- Laboratory of Cancer Genomics and Biology, Department of Urology, and Institute of Translational Medicine, Shanghai General Hospital, Shanghai Jiao Tong University School of Medicine, Shanghai, China
Tumor heterogeneity, a hallmark of cancer, impairs the efficacy of cancer therapy and drives tumor progression. Exploring inter- and intra-tumoral heterogeneity not only provides insights into tumor development and progression, but also guides the design of personalized therapies. Previously, high-throughput sequencing techniques have been used to investigate the heterogeneity of tumor ecosystems. However, they could not provide a high-resolution landscape of cellular components in tumor ecosystem. Recently, advance in single-cell technologies has provided an unprecedented resolution to uncover the intra-tumoral heterogeneity by profiling the transcriptomes, genomes, proteomes and epigenomes of the cellular components and also their spatial distribution, which greatly accelerated the process of basic and translational cancer research. Importantly, it has been demonstrated that some cancer cells are able to transit between different states in order to adapt to the changing tumor microenvironment, which led to increased cellular plasticity and tumor heterogeneity. Understanding the molecular mechanisms driving cancer cell plasticity is critical for developing precision therapies. In this review, we summarize the recent progress in dissecting the cancer cell plasticity and tumor heterogeneity by use of single-cell multi-omics techniques.
Introduction
Tumor heterogeneity, including genetic heterogeneity, epigenetic heterogeneity, phenotypic and functional heterogeneity, plays essential roles in tumor progression, especially in promoting resistance to treatment and driving metastasis (Giraudeau et al., 2019; Guo et al., 2019; Hinohara and Polyak, 2019). Investigating the origin of tumor heterogeneity has been the focus of research. For example, theories of cancer stem cell, clonal evolution and cellular plasticity have been proposed to explain the origin of heterogeneity (Michor and Polyak, 2010). Previously, high-throughput sequencing techniques have been employed to classify molecular subtypes, monitor the treatment response, identify new therapeutic targets and explore the tumor heterogeneity in the field of cancer research (Choi et al., 2017; Zhang et al., 2018; Zhao et al., 2020; Gay et al., 2021). However, these techniques have not been ideal tools to investigate the intra-tumoral heterogeneity since they usually detected the average signals of mixed cell populations rather than signals of individual cells within a tissue. For example, the bulk RNA sequencing cannot distinguish the proportions of distinct cellular components within a tumor (Roma-Rodrigues et al., 2019). Also, it is hard for the bulk sequencing techniques to determine the mutation status or transcriptome of distinct cell subpopulation in the tumor ecosystem. In contrast, single-cell techniques have shown great advantages in dissecting the cellular compositions and also their molecular features (Qian et al., 2020; Liu J. et al., 2021; Arora and Pal, 2021). Moreover, there are multiple hybrid or intermediate states of cells in the tumor ecosystem, such as hybrid epithelial/mesenchymal cells (Kroger et al., 2019; Thong et al., 2020). It is also hard for the bulk sequencing technologies to identify these hybrid cells. However, single-cell techniques have provided an opportunity to unmask hybrid states of individual cells (Williamson et al., 2016; Wouters et al., 2020; Gay et al., 2021). For example, multiple hybrid states of cancer cells have been discovered in human cancers by single-cell RNA sequencing (scRNA-seq), such as hybrid EMT cells and cancer/immune cells (Gay et al., 2021). In addition, single-cell techniques have great power to identify rare cell populations (Goveia et al., 2020; Kieffer et al., 2020; Pombo Antunes et al., 2021). Finally, single-cell techniques can distinguish tumor cells from non-tumor cell components, and also infer the interactions within or across these cellular components. Therefore, single-cell techniques provide more refined molecular features of tumor tissues compared with the bulk sequencing techniques.
Since the first application of single-cell transcriptome sequencing in 2009, single-cell techniques have evolved greatly and contributed tremendously in various fields of research (Tang et al., 2009; Navin et al., 2011; Hou et al., 2012; Liu J. et al., 2020; Zhu et al., 2020). Currently, single-cell techniques have been widely used in cancer research and shed light on the molecular underpins of tumor initiation and progression. For example, single-cell techniques have been used to analyze tumors at the levels of DNA (Gawad et al., 2016), RNA (Gonzalez-Silva et al., 2020), proteome (Wu and Singh, 2015), and epigenome (Schwartzman and Tanay, 2015). Recently, spatial transcriptomics (ST) techniques have enabled high-throughput sequencing of cellular components while preserving their spatial information within the tissue (Crosetto et al., 2015).
Cancer cell plasticity refers to some cancer cells transit dynamically between different cellular states, which results in increased tumor heterogeneity and promotes tumor progression (Gupta et al., 2011; Meacham and Morrison, 2013; Gunnarsson et al., 2020). However, molecular mechanisms that regulate cellular plasticity are still elusive. Recently, single-cell sequencing has been used to explore cancer cell plasticity (Su et al., 2017; Lourenco et al., 2020; Sacchetti et al., 2021). In this review, we summarize the recent progress in dissecting the cancer cell plasticity and tumor heterogeneity through single-cell multi-omics techniques, including the scRNA-seq, Single-Cell DNA Sequencing (scDNA-seq), single-cell proteomics and single-cell epigenomics (Figure 1).
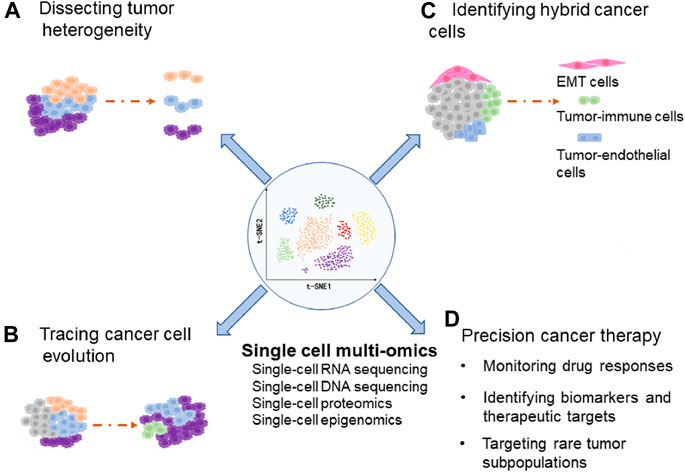
FIGURE 1. The diagram indicates applications of single-cell multi-omics in dissecting tumor heterogeneity (A), tracing cancer cell evolution (B), identifying hybrid cancer cells (C), and precision cancer therapy (D).
Dissection of Tumoral Heterogeneity
Single-Cell RNA Sequencing Analysis of Tumor Heterogeneity
ScRNA-seq has been widely used to explore the intra-tumoral heterogeneity (Peng et al., 2019; Robertson et al., 2020; Zhang et al., 2020; Zhou et al., 2020; Wang R. et al., 2021). For example, scRNA-seq revealed seven cancer cell subpopulations in pancreatic ductal adenocarcinoma (PDAC). However, only one subpopulation was shared in most PDAC patients, whereas the other six subpopulations existed in 1–2 patients (Peng et al., 2019). Moreover, tumor cells from different PDAC patients have been found to be hardly clustered together, indicating a high inter-tumor heterogeneity of PDAC (Lin et al., 2020). Consistently, similar findings have been identified in glioblastoma (Patel et al., 2014), melanoma (Tirosh et al., 2016), and breast cancer (Karaayvaz et al., 2018). Furthermore, scRNA-seq provided a refined resolution to dissect the tumor heterogeneity (Sottoriva et al., 2013; Gay et al., 2021; Riemondy et al., 2021). ScRNA-seq has also been used to identify rare cancer cell subpopulations, which previously hardly been identified by the bulk RNA-seq. For example, five cancer cell subpopulations were identified in primary gastric adenocarcinoma by scRNA-seq, three of which corresponded to histopathological features of Lauren’s subtypes whereas the other two were recognized as new subpopulations with different molecular characteristics (Zhang M. et al., 2021). In addition, scRNA-seq explored the functional heterogeneity of distinct cancer cell subpopulations. For example, scRNA-seq identified three different transcriptional states of lung adenocarcinoma (LUAD), tS1, tS2 and tS3 (Peng et al., 2019). The transcriptional state of tS1 and tS3 were similar to that of normal lung epithelial cells, suggesting that normal lung epithelial cells may be the source of LUAD. However, the tS2 showed a completely different transcription signature, characterized by the increased expression of genes associated with advanced tumors (Tirosh et al., 2016).
Single-Cell DNA Sequencing Analysis of Tumor Heterogeneity
ScDNA-seq has been used to identify single nucleotide variations (SNVs), copy number alterations (CNAs), and structure variants (SVs), as well as investigate the genetic heterogeneity of tumors. For instance, Duan et al. used scDNA-seq to study the CNA patterns of gastroesophageal junction cancer, and found that there are more than two subclones with different CNA patterns in both primary tumors and metastatic lymph nodes, suggesting that there is an extensive intra-tumor heterogeneity in both primary and metastatic tumors (Duan et al., 2021). Similar results have been identified in Hodgkin’s lymphoma (Mangano et al., 2019). Besides, scDNA-seq has been used to examine the heterogeneity of circulating tumor cells (CTCs) from liquid biopsies and monitor cancer genomes in a non-invasive manner. For example, scDNA-seq detected the same mutations of TP53, RB1, PIK3CA and ERBB2 genes in both biopsies and CTCs from patients with inflammatory breast cancer, suggesting that CTCs may reflect the genetic aberrations of primary tumors and act as an alternative resource of tumor heterogeneity (Bingham et al., 2017).
Single-Cell Proteomics Analysis of Tumor Heterogeneity
Recently, single-cell proteomics techniques have also been developed to investigate tumor heterogeneity and uncover the mechanisms of tumor progression (Wagner et al., 2019; Liu L. et al., 2020; Reza et al., 2021). For example, Wagner et al. analyzed 144 human breast tumors and 50 non-tumor tissues using cytometry by time-of-flight (CyTOF). Accordingly, they classified epithelial cells into seven luminal subgroups (L1–L7) and two basal subgroups (B1 and B2). Notably, the L3 luminal subgroup was observed to express high levels of EpCAM and CD49f but low level of ERα, which are characteristics of luminal progenitor cells. In contrast, the L4 luminal subgroup was shown to express high levels of the ERα, AR, HER2, EGFR and c-MET, which are involved in tumor cell proliferation and migration. Remarkably, they found that tumors recognized as ER+ by Immunohistochemical staining also contain a subset of ER− cell populations (Wagner et al., 2019). These findings could help to explain why nearly 30% of the ER+ breast cancers eventually develop endocrine resistance and progress to metastasis (Reinert and Barrios, 2015).
Furthermore, using RNAscope-based in situ hybridization protocol coupled with CyTOF, Schulz et al. have analyzed subcellular resolution mRNA and protein in breast cancer (Schulz et al., 2018). Similarly, the combination of CyTOF and immunohistochemical staining has enabled us to visualize the spatial distribution of distinct cellular compositions (Giesen et al., 2014). In addition to the CyTOF, multiple immunofluorescence imaging techniques have been used to detect multiple proteins in single cells (Lin et al., 2015; Pachynski et al., 2021). For example, cyclic immunofluorescence (CycIF) has been used to examine the formalin-fixed, paraffin-embedded (FFPE) specimens(Lin et al., 2018).
Single-Cell Epigenomics Analysis of Tumor Heterogeneity
Single-cell epigenomics techniques have also been developed to investigate epigenetic features of the cellular components within heterogenous tissues, such as single-cell DNA methylation sequencing and single-cell chromatin mapping (Schwartzman and Tanay, 2015), providing an opportunity to identify epigenetic regulation patterns and characterize epigenetic heterogeneity. For example, single-cell DNA methylation sequencing revealed that tumor-derived clonal organoids from different colorectal cancer patients show different epigenetic states, and one tumor encompasses multiple epigenetic states (Roerink et al., 2018). Furthermore, combinational single-cell RNA, DNA and methylation sequencing has been used to study the heterogeneity of hepatocellular carcinoma (Hou et al., 2016). In addition, single-cell ChIP-seq was employed to investigate the heterogeneity of chromatin states in breast cancer, which revealed that drug-resistant tumors show more heterogeneity than sensitive tumors. Notably, a small population of tumor cells with resistance signatures could also be detected in the sensitive tumor, indicating the pre-existence of drug resistant subpopulations (Grosselin et al., 2019).
Recently, single-cell sequencing assay for transposase-accessible chromatin (scATAC-seq) has also been employed to dissect the tumor heterogeneity (Wang et al., 2019; LaFave et al., 2020). For example, scATAC-seq identified three cancer cell subpopulations in glioblastoma, including pro-neural, mesenchymal and intermediate cell states (Wang et al., 2019). Moreover, dynamic evolution of cancer cells in a mouse model of lung adenocarcinoma has been investigated by scATAC-seq, which revealed an epigenetic continuum of cancer progression, characterized by loss of cellular identify and progression to a metastatic state (LaFave et al., 2020). Together, these findings indicate that single-cell epigenomics also have advantages to exploring tumor heterogeneity and identifying mechanisms underlying cancer cells evolution.
Dissecting Heterogeneity of Stromal Cells
Tumor microenvironment (TME) plays essential roles in cancer development and progression, which is composed of many types of cellular components and extracellular matrix (Hinshaw and Shevde, 2019). Importantly, single-cell sequencing has also been used to investigate the cellular heterogeneity of TME (Kieffer et al., 2020; Qian et al., 2020; Zhang et al., 2020). For example, a single-cell analysis of pan-cancer revealed a wide range of heterogeneity of stromal cells, including cancer-associated fibroblasts (CAFs), infiltrated immune cells, and endothelial cells (Qian et al., 2020). Moreover, a subset of FAP+ CAF could be further divided into eight subpopulations in Breast Cancers by scRNA-seq. Importantly, one CAF subpopulation characterized by high expression of genes encoding extracellular matrix proteins was revealed to drive immunotherapy resistance by increasing the protein levels of PD-L1 and CTLA4 in Treg cells through cell crosstalk (Kieffer et al., 2020). Similarly, scRNA-seq identified six CAF subpopulations in human intrahepatic cholangiocarcinoma, which could promote tumor progression by interacting with tumor cells (Zhang et al., 2020).
Furthermore, single-cell profiling of myeloid cells has been investigated in glioblastoma across species, which revealed that there are two distinct populations of tumor-associated macrophages, microglia- and monocyte-derived macrophages, which exist in the TME and compete for space (Pombo Antunes et al., 2021). Similarly, single-cell profiling of infiltrated T cells has been performed in multiple cancers (Zheng et al., 2017; Azizi et al., 2018; Li et al., 2019). For instance, scRNA-seq identified that a large number of CD8+ T cells exhibit continuous progression from an early effector state to dysfunctional T cell state in melanoma. Interestingly, this study also demonstrated that the dysfunctional CD8+ T cells are the major proliferating immune cells showing highly clonal and differentiating properties (Li et al., 2019). Moreover, scRNA-seq has been used to elucidate the heterogeneity of immune cells in treatment response to anti-PD1 in breast cancer, which revealed that PD1+ T cells undergo clonal expansion upon anti-PD1 treatment (Bassez et al., 2021). In addition, scRNA-seq has been employed to determine the heterogeneity of endothelial cells (ECs) in lung cancer, which identified 17 known and 16 unrecognized phenotypes of ECs (Goveia et al., 2020). Similarly, the subpopulations of ECs in tumors and their changes in gene expression following antiangiogenic treatment were analyzed by scRNA-seq (Zhao et al., 2018). Together, these studies demonstrated that single-cell technology greatly accelerates the understanding of stromal heterogeneity, providing new avenues to target these cellular components for precision cancer therapy.
Tracing Cancer Cell Evolution by Single-Cell Sequencing
Cancer cell evolution is a fundamental process during tumor progression (Black and McGranahan, 2021). Single-cell technologies have been used to trace the dynamic evolution of cancer cells (Wang et al., 2014; Davis et al., 2020; Ireland et al., 2020; Schlesinger et al., 2020; Liu R. et al., 2021; Su et al., 2021). For example, it has been considered that acinar metaplasia is the first step during pancreatic ductal adenocarcinoma tumorigenesis. However, using scRNA-seq and trajectory analysis, Schlesinger et al. found that acinar cells and early metaplastic cells show a continuous change to one of two fates, tumorigenic or stomach metaplastic, suggesting that metaplastic cells may not be involved in the evolution process from acinar cells, early metaplastic cells to tumor cells (Schlesinger et al., 2020). Moreover, small cell lung cancer (SCLC) has been classified into four molecular subtypes, including ASCL1+, NEUROD1+, POU2F3+ and YAP1+ SCLC (Rudin et al., 2019). However, the cellular origins of these SCLC subtypes are still elusive. Through time-series scRNA-seq analysis, Trudy Oliver and colleagues demonstrated that MYC can drive the dynamic evolution of SCLC subtypes, promoting a temporal shift from ASCL1+ to NEUROD1+ and YAP1+ SCLC states (Ireland et al., 2020).
In order to trace the clonal evolution of cancer cells from primary tumor to metastatic tumor, Davis et al. examined the heterogeneity of primary tumors and early metastases of triple-negative breast cancer by the scRNA-seq. They found that the heterogeneity of metastatic tumors is consistent with that of primary tumors, but the proportion of a subpopulation obviously increases in metastatic tumors, indicating an enrichment of this subpopulation during the process of metastasis (Davis et al., 2020). Moreover, clonal evolution of breast cancer has been investigated through the scDNA-seq, which found that chromosome rearrangements occur in the early stage of tumor evolution whereas point mutations evolve gradually over the long-term, generating extensive clonal diversity (Wang et al., 2014). Furthermore, scDNA-seq has been used to study genomic heterogeneity and clonal evolution of gastroesophageal junction cancer, which found that the similarity between lymph node metastasis and primary tumor is greater than that between different lymph node metastases, indicating that different lymph node metastases can originate from the same primary tumor but evolve independently (Duan et al., 2021). Similarly, Su et al. investigated the clonal evolution of liver cancer by scRNA-seq and scDNA-seq (Su et al., 2021). In addition, through examining genomic alterations of primary colorectal cancer tumor cells and CTCs from the same patient, Gao et al. revealed convergent evolution of copy number alterations from primary to circulating tumor cells (Gao et al., 2017).
Drug treatments have been shown to drive cancer evolution and increase the intra-tumor heterogeneity (Eyler et al., 2020; Vander Velde et al., 2020; Cohen et al., 2021). For example, longitudinal scRNA-seq has revealed that there are three main trajectories of tumor clonal evolution in patients with multiple myeloma, indicating that nearly half of the patients show clonal dynamics and transcriptional changes. Notably, one patient showed the transition from clone 1 with high expression of CSAG1 and MS4A1 genes at baseline treatment to clone 2 with downregulated expression of CSAG1 and MS4A1 after 4 cycles of treatment (Cohen et al., 2021). Furthermore, scRNA-seq revealed dynamic phenotypic changes in the evolution of drug resistance in ALK positive NSCLC, and even short-term Alectinib exposure can significantly affect cell phenotypes, suggesting a drug-induced direct cellular adaptation (Vander Velde et al., 2020). Additionally, scRNA-seq has been used to trace the emergence of drug resistance in glioblastoma cells after treatment of RTK inhibitors, which revealed the critical roles of interplay between genetic and epigenetic mechanisms in drug resistance (Eyler et al., 2020).
Hybrid Tumor Cell States Uncovered by Single-Cell Technologies
Hybrid Epithelial/Mesenchymal Cells
By use of single-cell sequencing, multiple hybrid states of tumor cells have been identified in various cancers, such as hybrid epithelial/mesenchymal cells, hybrid tumor/immune cells and hybrid tumor/endothelial cells. These hybrid states could confer tumor cells with different potentials to adapt to the changing microenvironments. EMT has been recognized as an important cellular program not only in normal embryonic development but also in many diseases, especially cancer initiation and progression (Brabletz et al., 2018). Recently, cancer cell subpopulations with EMT feature have been identified in multiple cancers by single-cell sequencing. For example, glioblatoma cells have been classified into four subtypes by scRNA-seq, including neural proenitor-like (NPC-like), oligodendrocyte-progenitor-like (OPC-like), astrocyte-like (AC-like) and mesenchymal like (MES-like) cells. Importantly, a dynamic transition from OPC-like or NPC-like cells to MES-like cells was revealed, indicating a high plasticity of glioblastoma cells (Neftel et al., 2019). Moreover, using scRNA-seq and ST analysis, Ji et al. dissected the cellular composition and architecture of cutaneous squamous cell carcinoma. They found that a tumor-specific keratinocyte (TSK) subpopulation, expressing classic EMT markers, localizes to a fibrovascular niche and functions as a hub for intercellular communication (Ji et al., 2020).
In addition, Wouters et al. reported an intermediate state of melanocyte and mesenchymal cell, which was regulated by a set of transcription factors, including SOX6, NFATC2, EGR3, ELF1, and ETV4. They also demonstrated that knockdown of the SOX10 gene is sufficient to switch melanocytic and intermediate cell state to mesenchymal-like cell state (Wouters et al., 2020). Notably, the cell origins of CAFs are still elusive. One of the cell origins has been proposed is that CAFs can be derived from tumor cells undergone a EMT program, which can be distinguished by analyzing the genomic alterations (Sahai et al., 2020). In summary, by use of single-cell technologies, these studies indicated that a subset of tumor cells with EMT feature has been widely existed in heterogenous populations of multiple Cancers.
Hybrid Tumor/Immune Cells
Immune Checkpoint Blockades (ICB) have been used in clinic to treat cancer patients. However, only a few patients respond to these ICBs. Unfortunately, the underlying mechanisms regarding immune evasion of tumor cells are largely unknown. Recently, a subpopulation of tumor cells expressing immune cell markers has been identified in several cancers by scRNA-seq. For example, Jin et al. identified a tumor cell population characterized by expression of epithelial-immune dual markers, such as classical epithelial marker EPCAM and immune markers, MHC-II and complement genes. The dual feature of tumor cells was observed to be positively correlated with the expression of co-inhibitory receptors on CD8+ T cells. Importantly, tumor cells with this dual feature exhibited a higher capacity for tumorigenesis and associated with poor prognosis of patients with nasopharyngeal carcinoma (Jin et al., 2020). Moreover, Miao et al. found that a subset of tumor-initiating stem cells in squamous cell carcinoma selectively express CD80, a previously identified immune cell surface ligand. They further demonstrated that CD80 is necessary for the tumor-initiating stem cells to endure immune attack and CD80 could dampen the activity of cytotoxic T cells through directly engaging with CTLA4 (Miao et al., 2019). Consistently, Wang et al. identified that cancer stem cells can upregulate another immune checkpoint molecule CD276 (B7–H3) in order to evade host immune attack. They found that CD274 is highly expressed by cancer stem cells of mouse and human head and neck squamous cell carcinoma, and anti-CD276 could eliminate these stem cells (Wang C. et al., 2021). Additionally, Chen et al. found that luminal prostate cancer cells express T-cell co-stimulatory genes, suggesting a potential role of tumor cells involved in antigen presentation (Chen et al., 2021). Taken together, these findings indicated that tumor cells expressing immune cell markers is one of the mechanisms by which tumor cells evade immunosurveillance, providing a new avenue for the development of immune checkpoint inhibitors and combined targeted therapy.
Hybrid Tumor/Endothelial Cells
Angiogenesis is one of the cancer hallmarks. It has been reported that tumor cells could transdifferentiate into endothelial cells and form vascular mimicry in order to feed rapidly growing tumors (Maniotis et al., 1999; Kirschmann et al., 2012). Recently, single-cell sequencing has been used to understand the tumor angiogenesis. For example, Caroline Dive and colleagues found that a rare subpopulation of CTCs from SCLC patients co-expresses vascular endothelial-cadherin (VE-cadherin) and cytokeratin, which is consistent with the process of vasculogenic mimicry, a process during which tumor cells form endothelial-like vessels. They also found that knockdown of the VE-cadherin could increase sensitivity of SCLC cells to chemotherapy (Williamson et al., 2016). Consistently, a rare subpopulation of tumor-derived endothelial cells was observed to contribute to vessels within the tumor tissues in a mouse model of glioma (Carlson et al., 2021). In addition, Li et al. showed that disseminated melanoma cells could transdifferentiate into endothelial cells in intravascular niches of various metastatic organs (Li et al., 2020). Altogether, these findings indicated that tumor cells with endothelial cell features might play important roles in tumor growth, drug resistance and metastasis.
Single-Cell Multi-Omics and Precision Cancer Therapy
Single-cell techniques have been applied in precision cancer therapy, such as tracing drug treatment responses and identifying novel therapeutic targets (Su et al., 2017; Yang et al., 2019; Jerby-Arnon et al., 2021). For example, melanoma cells with BRAF mutation often develope drug resistance after treatment with BRAF inhibitors. By use of single-cell functional proteomics, the activation of MEK/ERK and NFκB p65 pathways were revealed shortly after BRAF inhibition and before the emergence of drug resistance, suggesting that combining MEK and NFκB p65 inhibition with BRAF inhibitor could delay the adaptive cell state transition and development of resistant phenotypes (Su et al., 2017). Besides, HES6 was identified as a driver of metastasis in primary uveal melanoma by scRNA-seq, suggesting that HES6 may represent an actionable target of this tumor (Pandiani et al., 2021).
Moreover, single-cell techniques have been used to simultaneously determine the responses of heterogeneous tumors to multiple chemotherapeutic drugs, which could uncover the transcriptome networks underlying drug responses at single-cell resolution, and help to eliminate the effects of intra-tumoral heterogeneity on treatments (Roider et al., 2020; Srivatsan et al., 2020). Furthermore, single-cell techniques have advantages to identifying rare cancer cell subpopulations, which lead to the tumor progression and failure of cancer treatments (Kim et al., 2016; Miao et al., 2019; Prieto-Vila et al., 2019; Lee et al., 2020; Sehgal et al., 2021). For instance, scRNA-seq identified a subset of tumor-initiating stem cells in squamous cell carcinoma, which selectively express CD80 molecule and bind to the CTLA4 on cytotoxic T cells and thus damage the activity of T cells. Accordingly, blocking the binding of CTLA4 and CD80 could specifically eliminate these tumor-initiating stem cells and inhibit tumor relapse after immunotherapy (Miao et al., 2019). Besides, scRNA-seq revealed coexistence of multiple tumor cell subpopulations in metastatic renal cell carcinoma, whereas each tumor cell subpopulation showed distinct dysregulated signal pathways. This study further demonstrated that combinational inhibition of both EGFR and SRC signaling pathways could significantly enhance the therapeutic effect, indicating that single-cell sequencing can be used to optimize the strategy of targeted therapy (Kim et al., 2016). Similarly, scRNA-seq has been used to identify therapeutic targets for patients with refractory cancer (Lee et al., 2020).
In addition, single-cell techniques have been applied in identifying biomarkers to predict prognosis of cancer patients. For instance, by integrating large-scale bulk multi-omics and single-cell transcriptomic data of primary melanoma, a predictive model was constructed and 17 genes associated with the poor prognosis of patients were identified (Song et al., 2021). Finally, single-cell sequencing has been used to reveal the prognostic roles of stromal cell heterogeneity in multiple Cancers (Savas et al., 2018; Dominguez et al., 2020; Zhang Y. et al., 2021; Gong et al., 2021). For example, Gong et al. performed scRNA-seq of 66,627 cells from 14 nasopharyngeal carcinomas (NPCs), which revealed the stromal dynamics and NPC-specific characteristics in the TME of NPCs. Notably, they found that the dynamic immune signatures correlate with patient prognosis, such as increased infiltration of plasma B cells, dendritic cells and macrophages associated with a good prognosis (Gong et al., 2021). Moreover, scRNA-seq uncovered a subpopulation of CD8+ memory T cells in breast cancer, which showed high expression of immune checkpoint molecules and effector proteins. Importantly, this subset of T cells was observed to be significantly associated with an improved survival of patients with early-stage triple-negative breast cancer (Savas et al., 2018). Furthermore, Zhang et al. performed scRNA-seq analysis of renal cell carcinomas (RCC), which revealed that a higher fraction of endothelial cells associates with better overall survival of patients. Moreover, two macrophage subpopulations (macrophage-A and macrophage-B) were identified in the RCCs, high expression of the macrophage-A signature was observed to be associated with poor prognosis whereas high expression of the macrophage-B signature correlated with favorable prognosis (Zhang Y. et al., 2021). Besides, Dominguez et al. investigated the single-cell atlas of CAFs in pancreatic cancer by scRNA-seq, which revealed that a LRR15+ CAF subpopulation associates with a poor outcome of cancer immunotherapy (Dominguez et al., 2020). Taken together, single-cell technologies have shown great advantages in personalized therapy and prognosis prediction.
Limitations of Single-Cell Technologies
Although single-cell technologies have greatly enhanced our understanding of the tumor heterogeneity, there are still multiple limitations of these techniques, such as limited sensitivity, scale and accuracy, which need to be addressed by technological improvements or combined with other technologies (Lei et al., 2021). Furthermore, most single-cell techniques performed analysis on dissociated cells, which could not interrogate spatial architecture of tumor tissues. With the advance of new techniques, such as the spatial transcriptomics (ST), this issue can be partly solved. However, the resolution of the current ST platform is still low, the capture spot usually contains a couple of cells (Wu et al., 2021). Besides, the transcriptome and proteome of cells could be disturbed during the preparation of single-cell suspension. Moreover, most scRNA-seq approaches only detected protein-coding genes by capturing polyA RNAs, which excluded all the non-coding genes. In addition, interpretation of data generated from single-cell omics techniques has been a challenge, which heavily depended on bioinformatics methods. However, each bioinformatic algorithm has its own advantages and limitations. For example, the number of cell types identified within a tumor could be affected by using different parameters. Finally, the cost of current single-cell omics techniques is extremely expensive compared with the bulk omics approaches.
Conclusions
The dynamic evolution of tumor cells and their interactions with non-tumor cell components in the TME contributed to the tumor progression. Understating the heterogeneity of cellular compositions and their crosstalk in the TME will accelerate the development of personalized therapies. Fortunately, Single-cell multi-omics have shown great advantages in the dissecting the intra-tumoral heterogeneity. Importantly, the identification of cancer cell plasticity and their regulators have enabled us to understand the molecular underpinnings of cancer cell evolution during tumor progression.
Author Contributions
DJ conceived and designed the study. DP organized and wrote the manuscript. DJ and DP edited the manuscript.
Funding
This work was supported by National Natural Science Foundation of China (Grant No. 82072571), Shanghai Pujiang Scholar Program (Grant No. 19PJ1408500), Experimental Animal Research Fund, Science and Technology Commission of Shanghai Municipality (Grant No. 19140905600), and Shanghai Young Eastern Scholar Program.
Conflict of Interest
The authors declare that the research was conducted in the absence of any commercial or financial relationships that could be construed as a potential conflict of interest.
Publisher’s Note
All claims expressed in this article are solely those of the authors and do not necessarily represent those of their affiliated organizations, or those of the publisher, the editors and the reviewers. Any product that may be evaluated in this article, or claim that may be made by its manufacturer, is not guaranteed or endorsed by the publisher.
References
Arora, L., and Pal, D. (2021). Remodeling of Stromal Cells and Immune Landscape in Microenvironment during Tumor Progression. Front. Oncol. 11, 596798. doi:10.3389/fonc.2021.596798
Azizi, E., Carr, A. J., Plitas, G., Cornish, A. E., Konopacki, C., Prabhakaran, S., et al. (2018). Single-Cell Map of Diverse Immune Phenotypes in the Breast Tumor Microenvironment. Cell 174 (5), 1293–1308. e1236. doi:10.1016/j.cell.2018.05.060
Bassez, A., Vos, H., Van Dyck, L., Floris, G., Arijs, I., Desmedt, C., et al. (2021). A Single-Cell Map of Intratumoral Changes during Anti-PD1 Treatment of Patients with Breast Cancer. Nat. Med. 27 (5), 820–832. doi:10.1038/s41591-021-01323-8
Bingham, C., Fernandez, S. V., Fittipaldi, P., Dempsey, P. W., Ruth, K. J., Cristofanilli, M., et al. (2017). Mutational Studies on Single Circulating Tumor Cells Isolated from the Blood of Inflammatory Breast Cancer Patients. Breast Cancer Res. Treat. 163 (2), 219–230. doi:10.1007/s10549-017-4176-x
Black, J. R. M., and McGranahan, N. (2021). Genetic and Non-genetic Clonal Diversity in Cancer Evolution. Nat. Rev. Cancer 21 (6), 379–392. doi:10.1038/s41568-021-00336-2
Brabletz, T., Kalluri, R., Nieto, M. A., and Weinberg, R. A. (2018). EMT in Cancer. Nat. Rev. Cancer 18 (2), 128–134. doi:10.1038/nrc.2017.118
Carlson, J. C., Cantu Gutierrez, M., Lozzi, B., Huang-Hobbs, E., Turner, W. D., Tepe, B., et al. (2021). Identification of Diverse Tumor Endothelial Cell Populations in Malignant Glioma. Neuro Oncol. 23 (6), 932–944. doi:10.1093/neuonc/noaa297
Chen, S., Zhu, G., Yang, Y., Wang, F., Xiao, Y.-T., Zhang, N., et al. (2021). Single-cell Analysis Reveals Transcriptomic Remodellings in Distinct Cell Types that Contribute to Human Prostate Cancer Progression. Nat. Cel Biol 23 (1), 87–98. doi:10.1038/s41556-020-00613-6
Choi, W., Ochoa, A., McConkey, D. J., Aine, M., Höglund, M., Kim, W. Y., et al. (2017). Genetic Alterations in the Molecular Subtypes of Bladder Cancer: Illustration in the Cancer Genome Atlas Dataset. Eur. Urol. 72 (3), 354–365. doi:10.1016/j.eururo.2017.03.010
Cohen, Y. C., Zada, M., Wang, S.-Y., Bornstein, C., David, E., Moshe, A., et al. (2021). Identification of Resistance Pathways and Therapeutic Targets in Relapsed Multiple Myeloma Patients through Single-Cell Sequencing. Nat. Med. 27 (3), 491–503. doi:10.1038/s41591-021-01232-w
Crosetto, N., Bienko, M., and van Oudenaarden, A. (2015). Spatially Resolved Transcriptomics and beyond. Nat. Rev. Genet. 16 (1), 57–66. doi:10.1038/nrg3832
Davis, R. T., Blake, K., Ma, D., Gabra, M. B. I., Hernandez, G. A., Phung, A. T., et al. (2020). Transcriptional Diversity and Bioenergetic Shift in Human Breast Cancer Metastasis Revealed by Single-Cell RNA Sequencing. Nat. Cel Biol 22 (3), 310–320. doi:10.1038/s41556-020-0477-0
Dominguez, C. X., Müller, S., Keerthivasan, S., Koeppen, H., Hung, J., Gierke, S., et al. (2020). Single-Cell RNA Sequencing Reveals Stromal Evolution into LRRC15+ Myofibroblasts as a Determinant of Patient Response to Cancer Immunotherapy. Cancer Discov. 10 (2), 232–253. doi:10.1158/2159-8290.CD-19-0644
Duan, Q., Tang, C., Ma, Z., Chen, C., Shang, X., Yue, J., et al. (2021). Genomic Heterogeneity and Clonal Evolution in Gastroesophageal Junction Cancer Revealed by Single Cell DNA Sequencing. Front. Oncol. 11, 672020. doi:10.3389/fonc.2021.672020
Eyler, C. E., Matsunaga, H., Hovestadt, V., Vantine, S. J., van Galen, P., and Bernstein, B. E. (2020). Single-cell Lineage Analysis Reveals Genetic and Epigenetic Interplay in Glioblastoma Drug Resistance. Genome Biol. 21 (1), 174. doi:10.1186/s13059-020-02085-1
Gao, Y., Ni, X., Guo, H., Su, Z., Ba, Y., Tong, Z., et al. (2017). Single-cell Sequencing Deciphers a Convergent Evolution of Copy Number Alterations from Primary to Circulating Tumor Cells. Genome Res. 27 (8), 1312–1322. doi:10.1101/gr.216788.116
Gawad, C., Koh, W., and Quake, S. R. (2016). Single-cell Genome Sequencing: Current State of the Science. Nat. Rev. Genet. 17 (3), 175–188. doi:10.1038/nrg.2015.16
Gay, C. M., Stewart, C. A., Park, E. M., Diao, L., Groves, S. M., Heeke, S., et al. (2021). Patterns of Transcription Factor Programs and Immune Pathway Activation Define Four Major Subtypes of SCLC with Distinct Therapeutic Vulnerabilities. Cancer Cell 39 (3), 346–360. e347. doi:10.1016/j.ccell.2020.12.014
Giesen, C., Wang, H. A. O., Schapiro, D., Zivanovic, N., Jacobs, A., Hattendorf, B., et al. (2014). Highly Multiplexed Imaging of Tumor Tissues with Subcellular Resolution by Mass Cytometry. Nat. Methods 11 (4), 417–422. doi:10.1038/nmeth.2869
Giraudeau, M., Sepp, T., Ujvari, B., Renaud, F., Tasiemski, A., Roche, B., et al. (2019). Differences in Mutational Processes and Intra-Tumour Heterogeneity Between Organs. Evol. Med. Public Health 2019 (1), 139–146. doi:10.1093/emph/eoz017
Gong, L., Kwong, D. L.-W., Dai, W., Wu, P., Li, S., Yan, Q., et al. (2021). Comprehensive Single-Cell Sequencing Reveals the Stromal Dynamics and Tumor-specific Characteristics in the Microenvironment of Nasopharyngeal Carcinoma. Nat. Commun. 12 (1), 1540. doi:10.1038/s41467-021-21795-z
González-Silva, L., Quevedo, L., and Varela, I. (2020). Tumor Functional Heterogeneity Unraveled by scRNA-Seq Technologies. Trends Cancer 6 (1), 13–19. doi:10.1016/j.trecan.2019.11.010
Goveia, J., Rohlenova, K., Taverna, F., Treps, L., Conradi, L.-C., Pircher, A., et al. (2020). An Integrated Gene Expression Landscape Profiling Approach to Identify Lung Tumor Endothelial Cell Heterogeneity and Angiogenic Candidates. Cancer Cell 37 (1), 21–36. e13. doi:10.1016/j.ccell.2019.12.001
Grosselin, K., Durand, A., Marsolier, J., Poitou, A., Marangoni, E., Nemati, F., et al. (2019). High-throughput Single-Cell ChIP-Seq Identifies Heterogeneity of Chromatin States in Breast Cancer. Nat. Genet. 51 (6), 1060–1066. doi:10.1038/s41588-019-0424-9
Gunnarsson, E. B., De, S., Leder, K., and Foo, J. (2020). Understanding the Role of Phenotypic Switching in Cancer Drug Resistance. J. Theor. Biol. 490, 110162. doi:10.1016/j.jtbi.2020.110162
Guo, M., Peng, Y., Gao, A., Du, C., and Herman, J. G. (2019). Epigenetic Heterogeneity in Cancer. Biomark Res. 7, 23. doi:10.1186/s40364-019-0174-y
Gupta, P. B., Fillmore, C. M., Jiang, G., Shapira, S. D., Tao, K., Kuperwasser, C., et al. (2011). Stochastic State Transitions Give Rise to Phenotypic Equilibrium in Populations of Cancer Cells. Cell 146 (4), 633–644. doi:10.1016/j.cell.2011.07.026
Hinohara, K., and Polyak, K. (2019). Intratumoral Heterogeneity: More Than Just Mutations. Trends Cel Biol. 29 (7), 569–579. doi:10.1016/j.tcb.2019.03.003
Hinshaw, D. C., and Shevde, L. A. (2019). The Tumor Microenvironment Innately Modulates Cancer Progression. Cancer Res. 79 (18), 4557–4566. doi:10.1158/0008-5472.CAN-18-3962
Hou, Y., Song, L., Zhu, P., Zhang, B., Tao, Y., Xu, X., et al. (2012). Single-cell Exome Sequencing and Monoclonal Evolution of a JAK2-Negative Myeloproliferative Neoplasm. Cell 148 (5), 873–885. doi:10.1016/j.cell.2012.02.028
Hou, Y., Guo, H., Cao, C., Li, X., Hu, B., Zhu, P., et al. (2016). Single-cell Triple Omics Sequencing Reveals Genetic, Epigenetic, and Transcriptomic Heterogeneity in Hepatocellular Carcinomas. Cell Res 26 (3), 304–319. doi:10.1038/cr.2016.23
Ireland, A. S., Micinski, A. M., Kastner, D. W., Guo, B., Wait, S. J., Spainhower, K. B., et al. (2020). MYC Drives Temporal Evolution of Small Cell Lung Cancer Subtypes by Reprogramming Neuroendocrine Fate. Cancer Cell 38 (1), 60–78. e12. doi:10.1016/j.ccell.2020.05.001
Jerby-Arnon, L., Neftel, C., Shore, M. E., Weisman, H. R., Mathewson, N. D., McBride, M. J., et al. (2021). Opposing Immune and Genetic Mechanisms Shape Oncogenic Programs in Synovial Sarcoma. Nat. Med. 27 (2), 289–300. doi:10.1038/s41591-020-01212-6
Ji, A. L., Rubin, A. J., Thrane, K., Jiang, S., Reynolds, D. L., Meyers, R. M., et al. (2020). Multimodal Analysis of Composition and Spatial Architecture in Human Squamous Cell Carcinoma. Cell 182 (2), 497–514. e422. doi:10.1016/j.cell.2020.05.039
Jin, S., Li, R., Chen, M.-Y., Yu, C., Tang, L.-Q., Liu, Y.-M., et al. (2020). Single-cell Transcriptomic Analysis Defines the Interplay between Tumor Cells, Viral Infection, and the Microenvironment in Nasopharyngeal Carcinoma. Cel Res 30 (11), 950–965. doi:10.1038/s41422-020-00402-8
Karaayvaz, M., Cristea, S., Gillespie, S. M., Patel, A. P., Mylvaganam, R., Luo, C. C., et al. (2018). Unravelling Subclonal Heterogeneity and Aggressive Disease States in TNBC through Single-Cell RNA-Seq. Nat. Commun. 9 (1), 3588. doi:10.1038/s41467-018-06052-0
Kieffer, Y., Hocine, H. R., Gentric, G., Pelon, F., Bernard, C., Bourachot, B., et al. (2020). Single-Cell Analysis Reveals Fibroblast Clusters Linked to Immunotherapy Resistance in Cancer. Cancer Discov. 10 (9), 1330–1351. doi:10.1158/2159-8290.CD-19-1384
Kim, K.-T., Lee, H. W., Lee, H.-O., Song, H. J., Jeong, D. E., Shin, S., et al. (2016). Application of Single-Cell RNA Sequencing in Optimizing a Combinatorial Therapeutic Strategy in Metastatic Renal Cell Carcinoma. Genome Biol. 17, 80. doi:10.1186/s13059-016-0945-9
Kirschmann, D. A., Seftor, E. A., Hardy, K. M., Seftor, R. E. B., and Hendrix, M. J. C. (2012). Molecular Pathways: Vasculogenic Mimicry in Tumor Cells: Diagnostic and Therapeutic Implications. Clin. Cancer Res. 18 (10), 2726–2732. doi:10.1158/1078-0432.CCR-11-3237
Kröger, C., Afeyan, A., Mraz, J., Eaton, E. N., Reinhardt, F., Khodor, Y. L., et al. (2019). Acquisition of a Hybrid E/M State Is Essential for Tumorigenicity of Basal Breast Cancer Cells. Proc. Natl. Acad. Sci. USA 116 (15), 7353–7362. doi:10.1073/pnas.1812876116
LaFave, L. M., Kartha, V. K., Ma, S., Meli, K., Del Priore, I., Lareau, C., et al. (2020). Epigenomic State Transitions Characterize Tumor Progression in Mouse Lung Adenocarcinoma. Cancer Cell 38 (2), 212–228. e213. doi:10.1016/j.ccell.2020.06.006
Lee, H. W., Chung, W., Lee, H.-O., Jeong, D. E., Jo, A., Lim, J. E., et al. (2020). Single-cell RNA Sequencing Reveals the Tumor Microenvironment and Facilitates Strategic Choices to Circumvent Treatment Failure in a Chemorefractory Bladder Cancer Patient. Genome Med. 12 (1), 47. doi:10.1186/s13073-020-00741-6
Lei, Y., Tang, R., Xu, J., Wang, W., Zhang, B., Liu, J., et al. (2021). Applications of Single-Cell Sequencing in Cancer Research: Progress and Perspectives. J. Hematol. Oncol. 14 (1), 91. doi:10.1186/s13045-021-01105-2
Li, H., van der Leun, A. M., Yofe, I., Lubling, Y., Gelbard-Solodkin, D., van Akkooi, A. C. J., et al. (2019). Dysfunctional CD8 T Cells Form a Proliferative, Dynamically Regulated Compartment within Human Melanoma. Cell 176 (4), 775–789. e718. doi:10.1016/j.cell.2018.11.043
Li, X., Karras, P., Torres, R., Rambow, F., van den Oord, J., Marine, J.-C., et al. (2020). Disseminated Melanoma Cells Transdifferentiate into Endothelial Cells in Intravascular Niches at Metastatic Sites. Cel Rep. 31 (11), 107765. doi:10.1016/j.celrep.2020.107765
Lin, J.-R., Fallahi-Sichani, M., and Sorger, P. K. (2015). Highly Multiplexed Imaging of Single Cells Using a High-Throughput Cyclic Immunofluorescence Method. Nat. Commun. 6, 8390. doi:10.1038/ncomms9390
Lin, J.-R., Izar, B., Wang, S., Yapp, C., Mei, S., Shah, P. M., et al. (2018). Highly Multiplexed Immunofluorescence Imaging of Human Tissues and Tumors Using T-CyCIF and Conventional Optical Microscopes. Elife 7, e31657. doi:10.7554/eLife.31657
Lin, W., Noel, P., Borazanci, E. H., Lee, J., Amini, A., Han, I. W., et al. (2020). Single-cell Transcriptome Analysis of Tumor and Stromal Compartments of Pancreatic Ductal Adenocarcinoma Primary Tumors and Metastatic Lesions. Genome Med. 12 (1), 80. doi:10.1186/s13073-020-00776-9
Liu, J., Xu, T., Jin, Y., Huang, B., and Zhang, Y. (2020a). Progress and Clinical Application of Single-Cell Transcriptional Sequencing Technology in Cancer Research. Front. Oncol. 10, 593085. doi:10.3389/fonc.2020.593085
Liu, L., Yang, H., Men, D., Wang, M., Gao, X., Zhang, T., et al. (2020b). Development of Microfluidic Platform Capable of High-Throughput Absolute Quantification of Single-Cell Multiple Intracellular Proteins from Tumor Cell Lines and Patient Tumor Samples. Biosens. Bioelectron. 155, 112097. doi:10.1016/j.bios.2020.112097
Liu, J., Qu, S., Zhang, T., Gao, Y., Shi, H., Song, K., et al. (2021a). Applications of Single-Cell Omics in Tumor Immunology. Front. Immunol. 12, 697412. doi:10.3389/fimmu.2021.697412
Liu, R., Gao, Q., Foltz, S. M., Fowles, J. S., Yao, L., Wang, J. T., et al. (2021b). Co-evolution of Tumor and Immune Cells during Progression of Multiple Myeloma. Nat. Commun. 12 (1), 2559. doi:10.1038/s41467-021-22804-x
Lourenco, A. R., Ban, Y., Crowley, M. J., Lee, S. B., Ramchandani, D., Du, W., et al. (2020). Differential Contributions of Pre- and Post-EMT Tumor Cells in Breast Cancer Metastasis. Cancer Res. 80 (2), 163–169. doi:10.1158/0008-5472.CAN-19-1427
Mangano, C., Ferrarini, A., Forcato, C., Garonzi, M., Tononi, P., Lanzellotto, R., et al. (2019). Precise Detection of Genomic Imbalances at Single-Cell Resolution Reveals Intra-patient Heterogeneity in Hodgkin's Lymphoma. Blood Cancer J. 9 (12), 92. doi:10.1038/s41408-019-0256-y
Maniotis, A. J., Folberg, R., Hess, A., Seftor, E. A., Gardner, L. M. G., Pe'er, J., et al. (1999). Vascular Channel Formation by Human Melanoma Cells In Vivo and In Vitro: Vasculogenic Mimicry. Am. J. Pathol. 155 (3), 739–752. doi:10.1016/S0002-9440(10)65173-5
Meacham, C. E., and Morrison, S. J. (2013). Tumour Heterogeneity and Cancer Cell Plasticity. Nature 501 (7467), 328–337. doi:10.1038/nature12624
Miao, Y., Yang, H., Levorse, J., Yuan, S., Polak, L., Sribour, M., et al. (2019). Adaptive Immune Resistance Emerges from Tumor-Initiating Stem Cells. Cell 177 (5), 1172–1186. e1114. doi:10.1016/j.cell.2019.03.025
Michor, F., and Polyak, K. (2010). The Origins and Implications of Intratumor Heterogeneity: Fig. 1. Cancer Prev. Res. 3 (11), 1361–1364. doi:10.1158/1940-6207.CAPR-10-0234
Navin, N., Kendall, J., Troge, J., Andrews, P., Rodgers, L., McIndoo, J., et al. (2011). Tumour Evolution Inferred by Single-Cell Sequencing. Nature 472 (7341), 90–94. doi:10.1038/nature09807
Neftel, C., Laffy, J., Filbin, M. G., Hara, T., Shore, M. E., Rahme, G. J., et al. (2019). An Integrative Model of Cellular States, Plasticity, and Genetics for Glioblastoma. Cell 178 (4), 835–849. e821. doi:10.1016/j.cell.2019.06.024
Pachynski, R. K., Kim, E. H., Miheecheva, N., Kotlov, N., Ramachandran, A., Postovalova, E., et al. (2021). Single-cell Spatial Proteomic Revelations on the Multiparametric MRI Heterogeneity of Clinically Significant Prostate Cancer. Clin. Cancer Res. 27 (12), 3478–3490. doi:10.1158/1078-0432.CCR-20-4217
Pandiani, C., Strub, T., Nottet, N., Cheli, Y., Gambi, G., Bille, K., et al. (2021). Single-cell RNA Sequencing Reveals Intratumoral Heterogeneity in Primary Uveal Melanomas and Identifies HES6 as a Driver of the Metastatic Disease. Cell Death Differ 28 (6), 1990–2000. doi:10.1038/s41418-020-00730-7
Patel, A. P., Tirosh, I., Trombetta, J. J., Shalek, A. K., Gillespie, S. M., Wakimoto, H., et al. (2014). Single-cell RNA-Seq Highlights Intratumoral Heterogeneity in Primary Glioblastoma. Science 344 (6190), 1396–1401. doi:10.1126/science.1254257
Peng, J., Sun, B.-F., Chen, C.-Y., Zhou, J.-Y., Chen, Y.-S., Chen, H., et al. (2019). Single-cell RNA-Seq Highlights Intra-tumoral Heterogeneity and Malignant Progression in Pancreatic Ductal Adenocarcinoma. Cel Res 29 (9), 725–738. doi:10.1038/s41422-019-0195-y
Pombo Antunes, A. R., Scheyltjens, I., Lodi, F., Messiaen, J., Antoranz, A., Duerinck, J., et al. (2021). Single-cell Profiling of Myeloid Cells in Glioblastoma across Species and Disease Stage Reveals Macrophage Competition and Specialization. Nat. Neurosci. 24 (4), 595–610. doi:10.1038/s41593-020-00789-y
Prieto-Vila, M., Usuba, W., Takahashi, R.-u., Shimomura, I., Sasaki, H., Ochiya, T., et al. (2019). Single-Cell Analysis Reveals a Preexisting Drug-Resistant Subpopulation in the Luminal Breast Cancer Subtype. Cancer Res. 79 (17), 4412–4425. doi:10.1158/0008-5472.CAN-19-0122
Qian, J., Olbrecht, S., Boeckx, B., Vos, H., Laoui, D., Etlioglu, E., et al. (2020). A Pan-Cancer Blueprint of the Heterogeneous Tumor Microenvironment Revealed by Single-Cell Profiling. Cel Res 30 (9), 745–762. doi:10.1038/s41422-020-0355-0
Reinert, T., and Barrios, C. H. (2015). Optimal Management of Hormone Receptor Positive Metastatic Breast Cancer in 2016. Ther. Adv. Med. Oncol. 7 (6), 304–320. doi:10.1177/1758834015608993
Reza, K. K., Dey, S., Wuethrich, A., Jing Wang, W., Behren, A., Antaw, F., et al. (2021). In Situ Single Cell Proteomics Reveals Circulating Tumor Cell Heterogeneity during Treatment. ACS Nano 15, 11231–11243. doi:10.1021/acsnano.0c10008
Riemondy, K. A., Venkataraman, S., Willard, N., Nellan, A., Sanford, B., Griesinger, A. M., et al. (2021). Neoplastic and Immune Single-Cell Transcriptomics Define Subgroup-specific Intra-tumoral Heterogeneity of Childhood Medulloblastoma. Neuro Oncol. doi:10.1093/neuonc/noab135
Robertson, A. G., Groeneveld, C. S., Jordan, B., Lin, X., McLaughlin, K. A., Das, A., et al. (2020). Identification of Differential Tumor Subtypes of T1 Bladder Cancer. Eur. Urol. 78 (4), 533–537. doi:10.1016/j.eururo.2020.06.048
Roerink, S. F., Sasaki, N., Lee-Six, H., Young, M. D., Alexandrov, L. B., Behjati, S., et al. (2018). Intra-tumour Diversification in Colorectal Cancer at the Single-Cell Level. Nature 556 (7702), 457–462. doi:10.1038/s41586-018-0024-3
Roider, T., Seufert, J., Uvarovskii, A., Frauhammer, F., Bordas, M., Abedpour, N., et al. (2020). Dissecting Intratumour Heterogeneity of Nodal B-Cell Lymphomas at the Transcriptional, Genetic and Drug-Response Levels. Nat. Cel Biol 22 (7), 896–906. doi:10.1038/s41556-020-0532-x
Roma-Rodrigues, C., Mendes, R., Baptista, P., and Fernandes, A. (2019). Targeting Tumor Microenvironment for Cancer Therapy. Ijms 20 (4), 840. doi:10.3390/ijms20040840
Rudin, C. M., Poirier, J. T., Byers, L. A., Dive, C., Dowlati, A., George, J., et al. (2019). Molecular Subtypes of Small Cell Lung Cancer: a Synthesis of Human and Mouse Model Data. Nat. Rev. Cancer 19 (5), 289–297. doi:10.1038/s41568-019-0133-9
Sacchetti, A., Teeuwssen, M., Verhagen, M., Joosten, R., Xu, T., Stabile, R., et al. (2021). Phenotypic Plasticity Underlies Local Invasion and Distant Metastasis in colon Cancer. Elife 10, e61461. doi:10.7554/eLife.61461
Sahai, E., Astsaturov, I., Cukierman, E., DeNardo, D. G., Egeblad, M., Evans, R. M., et al. (2020). A Framework for Advancing Our Understanding of Cancer-Associated Fibroblasts. Nat. Rev. Cancer 20 (3), 174–186. doi:10.1038/s41568-019-0238-1
Savas, P., Virassamy, B., Virassamy, B., Ye, C., Salim, A., Mintoff, C. P., et al. (2018). Single-cell Profiling of Breast Cancer T Cells Reveals a Tissue-Resident Memory Subset Associated with Improved Prognosis. Nat. Med. 24 (7), 986–993. doi:10.1038/s41591-018-0078-7
Schlesinger, Y., Yosefov-Levi, O., Kolodkin-Gal, D., Granit, R. Z., Peters, L., Kalifa, R., et al. (2020). Single-cell Transcriptomes of Pancreatic Preinvasive Lesions and Cancer Reveal Acinar Metaplastic Cell's Heterogeneity. Nat. Commun. 11 (1), 4516. doi:10.1038/s41467-020-18207-z
Schulz, D., Zanotelli, V. R. T., Fischer, J. R., Schapiro, D., Engler, S., Lun, X.-K., et al. (2018). Simultaneous Multiplexed Imaging of mRNA and Proteins with Subcellular Resolution in Breast Cancer Tissue Samples by Mass Cytometry. Cel Syst. 6 (1), 25–36. doi:10.1016/j.cels.2017.12.001
Schwartzman, O., and Tanay, A. (2015). Single-cell Epigenomics: Techniques and Emerging Applications. Nat. Rev. Genet. 16 (12), 716–726. doi:10.1038/nrg3980
Sehgal, K., Portell, A., Ivanova, E. V., Lizotte, P. H., Mahadevan, N. R., Greene, J. R., et al. (2021). Dynamic Single-Cell RNA Sequencing Identifies Immunotherapy Persister Cells Following PD-1 Blockade. J. Clin. Invest. 131 (2), 1–17. doi:10.1172/JCI135038
Song, W.-M., Agrawal, P., Von Itter, R., Fontanals-Cirera, B., Wang, M., Zhou, X., et al. (2021). Network Models of Primary Melanoma Microenvironments Identify Key Melanoma Regulators Underlying Prognosis. Nat. Commun. 12 (1), 1214. doi:10.1038/s41467-021-21457-0
Sottoriva, A., Spiteri, I., Piccirillo, S. G. M., Touloumis, A., Collins, V. P., Marioni, J. C., et al. (2013). Intratumor Heterogeneity in Human Glioblastoma Reflects Cancer Evolutionary Dynamics. Proc. Natl. Acad. Sci. 110 (10), 4009–4014. doi:10.1073/pnas.1219747110
Srivatsan, S. R., McFaline-Figueroa, J. L., Ramani, V., Saunders, L., Cao, J., Packer, J., et al. (2020). Massively Multiplex Chemical Transcriptomics at Single-Cell Resolution. Science 367 (6473), 45–51. doi:10.1126/science.aax6234
Su, Y., Wei, W., Robert, L., Xue, M., Tsoi, J., Garcia-Diaz, A., et al. (2017). Single-cell Analysis Resolves the Cell State Transition and Signaling Dynamics Associated with Melanoma Drug-Induced Resistance. Proc. Natl. Acad. Sci. USA 114 (52), 13679–13684. doi:10.1073/pnas.1712064115
Su, X., Zhao, L., Shi, Y., Zhang, R., Long, Q., Bai, S., et al. (2021). Clonal Evolution in Liver Cancer at Single-Cell and Single-Variant Resolution. J. Hematol. Oncol. 14 (1), 22. doi:10.1186/s13045-021-01036-y
Tang, F., Barbacioru, C., Wang, Y., Nordman, E., Lee, C., Xu, N., et al. (2009). mRNA-Seq Whole-Transcriptome Analysis of a Single Cell. Nat. Methods 6 (5), 377–382. doi:10.1038/nmeth.1315
Thong, T., Wang, Y., Brooks, M. D., Lee, C. T., Scott, C., Balzano, L., et al. (2020). Hybrid Stem Cell States: Insights into the Relationship between Mammary Development and Breast Cancer Using Single-Cell Transcriptomics. Front. Cel Dev. Biol. 8, 288. doi:10.3389/fcell.2020.00288
Tirosh, I., Izar, B., Prakadan, S. M., Wadsworth, M. H., Treacy, D., Trombetta, J. J., et al. (2016). Dissecting the Multicellular Ecosystem of Metastatic Melanoma by Single-Cell RNA-Seq. Science 352 (6282), 189–196. doi:10.1126/science.aad0501
Vander Velde, R., Yoon, N., Marusyk, V., Durmaz, A., Dhawan, A., Miroshnychenko, D., et al. (2020). Resistance to Targeted Therapies as a Multifactorial, Gradual Adaptation to Inhibitor Specific Selective Pressures. Nat. Commun. 11 (1), 2393. doi:10.1038/s41467-020-16212-w
Wagner, J., Rapsomaniki, M. A., Chevrier, S., Anzeneder, T., Langwieder, C., Dykgers, A., et al. (2019). A Single-Cell Atlas of the Tumor and Immune Ecosystem of Human Breast Cancer. Cell 177 (5), 1330–1345. e1318. doi:10.1016/j.cell.2019.03.005
Wang, Y., Waters, J., Leung, M. L., Unruh, A., Roh, W., Shi, X., et al. (2014). Clonal Evolution in Breast Cancer Revealed by Single Nucleus Genome Sequencing. Nature 512 (7513), 155–160. doi:10.1038/nature13600
Wang, L., Babikir, H., Müller, S., Yagnik, G., Shamardani, K., Catalan, F., et al. (2019). The Phenotypes of Proliferating Glioblastoma Cells Reside on a Single Axis of Variation. Cancer Discov. 9 (12), 1708–1719. doi:10.1158/2159-8290.CD-19-0329
Wang, C., Li, Y., Jia, L., Kim, J. k., Li, J., Deng, P., et al. (2021a). CD276 Expression Enables Squamous Cell Carcinoma Stem Cells to Evade Immune Surveillance. Cell Stem Cell 28, 1597–1613. doi:10.1016/j.stem.2021.04.011
Wang, R., Dang, M., Harada, K., Han, G., Wang, F., Pool Pizzi, M., et al. (2021b). Single-cell Dissection of Intratumoral Heterogeneity and Lineage Diversity in Metastatic Gastric Adenocarcinoma. Nat. Med. 27 (1), 141–151. doi:10.1038/s41591-020-1125-8
Williamson, S. C., Metcalf, R. L., Trapani, F., Mohan, S., Antonello, J., Abbott, B., et al. (2016). Vasculogenic Mimicry in Small Cell Lung Cancer. Nat. Commun. 7, 13322. doi:10.1038/ncomms13322
Wouters, J., Kalender-Atak, Z., Minnoye, L., Spanier, K. I., De Waegeneer, M., Bravo González-Blas, C., et al. (2020). Robust Gene Expression Programs Underlie Recurrent Cell States and Phenotype Switching in Melanoma. Nat. Cel Biol 22 (8), 986–998. doi:10.1038/s41556-020-0547-3
Wu, M., and Singh, A. K. (2015). Microfluidic Flow Cytometry for Single-Cell Protein Analysis. Methods Mol. Biol. 1346, 69–83. doi:10.1007/978-1-4939-2987-0_6
Wu, Y., Yang, S., Ma, J., Chen, Z., Song, G., Rao, D., et al. (2021). Spatiotemporal Immune Landscape of Colorectal Cancer Liver Metastasis at Single-Cell Level. Cancer Discov., candisc.0316.2021. doi:10.1158/2159-8290.CD-21-0316
Yang, L., Zhang, X., Hou, Q., Huang, M., Zhang, H., Jiang, Z., et al. (2019). Single-cell RNA-Seq of Esophageal Squamous Cell Carcinoma Cell Line with Fractionated Irradiation Reveals Radioresistant Gene Expression Patterns. BMC Genomics 20 (1), 611. doi:10.1186/s12864-019-5970-0
Zhang, J., Späth, S. S., Marjani, S. L., Zhang, W., and Pan, X. (2018). Characterization of Cancer Genomic Heterogeneity by Next-Generation Sequencing Advances Precision Medicine in Cancer Treatment. Precis Clin. Med. 1 (1), 29–48. doi:10.1093/pcmedi/pby007
Zhang, M., Yang, H., Wan, L., Wang, Z., Wang, H., Ge, C., et al. (2020). Single-cell Transcriptomic Architecture and Intercellular Crosstalk of Human Intrahepatic Cholangiocarcinoma. J. Hepatol. 73 (5), 1118–1130. doi:10.1016/j.jhep.2020.05.039
Zhang, M., Hu, S., Min, M., Ni, Y., Lu, Z., Sun, X., et al. (2021a). Dissecting Transcriptional Heterogeneity in Primary Gastric Adenocarcinoma by Single Cell RNA Sequencing. Gut 70 (3), 464–475. doi:10.1136/gutjnl-2019-320368
Zhang, Y., Narayanan, S. P., Mannan, R., Raskind, G., Wang, X., Vats, P., et al. (2021b). Single-cell Analyses of Renal Cell Cancers Reveal Insights into Tumor Microenvironment, Cell of Origin, and Therapy Response. Proc. Natl. Acad. Sci. USA 118 (24), e2103240118. doi:10.1073/pnas.2103240118
Zhao, Q., Eichten, A., Parveen, A., Adler, C., Huang, Y., Wang, W., et al. (2018). Single-Cell Transcriptome Analyses Reveal Endothelial Cell Heterogeneity in Tumors and Changes Following Antiangiogenic Treatment. Cancer Res. 78 (9), 2370–2382. doi:10.1158/0008-5472.CAN-17-2728
Zhao, S., Zuo, W.-J., Shao, Z.-M., and Jiang, Y.-Z. (2020). Molecular Subtypes and Precision Treatment of Triple-Negative Breast Cancer. Ann. Transl Med. 8 (7), 499. doi:10.21037/atm.2020.03.194
Zheng, C., Zheng, L., Yoo, J.-K., Guo, H., Zhang, Y., Guo, X., et al. (2017). Landscape of Infiltrating T Cells in Liver Cancer Revealed by Single-Cell Sequencing. Cell 169 (7), 1342–1356. e1316. doi:10.1016/j.cell.2017.05.035
Zhou, Y., Yang, D., Yang, Q., Lv, X., Huang, W., Zhou, Z., et al. (2020). Single-cell RNA Landscape of Intratumoral Heterogeneity and Immunosuppressive Microenvironment in Advanced Osteosarcoma. Nat. Commun. 11 (1), 6322. doi:10.1038/s41467-020-20059-6
Keywords: single-cell techniques, tumor heterogeneity, cellular plasticity, clone evolution, precision therapy
Citation: Pan D and Jia D (2021) Application of Single-Cell Multi-Omics in Dissecting Cancer Cell Plasticity and Tumor Heterogeneity. Front. Mol. Biosci. 8:757024. doi: 10.3389/fmolb.2021.757024
Received: 11 August 2021; Accepted: 29 September 2021;
Published: 15 October 2021.
Edited by:
Abhijit DE, Tata Memorial Hospital, IndiaReviewed by:
Pritha Ray, Advanced Centre for Treatment, Research and Education in Cancer, IndiaSandeep Singh, National Institute of Biomedical Genomics (NIBMG), India
Copyright © 2021 Pan and Jia. This is an open-access article distributed under the terms of the Creative Commons Attribution License (CC BY). The use, distribution or reproduction in other forums is permitted, provided the original author(s) and the copyright owner(s) are credited and that the original publication in this journal is cited, in accordance with accepted academic practice. No use, distribution or reproduction is permitted which does not comply with these terms.
*Correspondence: Deshui Jia, RGVzaHVpLmppYUBzaGdoLmNu