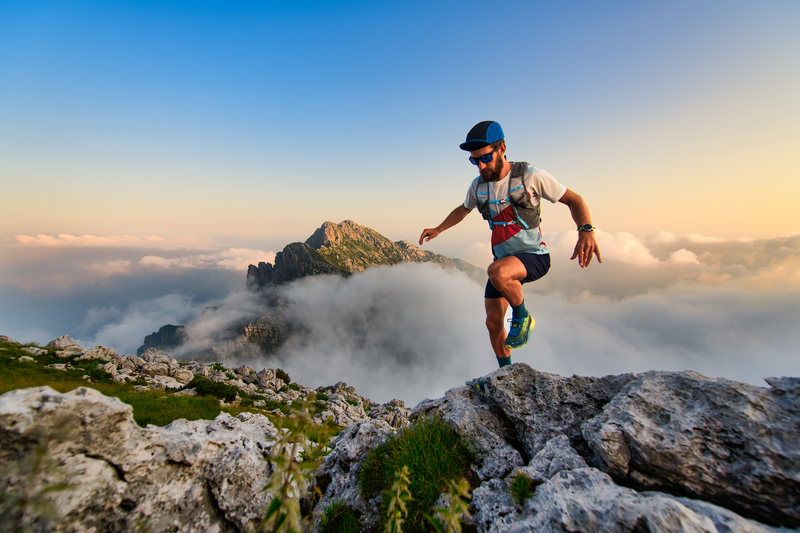
95% of researchers rate our articles as excellent or good
Learn more about the work of our research integrity team to safeguard the quality of each article we publish.
Find out more
ORIGINAL RESEARCH article
Front. Mol. Biosci. , 18 October 2021
Sec. Molecular Diagnostics and Therapeutics
Volume 8 - 2021 | https://doi.org/10.3389/fmolb.2021.720645
This article is part of the Research Topic Molecular Biomarkers for the Diagnosis, Prognosis, and Risk Prediction of Cancer View all 88 articles
Background: ARHGAP11A, belongs to RhoGAPs family, is vital for cell motility. However, the role of ARHGAP11A in gastric cancer is obscure.
Methods: The expression level of ARHGAP11A was analyzed by Oncomine database. The correlation of ARHGAP11A expression with immune infiltrates and associated gene markers was clarified by Tumor IMmune Estimation Resource and Gene Expression Profiling Interactive Analysis database. The correlation between ARHGAP11A expression and the patient prognosis was identified by Kaplan-Meier plotter and PrognoScan. Genetic changes of ARHGAP11A were analyzed by cBioPortal. The protein-protein interaction network and gene functional enrichment analysis were constructed and performed by GeneMANIA and Metascape.
Results: We found that the expression levels of ARHGAP11A were elevated in various cancers including gastric cancer when compared with normal tissues. High expression of ARHGAP11A was significantly correlated with a better prognosis in gastric cancer. We revealed that the expression of ARHGAP11A was negatively associated with infiltration levels of CD8+ T cells, CD4+ T cells, macrophages and dendritic cells. In addition, ARHGAP11A expression was significantly correlated with gene markers of these immune cells. Lastly, gene functional enrichment analysis indicated that ARHGAP11A involved in regulating lymphocyte activation, cell division, cell killing, myeloid leukocyte differentiation and leukocyte apoptosis.
Conclusion: Our findings demonstrated that ARHGAP11A was a valuable prognostic biomarker in gastric cancer. Further work is needed to validate its role and underlying mechanisms in regulating immune infiltrates.
Gastric cancer is a common cancer, especially in Asian countries (Sun et al., 2020; Yang et al., 2020; Cao et al., 2021). It is the fourth leading cause of cancer-related mortality worldwide (Sung et al., 2021). In recent years, the immunotherapy has bought revolutionary changes to the treatment of cancer (Ribas and Wolchok, 2018). However, the progress of immunotherapy in gastric cancer was hampered by a limited understanding of the immune microenvironment (Balkwill et al., 2012). The tumor immune microenvironment is crucial for tumor initiation and progression (Salmon et al., 2019). It is composed of all populations of tumor infiltrating cells including macrophages, T cells and dendritic cells (Bruni et al., 2020). Studies have shown that the tumor immune infiltrates correlated with prognosis and response to therapy (Zeng et al., 2019; Refolo et al., 2020). Therefore, there is a clear need to investigate the immune infiltrates related markers and to reveal the molecular mechanisms in gastric cancer.
Rho GTPases are a subfamily of the Ras superfamily proteins which play central roles in multiple biological processes, such as cell motility, cell polarity, cell cycle progression, cell adhesion, migration and invasion. Rho GTPase-activating proteins (RhoGAPs), upstream regulators of Rho GTPases, are frequently dysregulated in various cancers (Porter et al., 2016; Muller et al., 2020). Previous studies showed that Rho GTPases played a role in immune homeostasis, which involved in key processes for the T lymphocytes activation and differentiation (Saoudi et al., 2014). Tumors with abundant tumor-infiltrating lymphocytes (TILs) are associated with a better prognosis than tumors with scarce TILs in various cancers (Brambilla et al., 2016; Sudo et al., 2017). Low-grade TILs are associated with lymph node metastasis of early-stage cancer cells (Zhao et al., 2020). Whether RhoGAPs involve in the tumor immune microenvironment is still unknown. ARHGAP11A, a protein coding gene locates on chr15q13.3, encodes a member of RhoGAPs (ARHGAP11A). The role of ARHGAP11A in cancer is still controversial. In human glioma cells, ARHGAP11A binds to p53 and promotes its function eventually leading to cell-cycle arrest and apoptosis (Xu et al., 2013). ARHGAP11A is upregulated in liver cancer and proceeds the liver cell proliferation and migration via Rac1B (Dai et al., 2018). ARHGAP11A was found involved in the cell migration of breast cancer (Lawson et al., 2016). In our ongoing parallel study, using whole exon and whole genome sequencing, we characterized multiple metastases arising from gastric cancer in twelve patients. We found that high expression of ARHGAP11A, a representative gene with mutation characteristics in the clonal evolution of gastric cancer metastasis, appeared more frequently in gastric cancer with lymph node metastasis (unpublished data). ARHGAP11A might play a key role in lymph node metastasis of gastric cancer. Nevertheless, the prognostic significance of ARHGAP11A and its correlation with immune infiltrates including TILs in gastric cancer is obscure.
In this study, we analyzed the correlation between ARHGAP11A expression and patient prognosis using PrognoScan and Kaplan-Meier plotter. We next investigated the correlation of ARHGAP11A expression and tumor infiltrates in Tumor IMmune Estimation Resource (TIMER) and Gene Expression Profiling Interactive Analysis (GEPIA).
Oncomine (https://www.oncomine.org/resource/login.html) is a publicly available tumor microarray database and data mining platform that includes 715 datasets and 86,733 samples (includes tumor and normal tissue samples). Gene expression analyses for a single gene can be performed across various types of cancer and include comparisons relative to normal control (Rhodes et al., 2007). The expression of ARHGAP11A in different cancer tissues were analyzed by using Oncomine. The threshold was set as: p < 1.0E−04, fold change >2, gene rank: top 10% and data type: mRNA. One dataset (Cui Gastric Dataset: 80 gastric carcinoma and 80 normal paired gastric tissue samples were analyzed (Cui et al., 2011)) met the threshold when compared the ARHGAP11A expression in gastric cancer and normal tissue.
TIMER is a public resource for systematic analysis of immune infiltrates across 32 cancer types (10,897 tumor samples) from The Cancer Genome Atlas (TCGA) (https://cistrome.shinyapps.io/timer/) (Li et al., 2017). The DiffExp module of TIMER was used to identify the expression of ARHGAP11A in all TCGA tumors. Gene expression levels were displayed using box plots, with statistical significance of differential expression level of ARHGAP11A evaluated using the Wilcoxon test and marked with asterisk. The Gene module of TIMER database was used to clarify the correlation of ARHGAP11A expression with immune infiltration level, in which the scatterplots was generated and displayed, showed the purity-corrected partial Spearman’s rho value and statistical significance. The Survival module was used to explore the survival differences of patients with different immune infiltrates. The Correlation module of TIMER database was used to draw the expression scatterplots between ARHGAP11A and immune related markers together with the Spearman’s rho value and estimated statistical significance (Correlation adjusted by tumor purity). GEPIA (http://gepia.cancer-pku.cn/index.html) is an online database that includes 9,736 tumors and 8,587 normal samples from TCGA and the GTEx projects (Tang et al., 2017). It was used to further validate the significantly correlated genes in TIMER. Gene expression correlation analysis was performed for given sets of TCGA expression data. The correlation coefficient was determined by the Spearman method.
The Kaplan Meier plotter (https://kmplot.com/analysis/) is an online database capable to assess the effect of 54,675 genes (mRNA, miRNA, protein) on survival in 21 cancer types including gastric cancer (1,440 samples) (Szasz et al., 2016). Sources for the databases include GEO, EGA, and TCGA. It was used to analyze the relationship of ARHGAP11A expression with Overall Survival (OS) and Relapse Free Survival (RFS) in various cancer types, and hazard ratio (HR) values with 95% confidence intervals and log-rank p-values were calculated. Adjusted analyses were performed by using data from gastric cancer patients with different clinical parameters such as gender, tumor stage, Lauren classification, differentiation, treatment and HER2 status. PrognoScan (http://dna00.bio.kyutech.ac.jp/PrognoScan/index.html) provides a powerful platform to explore the relationships between gene expression and patient prognosis across a large collection of publicly available cancer microarray datasets (Mizuno et al., 2009). The threshold was set as: Corrected p-value and Cox p-value both <0.05. The impact of both ARHGAP11A expression level and clinical parameters was analyzed.
The cBio Cancer Genomics Portal (https://cbioportal.org) has multidimensional cancer genomics datasets (Gao et al., 2013). Data from 1,120 patients (TCGA datasets) was selected to analyze genetic changes of gastric cancer by using cBioPortal. Genetic alterations were shown in different colors.
GeneMANIA (http://genemania.org/) is an online tool uses bioinformatic methods to display a list of interacting genes, including gene co-expression, physical interactions, gene co-localization, gene enrichment analysis and website prediction. It always be used to construct a PPI network and analyze the function of interactive genes (Warde-Farley et al., 2010). Metascape (https://metascape.org/gp/index.html) is a gene function annotation website (Zhou et al., 2019). It integrates multiple authoritative data resources such as Gene Ontology (GO), Kyoto Encyclopedia of Genes and Genomes (KEGG) pathway, UniProt and DrugBank to complete thorough pathway enrichment and biological process annotation. A PPI network which contained genes interacting with ARHGAP11A was constructed by using GeneMANIA. Genes identified by the GeneMANIA PPI network and TIMER analysis were included in the GO function analyses by using Metascape.
Patient survival plots generated from the TIMER, GEPIA, PrognoScan and Kaplan-Meier plotter were displayed with HR, p or Cox p-value from a log-rank test. p < 0.05 was considered statistically significant.
We firstly analyzed the expression of ARHGAP11A in different tissues by using Oncomine. We revealed that expression levels of ARHGAP11A were elevated in breast, cervical, colorectal, gastric, ovarian cancers, lymphoma and sarcoma relative to normal tissues. In contrast, the ARHGAP11A expression was lower in kidney cancer when compared with normal kidney tissue (Figure 1A). Details were shown in Supplementary Table S1. Next, we assessed the transcriptional levels of ARHGAP11A by using RNA-sequencing data in TCGA and TIMER. Results showed significant differences in ARHGAP11A expression levels when compared tumor and normal tissues (Figure 1B). For example, the transcriptional expression of ARHGAP11A was significantly elevated relative to normal tissues in esophageal carcinoma (ESCA) and stomach adenocarcinoma (STAD). Moreover, the transcriptional expression level of ARHGAP11A in metastatic lesion of skin cutaneous melanoma (SKCM. Metastasis) was significantly higher than in the primary lesion of skin cutaneous melanoma (SKCM. Tumor). In consistent with high transcription level of ARHGAP11A in gastric cancer tissues shown in Figure 1; Cytoplasmic expression of ARHGAP11A was also detected in most cancers including gastric cancer. As shown in Supplementary Figure S1 7 of 9 gastric cancer patients show high/median expression (data from The Human Protein Atlas: https://www.proteinatlas.org/).
FIGURE 1. ARHGAP11A expression in different tumor and normal tissues. (A) Oncomine. (p-value < 1.0E−04), Cell color is determined by the best gene rank percentile for the analyses within the cell. An analysis may be counted in more than one cancer type. (B) TIMER. (*p-value < 0.05, *** p-value < 1.0E−03).
We next identified the prognostic value of ARHGAP11A in various cancers by using the Prognoscan and Kaplan-Meier plotter (Figure 2 and Supplementary Tables 2–6). Results revealed that high expression of ARHGAP11A was significantly associated with a better prognosis in gastric cancer (OS HR = 0.7, p = 6.4e−04) (Figure 2C) and blood cancer (The cohort GSE12417, OS HR = 0.47, Cox p = 0.047) (Figure 2J). In contrast, high expression of ARHGAP11A was correlated with poor prognosis in lung cancer (OS HR = 1.6, p = 3.8e−13; Progression free survival (PFS) HR = 1.61, P = 1e−06; The cohort GSE13213, OS HR = 1.80, Cox p = 0.0000) (Figures 2E,F, 2M), bladder cancer (The cohort GSE13507, disease Specific Survival (DSS) HR = 6.52, Cox p = 0.0046) (Figure 2I), breast cancer (The cohort GSE6532, RFS HR = 3.53, Cox p = 0.0037; The cohort GSE11121, Distant Metastasis Free Survival (DMFS) HR = 2.02, Cox p = 0.0176) (Figures 2K,L) and soft tissue cancer (The cohort GSE30929, Distant relapse free survival (DRFS) HR = 44.30, Cox p = 0.000052) (Figure 2N). No significant relationship was identified between the expression of ARHGAP11A and prognosis of breast and ovarian cancer patients (Figures 2A,B,G,H).
FIGURE 2. The prognostic value of ARHGAP11A expression in various types of cancer. (A–H) Kaplan-Meier Plotter. (I–N) PrognoScan. The cohort GSE12417: expression profiling by array; cancer subtype: acute myeloid leukemia; n = 163; endpoint: overall survival. The cohort GSE13213: expression profiling by array; cancer subtype: lung adenocarcinoma; n = 117; patient age: 32–84 years old; endpoint: overall survival. The cohort GSE13507: expression profiling by array; cancer subtype: bladder transitional cell carcinoma; n = 165; patient age: 27–88 years old; sample type: frozen tissue; endpoint: disease specific survival. The cohort GSE6532: expression profiling by array; cancer subtype: breast cancer; n = 87; endpoint: distant metastasis free survival. The cohort GSE11121: expression profiling by array; cancer subtype: breast cancer; n = 200; patient age: 34–89 years old; sample type: frozen; endpoint: distant metastasis free survival. The cohort GSE30929: expression profiling by array; cancer subtype: liposarcoma; n = 140; endpoint: distant recurrence free survival.
To further understand the role of ARHGAP11A in gastric cancer, we analyzed the correlation between the ARHGAP11A expression and clinical parameters by using the Kaplan-Meier plotter. High expression of ARHGAP11A was significantly correlated with better prognosis in patients with specific clinical parameters (p < 0.05) (Table 1). Interestingly, the therapeutic strategy and HER2 status influenced the prognostic value of ARHGAP11A. High expression level of ARHGAP11A was associated with better prognosis when the patient treated with surgery alone, while in contrast, associated with worse prognosis when treated with surgery and 5-Fu based adjuvant chemotherapy (Table 1). Moreover, High expression levels of ARHGAP11A indicated better prognosis in HER2 negative patients, while indicated worse prognosis in HER2 positive patients (Table 1).
TABLE 1. Correlation of ARHGAP11A and clinical parameters in gastric cancer from Kaplan-Meier Plotter.
We next analyzed the correlation between ARHGAP11A expression and immune infiltrates in gastric cancer by using TIMER (Figure 3). We found that the expression of ARHGAP11A was negatively associated with infiltration levels of CD8+ T cells (p = 1.38e−04), CD4+ T cells (p = 1.64e−03), macrophages (p = 4.56e−09) and dendritic cells (p = 1.51e−04) (Figure 3A). Moreover, the macrophage and dendritic cell infiltration significantly correlate with prognosis of gastric cancer patients in KM survival analysis (Figure 3B). The upper results implied ARHGAP11A might affect patient prognosis via regulating immune infiltrates in gastric cancer.
FIGURE 3. ARHGAP11A expression is correlated with immune infiltrates in gastric cancer. (A) Correlation of ARHGAP11A expression with immune cell infiltration. (B) Prognostic value of immune cell infiltration in gastric cancer.
We revealed the correlation between ARHGAP11A expression and gene markers of different types of immune cells by using the TIMER and GEPIA. As shown in Table 2 and Figures 4A–H, ARHGAP11A expression was significantly correlated with multiple immune markers, in particular, including the macrophage/TAM marker (CCL2, NOS2, and MS4A4A), the neutrophil marker (CEACAM8 and CCR7), the natural killer cell marker (KIR2DL3, KIR2DL4, and KIR3DL3), the dendritic cell marker (HLA-DPB1 and CD1C), the Th1/Th2 marker (STAT1, IFNG and GATA3), the exhausted T cell marker (CTLA4 and GZMB). We further evaluated the relationship between ARHGAP11A expression and these immune markers in gastric cancer using the GEPIA. Similar results were shown in Table 3. For example, the M1 Macrophage marker NOS2 and the dendritic cell marker, HLA-DPB1 and CD1C, were significantly correlated with ARHGAP11A expression in gastric cancer. Taken together, ARHGAP11A might involve in infiltration of M1 Macrophages and dendritic cells.
FIGURE 4. Correlation between ARHGAP11A expression and immune markers in gastric cancer. (A) Markers of macrophages/TMAs. (B) Markers of neutrophils. (C) Markers of nature killer cells. (D) Markers of dendritic cells. (E) Markers of Th1/Th2 cells. (F) Markers of exhausted T cells.
We used the cBioPortal to analyze genetic changes in gastric cancer. Among 1,120 gastric cancer patients, ARHGAP11A was changed in 22 samples (2%), including 13 mutations, 5 amplifications and 4 deep deletions (Figure 5A and Supplementary Figure S2). In addition, ARHGAP11A mutations did not affect the prognosis of gastric cancer (Supplementary Figures S3, 4). The difference of prognosis between ARHGAP11A altered and unaltered group with no statistical significance might due to low frequency of ARHGAP11A alteration. In GeneMANIA analysis, molecular signals interacting with ARHGAP11A included MKI67, MEK2, DLGAP5, KIF14, KIF18B, AURKB, RHOBTB2, PLK4, KIFC1, CDC20, CENPF, SFN, PLK1, WDHD1, KIF2C, CCNB1, KIF20B, TTK, OIP5 and CCNA2 (Figure 5B). Genes from the PPI network and TIMER analysis were included in the GO function and KEGG pathway analyses by using Metascape. Results showed that ARHGAP11A and its interacting signals involved in regulating of lymphocyte activation, immune effector process, cell killing, myeloid leukocyte differentiation, antigen receptor-mediated signaling pathway and leukocyte apoptotic process (Figure 5C).
FIGURE 5. Genetic alteration, PPI network and enrichment analyses of ARHGAP11A. (A) Genetic alteration of ARHGAP11A in gastric cancer. (B) PPI network of ARHGAP11A in GeneMANIA. (C) A heat map of GO function and KEGG pathway analysis of ARHGAP11A and its interacting proteins.
Dysregulation of Rho GTPases is identified in multiple cancers, and is associated with cancer development and malignant phenotypes. The activity of Rho GTPases (GDP/GTP cycling) is precisely controlled by regulators including GTPase-activating proteins (GAPs) (Lavanderos et al., 2020). Altered expression of GAPs is present in various cancers. ARHGAP10, a member of GAPs, is downregulated in ovarian and breast cancer (Luo et al., 2016; Li et al., 2019). ARHGAP5 is upregulated in metastatic colorectal cancers (Tian et al., 2020). In our study, we systematically analyzed the expression of ARHGAP11A in different tissue types. The expression of ARHGAP11A was found significantly higher in gastrointestinal cancers including stomach adenocarcinoma. Expression level of ARHGAP11A in the metastatic lesion of SKCM was higher than primary SKCM. In addition, the high expression level of ARHGAP11A was associated with better prognosis in gastric and blood cancer, while correlated with poor prognosis in lung, bladder, breast and soft tissue cancer. Taken together, ARHGAP11A revealed dual effects on different human cancers.
Interestingly, an interaction effect exists between ARHGAP11A expression and therapeutic strategy on the prognosis of gastric cancer. High expression level of ARHGAP11A was associated with better prognosis when the patient treated with surgery alone, while in contrast, associated with worse prognosis when treated with surgery and 5-Fu based adjuvant chemotherapy. Microsatellite instability (MSI) is a mature biomarker for predicting the efficacy of immune checkpoint inhibitors. Similar effects were reported when MSI status was applied to predict the chemo-sensitivity in locally advanced colorectal cancer. Efficacy of 5-Fu based adjuvant chemotherapy was significantly different in MSI-H and MSI-L/MSS tumors (Ribic et al., 2003; Klingbiel et al., 2015). We consequently explored the correlation between ARHGAP11A expression and immune infiltrates in gastric cancer.
The lymphocyte in the immune microenvironment is a predictor of sentinel lymph node metastasis and patient survival (Azimi et al., 2012). The evaluation of immune infiltrates in gastric cancer showed that ARHGAP11A expression was significantly associated CD8+ T cells, CD4+ T cells, macrophages and dendritic cells. Immune genes correlated with ARHGAP11A expression included CCL2, NOS2, CCR7, KIR2DL3, KIR2DL4, HLA-DPB1, CD1C, STAT1, IFNG, and GATA3 and CTLA4. CCL2 is an inflammatory chemokine that promotes the recruitment of Tumor-associated macrophages (TAMs) to tumor sites (Nakatsumi et al., 2017). Chen C, et al. identified a long noncoding RNA, termed Lymph Node Metastasis Associated Transcript 1 (LNMAT1). Mechanistically, LNMAT1 epigenetically activates CCL2 expression and recruits macrophages into bladder cancer, which promotes lymphatic metastasis via VEGF-C excretion (Chen et al., 2018). In our ongoing parallel study, high expression of ARHGAP11A appeared more frequently in gastric cancer with lymph node metastasis. Silencing ARHGAP11A in vitro, resulting in the decrease of the invasive ability of gastric cancer cells to lymphatic endothelial cells (unpublished data). ARHGAP11A might play a key role in lymph node metastasis of gastric cancer. Whether LNMAT1 and VEGF-C involves in the biomolecular process needs to be verified in the future study. M1 macrophage marker NOS2 can activate macrophages and causes tumor cell death (Brune et al., 2017). KIR2DL3 and KIR2DL4 are transmembrane glycoproteins expressed by natural killer cells (Gomez-Luque et al., 2021). We found that expression of ARHGAP11A was correlated with NOS2, KIR2DL3 and KIR2DL4. Whether ARHGAP11A involves in regulating macrophages and natural kill cells is worth to be explored. Studies have shown that T cell infiltration defined immune-evasive environment in gastric cancer patients (Gu et al., 2020). In our study, the expression of ARHGAP11A was correlated with multiple T cell markers including STAT1, IFNG, GATA3, and CTLA4. ARHGAP11A might involve in the T cell infiltration of gastric cancer.
Mutations of Rho GTPases have been identified in various cancers. Rac1 mutation was found in 4–9% of melanomas (Hodis et al., 2012). RhoA mutations were identified in over half of angioimmunoblastic T cell lymphomas (Sakata-Yanagimoto et al., 2014). In our study, genetic alterations of ARHGAP11A were identified in 2% of gastric cancer. Genes interacting with ARHGAP11A included DLGAP5, KIF14, AURKB, and TTK. Enhanced expression of DLGAP5 is observed in colorectal cancer. It defines a more aggressive type of colorectal cancer (Branchi et al., 2019). KIF14 is a potential oncogene, promotes gastric cancer progression and metastasis (Yang et al., 2019). AURKB and TTK participate in chromosomes segregation during mitosis via regulating kinetochore metaphase signaling (Su et al., 2021). Gene functional enrichment analysis showed ARHGAP11A and its interacting proteins involved in numerous processes including lymphocyte activation, cell division, cell killing, immune effector process regulating, and myeloid leukocyte differentiation.
There are still some limitations in our study. Kaplan Meier estimates are unadjusted in the Prognoscan and the results might be biased. Subgroup analysis is needed to confirm the prognostic value of ARHGAP11A expression in various types of cancer. More in vivo and in vitro experiments are needed to verify the abovementioned bioinformatic findings, especially the correlation between ARHGAP11A expression and immune infiltrates. On the other hand, the detailed mechanisms of ARHGAP11A in regulating gastric cancer metastasis needs further study.
In summary, ARHGAP11A might be a crucial regulator of immune infiltrates and a valuable prognostic marker in patients with gastric cancer. Additional studies are needed to validate its role both in vitro and in vivo.
The datasets presented in this study can be found in online repositories. The names of the repository/repositories and accession number(s) can be found in the article/Supplementary Material.
BF and XW designed the analytical strategies. The data analyses was conducted by BF, KJ, ZB, JZ, HY, and JL. BF wrote the manuscript. All authors have read and approved the manuscript.
This work is supported by the National Nature Science Foundation of China No. 81402308 and Science Foundation of Peking University Cancer Hospital No. 2021-24 and No.A001546.
The authors declare that the research was conducted in the absence of any commercial or financial relationships that could be construed as a potential conflict of interest.
All claims expressed in this article are solely those of the authors and do not necessarily represent those of their affiliated organizations, or those of the publisher, the editors and the reviewers. Any product that may be evaluated in this article, or claim that may be made by its manufacturer, is not guaranteed or endorsed by the publisher.
We would like to acknowledge the public databases including Oncomine, GEPIA, TIMER, Kaplan-Meier plotter and PrognoScan.
The Supplementary Material for this article can be found online at: https://www.frontiersin.org/articles/10.3389/fmolb.2021.720645/full#supplementary-material
Supplementary Figure 1 | Cytoplasmic expression of ARHGAP11A in various types of cancer.
Supplementary Figure 2 | Genetic alterations of ARHGAP11A in gastric cancer from TCGA database.
Supplementary Figure 3 | The overall survival of gastric cancer patients with/without ARHGAP11A alteration.
Supplementary Figure 4 | The disease-free survival of gastric cancer patients with/without ARHGAP11A alteration.
Azimi, F., Scolyer, R. A., Rumcheva, P., Moncrieff, M., Murali, R., McCarthy, S. W., et al. (2012). Tumor-infiltrating Lymphocyte Grade Is an Independent Predictor of sentinel Lymph Node Status and Survival in Patients with Cutaneous Melanoma. Jco 30, 2678–2683. doi:10.1200/jco.2011.37.8539
Balkwill, F. R., Capasso, M., and Hagemann, T. (2012). The Tumor Microenvironment at a Glance. J. Cel Sci 125, 5591–5596. doi:10.1242/jcs.116392
Brambilla, E., Le Teuff, G., Marguet, S., Lantuejoul, S., Dunant, A., Graziano, S., et al. (2016). Prognostic Effect of Tumor Lymphocytic Infiltration in Resectable Non-small-cell Lung Cancer. Jco 34, 1223–1230. doi:10.1200/jco.2015.63.0970
Branchi, V., García, S. A., Radhakrishnan, P., Győrffy, B., Hissa, B., Schneider, M., et al. (2019). Prognostic Value of DLGAP5 in Colorectal Cancer. Int. J. Colorectal Dis. 34, 1455–1465. doi:10.1007/s00384-019-03339-6
Brüne, B., Courtial, N., Dehne, N., Syed, S. N., and Weigert, A. (2017). Macrophage NOS2 in Tumor Leukocytes. Antioxid. Redox Signaling 26, 1023–1043. doi:10.1089/ars.2016.6811
Bruni, D., Angell, H. K., and Galon, J. (2020). The Immune Contexture and Immunoscore in Cancer Prognosis and Therapeutic Efficacy. Nat. Rev. Cancer 20, 662–680. doi:10.1038/s41568-020-0285-7
Cao, W., Chen, H.-D., Yu, Y.-W., Li, N., and Chen, W.-Q. (2021). Changing Profiles of Cancer burden Worldwide and in China: a Secondary Analysis of the Global Cancer Statistics 2020. Chin. Med. J. (Engl) 134, 783–791. doi:10.1097/cm9.0000000000001474
Chen, C., He, W., Huang, J., Wang, B., Li, H., Cai, Q., et al. (2018). LNMAT1 Promotes Lymphatic Metastasis of Bladder Cancer via CCL2 Dependent Macrophage Recruitment. Nat. Commun. 9, 3826. doi:10.1038/s41467-018-06152-x
Cui, J., Chen, Y., Chou, W.-C., Sun, L., Chen, L., Suo, J., et al. (2011). An Integrated Transcriptomic and Computational Analysis for Biomarker Identification in Gastric Cancer. Nucleic Acids Res. 39, 1197–1207. doi:10.1093/nar/gkq960
Dai, B., Zhang, X., Shang, R., Wang, J., Yang, X., Zhang, H., et al. (2018). Blockade of ARHGAP11A Reverses Malignant Progress via Inactivating Rac1B in Hepatocellular Carcinoma. Cell Commun Signal 16, 99. doi:10.1186/s12964-018-0312-4
Gao, J., Aksoy, B. A., Dogrusoz, U., Dresdner, G., Gross, B., Sumer, S. O., et al. (2013). Integrative Analysis of Complex Cancer Genomics and Clinical Profiles Using the cBioPortal. Sci. Signaling 6, pl1. doi:10.1126/scisignal.2004088
Gomez-Luque, J. M., Urrutia-Maldonado, E., Munoz de Rueda, P., Abril-Molina, A., and Ocete-Hita, E. (2021). Killer Immunoglobulin-like Receptor and Cancer. Pediatr. (Barc).
Gu, Y., Chen, Y., Jin, K., Cao, Y., Liu, X., Lv, K., et al. (2020). Intratumoral CD103+CD4+ T Cell Infiltration Defines Immunoevasive Contexture and Poor Clinical Outcomes in Gastric Cancer Patients. Oncoimmunology 9, 1844402. doi:10.1080/2162402x.2020.1844402
Hodis, E., Watson, I. R., Kryukov, G. V., Arold, S. T., Imielinski, M., Theurillat, J.-P., et al. (2012). A Landscape of Driver Mutations in Melanoma. Cell 150, 251–263. doi:10.1016/j.cell.2012.06.024
Klingbiel, D., Saridaki, Z., Roth, A. D., Bosman, F. T., Delorenzi, M., and Tejpar, S. (2015). Prognosis of Stage II and III colon Cancer Treated with Adjuvant 5-fluorouracil or FOLFIRI in Relation to Microsatellite Status: Results of the PETACC-3 Trial. Ann. Oncol. 26, 126–132. doi:10.1093/annonc/mdu499
Lavanderos, B., Silva, I., Cruz, P., Orellana-Serradell, O., Saldías, M. P., and Cerda, O. (2020). TRP Channels Regulation of Rho GTPases in Brain Context and Diseases. Front. Cel Dev. Biol. 8, 582975. doi:10.3389/fcell.2020.582975
Lawson, C. D., Fan, C., Mitin, N., Baker, N. M., George, S. D., Graham, D. M., et al. (2016). Rho GTPase Transcriptome Analysis Reveals Oncogenic Roles for Rho GTPase-Activating Proteins in Basal-like Breast Cancers. Cancer Res. 76, 3826–3837. doi:10.1158/0008-5472.can-15-2923
Li, T., Fan, J., Wang, B., Traugh, N., Chen, Q., Liu, J. S., et al. (2017). TIMER: A Web Server for Comprehensive Analysis of Tumor-Infiltrating Immune Cells. Cancer Res. 77, e108–e110. doi:10.1158/0008-5472.can-17-0307
Li, Y., Zeng, B., Li, Y., Zhang, C., and Ren, G. (2019). Downregulated Expression of ARHGAP10 Correlates with Advanced Stage and High Ki-67 index in Breast Cancer. PeerJ 7, e7431. doi:10.7717/peerj.7431
Luo, N., Guo, J., Chen, L., Yang, W., Qu, X., and Cheng, Z. (2016). ARHGAP10, Downregulated in Ovarian Cancer, Suppresses Tumorigenicity of Ovarian Cancer Cells. Cell Death Dis 7, e2157. doi:10.1038/cddis.2015.401
Mizuno, H., Kitada, K., Nakai, K., and Sarai, A. (2009). PrognoScan: a New Database for Meta-Analysis of the Prognostic Value of Genes. BMC Med. Genomics 2, 18. doi:10.1186/1755-8794-2-18
Müller, P. M., Rademacher, J., Bagshaw, R. D., Wortmann, C., Barth, C., van Unen, J., et al. (2020). Systems Analysis of RhoGEF and RhoGAP Regulatory Proteins Reveals Spatially Organized RAC1 Signalling from Integrin Adhesions. Nat. Cel Biol 22, 498–511. doi:10.1038/s41556-020-0488-x
Nakatsumi, H., Matsumoto, M., and Nakayama, K. I. (2017). Noncanonical Pathway for Regulation of CCL2 Expression by an mTORC1-FOXK1 Axis Promotes Recruitment of Tumor-Associated Macrophages. Cel Rep. 21, 2471–2486. doi:10.1016/j.celrep.2017.11.014
Porter, A. P., Papaioannou, A., and Malliri, A. (2016). Deregulation of Rho GTPases in Cancer. Small GTPases 7, 123–138. doi:10.1080/21541248.2016.1173767
Refolo, M. G., Lotesoriere, C., Messa, C., Caruso, M. G., and D'Alessandro, R. (2020). Integrated Immune Gene Expression Signature and Molecular Classification in Gastric Cancer: New Insights. J. Leukoc. Biol. 108, 633–646. doi:10.1002/jlb.4mr0120-221r
Rhodes, D. R., Kalyana-Sundaram, S., Mahavisno, V., Varambally, R., Yu, J., Briggs, B. B., et al. (2007). Oncomine 3.0: Genes, Pathways, and Networks in a Collection of 18,000 Cancer Gene Expression Profiles. Neoplasia 9, 166–180. doi:10.1593/neo.07112
Ribas, A., and Wolchok, J. D. (2018). Cancer Immunotherapy Using Checkpoint Blockade. Science 359, 1350–1355. doi:10.1126/science.aar4060
Ribic, C. M., Sargent, D. J., Moore, M. J., Thibodeau, S. N., French, A. J., Goldberg, R. M., et al. (2003). Tumor Microsatellite-Instability Status as a Predictor of Benefit from Fluorouracil-Based Adjuvant Chemotherapy for colon Cancer. N. Engl. J. Med. 349, 247–257. doi:10.1056/nejmoa022289
Sakata-Yanagimoto, M., Enami, T., Yoshida, K., Shiraishi, Y., Ishii, R., Miyake, Y., et al. (2014). Somatic RHOA Mutation in Angioimmunoblastic T Cell Lymphoma. Nat. Genet. 46, 171–175. doi:10.1038/ng.2872
Salmon, H., Remark, R., Gnjatic, S., and Merad, M. (2019). Host Tissue Determinants of Tumour Immunity. Nat. Rev. Cancer 19, 215–227. doi:10.1038/s41568-019-0125-9
Saoudi, A., Kassem, S., Dejean, A., and Gaud, G. (2014). Rho-GTPases as Key Regulators of T Lymphocyte Biology. Small GTPases 5 (4), e983862. doi:10.4161/sgtp.28208
Su, T., Qin, X. Y., Dohmae, N., Wei, F., Furutani, Y., Kojima, S., et al. (2021). Inhibition of Ganglioside Synthesis Suppressed Liver Cancer Cell Proliferation through Targeting Kinetochore Metaphase Signaling. Metabolites 11 (3), 167. doi:10.3390/metabo11030167
Sudo, T., Nishida, R., Kawahara, A., Saisho, K., Mimori, K., Yamada, A., et al. (2017). Clinical Impact of Tumor-Infiltrating Lymphocytes in Esophageal Squamous Cell Carcinoma. Ann. Surg. Oncol. 24, 3763–3770. doi:10.1245/s10434-017-5796-4
Sun, D., Cao, M., Cao, M., Li, H., He, S., and Chen, W. (2020). Cancer burden and Trends in China: A Review and Comparison with Japan and South Korea. Chin. J. Cancer Res. 32, 129–139. doi:10.21147/j.issn.1000-9604.2020.02.01
Sung, H., Ferlay, J., Siegel, R. L., Laversanne, M., Soerjomataram, I., Jemal, A., et al. (2021). Global Cancer Statistics 2020: GLOBOCAN Estimates of Incidence and Mortality Worldwide for 36 Cancers in 185 Countries. CA Cancer J. Clin. 71 (3), 209–249. doi:10.3322/caac.21660
Szász, A. M., Lánczky, A., Nagy, Á., Förster, S., Hark, K., Green, J. E., et al. (2016). Cross-validation of Survival Associated Biomarkers in Gastric Cancer Using Transcriptomic Data of 1,065 Patients. Oncotarget 7, 49322–49333. doi:10.18632/oncotarget.10337
Tang, Z., Li, C., Kang, B., Gao, G., Li, C., and Zhang, Z. (2017). GEPIA: a Web Server for Cancer and normal Gene Expression Profiling and Interactive Analyses. Nucleic Acids Res. 45, W98–W102. doi:10.1093/nar/gkx247
Tian, T., Chen, Z.-H., Zheng, Z., Liu, Y., Zhao, Q., Liu, Y., et al. (2020). Investigation of the Role and Mechanism of ARHGAP5-Mediated Colorectal Cancer Metastasis. Theranostics 10, 5998–6010. doi:10.7150/thno.43427
Warde-Farley, D., Donaldson, S. L., Comes, O., Zuberi, K., Badrawi, R., Chao, P., et al. (2010). The GeneMANIA Prediction Server: Biological Network Integration for Gene Prioritization and Predicting Gene Function. Nucleic Acids Res. 38, W214–W220. doi:10.1093/nar/gkq537
Xu, J., Zhou, X., Wang, J., Li, Z., Kong, X., Qian, J., et al. (2013). RhoGAPs Attenuate Cell Proliferation by Direct Interaction with P53 Tetramerization Domain. Cel Rep. 3, 1526–1538. doi:10.1016/j.celrep.2013.04.017
Yang, L., Ying, X., Ying, X., Liu, S., Lyu, G., Xu, Z., et al. (2020). Gastric Cancer: Epidemiology, Risk Factors and Prevention Strategies. Chin. J. Cancer Res. 32, 695–704. doi:10.21147/j.issn.1000-9604.2020.06.03
Yang, Z., Li, C., Yan, C., Li, J., Yan, M., Liu, B., et al. (2019). KIF14 Promotes Tumor Progression and Metastasis and Is an Independent Predictor of Poor Prognosis in Human Gastric Cancer. Biochim. Biophys. Acta (Bba) - Mol. Basis Dis. 1865, 181–192. doi:10.1016/j.bbadis.2018.10.039
Zeng, D., Li, M., Zhou, R., Zhang, J., Sun, H., Shi, M., et al. (2019). Tumor Microenvironment Characterization in Gastric Cancer Identifies Prognostic and Immunotherapeutically Relevant Gene Signatures. Cancer Immunol. Res. 7, 737–750. doi:10.1158/2326-6066.cir-18-0436
Zhao, Y., Xu, E., Yang, X., Zhang, Y., Chen, H., Wang, Y., et al. (2020). Tumor Infiltrative Growth Pattern Correlates with the Immune Microenvironment and Is an Independent Factor for Lymph Node Metastasis and Prognosis in Stage T1 Esophageal Squamous Cell Carcinoma. Virchows Arch. 477, 401–408. doi:10.1007/s00428-020-02801-z
Zhou, Y., Zhou, B., Pache, L., Chang, M., Khodabakhshi, A. H., Tanaseichuk, O., et al. (2019). Metascape Provides a Biologist-Oriented Resource for the Analysis of Systems-Level Datasets. Nat. Commun. 10, 1523. doi:10.1038/s41467-019-09234-6
ACC Adrenocortical carcinoma
BLCA Bladder urothelial carcinoma
BRCA Breast invasive carcinoma
CESC Cervical squamous cell carcinoma and endocervical adenocarcinoma
CHOL Cholangiocarcinoma
COAD Colon adenocarcinoma
DLBC Lymphoid Neoplasm Diffuse Large B-cell Lymphoma
DMFS Distant Metastasis Free Survival
DRFS Distant relapse free survival
DSS Disease Specific Survival
ESCA Esophageal carcinoma
GAPs GTPase-activating proteins
GBM Glioblastoma multiforme
GEPIA Gene Expression Profiling Interactive Analysis
HNSC Head and neck squamous cell carcinoma
HR Hazard ratio
KICH Kidney chromophobe
KIRC Kidney renal clear cell carcinoma
KIRP Kidney renal papillary cell carcinoma
LAML Acute Myeloid Leukemia
LGG Brain Lower Grade Glioma
LIHC Liver hepatocellular carcinoma
LUAD Lung adenocarcinoma
LUSC Lung squamous cell carcinoma
MESO Mesothelioma Rectum adenocarcinoma
MSI Microsatellite instability
OS Overall survival
OV Ovarian serous cystadenocarcinoma
PFS Progression free survival
PPI protein-protein interaction
PRAD Prostate adenocarcinoma
READ Rectum adenocarcinoma
RFS Relapse Free Survival
RhoGAPs Rho GTPase-activating proteins
SARC Sarcoma
SKCM Skin cutaneous melanoma
STAD Stomach adenocarcinoma
TAMs Tumor-associated macrophages
TCGA The Cancer Genome Atlas
TGCT Testicular Germ Cell Tumors
THCA Thyroid carcinoma
THYM Thymoma
TILs tumor-infiltrating lymphocytes
TIMER Tumor IMmune Estimation Resource
UCEC Uterine corpus endometrial carcinoma
UCS Uterine Carcinosarcoma
UVM Uveal Melanoma
Keywords: ARHGAP11A, immune infiltrates, gastric cancer, prognosis, biomarker
Citation: Fan B, Ji K, Bu Z, Zhang J, Yang H, Li J and Wu X (2021) ARHGAP11A Is a Prognostic Biomarker and Correlated With Immune Infiltrates in Gastric Cancer. Front. Mol. Biosci. 8:720645. doi: 10.3389/fmolb.2021.720645
Received: 04 June 2021; Accepted: 29 September 2021;
Published: 18 October 2021.
Edited by:
Ismail Hosen, University of Dhaka, BangladeshReviewed by:
Quan Zhou, China Three Gorges University, ChinaCopyright © 2021 Fan, Ji, Bu, Zhang, Yang, Li and Wu. This is an open-access article distributed under the terms of the Creative Commons Attribution License (CC BY). The use, distribution or reproduction in other forums is permitted, provided the original author(s) and the copyright owner(s) are credited and that the original publication in this journal is cited, in accordance with accepted academic practice. No use, distribution or reproduction is permitted which does not comply with these terms.
*Correspondence: Xiaojiang Wu, ZHJ3dXhpYW9qaWFuZ0AxNjMuY29t
†These authors have contributed equally to this work
Disclaimer: All claims expressed in this article are solely those of the authors and do not necessarily represent those of their affiliated organizations, or those of the publisher, the editors and the reviewers. Any product that may be evaluated in this article or claim that may be made by its manufacturer is not guaranteed or endorsed by the publisher.
Research integrity at Frontiers
Learn more about the work of our research integrity team to safeguard the quality of each article we publish.