver Biopsy. Eur. Radiol. 30, 1096–1104. doi:10.1007/s00330-019-06451-x
Han, H., Cho, J.-W., Lee, S., Yun, A., Kim, H., Bae, D., et al. (2018). Trrust V2: An Expanded Reference Database of Human and Mouse Transcriptional Regulatory Interactions. Nucleic Acids Res. 46, D380–D386. doi:10.1093/nar/gkx1013
Heathcote, E. J., Marcellin, P., Buti, M., Gane, E., De Man, R. A., Krastev, Z., et al. (2011). Three-Year Efficacy and Safety of Tenofovir Disoproxil Fumarate Treatment for Chronic Hepatitis B. Gastroenterology 140, 132–143. doi:10.1053/j.gastro.2010.10.011
Hegade, V. S., Speight, R. A., Etherington, R. E., and Jones, D. E. J. (2016). Novel Bile Acid Therapeutics for the Treatment of Chronic Liver Diseases. Therap Adv. Gastroenterol. 9, 376–391. doi:10.1177/1756283X16630712
Heymann, F., and Tacke, F. (2016). Immunology in the Liver - from Homeostasis to Disease. Nat. Rev. Gastroenterol. Hepatol. 13, 88–110. doi:10.1038/nrgastro.2015.200
Huang, D. W., Sherman, B. T., and Lempicki, R. A. (2009). Systematic and Integrative Analysis of Large Gene Lists Using DAVID Bioinformatics Resources. Nat. Protoc. 4, 44–57. doi:10.1038/nprot.2008.211
Iloeje, U. H., Yang, H. I., Su, J., Jen, C. L., You, S. L., and Chen, C. J. (2006). Predicting Cirrhosis Risk Based on the Level of Circulating Hepatitis B Viral Load. Gastroenterology 130, 678–686. doi:10.1053/j.gastro.2005.11.016
Jin, X., Chen, Y.-P., Yang, Y.-D., Li, Y.-M., Zheng, L., and Xu, C.-Q. (2012). Association between Hepatic Steatosis and Entecavir Treatment Failure in Chinese Patients with Chronic Hepatitis B. PLoS ONE 7, e34198. doi:10.1371/journal.pone.0034198
Karagkouni, D., Paraskevopoulou, M. D., Chatzopoulos, S., Vlachos, I. S., Tastsoglou, S., Kanellos, I., et al. (2018). DIANA-TarBase V8: A Decade-Long Collection of Experimentally Supported miRNA-Gene Interactions. Nucleic Acids Res. 46, D239–D245. doi:10.1093/nar/gkx1141
Kim, D., Langmead, B., and Salzberg, S. L. (2015). HISAT: A Fast Spliced Aligner with Low Memory Requirements. Nat. Methods 12, 357–360. doi:10.1038/nmeth.3317
Kim, D. S., Jeon, M. Y., Lee, H. W., Kim, B. K., Park, J. Y., Kim, D. Y., et al. (2019). Influence of Hepatic Steatosis on the Outcomes of Patients with Chronic Hepatitis B Treated with Entecavir and Tenofovir. Clin. Mol. Hepatol. 25, 283–293. doi:10.3350/cmh.2018.0054
Lampertico, P., Agarwal, K., Berg, T., Buti, M., Janssen, H. L. A., Papatheodoridis, G., et al. (2017). EASL 2017 Clinical Practice Guidelines on the Management of Hepatitis B Virus Infection. J. Hepatol. 67, 370–398. doi:10.1016/j.jhep.2017.03.021
Li, B., and Dewey, C. N. (2011). RSEM: Accurate Transcript Quantification from RNA-Seq Data with or without a Reference Genome. BMC Bioinformatics 12, 323. doi:10.1186/1471-2105-12-323
Liu, A., Le, A., Zhang, J., Wong, C., Wong, C., Henry, L., et al. (2018). Increasing Co-Morbidities in Chronic Hepatitis B Patients: Experience in Primary Care and Referral Practices during 2000-2015. Clin. Translational Gastroenterol. 9, e141. doi:10.1038/s41424-018-0007-6
Liu, K., Choi, J., Le, A., Yip, T. C.-F., Wong, V. W.-S., Chan, S. L., et al. (2019). Tenofovir Disoproxil Fumarate Reduces Hepatocellular Carcinoma, Decompensation and Death in Chronic Hepatitis B Patients with Cirrhosis. Aliment. Pharmacol. Ther. 50, 1037–1048. doi:10.1111/apt.15499
Marcellin, P., Gane, E., Buti, M., Afdhal, N., Sievert, W., Jacobson, I. M., et al. (2013). Regression of Cirrhosis during Treatment with Tenofovir Disoproxil Fumarate for Chronic Hepatitis B: A 5-year Open-Label Follow-Up Study. The Lancet 381, 468–475. doi:10.1016/S0140-6736(12)61425-1
Marcellin, P., Wong, D. K., Sievert, W., Buggisch, P., Petersen, J., Flisiak, R., et al. (2019). Ten‐Year Efficacy and Safety of Tenofovir Disoproxil Fumarate Treatment for Chronic Hepatitis B Virus Infection. Liver Int. 39, 1868–1875. doi:10.1111/liv.14155
Marra, F., and Tacke, F. (2014). Roles for Chemokines in Liver Disease. Gastroenterology 147, 577–594. e1. doi:10.1053/j.gastro.2014.06.043
Ng, H. H., Stock, H., Rausch, L., Bunin, D., Wang, A., Brill, S., et al. (2015). Tenofovir Disoproxil Fumarate. Int. J. Toxicol. 34, 4–10. doi:10.1177/1091581814565669
Oh, H., Jun, D. W., Lee, I.-H., Ahn, H. J., Kim, B. O., Jung, S., et al. (2020). Increasing Comorbidities in a South Korea Insured Population-Based Cohort of Patients with Chronic Hepatitis B. Aliment. Pharmacol. Ther. 52, 371–381. doi:10.1111/apt.15867
Perry, C. M., and Simpson, D. (2009). Tenofovir Disoproxil Fumarate. Drugs 69, 2245–2256. doi:10.2165/10482940-000000000-00000
Piñero, J., Ramírez-Anguita, J. M., Saüch-Pitarch, J., Ronzano, F., Centeno, E., Sanz, F., et al. (2019). The DisGeNET Knowledge Platform for Disease Genomics: 2019 Update. Nucleic Acids Res. 48 (D1), D845–D855. doi:10.1093/nar/gkz1021
Qian, F., Hu, Q., Tian, Y., Wu, J., Li, D., Tao, M., et al. (2019). ING4 Suppresses Hepatocellular Carcinoma via a NF-κB/miR-155/FOXO3a Signaling Axis. Int. J. Biol. Sci. 15, 369–385. doi:10.7150/ijbs.28422
Reagan‐Shaw, S., Nihal, M., and Ahmad, N. (2008). Dose Translation from Animal to Human Studies Revisited. FASEB j. 22, 659–661. doi:10.1096/fj.07-9574LSF
Seto, W.-K., Lo, Y.-R., Pawlotsky, J.-M., and Yuen, M.-F. (2018). Chronic Hepatitis B Virus Infection. The Lancet 392, 2313–2324. doi:10.1016/S0140-6736(18)31865-8
Shannon, P. (2003). Cytoscape: A Software Environment for Integrated Models of Biomolecular Interaction Networks. Genome Res. 13, 2498–2504. doi:10.1101/gr.1239303
Signal Transduction and Cell Function (2013). Signal Transduction and Cell Function. Hepatology 58, 459A–471A. doi:10.1002/hep.26848
Szklarczyk, D., Gable, A. L., Lyon, D., Junge, A., Wyder, S., Huerta-Cepas, J., et al. (2019). STRING V11: Protein-Protein Association Networks with Increased Coverage, Supporting Functional Discovery in Genome-wide Experimental Datasets. Nucleic Acids Res. 47, D607–D613. doi:10.1093/nar/gky1131
Tang, J., Xiong, K., Zhang, T., and Han, H. (2020). Application of Metabolomics in Diagnosis and Treatment of Chronic Liver Diseases. Crit. Rev. Anal. Chem., 1–11. doi:10.1080/10408347.2020.1842172
Terrault, N. A., Lok, A. S. F., McMahon, B. J., Chang, K.-M., Hwang, J. P., Jonas, M. M., et al. (2018). Update on Prevention, Diagnosis, and Treatment of Chronic Hepatitis B: AASLD 2018 Hepatitis B Guidance. Hepatology 67, 1560–1599. doi:10.1002/hep.29800
Wang, B., Majumder, S., Nuovo, G., Kutay, H., Volinia, S., Patel, T., et al. (2009). Role of microRNA-155 at Early Stages of Hepatocarcinogenesis Induced by Choline-Deficient and Amino Acid-Defined Diet in C57BL/6 Mice. Hepatology 50, 1152–1161. doi:10.1002/hep.23100
Wang, L., Feng, Z., Wang, X., Wang, X., and Zhang, X. (2010). DEGseq: An R Package for Identifying Differentially Expressed Genes from RNA-Seq Data. Bioinformatics 26, 136–138. doi:10.1093/bioinformatics/btp612
Yu, M.-W., Lin, C.-L., Liu, C.-J., Yang, S.-H., Tseng, Y.-L., and Wu, C.-F. (2017). Influence of Metabolic Risk Factors on Risk of Hepatocellular Carcinoma and Liver-Related Death in Men with Chronic Hepatitis B: A Large Cohort Study. Gastroenterology 153, 1006–1017. e5. doi:10.1053/j.gastro.2017.07.001
Yuan, K., Zhang, X., Lv, L., Zhang, J., Liang, W., and Wang, P. (2016). Fine-Tuning the Expression of microRNA-155 Controls Acetaminophen-Induced Liver Inflammation. Int. Immunopharmacology 40, 339–346. doi:10.1016/j.intimp.2016.09.011
Zhao, J., Han, M., Zhou, L., Liang, P., Wang, Y., Feng, S., et al. (2020). TAF and TDF Attenuate Liver Fibrosis through NS5ATP9, TGFβ1/Smad3, and NF-Κb/nlrp3 Inflammasome Signaling Pathways. Hepatol. Int. 14, 145–160. doi:10.1007/s12072-019-09997-6
Keywords: tenofovir disoproxil fumarate, chronic liver diseases, non-antiviral effect, immunity, inflammation, metabolism, miR-155-5p, NF-κB
Citation: Duan Y, Chen Z, Li H, Shen W, Zeng Y, Peng M and Hu P (2021) Potential Molecular Targets of Tenofovir Disoproxil Fumarate for Alleviating Chronic Liver Diseases via a Non-Antiviral Effect in a Normal Mouse Model. Front. Mol. Biosci. 8:763150. doi: 10.3389/fmolb.2021.763150
Received: 23 August 2021; Accepted: 25 October 2021;
Published: 16 November 2021.
Edited by:
Hua Wang, Anhui Medical University, ChinaReviewed by:
Jieliang Chen, Shanghai Medical College of Fudan University, ChinaSara Reis, Universidade do Porto, Portugal
Yu-Chen Fan, Shandong University, China
Copyright © 2021 Duan, Chen, Li, Shen, Zeng, Peng and Hu. This is an open-access article distributed under the terms of the Creative Commons Attribution License (CC BY). The use, distribution or reproduction in other forums is permitted, provided the original author(s) and the copyright owner(s) are credited and that the original publication in this journal is cited, in accordance with accepted academic practice. No use, distribution or reproduction is permitted which does not comply with these terms.
*Correspondence: Peng Hu, hp_cq@163.com