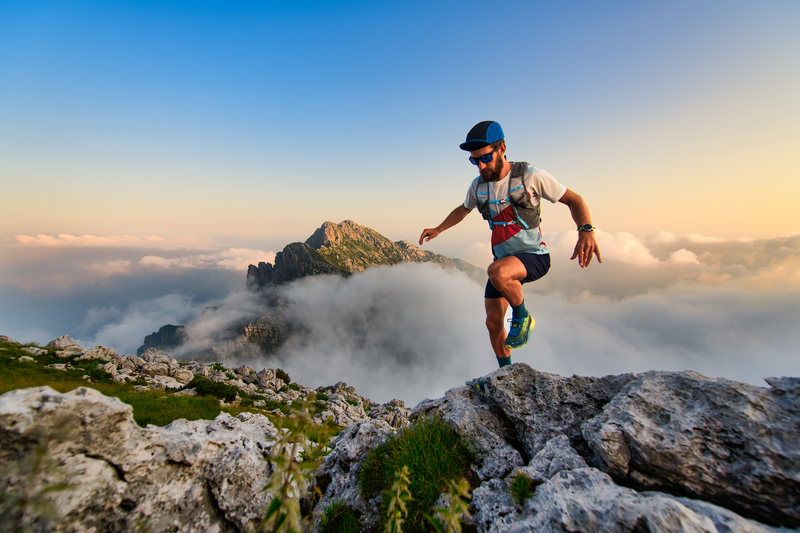
94% of researchers rate our articles as excellent or good
Learn more about the work of our research integrity team to safeguard the quality of each article we publish.
Find out more
ORIGINAL RESEARCH article
Front. Mol. Biosci. , 28 June 2021
Sec. Biological Modeling and Simulation
Volume 8 - 2021 | https://doi.org/10.3389/fmolb.2021.676235
This article is part of the Research Topic Experiments and Simulations: A Pas de Deux to Unravel Biological Function View all 15 articles
Ubiquitin is a small protein at the heart of many cellular processes, and several different protein domains are known to recognize and bind ubiquitin. A common motif for interaction with ubiquitin is the Ubiquitin Interacting Motif (UIM), characterized by a conserved sequence signature and often found in multi-domain proteins. Multi-domain proteins with intrinsically disordered regions mediate interactions with multiple partners, orchestrating diverse pathways. Short linear motifs for binding are often embedded in these disordered regions and play crucial roles in modulating protein function. In this work, we investigated the structural propensities of UIMs using molecular dynamics simulations and NMR chemical shifts. Despite the structural portrait depicted by X-crystallography of stable helical structures, we show that UIMs feature both helical and intrinsically disordered conformations. Our results shed light on a new class of disordered UIMs. This group is here exemplified by the C-terminal domain of one isoform of ataxin-3 and a group of ubiquitin-specific proteases. Intriguingly, UIMs not only bind ubiquitin. They can be a recruitment point for other interactors, such as parkin and the heat shock protein Hsc70-4. Disordered UIMs can provide versatility and new functions to the client proteins, opening new directions for research on their interactome.
Protein biochemistry relied for a long time on the paradigm that a protein’s function is tied to its three-dimensional structure. Over the past 20 years, several proteins or regions in proteins that do not fit within the structure-function paradigm have been reported (Wright and Dyson, 1999; Chen and Kriwacki, 2018; Milles et al., 2018). They are known as intrinsically disordered proteins (IDPs) or regions (IDRs). IDPs and IDRs lack stable tertiary contacts, are highly dynamic, pliable, and typically do not exhibit stable secondary structures. Proteins containing IDRs constitute 30–44% of eukaryotic proteomes (Perdigão et al., 2015). They attain multiple and chameleon conformations for interactions with different partners (Wright and Dyson, 2014; Bugge et al., 2020). Consequently, the modulation of the structural landscape of an IDP can result in opposing actions on different — or even the same — binding partners, making them elusive, but attractive targets to study (Metallo, 2010; Flock et al., 2014). IDPs and IDRs can also be involved in allosteric mechanisms with key roles in many processes, including modulation of protein-protein interactions and catalytic activities of enzymes (Ma et al., 2011; Li et al., 2017; Berlow et al., 2018; Guarnera and Berezovsky, 2019; Tee et al., 2020).
IDPs and IDRs often interact with binding partners through short stretches of conserved residues, called short linear motifs (SLiMs), embedded in otherwise non-conserved regions (Davey et al., 2012; Van Der Lee et al., 2014). The occurrence of two or more SLiMs in the same IDP/IDR can increase the interaction strength via avidity by multivalent interactions (Van Roey et al., 2014; Fung et al., 2018). Although individual SLiMs are short and mostly participate in transient interactions, they are essential to protein binding specificity and function (Bugge et al., 2020; Kumar et al., 2020).
Some functional motifs of proteins that were traditionally defined as helical elements have been recently reclassified as disordered SLiMs, such as the Bcl-2 Homology 3 motifs (Hinds et al., 2007; Aouacheria et al., 2015). Another well-known functional motif traditionally considered to have a high helical propensity (Scott et al., 2015) is the so-called Ubiquitin Interacting Motif (UIM) or ‘LALAL-motif’. UIMs are motifs of approximately 20 residues and were described for the first time in the 26S proteasome subunit PSD4/RPN-10 to bind ubiquitin (Young et al., 1998; Hofmann and Falquet, 2001), now representing the archetypal UIM in the families of ubiquitin binding domains (Scott et al., 2015). UIMs can be found, often in tandem or triplets, in a multitude of proteins involved in ubiquitination, ubiquitin metabolism, or that interact with ubiquitin-like modifiers (Buchberger, 2002). UIM binding partners are not limited to ubiquitin. As an example, ubiquitin-like proteins involved in autophagy feature an interface to recruit UIMs (Marshall et al., 2019; Sora et al., 2020). The UIM consensus motif is X-Ac-Ac-Ac-X-Φ-X-X-Ala-Φ-X-X-Ser-X-X-Ac-X, where Φ represents any hydrophobic residues (often Leu or Ile), Ac represents an acidic residue (Glu, Asp), and X loosely conserved positions (Hofmann and Falquet, 2001; Scott et al., 2015).
Among different UIMs, we focused our attention on the poorly characterized UIM within the C-terminus (residues 306–361) of the human ataxin-3 (AT-3). AT-3 is a multi-domain polyglutamine deubiquitinating enzyme used as a model system to study polyglutamine neurodegenerative diseases (Burnett et al., 2003; Carvalho et al., 2018; Invernizzi et al., 2012). AT-3 contains two UIM regions (UIM1 and UIM2) in the central part of the protein, surrounded by disordered regions (Burnett et al., 2003; Invernizzi et al., 2013; Masino et al., 2003; Sicorello et al., 2018, 2021). AT-3 also undergoes alternative splicing, and its isoforms differ in the C-terminus (Harris et al., 2010). Among the main isoforms, one isoform contains a third UIM, called UIM3 (Figure 1A (Goto et al., 1997; Bettencourt et al., 2010). The UIM3-containing isoform is widely expressed and appears to be the predominant form in the human brain (Ichikawa et al., 2001; Harris et al., 2010). Furthermore, AT-3 UIMs are involved in multivalent binding to the Ubl domain of the E3 ubiquitin ligase parkin (Bai et al., 2013; Aguirre et al., 2018). It has also been suggested that the three UIMs of AT-3 interact with the heat shock protein Hsc70-4 in Drosophila melanogaster (Johnson et al., 2020).
FIGURE 1. The C-terminus of the UIM3 isoform of ataxin-3 is predicted disordered and includes a UIM. (A) The figure illustrates the amino acid sequence of AT-3306-361. The blue box indicates UIM3 of AT-3 (residues E336-T350). (B) The panel shows the residue-wise prediction of the S2 order parameter in AT-3306-361 from DynaMine (purple). The dashed lines show the thresholds of S2 predicted scores to classify residues as ordered (S2 ≥ 0.75), context-dependent (0.65 < S2 < 0.75), and disordered (S2 ≤ 0.65). (C) The panel shows the residue-wise prediction of disorder propensity in AT-3306-361 from the Multilayered Fusion-based Disorder predictor v. 2.00 (MFDp2, green), and SPOT-Disorder2 (SPOTdis.2, yellow) predictors. The dashed line shows the threshold of disorder probability to classify the residues as disordered ( ≥ 0.5) and ordered ( < 0.5). (D) The plot shows the residue-wise prediction of secondary structure propensity (Coil orange, Helix violet, Strand teal) from PSI-PRED. The dashed line shows the threshold of secondary structure probability for each structural class to classify the residues as coil, helix or strand ( ≥ 0.5). The light blue box indicates UIM3. The predictors report AT-3306-361 as a mainly disordered tract with propensity to order and helical structures in proximity of UIM3.
Recent advances in all-atom molecular dynamics (MD) simulations in terms of enhanced sampling (Abrams and Bussi, 2013; Spiwok et al., 2015; Bonomi et al., 2017; Sugita et al., 2019; Bussi and Laio, 2020) and physical models for disordered proteins (Best, 2017; Huang and MacKerell, 2018) offer a possibility to unveil heterogeneous conformational ensembles at the atomic level. The presence of multiple UIMs in the disordered C-terminus of AT-3 that are involved in the binding of different interaction partners makes this protein a good model to investigate the structural propensities of UIM using molecular dynamics simulations and chemical shifts from NMR.
We here report a study on the structural propensity and dynamics of the C-terminus of the UIM3-containing isoform of AT-3 (residues 306–361, AT-3306-361). We used two different methods to enhance the sampling of the MD simulations based on temperature exchange or bias along with selected collective variables. We also employed three different force fields (available at the time we performed the simulations) suitable to study disordered/unfolded states of proteins (Best and Mittal, 2010; Knott and Best, 2012; Lindorff-Larsen et al., 2012; Best et al., 2014). The simulation results for AT-3306-361 were then been compared to NMR data for other UIMs in solution (Sgourakis et al., 2010; Lim et al., 2011; Lange et al., 2012; Anamika et al., 2014; Shi et al., 2014; Wen et al., 2014; Sicorello et al., 2018) or to NMR data recorded in this work. In addition, we validated the simulations against previously published NMR chemical shifts of a construct of AT-3 including UIM3 (Bai et al., 2013).
We find that UIM-containing regions can account for both stable helical conformations and more disordered ones, which, in turn, are the more pliable toward a wider range of interactors beyond ubiquitin itself. Thus, our study provides a broader view on the ubiquinome through uncovering an enhanced structural heterogeneity within the groups of UIMs.
For the sequence-based prediction of secondary structure propensity, we used the PSIPRED predictor (Jones, 1999). We performed disorder prediction from the amino acid sequence, using DynaMine (Cilia et al., 2013), Multilayered Fusion-based Disorder predictor v. 2.00 (MFDp2, (Mizianty et al., 2013), and SPOT-Disorder2 (Hanson et al., 2019). MFDp2 is a meta-method that combines disorder probabilities predicted at residue- and sequence-level by MFDp and DisCon, respectively, and uses post-processing filters and sequence alignment. SPOT-Disorder2 combines long short-term memory with deep bidirectional neural networks to capture non-local and long-range interactions, integrating information from evolutionary profiles of aligned sequences. DynaMine allows high-quality predictions of protein backbone dynamics using an accurate NMR data set for training.
REMD simulations were performed by GROMACS (Groninger MAchine for Chemical Simulation) using a conformation of the C-terminus of AT-3 (56 residues, 306–361, AT-3306-361) initially generated with Crystallography and NMR System version 1.3 (Brunger, 2007) as the starting structure. We further imposed a helical structure for the region E336-T357, according to the secondary structure prediction by PSIPRED, using MODELLER 9.14 (Eswar et al., 2007). In particular, we selected the model that lacked intermolecular side-chain contacts (defined as intramolecular contacts at a distance in sequence over three residues).
The models were soaked in a dodecahedron box of water molecules with periodic boundary conditions, with a minimal distance for the protein atoms from the box edges of at least 14 Å. We applied the Particle-Mesh Ewald method (Darden et al., 1993) with a 1.2 Å grid spacing. Van der Waals and Coulomb interactions were truncated at 12 Å. Na+ and Cl− counterions were added to the system to neutralize the overall charge and to simulate a physiological ionic strength (i.e., 150 mM).
Each system was initially relaxed by 10,000 steps of energy minimization by the steepest descent method. The optimization step was followed by 50 ps of solvent equilibration at 300 K, while restraining the protein atomic positions using a harmonic potential. The systems were subsequently simulated for five ns at 300 K at a constant pressure of 1 bar (NPT ensemble) with coupling constants of 5 and 10 ps, respectively. From the NPT trajectories, we selected a conformation with the volume close to the average volume of the trajectory and used as the starting point for the subsequent NVT preparatory step at 300 K for 20 ns. The 64 initial conformations for REMD simulations were selected from different points (between 10 and 20 ns) along the NVT trajectory using the v-rescale thermostat (Bussi et al., 2007). Other details are reported in the parameter files in the GitHub repository.
In the temperature REMD scheme a number of different copies (replicas) of the system were simulated in parallel at different temperatures and exchanges of configurations are attempted periodically between pairs of replicas. The advantage of this method is that if the trajectory is temporarily trapped in a local minimum can exchange with a replica at a higher temperature and cross high-energy barriers. We carried out REMD simulations using 64 replicas, each replica for 50 ns for a collective simulation time of 3.2 μs. Each replica was run at a different temperature in the range 299–360 K. We selected the temperature spacing between each neighboring replica to ensure an exchange probability higher than 0.2. The replica-exchanges were attempted every ten ps.
The WT-metaD (Barducci et al., 2008) simulations were performed using GROMACS and the open-source, community-developed PLUMED library (Bonomi et al., 2009; PLUMED Consortium, 2019). In the WT-metaD simulations, the sampling of the free energy surface is enhanced by adding a history-dependent potential to a set of collective variables (CVs). Similar approaches have been applied to simulations of other intrinsically disordered proteins and peptides (Do et al., 2014; Palazzesi et al., 2015). We employed two CVs in our simulations, i.e., 1) the Cα radius of gyration, and 2) alphabeta, a CV that measures the similarity of each ψ dihedral angle of AT-3306-361 to a reference value of 0.7854 rad, which corresponds to a-helix. Gaussian potentials with an initial height of 0.12 kcal/mol were added to the time-dependent potential every two ps. We used an initial bias factor of four for rescaling the Gaussian height following the WT-metaD scheme. In addition, we used Gaussian widths of 0.2 and one for each CV, respectively. We collected one-μs WT-metaD simulations. We used an extended and disordered conformation of the peptide generated by Profasi (Irbäck and Mohanty, 2006) as the initial structure for the WT-metaD simulations.
For the REMD simulations, we employed four different combinations of protein force fields and water models in our simulations: 1) Amber ff03w [ff03w (Best and Mittal, 2010)] with TIP4P/2005 (Abascal and Vega, 2005), 2) Amber ff03ws [ff03ws (Best et al., 2014)] with TIP4P/2005, in which the protein–water pair interactions have been modified to improve the description of disordered proteins, 3) CHARMM22* (Piana et al., 2011) with TIP3P (CHARMM22*1) (Jorgensen et al., 1983) or 4) TIPS3P (CHARMM22*2) (MacKerell, et al., 1998). WT-metaD was carried out only for ff03w, ff03ws, and CHARMM22*2.
The replica at 304 K was used for the analysis. To study the temperature distributions, we converted each replica to be continuous to the simulation time to follow each replica through the temperature space. We used DSSP (Kabsch and Sander, 1983) to estimate the helical content. We used MDAnalysis (Michaud-Agrawal et al., 2011) to calculate the root mean square deviation (RMSD) of UIM3 of AT-3306-361 with respect to the starting helical conformation. We considered the Cβ atom of A343 and the backbone (Cα, C, O, N) atoms of the residues E336-T350 of UIM3 for rigid body superposition and the RMSD calculation.
For the WT-metaD simulations, we reconstructed the one-dimensional free energy landscape from the deposited bias during the simulation with a stride value of 10,000. We extracted four ensembles of structures of AT-3306-361 from the CHARMM22*2 metadynamics trajectory with alphabeta values in the ranges of 1) 9–17, 2) 18–23, 3) 24–30, and 4) 31–34, respectively. On these ensembles, we estimated the propensity to helical structures using the DSSP dictionary (Kabsch and Sander, 1983) and including a-helix, π-helix and 3.10 helix in the analyses. We applied the MDplot R/CRAN package (Margreitter and Oostenbrink, 2017) to calculate a residue-wise persistence degree of helical secondary structures. On the ensembles selected from the CHARMM22*2 metadynamics trajectory, we used MDAnalysis to calculate the RMSD of UIM3 of AT-3306-361 (residues 336–350) with respect to: 1) the starting structure of AT-3306-361 used for the REMD simulations, 2) the experimental structure of yeast vps27 UIM1 [residue E259-E273, PDB entry 1Q0W (Swanson et al., 2003)], human proteasome subunit S5a UIM1 [residue A212-E226, PDB entry 1YX5 (Wang et al., 2005)] and UIM2 [residue E283-G297, PDB entry 1YX6 (Wang et al., 2005)], and mouse RAP80 UIM1 [residues E81-E95, PDB entry 3A1Q (Sato et al., 2009)] in complex with ubiquitin. We used the same subset of atoms for structural alignment and RMSD calculations, i.e., the Cβ atom of A343 and the backbone (Cα, C, O, N) atoms of residues E336-T350 of AT-3306-361.
To evaluate the REMD ensembles, we calculated the backbone chemical shifts as a function of the simulation time using PPM (Li and Brüschweiler, 2012) and compared them to the available NMR backbone chemical shifts for a construct of AT-3 including UIM3 [residues 194–361 (Bai et al., 2013)]. To compare the calculated backbone chemical shifts with the experimental ones, we used a reduced χ2 metric as previously described (Papaleo et al., 2018), using the Python package delta_cs (Sora et al., 2021). The reduced χ2 relates the squared deviation between the predicted and experimental value and normalized by the variance of the chemical shift predictor for each type of chemical shift and the total number of chemical shifts. Lower values of χ2red metric indicate a better agreement between experimental and calculated chemical shifts.
We produced recombinant yeast ubiquitin in E. coli strain BL21 using a pMCSG7vector. Ubiquitin was expressed as a 6X histidine (6His)-TEV N-tagged fusion protein by the addition of 1 mM IPTG and incubation 5 h at 37°C. Cells were harvested, resuspended in a lysis buffer (50 mM Tris pH 8.0, 150 mM NaCl, 10 mM imidazole) plus protease inhibitor mixture (Roche), and disrupted by sonication. 6His-TEV-Ubiquitin was affinity purified with Ni Sepharose 6Fast Flow (GE Healthcare) and eluted with 20 mM Na2HPO4.2H2O, 0.5M NaCl, 500 mM imidazole, pH7.4.
For the construct of human AT-3 including residues 182–291 (AT-3182–291) we cloned it in frame with glutathione S-transferase (GST) in a pGEX-6P-1 (GE Healthcare LifeSciences, Little Chalfont, England) plasmid and expressed in E. coli BL21 Codon Plus strain (Stratagene, La Jolla, CA, United States) in auto-inducing growth minimal medium (Tyler et al., 2005). For the production of 15N labeled proteins, we included 15NH4Cl or (15NH4)4SO4 1 g/l as the sole nitrogen source. For 15N13C labeled proteins, we added 15NH4Cl 1 g/l or (15NH4)4SO4 1 g/l and substituted the carbon source with a solution of 0.4% 13C-glucose. Cells were harvested, resuspended in a lysis buffer (50 mM KH2PO4, 50 mM Na2HPO4, 300 mM NaCl, pH 7.4) to which DNAse (10 μg/ml, Sigma-Aldrich, St. Louis, MO, United States) and PMSF (1 mM) were added and then disrupted by sonication. We purified the soluble protein fractions by affinity chromatography with Glutathione Sepharose four Fast Flow resin (GE Healthcare Bio-Sciences, Uppsala, Sweden) and subsequently in-site cleaved with 60 units of PreScission Protease (HRV 3C Protease Sino Biological inc., Beijing, P.R.China) per ml of resin. We then further purified the eluted samples by size-exclusion chromatography on a Superdex 75 10/300 GL column (GE Healthcare LifeSciences, Little Chalfont, England) in PBS buffer, pH 7.4, 150 mM NaCl.
We purchased peptide arrays from Intavis and modified the procedures for blocking and probing the arrays from (Frank and Dubel, 2006). Briefly, the peptide array was re-hydrated through incubation in 100% ethanol and transferred in TBS (137 mM NaCl, 2.7 mM KCl, and 50 mM Tris, pH 7.0) for 5 min at room temperature. The blocking was performed by incubating the membrane 4°C overnight in TBS with 5% nonfat dry milk (MBS). Membranes were then incubated with 10 ml MBS with 2 mg/ml of 6His-TEV-Ubiquitin for 3 h at room temperature. The peptide array was then rinsed with a blocking buffer and then incubated with anti-6His antibody (Sigma Aldrich C6594) diluted 1:1,000 in the blocking buffer for 2 h at room temperature. The membrane was washed in Tween TBS 3 times and then incubated 1 h at room temperature with the secondary antibody (anti-mouse AP from Immunstar kit 170–5010).
NMR samples were prepared by dissolving the purified protein in 90% PBS buffer, pH 7.4, 150 mM NaCl and 10% D2O with4,4-dimethyl-4-silapentane-1-sulfonic acid (DSS) added as internal calibration standard. Protein concentrations were from 0.5 to 1 mM in a volume of 400 μl. Assignment of backbone chemical shifts was performed on a 0.5 mM 13C, 15N AT-3182-291 sample and 1H, 15N-HSQC spectrum and the following triple resonance spectra were recorded, HNCA, HN(CO)CA, HNCO, HN(CA)CO, CBCA(CO)NH, CBCANH, CC(CO)NH and H(CCO)NH (all pulse programs from Agilent BioPack) at 25 °C on a Varian Unity Inova 750 and 800 Mhz instruments. NMR data were processed by NMRPipe (Delaglio et al., 1995) and analyzed using CCPNMR (Skinner et al., 2016). The chemical shift assignment for AT-3182-291 is deposited in the Biological Magnetic Resonance Bank (BMRB) with entry 50888.
We downloaded NMR chemical shift data from the Biological Magnetic Resonance Bank (BMRB) for STAM1 [BMRB entry 17065 (Lim et al., 2011)], STAM2 [BMRB entry 18403 (Lange et al., 2012)], vps27 [BMRB entry 16114 (Sgourakis et al., 2010)], USP25 [including UIM1 and UIM2, BMRB entry 19111 (Shi et al., 2014)], RAP80 ([including UIM1 and UIM2, BMRB entry 19774 (Anamika et al., 2014)], USP28 [BMRB entries 18560 and 19077 (Wen et al., 2014)], and AT-3 [including UIM1, UIM2, and UIM3, BMRB entry 27380 (Sicorello et al., 2018)]. Furthermore, we included in the analyses the chemical shifts for AT-3182-291 (including UIM1 and UIM2 of AT-3) from experiments performed in this study, along with previously published data for an AT-3 construct including the UIM3 residues 194–361 (Bai et al., 2013). We used the backbone chemical shifts from these NMR sets to predict the secondary structure propensity by δ2D (Camilloni et al., 2012).
We calculated the helical wheel projections of UIMs of the selected proteins by the freely available NetWheels web-based application (Mól et al., 2018).
We used NMR data for AT-3 UIM3 from a previous publication (including residues 194–361) (Bai et al., 2013). MD simulations of such a long and disordered region are challenging, due to several conformational transitions to sample and a large number of degrees of freedom involved. We thus focused on a shorter construct for MD simulations, i.e., AT-3306-361.
We employed two different methods to characterize the conformational ensemble of AT-3306-361 in solution, i.e., REMD and WT-metaD. These methods provide the possibility to enhance the sampling of the conformational space in MD simulations while keeping a description of both the protein and the solvent at the atomic level. We also evaluated the influence of different force-field descriptions for both the protein and the solvent: Amber ff03w-TIP4P/2005, Amber ff03ws-TIP4P/2005, CHARMM22*-TIP3P, CHARMM22*-TIPS3P (indicated as ff03w, ff03ws, CHARMM22*1, and CHARMM22*2, respectively) to assess the reproducibility of the result and identify force-field dependent properties. These approaches enabled us 1) to address if AT-3306-361 is stable or not in a helical conformation in solution, 2) to estimate the population of the helical conformations and compare them to the available experimental information on a variant of AT-3 (residues 194–361) characterized by NMR and on other known UIMs that have been similarly studied by solution NMR (see Materials and Methods) or recorded by us in this work ( AT-3182-291), 3) to identify conformations that resemble ubiquitin-bound states in the ensemble of the free AT-3306-361 region through the comparison of our ensembles to the experimentally known ubiquitin-bound UIM structures of other proteins.
UIMs are thought to assume an α-helical structure also in the absence of ubiquitin binding (Hofmann and Falquet, 2001; Scott et al., 2015). Nevertheless, many investigations on UIMs focus on characterizing the binding with ubiquitin, making it unclear if UIMs present transient propensity to disordered conformations in their free state, a typical trait of SLiMs (Davey et al., 2012; Van Roey et al., 2012, 2014). In AT-3306-361, UIM3 spans residues E336-T350 [(Donaldson et al., 2003), Figure 1A]. To identify inherent structural properties, we used four sequence-based methods to predict disorder or secondary structure propensity (Nielsen and Mulder, 2019). Overall, the predictors showed a disordered state for the AT-3306-361 region with propensity to order and helicity around UIM3 (Figures 1B–D).
We subsequently modeled this region as an α-helix in the starting structure for the REMD simulations. In the REMD simulations, the UIM3 region assumed both helical and non-helical conformations (Figure 2). The REMD simulations with ff03w and ff03ws showed higher helical content for UIM3 (∼60% and 56%, respectively) than with CHARMM22*1, and CHARMM22*2 (∼31% and 25%, respectively) (Figure 2). In the case of CHARMM22*2 simulation, we observed a more disordered ensemble for UIM3, with helical content < 20% in the region Q341-L348.
FIGURE 2. AT-3306-361 in the free state assumes both helical and non-helical conformations. The panels show the per-residue helical content of each replica at 304 K from the REMD simulations of the AT-3306-361 for each combination of protein force fields and water models: (A) CHARMM22*-TIP3P (CHARMM22*1, green), (B) CHARMM22*-TIPS3P (CHARMM22*2, pink), (C) Amber ff03w-TIP4P/2005 (ff03w, orange), and (D) Amber ff03ws-TIP4P/2005 (ff03ws, blue). The residues of UIM3 (residues 336–350) are highlighted by the black bars. The REMD simulations with ff03w and ff03ws show high helical content for UIM3 while the CHARMM22*2 simulation reports more disordered and heterogeneous conformations of UIM3.
An NMR backbone chemical shift assignment for AT-3306-361is available (Bai et al., 2013). We used this set of experimental data to evaluate the REMD structural ensembles. In particular, we calculated backbone chemical shifts as a function of the simulation time and compared these to the experimental values. The calculated chemical shifts from our simulations converged after only 5 ns of REMD simulations (Figure 3). They are in agreement with the experimental values with low χ2red values of CHARMM22* simulations, but not in the ff03w/ff03ws simulations (Figure 3). In ff03w/ff03ws, the simulations converged to structures that are unlikely to resemble the ones observed by solution NMR, probably due to the high helicity sampled by these trajectories.
FIGURE 3. The ensemble of AT-3306-361 from the CHARMM22*2 REMD simulation better resembles the experimental chemical shifts. The plot shows the comparison between experimental Cα chemical shifts and calculated Cα chemical shifts from the REMD simulations. Similar results have been achieved using the other backbone (N, HN, C, O, Hα) and Cβ chemical shifts. Among the protein force fields and water models tested in this study, the CHARMM22*2 REMD simulation shows a better agreement with the experimental NMR measurements.
The differences in the sampling of helical structures in the REMD simulations with different force fields could be ascribed to either force field differences or limitation of the conformational sampling. Since we started from an α-helical conformation the simulation time might not have been sufficient, even with the temperature replica-exchange, to allow the protein to exhaustively explore the conformational space in the different force-field simulations. Thus, to be able to discriminate between these two scenarios, we applied another method for enhanced sampling, based on metadynamics. In particular, we carried out simulations with WT-metaD, which should allow a more extensive exploration of the conformational space by using the Cα radius of gyration and alphabeta as collective variables (CVs) to bias the systems. Alphabeta is a collective variable in which we measured similarity for all ψ dihedral angles of the peptide to the ψ dihedral angles of an ideal α-helix (Figure 4). It is a suitable CV to enhance the sampling of disordered regions which might have local propensity for helical structures (Granata et al., 2015). The alphabeta estimated by the three different force fields were different with the CHARMM22*2 simulation providing more disordered conformations (i.e., alphabeta between 8 and 15 residues in Figure 4). As also observed in the REMD simulations, the ff03w ensemble was characterized by a higher helical content, suggesting that the difference observed is not necessarily related to limitations in the sampling or initial conformation, but to differences in force field parameters. In this context, overstabilization of helical conformations with ff03w has been observed also in other studies (Huang and Mackerell, 2014). The modification of the ff03ws force field with more balanced interactions between the protein and the solvent (Best et al., 2014) partially mitigates this effect, providing an ensemble of structures with a lower helical propensity, including also disordered states corresponding to the ones observed for CHARMM22*2 (Figure 4).
FIGURE 4. AT-3306-361 is characterized by a disordered ensemble with a low structural propensity and heterogeneous helical states. (A) The plot shows the one-dimensional free energy profile associated with the collective variable alphabeta for the ff03w (orange), ff03ws (blue), and CHARMM22*2 (pink) metadynamics simulations. (B) The plot shows the distribution of alphabeta values expressed as a percentage, i.e., alphabeta values divided by the total number of torsional angles considered. Ff03w and ff03ws show overstabilization of helical conformations while CHARMM22*2 better describes the disordered ensemble of AT-3306-361 in the free state.
The transition between more ordered and disordered states is favored in the description provided by CHARMM22*2 (with difference in free energy of 1.5 kcal/mol). In the ff03ws simulation, the two states were separated by a barrier of more than 8 kcal/mol. The intrinsic preference for helical conformations of ff03w/ff03ws is likely to make the sampling of disordered states more challenging even with an enhanced sampling approach. The high energy barriers observed are thus likely to be due to limitations of the sampling. Longer simulations or other enhanced sampling approaches could help to obtain free energy profiles with a larger number of order to disorder transitions for this peptide and ff0ws (Bussi and Laio, 2020).
In summary, AT-3306-361 is characterized by a disordered ensemble, which is better described by CHARMM22*2 among the force fields tested in this study. The UIM3 region of AT-3306-361 can interconvert between more disordered and partially helical states.
Both ordered and disordered proteins often sample bound-like states that could be important for their binding, which may sometimes occur via a mechanism known as conformational selection (Davey, 2019). We, therefore, asked if this was also the case for UIM3 of AT-3306-361. To this end, we compared conformations from the CHARMM22*2 WT-metaD simulation with the starting structure of AT-3306-361 for the REMD simulations, in which UIM3 is modeled as a well-folded a-helix (Figure 5). We identify partially folded states of UIM3 (around 3 Å of RMSD), characterized by helical conformations in the N-terminal region of the motif (residues 336–344) (Figure 5) and alphabeta values in the range of 24-30 and 31–34 residues (Supplementary Figure S1). We observed that the region with the highest propensity to fold to helix corresponds to the UIM3 region (residues 336–344). This accounts for approximately 20% of the structures from the entire WT-MetaD. We also observed a minor helical propensity in other regions of the peptide, especially around residues 320–334 (less than 10% of the structures from the metaD).
FIGURE 5. UIM3 of AT-3306-361 interconverts between disordered conformations and partially helical states in the free ensemble. The central panel shows the mono-dimensional free energy profile associated with alphabeta for CHARMM22*2 (pink) metadynamics simulation. The four side panels show the RMSD calculations of UIM3 structures from the CHARMM22*2 metadynamics trajectory, using as a reference the starting structure of AT-3306-361 for the REMD simulations, in which UIM3 is modeled as an α-helix. We used the Cβ atom of A343 and the backbone (Cα, C, O, N) atoms of the residues E336-T350 of UIM3 for rigid body superposition and the RMSD calculation. We calculated the RMSD of four ensembles of structures of UIM3 with alphabeta values in the ranges of: i) 9–17 (black), ii) 18–23 (purple), iii) 24–30 (red), and iv) 31–34 (yellow), respectively. The cartoon representations show the structures of UIM3 with the lowest RMSD in each of the four subsets. We identify partially helical states of UIM3 associated with alphabeta values in the range of 24-30 and 31–34.
We performed the same RMSD analysis on the replicas at 304 K from the REMD simulations (Supplementary Figure S2). In contrast with the results from WT-metaD, the REMD simulations tend to show a group of fully helical conformations of UIM3 (which are a minority of the frames in the CHARMM22*2 simulations, i.e. ∼ 3% of the frames) (Supplementary Figure S2). These analyses suggest that the REMD simulations provide a limited sampling and they are still biased by the initial helical conformation of UIM3. We thus relied on the WT-metaD results for the following analyses.
To identify the presence of bound-like states, we then compared the partially helical conformations of UIM3 of AT-3306-361 from the CHARMM22*2 WT-metaD simulation with the experimental structures of ubiquitin in complex with UIMs from other proteins (Figure 6).
FIGURE 6. UIM3 of AT-3306-361 samples states partially resembling ubiquitin-bound conformations. The plots show the RMSD calculations of UIM3 of AT-3306-361 from the CHARMM22*2 metadynamics trajectory, using as a reference the experimental structure of the UIMs of other proteins in complex with ubiquitin (A) VPS27 UIM (PDB entry 1Q0W, blue), (B) S5a UIM1 (PDB entry 1YX5, teal), (C) S5a UIM2 (PDB entry 1YX6, green), (D) RAP80 UIM1 (PDB entry 3A1Q, yellow). We calculated RMSD for the ensembles of structures of UIM3 with alphabeta values in the range of 31-34. The cartoon representations show the structures of the experimental complexes, with the ubiquitin monomers shown as gray cartoons. The white cartoon representation shows the conformations of UIM3 (residues 336–350) from the CHARMM22*2 metadynamics simulation with the lowest RMSD to each experimental structure.
UIMs are generally in folded helical conformations when bound to ubiquitin (Fisher et al., 2003; Swanson et al., 2003). We identified states of UIM3 partially resembling the bound conformations of other UIMs, characterized by RMSD around 3 Å with respect to the experimental complexes (Figure 6).
To discriminate if the low occurrence of a helical conformation in solution is a distinctive trait of UIM3 or a more common property of other UIMs, we searched the NMR database BMRB for chemical shift data on other UIMs in solution. We identified nine sets of released chemical shifts for AT-3 (including UIM1, UIM2, and UIM3), STAM1, STAM2, USP28, USP25, and RAP80 (holding two UIMs each) (Supplementary Figure S3). We also used a set of chemical shifts of VPS27 UIM1 in fusion with ubiquitin (Supplementary Figure S3). In addition, we recorded NMR experiments to collect backbone and side-chain chemical shifts for UIM1 and UIM2 of AT-3 in solution, using AT-3182-291. From the chemical shifts, we predicted the secondary structural propensity by δ2D (Figure 7A and Supplementary Figure S3). We observed UIMs with high helical content, such as UIM1 and UIM2 of AT-3, UIM1 and UIM2 of RAP80, and UIM1 of USP25 (average δ2D helix population higher than 0.3), and low helical content, as in the case of UIM3 of AT-3 and UIM2 of USP25 (average δ2D helix population lower than 0.1). USP28 has also a lower helical content compared to other UIMs suggesting a heterogeneous ensemble of conformations. We observe a lower helical content in the case of VPS27 UIM in fusion with ubiquitin, possibly suggesting that in the bound state some UIMs could retain disorder. Our NMR data of AT-3182-291 are in agreement with previously published sets of chemical shifts of AT-3, showing high helical content for UIM1 and UIM2 (average δ2D helix population above 0.3 for UIM1 and 0.4 for UIM2 in all datasets) (Supplementary Figure S3). Furthermore, our analysis on the two sets of chemical shifts of UIM3 shows low helical content for both of them (average δ2D helix population under 0.1 for each set) (Figure 7A and Supplementary Figure S3), confirming the presence of disordered conformations.
FIGURE 7. UIMs in the free state can vary from highly helical conformations to disordered counterparts. (A) Helical content for the UIMs predicted from chemical shifts by δ2D. We used nine sets of released chemical shifts of UIMs in the free state in solution from the BMRB database, including AT-3 UIM3, STAM1 UIM, STAM2 UIM, USP28 UIM, USP25 UIM1, and UIM2, and RAP80 UIM1 and UIM2. We also used a set of released chemical shifts of VPS27 UIM1 in fusion with the ubiquitin. In addition, we used the NMR chemical shifts that we recorded for AT-3182-291 UIM1 and UIM2. We highlighted in gray the residues for which there are not enough chemical shifts to run the prediction with δ2D. UIMs have a wide range of predicted helical content. (B) Consensus sequence for UIMs in the PFAM database. (C) Helical wheel representation of AT-3 UIM1, UIM2, and UIM3, and of USP25 UIM1 and UIM2.
Our results overall indicate that UIMs in the unbound state can span not only fully-formed helical conformations but also rather disordered counterparts. Moreover, a peptide SPOT arrays in which we studied the interaction of some representative UIMs with recombinant yeast ubiquitin shows that both disordered UIMs (AT-3 UIM3 and USP25 UIM1 and 2) and helical UIMs (STAM1, STAM2 and, AT-3 UIM1) interact with ubiquitin (Supplementary Figure S4). Thus, as all other UIMs tested are folded in the unbound state, these data suggest that a disordered UIM is not a barrier to bind ubiquitin.
To address if different classes of UIMs can be derived based on sequence and disorder, we compared the UIM3 sequence to other known UIMs and with the consensus sequence deposited in the Pfam database (entry PF02809) (Figure 7B). The 336–350 region of the C-terminus of AT-3 presents the typical signature of a UIM with conserved residues, such as L340, A343, S347, acidic residues in the N-terminal part of the motif (E336-D338), and the pattern of hydrophobic residues (Figure 7B). Moreover, in comparison to other UIMs, we should notice that suboptimal residues for helical formation are observed in the UIM3 sequence in comparison to other UIMs. For example, V344 and T345 are both at low helix propensity (Nick Pace and Martin Scholtz, 1998) and are localized in the region of UIM3 where the helix tend to break in some of the simulation frames (see above). Furthermore, USP25 has a valine replacing the invariant alanine of the motif and an insertion of an arginine in the N-terminal region of the motif which might alter the helical pattern.
To further identify if this is a common signature to other disordered UIMs, we carried out a helical wheel analysis of AT-3 UIM1, UIM2, UIM3, and of UIMs previously investigated (USP25 UIM1 and UIM2 Figure 7C). The analysis shows that when UIM3 assumes a helical conformation T345 is located on the face of the helix with one of the acidic residues (i.e., E337) that is conserved in UIMs. Moreover, T350 is located at the same face of the helix as A343 and S347; two residues that are strictly conserved in all UIMs since they are involved in the interaction with ubiquitin (Fisher et al., 2003). For the disordered UIM2 of USP25, a similar valine and isoleucine, two beta-branched amino acids, break the helicity. This means that disordered UIMs may carry similar sequence properties that allow for their identification. Our analysis and simulations overall suggest that the location of suboptimal residues, especially threonine and valine, coul be related to the low propensity to populate stable helical conformations in solution. A search based on regular expression with the motif x-[ED]-[ED]-[ED]-x-[AILVFWMP]-x-x(1,2)-[AVP]-[VPL]-[EDVNTCGPH]-x-S-x-x-[EDTVNCGPH]-x against the sequences associated with the Pfam entry PF02809 highlights other 1,614 hits in 626 sequences of UIMs with likelihood to be (partially) disordered in the unbound state (against 172 hits found in a randomized dataset from Uniprot). Among the disordered UIM candidates we find UBP37 from different species (residues 704–720), which feature patterns similar to USP25. The motif search suggests that disordered UIM could be a common class of SLiMs (see Supplementary Text S5).
We focused on the structural characterization of the conformational ensemble of a functional motif that has been classically defined for its helical conformation and originally associated with the binding of ubiquitin; the Ubiquitin Interacting Motif (UIM). We showed that the motifs could be more degenerate and account for both helical and more disordered members, a diversity that has functional implications. With an approach integrating simulations and experimental biophysical data, we showed that a C-terminal UIM of AT-3 is embedded in an intrinsically disordered region, bearing a predominantly disordered UIM of which a small fraction of the ensemble has helical propensity in the N-terminal region. An unbound ensemble as the one depicted by WT-metaD might suggest that a combination of conformational selection (i.e., pre-formed regions of the UIM in helical conformation) and folding upon binding could be in place for UIM3. The occurrence of one or the other mechanism might also depend on the nature of the client protein and help to confer UIM3 promiscuity toward different partners of interaction, an effect that can be further tuned by post-translational modifications. These mechanisms will require future investigations in which kinetics can be accounted for.
We also discovered that the disordered nature of UIM3, and low helical propensity in the free state, is not an isolated example, as shown by the analysis of the NMR data of USP25 UIM2, USP28 UIM, and VPS27 UIM1.
In our work, all the UIMs tested for binding with ubiquitin are either folded or disordered in the unbound state. These data suggest that a disordered UIM is not a barrier to bind ubiquitin. NMR measurements on UIM3 still suggest that the binding could be of lower affinity than what observed for helical UIMs (Bai et al., 2013), supporting a more pliable partner toward a different range of client proteins, at the cost of larger entropy loss in binding ubiquitin.
UIMs not only bind ubiquitin but can also be interfaces to recruit other proteins, such as the case of UIM and parkin. Proteins including disordered UIMs can have additional diversity in their protein-protein interactions and cellular functions. For example, it has been suggested by mass-spectrometry and co-immunoprecipitation assays that AT-3 isoforms differ in their interaction with other proteins (Weishäupl et al., 2019). Post-translational modifications are likely to add an extra level of regulation, and they could modulate the helical propensity of disordered UIMs and their preferences for binding partners, as seen for other IDRs (Mylona et al., 2016; Hendus-Altenburger et al., 2017; Csizmok and Forman-Kay, 2018; Marceau et al., 2019). For example, UIM3 is sumoylated at K356 and this enhances affinity for the binding to ATPase p97 to transfer proteins for proteasomal degradation (Almeida et al., 2015). Further experimental and computational studies of these disordered UIMs here identified and their post-translational regulation or the study of UIM from other proteins could contribute to clarify the structural and sequence features of disordered and folded UIMs, along with their connection with certain binding partners and biological functions.
Our analysis and simulations overall suggest that the location of suboptimal residues for helix formation, especially beta-branched residues as threonine, valine and isoleucine could play a role in gearing the low propensity of UIMs to populate stable helical conformations in solution and provide a gateway to multispecificity.
UIMs are not the only example of such structural duality. Some regions of proteins that were traditionally defined as helical elements, due to their conformation in the bound states, have been reclassified as disordered SLiMs, as in the case of the Bcl-2 Homology 3 motifs (Hinds et al., 2007; Aouacheria et al., 2015). The presence of this emerging higher structural variability into different classes of SLiMs is a shift in our view of functional protein regions that can account for both helical and more disordered counterparts. A better understanding of the structural diversity within each class of functional motifs could open new directions to understand biomolecular interactions and their specificity or flexibility toward multiple partners of interaction. SLiMs with disorder propensity and a more versatile interface could enhance the pool of functions of a certain protein, for example increasing the number of potential binding partners, allowing the protein to act at the cross-road among different biological processes, or allow for a fine regulation by post-translational modifications.
All the scripts, raw data, and outputs associated with this publication are available at the repository on GitHub https://github.com/ELELAB/disoUIM and on OSF https://osf.io/zfy9s/.
Conceived and designed the experiments: EP, GI, and ML. Performed the experiments: ML, EP, GI, EM, and BA. Analyzed the data: EP, GI, ML, EM, and KT. Discussion of the data: ML, EP, GI, BK, KT, KL-L, and GS. Contributed reagents/materials/analysis tools: ML, EP, BK, GS, and KT. Wrote the paper: ML, EP. All authors contributed to manuscript revision, read, and approved the submitted version.
EP group was supported by Carlsberg fondet Distinguished Fellowship (CF18-0314), Danmarks Grundforskningsfond (DNRF125) and NovoNordisk Fonden Bioscience and Basic Biomedicine NNF20OC0065262. BA was supported by COST-STSM-BM1405-34558. GI was supported by a Marie Curie IEF Fellowship. BK was supported by Novo Nordisk Foundation Challenge grant REPIN – rethinking protein interactions. GS was supported by Canadian Institutes of Health Research (PJT – 166019). The calculations described in this paper were performed using the ISCRA-CINECA grant diso-UIMs HP10C4LACQ.
The authors declare that the research was conducted in the absence of any commercial or financial relationships that could be construed as a potential conflict of interest.
The Supplementary Material for this article can be found online at: https://www.frontiersin.org/articles/10.3389/fmolb.2021.676235/full#supplementary-material
Abascal, J. L. F., and Vega, C. (2005). A General Purpose Model for the Condensed Phases of Water: TIP4P/2005. J. Chem. Phys. 123, 234505. doi:10.1063/1.2121687
Abrams, C., and Bussi, G. (2013). Enhanced Sampling in Molecular Dynamics Using Metadynamics, Replica-Exchange, and Temperature-Acceleration. Entropy. 16, 163–199. doi:10.3390/e16010163
Aguirre, J. D., Dunkerley, K. M., Lam, R., Rusal, M., and Shaw, G. S. (2018). Impact of Altered Phosphorylation on Loss of Function of Juvenile Parkinsonism-Associated Genetic Variants of the E3 Ligase Parkin. J. Biol. Chem. 293, 6337–6348. doi:10.1074/jbc.RA117.000605
Almeida, B., Abreu, I. A., Matos, C. A., Fraga, J. S., Fernandes, S., Macedo, M. G., et al. (2015). SUMOylation of the Brain-Predominant Ataxin-3 Isoform Modulates its Interaction with P97. Biochim. Biophys. Acta. 1852, 1950–1959. doi:10.1016/j.bbadis.2015.06.010
Anamika, S., Markin, C. J., Rout, M. K., and Spyracopoulos, L. (2014). Molecular Basis for Impaired DNA Damage Response Function Associated with the RAP80 ΔE81 Defect. J. Biol. Chem. 289, 12852–12862. doi:10.1074/jbc.M113.538280
Aouacheria, A., Combet, C., Tompa, P., and Hardwick, J. M. (2015). Redefining the BH3 Death Domain as a 'Short Linear Motif'. Trends Biochem. Sci. 40, 736–748. doi:10.1016/j.tibs.2015.09.007
Bai, J. J., Safadi, S. S., Mercier, P., Barber, K. R., and Shaw, G. S. (2013). Ataxin-3 Is a Multivalent Ligand for the Parkin Ubl Domain. Biochemistry. 52, 7369–7376. doi:10.1021/bi400780v
Barducci, A., Bussi, G., and Parrinello, M. (2008). Well-tempered Metadynamics: A Smoothly Converging and Tunable Free-Energy Method. Phys. Rev. Lett. 100, 1–4. doi:10.1103/PhysRevLett.100.020603
Berlow, R. B., Dyson, H. J., and Wright, P. E. (2018). Expanding the Paradigm: Intrinsically Disordered Proteins and Allosteric Regulation. J. Mol. Biol. 430, 2309–2320. doi:10.1016/j.jmb.2018.04.003
Best, R. B. (2017). Computational and Theoretical Advances in Studies of Intrinsically Disordered Proteins. Curr. Opin. Struct. Biol. 42, 147–154. doi:10.1016/j.sbi.2017.01.006
Best, R. B., and Mittal, J. (2010). Protein Simulations with an Optimized Water Model: Cooperative Helix Formation and Temperature-Induced Unfolded State Collapse. J. Phys. Chem. B. 114, 14916–14923. doi:10.1021/jp108618d
Best, R. B., Zheng, W., and Mittal, J. (2014). Balanced Protein-Water Interactions Improve Properties of Disordered Proteins and Non-specific Protein Association. J. Chem. Theor. Comput. 10, 5113–5124. doi:10.1021/ct500569b
Bettencourt, C., Santos, C., Montiel, R., Costa, M. d. C., Cruz-Morales, P., Santos, L. R., et al. (2010). Increased Transcript Diversity: Novel Splicing Variants of Machado-Joseph Disease Gene (ATXN3). Neurogenetics 11, 193–202. doi:10.1007/s10048-009-0216-y
Bonomi, M., Branduardi, D., Bussi, G., Camilloni, C., Provasi, D., Raiteri, P., et al. (2009). PLUMED: A Portable Plugin for Free-Energy Calculations with Molecular Dynamics. Computer Phys. Commun. 180, 1961–1972. doi:10.1016/j.cpc.2009.05.011
Bonomi, M., Heller, G. T., Camilloni, C., and Vendruscolo, M. (2017). Principles of Protein Structural Ensemble Determination. Curr. Opin. Struct. Biol. 42, 106–116. doi:10.1016/j.sbi.2016.12.004
Brunger, A. T. (2007). Version 1.2 of the Crystallography and Nmr System. Nat. Protoc. 2, 2728–2733. doi:10.1038/nprot.2007.406
Buchberger, A. (2002). From UBA to UBX: New Words in the Ubiquitin Vocabulary. Trends Cel Biol 12, 216–221. doi:10.1016/S0962-8924(02)02269-9
Bugge, K., Brakti, I., Fernandes, C. B., Dreier, J. E., Lundsgaard, J. E., Olsen, J. G., et al. (2020). Interactions by Disorder - A Matter of Context. Front. Mol. Biosci. 7, 110. doi:10.3389/fmolb.2020.00110
Burnett, B., Li, F., and Pittman, R. N. (2003). The Polyglutamine Neurodegenerative Protein Ataxin-3 Binds Polyubiquitylated Proteins and Has Ubiquitin Protease Activity. Hum. Mol. Genet. 12, 3195–3205. doi:10.1093/hmg/ddg344
Bussi, G., Donadio, D., and Parrinello, M. (2007). Canonical Sampling through Velocity Rescaling. J. Chem. Phys. 126, 014101. doi:10.1063/1.2408420
Bussi, G., and Laio, A. (2020). Using Metadynamics to Explore Complex Free-Energy Landscapes. Nat. Rev. Phys. 2, 200–212. doi:10.1038/s42254-020-0153-0
Camilloni, C., De Simone, A., Vranken, W. F., and Vendruscolo, M. (2012). Determination of Secondary Structure Populations in Disordered States of Proteins Using Nuclear Magnetic Resonance Chemical Shifts. Biochemistry. 51, 2224–2231. doi:10.1021/bi3001825
Carvalho, A. L., Silva, A., and Macedo-Ribeiro, S. (2018). Polyglutamine-independent Features in Ataxin-3 Aggregation and Pathogenesis of Machado-Joseph Disease. Adv. Exp. Med. Biol. 1049, 275–288. doi:10.1007/978-3-319-71779-1_14
Chen, J., and Kriwacki, R. W. (2018). Intrinsically Disordered Proteins: Structure, Function and Therapeutics. J. Mol. Biol. 430, 2275–2277. doi:10.1016/j.jmb.2018.06.012
Cilia, E., Pancsa, R., Tompa, P., Lenaerts, T., and Vranken, W. F. (2013). From Protein Sequence to Dynamics and Disorder with DynaMine. Nat. Commun. 4, 2741. doi:10.1038/ncomms3741
Csizmok, V., and Forman-Kay, J. D. (2018). Complex Regulatory Mechanisms Mediated by the Interplay of Multiple post-translational Modifications. Curr. Opin. Struct. Biol. 48, 58–67. doi:10.1016/j.sbi.2017.10.013
Darden, T., York, D., and Pedersen, L. (1993). Particle Mesh Ewald: AnN⋅Log(N) Method for Ewald Sums in Large Systems. J. Chem. Phys. 98, 10089–10092. doi:10.1063/1.464397
Davey, N. E. (2019). The Functional Importance of Structure in Unstructured Protein Regions. Curr. Opin. Struct. Biol. 56, 155–163. doi:10.1016/j.sbi.2019.03.009
Davey, N. E., Van Roey, K., Weatheritt, R. J., Toedt, G., Uyar, B., Altenberg, B., et al. (2012). Attributes of Short Linear Motifs. Mol. Biosyst. 8, 268–281. doi:10.1039/C1MB05231D
Delaglio, F., Grzesiek, S., Vuister, G., Zhu, G., Pfeifer, J., and Bax, A. (1995). NMRPipe: A Multidimensional Spectral Processing System Based on UNIX Pipes. J. Biomol. NMR. 6, 277–293. doi:10.1007/BF00197809
Do, T. N., Choy, W.-Y., and Karttunen, M. (2014). Accelerating the Conformational Sampling of Intrinsically Disordered Proteins. J. Chem. Theor. Comput. 10, 5081–5094. doi:10.1021/ct5004803
Donaldson, K. M., Li, W., Ching, K. A., Batalov, S., Tsai, C.-C., and Joazeiro, C. A. P. (2003). Ubiquitin-mediated Sequestration of normal Cellular Proteins into Polyglutamine Aggregates. Proc. Natl. Acad. Sci. 100, 8892–8897. doi:10.1073/pnas.1530212100
Eswar, N., Webb, B., Marti‐Renom, M. A., Madhusudhan, M. S., Eramian, D., Shen, M. y., et al. (2007). Comparative Protein Structure Modeling Using MODELLER. Curr. Protoc. Protein Sci. 50. doi:10.1002/0471140864.ps0209s50
Fisher, R. D., Wang, B., Alam, S. L., Higginson, D. S., Robinson, H., Sundquist, W. I., et al. (2003). Structure and Ubiquitin Binding of the Ubiquitin-Interacting Motif. J. Biol. Chem. 278, 28976–28984. doi:10.1074/jbc.M302596200
Flock, T., Weatheritt, R. J., Latysheva, N. S., and Babu, M. M. (2014). Controlling Entropy to Tune the Functions of Intrinsically Disordered Regions. Curr. Opin. Struct. Biol. 26, 62–72. doi:10.1016/j.sbi.2014.05.007
Frank, R., and Dubel, S. (2006). Analysis of Protein Interactions with Immobilized Peptide Arrays Synthesized on Membrane Supports. Cold Spring Harbor Protoc. 2006, prot4566. doi:10.1101/pdb.prot4566
Fung, H. Y. J., Birol, M., and Rhoades, E. (2018). IDPs in Macromolecular Complexes: the Roles of Multivalent Interactions in Diverse Assemblies. Curr. Opin. Struct. Biol. 49, 36–43. doi:10.1016/j.sbi.2017.12.007
Goto, J., Watanabe, M., Ichikawa, Y., Yee, S.-B., Ihara, N., Endo, K., et al. (1997). Machado-Joseph Disease Gene Products Carrying Different Carboxyl Termini. Neurosci. Res. 28, 373–377. doi:10.1016/S0168-0102(97)00056-4
Granata, D., Baftizadeh, F., Habchi, J., Galvagnion, C., De Simone, A., Camilloni, C., et al. (2015). The Inverted Free Energy Landscape of an Intrinsically Disordered Peptide by Simulations and Experiments. Sci. Rep. 5, 1–15. doi:10.1038/srep15449
Guarnera, E., and Berezovsky, I. N. (2019). On the Perturbation Nature of Allostery: Sites, Mutations, and Signal Modulation. Curr. Opin. Struct. Biol. 56, 18–27. doi:10.1016/j.sbi.2018.10.008
Hanson, J., Paliwal, K. K., Litfin, T., and Zhou, Y. (2019). SPOT-Disorder2: Improved Protein Intrinsic Disorder Prediction by Ensembled Deep Learning. Genomics, Proteomics & Bioinformatics. 17, 645–656. doi:10.1016/j.gpb.2019.01.004
Harris, G. M., Dodelzon, K., Gong, L., Gonzalez-Alegre, P., and Paulson, H. L. (2010). Splice Isoforms of the Polyglutamine Disease Protein Ataxin-3 Exhibit Similar Enzymatic yet Different Aggregation Properties. PLoS One. 5, e13695. doi:10.1371/journal.pone.0013695
Hendus-Altenburger, R., Lambrughi, M., Terkelsen, T., Pedersen, S. F., Papaleo, E., Lindorff-Larsen, K., et al. (2017). A Phosphorylation-Motif for Tuneable helix Stabilisation in Intrinsically Disordered Proteins - Lessons from the Sodium Proton Exchanger 1 (NHE1). Cell Signal. 37, 40–51. doi:10.1016/j.cellsig.2017.05.015
Hinds, M. G., Smits, C., Fredericks-Short, R., Risk, J. M., Bailey, M., Huang, D. C. S., et al. (2007). Bim, Bad and Bmf: Intrinsically Unstructured BH3-Only Proteins that Undergo a Localized Conformational Change upon Binding to Prosurvival Bcl-2 Targets. Cell Death Differ. 14, 128–136. doi:10.1038/sj.cdd.4401934
Hofmann, K., and Falquet, L. (2001). A Ubiquitin-Interacting Motif Conserved in Components of the Proteasomal and Lysosomal Protein Degradation Systems. Trends Biochem. Sci. 26, 347–350. doi:10.1016/S0968-0004(01)01835-7
Huang, J., and Mackerell, A. D. (2014). Induction of Peptide Bond Dipoles Drives Cooperative helix Formation in the (AAQAA)3 Peptide. Biophysical J. 107, 991–997. doi:10.1016/j.bpj.2014.06.038
Huang, J., and MacKerell, A. D. (2018). Force Field Development and Simulations of Intrinsically Disordered Proteins. Curr. Opin. Struct. Biol. 48, 40–48. doi:10.1016/j.sbi.2017.10.008
Ichikawa, Y., Goto, J., Hattori, M., Toyoda, A., Ishii, K., Jeong, S.-Y., et al. (2001). The Genomic Structure and Expression of MJD, the Machado-Joseph Disease Gene. J. Hum. Genet. 46, 413–422. doi:10.1007/s100380170060
Invernizzi, G., Lambrughi, M., Regonesi, M. E., Tortora, P., and Papaleo, E. (2013). The Conformational Ensemble of the Disordered and Aggregation-Protective 182-291 Region of Ataxin-3. Biochim. Biophys. Acta. 1830, 5236–5247. doi:10.1016/j.bbagen.2013.07.007
Invernizzi, G., Papaleo, E., Sabate, R., and Ventura, S. (2012). Protein Aggregation: Mechanisms and Functional Consequences. Int. J. Biochem. Cel Biol. 44, 1541–1554. doi:10.1016/j.biocel.2012.05.023
Irbäck, A., and Mohanty, S. (2006). PROFASI: A Monte Carlo Simulation Package for Protein Folding and Aggregation. J. Comput. Chem. 27, 1548–1555. doi:10.1002/jcc.20452
Johnson, S. L., Ranxhi, B., Libohova, K., Tsou, W.-L., and Todi, S. V. (2020). Ubiquitin-interacting Motifs of Ataxin-3 Regulate its Polyglutamine Toxicity through Hsc70-4-dependent Aggregation. Elife. 9, 1–22. doi:10.7554/ELIFE.60742
Jones, D. T. (1999). Protein Secondary Structure Prediction Based on Position-specific Scoring Matrices 1 1Edited by G. Von Heijne. J. Mol. Biol. 292, 195–202. doi:10.1006/jmbi.1999.3091
Jorgensen, W. L., Chandrasekhar, J., Madura, J. D., Impey, R. W., and Klein, M. L. (1983). Comparison of Simple Potential Functions for Simulating Liquid Water. J. Chem. Phys. 79, 926–935. doi:10.1063/1.445869
Kabsch, W., and Sander, C. (1983). Dictionary of Protein Secondary Structure: Pattern Recognition of Hydrogen-Bonded and Geometrical Features. Biopolymers. 22, 2577–2637. doi:10.1002/bip.360221211
Knott, M., and Best, R. B. (2012). A Preformed Binding Interface in the Unbound Ensemble of an Intrinsically Disordered Protein: Evidence from Molecular Simulations. Plos Comput. Biol. 8, e1002605. doi:10.1371/journal.pcbi.1002605
Kumar, M., Gouw, M., Michael, S., Sámano-Sánchez, H., Pancsa, R., Glavina, J., et al. (2020). ELM-the Eukaryotic Linear Motif Resource in 2020. Nucleic Acids Res. 48, D296–D306. doi:10.1093/nar/gkz1030
Lange, A., Ismail, M.-B., Rivière, G., Hologne, M., Lacabanne, D., Guillière, F., et al. (2012). Competitive Binding of UBPY and Ubiquitin to the STAM2 SH3 Domain Revealed by NMR. FEBS Lett. 586, 3379–3384. doi:10.1016/j.febslet.2012.07.047
Li, D.-W., and Brüschweiler, R. (2012). PPM: A Side-Chain and Backbone Chemical Shift Predictor for the Assessment of Protein Conformational Ensembles. J. Biomol. NMR. 54, 257–265. doi:10.1007/s10858-012-9668-8
Li, J., White, J. T., Saavedra, H., Wrabl, J. O., Motlagh, H. N., Liu, K., et al. (2017). Genetically Tunable Frustration Controls Allostery in an Intrinsically Disordered Transcription Factor. Elife. 6, e30688. doi:10.7554/eLife.30688
Lim, J., Hong, Y.-H., Lee, B.-J., and Ahn, H.-C. (2011). Backbone 1H, 13C, and 15N Assignments for the Tandem Ubiquitin Binding Domains of Signal Transducing Adapter Molecule 1. Biomol. NMR Assign. 5, 51–54. doi:10.1007/s12104-010-9265-2
Lindorff-Larsen, K., Trbovic, N., Maragakis, P., Piana, S., and Shaw, D. E. (2012). Structure and Dynamics of an Unfolded Protein Examined by Molecular Dynamics Simulation. J. Am. Chem. Soc. 134, 3787–3791. doi:10.1021/ja209931w
Ma, B., Tsai, C.-J., Haliloğlu, T., and Nussinov, R. (2011). Dynamic Allostery: Linkers Are Not Merely Flexible. Structure. 19, 907–917. doi:10.1016/j.str.2011.06.002
MacKerell, A. D., Bashford, D., Bellott, M., Dunbrack, R. L., Evanseck, J. D., Field, M. J., et al. (1998). All-Atom Empirical Potential for Molecular Modeling and Dynamics Studies of Proteins. J. Phys. Chem. B 102, 3586–3616. doi:10.1021/jp973084f
Marceau, A. H., Brison, C. M., Nerli, S., Arsenault, H. E., McShan, A. C., Chen, E., et al. (2019). An Order-To-Disorder Structural Switch Activates the Foxm1 Transcription Factor. Elife. 8, e46131. doi:10.7554/eLife.46131
Margreitter, C., and Oostenbrink, C. (2017). MDplot: Visualise Molecular Dynamics. R. J. 9, 164–186. doi:10.32614/rj-2017-007
Marshall, R. S., Hua, Z., Mali, S., McLoughlin, F., and Vierstra, R. D. (2019). ATG8-Binding UIM Proteins Define a New Class of Autophagy Adaptors and Receptors. Cell. 177, 766–781. doi:10.1016/j.cell.2019.02.009
Masino, L., Musi, V., Menon, R. P., Fusi, P., Kelly, G., Frenkiel, T. A., et al. (2003). Domain Architecture of the Polyglutamine Protein Ataxin-3: A Globular Domain Followed by a Flexible Tail. FEBS Lett. 549, 21–25. doi:10.1016/S0014-5793(03)00748-8
Metallo, S. J. (2010). Intrinsically Disordered Proteins Are Potential Drug Targets. Curr. Opin. Chem. Biol. 14, 481–488. doi:10.1016/j.cbpa.2010.06.169
Michaud-Agrawal, N., Denning, E. J., Woolf, T. B., and Beckstein, O. (2011). MDAnalysis: A Toolkit for the Analysis of Molecular Dynamics Simulations. J. Comput. Chem. 32, 2319–2327. doi:10.1002/jcc.21787
Milles, S., Salvi, N., Blackledge, M., and Jensen, M. R. (2018). Characterization of Intrinsically Disordered Proteins and Their Dynamic Complexes: From In Vitro to Cell-like Environments. Prog. Nucl. Magn. Reson. Spectrosc. 109, 79–100. doi:10.1016/j.pnmrs.2018.07.001
Mizianty, M. J., Peng, Z., and Kurgan, L. (2013). MFDp2. Intrinsically Disordered ProteinsProteins. 1, e24428. doi:10.4161/idp.24428
Mól, A. R., Castro, M. S., and Fontes, W. (2018). NetWheels: A Web Application to Create High Quality Peptide Helical Wheel and Net Projections. bioRxiv. doi:10.1101/416347
Mylona, A., Theillet, F.-X., Foster, C., Cheng, T. M., Miralles, F., Bates, P. A., et al. (2016). Opposing Effects of Elk-1 Multisite Phosphorylation Shape its Response to ERK Activation. Science. 354, 233–237. doi:10.1126/science.aad1872
Nick Pace, C., and Martin Scholtz, J. (1998). A Helix Propensity Scale Based on Experimental Studies of Peptides and Proteins. Biophys. J. 75 (1), 422–427. doi:10.1016/s0006-3495(98)77529-0
Nielsen, J. T., and Mulder, F. A. A. (2019). Quality and Bias of Protein Disorder Predictors. Sci. Rep. 9, 5137. doi:10.1038/s41598-019-41644-w
Palazzesi, F., Prakash, M. K., Bonomi, M., and Barducci, A. (2015). Accuracy of Current All-Atom Force-fields in Modeling Protein Disordered States. J. Chem. Theor. Comput. 11, 2–7. doi:10.1021/ct500718s
Papaleo, E., Camilloni, C., Teilum, K., Vendruscolo, M., and Lindorff-Larsen, K. (2018). Molecular Dynamics Ensemble Refinement of the Heterogeneous Native State of NCBD Using Chemical Shifts and NOEs. PeerJ. 6, e5125. doi:10.7717/peerj.5125
Perdigão, N., Heinrich, J., Stolte, C., Sabir, K. S., Buckley, M. J., Tabor, B., et al. (2015). Unexpected Features of the Dark Proteome. Proc. Natl. Acad. Sci. USA. 112, 15898–15903. doi:10.1073/pnas.1508380112
Piana, S., Lindorff-Larsen, K., and Shaw, D. E. (2011). How Robust Are Protein Folding Simulations with Respect to Force Field Parameterization?. Biophysical J. 100, L47–L49. doi:10.1016/j.bpj.2011.03.051
PLUMED Consortium (2019). Promoting Transparency and Reproducibility in Enhanced Molecular Simulations. Nat. Methods. 16, 670–673. doi:10.1057/palcomms.2015.1310.1038/s41592-019-0506-8
Sato, Y., Yoshikawa, A., Mimura, H., Yamashita, M., Yamagata, A., and Fukai, S. (2009). Structural Basis for Specific Recognition of Lys 63-linked Polyubiquitin Chains by Tandem UIMs of RAP80. EMBO J. 28, 2461–2468. doi:10.1038/emboj.2009.160
Scott, D., Oldham, N. J., Strachan, J., Searle, M. S., and Layfield, R. (2015). Ubiquitin-binding Domains: Mechanisms of Ubiquitin Recognition and Use as Tools to Investigate Ubiquitin-Modified Proteomes. Proteomics. 15, 844–861. doi:10.1002/pmic.201400341
Sgourakis, N. G., Patel, M. M., Garcia, A. E., Makhatadze, G. I., and McCallum, S. A. (2010). Conformational Dynamics and Structural Plasticity Play Critical Roles in the Ubiquitin Recognition of a UIM Domain. J. Mol. Biol. 396, 1128–1144. doi:10.1016/j.jmb.2009.12.052
Shi, L., Wen, Y., and Zhang, N. (2014). 1H, 13C and 15N Backbone and Side-Chain Resonance Assignments of the N-Terminal Ubiquitin-Binding Domains of USP25. Biomol. NMR Assign. 8, 255–258. doi:10.1007/s12104-013-9495-1
Sicorello, A., Kelly, G., Oregioni, A., Nováček, J., Sklenář, V., and Pastore, A. (2018). The Structural Properties in Solution of the Intrinsically Mixed Folded Protein Ataxin-3. Biophysical J. 115, 59–71. doi:10.1016/j.bpj.2018.05.029
Sicorello, A., Różycki, B., Konarev, P. V., Svergun, D. I., and Pastore, A. (2021). Capturing the Conformational Ensemble of the Mixed Folded Polyglutamine Protein Ataxin-3. Structure. 29, 70–81. doi:10.1016/j.str.2020.09.010
Skinner, S. P., Fogh, R. H., Boucher, W., Ragan, T. J., Mureddu, L. G., and Vuister, G. W. (2016). CcpNmr AnalysisAssign: a Flexible Platform for Integrated NMR Analysis. J. Biomol. NMR. 66, 111–124. doi:10.1007/s10858-016-0060-y
Sora, V., Kumar, M., Maiani, E., Lambrughi, M., Tiberti, M., and Papaleo, E. (2020). Structure and Dynamics in the ATG8 Family from Experimental to Computational Techniques. Front. Cel Dev. Biol. 8, 1–28. doi:10.3389/fcell.2020.00420
Sora, V., Sanchez, D., and Papaleo, E. (2021). Bcl-xL Dynamics under the Lens of Protein Structure Networks. J. Phys. Chem. B. 125, 4308–4320. doi:10.1021/acs.jpcb.0c11562
Spiwok, V., Sucur, Z., and Hosek, P. (2015). Enhanced Sampling Techniques in Biomolecular Simulations. Biotechnol. Adv. 33, 1130–1140. doi:10.1016/j.biotechadv.2014.11.011
Sugita, Y., Kamiya, M., Oshima, H., and Re, S. (2019). Replica-Exchange Methods for Biomolecular Simulations. Methods Mol. Biol. 2022, 155–177. doi:10.1007/978-1-4939-9608-7_7
Swanson, K. A., Kang, R. S., Stamenova, S. D., Hicke, L., and Radhakrishnan, I. (2003). Solution Structure of Vps27 UIM-Ubiquitin Complex Important for Endosomal Sorting and Receptor Downregulation. EMBO J. 22, 4597–4606. doi:10.1093/emboj/cdg471
Tee, W.-V., Guarnera, E., and Berezovsky, I. N. (2020). Disorder Driven Allosteric Control of Protein Activity. Curr. Res. Struct. Biol. 2, 191–203. doi:10.1016/j.crstbi.2020.09.001
Tyler, R. C., Sreenath, H. K., Singh, S., Aceti, D. J., Bingman, C. A., Markley, J. L., et al. (2005). Auto-induction Medium for the Production of [U-15N]- and [U-13C, U-15n]-Labeled Proteins for NMR Screening and Structure Determination. Protein Expr. Purif. 40, 268–278. doi:10.1016/j.pep.2004.12.024
Van Der Lee, R., Buljan, M., Lang, B., Weatheritt, R. J., Daughdrill, G. W., Dunker, A. K., et al. (2014). Classification of Intrinsically Disordered Regions and Proteins. Chem. Rev. 114, 6589–6631. doi:10.1021/cr400525m
Van Roey, K., Gibson, T. J., and Davey, N. E. (2012). Motif Switches: Decision-Making in Cell Regulation. Curr. Opin. Struct. Biol. 22, 378–385. doi:10.1016/j.sbi.2012.03.004
Van Roey, K., Uyar, B., Weatheritt, R. J., Dinkel, H., Seiler, M., Budd, A., et al. (2014). Short Linear Motifs: Ubiquitous and Functionally Diverse Protein Interaction Modules Directing Cell Regulation. Chem. Rev. 114, 6733–6778. doi:10.1021/cr400585q
Wang, Q., Young, P., and Walters, K. J. (2005). Structure of S5a Bound to Monoubiquitin Provides a Model for Polyubiquitin Recognition. J. Mol. Biol. 348, 727–739. doi:10.1016/j.jmb.2005.03.007
Weishäupl, D., Schneider, J., Peixoto Pinheiro, B., Ruess, C., Dold, S. M., von Zweydorf, F., et al. (2019). Physiological and Pathophysiological Characteristics of Ataxin-3 Isoforms. J. Biol. Chem. 294, 644–661. doi:10.1074/jbc.RA118.005801
Wen, Y., Cui, R., Zhang, H., and Zhang, N. (2014). 1H, 13C and 15N Backbone and Side-Chain Resonance Assignments of the N-Terminal Ubiquitin-Binding Domains of the Human Deubiquitinase Usp28. Biomol. NMR Assign. 8, 251–254. doi:10.1007/s12104-013-9494-2
Wright, P. E., and Dyson, H. J. (2014). Intrinsically Disordered Proteins in Cellular Signalling and Regulation. Nat. Rev. Mol. Cel Biol. 16, 18–29. doi:10.1038/nrm3920
Wright, P. E., and Dyson, H. J. (1999). Intrinsically Unstructured Proteins: Re-assessing the Protein Structure-Function Paradigm. J. Mol. Biol. 293, 321–331. doi:10.1006/jmbi.1999.3110
Keywords: molecular dynamics, peptide arrays, ubiquitin, short linear motifs, moonlight functions, intrinsic disorder
Citation: Lambrughi M, Maiani E, Aykac Fas B, Shaw GS, Kragelund BB, Lindorff-Larsen K, Teilum K, Invernizzi G and Papaleo E (2021) Ubiquitin Interacting Motifs: Duality Between Structured and Disordered Motifs. Front. Mol. Biosci. 8:676235. doi: 10.3389/fmolb.2021.676235
Received: 04 March 2021; Accepted: 14 May 2021;
Published: 28 June 2021.
Edited by:
Gregory Bowman, Washington University School of Medicine in St. Louis, United StatesReviewed by:
Sophie Sacquin-Mora, UPR9080 Laboratoire de Biochimie Théorique (LBT), FranceCopyright © 2021 Lambrughi, Maiani, Aykac Fas, Shaw, Kragelund, Lindorff-Larsen, Teilum, Invernizzi and Papaleo. This is an open-access article distributed under the terms of the Creative Commons Attribution License (CC BY). The use, distribution or reproduction in other forums is permitted, provided the original author(s) and the copyright owner(s) are credited and that the original publication in this journal is cited, in accordance with accepted academic practice. No use, distribution or reproduction is permitted which does not comply with these terms.
*Correspondence: Elena Papaleo, ZWxlbmFwQGNhbmNlci5kaw==, ZWxwYXBAZHR1LmRr
†Present address: Department of Proteins and Peptides Biophysics, Novo Nordisk A/S, Måløv, Denmark
Disclaimer: All claims expressed in this article are solely those of the authors and do not necessarily represent those of their affiliated organizations, or those of the publisher, the editors and the reviewers. Any product that may be evaluated in this article or claim that may be made by its manufacturer is not guaranteed or endorsed by the publisher.
Research integrity at Frontiers
Learn more about the work of our research integrity team to safeguard the quality of each article we publish.