- Department of Gynecology and Obstetrics, Beijing Friendship Hospital, Capital Medical University, Beijing, China
Objective: Immunity plays a vital role in the human papilloma virus (HPV) persistent infection, and closely associates with occurrence and development of cervical squamous cell carcinoma (CSCC). Herein, we performed an integrated bioinformatics analysis to establish an immune-gene signature and immune-associated nomogram for predicting prognosis of CSCC patients.
Methods: The list of immunity-associated genes was retrieved from ImmPort database. The gene and clinical information of CSCC patients were obtained from The Cancer Genome Atlas (TCGA) website. The immune gene signature for predicting overall survival (OS) of CSCC patients was constructed using the univariate Cox-regression analysis, random survival forests, and multivariate Cox-regression analysis. This signature was externally validated in GSE44001 cohort from Gene Expression Omnibus (GEO). Then, based on the established signature and the TCGA cohort with the corresponding clinical information, a nomogram was constructed and evaluated via Cox regression analysis, concordance index (C-index), receiver operating characteristic (ROC) curves, calibration plots and decision curve analyses (DCAs).
Results: A 5-immune-gene prognostic signature for CSCC was established. Low expression of ICOS, ISG20 and high expression of ANGPTL4, SBDS, LTBR were risk factors for CSCC prognosis indicating poor OS. Based on this signature, the OS was significantly worse in high-risk group than in low-risk group (p-value < 0.001), the area under curves (AUCs) for 1-, 3-, 5-years OS were, respectively, 0.784, 0.727, and 0.715. A nomogram incorporating the risk score of signature and the clinical stage was constructed. The C-index of this nomogram was 0.76. AUC values were 0.811, 0.717, and 0.712 for 1-, 3-, 5-years OS. The nomogram showed good calibration and gained more net benefits than the 5-immune-gene signature and the clinical stage.
Conclusion: The 5-immune-gene signature may serve as a novel, independent predictor for prognosis in patients with CSCC. The nomogram incorporating the signature risk score and clinical stage improved the predictive performance than the signature and clinical stage alone for predicting 1-year OS.
Introduction
Cervical squamous cell carcinoma (CSCC) is the major pathological type in cervical cancer (CC), accounting for approximately 75–80% of all CC (Intaraphet et al., 2013). In developing countries without a comprehensive CC screening and anti-human papilloma virus (HPV) vaccination program, the incidence and mortality of CC remains high, and the age of CC onset tends to be younger (Olson et al., 2016). Prognostic evaluation is essential for selecting patients for adjuvant therapy, setting goals for patient treatment and counselling patients (Grobmyer and Brennan, 2003). The tumor-node-metastasis (TNM) system is the most frequently utilized prognostic indicator in clinical practice. Nevertheless, the clinical TNM staging system narrowly focuses on tumor cells without considering other factors such as host immunity and inflammation responses; even patients with the same stage disease might face distinctly different outcomes (Hu et al., 2018).
It is well recognized that high-risk HPV is a key cause of CC (Evander et al., 1995; Walboomers et al., 1999). While around 90% of women contract HPV infection during their lifetimes (Rodríguez et al., 2008), only a small percentage of these infections will progress to premalignant lesion/cancer (Kulasingam et al., 2002; Dalstein et al., 2003). Recent studies pointed out that HPV infection alone is insufficient for CC; factors such as abnormal host genes (e.g., immune genes polymorphisms) and dysfunctional host immunity may also play synergistic roles in persistent HPV infection and the development of CC (Grulich et al., 2007; Wang et al., 2009; De Freitas et al., 2014; Zhang et al., 2014; Song et al., 2015). The immunity-related mechanisms behind CC remains to be further explored.
A number of immunity-related prognostic markers for CC have been identified, including interferon gamma (IFNG) (Ivansson et al., 2010), cytotoxic T-lymphocyte associated protein 4 (CTLA4) (Rahimifar et al., 2010), transporter 1, ATP binding cassette subfamily B member (TAP1), and transporter 2, ATP binding cassette subfamily B member (TAP2) (Gostout et al., 2003). However, all of these are single gene markers that can be less prognostic and less stable than multi-gene signatures (Grzadkowski et al., 2018). Immune-related prognostic gene signatures based on breast cancer, lung cancer, hepatocellular carcinoma have been reported (Budhu et al., 2006; Roepman et al., 2009; Bedognetti et al., 2015). Immune-related prognostic nomogram incorporating immune gene signature and the other clinical information were also shown good performance in colorectal cancer (Song et al., 2020) and lower-grade glioma (Zhang et al., 2020). For the CC, Liu and colleagues (Liu et al., 2020) established a two-immune-gene signature (CD6 and CD1C) for predicting prognosis. This signature showed good performance in TCGA cohort, even though lacking the external validation. Another study also developed a 29-immune-related gene-pairs signature for predicting the prognosis of CC (Nie et al., 2020). Nevertheless, the nomogram incorporating immune gene signature expression and other potential clinical information for evaluating the prognosis of CSCC remains to be investigated.
Given the available evidence, we proposed two hypotheses to be tested. The first hypothesis was that immune gene signature may also show prognostic significance for CSCC patients; the second was that the combination of signature and clinical information could further improve the prediction performance.
Methods
Data Downloads
The Gene symbol IDs of all immune-related genes were retrieved from Immunology Database and Analysis Portal (ImmPort, https://www.immport.org/home). The gene expression profiles and corresponding clinical information for CSCC patients (n = 254) were obtained from The Cancer Genome Atlas (TCGA, https://tcga-data.nci.nih.gov/tcga/) and further served as training dataset. In addition, the GSE44001 (n = 300) was retrieved from NCBI Gene Expression Omnibus (GEO, http://www.ncbi.nlm.nih.gov/geo/) and served as external validation dataset. Data were downloaded on 14 December, 2020.
Data Preprocessing
Data From TCGA
The RNA-sequencing data were preprocessed following these steps:
1) Samples with overall survival (OS) of less than 30 days or with no clinical information were excluded.
2) Genes with expression levels less than one were excluded.
3) The expression profiles of immune genes were included for analysis.
Data From GEO
The GSE44001 data were preprocessed following these steps:
1) Probe sets were mapped to the human gene SYMBOL by the R-package ‘GEOquery’ (version 2.58). Probes without gene symbols or matching two or more gene symbols were excluded. When two or more probes matched one gene symbol, the probe with the highest value of |log fold change| was selected.
2) Genes with expression levels less than one were excluded.
3) The expression profiles of immune genes were included for analysis.
To make the expression data from two databases comparable, the “scale” function in R was used for centring and standardising.
Construction and Evaluation of the Immune Gene Signature
After excluding 27 cases of CSCC patients with overall survival time less than 30 days, a total of 227 CSCC patients from TCGA were included in the construction of signature. Firstly, univariate Cox-regression analysis was conducted to identify survival-associated immune genes via R-package “survival” (version 3.2-11). Secondly, to guarantee sufficient statistical power for multivariate stepwise cox-regression, the R-package “random-ForestSRC” (version 2.9.3) (Ishwaran et al., 2008) was utilized to make a feature selection from a large number of survival-related immune genes. The random forest resembled the gating hierarchy of flow cytometry (Popescu et al., 2019). It is an ensemble classifier with a decision tree as base classifier (Cheng et al., 2018), has been well established in bioinformatics (Gosink et al., 2017). We applied the random Survival Forest (RSF) method to rank the gene importance. The genes with relative importance over 0.4 were selected in multivariate stepwise Cox-regression analysis. Based on the coefficients calculated by multivariate Cox-regression analysis, an immune-gene signature for predicting the prognosis of CSCC patients was established. Based on the expression of signature, the risk score was calculated for each case from TCGA cohort, and risk-score distribution diagrams, Kaplan–Meier (KM) survival curves, and receiver-operating characteristic (ROC) curves at the 1-, 3-, 5-years time points were conducted to evaluate the performance of the signature. We also performed KM survival curves of every single gene from the signature to explore their prognostic values. In addition, to verify the stability, this signature was further validated in the GSE44001 cohort and disease-free survival (DFS) dataset of CSCC via risk-score distribution diagrams, KM survival curves, and ROC curves.
Construction and Evaluation of Nomogram
To better predict the prognosis of CSCC patients, a nomogram incorporating the signature risk score and other clinical characteristics was developed based on TCGA CSCC cohort. After excluding cases without detailed clinical information, a total of 220 CSCC patients were included in analysis. To be specific, variables including age, smoking status, pathological grades, clinical stages, and the signature risk score were included in the univariate Cox-regression analysis. Then, statistically significant variables were included in multivariate Cox-regression analysis, to screen independent prognostic variables for the OS of CSCC patients. The significant factors were screened based on the p-value < 0.05. To assess the performance (discrimination ability and calibration) of the established nomogram, concordance index (C-index) and calibration plots were utilized (Hanley and McNeil, 1982; Coutant et al., 2009; Wolbers et al., 2009). The C-index is a widely applicable measure of predictive discrimination (Harrell et al., 1996). The calibration curves were employed to compare the probability between nomogram-predicted survival with the observed survival. Moreover, the 1-, 3-, 5-years ROC curves and the 1-, 3-, 5-years decision curve analysis (DCA) (Vickers and Elkin, 2006) curves were used to compare the prediction performance of the established nomogram, immune-gene signature, and the clinical stage. DCA is a method for evaluating the clinical usefulness through quantifying net benefits at a range of threshold probabilities. Both the calibration and discrimination were evaluated through bootstrap analysis with 1,000 resamples.
Gene Ontology and KEGG Pathway Enrichment
To explore the biological function of the immune-gene signature, GO and KEGG enrichment analysis were carried out via R-package “Clusterprofiler” (version 3.10.1) (Yu et al., 2012a), with the immune genes from the signature as gene-list and with the whole transcriptome as background. The pathway or function with adjusted p-value < 0.05 was selected as significantly enriched GO category/KEGG pathway.
Gene Set Enrichment Analysis
To better understand the biological features of the immune-gene signature and every prognostic gene in this signature. Gene set enrichment analysis (GSEA) (Subramanian et al., 2005) was applied to screen significant signalling pathways based on the CSCC cohort from TCGA, with the whole transcriptome as background and with the signal-to-noise ratio as ranking criterion. Based on the median signature score or the median gene expression level (Song et al., 2019), patients were divided into two groups (i.e., high/low-risk groups classified by the established signature and high/low-expression group classified by the prognostic gene). GSEA was performed via GSEA 4.1.0 software (http://software.broadinstitute.org/gsea/). The significant pathways were screened based on false discovery rate (FDR) < 0.05.
Immunotherapy Sensitivity Analysis
To investigate the immunotherapy sensitivity of high/low-risk groups classified by the established immune-gene signature, a published dataset containing 47 melanoma patients treated with cytotoxic T lymphocyte-associated molecule-4 (CTLA-4) and programmed cell death receptor-1 (PD-1) inhibitors (Roh et al., 2017) was used as the reference dataset as previously described (Lu et al., 2019). Then, the unsupervised Subclass Mapping (Submap) algorithm (Hoshida et al., 2007) in GenePattern (https://www.genepattern.org/) was adopted to compare the sensitivity to immune-checkpoint inhibitor therapy between high/low-risk groups classified by the signature. The Submap algorithm provided the p-value, demonstrating the likelihood of molecular similarity among different subclasses (Reich et al., 2006).
Lymphocytes Infiltration Analysis
To estimate the relative abundance of 22 types of immune cells in CSCC samples, Cell type Identification By Estimating Relative Subsets Of known RNA Transcripts (CIBERSORT, https://cibersortx.stanford.edu/) was used for lymphocytes infiltration analysis. This is a method for exploring cell composition from complex tissues based on their gene expression profiles (Newman et al., 2015). The 22 immune cells include different subtypes of T cells, B cells, natural killer cells (NKs), plasma, dendritic, and mast cells, macrophages, neutrophils, and eosinophils.
Prognostic Analysis in Gynecological Cancers
To further explore the relationship of every single gene from the immune-gene signature with the prognosis of gynecological cancers (including breast invasive carcinoma [BRCA], cervical squamous cell carcinoma and endocervical adenocarcinoma [CESC], ovarian serous cystadenocarcinoma [OV], uterine corpus endometrial carcinoma [UCEC], and uterine carcinosarcoma [UCS]), univariate survival analysis was used to analyze the correlation of patient overall survival with gene expression. Univariate Cox analysis was employed via R package “survival” (version 3.2-11).
Statistical Analysis
R 3.4.0 software and related statistical packages were utilized for statistical analysis. Chi-square test, or Fisher-exact test (if any of the variables with value less than 5), was performed for categorical variables. The p-value less than 0.05 was regarded as statistically significant.
Results
A 5-Immune-gene Prognostic Signature was Established for CSCC Patients
An overview of the analysis procedure of the current study was shown in Supplementary Figure S1. The random forest analysis was performed and identified eight survival-related immune genes with out-of-bag importance over 0.4 (Figure 1). After multivariable stepwise regression analysis based on the eight survival-related immune genes, a 5-immune-gene prognostic signature was finally constructed. These genes included Inducible T Cell Costimulator (ICOS, average TPM: 3.711), Interferon Stimulated Exonuclease Gene 20 (ISG20, average TPM: 19.819), Angiopoietin Like 4 (ANGPTL4, average TPM: 72.436), SBDS Ribosome Maturation Factor (SBDS, average TPM: 150.027), Lymphotoxin Beta Receptor (LTBR, average TPM: 79.407). For each individual CSCC patient, a signature risk score was calculated via the following formula: Score = ICOS × (−0.34244) + ISG20 × (−0.24956) + ANGPTL4 × 0.270329 + SBDS × 0.423242 + LTBR × 0.401314. Patients were divided into high/low-risk groups via the median risk score (0.971). The clinical characteristics of the high/low-risk groups in TCGA dataset were shown in Table 1. Based on the 5-immune-gene signature, the area under curves (AUCs) for 1-, 3-, and 5-years OS were respectively 0.784, 0.727, and 0.715 (Figure 2A). Compared with the low-risk group, the high-risk group showed significantly worse OS (p-value < 0.001; Figures 2C,E). This 5-immune-gene signature was externally validated in the GEO cohort with DFS information. The clinical information of the GEO cohort was shown in Table 1. The AUCs were 0.624 at 1 year, 0.648 at 3 years, and 0.627 at 5 years. (Figure 2B). Compared with low-risk group, the high-risk group of the GEO cohort had significantly worse probability of DFS (p-value = 0.001; Figures 2D,F). The 5-immune-gene signature was also evaluated in the TCGA cohort with DFS information. The AUC of DFS was 0.695 (Supplementary Figure S2A), the high-risk group had significantly worse probability of DFS than low-risk group (p-value = 0.043; Supplementary Figures S2B,C). Taken together, the 5-immune-gene signature exhibited good performance of prognostic prediction. In this signature, low expression levels of ICOS (HR = 0.48, 95% CI = 0.29–0.80, p-value = 0.007), ISG20 (HR = 0.44, 95% CI = 0.26–0.74, p-value = 0.010) and high expression levels of ANGPTL4 (HR = 2.08, 95% CI = 1.20–3.59, p-value = 0.004), SBDS (HR = 1.96, 95% CI = 1.18–3.26, p-value = 0.015), and LTBR (HR = 1.73, 95% CI = 1.04–2.88, p-value = 0.045) were risk factors for CSCC prognosis, suggesting poor OS (Figures 3A–E).
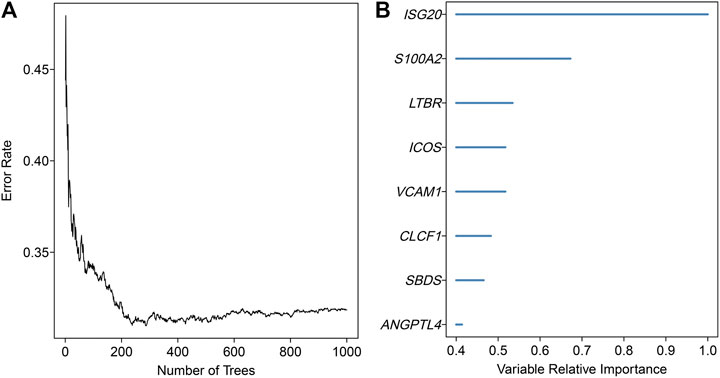
FIGURE 1. Random survival forests variable hunting analysis. (A) The relationship between the number of classification trees and the error rate. This random forest consisted of 1,000 decision trees and analysed all the significant survival-related genes in univariate Cox-regression analysis. (B) Out-of-bag importance of the survival-related genes with values over 0.4. The x-axis represented the relative importance values of variables estimated by random forest.
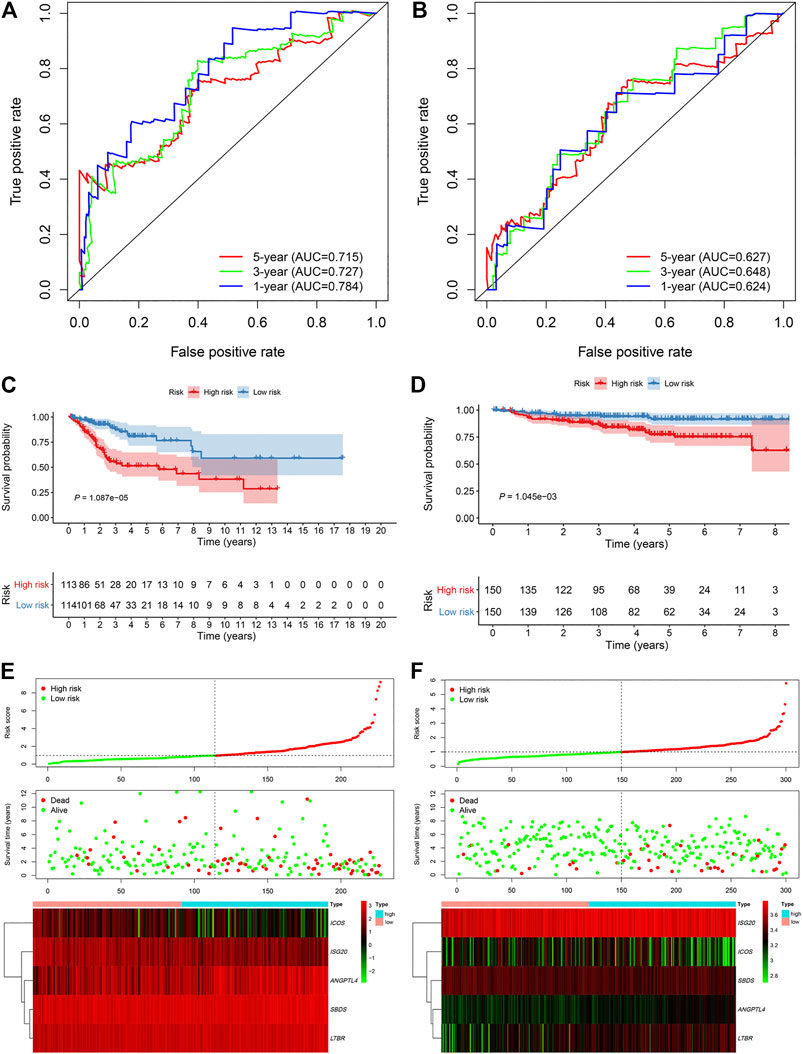
FIGURE 2. The 5-immune-gene signature showed good performance in TCGA and GEO cohort. Time-dependent ROC analysis in (A) TCGA cohort and (B) GEO cohort. Kaplan–Meier survival analysis in (C) TCGA and (D) GEO cohort. Signature risk score analysis in (E) TCGA and (F) GEO cohort. TCGA, The Cancer Genome Atlas; GEO, Gene Expression Omnibus; ROC, receiver-operating characteristic.
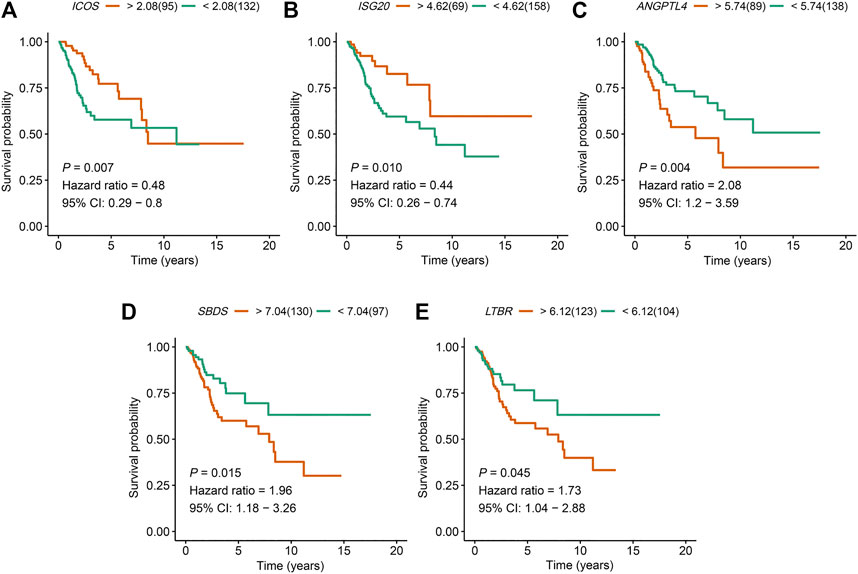
FIGURE 3. The expression of the five immune genes ICOS, ISG20, ANGPTL4, SBDS, and LTBR were significantly associated with the prognosis of CSCC patients. Kaplan–Meier OS curves for high and low expression of (A)ICOS, (B)ISG20, (C)ANGPTL4, (D)SBDS, and (E)LTBR in CSCC patients. ICOS, Inducible T Cell Costimulator; ISG20, Interferon Stimulated Exonuclease Gene 20; ANGPTL4, Angiopoietin Like 4; SBDS, SBDS Ribosome Maturation Factor; LTBR, Lymphotoxin Beta Receptor; CSCC, cervical squamous cell carcinoma; OS, overall survival.
Prognostic Nomogram Incorporating Signature Risk Score and Clinical Stage was Constructed for CSCC Patients
In univariate Cox regression analysis (Figure 4A), the 5-immune-gene signature risk scores (HR = 1.56, 95% CI = 1.37–1.79, p-value < 0.001) and the clinical stages (HR = 1.51, 95% CI = 1.18–1.92, p-value < 0.001) were significantly correlated with the OS in CSCC patients. In multivariate Cox regression analysis (Figure 4B), the signature risk scores (HR = 1.63, 95% CI = 1.41–1.87, p-value < 0.001) and the clinical stages (HR = 1.57, 95% CI = 1.23–2.00, p-value < 0.001) turned out to be the independent predictors for the OS of CSCC patients. We further constructed and evaluated a nomogram (Figure 5) incorporating signature risk score and clinical stage. The nomogram had favorable discrimination (C-index = 0.76; 95% CI = 0.70–0.82). The calibration curves of the nomogram showed favorable agreement between actual OS probability and nomogram-predicted OS probability (Figures 6A–C). When compared with 5-immune-gene signature and clinical stage, the nomogram (AUC = 0.811) showed better predictive power than signature risk score (AUC = 0.782) and clinical stage for 1-year OS (AUC = 0.660; Figure 7A). For the OS at 3- and 5-years, the predictive power of the prognostic nomogram was similar to that of the signature risk score and better than that of clinical stage (AUC at 3 years = 0.566, AUC at 5 years = 0.576, Figures 7B,C). The DCA curves at 1-, 3-, and 5- years revealed that the nomogram had more net benefits than the signature risk score and the clinical stage (range: 0–0.5; Figures 7D–F).
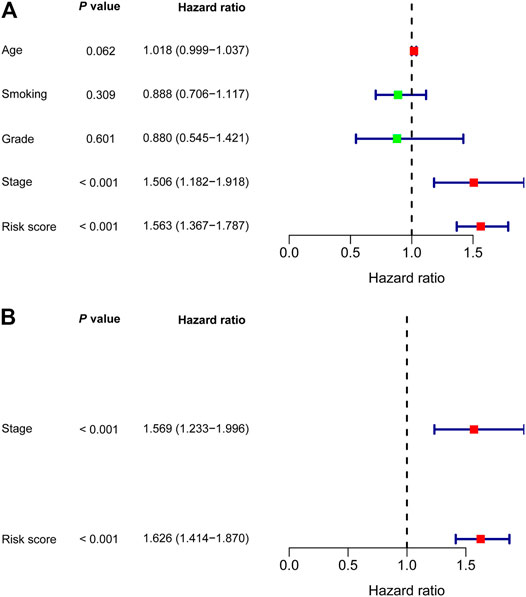
FIGURE 4. Nomogram was developed based on (A) Univariate and (B) multivariate Cox regression analysis.
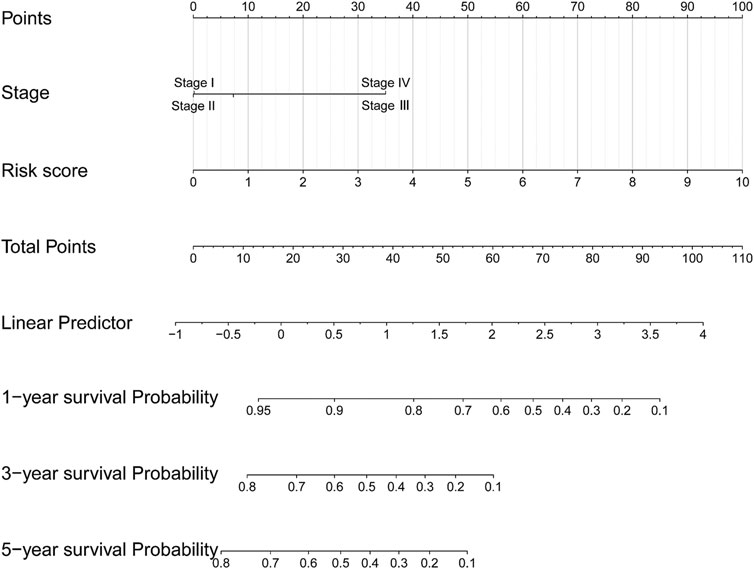
FIGURE 5. A nomogram incorporating 5-immune-gene signature and clinical stage was constructed for predicting OS of CSCC patients.
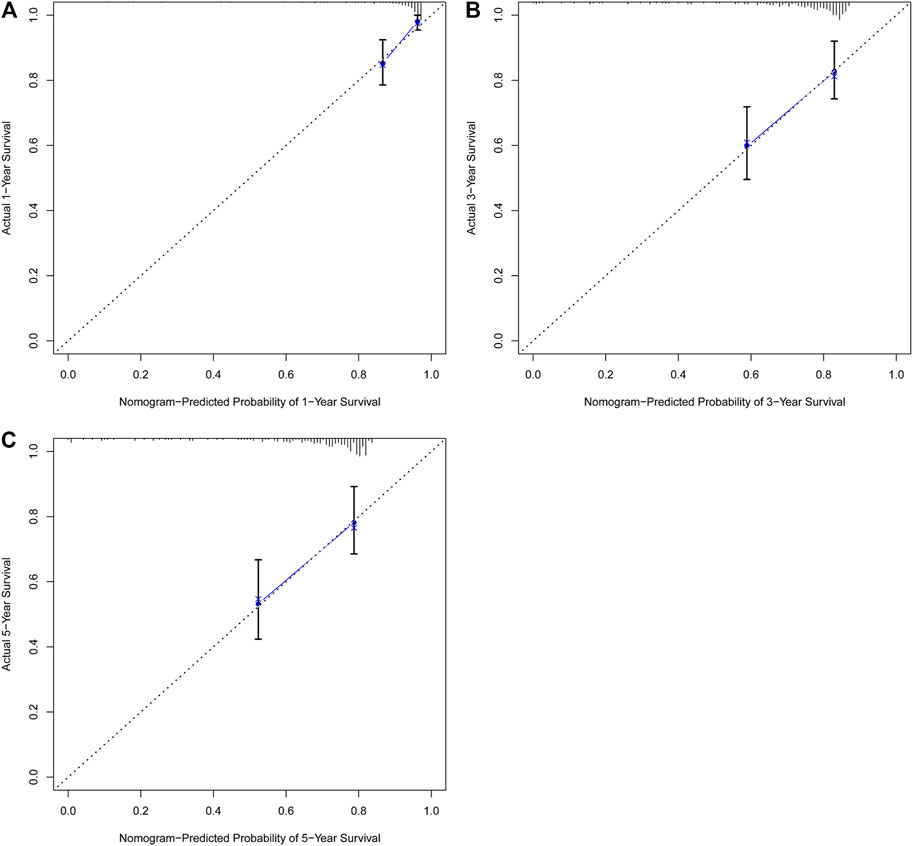
FIGURE 6. The nomogram showed favorable agreement between nomogram-predicted OS probability and actual OS probability. Calibration plots for predicting (A) 1-year, (B) 3-years, and (C) 5-years OS for CSCC patients based on the nomogram. The 45-degree dotted line represents the “ideal” prediction. The blue line represents the performance of the nomogram. The blue line closer to the ideal prediction has a higher predictive accuracy of the nomogram.
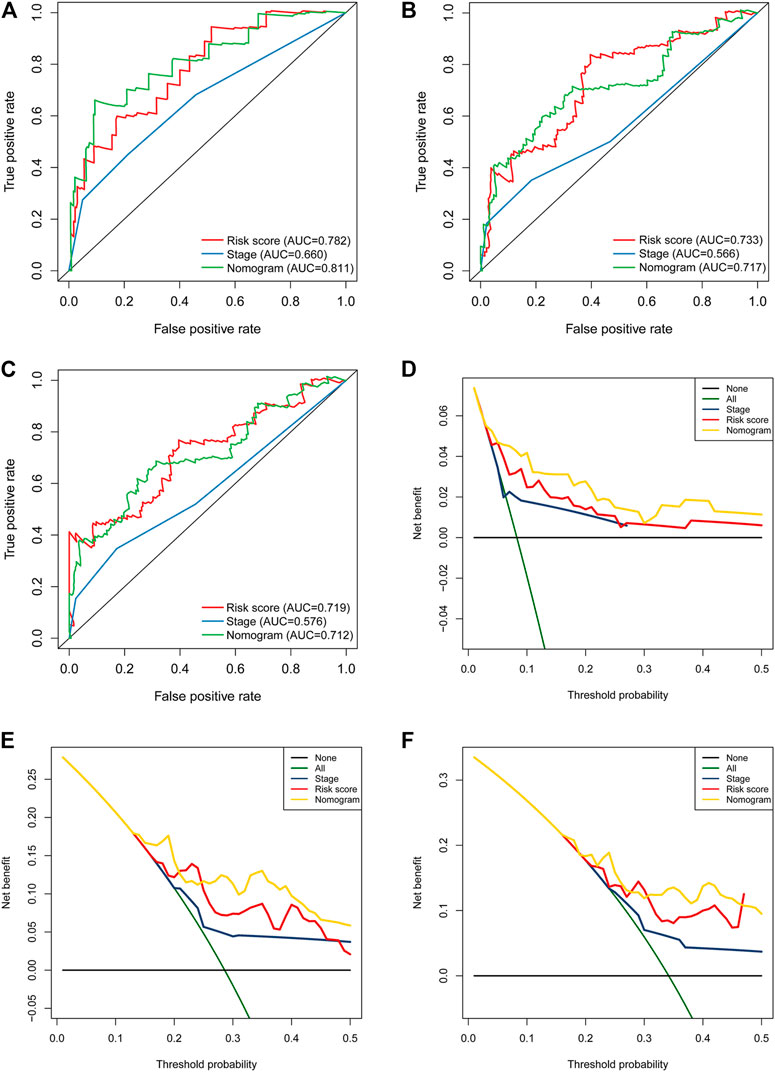
FIGURE 7. The nomogram showed better predictive power than the 5-immune-gene signature and clinical stage for 1-year OS. Time-dependent ROC curves of the nomograms, 5-immune-gene signature, and clinical stage in prediction of (A) 1-year, (B) 3-years, and (C) 5-years OS for CSCC patients. DCA curves for the nomogram, 5-immune-gene signature, and clinical stage in prediction of (D) 1-year, (E) 3-years, and (F) 5-years OS for CSCC patients. DCA, decision curve analysis.
Enrichment Analysis Based on the 5-Immune-gene Signature
The GSEA analysis result based on the signature revealed that “adherens junction,” “basal cell carcinoma,” “pentose phosphate pathway,” and “renal cell carcinoma” signalling pathways were significantly enriched (p-value < 0.05) in the high-risk group of CSCC patients (Figure 8A). The “B cell receptor signalling pathway,” “primary immunodeficiency,” and “T cell receptor signalling pathway” were significantly enriched (p-value < 0.05) in the low-risk group (Figure 8B).
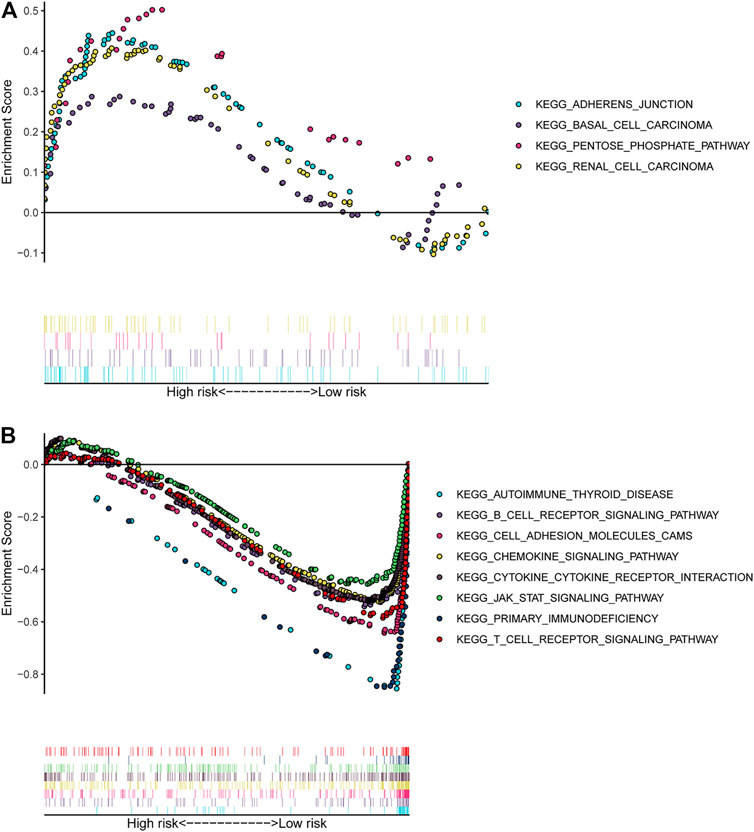
FIGURE 8. GSEA analysis based on the 5-immune-gene signature. (A) Significantly enriched pathways in the high-risk group of CSCC; (B) significantly enriched pathways in the low-risk group of CSCC. The y-axis represented the enrichment score calculated by GSEA. Vertical bars under the x-axis represented the positions of genes in the given set. Positive enrichment scores denoted the pathways were significantly enriched in high-risk group, while negative enrichment scores denoted the pathways were significantly enriched in low-risk group. GSEA, gene set enrichment analysis.
GO analysis revealed that the signature was mainly enriched in “T cell activation” for biological process (BP) (Supplementary Figure S3). The KEGG pathway analysis suggested that the signature was predominantly enriched in “T cell receptor signalling pathway,” “Th1, Th2, and Th17 cell differentiation,” and “PD-L1 expression and PD-1 checkpoint pathway in cancer” (Supplementary Figure S4).
Immunotherapy Sensitivity Analysis Based on the 5-Immune-gene Signature
To further investigate the clinical implication of 5-immune-gene signature, we used SubMap algorithm to compare the immunotherapy sensitivity between high/low-risk groups classified by this signature. As shown in Figure 9, the low-risk group was more likely to respond to PD-1 checkpoint immunotherapies (Bonferroni-corrected p-value = 0.006).
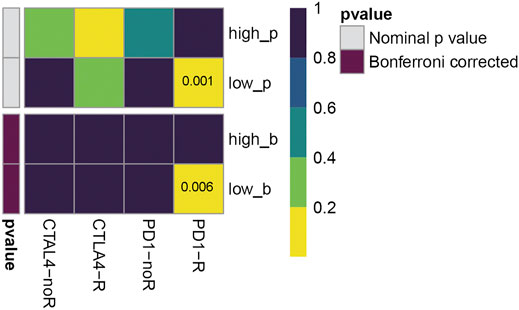
FIGURE 9. Effect prediction of immunotherapy response in TCGA cohort. The horizontal axis shows the groups placed on CTLA-4 and PD-1 immunotherapies. PD1-R, patients respond to PD-1 therapy, PD1-noR, patients do not respond to PD-1 therapy. The vertical axis shows the high/low-risk groups classified by five-gene prognostic signature, p refers to nominal p-value, b refers to Bonferroni-corrected p-value. The color represents the significance levels (p-values).
Lymphocytes Infiltration and GSEA and Gynecological-Cancer Prognostic Analysis Based on the Five Immune Genes
The correlation between the five immune genes and the infiltration of 22 lymphocytes was shown in Supplementary Figure S5. This analysis demonstrated that these five genes were all significantly associated with the infiltration of one or more lymphocytes of 22 lymphocytes. It should be noted that both of the ISG20 and ICOS showed negative correlations with “naïve B cells,” “Eosinophils,” “M0 Macrophages,” and “CD4+ resting memory T cells,” and showed positive correlations with “activated memory CD4+ T cells,” and “CD8+ T cells” (p-value < 0.05). The SBDS and ANGPTL4 were positively correlated with “activated mast cells”, and ICOS was negatively correlated with “activated mast cells” (p-values < 0.05).
Supplementary Figures S6–8 showed the GSEA results for each prognostic gene with statistical significance. GSEA of ICOS (Supplementary Figure S6) revealed that “T cell receptor signalling pathway” was significantly enriched in ICOS-high-expressing group (p-value < 0.05). GSEA of LTBR (Supplementary Figure S8) found that “galactose metabolism” pathway was significantly enriched in LTBR-high-expressing group (p-value < 0.05).
As shown in Supplementary Figure S9, in terms of overall survival, not only CESC patients, but also OV patients, were significantly associated with ICOS, ISG20, ANGPTL4, and SBDS (all p-values < 0.05).
Discussion
In this study, to predict prognosis of CSCC patients, we established a 5-immune-gene signature (including ICOS, ISG20, ANGPTL4, SBDS, and LTBR) in TCGA cohort and externally validated it in GEO cohorts. Based on the 5-immune-gene signature, we constructed a nomogram incorporating signature risk score and clinical stage. This nomogram showed good accuracy for predicting overall survival of CSCC patients (1-, 3-, 5-years AUC were 0.811, 0.717, and 0.712, respectively).
Among the five immune genes from the established signature, several genes have been explored in previous studies. ISG20 is an RNA exonuclease that exhibits inhibitory activity against various viruses (e.g., human immunodeficiency virus (Espert et al., 2005), influenza virus, vesicular stomatitis virus, and encephalomyocarditis virus (Zhou et al., 2011)). In our analysis, downregulation of ISG20 was associated with poor outcome of CSCC. This result appears to be in discordance with that of Rajkumar et al. (2011), who found that ISG20 was up-regulated in CC and may get involved in the progression of cervical disease (Rajkumar et al., 2011). But to be noted, Rajkumar et al. (2011) included all pathological types of CC, the sample size was limited (n = 28), follow-up information of CC patients was lacking, and up-regulation of ISG20 was regarded as being involved in cervical tumorigenesis, not for predicting outcome.
We also found that ANGPTL4 (angiopoietin-like protein 4) was up-regulated in CSCC tissues with poor prognosis. This finding is in accordance with that of Nie et al., who reported that ANGPTL4 was up-regulated in CC samples, and this up-regulation was associated with lymph node metastasis, deep stromal invasion, lympho-vascular space invasion, and advanced tumor stage, as well as poor OS and DFS (Nie et al., 2019). ANGPTL4 is a member of the angiopoietin-related family, and involves the regulation of glucolipid and angiogenesis metabolism (Hato et al., 2008; Li and Teng, 2014). The up-regulated ANGPTL4 contributed to tumor angiogenesis, growth, metastasis, invasion, and reduced OS of patients (Shibata et al., 2010; Li et al., 2011; Tanaka et al., 2015; Huang et al., 2016; Zhu et al., 2016). Meanwhile, ANGPTL4 polymorphism was found to be associated with cervical neoplasia development (Rahmani et al., 2020). For patients with CC, ANGPTL4 might serve as a marker for poor survival (Nie et al., 2019). Nevertheless, there remains a need to elucidate the exact tumorigenesis mechanism of ANGPTL4 in CC.
SBDS encodes a highly conserved protein in archaebacteria and eukaryotes (Boocock et al., 2003). It is multifunctional, involved in ribosome synthesis, chemotaxis, telomerase recruitment, assembly of mitotic spindle, and regulation of reactive oxygen species generation (Wessels et al., 2006; Austin et al., 2008; Ambekar et al., 2010; Spalinger et al., 2018). The role of SBDS in cancer is not well clarified. In the current analysis, the SBDS was highly expressed in CSCC tissues and predicted worse OS. This finding accords with those of Hao et al. (2020). But, to be noted, Hao et al. (2020) also found that SBDS played dual roles in cancer development (i.e., both tumor-promoting and tumor-suppressive roles). SBDS is essential for ribosome assembly in cytoplasm and rRNA synthesis in nucleolus, which may confer its oncogenic action in cancer. By contrast, ectopic SBDS in the nucleoplasm may exert a tumor-suppressive effect by activating p53 (Ganapathi et al., 2007; Finch et al., 2011; Hao et al., 2020).
The associations between ICOS, LTBR with CSCC prognosis have not been completely clarified; however, one study reported that ICOS gene polymorphisms showed no association with susceptibility to CSCC (Pawlak et al., 2010). Our study revealed that low expression of ICOS was risk factor for CSCC prognosis. Moreover, we found the ICOS expression was associated with the prognosis of CESC, OV, and UCEC. Based on the GSEA of ICOS and the enrichment analysis of the 5-immune-gene signature (i.e., GO, KEGG, and GSEA), it seems that ICSO combining with other four immune genes may enrich in the pathway of T cell receptor and play a role in regulating the activation of T cell. In the cancer immunotherapy, T cell activation is critical for therapeutic efficacy (Nam et al., 2018). The well-known PD-1 inhibitor is designed to interrupt the interactions of PD-1 receptor with ligand and thus restore the anti-tumor responses from T cell (Agulló-Ortuño et al., 2020).
In addition, through the submap algorithm, we were delighted to see the low-risk group classified by the 5-immune-gene signature was more likely to benefit from PD-1 checkpoint immunotherapies. Over the past decades, immunotherapies have dramatically changed the treatment algorithm for melanoma. Immune-checkpoint inhibitors (Schumacher and Schreiber, 2015), particularly PD-1/programmed cell death ligand-1(PD-L1) inhibitors (Constantinidou et al., 2019), have exhibited favorable efficacy against various solid tumors (Gettinger et al., 2018). It has also been revealed that the inhibitors of PD-1/PD-L1 might be a promising method for treatment for CC (Liu et al., 2019), while the reported response rates of PD-1/PD-L1 inhibitors against recurrent or metastatic CC were under 30% (Frenel et al., 2017; Hollebecque et al., 2017; Schellens et al., 2017). Cancer patients’ response to immunotherapies remains a challenging field of research. Our study found that the 5-immune-gene signature was correlated with response to PD-1 inhibitors, indicating this 5-immune-gene signature may help doctors to screen for patients who have more potential to respond to anti-PD-1 immunotherapy, achieving more accurate and personalised treatment plans.
Based on the CIBERSORT algorithm, the current analysis found that expression of all five genes was significantly correlated with the lymphocyte infiltration. These results reveal that the five immune genes play significant roles in the immune microenvironment, and the dysregulated immune systems may be involved in the progression of CSCC. “Activated mast cells” was positively associated with SBDS and ANGPTL4 but negatively associated with ICOS, suggesting that the infiltration levels of activated mast cells (MCs) were increased in the high-risk group of CSCC patients. This is in accordance with the study of Wang et al., who found that the higher fraction of activated MCs were independent risk factors for CC, indicating worse OS. MCs have been found to be involved in inflammatory, immune reactions, angiogenesis, tissue remodelling, immune-modulation, and carcinogenesis of various cancers, including CC (Maltby et al., 2009; Utrera-Barillas et al., 2010). In cervical tissues with intra-epithelial lesions or invasive lesions, microvessel density significantly correlated with the density of MCs (Wilk et al., 2010). MCs play several roles in both physiological and pathological processes. Normally, MCs are located at the boundary of the external environment, where they serve as sentinels for pathogen invasion and tissue damage (Galli and Tsai, 2012); however, when MCs become over-activated, such as during systemic or chronic infections, they often cause pathogenic sequelae (Choi et al., 2020). One study found both a pro-tumorigenic and anti-tumorigenic role of MCs (Varricchi et al., 2017a). Overall, the impacts of MCs are complicated and controversial, deserving further investigation (Theoharides and Conti, 2004; Varricchi et al., 2017b).
The potential study limitations: in this study, we adopted the random forest and COX regression analysis to select hub genes for the prognostic signature, and established a 5-immune-gene signature with good performance; however, due to the limited sample size of the study cohort and the complex nature of biology, we could not guarantee good stability of this signature in the real-world cohort. Despite the fact that an external GEO data-set of gene expression profiles of CSCC was obtained for validation, it remains essential that the findings of the current study should be validated in large-scale clinical cohort studies. For the future studies aiming at the establishment of prognostic signature, not only the mechanisms of the feature selection methods, but also the sample size and the data distribution should be considered (Yu et al., 2012b).
Conclusion
In summary, we established an immune gene-based signature for predicting prognosis of CSCC patients; besides, this signature could also help in predicting response to anti-PD-1 immunotherapy. Further, we developed a nomogram combining the signature and clinical stage, which showed better prediction performance than the individual signature and clinical stage. Our findings give the important indication that the immune gene-based classifier should prove valuable in prognosis prediction and clinical decision-making for CSCC patients.
Data Availability Statement
The datasets presented in this study can be found in online repositories. The names of the repository/repositories and accession number(s) can be found in the article/Supplementary Material.
Ethics Statement
Ethical review and approval was not required for the study on human participants in accordance with the local legislation and institutional requirements. Written informed consent for participation was not required for this study in accordance with the national legislation and the institutional requirements.
Author Contributions
All authors listed have made a substantial, direct, and intellectual contribution to the work and approved it for publication.
Conflict of Interest
The authors declare that the research was conducted in the absence of any commercial or financial relationships that could be construed as a potential conflict of interest.
Acknowledgments
The authors appreciated the editors and the reviewers for constructive comments.
Supplementary Material
The Supplementary Material for this article can be found online at: https://www.frontiersin.org/articles/10.3389/fmolb.2021.679474/full#supplementary-material
Supplementary Figure 1 | Flowchart of this study. TCGA, The Cancer Genome Atlas; GEO, Gene Expression Omnibus; CESC, cervical squamous cell carcinoma and endocervical adenocarcinoma; CSCC, cervical squamous cell carcinoma; DFS, disease-free survival; GO, Gene Ontology; GSEA, gene set enrichment analysis; ROC, receiver-operating characteristic; DCA, decision curve analysis; ICOS, Inducible T Cell Costimulator; ISG20, Interferon Stimulated Exonuclease Gene 20; ANGPTL4, Angiopoietin Like 4; SBDS, SBDS Ribosome Maturation Factor; LTBR, Lymphotoxin Beta Receptor.
Supplementary Figure 2 | Prognostic value of the 5-immune-gene signature for predicting DFS of TCGA CSCC patients. (A) ROC analysis, (B) Kaplan–Meier survival analysis and (C) signature risk score analysis.
Supplementary Figure 3 | GO enrichment analysis of the 5-immune-gene signature. The y-axis represented the top 10 most significant terms in BP, CC and MF; the x-axis represented counts of genes enriched in GO terms. The color gradient from red to blue meant the adjusted p-value from low to high. BP, biological process; CC, cellular component; MF, molecular function.
Supplementary Figure 4 | KEGG pathway enrichment analysis of the 5-immune-gene signature. The y-axis represented the top 30 most significant KEGG terms; the x-axis represented counts of genes enriched in KEGG terms. The color gradient from red to blue meant the adjusted p-value from low to high.
Supplementary Figure 5 | Lymphocyte infiltration analysis. The association between the five immune genes and infiltration of 22 lymphocytes in CSCC samples. Red, positive correlation; blue, negative correlation.
Supplementary Figure 6 | Single-gene GSEA analysis of ICOS discovered seven pathways significantly upregulated in the ICOS-high-expressing group. The y-axis represented the enrichment score calculated by GSEA. Vertical bars under the x-axis represented the positions of genes in the given set. Positive enrichment scores denoted the pathways were significantly enriched in ICOS-high-expressing group, while negative enrichment scores denoted the pathways were significantly enriched in ICOS-low-expressing group.
Supplementary Figure 7 | Single-gene GSEA analysis of ISG20 discovered seven pathways significantly upregulated in the ISG20-high-expressing group. The y-axis represented the enrichment score calculated by GSEA. Vertical bars under the x-axis represented the positions of genes in the given set. Positive enrichment scores denoted the pathways were significantly enriched in ISG20-high-expressing group, while negative enrichment scores denoted the pathways were significantly enriched in ISG20-low-expressing group.
Supplementary Figure 8 | Single-gene GSEA analysis of LTBR discovered one pathway significantly upregulated in the LTBR-high-expressing group. The y-axis represented the enrichment score calculated by GSEA. Vertical bars under the x-axis represented the positions of genes in the given set. Positive enrichment scores denoted the pathways were significantly enriched in LTBR-high-expressing group, while negative enrichment scores denoted the pathways were significantly enriched in LTBR-low-expressing group.
Supplementary Figure 9 | Forest plot of the relationship between ICOS, ISG20, ANGPTL4, SBDS, and LTBR expression with the overall survival of gynecological cancers. BRCA, breast invasive carcinoma; CESC, cervical squamous cell carcinoma and endocervical adenocarcinoma; OV, ovarian serous cystadenocarcinoma; UCEC, uterine corpus endometrial carcinoma; UCS, uterine carcinosarcoma; HR, hazard ratio.
References
Agulló-Ortuño, M. T., Gómez-Martín, Ó., Ponce, S., Iglesias, L., Ojeda, L., Ferrer, I., et al. (2020). Blood Predictive Biomarkers for Patients With Nony–small–cell Lung Cancer Associated With Clinical Response to Nivolumab. Clin. Lung Cancer 21, 75–85. doi:10.1016/j.cllc.2019.08.006
Ambekar, C., Das, B., Yeger, H., and Dror, Y. (2010). SBDS-deficiency Results in Deregulation of Reactive Oxygen Species Leading to Increased Cell Death and Decreased Cell Growth. Pediatr. Blood Cancer 55, 1138–1144. doi:10.1002/pbc.22700
Austin, K. M., Gupta, M. L., Coats, S. A., Tulpule, A., Mostoslavsky, G., Balazs, A. B., et al. (2008). Mitotic Spindle Destabilization and Genomic Instability in Shwachman-Diamond Syndrome. J. Clin. Invest. 118, 1511–1518. doi:10.1172/JCI33764
Bedognetti, D., Hendrickx, W., Marincola, F. M., and Miller, L. D. (2015). Prognostic and Predictive Immune Gene Signatures in Breast Cancer. Curr. Opin. Oncol. 27, 433–444. doi:10.1097/CCO.0000000000000234
Boocock, G. R. B., Morrison, J. A., Popovic, M., Richards, N., Ellis, L., Durie, P. R., et al. (2003). Mutations in SBDS Are Associated with Shwachman-Diamond Syndrome. Nat. Genet. 33, 97–101. doi:10.1038/ng1062
Budhu, A., Forgues, M., Ye, Q.-H., Jia, H.-L., He, P., Zanetti, K. A., et al. (2006). Prediction of Venous Metastases, Recurrence, and Prognosis in Hepatocellular Carcinoma Based on a Unique Immune Response Signature of the Liver Microenvironment. Cancer Cell 10, 99–111. doi:10.1016/j.ccr.2006.06.016
Cheng, Y., Qiao, X., Wang, X., and Yu, Q. (2018). Random forest Classifier for Zero-Shot Learning Based on Relative Attribute. IEEE Trans. Neural Netw. Learn. Syst. 29, 1662–1674. doi:10.1109/TNNLS.2017.2677441
Choi, H. W., Johnson-Weaver, B., Staats, H. F., and Abraham, S. N. (2020). Mast Cells for the Control of Mucosal Immunity. Mucosal Vaccin. Academic Press 13, 213–228. doi:10.1016/b978-0-12-811924-2.00013-4
Constantinidou, A., Alifieris, C., and Trafalis, D. T. (2019). Targeting Programmed Cell Death -1 (PD-1) and Ligand (PD-L1): A new era in Cancer Active Immunotherapy. Pharmacol. Ther. 194, 84–106. doi:10.1016/j.pharmthera.2018.09.008
Coutant, C., Olivier, C., Lambaudie, E., Fondrinier, E., Marchal, F., Guillemin, F., et al. (2009). Comparison of Models to Predict Nonsentinel Lymph Node Status in Breast Cancer Patients with Metastatic sentinel Lymph Nodes: A Prospective Multicenter Study. Jco 27, 2800–2808. doi:10.1200/JCO.2008.19.7418
Dalstein, V., Riethmuller, D., Prétet, J.-L., Le Bail Carval, K., Sautière, J.-L., Carbillet, J.-P., et al. (2003). Persistence and Load of High-Risk HPV Are Predictors for Development of High-Grade Cervical Lesions: A Longitudinal French Cohort Study. Int. J. Cancer 106, 396–403. doi:10.1002/ijc.11222
De Freitas, A. C., Coimbra, E. C., and Leitão Mda, C. (2014). Molecular Targets of HPV Oncoproteins: Potential Biomarkers for Cervical Carcinogenesis. Biochim. Biophys. Acta (Bba) - Rev. Cancer 1845, 91–103. doi:10.1016/j.bbcan.2013.12.004
Espert, L., Degols, G., Lin, Y.-L., Vincent, T., Benkirane, M., and Mechti, N. (2005). Interferon-induced Exonuclease ISG20 Exhibits an Antiviral Activity against Human Immunodeficiency Virus Type 1. J. Gen. Virol. 86, 2221–2229. doi:10.1099/vir.0.81074-0
Evander, M., Edlund, K., Gustafsson, A., Jonsson, M., Karlsson, R., Rylander, E., et al. (1995). Human Papillomavirus Infection Is Transient in Young Women: A Populationbased Cohort Study. J. Infect. Dis. 171, 1026–1030. doi:10.1093/infdis/171.4.1026
Finch, A. J., Hilcenko, C., Basse, N., Drynan, L. F., Goyenechea, B., Menne, T. F., et al. (2011). Uncoupling of GTP Hydrolysis from eIF6 Release on the Ribosome Causes Shwachman-diamond Syndrome. Genes Dev. 25, 917–929. doi:10.1101/gad.623011
Frenel, J.-S., Le Tourneau, C., O’Neil, B., Ott, P. A., Piha-Paul, S. A., Gomez-Roca, C., et al. (2017). Safety and Efficacy of Pembrolizumab in Advanced, Programmed Death Ligand 1-positive Cervical Cancer: Results from the Phase IB KEYNOTE-028 Trial. Jco 35, 4035–4041. doi:10.1200/JCO.2017.74.5471
Galli, S. J., and Tsai, M. (2012). IgE and Mast Cells in Allergic Disease. Nat. Med. 18, 693–704. doi:10.1038/nm.2755
Ganapathi, K. A., Austin, K. M., Lee, C.-S., Dias, A., Malsch, M. M., Reed, R., et al. (2007). The Human Shwachman-Diamond Syndrome Protein, SBDS, Associates with Ribosomal RNA. Blood 110, 1458–1465. doi:10.1182/blood-2007-02-075184
Gettinger, S., Horn, L., Jackman, D., Spigel, D., Antonia, S., Hellmann, M., et al. (2018). Five-Year Follow-Up of Nivolumab in Previously Treated Advanced Non-small-cell Lung Cancer: Results from the CA209-003 Study. Jco 36, 1675–1684. doi:10.1200/JCO.2017.77.0412
Gosink, L. J., Overall, C. C., Reehl, S. M., Whitney, P. D., Mobley, D. L., and Baker, N. A. (2017). Bayesian Model Averaging for Ensemble-Based Estimates of Solvation-free Energies. J. Phys. Chem. B 121, 3458–3472. doi:10.1021/acs.jpcb.6b09198
Gostout, B. S., Poland, G. A., Calhoun, E. S., Sohni, Y. R., Giuntoli II, R. L., McGovern, R. M., et al. (2003). TAP1, TAP2, and HLA-DR2 Alleles Are Predictors of Cervical Cancer Risk☆. Gynecol. Oncol. 88, 326–332. doi:10.1016/S0090-8258(02)00074-4
Grobmyer, S. R., and Brennan, M. F. (2003). Predictive Variables Detailing the Recurrence Rate of Soft Tissue Sarcomas. Curr. Opin. Oncol. 15, 319–326. doi:10.1097/00001622-200307000-00007
Grulich, A. E., van Leeuwen, M. T., Falster, M. O., and Vajdic, C. M. (2007). Incidence of Cancers in People with HIV/AIDS Compared with Immunosuppressed Transplant Recipients: a Meta-Analysis. The Lancet 370, 59–67. doi:10.1016/S0140-6736(07)61050-2
Grzadkowski, M. R., Sendorek, D. H., P’ng, C., Huang, V., and Boutros, P. C. (2018). A Comparative Study of Survival Models for Breast Cancer Prognostication Revisited: The Benefits of Multi-Gene Models. BMC Bioinformatics 19, 400. doi:10.1186/s12859-018-2430-9
Hanley, J. A., and McNeil, B. J. (1982). The Meaning and Use of the Area under a Receiver Operating Characteristic (ROC) Curve. Radiology 143, 29–36. doi:10.1148/radiology.143.1.7063747
Hao, Q., Wang, J., Chen, Y., Wang, S., Cao, M., Lu, H., et al. (2020). Dual Regulation of P53 by the Ribosome Maturation Factor SBDS. Cell Death Dis 11, 197. doi:10.1038/s41419-020-2393-4
Harrell, F. E., Lee, K. L., and Mark, D. B. (1996). Multivariable Prognostic Models: Issues in Developing Models, Evaluating Assumptions and Adequacy, and Measuring and Reducing Errors. Stat. Med. 15, 361–387. doi:10.1002/(SICI)1097-025810.1002/(sici)1097-0258(19960229)15:4<361::aid-sim168>3.0.co;2-4
Hato, T., Tabata, M., and Oike, Y. (2008). The Role of Angiopoietin-like Proteins in Angiogenesis and Metabolism. Trends Cardiovasc. Med. 18, 6–14. doi:10.1016/j.tcm.2007.10.003
Hollebecque, A., Meyer, T., Moore, K. N., Machiels, J.-P. H., De Greve, J., López-Picazo, J. M., et al. (2017). An Open-Label, Multicohort, Phase I/II Study of Nivolumab in Patients with Virus-Associated Tumors (CheckMate 358): Efficacy and Safety in Recurrent or Metastatic (R/M) Cervical, Vaginal, and Vulvar Cancers. Jco 35, 5504. doi:10.1200/jco.2017.35.15_suppl.5504
Hoshida, Y., Brunet, J.-P., Tamayo, P., Golub, T. R., and Mesirov, J. P. (2007). Subclass Mapping: Identifying Common Subtypes in Independent Disease Data Sets. PLoS One 2, e1195. doi:10.1371/journal.pone.0001195
Hu, X., Li, Y.-Q., Li, Q.-G., Ma, Y.-L., Peng, J.-J., and Cai, S.-J. (2018). Baseline Peripheral Blood Leukocytosis Is Negatively Correlated with T-Cell Infiltration Predicting Worse Outcome in Colorectal Cancers. Front. Immunol. 9, 2354. doi:10.3389/fimmu.2018.02354
Huang, Z., Xie, J., Lin, S., Li, S., Huang, Z., Wang, Y., et al. (2016). The Downregulation of ANGPTL4 Inhibits the Migration and Proliferation of Tongue Squamous Cell Carcinoma. Arch. Oral Biol. 71, 144–149. doi:10.1016/j.archoralbio.2016.07.011
Intaraphet, S., Kasatpibal, N., Siriaunkgul, S., Sogaard, M., Patumanond, J., Khunamornpong, S., et al. (2013). Prognostic Impact of Histology in Patients with Cervical Squamous Cell Carcinoma, Adenocarcinoma and Small Cell Neuroendocrine Carcinoma. Asian Pac. J. Cancer Prev. 14, 5355–5360. doi:10.7314/APJCP.2013.14.9.5355
Ishwaran, H., Kogalur, U. B., Blackstone, E. H., and Lauer, M. S. (2008). Random Survival Forests. Ann. Appl. Stat. 2, 841–860. doi:10.1214/08-AOAS169
Ivansson, E. L., Juko-Pecirep, I., and Gyllensten, U. B. (2010). Interaction of Immunological Genes on Chromosome 2q33 and IFNG in Susceptibility to Cervical Cancer. Gynecol. Oncol. 116, 544–548. doi:10.1016/j.ygyno.2009.10.084
Kulasingam, S. L., Hughes, J. P., Kiviat, N. B., Mao, C., Weiss, N. S., Kuypers, J. M., et al. (2002). Evaluation of Human Papillomavirus Testing in Primary Screening for Cervical Abnormalities. Jama 288, 1749–1757. doi:10.1001/jama.288.14.1749
Li, H., Ge, C., Zhao, F., Yan, M., Hu, C., Jia, D., et al. (2011). Hypoxia-inducible Factor 1 Alpha-Activated Angiopoietin-like Protein 4 Contributes to Tumor Metastasis via Vascular Cell Adhesion Molecule-1/integrin β1 Signaling in Human Hepatocellular Carcinoma. Hepatology 54, 910–919. doi:10.1002/hep.24479
Li, Y., and Teng, C. (2014). Angiopoietin-like Proteins 3, 4 and 8: Regulating Lipid Metabolism and Providing new hope for Metabolic Syndrome. J. Drug Target. 22, 679–687. doi:10.3109/1061186X.2014.928715
Liu, J., Wu, Z., Wang, Y., Nie, S., Sun, R., Yang, J., et al. (2020). A Prognostic Signature Based on Immune-Related Genes for Cervical Squamous Cell Carcinoma and Endocervical Adenocarcinoma. Int. Immunopharmacology 88, 106884. doi:10.1016/j.intimp.2020.106884
Liu, Y., Wu, L., Tong, R., Yang, F., Yin, L., Li, M., et al. (2019). PD-1/PD-L1 Inhibitors in Cervical Cancer. Front. Pharmacol. 10, 65. doi:10.3389/fphar.2019.00065
Lu, X., Jiang, L., Zhang, L., Zhu, Y., Hu, W., Wang, J., et al. (2019). Immune Signature-Based Subtypes of Cervical Squamous Cell Carcinoma Tightly Associated with Human Papillomavirus Type 16 Expression, Molecular Features, and Clinical Outcome. Neoplasia 21, 591–601. doi:10.1016/j.neo.2019.04.003
Maltby, S., Khazaie, K., and McNagny, K. M. (2009). Mast Cells in Tumor Growth: Angiogenesis, Tissue Remodelling and Immune-Modulation. Biochim. Biophys. Acta (Bba) - Rev. Cancer 1796, 19–26. doi:10.1016/j.bbcan.2009.02.001
Nam, G.-H., Lee, E. J., Kim, Y. K., Hong, Y., Choi, Y., Ryu, M.-J., et al. (2018). Combined Rho-Kinase Inhibition and Immunogenic Cell Death Triggers and Propagates Immunity against Cancer. Nat. Commun. 9, 2165. doi:10.1038/s41467-018-04607-9
Newman, A. M., Liu, C. L., Green, M. R., Gentles, A. J., Feng, W., Xu, Y., et al. (2015). Robust Enumeration of Cell Subsets from Tissue Expression Profiles. Nat. Methods 12, 453–457. doi:10.1038/nmeth.3337
Nie, D., Zheng, Q., Liu, L., Mao, X., and Li, Z. (2019). Up-regulated of Angiopoietin-like Protein 4 Predicts Poor Prognosis in Cervical Cancer. J. Cancer 10, 1896–1901. doi:10.7150/jca.29916
Nie, H., Bu, F., Xu, J., Li, T., and Huang, J. (2020). 29 Immune-Related Genes Pairs Signature Predict the Prognosis of Cervical Cancer Patients. Sci. Rep. 10, 14152. doi:10.1038/s41598-020-70500-5
Olson, B., Gribble, B., Dias, J., Curryer, C., Vo, K., Kowal, P., et al. (2016). Cervical Cancer Screening Programs and Guidelines in Low- and Middle-Income Countries. Int. J. Gynecol. Obstet. 134, 239–246. doi:10.1016/j.ijgo.2016.03.011
Pawlak, E., Karabon, L., Wlodarska-Polinska, I., Jedynak, A., Jonkisz, A., Tomkiewicz, A., et al. (2010). Influence of CTLA-4/CD28/ICOS Gene Polymorphisms on the Susceptibility to Cervical Squamous Cell Carcinoma and Stage of Differentiation in the Polish Population. Hum. Immunol. 71, 195–200. doi:10.1016/j.humimm.2009.11.006
Popescu, D.-M., Botting, R. A., Stephenson, E., Green, K., Webb, S., Jardine, L., et al. (2019). Decoding Human Fetal Liver Haematopoiesis. Nature 574, 365–371. doi:10.1038/s41586-019-1652-y
Rahimifar, S., Erfani, N., Sarraf, Z., and Ghaderi, A. (2010). CTLA-4 Gene Variations May Influence Cervical Cancer Susceptibility. Gynecol. Oncol. 119, 136–139. doi:10.1016/j.ygyno.2010.06.006
Rahmani, F., Hasanzadeh, M., Hassanian, S. M., Khazaei, M., Esmaily, H., Asef-Agah, s. A., et al. (2020). Association of a Genetic Variant in the Angiopoietin-like Protein 4 Gene with Cervical Cancer. Pathol. - Res. Pract. 216, 153011. doi:10.1016/j.prp.2020.153011
Rajkumar, T., Sabitha, K., Vijayalakshmi, N., Shirley, S., Bose, M. V., Gopal, G., et al. (2011). Identification and Validation of Genes Involved in Cervical Tumourigenesis. BMC Cancer 11, 80. doi:10.1186/1471-2407-11-80
Reich, M., Liefeld, T., Gould, J., Lerner, J., Tamayo, P., and Mesirov, J. P. (2006). GenePattern 2.0. Nat. Genet. 38, 500–501. doi:10.1038/ng0506-500
Rodríguez, A. C., Schiffman, M., Herrero, R., Wacholder, S., Hildesheim, A., Castle, P. E., et al. (2008). Rapid Clearance of Human Papillomavirus and Implications for Clinical Focus on Persistent Infections. JNCI J. Natl. Cancer Inst. 100, 513–517. doi:10.1093/jnci/djn044
Roepman, P., Jassem, J., Smit, E. F., Muley, T., Niklinski, J., van de Velde, T., et al. (2009). An Immune Response Enriched 72-gene Prognostic Profile for Early-Stage Non-small-cell Lung Cancer. Clin. Cancer Res. 15, 284–290. doi:10.1158/1078-0432.CCR-08-1258
Roh, W., Chen, P.-L., Reuben, A., Spencer, C. N., Prieto, P. A., Miller, J. P., et al. (2017). Integrated Molecular Analysis of Tumor Biopsies on Sequential CTLA-4 and PD-1 Blockade Reveals Markers of Response and Resistance. Sci. Transl. Med. 9, eaah3560. doi:10.1126/scitranslmed.aah3560
Schellens, J. H. M., Marabelle, A., Zeigenfuss, S., Ding, J., Pruitt, S. K., and Chung, H. C. (2017). Pembrolizumab for Previously Treated Advanced Cervical Squamous Cell Cancer: Preliminary Results from the Phase 2 KEYNOTE-158 Study. Jco 35, 5514. doi:10.1200/jco.2017.35.15_suppl.5514
Schumacher, T. N., and Schreiber, R. D. (2015). Neoantigens in Cancer Immunotherapy. Science 348, 69–74. doi:10.1126/science.aaa4971
Shibata, K., Nakayama, T., Hirakawa, H., Hidaka, S., and Nagayasu, T. (2010). Clinicopathological Significance of Angiopoietin-like Protein 4 Expression in Oesophageal Squamous Cell Carcinoma. J. Clin. Pathol. 63, 1054–1058. doi:10.1136/jcp.2010.078600
Song, D., Li, H., Li, H., and Dai, J. (2015). Effect of Human Papillomavirus Infection on the Immune System and its Role in the Course of Cervical Cancer. Oncol. Lett. 10, 600–606. doi:10.3892/ol.2015.3295
Song, W., Ren, J., Wang, C., Ge, Y., and Fu, T. (2020). Analysis of Circular RNA-Related Competing Endogenous RNA Identifies the Immune-Related Risk Signature for Colorectal Cancer. Front. Genet. 11, 505. doi:10.3389/fgene.2020.00505
Song, Z.-y., Chao, F., Zhuo, Z., Ma, Z., Li, W., and Chen, G. (2019). Identification of Hub Genes in Prostate Cancer Using Robust Rank Aggregation and Weighted Gene Co-expression Network Analysis. Aging 11, 4736–4756. doi:10.18632/aging.102087
Spalinger, M. R., Manzini, R., Hering, L., Riggs, J. B., Gottier, C., Lang, S., et al. (2018). PTPN2 Regulates Inflammasome Activation and Controls Onset of Intestinal Inflammation and Colon Cancer. Cel Rep. 22, 1835–1848. doi:10.1016/j.celrep.2018.01.052
Subramanian, A., Tamayo, P., Mootha, V. K., Mukherjee, S., Ebert, B. L., Gillette, M. A., et al. (2005). Gene Set Enrichment Analysis: A Knowledge-Based Approach for Interpreting Genome-wide Expression Profiles. Proc. Natl. Acad. Sci. 102, 15545–15550. doi:10.1073/pnas.0506580102
Tanaka, J., Irié, T., Yamamoto, G., Yasuhara, R., Isobe, T., Hokazono, C., et al. (2015). ANGPTL4 Regulates the Metastatic Potential of Oral Squamous Cell Carcinoma. J. Oral Pathol. Med. 44, 126–133. doi:10.1111/jop.12212
Theoharides, T. C., and Conti, P. (2004). Mast Cells: The JEKYLL and HYDE of Tumor Growth. Trends Immunol. 25, 235–241. doi:10.1016/j.it.2004.02.013
Utrera-Barillas, D., Castro-Manrreza, M., Castellanos, E., Gutiérrez-Rodríguez, M., Arciniega-Ruíz de Esparza, O., García-Cebada, J., et al. (2010). The Role of Macrophages and Mast Cells in Lymphangiogenesis and Angiogenesis in Cervical Carcinogenesis. Exp. Mol. Pathol. 89, 190–196. doi:10.1016/j.yexmp.2010.06.002
Varricchi, G., Galdiero, M. R., Loffredo, S., Marone, G., Iannone, R., Marone, G., et al. (2017a). Are Mast Cells MASTers in Cancer? Front. Immunol. 8, 424. doi:10.3389/fimmu.2017.00424
Varricchi, G., Galdiero, M. R., Marone, G., Granata, F., Borriello, F., and Marone, G. (2017b). Controversial Role of Mast Cells in Skin Cancers. Exp. Dermatol. 26, 11–17. doi:10.1111/exd.13107
Vickers, A. J., and Elkin, E. B. (2006). Decision Curve Analysis: A Novel Method for Evaluating Prediction Models. Med. Decis. Making 26, 565–574. doi:10.1177/0272989X06295361
Walboomers, J. M. M., Jacobs, M. V., Manos, M. M., Bosch, F. X., Kummer, J. A., Shah, K. V., et al. (1999). Human Papillomavirus Is a Necessary Cause of Invasive Cervical Cancer Worldwide. J. Pathol. 189, 12–19. doi:10.1002/(SICI)1096-989610.1002/(sici)1096-9896(199909)189:1<12::aid-path431>3.0.co;2-f
Wang, S. S., Bratti, M. C., Rodríguez, A. C., Herrero, R., Burk, R. D., Porras, C., et al. (2009). Common Variants in Immune and DNA Repair Genes and Risk for Human Papillomavirus Persistence and Progression to Cervical Cancer. J. Infect. Dis. 199, 20–30. doi:10.1086/595563
Wessels, D., Srikantha, T., Yi, S., Kuhl, S., Aravind, L., and Soll, D. R. (2006). The Shwachman-Bodian-Diamond Syndrome Gene Encodes an RNA-Binding Protein that Localizes to the Pseudopod of Dictyostelium Amoebae during Chemotaxis. J. Cel Sci. 119, 370–379. doi:10.1242/jcs.02753
Wilk, M., Liszka, ł., Paleń, P., Gabriel, A., and Laudański, P. (2010). Intensity of Angiogenesis and Mast Cell Infiltration in Cervical Intraepithelial and Invasive Lesions - Are They Correlated? Pathol. - Res. Pract. 206, 217–222. doi:10.1016/j.prp.2009.10.005
Wolbers, M., Koller, M. T., Witteman, J. C. M., and Steyerberg, E. W. (2009). Prognostic Models with Competing Risks. Epidemiology 20, 555–561. doi:10.1097/EDE.0b013e3181a39056
Yu, G., Wang, L.-G., Han, Y., and He, Q.-Y. (2012a). ClusterProfiler: An R Package for Comparing Biological Themes Among Gene Clusters. OMICS: A J. Integr. Biol. 16, 284–287. doi:10.1089/omi.2011.0118
Yu, L., Han, Y., and Berens, M. E. (2012b). Stable Gene Selection from Microarray Data via Sample Weighting. Ieee/acm Trans. Comput. Biol. Bioinforma. 9, 262–272. doi:10.1109/TCBB.2011.47
Zhang, M., Wang, X., Chen, X., Zhang, Q., and Hong, J. (2020). Novel Immune-Related Gene Signature for Risk Stratification and Prognosis of Survival in Lower-Grade Glioma. Front. Genet. 11, 363. doi:10.3389/fgene.2020.00363
Zhang, Z., Fye, S., Borecki, I. B., and Rader, J. S. (2014). Polymorphisms in Immune Mediators Associate with Risk of Cervical Cancer. Gynecol. Oncol. 135, 69–73. doi:10.1016/j.ygyno.2014.07.106
Zhou, Z., Wang, N., Woodson, S. E., Dong, Q., Wang, J., Liang, Y., et al. (2011). Antiviral Activities of ISG20 in Positive-Strand RNA Virus Infections. Virology 409, 175–188. doi:10.1016/j.virol.2010.10.008
Keywords: cervical squamous cell carcinoma, immune, gene, PD1/PD-L1, prognostic, nomogram, signature
Citation: Yang H, Han X and Hao Z (2021) An Immune-Gene-Based Classifier Predicts Prognosis in Patients With Cervical Squamous Cell Carcinoma. Front. Mol. Biosci. 8:679474. doi: 10.3389/fmolb.2021.679474
Received: 11 March 2021; Accepted: 21 June 2021;
Published: 05 July 2021.
Edited by:
Matteo Becatti, University of Firenze, ItalyReviewed by:
Zeeshan Ahmed, The State University of New Jersey, United StatesVishal Acharya, Institute of Himalayan Bioresource Technology, India
Copyright © 2021 Yang, Han and Hao. This is an open-access article distributed under the terms of the Creative Commons Attribution License (CC BY). The use, distribution or reproduction in other forums is permitted, provided the original author(s) and the copyright owner(s) are credited and that the original publication in this journal is cited, in accordance with accepted academic practice. No use, distribution or reproduction is permitted which does not comply with these terms.
*Correspondence: Zengping Hao, aF96ZW5ncGluZ0BvdXRsb29rLmNvbQ==
†These authors have contributed equally to this work