- 1Biomedical Research Center, Qatar University, Doha, Qatar
- 2Department of Drug Design, University of Groningen, Groningen, Netherlands
- 3Department of Physical Education (PE), College of Education, Qatar University, Doha, Qatar
- 4Department of Pharmaceutical Sciences, College of Pharmacy, QU Health, Qatar University, Doha, Qatar
- 5Biomedical and Pharmaceutical Research Unit (BPRU), QU Health, Qatar University, Doha, Qatar
- 6Anti-Doping Lab Qatar, Doha, Qatar
- 7Laboratorio Antidoping, Federazione Medico Sportiva Italiana, Rome, Italy
- 8College of Health and Life Sciences, Hamad Bin Khalifa University (HBKU), Doha, Qatar
Introduction: Biological aging is associated with changes in the metabolic pathways. Leukocyte telomere length (LTL) is a predictive marker of biological aging; however, the underlying metabolic pathways remain largely unknown. The aim of this study was to investigate the metabolic alterations and identify the metabolic predictors of LTL in elite male soccer players.
Methods: Levels of 837 blood metabolites and LTL were measured in 126 young elite male soccer players who tested negative for doping abuse at anti-doping laboratory in Italy. Multivariate analysis using orthogonal partial least squares (OPLS), univariate linear models and enrichment analyses were conducted to identify metabolites and metabolic pathways associated with LTL. Generalized linear model followed by receiver operating characteristic (ROC) analysis were conducted to identify top metabolites predictive of LTL.
Results: Sixty-seven metabolites and seven metabolic pathways showed significant associations with LTL. Among enriched pathways, lysophospholipids, benzoate metabolites, and glycine/serine/threonine metabolites were elevated with longer LTL. Conversely, monoacylglycerols, sphingolipid metabolites, long chain fatty acids and polyunsaturated fatty acids were enriched with shorter telomeres. ROC analysis revealed eight metabolites that best predict LTL, including glutamine, N-acetylglutamine, xanthine, beta-sitosterol, N2-acetyllysine, stearoyl-arachidonoyl-glycerol (18:0/20:4), N-acetylserine and 3-7-dimethylurate with AUC of 0.75 (0.64–0.87, p < 0.0001).
Conclusion: This study characterized the metabolic activity in relation to telomere length in elite soccer players. Investigating the functional relevance of these associations could provide a better understanding of exercise physiology and pathophysiology of elite athletes.
Introduction
Telomeres are repetitive non-coding DNA sequences located at the end of chromosomes, which protect against DNA damage and preserve genome integrity as they shorten during cell division (Blackburn and Gall, 1978; Blackburn et al., 2006). When the mean telomere length reaches a critical value, replicative senescence and cell death occurs. Therefore, telomere length is regarded as a marker of biological aging (Blackburn, 2001; Campisi and d'Adda di Fagagna, 2007; Abdallah et al., 2009). Short leukocyte telomere length (LTL) has been associated with age (Lindsey et al., 1991; Slagboom et al., 1994; Abdallah et al., 2009; Broer et al., 2013) as well as multiple age-related diseases such as diabetes (Jeanclos et al., 1998; Fitzpatrick et al., 2007; Zhao et al., 2013), cardiovascular disease (Brouilette et al., 2003; Brouilette et al., 2007; Fitzpatrick et al., 2007; Maubaret et al., 2010; Haycock et al., 2014; Rehkopf et al., 2016) and dementia (Martin-Ruiz et al., 2006; Honig et al., 2012). Short LTL was also linked to increased risk of mortality (Cawthon et al., 2003; Bakaysa et al., 2007; Kimura et al., 2008; Ehrlenbach et al., 2009; Fitzpatrick et al., 2011; Deelen et al., 2014; Rode et al., 2015; Marioni et al., 2018), although such association was not supported by other studies (Martin-Ruiz et al., 2005; Bischoff et al., 2006; Harris et al., 2006; Njajou et al., 2009; Houben et al., 2011; Strandberg et al., 2011). Despite the large number of studies establishing the link between LTL and wellbeing, the molecular pathways underlying these associations are still largely unknown.
Various alterations of metabolic pathways constitute key features of longevity (Gonzalez-Covarrubias et al., 2013; Montoliu et al., 2014; Cheng et al., 2015). Metabolic changes were also linked with age-related diseases, such as type-2 diabetes, atherosclerosis, cancer, and Alzheimer’s disease (Matsumoto et al., 2007; Suhre et al., 2010; Oresic et al., 2011; Cao et al., 2012; Wang-Sattler et al., 2012; Yu et al., 2012; Floegel et al., 2013; Liu et al., 2017; Al-Khelaifi et al., 2018; Al-Khelaifi et al., 2019; Al-Sulaiti et al., 2019; Ilhame et al., 2020; Mohamed et al., 2020). However, a limited number of studies investigated metabolic pathways associated with LTL (Menni et al., 2013; Zhao et al., 2014; Zierer et al., 2016). One study investigated the metabolic biomarkers of aging in six thousand individuals from TwinsUK registry and identified of 22 metabolites that strongly correlated with age and related traits, but not with LTL (Menni et al., 2013). Another study of 423 American Indians identified 19 metabolites associated with LTL (Zhao et al., 2014). A more recent study tested the association between 280 blood metabolites and LTL in 3,511 females from TwinsUK and replicated results in the KORA cohort. The results confirmed the association of 1-stearoylglycerophosphoinositol and 1-palmitoylglycerophosphoinositol with LTL, suggesting involvement of fatty acid metabolism and particularly membrane composition in biological aging. The study also reported the association of gamma-glutamyltyrosine and gamma-glutamylphenylalanine with LTL, suggesting the involvement of the glutathione cycle and markers of increased oxidative stress (Zierer et al., 2016).
Exercise training was shown to reduce the rate of telomere shortening during the aging process (Shammas, 2011; Balan et al., 2018). Studies have suggested that regular exercise training is associated with longer telomeres (Arsenis et al., 2017); however, the metabolic signature of telomere length in long-term exercising elite endurance athletes (such as soccer players) has not been described. In the current study, an untargeted metabolomics approach was used to investigate the association between LTL and 837 serum metabolites in 126 young male elite soccer players and the metabolic pathways associated with LTL.
Methods
Cohort
126 young elite male soccer players who participated in national or international sports events and tested negative for doping substances at anti-doping laboratories in Italy were included in this study. Metabolomics study utilized spare serum samples collected for human growth hormone anti-doping tests. Briefly, samples were delivered to the anti-doing laboratory within 36 h under cooling conditions. Once received, samples were immediately centrifuged to separate the serum and then stored at −20°C until analysis. Due to the strict anonymization process undertaken by anti-doping laboratories and as per study’s ethics, only information related to the type of sport and athlete’s gender were available to researchers. All other information were not available, including age, ethnicity, or the time of recruitment (pre or post exercise). This study was performed in line with the World Medical Association Declaration of Helsinki–Ethical Principles for medical research involving human subjects. All protocols were approved by the Institutional Research Board of Qatar University (QU-IRB 1277-E/20). All participants consented for the use of their samples for research.
Metabolomics
Metabolic profiling of participants’ serum samples was conducted using established protocols at Metabolon’s established protocols using a Waters ACQUITY ultra-performance liquid chromatography (UPLC) and a Thermo Scientific Q-Exactive high resolution/accurate mass spectrometer interfaced with a heated electrospray ionization (HESI-II) source and Orbitrap mass analyzer operated at 35,000 mass resolution. The detailed description of the liquid chromatography-mass spectrometry (LC-MS) methodology was previously reported (Evans et al., 2014). Briefly, 100 μL of sample was used for each analysis. Small molecules were extracted in an 80% methanol solution containing recovery standards. The resulting extract was divided into five fractions: two for analysis by two separate reverse phase (RP)/UPLC-MS/MS methods with positive ion mode electrospray ionization (ESI), one for analysis by RP/UPLC-MS/MS with negative ion mode ESI, one for analysis by hydrophilic interaction chromatography (HILIC)/UPLC-MS/MS with negative ion mode ESI, and one sample was reserved for backup. On average, 1,009 features per sample (ranges from 906 to 1,038) are measured above the detection limits (Kong and Hernandez-Ferrer, 2020). Raw data were extracted, peak-identified and quality control processed using Metabolon’s hardware and software (DeHaven et al., 2012). Compounds were identified by comparison to library entries of purified standards or recurrent unknown entities with more than 3,300 commercially available purified standard compounds. Library matches for each compound were checked for each sample and corrected if necessary (Evans et al., 2014). Further details of raw data extraction and types of quality control samples and the parameters used to assure data collection quality were previously described (Montrose et al., 2012).
Measurement of Telomere Length
DNA was extracted from whole blood using DNeasy Blood and Tissue kit according to manufacturer’s instructions (Qiagen, Germany). Nanodrop was used to assess the concentration/quality of DNA. The average LTL in extracted DNA samples were assessed using Absolute Human Telomere Length Quantification qPCR Assay Kit according to manufacturer’s instructions (ScienCell, United States). The kit includes telomere primer set that amplifies telomere sequences, a single copy reference region for data normalization and a reference genomic DNA sample with known telomere length as a reference for calculating the telomere length of target samples.
Statistical Analysis
Metabolomics data were log-transformed to ensure normality of distribution. Batch correction was performed by Metabolon by rescaling the median of each metabolite to 1. LTL was log transformed to alleviate the original skewing of the distribution. Principal component analysis (PCA) was performed as an exploratory approach for finding out the main factors contributing to the observed variation in the data. Orthogonal partial least square (OPLS) was performed to identify components that best differentiate LTL whilst dissecting orthogonal components that do not differentiate LTL. Both PCA and OPLS were run using SIMCA 16 with the default metabolite-wise metabolite missingness threshold of 50%. Linear models for association analysis were run using the R statistical package (version 2.14, www.r-project.org/) to identify metabolites associated with LTL. The model also corrected for hemolysis levels (determined visually by Metabolon) and PCs from PCA analysis. Function enrichment analysis was performed using the one tailed Wilcoxon sum of the ranks test. The LTL variable was then categorized into low (below mean) and high (above mean) levels. A logistic regression (or Generalised linear model based on the Binomial family) was then used together with a step-wise procedure to determine a subset of metabolites (of those with the nominal p value ≤ 0.05 from the linear model) that best predicts the categorized LTL (Y variable). Using SPSS statistical package (version 27), the receiver operating characteristic (ROC) analysis was used to assess the discriminatory capacity of the identified subset of metabolites. Kyoto Encyclopedia of Genes and Genomes (KEGG) pathways were utilized in order to gain further insight into the biochemistry of identified metabolites.
Results
Multivariate Analysis of Metabolomics Data of Elite Soccer Players
Non-targeted metabolomics was applied to determine the metabolic signatures of 126 elite male soccer players. An OPLS analysis revealed one class-discriminatory component accounting for 69% of the variation in the LTL data (Figure 1A). The corresponding loading score, shown in Figure 1B, suggests enrichment of lysophosolipids, benzoate metabolites and glycine/serine/threonine metabolites with longer LTL, whereas monoacylglycerols, long change fatty acids, polyunsaturated fatty acids and sphingolipids were enriched with shorter LTL.
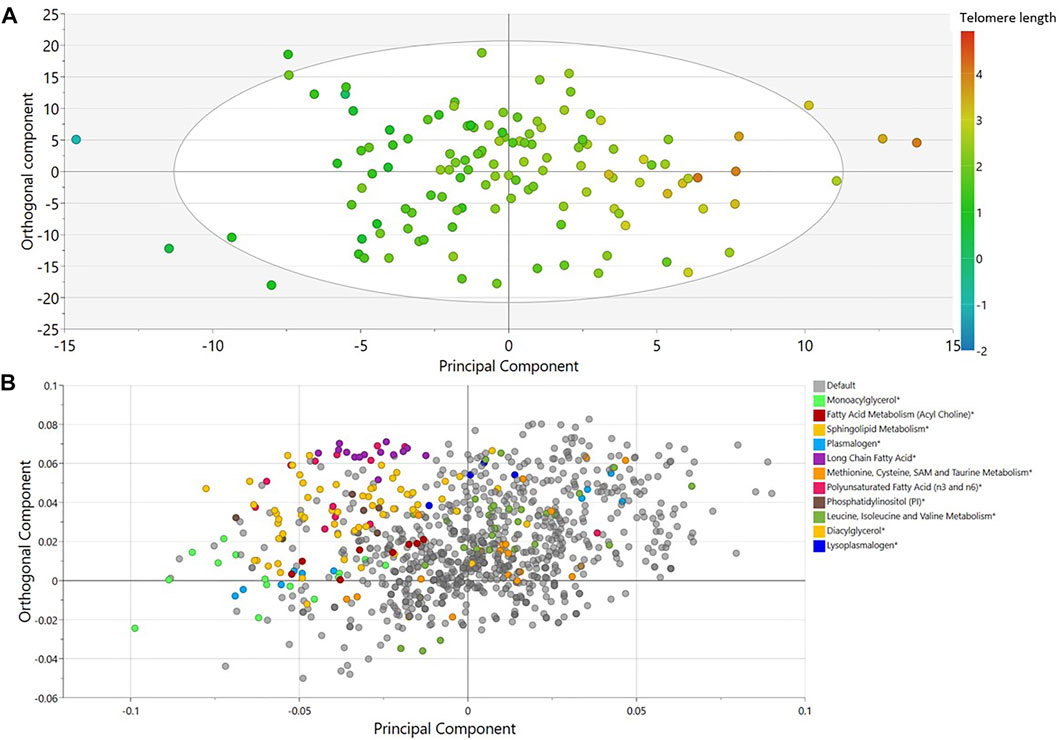
FIGURE 1. OPLS model of metabolites associated with LTL in elite male soccer players. (A) Score plot showing the class-discriminatory component 1 (x-axis) versus orthogonal component (y-axis) for LTL. (B) The corresponding loading plots showing top associated metabolites differentiating short from long LTL. Sub-pathway categories enriched in significant metabolites from regression analysis are shown in color.
Univariate Association and Function Enrichment Analyses
A linear model was used to assess the significance of metabolite-associations with the LTL after correcting for hemolysis levels, PC1 and PC2. Sixty-seven metabolites associated with LTL (p < 0.05) were identified and their associated pathways listed (Table 1). Enrichment analysis confirmed an over-representation of lysophosolipids, benzoate metabolites and glycine/serine/threonine metabolites with longer LTL, and monoacylglycerols, long change fatty acids, polyunsaturated fatty acids and sphingolipids with shorter LTL (Figure 2). Scatter plots of metabolites representing these pathways, which either decreased (Figure 3A) or increased (Figure 3B) with LTL, are shown in Figure 3.
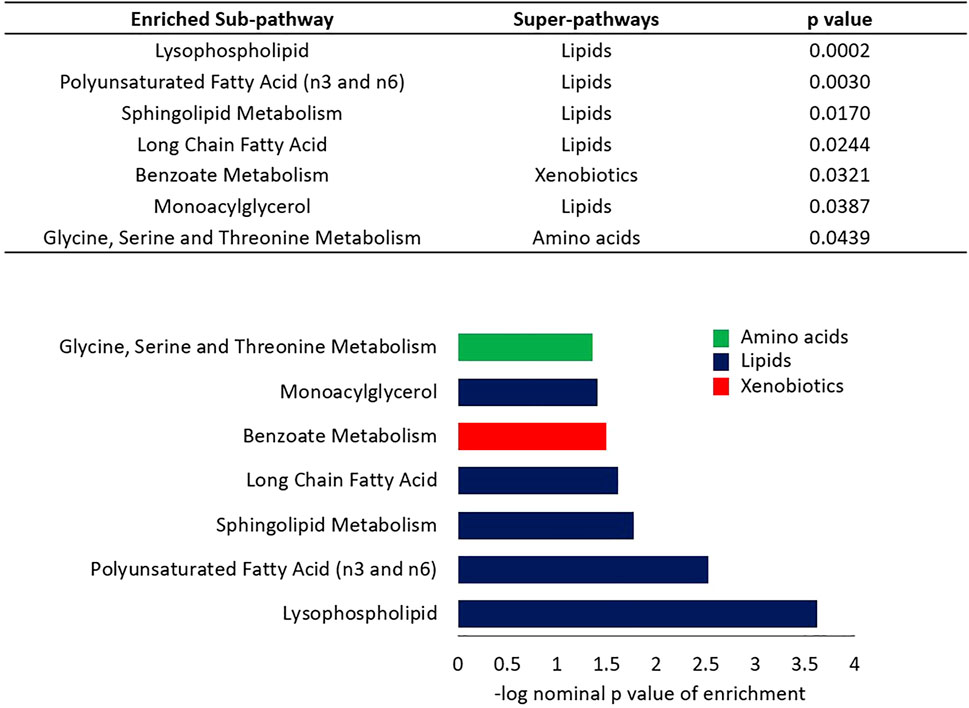
FIGURE 2. Enrichment analysis of sub-pathways based on regression nominal p value. Wilcoxon sum of the ranks test was used to assess the probability of the observed ranks of sub-pathway metabolites when ordered by nominal p values from the linear model analysis. Colors indicate the super-pathways of enriched sub-pathways.
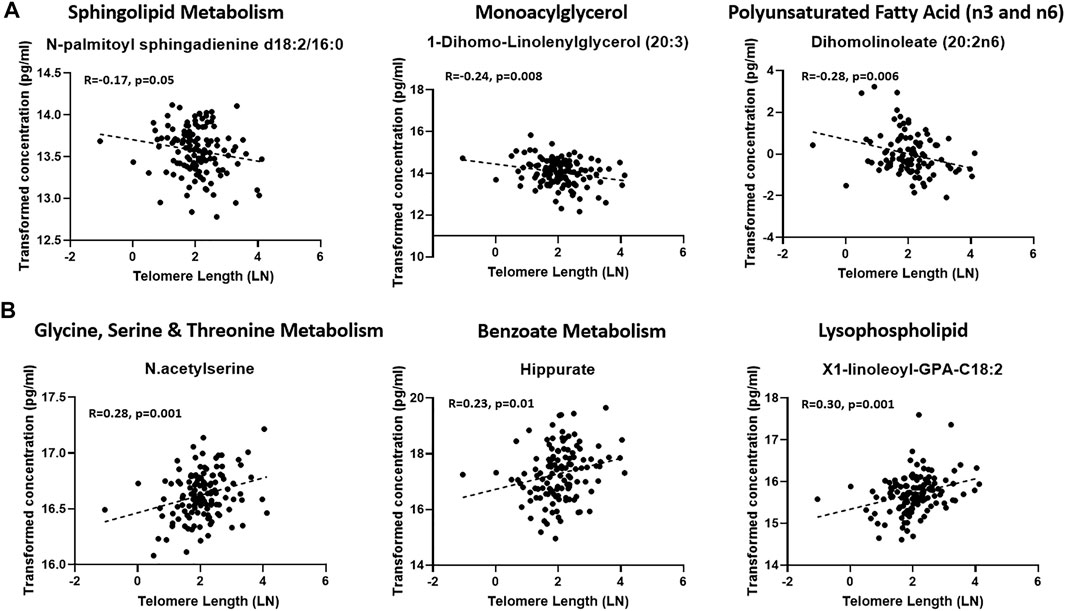
FIGURE 3. Scatter plots of representatives of enriched pathways that were reduced (A) or increased (B) with LTL.
Predictive Metabolites of LTL
A Generalized Linear Model featuring the mean-dichotomized LTL as the y-variable and top metabolites from the linear model as explanatory variables revealed a set of eight best predictive metabolites, including glutamine, N-acetylglutamine, xanthine, beta-sitosterol, N2-acetyllysine, stearoyl-arachidonoyl-glycerol (18:0/20:4), N-acetylserine and 3-7-dimethylurate. The area under curve (AUC) value from the ROC curve analysis was 0.75 (0.64–0.87, p < 0.0001) (Figure 4).
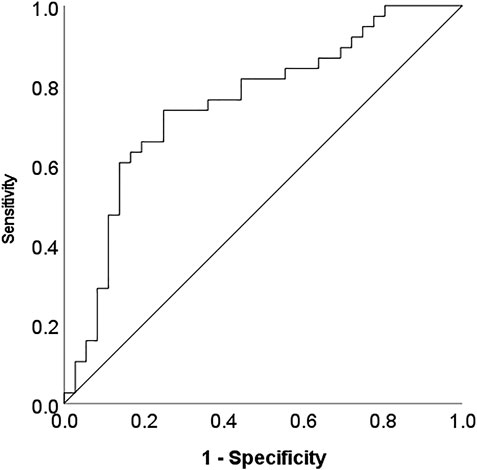
FIGURE 4. ROC curve analysis indicating the discriminatory power of a subset of metabolites differentiating short and long LTL (below/above mean respectively).
Discussion
Studies performed in human cell lines and various model organisms, including non-human primates, have revealed several longevity regulatory pathways involved in genome stability, energy metabolism and self-recognition (Riera et al., 2016; Campisi et al., 2019). Together, these pathways promote somatic maintenance via normal stem cell function and activation of autophagy, defense mechanisms against infectious agents, and survival pathways, while attenuating pro-inflammatory mediators, deregulated cell growth and senescence (Riera et al., 2016; Campisi et al., 2019). Previous studies have shown that exercise-mediated telomere preservation is associated with activation of specific metabolic pathways that could play a major role in disease prevention through exercise (Denham et al., 2016). However, several questions remain unanswered about how these longevity regulatory pathways are interconnected, or even hierarchical, to determine the telomere attrition through time and how they are related to regular practice of sport. In this study, the metabolic predictors of LTL were determined in 126 young elite male soccer players. Several metabolites and metabolic pathways were increased or decreased with LTL. Among these, top predictors of LTL were determined, providing a better understanding of exercise physiology and pathophysiology of elite athletes.
Changes in Various Lipids Are Associated With LTL
Whereas levels of lysophospholipids were elevated with longer LTL, other lipid species, including monoacylglycerols, long chain fatty acids, polyunsaturated fatty acid (n3 and n6) and sphingolipids, were reduced. Previous studies have shown that blood lysophosphatidylcholine levels tend to decrease with age (Johnson and Stolzing, 2019). Our data are in agreement with these findings as lower levels of lysophatidylcholines were associated with shorter LTL in elite soccer players. The blood profile of other phospholipids and sphingolipids was also shown to change with age, suggesting that blood lipids provide a rich source of biomarkers of human aging and potently regulators of aging and lifespan. Plasma lipidomics of 11 mammalian species ranging in longevity from 3.5 to 120 years has identified a predictive metabolic signature of the mammal’s lifespan, including long‐chain free fatty acids, lipid peroxidation index, and lipid peroxidation‐derived content, which were inversely correlated with longevity (Jové et al., 2013). These data confirm our findings as elevated levels of long chain fatty acids, polyunsaturated fatty acid (n3 and n6) and sphingolipids were associated with shorter telomeres. Further evidence linking lipid metabolism to aging came from animals with extreme longevity. The very long‐lived (over 500 years) quahog clam Arctica islandica was shown to have an exceptional resistance to lipid peroxidation in mitochondrial membranes (Munro and Blier, 2012). The lens membranes of the bowhead whale that can live longer than 200 years are highly enriched with phospholipids, providing resistance to the age‐related cataracts disease (Borchman et al., 2017). Hence, our emerging data confirm the important role of lipids in longevity and suggest the ability of lipid‐based interventions to modulate longevity in model organisms (Huang et al., 2014).
Changes in Benzoate and Glycine Metabolism Are Associated With Longer LTL
Our data showed elevation in betaine (dimethylglycine precursor), dimethylglycine (sarcosine precursor), sarcosine (glycine precursor), hippurate (glycine product) and 3-hydroxyhippurate (hippurate product), suggesting increased hippurate synthesis from glycine in athletes with longer LTL (Pallister et al., 2017; Tahir et al., 2019). Hippurate is a glycine conjugate of benzoate and is related to the gut microbiome and correlated to kidney function. Previous studies have identified higher hippurate levels in younger subjects (Lees et al., 2013; Adav and Wang, 2021), suggesting that elevated hippurate synthesis could be a biomarker of slower aging. The functional relevance of these metabolic changes requires further investigation.
Metabolic Predictors of LTL
Our data has also identified a metabolic classifier based on eight metabolites that can predict LTL in our cohort. Selected metabolites included four amino acids (glutamine, N-acetylglutamine, N-acetylserine, N2.acetyllysine), two lipids (stearoyl-arachidonoyl-glycerol (18:0/20:4), beta-sitosterol) and one nucleotide (xanthine). In this model, reduced glutamine levels were associated with longer LTL. Previous studies have identified that older subjects have higher glutamine compared to younger subjects (Kaiser et al., 2005), confirming our observation. The classifier also identified that elevated xanthine was associated with increased LTL, confirming a multi-omics study that showed xanthine as a pro-survival metabolite with aberrant mitochondrial function (Gioran et al., 2019). Similarly, our model also suggested that elevated beta-sitosterol is increased with longer LTL. Previous reports have shown that beta-sitosterol provides immunomodulatory, anti-microbial, anti-cancer, anti-inflammatory, lipid-lowering, hepato-protective, protective effect against respiratory diseases, wound-healing, anti-oxidant and anti-diabetic activities (Babu and Jayaraman, 2020). The association of the other metabolites included in the metabolic classifier of LTL was not reported before, therefore requires further confirmation in other cohorts.
Study Limitations
In this pilot study, the relatively small number of participants and the variation in sample collection, transportation and storage could have affected the results. Additionally, the limited available information of athletes’ anthropometric, physiological, and nutritional data during sampling as well as the resting time since their last exercise has hindered attempts to consider other important potential confounders such as body mass index and diet in our analysis. Furthermore, the use of young elite athletes without inclusion of non-athlete controls has limited the usefulness of the data, although the current study used a “within-subjects” design to test the effect of long-term soccer practice on LTL and metabolic profile without a control group.
Despite all these limitations, our novel data has revealed significant, although differences were only nominally significant, and pronounced alterations in metabolites with LTL in elite male soccer players. However, replication of data in other cohorts with controlled experimental design, including having non-elite athlete controls, is warranted to validate the emerging metabolites set as potential predictive biomarkers of exercise-associated reduced telomere attrition.
Conclusion
Our novel data suggest that elite soccer players exhibit a unique metabolic signature associated with LTL, including specific metabolites that can best predict LTL. The functional relevance of the emerging data is to highlight the importance of lipids, glutamine, xanthine, beta-sitosterol, among other metabolites, on longevity in response to chronic exercise, potentially through triggering a pro-survival, immunomodulatory and anti-oxidant effect. Confirming the predictive power of these metabolites and their functional relevance in different cohorts could help in understanding the aging process and the metabolic pathways underlying exercise-associated reduced telomere attrition.
Data Availability Statement
The datasets presented in this study can be found in online repositories. The names of the repository/repositories and accession number(s) can be found below: https://www.ebi.ac.uk/metabolights/, MTBLS2976.
Ethics Statement
The studies involving human participants were reviewed and approved by the Institutional Research Board of Qatar University. The patients/participants provided their written informed consent to participate in this study.
Author Contributions
SA-M carried out the experimental part and wrote the paper. MS helped with study design, data analysis and writing the manuscript. ID, AD, NR, and AA helped with data analysis and revised the manuscript critically for important intellectual content. FA-K helped with experimental part. FD and FB were involved in study design, sample collection and data analysis. ME was lead principal investigator, designed the experiments, supervised progress, analyzed data and reviewed and approved the final version of the article. ME is responsible for the integrity of the work as a whole. All authors have read and approved the manuscript.
Funding
This research was sponsored by award no. UREP 26-043-3-018 (ME, MS) from Qatar National Research Fund (a member of Qatar Foundation). The statements made herein are solely the responsibility of the authors.
Conflict of Interest
The authors declare that the research was conducted in the absence of any commercial or financial relationships that could be construed as a potential conflict of interest.
Publisher’s Note
All claims expressed in this article are solely those of the authors and do not necessarily represent those of their affiliated organizations, or those of the publisher, the editors and the reviewers. Any product that may be evaluated in this article, or claim that may be made by its manufacturer, is not guaranteed or endorsed by the publisher.
Supplementary Material
The Supplementary Material for this article can be found online at: https://www.frontiersin.org/articles/10.3389/fmolb.2021.727144/full#supplementary-material
References
Abdallah, P., Luciano, P., Runge, K. W., Lisby, M., Géli, V., Gilson, E., et al. (2009). A Two-step Model for Senescence Triggered by a Single Critically Short Telomere. Nat. Cel Biol. 11, 988–993. doi:10.1038/ncb1911
Adav, S. S., and Wang, Y. (2021). Metabolomics Signatures of Aging: Recent Advances. Aging Dis. 12, 646–661. doi:10.14336/ad.2020.0909
Al-Khelaifi, F., Diboun, I., Donati, F., Botrè, F., Alsayrafi, M., Georgakopoulos, C., et al. (2018). Metabolomics Profiling of Xenobiotics in Elite Athletes: Relevance to Supplement Consumption. J. Int. Soc. Sports Nutr. 15, 48. doi:10.1186/s12970-018-0254-7
Al-Khelaifi, F., Donati, F., Botrè, F., Latiff, A., Abraham, D., Hingorani, A., et al. (2019). Metabolic Profiling of Elite Athletes with Different Cardiovascular Demand. Scand. J. Med. Sci. Sports 29, 933–943. doi:10.1111/sms.13425
Al-Sulaiti, H., Diboun, I., Agha, M. V., Mohamed, F. F. S., Atkin, S., Dömling, A. S., et al. (2019). Metabolic Signature of Obesity-Associated Insulin Resistance and Type 2 Diabetes. J. Transl Med. 17, 348. doi:10.1186/s12967-019-2096-8
Arsenis, N. C., You, T., Ogawa, E. F., Tinsley, G. M., and Zuo, L. (2017). Physical Activity and Telomere Length: Impact of Aging and Potential Mechanisms of Action. Oncotarget 8, 45008–45019. doi:10.18632/oncotarget.16726
Babu, S., and Jayaraman, S. (2020). An Update on β-sitosterol: A Potential Herbal Nutraceutical for Diabetic Management. Biomed. Pharmacother. 131, 110702. doi:10.1016/j.biopha.2020.110702
Bakaysa, S. L., Mucci, L. A., Slagboom, P. E., Boomsma, D. I., McClearn, G. E., Johansson, B., et al. (2007). Telomere Length Predicts Survival Independent of Genetic Influences. Aging Cel. 6, 769–774. doi:10.1111/j.1474-9726.2007.00340.x
Balan, E., Decottignies, A., and Deldicque, L. (2018). Physical Activity and Nutrition: Two Promising Strategies for Telomere Maintenance? Nutrients 10, 1942. doi:10.3390/nu10121942
Bischoff, C., Petersen, H. C., Graakjaer, J., Andersen-Ranberg, K., Vaupel, J. W., Bohr, V. A., et al. (2006). No Association between Telomere Length and Survival Among the Elderly and Oldest Old. Epidemiology 17, 190–194. doi:10.1097/01.ede.0000199436.55248.10
Blackburn, E. H., and Gall, J. G. (1978). A Tandemly Repeated Sequence at the Termini of the Extrachromosomal Ribosomal RNA Genes in Tetrahymena. J. Mol. Biol. 120, 33–53. doi:10.1016/0022-2836(78)90294-2
Blackburn, E. H., Greider, C. W., and Szostak, J. W. (2006). Telomeres and Telomerase: the Path from maize, Tetrahymena and Yeast to Human Cancer and Aging. Nat. Med. 12, 1133–1138. doi:10.1038/nm1006-1133
Blackburn, E. H. (2001). Switching and Signaling at the Telomere. Cell 106, 661–673. doi:10.1016/s0092-8674(01)00492-5
Borchman, D., Stimmelmayr, R., and George, J. C. (2017). Whales, Lifespan, Phospholipids, and Cataracts. J. Lipid Res. 58, 2289–2298. doi:10.1194/jlr.m079368
Broer, L., Codd, V., Nyholt, D. R., Deelen, J., Mangino, M., Willemsen, G., et al. (2013). Meta-analysis of Telomere Length in 19 713 Subjects Reveals High Heritability, Stronger Maternal Inheritance and a Paternal Age Effect. Eur. J. Hum. Genet. 21, 1163–1168. doi:10.1038/ejhg.2012.303
Brouilette, S., Singh, R. K., Thompson, J. R., Goodall, A. H., and Samani, N. J. (2003). White Cell Telomere Length and Risk of Premature Myocardial Infarction. Atvb 23, 842–846. doi:10.1161/01.atv.0000067426.96344.32
Brouilette, S. W., Moore, J. S., McMahon, A. D., Thompson, J. R., Ford, I., Shepherd, J., et al. (2007). Telomere Length, Risk of Coronary Heart Disease, and Statin Treatment in the West of Scotland Primary Prevention Study: a Nested Case-Control Study. Lancet 369, 107–114. doi:10.1016/s0140-6736(07)60071-3
Campisi, J., and d'Adda di Fagagna, F. (2007). Cellular Senescence: when Bad Things Happen to Good Cells. Nat. Rev. Mol. Cel Biol. 8, 729–740. doi:10.1038/nrm2233
Campisi, J., Kapahi, P., Lithgow, G. J., Melov, S., Newman, J. C., and Verdin, E. (2019). From Discoveries in Ageing Research to Therapeutics for Healthy Ageing. Nature 571, 183–192. doi:10.1038/s41586-019-1365-2
Cao, M., Zhao, L., Chen, H., Xue, W., and Lin, D. (2012). NMR-based Metabolomic Analysis of Human Bladder Cancer. Anal. Sci. 28, 451–456. doi:10.2116/analsci.28.451
Cawthon, R. M., Smith, K. R., O'Brien, E., Sivatchenko, A., and Kerber, R. A. (2003). Association between Telomere Length in Blood and Mortality in People Aged 60 Years or Older. Lancet 361, 393–395. doi:10.1016/s0140-6736(03)12384-7
Cheng, S., Larson, M. G., McCabe, E. L., Murabito, J. M., Rhee, E. P., Ho, J. E., et al. (2015). Distinct Metabolomic Signatures Are Associated with Longevity in Humans. Nat. Commun. 6, 6791. doi:10.1038/ncomms7791
Deelen, J., Beekman, M., Codd, V., Trompet, S., Broer, L., Hägg, S., et al. (2014). Leukocyte Telomere Length Associates with Prospective Mortality Independent of Immune-Related Parameters and Known Genetic Markers. Int. J. Epidemiol. 43, 878–886. doi:10.1093/ije/dyt267
DeHaven, C. D., Evans, J. M., Dai, H., and Lawton, K. A. (2012). “Software Techniques for Enabling High-Throughput Analysis of Metabolomic Datasets,” in Metabolomics. Editor Dr. U. Roessner (InTech Chapter), 7. doi:10.5772/31277
Denham, J., O’Brien, B. J., and Charchar, F. J. (2016). Telomere Length Maintenance and Cardio-Metabolic Disease Prevention through Exercise Training. Sports Med. 46, 1213–1237. doi:10.1007/s40279-016-0482-4
Ehrlenbach, S., Willeit, P., Kiechl, S., Willeit, J., Reindl, M., Schanda, K., et al. (2009). Influences on the Reduction of Relative Telomere Length over 10 Years in the Population-Based Bruneck Study: Introduction of a Well-Controlled High-Throughput Assay. Int. J. Epidemiol. 38, 1725–1734. doi:10.1093/ije/dyp273
Evans, A. M., Bridgewater, B. R., Liu, Q., Mitchell, M. W., Robinson, R. J., Dai, H., et al. (2014). High Resolution Mass Spectrometry Improves Data Quantity and Quality as Compared to Unit Mass Resolution Mass Spectrometry in High- Throughput Profiling Metabolomics. Metabolomics 2014 (4), 2. doi:10.4172/2153-0769.1000132
Fitzpatrick, A. L., Kronmal, R. A., Gardner, J. P., Psaty, B. M., Jenny, N. S., Tracy, R. P., et al. (2007). Leukocyte Telomere Length and Cardiovascular Disease in the Cardiovascular Health Study. Am. J. Epidemiol. 165, 14–21. doi:10.1093/aje/kwj346
Fitzpatrick, A. L., Kronmal, R. A., Kimura, M., Gardner, J. P., Psaty, B. M., Jenny, N. S., et al. (2011). Leukocyte Telomere Length and Mortality in the Cardiovascular Health Study. Journals Gerontol. Ser. A: Biol. Sci. Med. Sci. 66A, 421–429. doi:10.1093/gerona/glq224
Floegel, A., Stefan, N., Yu, Z., Muhlenbruch, K., Drogan, D., Joost, H.-G., et al. (2013). Identification of Serum Metabolites Associated with Risk of Type 2 Diabetes Using a Targeted Metabolomic Approach. Diabetes 62, 639–648. doi:10.2337/db12-0495
Gioran, A., Piazzesi, A., Bertan, F., Schroer, J., Wischhof, L., Nicotera, P., et al. (2019). Multi-Omics Identify Xanthine as a Pro-survival Metabolite for Nematodes with Mitochondrial Dysfunction. EMBO J. 38, e99558. doi:10.15252/embj.201899558
Gonzalez-Covarrubias, V., Beekman, M., Uh, H. W., Dane, A., Troost, J., Paliukhovich, I., et al. (2013). Lipidomics of Familial Longevity. Aging Cell 12, 426–434. doi:10.1111/acel.12064
Harris, S. E., Deary, I. J., MacIntyre, A., Lamb, K. J., Radhakrishnan, K., Starr, J. M., et al. (2006). The Association between Telomere Length, Physical Health, Cognitive Ageing, and Mortality in Non-demented Older People. Neurosci. Lett. 406, 260–264. doi:10.1016/j.neulet.2006.07.055
Haycock, P. C., Heydon, E. E., Kaptoge, S., Butterworth, A. S., Thompson, A., and Willeit, P. (2014). Leucocyte Telomere Length and Risk of Cardiovascular Disease: Systematic Review and Meta-Analysis. BMJ 349, g4227. doi:10.1136/bmj.g4227
Honig, L. S., Kang, M. S., Schupf, N., Lee, J. H., and Mayeux, R. (2012). Association of Shorter Leukocyte Telomere Repeat Length with Dementia and Mortality. Arch. Neurol. 69, 1332–1339. doi:10.1001/archneurol.2012.1541
Houben, J. M. J., Giltay, E. J., Rius-Ottenheim, N., Hageman, G. J., and Kromhout, D. (2011). Telomere Length and Mortality in Elderly Men: the Zutphen Elderly Study. Journals Gerontol. Ser. A: Biol. Sci. Med. Sci. 66A, 38–44. doi:10.1093/gerona/glq164
Huang, X., Withers, B. R., and Dickson, R. C. (2014). Sphingolipids and Lifespan Regulation. Biochim. Biophys. Acta (Bba) - Mol. Cel Biol. Lipids 1841, 657–664. doi:10.1016/j.bbalip.2013.08.006
Ilhame, D., Layla, A.-M., Hend, A.-J., Omar, A., and Mohamed, E. (2020). Metabolomics of Lean/Overweight Insulin Resistant Females Reveals Alterations in Steroids and Fatty Acids: Supplementary Tables S1-S3. Figshare. Dataset. doi:10.6084/m9.figshare.12857456.v1
Jeanclos, E., Krolewski, A., Skurnick, J., Kimura, M., Aviv, H., Warram, J. H., et al. (1998). Shortened Telomere Length in white Blood Cells of Patients with IDDM. Diabetes 47, 482–486. doi:10.2337/diabetes.47.3.482
Johnson, A. A., and Stolzing, A. (2019). The Role of Lipid Metabolism in Aging, Lifespan Regulation, and Age-Related Disease. Aging Cell 18, e13048. doi:10.1111/acel.13048
Jové, M., Naudí, A., Aledo, J. C., Cabré, R., Ayala, V., Portero-Otin, M., et al. (2013). Plasma Long-Chain Free Fatty Acids Predict Mammalian Longevity. Sci. Rep. 3, 3346. doi:10.1038/srep03346
Kaiser, L. G., Schuff, N., Cashdollar, N., and Weiner, M. W. (2005). Age-related Glutamate and Glutamine Concentration Changes in normal Human Brain: 1H MR Spectroscopy Study at 4 T. Neurobiol. Aging 26, 665–672. doi:10.1016/j.neurobiolaging.2004.07.001
Kimura, M., Hjelmborg, J. v. B., Gardner, J. P., Bathum, L., Brimacombe, M., Lu, X., et al. (2008). Telomere Length and Mortality: a Study of Leukocytes in Elderly Danish Twins. Am. J. Epidemiol. 167, 799–806. doi:10.1093/aje/kwm380
Kong, S. W., and Hernandez-Ferrer, C. (2020). Assessment of Coverage for Endogenous Metabolites and Exogenous Chemical Compounds Using an Untargeted Metabolomics Platform. Pac. Symp. Biocomput. 25, 587–598. doi:10.1142/9789811215636_0052
Lees, H. J., Swann, J. R., Wilson, I. D., Nicholson, J. K., and Holmes, E. (2013). Hippurate: the Natural History of a Mammalian-Microbial Cometabolite. J. Proteome Res. 12, 1527–1546. doi:10.1021/pr300900b
Lindsey, J., McGill, N. I., Lindsey, L. A., Green, D. K., and Cooke, H. J. (1991). In Vivo loss of Telomeric Repeats with Age in Humans. Mutat. Research/DNAging 256, 45–48. doi:10.1016/0921-8734(91)90032-7
Liu, J., Semiz, S., van der Lee, S. J., van der Spek, A., Verhoeven, A., van Klinken, J. B., et al. (2017). Metabolomics Based Markers Predict Type 2 Diabetes in a 14-year Follow-Up Study. Metabolomics 13, 104. doi:10.1007/s11306-017-1239-2
Marioni, R. E., Harris, S. E., Shah, S., McRae, A. F., von Zglinicki, T., Martin-Ruiz, C., et al. (2018). The Epigenetic Clock and Telomere Length Are Independently Associated with Chronological Age and Mortality. Int. J. Epidemiol. 47, 356. doi:10.1093/ije/dyx233
Martin-Ruiz, C. M., Gussekloo, J., Heemst, D., Zglinicki, T., and Westendorp, R. G. J. (2005). Telomere Length in white Blood Cells Is Not Associated with Morbidity or Mortality in the Oldest Old: a Population-Based Study. Aging Cell 4, 287–290. doi:10.1111/j.1474-9726.2005.00171.x
Martin-Ruiz, C., Dickinson, H. O., Keys, B., Rowan, E., Kenny, R. A., and Von Zglinicki, T. (2006). Telomere Length Predicts Poststroke Mortality, Dementia, and Cognitive Decline. Ann. Neurol. 60, 174–180. doi:10.1002/ana.20869
Matsumoto, T., Kobayashi, T., and Kamata, K. (2007). Role of Lysophosphatidylcholine (LPC) in Atherosclerosis. Cmc 14, 3209–3220. doi:10.2174/092986707782793899
Maubaret, C. G., Salpea, K. D., Salpea, K. D., Jain, A., Cooper, J. A., Hamsten, A., et al. (2010). Telomeres Are Shorter in Myocardial Infarction Patients Compared to Healthy Subjects: Correlation with Environmental Risk Factors. J. Mol. Med. 88, 785–794. doi:10.1007/s00109-010-0624-3
Menni, C., Kastenmüller, G., Petersen, A. K., Bell, J. T., Psatha, M., Tsai, P.-C., et al. (2013). Metabolomic Markers Reveal Novel Pathways of Ageing and Early Development in Human Populations. Int. J. Epidemiol. 42, 1111–1119. doi:10.1093/ije/dyt094
Mohamed, E., Ilhame, D., Manjunath, R., Yasser, M., Lina, A., Mohammed, B., et al. (2020). Metabolic Profiling of Pre-gestational and Gestational Diabetes Mellitus Identifies Novel Predictors of Pre-term Labor: Supplementary Tables S1-S5. Figshare. Dataset.
Montoliu, I., Scherer, M., Beguelin, F., DaSilva, L., Mari, D., Salvioli, S., et al. (2014). Serum Profiling of Healthy Aging Identifies Phospho- and Sphingolipid Species as Markers of Human Longevity. Aging 6, 9–25. doi:10.18632/aging.100630
Montrose, D. C., Zhou, X. K., Kopelovich, L., Yantiss, R. K., Karoly, E. D., Subbaramaiah, K., et al. (2012). Metabolic Profiling, a Noninvasive Approach for the Detection of Experimental Colorectal Neoplasia. Cancer Prev. Res. 5, 1358–1367. doi:10.1158/1940-6207.capr-12-0160
Munro, D., and Blier, P. U. (2012). The Extreme Longevity ofArctica Islandicais Associated with Increased Peroxidation Resistance in Mitochondrial Membranes. Aging Cell 11, 845–855. doi:10.1111/j.1474-9726.2012.00847.x
Njajou, O. T., Hsueh, W.-C., Blackburn, E. H., Newman, A. B., Wu, S.-H., Li, R., et al. A.B.C.s. Health (2009). Association between Telomere Length, Specific Causes of Death, and Years of Healthy Life in Health, Aging, and Body Composition, a Population-Based Cohort Study. J. Gerontol. A. Biol. Sci. Med. Sci. 64A, 860–864. doi:10.1093/gerona/glp061
Oresic, M., Hyotylainen, T., Herukka, S. K., Sysi-Aho, M., Mattila, I., Seppanan-Laakso, T., et al. (2011). Metabolome in Progression to Alzheimer's Disease. Transl Psychiatry 1, e57. doi:10.1038/tp.2011.55
Pallister, T., Jackson, M. A., Martin, T. C., Zierer, J., Jennings, A., Mohney, R. P., et al. (2017). Hippurate as a Metabolomic Marker of Gut Microbiome Diversity: Modulation by Diet and Relationship to Metabolic Syndrome. Sci. Rep. 7, 13670. doi:10.1038/s41598-017-13722-4
Rehkopf, D. H., Needham, B. L., Lin, J., Blackburn, E. H., Zota, A. R., Wojcicki, J. M., et al. (2016). Leukocyte Telomere Length in Relation to 17 Biomarkers of Cardiovascular Disease Risk: A Cross-Sectional Study of US Adults. Plos Med. 13, e1002188. doi:10.1371/journal.pmed.1002188
Riera, C. E., Merkwirth, C., De Magalhaes Filho, C. D., and Dillin, A. (2016). Signaling Networks Determining Life Span. Annu. Rev. Biochem. 85, 35–64. doi:10.1146/annurev-biochem-060815-014451
Rode, L., Nordestgaard, B. G., and Bojesen, S. E. (2015). Peripheral Blood Leukocyte Telomere Length and Mortality Among 64,637 Individuals from the General Population. J. Natl. Cancer Inst. 107, djv074. doi:10.1093/jnci/djv074
Shammas, M. A. (2011). Telomeres, Lifestyle, Cancer, and Aging. Curr. Opin. Clin. Nutr. Metab. Care 14, 28–34. doi:10.1097/mco.0b013e32834121b1
Slagboom, P. E., Droog, S., and Boomsma, D. I. (1994). Genetic Determination of Telomere Size in Humans: a Twin Study of Three Age Groups. Am. J. Hum. Genet. 55, 876–882.
Strandberg, T. E., Saijonmaa, O., Tilvis, R. S., Pitkala, K. H., Strandberg, A. Y., Miettinen, T. A., et al. (2011). Association of Telomere Length in Older Men with Mortality and Midlife Body Mass index and Smoking. Journals Gerontol. Ser. A: Biol. Sci. Med. Sci. 66A, 815–820. doi:10.1093/gerona/glr064
Suhre, K., Meisinger, C., Döring, A., Altmaier, E., Belcredi, P., Gieger, C., et al. (2010). Metabolic Footprint of Diabetes: a Multiplatform Metabolomics Study in an Epidemiological Setting. PLoS One 5, e13953. doi:10.1371/journal.pone.0013953
Tahir, T., Ashfaq, M., Asghar, H., Shahzad, M. I., Tabassum, R., and Ashfaq, A. (2019). Medicinal Importance of Azo and Hippuric Acid Derivatives. Mrmc 19, 708–719. doi:10.2174/1389557518666180727162018
Wang-Sattler, R., Yu, Z., Herder, C., Messias, A. C., Floegel, A., He, Y., et al. (2012). Novel Biomarkers for Pre-diabetes Identified by Metabolomics. Mol. Syst. Biol. 8, 615. doi:10.1038/msb.2012.43
Yu, Z., Zhai, G., Singmann, P., He, Y., Xu, T., Prehn, C., et al. (2012). Human Serum Metabolic Profiles Are Age Dependent. Aging Cell 11, 960–967. doi:10.1111/j.1474-9726.2012.00865.x
Zhao, J., Miao, K., Wang, H., Ding, H., and Wang, D. W. (2013). Association between Telomere Length and Type 2 Diabetes Mellitus: a Meta-Analysis. PLoS One 8, e79993. doi:10.1371/journal.pone.0079993
Zhao, J., Zhu, Y., Uppal, K., Tran, V. T., Yu, T., Lin, J., et al. (2014). Metabolic Profiles of Biological Aging in American Indians: the Strong Heart Family Study. Aging 6, 176–186. doi:10.18632/aging.100644
Keywords: telomere length, elite athletes, soccer, aging, metabolomics
Citation: Al-Muraikhy S, Sellami M, Domling AS, Rizwana N, Agouni A, Al-Khelaifi F, Donati F, Botre F, Diboun I and Elrayess MA (2021) Metabolic Signature of Leukocyte Telomere Length in Elite Male Soccer Players. Front. Mol. Biosci. 8:727144. doi: 10.3389/fmolb.2021.727144
Received: 18 June 2021; Accepted: 02 December 2021;
Published: 16 December 2021.
Edited by:
Luciana Hannibal, University of Freiburg Medical Center, GermanyReviewed by:
Maheshwor Thapa, Jackson Laboratory for Genomic Medicine, United StatesKayvan Khoramipour, University of Tehran, Iran
Copyright © 2021 Al-Muraikhy, Sellami, Domling, Rizwana, Agouni, Al-Khelaifi, Donati, Botre, Diboun and Elrayess. This is an open-access article distributed under the terms of the Creative Commons Attribution License (CC BY). The use, distribution or reproduction in other forums is permitted, provided the original author(s) and the copyright owner(s) are credited and that the original publication in this journal is cited, in accordance with accepted academic practice. No use, distribution or reproduction is permitted which does not comply with these terms.
*Correspondence: Ilhame Diboun, idiboun@hbku.edu.qa; Mohamed A Elrayess, m.elrayess@qu.edu.qa
†These authors have contributed equally to this work