- 1Department of Breast Surgery, General Surgery, Qilu Hospital, Cheeloo College of Medicine, Shandong University, Jinan, China
- 2Department of Pathology, Qilu Hospital, Cheeloo College of Medicine, Shandong University, Jinan, China
- 3Department of Radiation Oncology, Shandong Cancer Hospital and Institute, Shandong First Medical University and Shandong Academy of Medical Sciences, Jinan, China
Background: Circular RNAs (circRNAs) have been shown to play important roles in the development and progression of human cancers. Emerging evidence shows that circRNAs have the potential to be promising biomarkers for cancer diagnosis and prognosis. However, the roles of circRNAs in breast cancer axillary lymph node metastasis (ALNM) remain to be determined.
Methods: Transcriptome sequencing was utilized to screen the differentially expressed circRNAs in three breast cancer tissues with ALNM and three without ALNM. Differentially expressed circRNAs were further verified by quantitative real-time PCR. Moreover, receiver operating characteristic (ROC) curve analysis was performed to calculate the value of circRNAs to distinguish breast cancer tissues with ALNM and those without ALNM. To explore the potential mechanism of the circRNAs, a circRNA–miRNA–mRNA network was constructed based on the CircInteractome, circBank, and mirDIP online software.
Results: In total, 31 differentially expressed circRNAs were identified by transcriptome sequencing; among them, 21 were upregulated and 10 were downregulated in breast cancer with ALNM compared to those without ALNM. Circ_0000160 was validated to be downregulated in breast cancer tissues with ALNM compared with those without ALNM. The ROC curve showed the ability of circ_0000160 to distinguish breast cancer tissues with ALNM and those without ALNM, with an area under the curve of 0.7435. Furthermore, bioinformatics analysis revealed that the predicted mRNAs for circ_0000160 may be related to lymph node metastasis. The predicted mRNAs for circ_0000160 may be involved in many cancer-related pathways.
Conclusion: A decreased expression of circ_0000160 was found in breast cancer with axillary lymph node metastasis. Circ_0000160 may have the potential to distinguish breast cancer with axillary lymph node metastasis from those without axillary lymph node metastasis.
Introduction
Breast cancer is one of the most common malignant tumors and the leading cause of cancer-related death in women. For breast cancer, deaths are usually caused not by the primary tumor but by metastases (Wang et al., 2020; Kim, 2021). Axillary lymph node metastasis (ALNM) is one of the most important prognostic indicators and a crucial component in the staging system (Sawaki et al., 2019) Metastasis to lymph nodes is a high-risk factor for relapse and poor survival (Si et al., 2019). Additionally, identifying metastasis is essential for treatment regimens (Choi et al., 2017). Currently, ideal biomarkers are needed for ALNM prediction (Lee et al., 2018; Chang et al., 2020).
Circular RNAs (circRNAs) are single-stranded, covalently closed RNA molecules and are the downstream products of precursor mRNA back-splicing (Vo et al., 2019). Their high abundance, stability, evolutionary conservation among species, and high cell and tissue specificity endow circRNAs with the potential as molecular biomarkers for cancer diagnosis (Wang S. et al., 2021; Wei et al., 2021). For example, circEHD2, circENGLN3, and circNETO2 were found to be upregulated in clear cell renal carcinoma and showed diagnostic and prognostic values (Frey et al., 2021). Recently, Mani et al. identified hsa_circ_0006743 and hsa_circ_0002496 to be significantly upregulated in early-stage breast cancer tissues compared to normal samples (Rao et al., 2021). We have previously found that circ_0000745, circ_0001531, and circ_0001640 were upregulated in breast cancer, compared with benign tumor and healthy control (Wang Y.-W. et al., 2021). However, the profile of ALNM-related circRNAs in breast cancer has not been well established.
In this study, we investigated the profile of circRNAs using transcriptome sequencing and quantitative real-time PCR (qRT–PCR) in breast cancer tissues with and without ALNM.
Materials and Methods
Tissue Samples
For transcriptome sequencing, breast cancer tissues were collected from six patients, including three with ALNM and three without ALNM, at the Qilu Hospital of Shandong University (Shandong, China). The inclusion criteria were breast cancer patients who had undergone mastectomy, were pathologically diagnosed with ALNM or without ALNM, and signed informed consent. Patients who had preoperative chemotherapy or radiotherapy were excluded. Patients with ALNM were matched with patients without ALNM with respect to the timing of the sampling and duration of specimen storage. In the validation stage, another 56 breast cancer tissues (24 with ALNM and 32 without ALNM) were collected from the Qilu Hospital of Shandong University. Typical figures of hematoxylin and eosin (H&E)-stained sections of breast cancer with ALNM and without ALNM are provided in Supplementary Figure S1. All cases of breast cancer were evaluated histologically according to the WHO Classification of Tumors of the Breast (4th edition, 2012) and staged using the Tumor–Node–Metastasis (TNM) staging of the American Joint Committee on Cancer (AJCC, 7th edition, 2010). All fresh specimens were frozen in liquid nitrogen until further use. Written informed consent was obtained from all of the enrolled participants. The present study was approved by the Ethics Committee of the Qilu Hospital of Shandong University.
Transcriptome Sequencing for circRNA Profiling
Total RNA was isolated from breast cancer tissues using TRIzol reagent (Invitrogen, Carlsbad, CA, United States) according to the manufacturer’s protocol. RNA quality and quantity were measured on a Nanodrop spectrophotometer (ND-1000, Nanodrop Technologies), and RNA integrity was determined by gel electrophoresis. Total RNA was used to remove the rRNAs using Ribo-Zero rRNA Removal Kits (Illumina, United States) following the manufacturer’s instructions. RNA libraries were constructed by using rRNA-depleted RNAs with the TruSeq Stranded Total RNA Library Prep Kit (Illumina, United States) according to the manufacturer’s instructions. Libraries were controlled for quality and quantified using the BioAnalyzer 2,100 system (Agilent Technologies, United States). Ten pM libraries were denatured as single-stranded DNA molecules, captured on Illumina flow cells, amplified in situ as clusters, and finally sequenced for 150 cycles on an Illumina HiSeq Sequencer according to the manufacturer’s instructions. Paired-end reads were harvested from an Illumina HiSeq Sequencer after quality filtering. The reads were aligned to the reference genome/transcriptome with Bowtie2 software, and circRNAs were detected and identified with find_circ software. Raw junction reads for all samples were normalized by a total read number and log2 transformed. Differentially expressed circRNAs were identified by the t test between two groups. The transcriptome sequencing data were deposited in the Gene Expression Omnibus (GEO) database under the accession number GSE173766.
Quantitative Real-Time PCR
Briefly, total RNA was extracted from breast cancer tissues using TRIzol reagent (Invitrogen, Carlsbad, CA, United States) according to the manufacturer’s protocol. Reverse transcription was performed using the Invitrogen SuperScript III Reverse Transcriptase kit (Invitrogen). qRT–PCR was performed on a ViiA 7 Real-time PCR system (Applied Biosystems) using the AceQ qPCR SYBR Green Master Mix (Vazyme, Nanjing, China). ACTB levels were used to normalize the expression of circRNAs. The data were analyzed by the 2−ΔΔCT method. Related primers are shown in Supplementary Table S1.
CircRNA–miRNA–mRNA ceRNA (Competing Endogenous RNA) Network Analysis
The circRNA–miRNA interaction was predicted using CircInteractome (Dudekula et al., 2016) and circBank (Liu et al., 2019). miRNAs shared by the two software programs were selected for further mRNA predictions. The mRNAs targeted by miRNAs were predicted using mirDIP v4.1 (Tokar et al., 2018). The top 10 mRNA targets of miRNAs were first screened based on the integrated score provided by mirDIP. The circRNA–miRNA–mRNA ceRNA network was visualized by Cytoscape software (Shannon et al., 2003). KEGG pathway analysis for mRNAs was performed by the WebGestalt online tool (Liao et al., 2019), and the top 10 pathways based on the p value are presented.
Bioinformatics Analysis
For analysis of the predicted miRNAs, mRNAs and host gene for circ_0000160, miRNA, and mRNA expression profiles and the corresponding clinicopathological information [lymph node metastasis (LNM) status] of the breast cancer patients were obtained from The Cancer Genome Atlas (TCGA) (https://portal.gdc.cancer.gov/). For further analysis, the RNA-sequencing data were normalized as TPM (transcripts per kilobase of exon model per million mapped reads) for mRNA or reads of exon model per million mapped reads for miRNA. Log2-transformed normalized values were used for identification of differentially expressed genes between two groups (with LNM and without LNM) using the Wilcoxon rank sum test.
Statistical Analysis
Statistical analyses were performed using GraphPad Prism 5.0 (GraphPad Software, Inc., San Diego, CA, United States) and SPSS 20.0 (SPSS, Chicago, IL, United States). The significance of the differences was determined by a nonparametric test between two groups and one-way analysis of variance between multiple groups. The chi-square test or Fisher’s exact test was used, as appropriate, to analyze the relationship between the circRNA expression and clinicopathological variables. p < 0.05 was considered statistically significant.
Results
Expression Profiling of circRNAs in Breast Cancer With Axillary Lymph Node Metastasis and Without ALNM
To identify dysregulated circRNAs associated with breast cancer ALNM, transcriptome sequencing was performed on three breast cancer tissues with ALNM and three without ALNM (Figure 1A). In total, 31 circRNAs were found to be differentially expressed in breast cancer with ALNM vs. without ALNM (fold change >2, p < 0.05) (Figure 1B). Among the 31 circRNAs, 21 were upregulated and 10 were downregulated in breast cancer with ALNM compared with those without ALNM.
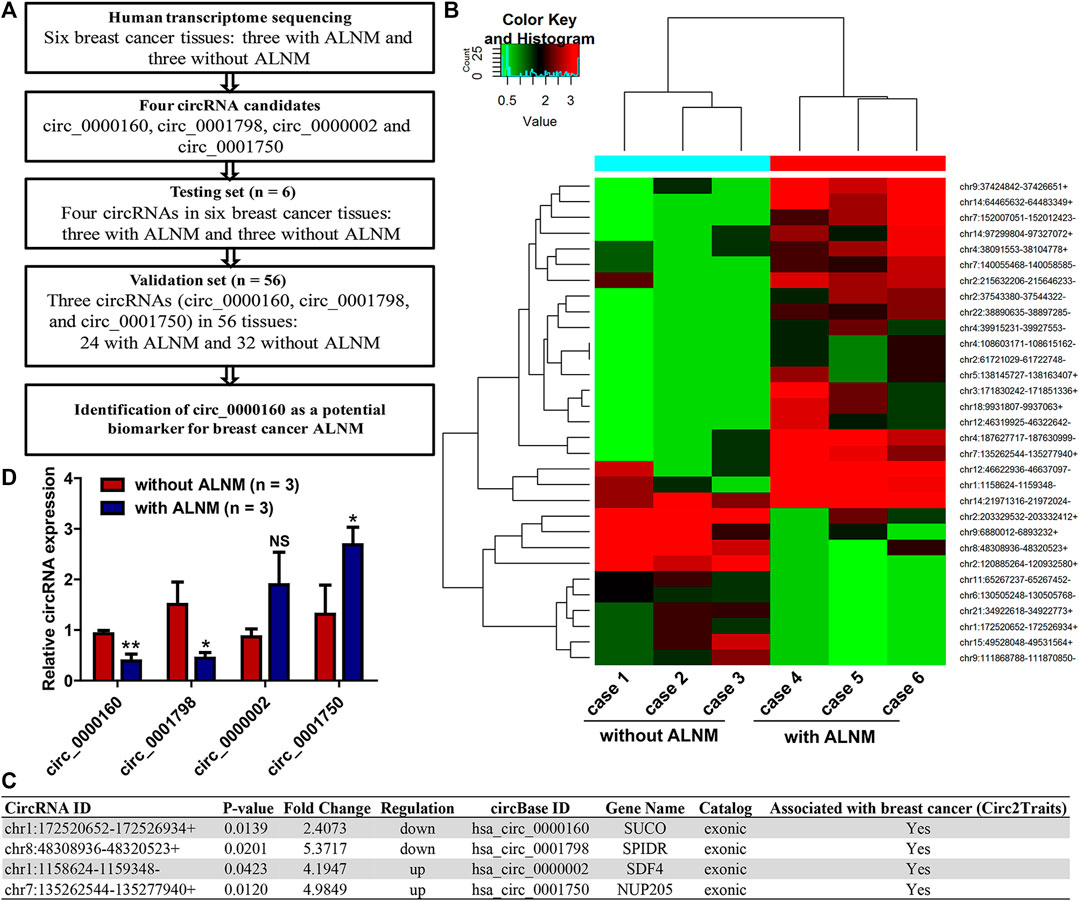
FIGURE 1. Screening axillary lymph node metastasis (ALNM)-related circRNAs in breast cancer using transcriptome sequencing. (A) The flow diagrams of the current study. (B) Human transcriptome sequencing was used to screen dysregulated circRNAs associated with breast cancer axillary ALNM in three breast cancer tissues with ALNM and three without ALNM. In total, 21 circRNAs were upregulated and 10 were downregulated in breast cancer with ALNM compared with those without ALNM. (C) Four circRNAs were selected for further investigation due to their high raw signal (junction reads) in transcriptome sequencing and potential association with breast cancer determined by the Circ2Traits database. (D) qRT–PCR was used to detect the expression of the four circRNAs in three breast cancer tissues with ALNM and three without ALNM. Circ_0000160 and circ_0001798 were downregulated, and circ_0001750 was upregulated in three breast cancer tissues with ALNM compared with three breast cancer tissues without ALNM (*p < 0.05, **p < 0.01). Circ_0000002 did not show significant differences between the two groups.
Next, we utilized Circ2Traits (Ghosal et al., 2013), a database collecting disease-associated circRNAs based on bioinformatics analysis and publications, to screen potential disease-associated circRNAs. Nine circRNAs were included in the Circ2Traits database, which were supposed to be associated with human cancers, cardiac hypertrophy, nonalcoholic fatty liver disease, Duchenne muscular dystrophy, and other human diseases. Finally, four of the nine circRNAs were selected for further investigation. These four circRNAs (upregulated: circ_0000002 and circ_0001750; downregulated: circ_0000160, circ_0001798) showed relatively high raw signals (junction reads) in transcriptome sequencing and were thought to be associated with breast cancer, as determined by the Circ2Traits database (Figure 1C).
Validation of Differentially Expressed circRNAs Using qRT–PCR
First, qRT–PCR was used to detect the expression of circ_0000002 and circ_0001750, circ_0000160, and circ_0001798 in the testing set (the six patients used in the transcriptome sequencing). The data showed that circ_0000160 and circ_0001798 were downregulated and that circ_0001750 was upregulated in three breast cancer tissues with ALNM compared with three breast cancer tissues without ALNM (Figure 1D, p < 0.05). The expression of circ_0000002 did not show significant differences between the two groups (Figure 1D).
Then, the differential expression of circ_0000160, circ_0001798, and circ_0001750 was further validated in a larger cohort containing 56 patients. Only circ_0000160 was downregulated in 24 breast cancer tissues with ALNM compared with 32 breast cancer tissues without ALNM (Figures 2A–C). The receiver operating characteristic (ROC) curve reflected strong separation between tissues with ALNM and those without ALNM, with an area under the curve (AUC) of 0.7435 (p = 0.0020) (Figure 2D). A decreasing trend of circ_0000160 was found in breast cancer tissues with more advanced N stages (increasing ALNM number, Figure 3A, one-way analysis of variance, p = 0.0157); however, the comparison for every two stages did not reach statistical significance (N1 vs. N2, p = 0.8520; N1 vs. N3, p = 0.4128; N2 vs. N3, p = 0.5176).
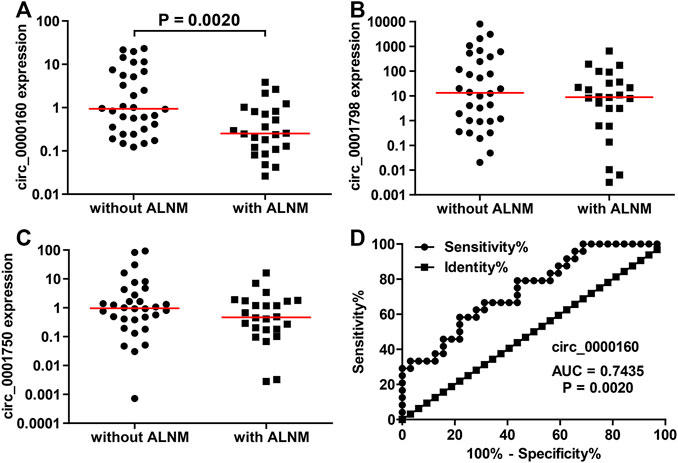
FIGURE 2. Decreased expression of circ_0000160 was found in breast cancer with ALNM in the validation cohort containing 56 patients. (A) Circ_0000160 was downregulated in 24 breast cancer tissues with ALNM compared to 32 tissues without ALNM. (B,C) Circ_0001798 and circ_0001750 did not show significant differences between the two groups. (D) ROC analysis showed that circ_0000160 had an area under the curve of 0.7435 (p = 0.0020) to discriminate tissues with ALNM from those without ALNM.
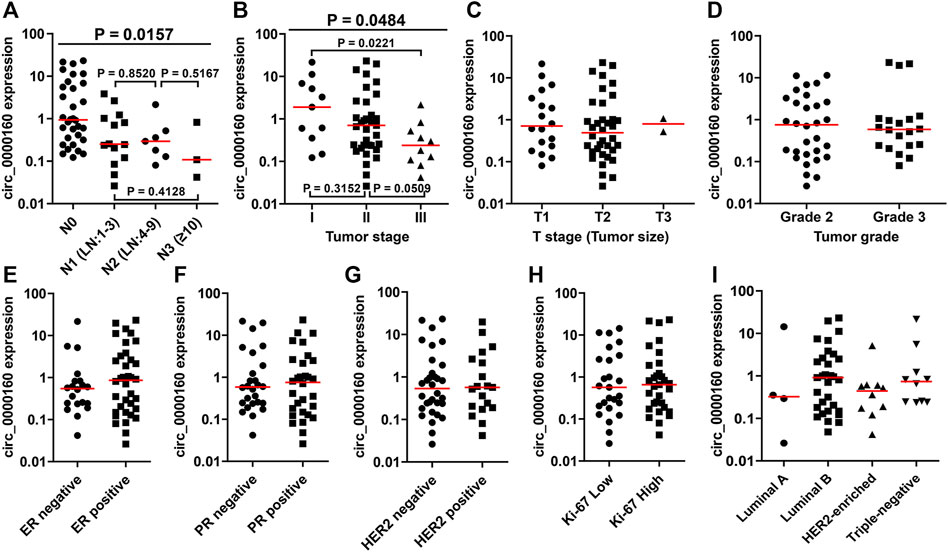
FIGURE 3. Association between the circ_0000160 expression and clinicopathological parameters in the validation cohort containing 56 patients. (A) A decreasing trend of circ_0000160 was found in breast cancer tissues with more advanced N stages (increasing ALNM number, one-way analysis of variance, p = 0.0157); however, the comparison for each two stages did not reach statistical significance (N1 vs. N2, p = 0.8520; N1 vs. N3, p = 0.4128; N2 vs. N3, p = 0.5176). (B) Circ_0000160 was even decreased in breast cancer with a more advanced tumor stage (one-way analysis of variance, p = 0.0484; stage I vs. stage II, p = 0.3152; II vs. III, p = 0.0509; I vs. III, p = 0.0221). (C)-(I) Circ_0000160 expression showed no relationship with the tumor size, tumor grade, ER/PR/HER2/Ki-67 expression, or molecular type (ER, estrogen receptor; PR, progesterone receptor).
Association Between the circRNA Expression and Clinicopathological Parameters of Breast Cancer Patients
To further determine the clinical significance of circRNAs, we analyzed the association between the circRNA expression and the clinicopathological parameters of breast cancer patients (Table 1). Here, the patients were divided into a high-expression group and a low-expression group based on the median expression value of circRNA. Our results showed that the low expression of circ_0000160 was correlated with axillary lymph node metastasis (p = 0.031). Additionally, circ_0000160 was even decreased in breast cancer with a more advanced tumor stage (Figure 3B, one-way analysis of variance, p = 0.0484; Stage I vs. Stage II, p = 0.3152; II vs. III, p = 0.0509; I vs. III, p = 0.0221). For circ_0001750, a higher expression was found in breast cancer tissues with a higher tumor grade, negative progesterone receptor (PR), or higher Ki-67 expression (Supplementary Figure S2; Table 1). No association was found between the circ_0001798 expression and any clinicopathological parameters (Supplementary Figure S3).
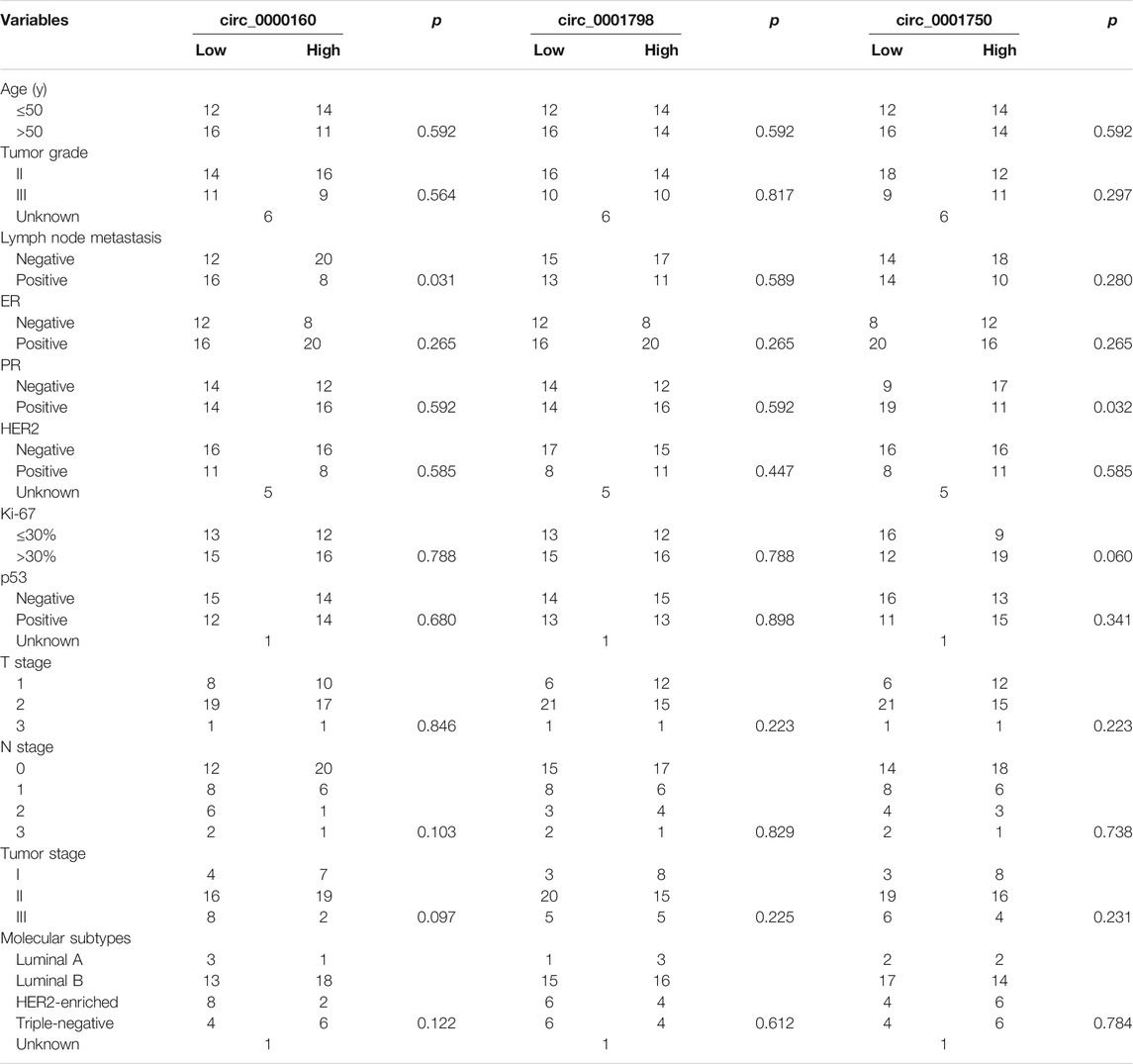
TABLE 1. Association between the circRNA expression and clinicopathologic features in breast cancer.
CircRNA–miRNA–mRNA ceRNA Network Construction
Circ_0000160 was subjected to further bioinformatics analysis to explore the potential mechanisms in breast cancer. Based on the prediction of CircInteractome and circBank, eight miRNAs (miR-607, miR-127-5p, miR-1272, miR-1299, miR-1183, miR-217, miR-767-3p, and miR-1256) could potentially interact with circ_0000160. Then, the mRNA targets of these miRNAs were predicted using mirDIP v4.1, and the top 10 mRNAs of each miRNA were included in the circRNA–miRNA–mRNA network construction (Figure 4). The potential targets of miRNAs were enriched in cancer-related pathways, such as pathways in cancer, the cell cycle, cellular senescence, and the JAK-STAT signaling pathway (Table 2).
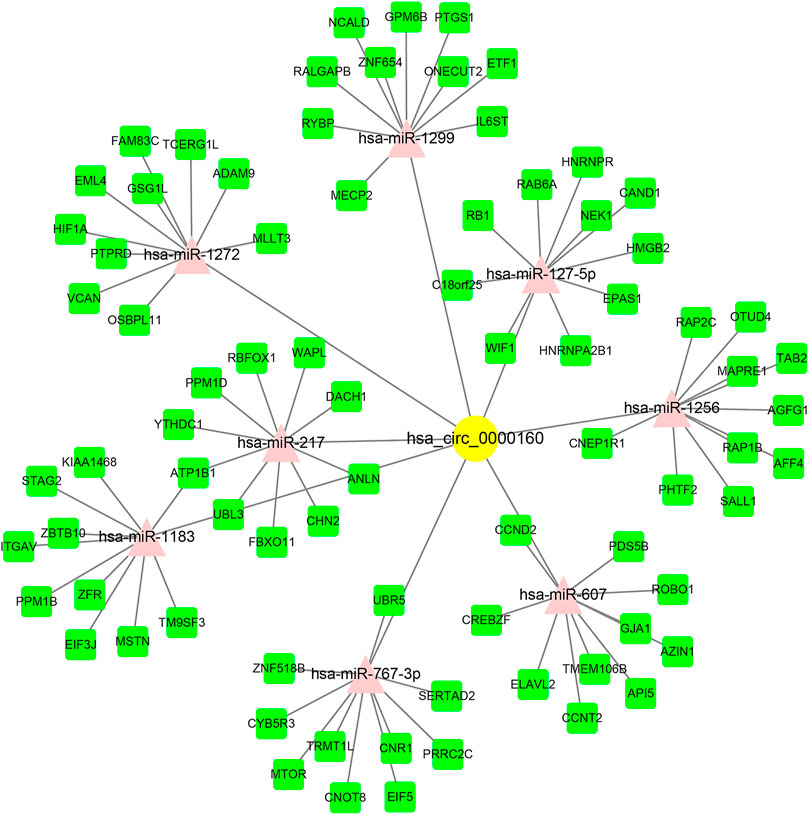
FIGURE 4. circRNA–miRNA–mRNA ceRNA network for circ_0000160. miRNAs were predicted by CircInteractome and circBank. mRNAs were predicted using mirDIP v4.1. The top 10 mRNA targets of each miRNA were selected based on the integrated score provided by mirDIP. Cytoscape software was used to visualize the network.
TCGA data were used to test whether the predicted miRNAs and mRNAs (and the host gene SUCO) for circ_0000160 are deregulated in breast cancers with lymph node metastasis. Among the predicted miRNAs and mRNAs, ADAM9, CAND1, CNOT8, DACH1, EIF3J, RAP2C, RB1, and VCAN were found to be deregulated in breast cancer with lymph node metastasis compared with those without lymph node metastasis (Figures 5A-H). Of note, DACH1 and VCAN showed obvious upregulation in breast cancer with lymph node metastasis. However, the host gene SUCO (Figure 5I) and the analyzed miRNAs (data not shown) showed no obvious difference in breast cancer with lymph node metastasis and those without lymph node metastasis.
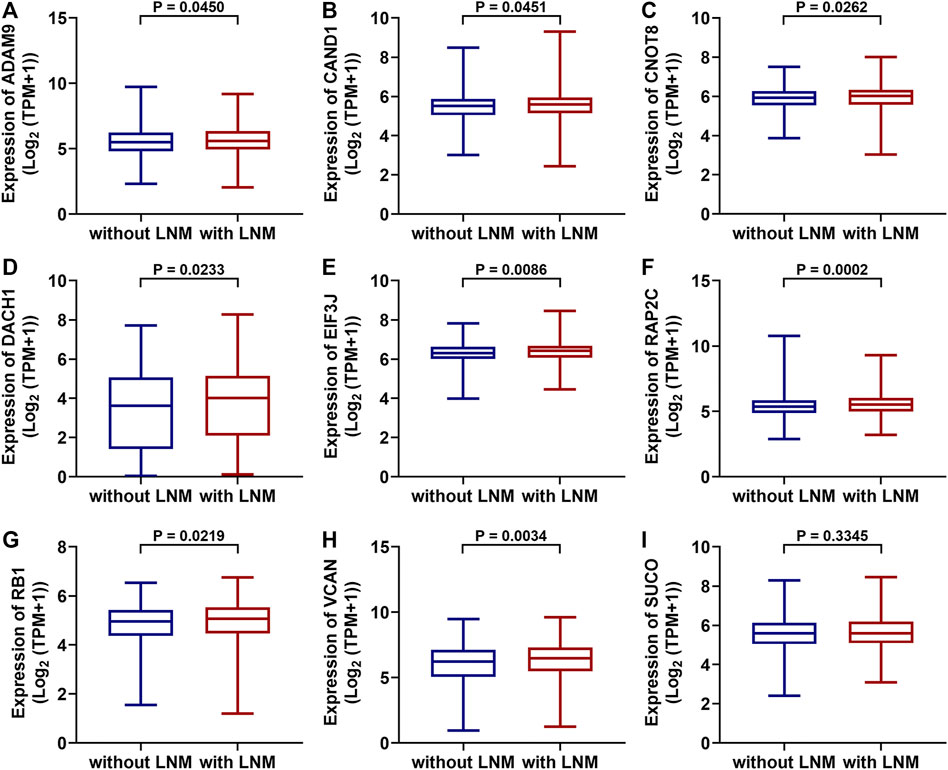
FIGURE 5. Differentially expressed mRNAs between breast cancer with lymph node metastasis and those without lymph node metastasis based on the TGCA dataset (BRCA). ADAM9, CAND1, CNOT8, DACH1, EIF3J, RAP2C, RB1, and VCAN were upregulated in breast cancer with lymph node metastasis (n = 551) compared with those without lymph node metastasis (A-H, n = 505). The SUCO expression showed no obvious difference in breast cancer with lymph node metastasis and those without lymph node metastasis (I).
Discussion
Breast cancer is one of the most common cancers. Recently, circRNAs have shown emerging promise as potential disease biomarkers (Verduci et al., 2021). Based on transcriptome sequencing followed by qRT–PCR confirmation, we identified circ_0000160 as significantly associated with ALNM in breast cancer. Circ_0000160 showed a relatively high predictive value to distinguish breast cancer patients with and without ALNM. It is noted that three pairs were evaluated by transcriptome sequencing as a discovery set, which may result in bias, which means that a small number of samples in high-throughput screening may produce “false positive” and “false negative” results. First, potentially differentially expressed circRNAs revealed by transcriptome sequencing may not be truly differential and should be further validated in a larger cohort. Second, we cannot exclude the possibility that there are other ALNM-related circRNAs other than the 31 circRNAs revealed by our transcriptome sequencing data. More ALNM-related circRNAs should be explored with a larger cohort using high-throughput technology.
Circ_0000160 is a novel circRNA that has not ever been investigated in human diseases, including cancers. The host gene SUCO (the SUN domain containing the ossification factor) has rarely been investigated in human cancers. Harland et al. found that mice lacking SUCO showed impaired bone formation and spontaneous fractures, and their results suggested SUCO as a candidate gene for brittle bone disorders (Sohaskey et al., 2010). Recently, exome sequencing identified SUCO mutations in mesial temporal lobe epilepsy, and a lack of SUCO led to abnormal development of neurons (Sha et al., 2015). SUCO was found to be upregulated in hepatocellular carcinoma (Yue et al., 2019) and may be targeted by miR-497 (Chen et al., 2021). Our data showed that circ_0000160 was downregulated, while the host gene SUCO showed no significant change in breast cancer with lymph node metastasis compared with those without lymph node metastasis. Recently, Kristensen et al. found that the expression of circRNAs does not often correlate well with the expression of host genes linearly (Kristensen et al., 2018). This argues that circRNAs are not merely steady-state byproducts of mRNA splicing but rather the product of a new type of regulated alternative splicing. We then analyzed the isoform expression data of the SUCO gene in breast cancer based on the GEPIA2 database (Tang et al., 2017). SUCO has 10 main isoforms (transcripts), and they showed distinct expression distributions in breast cancer (Supplementary Figure S4A). Overall, SUCO was upregulated in breast cancer tissues compared with normal breast tissues (Supplementary Figure S4B). However, some transcripts (e.g., ENST00000263688.3, circ_0000160 was derived from this transcript) were upregulated, some (e.g., ENST00000608566.1) showed no obvious change, and some (e.g., ENST00000609685.1) even showed a downregulation trend in breast cancer (Supplementary Figures S4C–L). These results suggested that different isoforms may show distinct expression patterns. Further investigation on the function and mechanism of circ_0000160, as well as its relationship with SUCO, is needed in the future.
There were a few limitations in our study. First, the sample size was small. Circ_0000160 and the other two circRNAs circ_0001798 and circ_0001750, which did not show a relationship with ALNM, need further confirmation, ideally, with varied geographic and ethnic populations, with different stages, subtypes, and treatment regimens. Second, the present study mainly focused on clinically significant circRNAs in breast cancer. The function and mechanism of circRNAs in breast cancer need further investigation. Third, a single marker is not sufficiently accurate to predict ALNM. In future studies, the integration of multiple biomarkers to predict lymph node metastasis may supersede their individual predictive value.
In conclusion, we revealed the circRNA profile related to breast cancer ALNM using transcriptome sequencing. A decreased expression of circ_0000160 was found in breast cancer with axillary lymph node metastasis. Bioinformatics analysis showed that circ_0000160 may interact with numerous miRNAs and mRNAs and participate in many cancer-related pathways. The predicted mRNAs for circ_0000160 may be related to lymph node metastasis. This study provides a framework for understanding the mechanisms of breast cancer ALNM from the perspective of circRNAs. Circ_0000160 may have the potential to distinguish breast cancer with axillary lymph node metastasis from those without axillary lymph node metastasis.
Data Availability Statement
The datasets presented in this study can be found in online repositories. The names of the repository/repositories and accession number(s) can be found below: https://www.ncbi.nlm.nih.gov/geo/query/acc.cgi?acc=GSE173766.
Ethics Statement
The studies involving human participants were reviewed and approved by the Ethics Committee of the Qilu Hospital of Shandong University. The patients/participants provided their written informed consent to participate in this study.
Author Contributions
Y-WW and PS conceptualized the study. XC, YT, and LL helped with data collection. Y-WW and XC carried out data analysis and figure preparation. Y-WW and PS contributed to data interpretation and manuscript preparation. PS assisted with supervision. All authors contributed to the manuscript and approved the submitted version.
Funding
This work was supported by the National Natural Science Foundation of China (Nos. 81902698 and 81802406), the Shandong Provincial Natural Science Foundation (Nos. ZR2019BH061, ZR2019BH034, and ZR2020LZL009) and Special Funds for Scientific Research on Breast Diseases of Shandong Medical Association (No. YXH2021ZX058).
Conflict of Interest
The authors declare that the research was conducted in the absence of any commercial or financial relationships that could be construed as a potential conflict of interest.
Publisher’s Note
All claims expressed in this article are solely those of the authors and do not necessarily represent those of their affiliated organizations, or those of the publisher, the editors, and the reviewers. Any product that may be evaluated in this article, or claim that may be made by its manufacturer, is not guaranteed or endorsed by the publisher.
Acknowledgments
The authors thank Cloud-Seq Company (Shanghai, China) for the transcriptome sequencing service.
Supplementary Material
The Supplementary Material for this article can be found online at: https://www.frontiersin.org/articles/10.3389/fmolb.2021.690826/full#supplementary-material
Supplementary Figure S1 | Typical figures of hematoxylin and eosin (H&E)-stained sections of breast cancer with ALNM (axillary lymph node metastasis) and without ALNM. Two cases were used as examples, and their sections of primary breast cancer and the corresponding axillary lymph node were provided (A,B,E,F, primary breast invasive ductal carcinoma; C,D, without ALNM; G,H, with ALNM) (A,C,E,G, magnification × 40; B,D,F,H, magnification × 200).
Supplementary Figure S2 | Association between the circ_0001750 expression and clinicopathological parameters in the validation cohort containing 56 patients. Circ_0001750 displayed a higher expression in tissues with a higher grade (grade 3 vs. grade 2), negative PR status, or higher Ki-67 expression. The circ_0001750 expression showed no relationship with other parameters (ER, estrogen receptor; PR, progesterone receptor).
Supplementary Figure S3 | Circ_0001798 expression showed no relationship with clinicopathological parameters in the validation cohort containing 56 patients (ER, estrogen receptor; PR, progesterone receptor).
Supplementary Figure S4 | Isoform expression data of the SUCO gene in breast cancer based on the GEPIA2 database. (A) Isoform expression distribution of SUCO in breast cancer. (B–L) Comparison of the SUCO isoform expression in breast cancer and normal tissues (*p < 0.01).
References
Chang, J. M., Leung, J. W. T., Moy, L., Ha, S. M., and Moon, W. K. (2020). Axillary Nodal Evaluation in Breast Cancer: State of the Art. Radiology 295 (3), 500–515. doi:10.1148/radiol.2020192534
Chen, S., Fu, Z., Wen, S., Yang, X., Yu, C., Zhou, W., et al. (2021). Expression and Diagnostic Value of miR-497 and miR-1246 in Hepatocellular Carcinoma. Front. Genet. 12, 666306. doi:10.3389/fgene.2021.666306
Choi, H. Y., Park, M., Seo, M., Song, E., Shin, S. Y., and Sohn, Y.-M. (2017). Preoperative Axillary Lymph Node Evaluation in Breast Cancer. Ultrasound Q. 33 (1), 6–14. doi:10.1097/ruq.0000000000000277
Dudekula, D. B., Panda, A. C., Grammatikakis, I., De, S., Abdelmohsen, K., and Gorospe, M. (2016). CircInteractome: A Web Tool for Exploring Circular RNAs and Their Interacting Proteins and microRNAs. RNA Biol. 13 (1), 34–42. doi:10.1080/15476286.2015.1128065
Frey, L., Klümper, N., Schmidt, D., Kristiansen, G., Toma, M., Ritter, M., et al. (2021). CircEHD2, CircNETO2 and CircEGLN3 as Diagnostic and Prognostic Biomarkers for Patients with Renal Cell Carcinoma. Cancers 13 (9), 2177. doi:10.3390/cancers13092177
Ghosal, S., Das, S., Sen, R., Basak, P., and Chakrabarti, J. (2013). Circ2Traits: a Comprehensive Database for Circular RNA Potentially Associated with Disease and Traits. Front. Genet. 4, 283. doi:10.3389/fgene.2013.00283
Kim, M. Y. (2021). Breast Cancer Metastasis. Adv. Exp. Med. Biol. 1187, 183–204. doi:10.1007/978-981-32-9620-6_9
Kristensen, L. S., Hansen, T. B., Venø, M. T., and Kjems, J. (2018). Circular RNAs in Cancer: Opportunities and Challenges in the Field. Oncogene 37 (5), 555–565. doi:10.1038/onc.2017.361
Lee, J.-H., Jeong, H., Choi, J.-W., Oh, H. E., and Kim, Y.-S. (2018). Liquid Biopsy Prediction of Axillary Lymph Node Metastasis, Cancer Recurrence, and Patient Survival in Breast Cancer. Medicine (Baltimore) 97 (42), e12862. doi:10.1097/md.0000000000012862
Liao, Y., Wang, J., Jaehnig, E. J., Shi, Z., and Zhang, B. (2019). WebGestalt 2019: Gene Set Analysis Toolkit with Revamped UIs and APIs. Nucleic Acids Res. 47 (W1), W199–w205. doi:10.1093/nar/gkz401
Liu, M., Wang, Q., Shen, J., Yang, B. B., and Ding, X. (2019). Circbank: a Comprehensive Database for circRNA with Standard Nomenclature. RNA Biol. 16 (7), 899–905. doi:10.1080/15476286.2019.1600395
Rao, A. K. D. M., Arvinden, V. R., Ramasamy, D., Patel, K., Meenakumari, B., Ramanathan, P., et al. (2021). Identification of Novel Dysregulated Circular RNAs in Early-Stage Breast Cancer. J. Cel. Mol. Med. 25 (8), 3912–3921. doi:10.1111/jcmm.16324
Sawaki, M., Shien, T., and Iwata, H. (2019). TNM Classification of Malignant Tumors (Breast Cancer Study Group). Jpn. J. Clin. Oncol. 49 (3), 228–231. doi:10.1093/jjco/hyy182
Sha, Z., Sha, L., Li, W., Dou, W., Shen, Y., Wu, L., et al. (2015). Exome Sequencing Identifies SUCO Mutations in Mesial Temporal Lobe Epilepsy. Neurosci. Lett. 591, 149–154. doi:10.1016/j.neulet.2015.02.009
Shannon, P., Markiel, A., Ozier, O., Baliga, N. S., Wang, J. T., Ramage, D., et al. (2003). Cytoscape: a Software Environment for Integrated Models of Biomolecular Interaction Networks. Genome Res. 13 (11), 2498–2504. doi:10.1101/gr.1239303
Si, P., Zhang, P., Chen, T., Liu, G., Lu, H., Chen, H., et al. (2019). Positive Nonsentinel Lymph Nodes Are Associated with Poor Survival in Breast Cancer: Results from a Retrospective Study. Clin. Transl. Oncol. 21 (8), 1085–1092. doi:10.1007/s12094-018-02031-5
Sohaskey, M. L., Jiang, Y., Zhao, J. J., Mohr, A., Roemer, F., and Harland, R. M. (2010). Osteopotentia Regulates Osteoblast Maturation, Bone Formation, and Skeletal Integrity in Mice. J. Cel. Biol. 189 (3), 511–525. doi:10.1083/jcb.201003006
Tang, Z., Li, C., Kang, B., Gao, G., Li, C., and Zhang, Z. (2017). GEPIA: a Web Server for Cancer and normal Gene Expression Profiling and Interactive Analyses. Nucleic Acids Res. 45 (W1), W98–w102. doi:10.1093/nar/gkx247
Tokar, T., Pastrello, C., Rossos, A. E. M., Abovsky, M., Hauschild, A.-C., Tsay, M., et al. (2018). mirDIP 4.1-integrative Database of Human microRNA Target Predictions. Nucleic Acids Res. 46 (D1), D360–d370. doi:10.1093/nar/gkx1144
Verduci, L., Tarcitano, E., Strano, S., Yarden, Y., and Blandino, G. (2021). CircRNAs: Role in Human Diseases and Potential Use as Biomarkers. Cell Death Dis. 12 (5), 468. doi:10.1038/s41419-021-03743-3
Vo, J. N., Cieslik, M., Zhang, Y., Shukla, S., Xiao, L., Zhang, Y., et al. (2019). The Landscape of Circular RNA in Cancer. Cell 176 (4), 869–881. e813. doi:10.1016/j.cell.2018.12.021
Wang, L., Zhang, S., and Wang, X. (2020). The Metabolic Mechanisms of Breast Cancer Metastasis. Front. Oncol. 10, 602416. doi:10.3389/fonc.2020.602416
Wang, S., Zhang, K., Tan, S., Xin, J., Yuan, Q., Xu, H., et al. (2021a). Circular RNAs in Body Fluids as Cancer Biomarkers: the New Frontier of Liquid Biopsies. Mol. Cancer 20 (1), 13. doi:10.1186/s12943-020-01298-z
Wang, Y.-W., Xu, Y., Wang, Y.-Y., Zhu, J., Gao, H.-D., Ma, R., et al. (2021b). Elevated circRNAs Circ_0000745, Circ_0001531 and Circ_0001640 in Human Whole Blood: Potential Novel Diagnostic Biomarkers for Breast Cancer. Exp. Mol. Pathol. 121, 104661. doi:10.1016/j.yexmp.2021.104661
Wei, G., Zhu, J., Hu, H.-B., and Liu, J.-Q. (2021). Circular RNAs: Promising Biomarkers for Cancer Diagnosis and Prognosis. Gene 771, 145365. doi:10.1016/j.gene.2020.145365
Keywords: breast cancer, circRNA, lymph node metastasis, circ_0000160, transcriptome sequencing
Citation: Wang Y-, Chen X, Tian Y, Liu L and Su P (2022) Decreased Expression of circ_0000160 in Breast Cancer With Axillary Lymph Node Metastasis. Front. Mol. Biosci. 8:690826. doi: 10.3389/fmolb.2021.690826
Received: 04 April 2021; Accepted: 30 December 2021;
Published: 08 February 2022.
Edited by:
William C. Cho, QEH, ChinaReviewed by:
Didik Setyo Heriyanto, Gadjah Mada University, IndonesiaSandra Romero-CÃrdoba, Universidad Nacional Autónoma de México, Mexico
Kafil Akhtar, Aligarh Muslim University, India
Copyright © 2022 Wang, Chen, Tian, Liu and Su. This is an open-access article distributed under the terms of the Creative Commons Attribution License (CC BY). The use, distribution or reproduction in other forums is permitted, provided the original author(s) and the copyright owner(s) are credited and that the original publication in this journal is cited, in accordance with accepted academic practice. No use, distribution or reproduction is permitted which does not comply with these terms.
*Correspondence: Peng Su, c3VwZW5nX3NkcWxAMTYzLmNvbQ==
†These authors have contributed equally to this work and share first authorship