- 1Department of Vascular and Endovascular Surgery, First Affiliated Hospital of Zhengzhou University, Zhengzhou, Henan, China
- 2Science China Press, Beijing, China
- 3Key Laboratory of Advanced Drug Preparation Technologies, School of Pharmaceutical Sciences, Ministry of Education, Zhengzhou University, Zhengzhou, China
- 4Laboratory of Synthetic Biology, Zhengzhou University, Zhengzhou, China
- 5Oncology Department, Colorectal and Anal Surgery Department, The First Affiliated Hospital of Zhengzhou University, Zhengzhou, Henan, China
- 6Department of Biology and Biological Engineering, Chalmers University of Technology, Gothenburg, Sweden
The gastrointestinal tract, the largest human microbial reservoir, is highly dynamic. The gut microbes play essential roles in causing colorectal diseases. In the present study, we explored potential keystone taxa during the development of colorectal diseases in central China. Fecal samples of some patients were collected and were allocated to the adenoma (Group A), colorectal cancer (Group C), and hemorrhoid (Group H) groups. The 16S rRNA amplicon and shallow metagenomic sequencing (SMS) strategies were used to recover the gut microbiota. Microbial diversities obtained from 16S rRNA amplicon and SMS data were similar. Group C had the highest diversity, although no significant difference in diversity was observed among the groups. The most dominant phyla in the gut microbiota of patients with colorectal diseases were Bacteroidetes, Firmicutes, and Proteobacteria, accounting for >95% of microbes in the samples. The most abundant genera in the samples were Bacteroides, Prevotella, and Escherichia/Shigella, and further species-level and network analyses identified certain potential keystone taxa in each group. Some of the dominant species, such as Prevotella copri, Bacteroides dorei, and Bacteroides vulgatus, could be responsible for causing colorectal diseases. The SMS data recovered diverse antibiotic resistance genes of tetracycline, macrolide, and beta-lactam, which could be a result of antibiotic overuse. This study explored the gut microbiota of patients with three different types of colorectal diseases, and the microbial diversity results obtained from 16S rRNA amplicon sequencing and SMS data were found to be similar. However, the findings of this study are based on a limited sample size, which warrants further large-scale studies. The recovery of gut microbiota profiles in patients with colorectal diseases could be beneficial for future diagnosis and treatment with modulation of the gut microbiota. Moreover, SMS data can provide accurate species- and gene-level information, and it is economical. It can therefore be widely applied in future clinical metagenomic studies.
Introduction
According to global cancer statistics 2020 by GLOBOCAN, among the 36 kinds of cancers, colorectal cancer is the third most common malignancy and the second most lethal tumor in 185 countries (Sung et al., 2021). It is associated with a high mortality rate, with 0.93 million deaths reported worldwide in 2020 (Sung et al., 2021). Research suggests that genetic factors are responsible for 12–35% of all colorectal cancers (Lichtenstein et al., 2000; Czene et al., 2002; Song et al., 2020; Rajamäki et al., 2021). The human intestine is the largest microbial reservoir. The number of gut microbes is nearly equal to that of the cells present in the entire body (Wang et al., 2017), while the number of genes from the gut microbiota is 100-fold greater than that from the human genome (Bilen et al., 2018). Intestinal microbes are associated with diverse colorectal diseases, including colorectal carcinoma (Wong and Yu, 2019; Schmitt and Greten, 2021; Xing et al., 2021).
Previous studies have reported the association of some important gut microbial species with colorectal cancer (Dai et al., 2018; Wirbel et al., 2019; Yachida et al., 2019). Fusobacterium nucleatum (Brennan and Garrett, 2019; Rubinstein et al., 2019), certain Escherichia coli strains (Wassenaar, 2018), some Bacteroides fragilis strains (Sears et al., 2014; Chung et al., 2018), and several other bacterial species have been linked to colorectal cancer (Dai et al., 2018; Butt et al., 2021). Some of these strains induce colorectal carcinogenesis by generating metabolites or toxins (Wong and Yu, 2019). A driver–passenger model was proposed for assessing the genetic pattern of colorectal cancer progression (Tjalsma et al., 2012). The driver microbes, including the toxigenic microbes of E. coli and B. fragilis strains, damaged the host DNA and disrupted colonic barriers (Cuevas-Ramos et al., 2010; Goodwin et al., 2011; Pleguezuelos-Manzano et al., 2020; Butt et al., 2021; Lopez et al., 2021), whereas the passenger microbes, such as F. nucleatum, proliferated in the suitable niche created by the driver microbes (Tjalsma et al., 2012; Shang and Liu, 2018). The dysbiosis of the micro-niche may lead to colorectal cancer (Brennan and Garrett, 2016; Tilg et al., 2018). Similarly, microbial profiling of gastric cancer samples suggested that Helicobacter pylori may be the driver microbe and several opportunistic pathogenic microbes may directly cause gastric cancer (Li et al., 2021).
Colorectal adenoma is the main precursor of most colorectal cancers (Corley et al., 2014; Click et al., 2018). Hemorrhoid is a common colorectal disease with symptoms different from those of colorectal adenoma and colorectal cancer (Jandhyala et al., 2015). Therefore, exploring the gut microbiota of different colorectal diseases could reveal the microbial mechanism of action in colorectal carcinogenesis. The 16S rRNA gene amplicon and metagenomic strategies are commonly used to study the complex gut microbiota involved in colorectal diseases, including colorectal cancer (Flemer et al., 2017; Saito et al., 2019). The 16S rRNA gene amplicon sequencing provides insight into the diversity of microbial communities at the genus level, whereas the metagenomic strategy provides the species- and gene-level information of the gut microbiota (Wei et al., 2020b; Liang et al., 2021). Typically, the 16S rRNA gene amplicon strategy is economical than the metagenomic strategy and the associated library construction is complex (Laudadio et al., 2018; Rausch et al., 2019; Shi et al., 2020). The shallow metagenomic sequencing (SMS) strategy has been preferred to the 16S rRNA gene amplicon strategy in certain microbiome studies (Zhu et al., 2021). The use of the SMS strategy may decrease the costs associated with metagenomic sequencing strategy (Hillmann et al., 2018; Santiago-Rodriguez et al., 2020). A comparative analysis of infant gut microbiota indicated that 16S rRNA gene amplicon and metagenomic sequencing approaches can provide similar alpha and beta diversity of the gut microbiota (Peterson et al., 2021).
In this study, we used 16S rRNA gene amplicon and SMS strategies to provide insights into the gut microbiota of patients with three colorectal diseases, namely colorectal cancer, adenoma, and hemorrhoid. We compared alpha and beta diversities between the two strategies and identified potential keystone taxa associated with colorectal diseases. Moreover, antibiotic resistant and other functional gene profiles were recovered using SMS data. We also discuss the future prospects of the use of sequencing strategies in determination of the gut microbiota in colorectal diseases.
Materials and Methods
Ethics Statement and Sample Collection
This study was approved by the Institutional Review Board of the First Affiliated Hospital, Zhengzhou University, Zhengzhou, Henan Province, China. All patients agreed to participate and provided written informed consents. Patient details were anonymized for all analyses.
A total of 16 fecal samples were collected from 16 hospitalized patients. The 16 patients were allocated to the Group A (adenoma), Group C (colorectal cancer), or Group H (hemorrhoids; Supplementary Table S1).
The fecal samples were collected in sterile 5-ml tubes containing 2 ml DNA/RNA shield liquids, which were provided by DeepBiome Co., Ltd. The collected samples were sent to DeepBiome Co., Ltd. for 16S rRNA gene amplicon and SMS analyses. Patient information including smoking and alcohol intake history and antibiotic use history was obtained.
16S rRNA Gene Amplicon Sequencing
The DNA from samples was extracted using DNeasy PowerSoil Kit (Qiagen, Germany). Approximately 500 µL of the fecal sample from each tube was used for DNA extraction. The DNA was quantified using Nanodrop 2000 (Thermo Fisher Scientific, United States), and the V3-V4 regions of the 16S rRNA gene sequences were amplified using KAPA HiFi HotStart ReadyMix PCR Kit KK2600 (Roche Sequencing, South Africa), according to the manufacturer’s instructions. The amplified PCR fragments were purified and mixed (Liang et al., 2019; Jiang et al., 2020; Mai et al., 2020). The sequencing of mixed PCR fragments was performed by DeepBiome Co., Ltd.
16S rRNA Amplicon Data Analyses
The raw fastq files were processed using atlas-utils, and barcode and low-quality sequences were filtered using Trimmomatic (Wei et al., 2020a). After filtering, the chimeric reads were removed using atlas-utilis. Paired-end reads were merged with default parameters by using Usearch software v.11.0.667. Finally, the sequences were classified into operational taxonomic units (OTUs), with a cutoff of 97% identity (Cole et al., 2014). The OTU tables were generated using Usearch software. The alpha parameters of the samples were generated using the Usearch alpha_div tool (Wei et al., 2020a). The beta-diversity distance and differences between the samples and the OTU table were evaluated using the Usearch beta_div tool. The unweighted pair–group method with arithmetic mean (UPGMA) was used, and principal coordinates analysis (PCoA) was performed using R software. MetagenoNets was used to infer microbial networks in the study groups (Nagpal et al., 2020). A Pearson vanilla algorithm was used for network construction. The p value was significant at 0.05, and the critical r-value cutoff was 0.7 (Li et al., 2021). The network figures were generated according to the instructions of MetagenoNets (Nagpal et al., 2020).
Metagenomic Sequencing
The DNA used for the SMS was the same as that used for 16S rRNA amplicon sequencing. The DNA was quantified using Qubit dsDNA HS assay kit (12640ES60, Yeason Biotechnology, Shanghai, China). KAPA HyperPrep Kit (Illumina; KK8504), KAPA Dual-Indexed Adapters (KK8722), KAPA pure beads, and KAPA Library Quantification Kit (KK4824) were used to construct the SMS library (Liang et al., 2021). The SMS library was sequenced using the Illumina platform, and approximately 1 Gbp data were generated after sequencing.
SMS Data Analyses
The quality of raw SMS data was evaluated using FastqQC (version 0.11.9), and adaptor sequences in raw reads were removed using Trimmomatic (version 0.38 (Bolger et al., 2014). Kraken2 was used for taxonomic classification of SMS data. The sequence reads were assembled using metaSPAdes (version 3.13.2) with the parameters of −k 21,33,55 (Nurk et al., 2017). eggnog-mapper (version 0.12.7) was used for COG term assignment, with KOfam parameters of −e 1e-3 (Huerta-Cepas et al., 2017). The gene-level TPM values of the genes were calculated (Wei et al., 2019). AMRFinder Plus (version 3.1.1b) was used to annotate the antimicrobial resistance genes in the SMS data (Feldgarden et al., 2019).
All 16S rRNA gene amplicon and SMS data were deposited in the GenBank database, with the BioProject accession number of PRJNA725613, with the BioSample accession numbers of SAMN1889570-SAMN18895718, the SRA accession numbers of 16S rRNA data are SRR14410510-SRR14410525, the SRA accession numbers of SMS data are SRR14460530-SRR14460545.
Results
Microbial Profiles of the Samples
The numbers of samples obtained from Groups A, C, and H were 6, 4, and 6, respectively (Supplementary Table S1). The 16S rRNA gene fragment sequencing generated 1,457,234 reads, with an average of 91,077 sequences per sample (Supplementary Table S1). The sizes of SMS data for these samples were 0.45–5.76 G (average: 1.6 G) (Supplementary Table S2).
The sequences of these samples were classified into OTUs based on 97% identity. The OTUs ranged from 105 to 314 (Table 1). The average OTUs of Groups A, C, and H were 162.17 ± 32.79, 251.75 ± 21.36, and 239.83 ± 52.05, respectively (Table 1). Group C demonstrated to have the highest diversity (Table 1), although no significant difference in diversity was observed among the groups. SMS data also demonstrated similar results, with Group C having the highest diversity (Supplementary Table S3).
Microbial Diversity at the Phylum and Genus Levels
At the phylum level, the most dominant phyla were Bacteroidetes, Firmicutes, and Proteobacteria (Table 2). The most dominant phylum in all the groups was Bacteroidetes, with the following compositions: 44.41 ± 19.76% in Group A, 52.95 ± 7.33% in Group C, and 59.04 ± 17.41% in Group H (Table 2). The second most dominant phylum in all the groups was Firmicutes, and its percentage in Group C was higher (40.44 ± 6.63%) than in Groups A and H. Proteobacteria was the third most dominant phylum, with a higher percentage in Group A (24.97 ± 20.75%) than in Group C (3.95 ± 1.74%) and Group H (8.28 ± 12.12%; Table 2). The percentage of Fusobacteria in Group A (3.16 ± 6.20%) was higher than in Group C (0.4 ± 0.77%) and Group H (0.07 ± 0.08%; Table 2). The 16S rRNA gene data revealed that the microbial distribution was different in samples from the same groups at phylum and genus levels (Supplementary Figure S1).
The SMS data were similar to the 16S rRNA data, although the richness of SMS data was higher than that of 16S rRNA data (Table 1 and Supplementary Table S2). Bacteroidetes, Firmicutes, and Proteobacteria (Supplementary Table S4) were the most dominant phyla in the samples. However, their compositions in the groups were found to differ between 16S rRNA and SMS data (Table 2 and Supplementary Table S4). Importantly, the percentage of Bacteroidota in Group A was 49.76%, whereas it was 61.66 and 75.79% in Groups C and H, respectively (Supplementary Table S4). The percentage of Bacteroidota in Groups C and H based on SMS data was higher than its corresponding composition in each group based on 16S rRNA data (Table 2 and Supplementary Table S4).
Based on 16S rRNA data, the most dominant genera were assigned to Bacteroides, Prevotella, Escherichia or Shigella, Faecalibacterium, and Blautia (Table 3). Bacteroides and Prevotella were the most dominant genera in all the groups (Table 3). The percentages of Bacteroides in Groups A, C, and H were 28.88 ± 23.70%, 22.2 ± 19.66%, and 33.8 ± 25.05%, respectively (Table 3). The percentages of Prevotella in Groups A, C, and H were 14.41 ± 27.19%, 26.28 ± 24.78%, and 23.12 ± 28.32%, respectively (Table 3). Although the genus compositions of the groups were different, no significant difference was observed between each group. The SMS data were similar to 16S rRNA data in terms of genus compositions. According to SMS data, the most dominant genera in all the groups were Bacteroides and Prevotella, and their compositions in each group were similar (Table 3 and Supplementary Table S5). Overall, microbial compositions at the gene level were consistent between 16S rRNA and SMS data (Table 3 and Supplementary Table S5).
Dominant Species in the Samples
Nearly all the representative sequences of dominant OTUs demonstrated >97% identity with known isolates, indicating that most microbes in the samples could be cultured (Table 4) (Lagier et al., 2016; Bilen et al., 2018). The most dominant OTU, ZOTU_4, in the groups was assigned as Prevotella copri, which is a common gut microbe. P. copri is associated with host health, and its diversity can be affected by the diet (De Filippis et al., 2019). The microbe has strong carbohydrate metabolism ability; however, its composition in the western populations is underrepresented (Tett et al., 2019). In our study, all the samples were from patients of central China. The high-level compositions of P. copri were in accordance with the vegetarian habit in this region. Other dominant OTUs were assigned to the Bacteroides or Escherichia strains (Table 4). Two OTUs, namely ZOTU_8 and ZOTU_5, were assigned to the pathogenic microbes, and their percentages in Group A were high. The ZOTU_8 composition in sample A5 of Group A was 43.72% and the ZOTU_5 composition in sample A3 of Group A was 29.76%. These patients (A3 and A5) had symptoms of inflammation, and it was presumed that strains representing ZOTU_8 and ZOTU_5 caused these symptoms (Table 4). In addition, ZOTU_6, ZOTU_10, and ZOTU_17 percentages in sample A6 of Group A were 29.29, 17.44, and 14.95%, respectively, which were higher than the corresponding OTU percentages of other samples in Group A (Table 4). These three dominant OTUs in sample A6 represented potential opportunistic pathogenic bacteria, which were possibly responsible for the serious adenoma symptoms (Table 4).
Compared with 16S rRNA gene amplicon data, SMS data can provide species-level information. Based on SMS data, the most dominant species were assigned to P. copri, B. dorei, B. vulgatus, and few other Bacteroides genera (Supplementary Table S6). The percentages of Streptococcus pasteurianus and Klebsiella quasipneumoniae in samples A3 and A5 were 18.48 and 29.17%, respectively, which were similar to those obtained with 16S rRNA data. Some B. fragilis strains could serve as driver species in colorectal cancer (Haghi et al., 2019; Wong and Yu, 2019; Butt et al., 2021). The percentages of B. fragilis in Groups A, C, and H were 3.96, 0.17, and 0.43%, respectively. The percentages of another potential colorectal cancer driver species of E. coli in Groups A, C, and H were 2.83, 0.02, and 0.11%, respectively (Supplementary Table S6). The F. nucleatum percentage was not high (<0.01%) in the groups (Shang and Liu, 2018; Brennan and Garrett, 2019), suggesting the presence of other passenger opportunistic species in patients with colorectal cancer (Wong and Yu, 2019). The microbial compositions in 16S rRNA and SMS data were different, although the microbial structures were similar (Table 4 and Supplementary Table S6). Most microbes could be assigned at the species level, suggesting the possibility of isolating the human gut bacteria (Lagier et al., 2016; Bilen et al., 2018).
Group Analysis of the Samples Based on 16S rRNA Data
The beta-diversity analyses based on both 16S rRNA and SMS data revealed that samples from Groups A, C, and H overlapped with each other, with no significant difference (Figure 1). The UPGMA analysis based on 16S rRNA data demonstrated that samples from the same groups had different microbial profiles (Figure 1A). Samples H2, A4, A5, H4, and C4 were clustered, and so were samples H1, C2, and C3. Samples A1 and A3 and samples H6 and A6 were clustered (Figure 1A). The SMS data indicated the same results (Figure 1B). Samples A1 and A3 were clustered, and so were samples H2, A4, H4, and C4 (Figure 1B). The 16S rRNA data and the SMS data were similar (Figure 1). The phylum level distribution of these samples used for the UPGMA analysis further suggests that the 16S rRNA data were similar to the SMS data (Figure 1). The PCoA analysis was consistent with the UPGMA analysis (Figure 1 and Supplementary Figure S2).
The network analysis can indicate potential microbes associated with the disease, and keystone taxa can be predicted based on the microbiota data (Li et al., 2021). The most dominant 54 OTUs with a composition of >0.5% in the samples were selected for network construction. Three networks were generated (Figure 2). The edges for Groups A, C, and H were 247, 401, and 241, respectively (Figure 2 and Supplementary Table S7), and the diameters of the three groups were different. The densities of Groups A and Group H were similar, whereas that of Group C was higher than those of other groups. The average network degrees of Groups A and H were nearly 9, and that of Group C was 14.6 (Supplementary Table S7). These data revealed that the association in Group C was more complex than that in the other groups. These findings suggest the complex microbiota in patients with colorectal cancer and the highly connected correlation between the species of the microbiota (Figure 2).
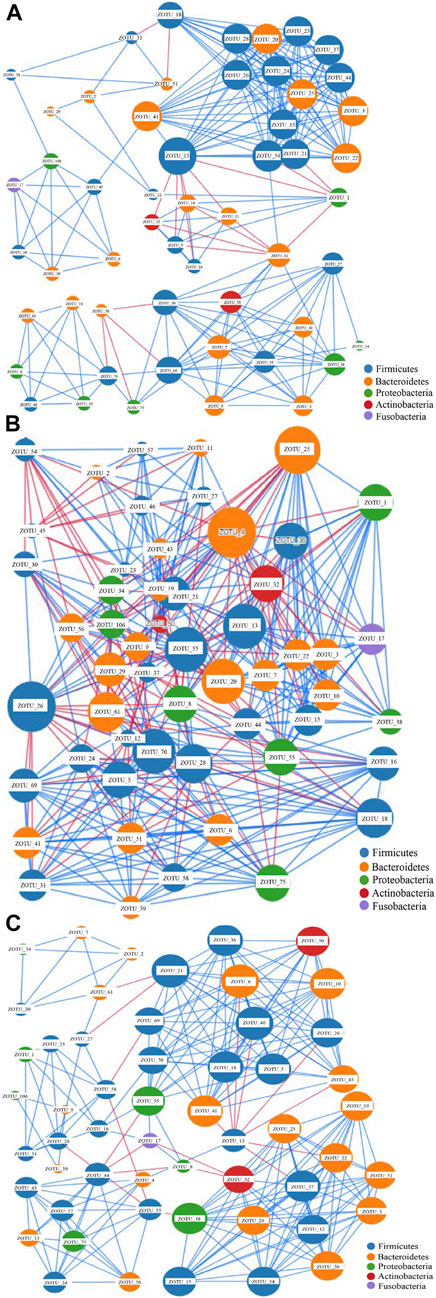
FIGURE 2. Network analysis of the gut microbiota of the three groups based on correlation analysis. (A) the network analysis of group A; (B) The network analysis of Group C; (C). The network analysis of Group H.The size of each node is proportional to the node degree. The lines between two nodes colored in blue indicate positive correlation, whereas the lines between two nodes colored in blue indicate negative correlation.
The nodes in all these networks were assigned to Firmicutes, Bacteroidetes, Proteobacteria, Actinobacteria, and Fusobacteria. In Group A, the most dominant OTUs demonstrated positive correlations with other OTUs. Only four OTUs, namely ZOTU_13, ZOTU_1, ZOTU_61, and ZOTU_58, demonstrated a negative correlation with other OTUs (Figure 2A). These four OTUs may represent pathogenic species and be associated with adenoma in these patients. The OTUs ZOTU_3, ZOTU_15, ZOTU_24, and ZOTU_25 had more positive correlations than other OTUs, suggesting their importance in these samples. In Group C, both positive and negative correlations were present, and the correlations between different species were complex (Figure 2B). The keystone taxa in Group C could be ZOTU_4, ZOTU_20, ZOTU_7, ZOTU_13, and other OTUs. These OTUs are not potential pathogens, but some of them may serve as passenger microbes. Similar to Group A, the main correlations in Group H were positive (Figure 2C). Only ZOTU_13, ZOTU_44, and ZOTU_21 had negative correlations with other species (Figure 2C). Compared with the network of Group C, that of Group H was simple. The predicted keystone taxa of Group H were not pathogens, and most of them were normal human gut microbes (Figure 2C).
Functional Profiles of the Microbiota
The assembly of SMS data generated large amounts of contigs. The assembly quality was related to the generated data. The contig N50 of all the samples ranged from 2,352 to 9,593 bp (Supplementary Table S8). The largest contigs were as high as 64.33 kbp (Supplementary Table S8). The open reading frame (ORF) in the contigs was annotated using the KEGG database. At the catalog level, the TPM values of Groups A and H were higher than that of Group C (Figure 3A). Specifically, the carbohydrate metabolism and other energy and nutrient uptake metabolism are important for microbial growth, suggesting the possible dysfunctional microbiota of Group C (Figure 3A). At the module and pathway levels, the distribution of Group C was lower than that of other two groups (Figures 3B,C). The low distribution of these metabolic catalogs in Group C suggests that the metabolic ability of gut microbiota in Group C was weak, and the microbiota of patients in Group C could be imbalanced (Figure 3B) (Gagnière et al., 2016). Some antimicrobial resistance genes were identified in some samples, and these included the antibiotic resistance genes for tetracycline, macrolide, beta-lactam, and aminoglycoside (Figure 4) (Feldgarden et al., 2019). The TPM value of tetracycline resistance gene in Group H was higher than the corresponding values in Groups A and C. For other antibiotic resistance genes, most TPM values of Group A were higher than the corresponding values of Groups C and H (Figure 4). The antibiotics tetracycline, macrolide, and beta-lactam are commonly used in clinical settings; however, their overuse could result in the high availability of corresponding antibiotic resistance genes in such samples.
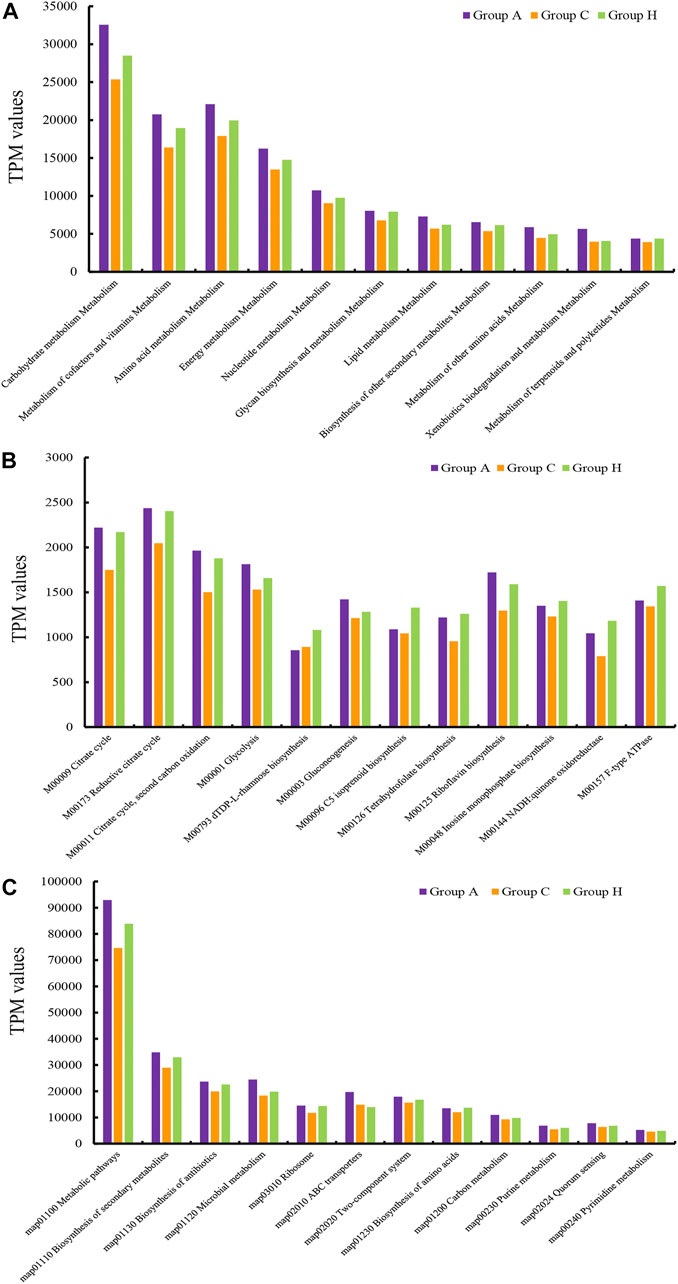
FIGURE 3. TPM values of the annotated ORFs at the (A) catalog level, (B) module level, and (C) pathway level.
Discussion
Colorectal cancer is the third most common cancer and the second leading cause of cancer-related mortality, which suggests the importance of investigating the molecular mechanisms of colorectal carcinogenesis (Sung et al., 2021). Environmental factors, particularly gut microbes, play pivotal roles in causing colorectal diseases (Czene et al., 2002; Song and Chan, 2019). Core microbiota has been found in the gut microbiota of patients with colorectal cancer, and a study recovered the microbiota of patients with adenoma (Click et al., 2018; Saito et al., 2019). Colorectal cancer typically develops from adenoma, whereas hemorrhoids are a common colorectal disease. Comparison of these colorectal diseases may provide insights into the causative factors for colorectal carcinogenesis (Wong and Yu, 2019). In this study, we used 16S rRNA gene amplicon and SMS strategies to investigate the gut microbiota of patients with colorectal cancer, adenoma, and hemorrhoids.
Microbial profiling revealed higher diversity in Group C than in other groups, which is consistent with previous results that indicated higher microbiota diversity in patients with colorectal cancer than in healthy individuals (Tilg et al., 2018; Reis et al., 2019). The difference in microbiota diversity could be attributed to the increase in microbial diversity due to dysbiosis of gut microbiota of patients with colorectal cancer (Gao et al., 2015). The richness based on SMS data was higher than that based on 16S rRNA gene amplicon data. Metagenomic data can provide species-level microbial information, but it can lead to the overestimation of microbial richness (Wang et al., 2015). Some driver and passenger microbes were identified in the samples, but the percentages of certain known passenger species, such as F. nucleatum, were low (Tjalsma et al., 2012; Wong and Yu, 2019). Different ethnic groups of patients living in different areas may have diverse gut microbiota (Dai et al., 2018; Deschasaux et al., 2018; Verhaar et al., 2020). Therefore, it is possible that unknown passenger species may be present in patients of central China with colorectal disease. Although certain potential species were identified according to the network analysis, further studies based on pure cultures isolated from the gut microbiota are required to investigate their functional roles (Lagier et al., 2016; Bilen et al., 2018). In future, verification of the keystone taxa in colorectal carcinogenesis could help in identifying biomarkers for the diagnosis and prevention of colorectal diseases, including colorectal cancer (Lagier et al., 2016; Bilen et al., 2018). Moreover, modulation of the colorectal disease microbiota with probiotics or other strategies will confer health benefits to humans, which will be a value addition to the treatment paradigm (Durack and Lynch, 2019; Peng et al., 2020).
The gut microbiota is affected by diet and other environmental factors (Grazioso et al., 2019; Illiano et al., 2020). The functional profiles of the gut microbiota in the groups were different, and the metabolic catalogs of Group C were lower than those of Groups A and H. This finding indicates that the high microbial diversity of Group C could have caused dysbiosis of the microbial metabolism (Gagnière et al., 2016; Song and Chan, 2019). The identification of certain pathogenic microbes, such as K. pneumoniae and S. pasteurianus, in the samples revealed the potential of microbial analyses to recognize infective microbes in patients (Gu et al., 2021). Diverse antibiotic resistance genes were identified in SMS data. This could be due to the fact that antibiotics were administered to the hospitalized patients (Bullman et al., 2017; Cao et al., 2018). Prevotella and other gut microbes can be identified in oral microbiota (Yamashita and Takeshita, 2017; Qiao et al., 2020), and the oral microbiota of patients with colorectal diseases are different from that of healthy individuals (Russo et al., 2017; Flemer et al., 2018). Therefore, it is possible to classify or predict potential patients with colorectal diseases based on oral microbiota (Yamashita and Takeshita, 2017; Flemer et al., 2018). Moreover, diets can reshape the gut microbiota, implying that designing and implementing an optimized diet by using active components of plants can be an effective therapeutic strategy for colorectal diseases in the near future (Guan et al., 2020; Wang et al., 2020).
The metagenomic strategy had been widely used to identify keystone taxa in human microbiome (Saito et al., 2019). The metagenomic strategy is rapid and accurate, and could provide species- and gene-level information of the microbiota (Wang et al., 2015). However, the associated cost is high, and analysis of these data requires investment of massive computer resources (Zhu et al., 2021). The SMS approach is an affordable and rapid microbial detection strategy. Although phylum- and genus-level data are slightly different, SMS and 16S rRNA amplicon data provided similar microbial structures in this study. In addition, SMS data shed light into the species- and gene-level information, which can drive future identification of reliable microbial or gene biomarkers for diagnosis and treatment of colorectal diseases (Hillmann et al., 2018; Zhu et al., 2021).
In this study, we recovered gut microbiota from patients with three different colorectal diseases and identified the potential keystone taxa. In addition to pathogenic microbes in a few adenoma disease samples, certain passenger microbes could have roles in causing colorectal diseases. The 16S rRNA gene amplicon and SMS data provide similar alpha- and beta-diversity results, and SMS data may be applied for future clinical use in colorectal diseases. In future, designing an appropriate diet to modulate the gut microbiota may be beneficial for the prevention or treatment of colorectal diseases.
Data Availability Statement
The original contributions presented in the study are publicly available. This data can be found here: https://www.ncbi.nlm.nih.gov/bioproject/PRJNA725613.
Ethics Statement
The studies involving human participants were reviewed and approved by the institutional review boards of the First Affiliated Hospital, Zhengzhou University. The patients/participants provided their written informed consent to participate in this study.
Author Contributions
YW and BJ conceived the study. YuL, YeL, SW, and YY conducted experiments. YW, BJ, and XL analyzed the data. YW and BJ drafted the manuscript. All the authors read, revised, and approved the manuscript.
Funding
This work was supported by Henan Provincial Key Laboratory of Children's Genetics and Metabolic Diseases Foundation (NO. SS201909), the National Natural Science Foundation of China (No. 31800079 and 32111530179) and Clinical Laboratories, Shenyou Bio.
Conflict of Interest
XL was employed by Science China Press.
The remaining authors declare that the research was conducted in the absence of any commercial or financial relationships that could be construed as a potential conflict of interest.
Acknowledgments
We appreciate the linguistic assistance provided by TopEdit (www.topeditsci.com) during the preparation of this manuscript.
Supplementary Material
The Supplementary Material for this article can be found online at: https://www.frontiersin.org/articles/10.3389/fmolb.2021.703638/full#supplementary-material
References
Bilen, M., Dufour, J.-C., Lagier, J.-C., Cadoret, F., Daoud, Z., Dubourg, G., et al. (2018). The Contribution of Culturomics to the Repertoire of Isolated Human Bacterial and Archaeal Species. Microbiome 6, 94. doi:10.1186/s40168-018-0485-5
Bolger, A. M., Lohse, M., and Usadel, B. (2014). Trimmomatic: a Flexible Trimmer for Illumina Sequence Data. Bioinformatics 30, 2114–2120. doi:10.1093/bioinformatics/btu170
Brennan, C. A., and Garrett, W. S. (2019). Fusobacterium Nucleatum - Symbiont, Opportunist and Oncobacterium. Nat. Rev. Microbiol. 17, 156–166. doi:10.1038/s41579-018-0129-6
Brennan, C. A., and Garrett, W. S. (2016). Gut Microbiota, Inflammation, and Colorectal Cancer. Annu. Rev. Microbiol. 70, 395–411. doi:10.1146/annurev-micro-102215-095513
Bullman, S., Pedamallu, C. S., Sicinska, E., Clancy, T. E., Zhang, X., Cai, D., et al. (2017). Analysis ofFusobacteriumpersistence and Antibiotic Response in Colorectal Cancer. Science 358, 1443–1448. doi:10.1126/science.aal5240
Butt, J., Jenab, M., Werner, J., Fedirko, V., Weiderpass, E., Dahm, C. C., et al. (2021). Association of Pre-diagnostic Antibody Responses to Escherichia coli and Bacteroides Fragilis Toxin Proteins with Colorectal Cancer in a European Cohort. Gut Microbes 13, 1–14. doi:10.1080/19490976.2021.1903825
Cao, Y., Wu, K., Mehta, R., Drew, D. A., Song, M., Lochhead, P., et al. (2018). Long-term Use of Antibiotics and Risk of Colorectal Adenoma. Gut 67, 672–678. doi:10.1136/gutjnl-2016-313413
Chung, L., Thiele Orberg, E., Geis, A. L., Chan, J. L., Fu, K., Destefano Shields, C. E., et al. (2018). Bacteroides Fragilis Toxin Coordinates a Pro-carcinogenic Inflammatory Cascade via Targeting of Colonic Epithelial Cells. Cell Host Microbe. 23, 203–214. doi:10.1016/j.chom.2018.01.007
Click, B., Pinsky, P. F., Hickey, T., Doroudi, M., and Schoen, R. E. (2018). Association of Colonoscopy Adenoma Findings With Long-Term Colorectal Cancer Incidence. Jama 319, 2021–2031. doi:10.1001/jama.2018.5809
Cole, J. R., Wang, Q., Fish, J. A., Chai, B., Mcgarrell, D. M., Sun, Y., et al. (2014). Ribosomal Database Project: Data and Tools for High Throughput rRNA Analysis. Nucl. Acids Res. 42, D633–D642. doi:10.1093/nar/gkt1244
Corley, D. A., Jensen, C. D., Marks, A. R., Zhao, W. K., Lee, J. K., Doubeni, C. A., et al. (2014). Adenoma Detection Rate and Risk of Colorectal Cancer and Death. N. Engl. J. Med. 370, 1298–1306. doi:10.1056/nejmoa1309086
Cuevas-Ramos, G., Petit, C. R., Marcq, I., Boury, M., Oswald, E., and Nougayrède, J.-P. (2010). Escherichia coli Induces DNA Damage In Vivo and Triggers Genomic Instability in Mammalian Cells. Proc. Natl. Acad. Sci. 107, 11537–11542. doi:10.1073/pnas.1001261107
Czene, K., Lichtenstein, P., and Hemminki, K. (2002). Environmental and Heritable Causes of Cancer Among 9.6 Million Individuals in the Swedish Family-Cancer Database. Int. J. Cancer 99, 260–266. doi:10.1002/ijc.10332
Dai, Z., Coker, O. O., Nakatsu, G., Wu, W. K. K., Zhao, L., Chen, Z., et al. (2018). Multi-cohort Analysis of Colorectal Cancer Metagenome Identified Altered Bacteria across Populations and Universal Bacterial Markers. Microbiome 6, 70. doi:10.1186/s40168-018-0451-2
De Filippis, F., Pasolli, E., Tett, A., Tarallo, S., Naccarati, A., De Angelis, M., et al. (2019). Distinct Genetic and Functional Traits of Human Intestinal Prevotella Copri Strains Are Associated with Different Habitual Diets. Cell Host Microbe 25, 444–453. doi:10.1016/j.chom.2019.01.004
Deschasaux, M., Bouter, K. E., Prodan, A., Levin, E., Groen, A. K., Herrema, H., et al. (2018). Depicting the Composition of Gut Microbiota in a Population with Varied Ethnic Origins but Shared Geography. Nat. Med. 24, 1526–1531. doi:10.1038/s41591-018-0160-1
Durack, J., and Lynch, S. V. (2019). The Gut Microbiome: Relationships with Disease and Opportunities for Therapy. J. Exp. Med. 216, 20–40. doi:10.1084/jem.20180448
Feldgarden, M., Brover, V., Haft, D. H., Prasad, A. B., Slotta, D. J., Tolstoy, I., et al. (2019). Validating the AMRFinder Tool and Resistance Gene Database by Using Antimicrobial Resistance Genotype-Phenotype Correlations in a Collection of Isolates. Antimicrob. Agents Chemother. 63. doi:10.1128/AAC.00483-19
Flemer, B., Lynch, D. B., Brown, J. M. R., Jeffery, I. B., Ryan, F. J., Claesson, M. J., et al. (2017). Tumour-associated and Non-tumour-associated Microbiota in Colorectal Cancer. Gut 66, 633–643. doi:10.1136/gutjnl-2015-309595
Flemer, B., Warren, R. D., Barrett, M. P., Cisek, K., Das, A., Jeffery, I. B., et al. (2018). The Oral Microbiota in Colorectal Cancer Is Distinctive and Predictive. Gut 67, 1454–1463. doi:10.1136/gutjnl-2017-314814
Gagnière, J., Raisch, J., Veziant, J., Barnich, N., Bonnet, R., Buc, E., et al. (2016). Gut Microbiota Imbalance and Colorectal Cancer. World J. Gastroenterol. 22, 501–518. doi:10.3748/wjg.v22.i2.501
Gao, Z., Guo, B., Gao, R., Zhu, Q., and Qin, H. (2015). Microbiota Disbiosis Is Associated with Colorectal Cancer. Front. Microbiol. 6. doi:10.3389/fmicb.2015.00020
Goodwin, A. C., Shields, C. E. D., Wu, S., Huso, D. L., Wu, X., Murray-Stewart, T. R., et al. (2011). Polyamine Catabolism Contributes to Enterotoxigenic Bacteroides Fragilis-Induced colon Tumorigenesis. Proc. Natl. Acad. Sci. 108, 15354–15359. doi:10.1073/pnas.1010203108
Grazioso, T. P., Brandt, M., and Djouder, N. (2019). Diet, Microbiota, and Colorectal Cancer. iScience 21, 168–187. doi:10.1016/j.isci.2019.10.011
Gu, W., Deng, X., Lee, M., Sucu, Y. D., Arevalo, S., Stryke, D., et al. (2021). Rapid Pathogen Detection by Metagenomic Next-Generation Sequencing of Infected Body Fluids. Nat. Med. 27, 115–124. doi:10.1038/s41591-020-1105-z
Guan, R., Wang, M., Guan, Z., Jin, C.-Y., Lin, W., Ji, X., et al. (2020). Metabolic Engineering for Glycyrrhetinic Acid Production in Saccharomyces cerevisiae. Front. Bioeng. Biotechnol. 8, 1318. doi:10.3389/fbioe.2020.588255
Haghi, F., Goli, E., Mirzaei, B., and Zeighami, H. (2019). The Association between Fecal Enterotoxigenic B. Fragilis with Colorectal Cancer. BMC Cancer 19, 879. doi:10.1186/s12885-019-6115-1
Hillmann, B., Al-Ghalith, G. A., Shields-Cutler, R. R., Zhu, Q., Gohl, D. M., Beckman, K. B., et al. (2018). Evaluating the Information Content of Shallow Shotgun Metagenomics. mSystems 3. doi:10.1128/mSystems.00069-18
Huerta-Cepas, J., Forslund, K., Coelho, L. P., Szklarczyk, D., Jensen, L. J., Von Mering, C., et al. (2017). Fast Genome-Wide Functional Annotation through Orthology Assignment by eggNOG-Mapper. Mol. Biol. Evol. 34, 2115–2122. doi:10.1093/molbev/msx148
Illiano, P., Brambilla, R., and Parolini, C. (2020). The Mutual Interplay of Gut Microbiota, Diet and Human Disease. Febs j 287, 833–855. doi:10.1111/febs.15217
Jandhyala, S. M., Talukdar, R., Subramanyam, C., Vuyyuru, H., Sasikala, M., and Nageshwar Reddy, D. (2015). Role of the normal Gut Microbiota. World J. Gastroenterol. 21, 8787–8803. doi:10.3748/wjg.v21.i29.8787
Jiang, S., Zhang, Y., Jin, J., Wu, Y., Wei, Y., Wang, X., et al. (2020). Organic Carbon in a Seepage Face of a Subterranean Estuary: Turnover and Microbial Interrelations. Sci. Total Environ. 725, 138220. doi:10.1016/j.scitotenv.2020.138220
Lagier, J.-C., Khelaifia, S., Alou, M. T., Ndongo, S., Dione, N., Hugon, P., et al. (2016). Culture of Previously Uncultured Members of the Human Gut Microbiota by Culturomics. Nat. Microbiol. 1, 16203. doi:10.1038/nmicrobiol.2016.203
Laudadio, I., Fulci, V., Palone, F., Stronati, L., Cucchiara, S., and Carissimi, C. (2018). Quantitative Assessment of Shotgun Metagenomics and 16S rDNA Amplicon Sequencing in the Study of Human Gut Microbiome. OMICS: A J. Integr. Biol. 22, 248–254. doi:10.1089/omi.2018.0013
Li, Y., Wang, J., Wang, M., Gao, Y., Jin, C.-Y., Shi, X., et al. (2021). Microbial Profiling Identifies Potential Key Drivers in Gastric Cancer Patients. Biotechnol. Biotechnological Equipment 35, 496–503. doi:10.1080/13102818.2021.1896384
Liang, J., Mai, W., Tang, J., and Wei, Y. (2019). Highly Effective Treatment of Petrochemical Wastewater by a Super-sized Industrial Scale Plant with Expanded Granular Sludge Bed Bioreactor and Aerobic Activated Sludge. Chem. Eng. J. 360, 15–23. doi:10.1016/j.cej.2018.11.167
Liang, J., Mai, W., Wang, J., Li, X., Su, M., Du, J., et al. (2021). Performance and Microbial Communities of a Novel Integrated Industrial-Scale Pulp and Paper Wastewater Treatment Plant. J. Clean. Prod. 278, 123896. doi:10.1016/j.jclepro.2020.123896
Lichtenstein, P., Holm, N. V., Verkasalo, P. K., Iliadou, A., Kaprio, J., Koskenvuo, M., et al. (2000). Environmental and Heritable Factors in the Causation of Cancer - Analyses of Cohorts of Twins from Sweden, Denmark, and Finland. N. Engl. J. Med. 343, 78–85. doi:10.1056/nejm200007133430201
Lopez, L. R., Bleich, R. M., and Arthur, J. C. (2021). Microbiota Effects on Carcinogenesis: Initiation, Promotion, and Progression. Annu. Rev. Med. 72, 243–261. doi:10.1146/annurev-med-080719-091604
Mai, W., Hu, T., Li, C., Wu, R., Chen, J., Shao, Y., et al. (2020). Effective Nitrogen Removal of Wastewater from Vitamin B2 Production by a Potential Anammox Process. J. Water Process Eng. 37, 101515. doi:10.1016/j.jwpe.2020.101515
Nagpal, S., Singh, R., Yadav, D., and Mande, S. S. (2020). MetagenoNets: Comprehensive Inference and Meta-Insights for Microbial Correlation Networks. Nucleic Acids Res. 48, W572–W579. doi:10.1093/nar/gkaa254
Nurk, S., Meleshko, D., Korobeynikov, A., and Pevzner, P. A. (2017). metaSPAdes: a New Versatile Metagenomic Assembler. Genome Res. 27, 824–834. doi:10.1101/gr.213959.116
Peng, M., Tabashsum, Z., Patel, P., Bernhardt, C., Biswas, C., Meng, J., et al. (2020). Prevention of Enteric Bacterial Infections and Modulation of Gut Microbiota with Conjugated Linoleic Acids Producing Lactobacillus in Mice. Gut Microbes 11, 433–452. doi:10.1080/19490976.2019.1638724
Peterson, D., Bonham, K. S., Rowland, S., Pattanayak, C. W., and Klepac-Ceraj, V. (2021). Comparative Analysis of 16S rRNA Gene and Metagenome Sequencing in Pediatric Gut Microbiomes. bioRxiv. doi:10.1101/2021.02.20.432118
Pleguezuelos-Manzano, C., Puschhof, J., Puschhof, J., Rosendahl Huber, A., van Hoeck, A., Wood, H. M., et al. (2020). Mutational Signature in Colorectal Cancer Caused by Genotoxic Pks+ E. coli. Nature 580, 269–273. doi:10.1038/s41586-020-2080-8
Qiao, S., Wu, D., Wang, M., Qian, S., Zhu, Y., Shi, J., et al. (2020). Oral Microbial Profile Variation during Canine Ligature-Induced Peri-Implantitis Development. BMC Microbiol. 20, 293. doi:10.1186/s12866-020-01982-6
Rajamäki, K., Taira, A., Katainen, R., Välimäki, N., Kuosmanen, A., Plaketti, R. M., et al. (2021). Genetic and Epigenetic Characteristics of Inflammatory Bowel Disease Associated Colorectal Cancer. Gastroenterology. doi:10.1053/j.gastro.2021.04.042
Rausch, P., Rühlemann, M., Hermes, B. M., Doms, S., Dagan, T., Dierking, K., et al. (2019). Comparative Analysis of Amplicon and Metagenomic Sequencing Methods Reveals Key Features in the Evolution of Animal Metaorganisms. Microbiome 7 (1), 133. doi:10.1186/s40168-019-0743-1
Reis, S. A. d., da Conceição, L. L., and Peluzio, M. d. C. G. (2019). Intestinal Microbiota and Colorectal Cancer: Changes in the Intestinal Microenvironment and Their Relation to the Disease. J. Med. Microbiol. 68, 1391–1407. doi:10.1099/jmm.0.001049
Rubinstein, M. R., Baik, J. E., Lagana, S. M., Han, R. P., Raab, W. J., Sahoo, D., et al. (2019). Fusobacterium Nucleatum Promotes Colorectal Cancer by Inducing Wnt/β-Catenin Modulator Annexin A1. EMBO Rep. 20. doi:10.15252/embr.201847638
Russo, E., Bacci, G., Chiellini, C., Fagorzi, C., Niccolai, E., Taddei, A., et al. (2017). Preliminary Comparison of Oral and Intestinal Human Microbiota in Patients with Colorectal Cancer: A Pilot Study. Front. Microbiol. 8, 2699. doi:10.3389/fmicb.2017.02699
Saito, K., Koido, S., Odamaki, T., Kajihara, M., Kato, K., Horiuchi, S., et al. (2019). Metagenomic Analyses of the Gut Microbiota Associated with Colorectal Adenoma. PLoS One 14, e0212406. doi:10.1371/journal.pone.0212406
Santiago-Rodriguez, T. M., Garoutte, A., Adams, E., Nasser, W., Ross, M. C., La Reau, A., et al. (2020). Metagenomic Information Recovery from Human Stool Samples Is Influenced by Sequencing Depth and Profiling Method. Genes (Basel) 11. doi:10.3390/genes11111380
Schmitt, M., and Greten, F. R. (2021). The Inflammatory Pathogenesis of Colorectal Cancer. Nat. Rev. Immunol. doi:10.1038/s41577-021-00534-x
Sears, C. L., Geis, A. L., and Housseau, F. (2014). Bacteroides Fragilis Subverts Mucosal Biology: from Symbiont to colon Carcinogenesis. J. Clin. Invest. 124, 4166–4172. doi:10.1172/jci72334
Shang, F.-M., and Liu, H.-L. (2018). Fusobacterium Nucleatumand Colorectal Cancer: A Review. World J. Gastrointest. Oncol. 10, 71–81. doi:10.4251/wjgo.v10.i3.71
Shi, X.-J., Wei, Y., and Ji, B. (2020). Systems Biology of Gastric Cancer: Perspectives on the Omics-Based Diagnosis and Treatment. Front. Mol. Biosci. 7, 203. doi:10.3389/fmolb.2020.00203
Song, M., and Chan, A. T. (2019). Environmental Factors, Gut Microbiota, and Colorectal Cancer Prevention. Clin. Gastroenterol. Hepatol. 17, 275–289. doi:10.1016/j.cgh.2018.07.012
Song, M., Chan, A. T., and Sun, J. (2020). Influence of the Gut Microbiome, Diet, and Environment on Risk of Colorectal Cancer. Gastroenterology 158, 322–340. doi:10.1053/j.gastro.2019.06.048
Sung, H., Ferlay, J., Siegel, R. L., Laversanne, M., Soerjomataram, I., Jemal, A., et al. (2021). Global Cancer Statistics 2020: GLOBOCAN Estimates of Incidence and Mortality Worldwide for 36 Cancers in 185 Countries. CA Cancer J. Clin. 71 (3), 209–249. doi:10.3322/caac.21660
Tett, A., Huang, K. D., Asnicar, F., Fehlner-Peach, H., Pasolli, E., Karcher, N., et al. (2019). The Prevotella Copri Complex Comprises Four Distinct Clades Underrepresented in Westernized Populations. Cell Host & Microbe 26, 666–679. doi:10.1016/j.chom.2019.08.018
Tilg, H., Adolph, T. E., Gerner, R. R., and Moschen, A. R. (2018). The Intestinal Microbiota in Colorectal Cancer. Cancer Cell 33, 954–964. doi:10.1016/j.ccell.2018.03.004
Tjalsma, H., Boleij, A., Marchesi, J. R., and Dutilh, B. E. (2012). A Bacterial Driver-Passenger Model for Colorectal Cancer: beyond the Usual Suspects. Nat. Rev. Microbiol. 10, 575–582. doi:10.1038/nrmicro2819
Verhaar, B. J. H., Collard, D., Prodan, A., Levels, J. H. M., Zwinderman, A. H., Bäckhed, F., et al. (2020). Associations between Gut Microbiota, Faecal Short-Chain Fatty Acids, and Blood Pressure across Ethnic Groups: the HELIUS Study. Eur. Heart J. 41, 4259–4267. doi:10.1093/eurheartj/ehaa704
Wang, B., Yao, M., Lv, L., Ling, Z., and Li, L. (2017). The Human Microbiota in Health and Disease. Engineering 3, 71–82. doi:10.1016/j.eng.2017.01.008
Wang, M., Wei, Y., Ji, B., and Nielsen, J. (2020). Advances in Metabolic Engineering of Saccharomyces cerevisiae for Cocoa Butter Equivalent Production. Front. Bioeng. Biotechnol. 8. doi:10.3389/fbioe.2020.594081
Wang, W.-L., Xu, S.-Y., Ren, Z.-G., Tao, L., Jiang, J.-W., and Zheng, S.-S. (2015). Application of Metagenomics in the Human Gut Microbiome. World J. Gastroenterol. 21, 803–814. doi:10.3748/wjg.v21.i3.803
Wassenaar, T. M. (2018). E. coli and Colorectal Cancer: a Complex Relationship that Deserves a Critical Mindset. Crit. Rev. Microbiol. 44, 619–632. doi:10.1080/1040841x.2018.1481013
Wei, Y., Ji, B., Siewers, V., Xu, D., Halkier, B. A., and Nielsen, J. (2019). Identification of Genes Involved in Shea Butter Biosynthesis from Vitellaria Paradoxa Fruits through Transcriptomics and Functional Heterologous Expression. Appl. Microbiol. Biotechnol. 103, 3727–3736. doi:10.1007/s00253-019-09720-3
Wei, Y., Ren, T., and Zhang, L. (2020a). Dix-seq: An Integrated Pipeline for Fast Amplicon Data Analysis. bioRxiv. doi:10.1101/2020.05.11.089748,
Wei, Y., Wu, Y., Zhang, L., Zhou, Z., Zhou, H., and Yan, X. (2020b). Genome Recovery and Metatranscriptomic Confirmation of Functional Acetate-Oxidizing Bacteria from Enriched Anaerobic Biogas Digesters. Environ. Pollut., 265, 114843. doi:10.1016/j.envpol.2020.114843
Wirbel, J., Pyl, P. T., Kartal, E., Zych, K., Kashani, A., Milanese, A., et al. (2019). Meta-analysis of Fecal Metagenomes Reveals Global Microbial Signatures that Are Specific for Colorectal Cancer. Nat. Med. 25, 679–689. doi:10.1038/s41591-019-0406-6
Wong, S. H., and Yu, J. (2019). Gut Microbiota in Colorectal Cancer: Mechanisms of Action and Clinical Applications. Nat. Rev. Gastroenterol. Hepatol. 16, 690–704. doi:10.1038/s41575-019-0209-8
Xing, C., Wang, M., Ajibade, A. A., Tan, P., Fu, C., Chen, L., et al. (2021). Microbiota Regulate Innate Immune Signaling and Protective Immunity against Cancer. Cell Host Microbe 29 (6), 959–974. doi:10.1016/j.chom.2021.03.016
Yachida, S., Mizutani, S., Shiroma, H., Shiba, S., Nakajima, T., Sakamoto, T., et al. (2019). Metagenomic and Metabolomic Analyses Reveal Distinct Stage-specific Phenotypes of the Gut Microbiota in Colorectal Cancer. Nat. Med. 25, 968–976. doi:10.1038/s41591-019-0458-7
Yamashita, Y., and Takeshita, T. (2017). The Oral Microbiome and Human Health. J. Oral Sci. 59, 201–206. doi:10.2334/josnusd.16-0856
Keywords: gut microbiota, colorectal diseases, 16S amplicon, network analysis, shallow metagenomic sequencing
Citation: Liu Y, Li X, Yang Y, Liu Y, Wang S, Ji B and Wei Y (2021) Exploring Gut Microbiota in Patients with Colorectal Disease Based on 16S rRNA Gene Amplicon and Shallow Metagenomic Sequencing. Front. Mol. Biosci. 8:703638. doi: 10.3389/fmolb.2021.703638
Received: 30 April 2021; Accepted: 15 June 2021;
Published: 09 July 2021.
Edited by:
Shuyi Zhang, Tsinghua University, ChinaReviewed by:
Yong Liu, Xuzhou Medical University, ChinaShuangling Luo, The Sixth Affiliated Hospital of Sun Yat-sen University, China
Copyright © 2021 Liu, Li, Yang, Liu, Wang, Ji and Wei. This is an open-access article distributed under the terms of the Creative Commons Attribution License (CC BY). The use, distribution or reproduction in other forums is permitted, provided the original author(s) and the copyright owner(s) are credited and that the original publication in this journal is cited, in accordance with accepted academic practice. No use, distribution or reproduction is permitted which does not comply with these terms.
*Correspondence: Yongjun Wei, eW9uZ2p1bndlaUB6enUuZWR1LmNu