- 1Laboratory of Human Molecular Genetics, Postgraduate Program in Genetics, Department of Genetics, Federal University of Paraná, Curitiba, Brazil
- 2Laboratory of Polymorphism and Linkage, Postgraduate Program in Genetics, Department of Genetics, Federal University of Paraná, Curitiba, Brazil
Several genome-wide association studies (GWAS) have been carried out with late-onset Alzheimer’s disease (LOAD), mainly in European and Asian populations. Different polymorphisms were associated, but several of them without a functional explanation. GWAS are fundamental for identifying loci associated with diseases, although they often do not point to causal polymorphisms. In this sense, functional investigations are a fundamental tool for discovering causality, although the failure of this validation does not necessarily indicate a non-causality. Furthermore, the allele frequency of associated genetic variants may vary widely between populations, requiring replication of these associations in other ethnicities. In this sense, our study sought to replicate in 150 AD patients and 114 elderly controls from the South Brazilian population 18 single-nucleotide polymorphisms (SNPs) associated with AD in European GWAS, with further functional investigation using bioinformatic tools for the associated SNPs. Of the 18 SNPs investigated, only four were associated in our population: rs769449 (APOE), rs10838725 (CELF1), rs6733839, and rs744373 (BIN1–CYP27C1). We identified 54 variants in linkage disequilibrium (LD) with the associated SNPs, most of which act as expression or splicing quantitative trait loci (eQTLs/sQTLs) in genes previously associated with AD or with a possible functional role in the disease, such as CELF1, MADD, MYBPC3, NR1H3, NUP160, SPI1, and TOMM40. Interestingly, eight of these variants are located within long non-coding RNA (lncRNA) genes that have not been previously investigated regarding AD. Some of these polymorphisms can result in changes in these lncRNAs’ secondary structures, leading to either loss or gain of microRNA (miRNA)-binding sites, deregulating downstream pathways. Our pioneering work not only replicated LOAD association with polymorphisms not yet associated in the Brazilian population but also identified six possible lncRNAs that may interfere in LOAD development. The results lead us to emphasize the importance of functional exploration of associations found in large-scale association studies in different populations to base personalized and inclusive medicine in the future.
Introduction
Late-onset Alzheimer’s disease (LOAD) is a neurodegenerative disease responsible for most dementia cases worldwide in the elderly population (Lane et al., 2018). The neuropathological features are the accumulation of β-amyloid (Aβ) plaques and neurofibrillary tangles (NFTs), leading to neuronal death and cerebral atrophy (Braak and Braak, 1991). LOAD is a complex disease, with the influence of several genetic factors, in addition to environmental factors. Although there are numerous studies on LOAD with the most diverse approaches, the mechanisms involved in this disease remain poorly understood.
Genome-wide association studies (GWAS) started in 2006 and have grown exponentially in number (Buniello et al., 2019), allowing for the identification of countless genetic variants associated with diseases and phenotypic traits. However, most of them are not directly implicated in the phenotype, being markers in high linkage disequilibrium (LD) with the unknown regulatory or structural causal variant (Maurano et al., 2012; Zhu et al., 2016). Besides, most GWAS were performed in European and Asian populations, hindering the extrapolation of results for populations of other or mixed ancestries, given the possible difference of gene or haplotype frequencies (Nédélec et al., 2016). Currently, over 90 GWAS have been performed with LOAD (GWAS Catalog). They led to the identification of different polymorphisms, mostly in intronic or intergenic regions, possibly modulating the susceptibility to disease in the populations where they were analyzed, but most were not investigated nor replicated yet in Latin American populations (Kretzschmar et al., 2020).
In this context, our study sought to replicate in the South Brazilian population some of the single-nucleotide polymorphism (SNP) alleles found associated with LOAD in GWAS performed in European-derived populations, as well as to evaluate possible functional explanations for these associations.
Materials and Methods
To understand this work’s multistep nature, we represent it as a two-stage workflow (Figure 1), showing first, the replication in patients from South Brazil of European-associated LOAD variants, followed by the in silico investigation of replicated associations.
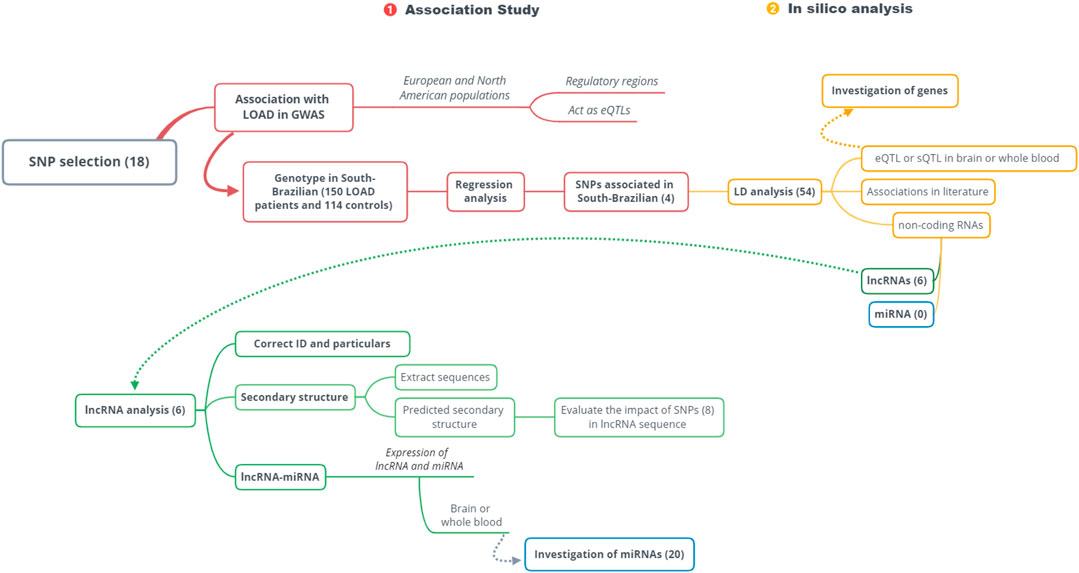
FIGURE 1. Workflow. This study is divided into two stages: 1, association study; 2, in silico analysis. Only the associated variants in the studied population (South Brazilian) followed in silico analysis. SNP: single-nucleotide polymorphism; LOAD: late-onset Alzheimer’s disease; GWAS: genome-wide association studies; eQTL: expression quantitative trait locus; sQTL: splicing quantitative trait locus; LD: linkage disequilibrium; lncRNAs: long non-coding RNAs; miRNAs: microRNAs.
Ethical Approval
This study was performed in accordance with the ethical standards of the Research Ethics Committee of the Health Sciences Sector (Federal University of Paraná) (CAAE: 55965316.1.0000.0102), according to Resolution 466/2012 of the National Health Council and the 1964 Helsinki Declaration and its later amendments or comparable ethical standards. Informed consent was obtained from all participants included in the study.
Association Study
Research Participants
The 150 LOAD patients were recruited from the Clinical Hospital of the Federal University of Paraná (HC-UFPR) (n = 97) and the Institute of Neurology of Paraná (INC) (n = 53). To be included, patients should be diagnosed with LOAD based on the clinical history and cognitive tests (Frota et al., 2011). Forms of dementia other than LOAD, inconclusive diagnosis, and less than 60 years of age were exclusion criteria. The elderly controls (114 individuals) were confirmed to be healthy and neurologically normal, according to their medical history and scores in the Mini–Mental State Examination (MMSE) scale. Individuals with infectious diseases or of 60–65 years of age and family history of AD were excluded from both groups. Since APOE (apolipoprotein E) rs7412 and rs429358 polymorphisms are strongly associated with LOAD (Lane et al., 2018), we previously genotyped the samples by TaqMan real-time PCR (Life Technologies 4351379) to correct for the presence of the LOAD-associated alleles in logistic regression analyses. Further sample descriptions can be found in Table 1.
Polymorphism Selection and Genotyping
Total DNA was extracted from peripheral blood using the salting-out standard protocol (Lahiri and Numberger, 1991). We genotyped eighteen single-nucleotide polymorphisms (SNPs), listed in Table 2. SNP selection was based on their association with LOAD in GWAS conducted with European and North American populations with European ancestry, accessing the GWAS Catalog (Buniello et al., 2019). These populations are more similar to the South Brazilian population, whose ancestry has a strong European component, mainly from the Iberian and Tuscany regions (Lima-Costa et al., 2015). Selected SNPs should also be located in regulatory regions or act as expression quantitative trait loci (eQTLs). SNP rs3857059 was the only exception, since it was originally associated with Parkinson’s disease in a GWAS. We selected it to evaluate if it could be associated with LOAD in our population.
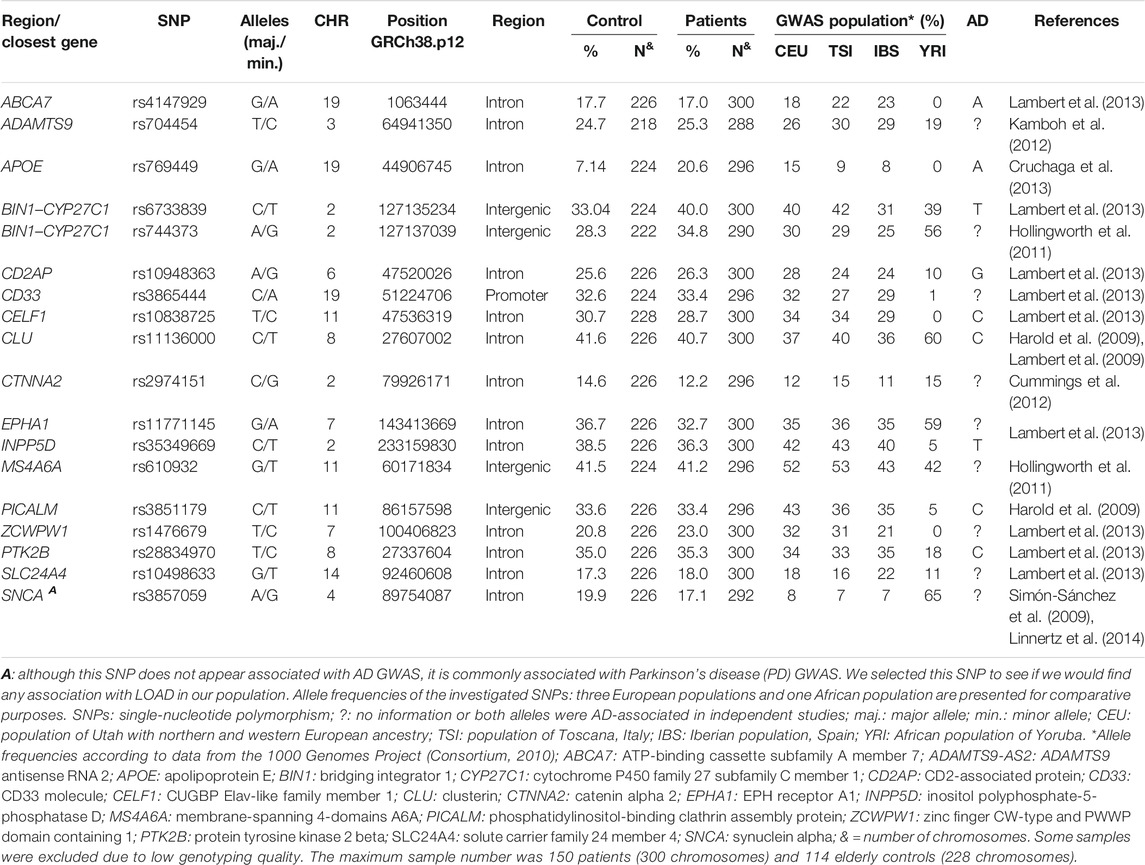
TABLE 2. Single-nucleotide polymorphisms (SNPs) selected for this study and their minor allele frequencies.
Genotyping was performed by mass spectrometry using the iPLEX MassARRAY Platform (Sequenom, San Diego, CA) at Auckland University (NZ).
Association Analysis
Allelic and genotypic frequencies were obtained by direct counting, and their distribution was evaluated according to the Hardy–Weinberg equilibrium hypothesis (applied by PLINK v.1). Differences between the distributions of polymorphisms in patients and controls were compared using the exact Fisher test and binary multivariate logistic regression (dominant, recessive, and additive models) with STATA v.9.2 (Statacorps, Lakeway Drive, TX), correcting for independent variables (Table 3). Only independent variables with p-values lower than 0.22 were considered for multivariate regression analysis. Only models with intercept values corresponding to p ≤ 0.05 were considered. The p-values were corrected for multiple testing using the false discovery rate (FDR) method (Benjamini and Hochberg, 1995), performed in R language 3.6.1, through the Stats package (R Development Core Team, 2011). Corrected p-values (Pc) lower than 0.05 were considered significant.
In Silico Analysis
To further explore the genetic associations found in the South Brazilian population, we performed in silico analysis using publicly available tools and databases (cited in Supplementary Table S1).
Linkage Disequilibrium
Since many of the variants found associated in a GWAS are not responsible for the disease and probably act as tag SNPs, we performed the LD analysis for the variants that remained associated after correction for independent variables and FDR. We used LDlink, a web-based tool that uses the publicly available reference haplotypes from Phase 3 of the 1000 Genomes Project to calculate population-specific measures of LD. Using the proxy tool of LDlink, we considered as LD variants only those with r2 > 0.8 in at least one of the following populations: from Utah with northern and western European ancestry (CEU), from Tuscany (TSI), and from the Iberian Peninsula (IBS).
Search for Associations in the Literature
For all variants considered in LD and the SNPs associated in this study, we searched for previous associations with AD in the literature through PubMed, Web of Science, and Google Scholar databases. As search terms, we initially used only each variant to check all the associations already reported. Later, we filtered for articles that included the word “Alzheimer” in the title or abstract.
Expression and Splicing Quantitative Trait Loci (eQTL/sQTL)
For SNPs associated in this study and all variants in LD with them, we evaluated their possible role as eQTL or sQTL in brain tissue and/or whole blood (GTEx and Braineac). All genes whose expression level was associated with these eQTLs and sQTLs, as well as the genes where the variants in LD were located, were investigated for their characteristics, expression, regulation, structure, function of the encoded protein, protein network, and possible associations with diseases reported in the literature.
Investigation of Non-Coding RNAs Possibly Related to AD Through the Associated or Linkage Disequilibrium Variants
For all the SNPs associated in this study and their respective variants in LD, we evaluated the physical location, especially if they occur within the sequence of non-coding RNA (ncRNA) genes, where they may affect the structure and function of the ncRNA. For variants in long non-coding RNAs (lncRNAs), we analyzed whether they could lead to a change in their secondary structure or a gain/loss of microRNA (miRNA)-binding sites, disturbing lncRNA–miRNA interactions (since both can be endogenous competitors). We also searched for information on expression, function, and previous associations of these lncRNAs in the literature and corresponding databases. All lncRNAs were mapped according to their genomic coordinates using UCSC (GRch38. p13). We also looked for genes within 2 kb distance from the 5′ and 3′ sequence limits of these lncRNA genes.
Secondary Structure of Long Non-Coding RNA Prediction
All variants found in the sequence of lncRNA genes occurred within exons, possibly resulting in a structural and, consequently, a functional change. The secondary structure of the lncRNAs was predicted through the online version of the RNAfold web server, based on the Vienna RNA package. We obtained the lncRNA sequences through NONCODE and searched for the variant’s location within the lncRNA using the Ensembl and UCSC databases (GRch38. p13). For each lncRNA, we generated secondary structures for both alleles of the variant, using the calculation of minimum free energy (MFE) and positional entropy (the input sequences are available at https://osf.io/njau3/?view_only=949c6b1c91c94e33b2c3e4d152f82a0e). To assess the mutation’s possible impact on the structure, we considered the conformational change of the molecule and the p-value provided by the lncRNASNP2 database. This p-value is empirical, generated by the SNP’s position, the GC content of the molecule, and the size of the sequence (p < 0.2 indicating possibly harmful).
Investigation of MicroRNAs That Are Possibly Affected by the Presence of Variants in Long Non-Coding RNAs
For the miRNAs affected by the variants identified in the lncRNA gene sequences, we investigated their tissue expression, lncRNA–miRNA interaction, pathway enrichment, and genes regulated by them, as well as previous associations in the literature. For the analysis of pathways, we considered only those related to Alzheimer’s disease pathophysiology through miRPath (KEGG and GO pathways).
Results
Association Study
Genotype distributions were in Hardy–Weinberg equilibrium for both patients and controls (Table 2, Table 4, and Supplementary Table S2). Using binary logistic regression, we selected nine independent variables with a tendency or an association with LOAD (p-value < 0.220). We used them in multiple regression models to correct any associations with polymorphisms (Table 3, Table 4, and Supplementary Table S2). Of the 18 selected SNPs, only four remained associated after correction for independent variables and FDR: rs769449 (APOE); rs6733839 and rs744373 (BIN1–CYP27C1); and rs10838725 (CELF1) (Table 4).
In Silico Analysis
Identification of Variants in Linkage Disequilibrium, Expression and Splicing Quantitative Trait Locus Effect, and Interference in Non-Coding RNAs
We investigated variants in LD with the four associated SNPs in the CEU, TSI, and IBS populations and found a total of 54 SNPs in LD with them (r2 > 0.8, Supplementary Table S3). For rs10838725 (CELF1), we found 49 variants in LD, present in the following genes: CELF1, MTCH2, AGBL2, FNBP4, and NUP160, and in intergenic regions: NDUFS3–FAM180B, C1QTNF4–MTCH2, and NUP160—PTPRJ (Supplementary Figure S1). In addition to rs10838725 (CELF1) itself, of the 49 variants in LD with this SNP, 40 act as eQTLs for NDUFS3, FAM180B, SLC39A13, C1QTNF4, MYBPC3, PTPRJ, FNBP4, MADD, ARHGAP1, ARFGAP2, PTPMT1, and ACP2 and sQTLs for SLC39A13 and SPI1 in brain regions and/or whole blood. Two variants in LD with rs10838725 (rs71457224 and rs10769282) occur within a lncRNA (NONHSAT021264.2) gene, where probably rs10769282 results in the loss of a binding site for hsa-miR-373-5p.
There are three SNPs in LD with rs769449 (APOE), one of which (rs429358) is responsible for the APOE*ε4 allele. rs7256200 and rs10414043 act as eQTLs for RSPH6A in intralobular white matter (Supplementary Figure S2). Besides that, rs769449 itself, rs10414043, and rs429358 can lead to alternative splicing (sQTL) of the TOMM40 pre-mRNA in the cerebellar hemisphere and cerebellum. In addition, rs429358 is also an sQTL for the APOE gene in the basal ganglia. rs10414043 and rs7256200 occur within the NONHSAT179794.1 lncRNA gene. According to in silico prediction, rs10414043 leads to a gain (hsa-miR-5089-3p) and a loss (hsa-miR-1273g-3p, hsa-miR-4252, and hsa-miR-1227-3p) of miRNA-binding sites in this lncRNA, whereas rs7256200 leads to a loss of the hsa-miR-4284–binding site. Furthermore, rs429358 is located within two lncRNA genes (NONHSAT066732.2 and NONHSAT179793.1), resulting in a gain (hsa-miR-4479) and a loss (hsa-miR-4479) of miRNA-binding sites in NONHSAT066732.2 and a gain (hsa-miR-6869-3p) in NONHSAT179793.1.
rs6733839 (BIN1–CYP27C1) is in LD only with rs4663105. This SNP acts as an eQTL for the BIN1 gene in the cerebellum and whole blood. This SNP also occurs within a lncRNA gene (NONHSAT187478.1), resulting in a gain (hsa-miR-6776-5p and hsa-miR-4455) and a loss (hsa-miR-6839-3p) of miRNA sites. Another SNP investigated in the BIN1–CYP27C1 region (rs744373) is in LD only with rs730482. None of them act like an eQTL/sQTL, but both occur within the NONHSAT182593.1 lncRNA gene, where rs744373 can lead to a gain (hsa-miR-5008-5p) or loss (hsa-miR-2467-5p, hsa-miR-657, and hsa-miR-6822-3p) of miRNA-binding sites and rs730482 can result in the loss of various miRNA-binding sites (hsa-miR-192-5p, hsa-miR-215-5p, hsa-miR-4766-3p, and hsa-miR-1224-3p).
Characterization and Secondary Structure of Candidate Long Non-Coding RNAs
We found six lncRNAs potentially involved in LOAD, carrying eight investigated variants, of which only rs744373 was associated in the South Brazilian population (Table 5). There is a general lack of information about these lncRNAs, mostly derived from databases. Except for NONHSAT066732.2, for which it is unknown whether it is not expressed in this tissue or has just not been analyzed, all others are expressed in the brain.
All the variants occur within the mature lncRNA sequence. According to results from the lncRNASNP2 database, which provides an empirical p-value for structural lncRNA damage (p < 0.2 = possibly harmful), we predicted a possible harmful shift in the secondary structure of NONHSAT179794.1 (due to rs7256200, p = 0.2019), NONHSAT066732.2, and NONHSAT179793.1 (due to rs429358, p = 0.0666 for both). Besides, a structural variation is clearly noticeable for NONHSAT021264.2 (rs71457224 and rs10769282) and NONHSAT182593.1 (rs744373 and rs730482) but possibly is not harmful (Figure 2).
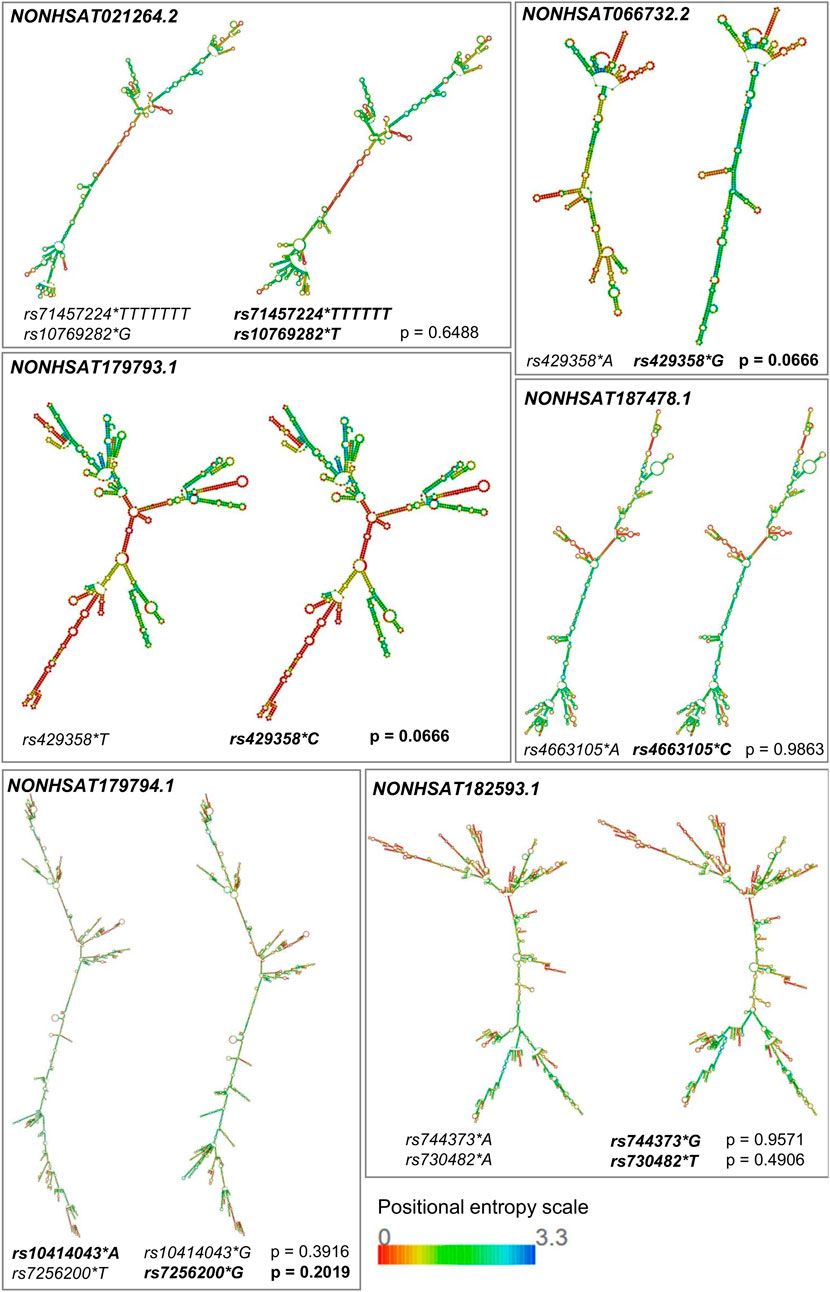
FIGURE 2. Prediction of secondary structures of lncRNAs possibly involved in AD. The secondary structures of the lncRNAs possibly involved in AD were predicted by the RNAfold web server based on the Vienna RNA package, considering the calculation of minimum free energy (MFE) and positional entropy. Structures were generated for both alleles (alleles in bold are deemed to have a possible harmful role). The impact of these mutations on the structures was established by visually changing the molecule and the p-value provided by lncRNASNP2. This p-value is empirical, being generated in silico, through the SNP’s position, the GC content of the molecule, and the size of the sequence (p < 0.2 = possibly harmful). Within the positional entropy scale, low entropy (red) leads to little structural flexibility, making the prediction more reliable, while regions of high entropy (violet) may have several alternative structures.
Through ncRPheno, a comprehensive database that provides experimentally supported associations between non-coding RNAs and disease phenotypes, we found that NONHSAT179794.1, NONHSAT179793.1, NONHSAT187478.1, and NONHSAT182593.1 could lead to neurodegenerative disorders (including LOAD) (Supplementary Figure S3).
MicroRNA-Binding Sites Affected by Single-Nucleotide Polymorphisms in Long Non-Coding RNAs
The presence of SNPs in lncRNAs could create or disrupt a miRNA-binding site. We identified 20 miRNAs whose binding sites were affected by SNPs located within lncRNAs, of which seven gained and thirteen lost a binding site (Table 5).
Of the 20 miRNAs, 14 are known to be expressed in the brain. All others do not have enough data to determine whether they are expressed or not in the brain. Only four were previously associated with any neurological disease or with AD risk factors: hsa-miR-373-5p with schizophrenia (Pala and Denkçeken, 2020); hsa-miR-657 with type 2 diabetes (Lv et al., 2008); hsa-miR-192-5p with venous thrombosis and type 2 diabetes (Lu et al., 2020; Rodriguez-Rius et al., 2020); and has-miR-147b with negative regulation of the inflammatory response (van Scheppingen et al., 2018).
Discussion
GWAS are extremely relevant for identifying genome regions associated with a disease. However, most of the associated loci occur in non-coding areas, turning it difficult to establish causality with the disease (Albert and Kruglyak, 2015). Often, the associations reflect the deregulation of gene expression, resulting from changes caused by the presence of variants in regulatory regions (promoters, enhancers) or ncRNA genes, or even from LD with variants that act as eQTLs/sQTLs (Albert and Kruglyak, 2015; Hu et al., 2019). Also, the associations found are closely related to the genetic background of the assessed population. Most of the GWAS carried out with LOAD used samples from European populations or with European ancestry (GWAS Catalog), which do not necessarily reflect the genetic diversity of other populations. Thus, we sought to replicate in the South Brazilian sample some of the main associations reported in LOAD-GWAS performed with European-derived populations, investigating the possible functional role of these variants in LOAD development.
The APOE (apolipoprotein E) gene presents three distinct allelic variants (ε2, ε3, and ε4). Their product is probably involved in Aβ production and/or clearance, neuroinflammation, synaptic loss, and tau hyperphosphorylation, important for the development and progression of LOAD (Yu et al., 2014; Yamazaki et al., 2019). The rs429358*C allele corresponds to the ε4 isoform and is considered the most critical genetic susceptibility factor for LOAD development (Corder et al., 2008; Castellano et al., 2011; Lambert et al., 2013). This SNP allele is in LD with rs769449*A, associated in our sample with susceptibility to LOAD. rs429358 is located within two lncRNAs genes (NONHSAT179793.1 and NONHSAT066732.2), leading to a change in the secondary structure of NONHSAT066732.2, resulting in the loss of the hsa-miR-147b–binding site and the gain of the hsa-miR-4479–binding site. Increased expression of hsa-miR-147b is associated with down-regulation of the inflammation driven by activated astrocytes (van Scheppingen et al., 2018). Due to the loss of miRNA–lncRNA interaction caused by rs429358*G, there is possibly a greater availability of hsa-miRNA-147b, reducing the inflammatory response. While this may seem beneficial, it possibly harms the clearance of Aβ plaques promoted by inflammatory elements. Also, through pathway enrichment analysis, we observed that hsa-miR-4479 is involved in the GABAergic pathway regulating the expression of CACNA1A, SLC32A1, PRKX, and SLC12A5 genes (miRPath). With the addition of a binding site in NONHSAT066732.2, this miRNA may be sequestered, leading to an imbalance in GABAergic signaling, which has been considered to be involved in LOAD pathology (Li et al., 2016). Besides, rs429358 is located in a CpG island and may impact DNA methylation. Foraker et al. (2015) demonstrated a difference in this region’s methylation profile between individuals with AD and controls. rs769449 is also in LD with two other variants (rs10414043 and rs7256200) located in the lncRNA NONHSAT179794.1, with rs7256200 leading to a structural change in the lncRNA molecule. Recent studies have shown that lncRNAs can affect expression of genes found in the proximity (within 2 Kb) (Engreitz et al., 2016). Thus, both NONHSAT179793.1 and NONHSAT066732.2 may interfere with APOE regulation. However, the association observed in our study with rs769449 is possibly related to its LD with other variants having high regulatory potential. Other studies have already shown that the change of G for an A allele, creating the rs769449*A allele, may favor an open chromatin state for the APOE gene, along with a correspondent strong H3K4Me3 signal (trimethylation of lysine 4 in histone H3) (Ryu et al., 2016; Babenko et al., 2018). Furthermore, the rs769449*A allele is absent in older people with greater longevity, being related to poor LOAD prognoses, such as inferior recovery of late verbal memory and faster cognitive decline (Soerensen et al., 2013; Zhang and Pierce, 2014; Arpawong et al., 2017).
Variants of BIN1 (bridging integrator 1) commonly show the second highest odds ratios for LOAD, lagging only behind APOE variants (Tan et al., 2013; Crotti et al., 2019; Franzmeier et al., 2019). It is involved in endocytosis, sustained cytoskeleton integrity, regulation of the tau peptide, and probably inflammation, calcium homeostasis, and apoptosis (Tan et al., 2013; Crotti et al., 2019; Franzmeier et al., 2019; Sartori et al., 2019; Thomas et al., 2019). Tau is a microtubule-associated protein which, under a pathological condition, is phosphorylated (pTau) and assembles into insoluble aggregates (neurofibrillary tangles), leading to synaptic dysfunction and neural cell death, which plays an essential role in the development and progression of LOAD (Franzmeier et al., 2019; Thomas et al., 2019). Our study validated the association of the two rs6733839 and rs744373 SNPs, located in the BIN1–CYP27C1 region. rs6733839 carriage is associated with higher pTau181 levels in CSF (Crotti et al., 2019). Homozygote individuals for rs6733839*T show worse episodic memory (Greenbaum et al., 2016). This SNP is in LD with rs4663105, which occurs in the NONHSAT187478.1 lncRNA gene, possibly associated with neurodegenerative diseases (ncRPheno). Also, rs4663105 is an eQTL for BIN1 in the cerebellum and whole blood (GTEx). rs744373*G was recently associated with LOAD in the Colombian population (Moreno et al., 2017) and was found to increase tau pathology in LOAD (Franzmeier et al., 2019). We found this SNP and rs730482 (both in LD) in NONHSAT182593.1 lncRNA, possibly associated with neurodegenerative diseases (ncRPheno).
The CELF1 (CUGBP Elav-like family member 1) gene encodes CELF1 protein, a RNA-binding protein related to the regulation of different post-transcriptional events and alternative splicing, mRNA translation, and mRNA stability (Beisang et al., 2012; Bateman et al., 2017). During alternative RNA processing, the protein can select the splicing target site by binding to U/G-rich elements in the transcript sequence, leading to mRNA decay and controlling translation efficiency (preprint David et al., 2020). The rs10838725 polymorphism has been associated with LOAD in a GWAS (Lambert et al., 2013; Karch et al., 2016; Marioni et al., 2018). However, this SNP possibly is not the only causal SNP, since it occurs in LD with several variants distributed in distinct genes, intergenic regions, and NONHSAT021264.2 lncRNA region (rs71457224 and rs10769282). Besides that, in this LD block, several variants act as eQTLs/sQTLs in the brain and whole blood, altering the expression of many genes already related to LOAD or other neurological diseases in human or animal studies, such as CELF1 itself, MADD, MYBPC3, NR1H3, NUP160, SPI1, and TOMM40 (Natunen et al., 2013; Karch et al., 2016; Dourlen et al., 2017; Huang et al., 2017; Katsumata et al., 2019; Zhu et al., 2019, 2020; Lutz et al., 2020).
Our work has some limitations: it does not share the statistical power of GWAS for validation of allelic associations, and we might have missed some true associations with alleles of lower frequency due to the smaller sample size. Furthermore, the secondary structures of lncRNAs are the result of an in silico analysis. This structural prediction does not consider the huge complexity of possible interactions within a RNA molecule and its interactions with other molecules, which can dramatically alter its structure. The in silico analysis results from the compilation of information obtained in online databases, some of which lack experimental validation.
Nonetheless, we replicated in the South Brazilian population the associations already reported with LOAD in a European GWAS for APOE, BIN1, and CELF1. Of the eighteen polymorphisms analyzed, only four remained associated with the South Brazilian population (these are the first confirmatory results for these polymorphisms in the Brazilian population), corroborating previous studies of our group (Kretzschmar et al., 2020). The low replication rate in South Brazilians is due to the admixture with other human groups, such as Amerindians and Africans, which causes South Brazilians to differ from Europeans, despite their major Iberian and Tuscany origin (Braun-Prado et al., 2000; Probst et al., 2000; Pena et al., 2020). This highlights the importance of replication of associated variants in different ethnicities, to contribute to a more personalized and inclusive medicine.
Furthermore, the need for functional exploration of the genetic associations found in large-scale studies is explicit, since most are not causal. Many of the associated variants are in LD with causal polymorphisms. They may act as eQTLs/sQTLs for other genes (as observed for CELF1 rs10838725), located in regions of regulation of gene expression or ncRNA genes. The influence of lncRNAs on the regulation of genes, which can cause pathological disorders, is becoming increasingly evident (Cipolla et al., 2018; Salviano-Silva et al., 2018). Through the LD analysis performed for the four associated SNPs in our study, we were able to find six lncRNAs that are possibly playing a role in LOAD and which have not been analyzed until now. Some polymorphisms can lead to changes in the secondary structure of these lncRNAs, resulting in the loss or gain in the binding of miRNAs, probably deregulating essential pathways and, consequently, causing the disease. Experimental validation studies of these lncRNAs and their alleles in LOAD can contribute to a better understanding of the disease. Thus, our study brings new promising targets for future research on Alzheimer’s disease.
Data Availability Statement
The genotype data are available in the Supplementary Material and also at OSF via the link: https://osf.io/njau3/?view_only=949c6b1c91c94e33b2c3e4d152f82a0e.
Ethics Statement
The studies involving human participants were reviewed and approved by the Research Ethics Committee of the Health Sciences Sector (Federal University of Paraná). The patients/participants provided their written informed consent to participate in this study.
Author Contributions
AB administered the project and supervised this work. GK and CM contributed to the conception of the work and curated and analyzed the data. SS and CS obtained and prepared the samples. GK, NA, SS, and CS performed the investigation. RS provided the samples, and MP-E and AB provided resources and funding for analysis. GK drafted and edited the letter, after critical review for intellectual content by all co-authors. All authors approved the final version of the work.
Funding
This work was supported by grants of Fundação Araucária (F.A. protocol 39894.413.43926.1904/2013 and 116/2018, protocol 50.530) and by Coordenação de Aperfeiçoamento de Pessoal de Nível Superior–Brasil (CAPES) (40001016006P1) and CAPES/PROAP Finance Code 001. ABWB receives a research productivity scholarship from Conselho Nacional de Desenvolvimento Científico e Tecnológico (CNPq 314288/2018-0). The funding agencies had no role in study design, in the collection, analysis, and interpretation of data, in the writing of the manuscript, and in the decision to submit it for publication.
Conflict of Interest
The authors declare that the research was conducted in the absence of any commercial or financial relationships that could be construed as a potential conflict of interest.
Acknowledgments
We are deeply grateful to all the research participants, especially to the patients. We thank the Polymorphism and Linkage Laboratory/UFPR staff for their assistance in DNA extraction.
Supplementary Material
The Supplementary Material for this article can be found online at: https://www.frontiersin.org/articles/10.3389/fmolb.2021.632314/full#supplementary-material
References
Albert, F. W., and Kruglyak, L. (2015). The Role of Regulatory Variation in Complex Traits and Disease. Nat. Rev. Genet. 16, 197–212. doi:10.1038/nrg3891
Arpawong, T. E., Pendleton, N., Mekli, K., McArdle, J. J., Gatz, M., Armoskus, C., et al. (2017). Genetic Variants Specific to Aging-Related Verbal Memory: Insights from GWASs in a Population-Based Cohort. PLoS One 12, e0182448. doi:10.1371/journal.pone.0182448
Babenko, V. N., Afonnikov, D. A., Ignatieva, E. V., Klimov, A. V., Gusev, F. E., and Rogaev, E. I. (2018). Haplotype Analysis of APOE Intragenic SNPs. BMC Neurosci. 19, 16. doi:10.1186/s12868-018-0413-4
Bateman, A., Martin, M. J., O’Donovan, C., Magrane, M., Alpi, E., Antunes, R., et al. (2017). UniProt: The Universal Protein Knowledgebase. Nucleic Acids Res. 45, D158–D169. doi:10.1093/nar/gkw1099
Beisang, D., and Vlasova-St. LouisLouis, I. A. I. A. (2012). “CELF1, a Multifunctional Regulator of Posttranscriptional Networks,” in Binding Protein. InTech. doi:10.5772/48780
Benjamini, Y., and Hochberg, Y. (1995). Controlling the False Discovery Rate: A Practical and Powerful Approach to Multiple Testing. J. R. Stat. Soc. Ser. B (Methodological) 57, 289–300. doi:10.1111/j.2517-6161.1995.tb02031.x
Braak, H., and Braak, E. (1991). Neuropathological Stageing of Alzheimer-Related Changes. Acta Neuropathol. 82, 239–259. doi:10.1007/BF00308809
Braun-Prado, K., Vieira Mion, A. L., Farah Pereira, N., Culpi, L., and Petzl-Erler, M. L. (2000). HLA Class I Polymorphism, as Characterised by PCR-SSOP, in a Brazilian Exogamic Population. Tissue Antigens 56, 417–427. doi:10.1034/j.1399-0039.2000.560504.x
Buniello, A., MacArthur, J. A. L., Cerezo, M., Harris, L. W., Hayhurst, J., Malangone, C., et al. (2019). The NHGRI-EBI GWAS Catalog of Published Genome-wide Association Studies, Targeted Arrays and Summary Statistics 2019. Nucleic Acids Res. 47, D1005–D1012. doi:10.1093/nar/gky1120
Castellano, J. M., Kim, J., Stewart, F. R., Jiang, H., DeMattos, R. B., Patterson, B. W., et al. (2011). Human apoE Isoforms Differentially Regulate Brain Amyloid- Peptide Clearance. Sci. Translational Med. 3, 89ra57. doi:10.1126/scitranslmed.3002156
Cipolla, G., de Oliveira, J., Salviano-Silva, A., Lobo-Alves, S., Lemos, D., Oliveira, L., et al. (2018). Long Non-coding RNAs in Multifactorial Diseases: Another Layer of Complexity. ncRNA 4, 13. doi:10.3390/ncrna4020013
Consortium, T. 1000 G. P., Abecasis, G. R., Altshuler, D., Auton, A., Brooks, L. D., Durbin, R. M., et al. (2010). A Map of Human Genome Variation from Population-Scale Sequencing. Nature 467, 1061–1073. doi:10.1038/nature09534
Corder, E. H., Saunders, A. M., Strittmatter, W. J., Schmechel, D. E., Gaskell, P. C., Small, G. W., et al. (2008). Gene Dose of Apolipoprotein E Type 4 Allele and the Risk of Alzheimer ’ S Disease in Late Onset Families Published by: American Association for the Advancement of Science Stable URL, 261. Adv. Sci, 921–923. http://www.jstor.org/stable/2882127.
Crotti, A., Sait, H. R., McAvoy, K. M., Estrada, K., Ergun, A., Szak, S., et al. (2019). BIN1 Favors the Spreading of Tau via Extracellular Vesicles. Sci. Rep. 9, 9477. doi:10.1038/s41598-019-45676-0
Cruchaga, C., Kauwe, J. S. K., Harari, O., Jin, S. C., Cai, Y., Karch, C. M., et al. (2013). GWAS of Cerebrospinal Fluid Tau Levels Identifies Risk Variants for Alzheimer's Disease. Neuron 78, 256–268. doi:10.1016/j.neuron.2013.02.026
Cummings, A. C., Jiang, L., Velez Edwards, D. R., Mccauley, J. L., Laux, R., Mcfarland, L. L., et al. (2012). Genome-Wide Association and Linkage Study in the Amish Detects a Novel Candidate Late-Onset Alzheimer Disease Gene. Ann. Hum. Genet. 76, 342–351. doi:10.1111/j.1469-1809.2012.00721.x
David, G., Reboutier, D., Deschamps, S., Méreau, A., Taylor, W., Padilla-Parra, S., et al. (2020). The RNA-Binding Proteins CELF1 and ELAVL1 Cooperatively Control RNA Isoform Production. bioRxiv. doi:10.1101/373704
Dourlen, P., Fernandez-Gomez, F. J., Dupont, C., Grenier-Boley, B., Bellenguez, C., Obriot, H., et al. (2017). Functional Screening of Alzheimer Risk Loci Identifies PTK2B as an In Vivo Modulator and Early Marker of Tau Pathology. Mol. Psychiatry 22, 874–883. doi:10.1038/mp.2016.59
Engreitz, J. M., Haines, J. E., Perez, E. M., Munson, G., Chen, J., Kane, M., et al. (2016). Local Regulation of Gene Expression by lncRNA Promoters, Transcription and Splicing. Nature 539, 452–455. doi:10.1038/nature20149
Foraker, J., Millard, S. P., Leong, L., Thomson, Z., Chen, S., Keene, C. D., et al. (2015). The APOE Gene Is Differentially Methylated in Alzheimer's Disease. Jad 48, 745–755. doi:10.3233/JAD-143060
Franzmeier, N., Rubinski, A., Rubinski, A., Neitzel, J., and Ewers, M. (2019). The BIN1 Rs744373 SNP Is Associated with Increased Tau-PET Levels and Impaired Memory. Nat. Commun. 10, 1766. doi:10.1038/s41467-019-09564-5
Frota, N. A. F., Nitrini, R., Damasceno, B. P., Forlenza, O. V., Dias-Tosta, E., Silva, A. B. d., et al. (2011). Criteria for the Diagnosis of Alzheimer's Disease: Recommendations of the Scientific Department of Cognitive Neurology and Aging of the Brazilian Academy of Neurology. Dement. Neuropsychol. 5, 146–152. doi:10.1590/S1980-57642011DN05030002
Greenbaum, L., Ravona-Springer, R., Lubitz, I., Schmeidler, J., Cooper, I., Sano, M., et al. (2016). Potential Contribution of the Alzheimer׳s Disease Risk Locus BIN1 to Episodic Memory Performance in Cognitively normal Type 2 Diabetes Elderly. Eur. Neuropsychopharmacol. 26, 787–795. doi:10.1016/j.euroneuro.2015.11.004
Harold, D., Abraham, R., Hollingworth, P., Sims, R., Gerrish, A., Hamshere, M. L., et al. (2009). Genome-wide Association Study Identifies Variants at CLU and PICALM Associated with Alzheimer's Disease. Nat. Genet. 41, 1088–1093. doi:10.1038/ng.440
Hollingworth, P., Harold, D., Sims, R., Gerrish, A., Lambert, J. C., Carrasquillo, M. M., et al. (2011). Common Variants at ABCA7, MS4A6A/MS4A4E, EPHA1, CD33 and CD2AP Are Associated with Alzheimer's Disease. Nat. Genet. 43, 429–435. doi:10.1038/ng.803.Common
Hu, Y., Zhao, T., Zang, T., Zhang, Y., and Cheng, L. (2019). Identification of Alzheimer's Disease-Related Genes Based on Data Integration Method. Front. Genet. 9, 1–7. doi:10.3389/fgene.2018.00703
Huang, K.-l., Marcora, E., Marcora, E., Pimenova, A. A., Di Narzo, A. F., Kapoor, M., et al. (2017). A Common Haplotype Lowers PU.1 Expression in Myeloid Cells and Delays Onset of Alzheimer's Disease. Nat. Neurosci. 20, 1052–1061. doi:10.1038/nn.4587
Kamboh, M. I., Barmada, M. M., Barmada, M. M., Demirci, F. Y., Minster, R. L., Carrasquillo, M. M., et al. (2012). Genome-wide Association Analysis of Age-At-Onset in Alzheimer's Disease. Mol. Psychiatry 17, 1340–1346. doi:10.1038/mp.2011.135
Karch, C. M., Ezerskiy, L. A., Bertelsen, S., and Goate, A. M. (2016). Alzheimer's Disease Risk Polymorphisms Regulate Gene Expression in the ZCWPW1 and the CELF1 Loci. PLoS One 11, e0148717. doi:10.1371/journal.pone.0148717
Katsumata, Y., Nelson, P. T., Estus, S., and Fardo, D. W. (2019). Translating Alzheimer's Disease-Associated Polymorphisms into Functional Candidates: a Survey of IGAP Genes and SNPs. Neurobiol. Aging 74, 135–146. doi:10.1016/j.neurobiolaging.2018.10.017
Kretzschmar, G. C., Antoniazzi, A. A. H., Oliveira, L. C., Nisihara, R. M., Petzl-Erler, M. L., de Souza, R. L. R., et al. (2020). First Report of CR1 Polymorphisms and Soluble CR1 Levels Associated with Late Onset Alzheimer's Disease (LOAD) in Latin America. J. Mol. Neurosci. 70, 1338–1344. doi:10.1007/s12031-020-01547-2
Lahiri, D. K., and Numberger, J. I. (1991). A Rapid Non-enzymatic Method for the Preparation of HMW DNA from Blood for RFLP Studies. Nucl. Acids Res. 19, 5444. doi:10.1093/nar/19.19.5444
Lambert, J.-C., Heath, S., Heath, S., Even, G., Campion, D., Sleegers, K., et al. (2009). Genome-wide Association Study Identifies Variants at CLU and CR1 Associated with Alzheimer's Disease. Nat. Genet. 41, 1094–1099. doi:10.1038/ng.439
Lambert, J. C., Ibrahim-Verbaas, C. A., Harold, D., Naj, A. C., Sims, R., Bellenguez, C., et al. (2013). Meta-analysis of 74,046 Individuals Identifies 11 New Susceptibility Loci for Alzheimer's Disease. Nat. Genet. 45, 1452–1458. doi:10.1038/ng.2802
Lane, C. A., Hardy, J., and Schott, J. M. (2018). Alzheimer's Disease. Eur. J. Neurol. 25, 59–70. doi:10.1111/ene.13439
Li, Y., Sun, H., Chen, Z., Xu, H., Bu, G., and Zheng, H. (2016). Implications of GABAergic Neurotransmission in Alzheimer's Disease. Front. Aging Neurosci. 8, 31. doi:10.3389/fnagi.2016.00031
Lima-Costa, M. F., Rodrigues, L. C., Rodrigues, L. C., Barreto, M. L., Gouveia, M., Horta, B. L., et al. (2015). Genomic Ancestry and Ethnoracial Self-Classification Based on 5,871 Community-Dwelling Brazilians (The Epigen Initiative). Sci. Rep. 5, 9812. doi:10.1038/srep09812
Linnertz, C., Lutz, M. W., Ervin, J. F., Allen, J., Miller, N. R., Welsh-Bohmer, K. A., et al. (2014). The Genetic Contributions of SNCA and LRRK2 Genes to Lewy Body Pathology in Alzheimer's Disease. Hum. Mol. Genet. 23, 4814–4821. doi:10.1093/hmg/ddu196
Lu, Y., Li, Y., Li, G., and Lu, H. (2020). Identification of Potential Markers for Type 2 Diabetes Mellitus via Bioinformatics Analysis. Mol. Med. Rep. 22, 1868–1882. doi:10.3892/mmr.2020.11281
Lutz, M. W., Sprague, D., Barrera, J., and Chiba-Falek, O. (2020). Shared Genetic Etiology Underlying Alzheimer's Disease and Major Depressive Disorder. Transl. Psychiatry 10. doi:10.1038/s41398-020-0769-y
Lv, K., Guo, Y., Zhang, Y., Wang, K., Jia, Y., and Sun, S. (2008). Allele-specific Targeting of Hsa-miR-657 to Human IGF2R Creates a Potential Mechanism Underlying the Association of ACAA-Insertion/deletion Polymorphism with Type 2 Diabetes. Biochem. Biophysical Res. Commun. 374, 101–105. doi:10.1016/j.bbrc.2008.06.102
Marioni, R. E., Harris, S. E., Zhang, Q., McRae, A. F., Hagenaars, S. P., Hill, W. D., et al. (2018). GWAS on Family History of Alzheimer's Disease. Transl. Psychiatry 8, 99. doi:10.1038/s41398-018-0150-6
Maurano, M. T., Humbert, R., Rynes, E., Thurman, R. E., Haugen, E., Wang, H., et al. (2012). Systematic Localization of Common Disease-Associated Variation in Regulatory DNA. Science 337, 1190–1195. doi:10.1126/science.1222794
Moreno, D. J., Ruiz, S., Ríos, Á., Lopera, F., Ostos, H., Via, M., et al. (2017). Association of GWAS Top Genes with Late-Onset Alzheimer's Disease in Colombian Population. Am. J. Alzheimers Dis. Other Demen. 32, 27–35. doi:10.1177/1533317516679303
Natunen, T., Martiskainen, H., Sarajärvi, T., Helisalmi, S., Pursiheimo, J.-P., Viswanathan, J., et al. (2013). Effects of NR1H3 Genetic Variation on the Expression of Liver X Receptor α and the Progression of Alzheimer's Disease. PLoS One 8, e80700. doi:10.1371/journal.pone.0080700
Nédélec, Y., Sanz, J., Baharian, G., Szpiech, Z. A., Pacis, A., Dumaine, A., et al. (2016). Genetic Ancestry and Natural Selection Drive Population Differences in Immune Responses to Pathogens. Cell 167, 657–669. e21. doi:10.1016/j.cell.2016.09.025
Pala, E., and Denkçeken, T. (2020). Evaluation of miRNA Expression Profiles in Schizophrenia Using Principal-Component Analysis-Based Unsupervised Feature Extraction Method. J. Comput. Biol. 27, 1253–1263. doi:10.1089/cmb.2019.0412
Pena, S. D. J., Santos, F. R., and Tarazona‐Santos, E. (2020). Genetic Admixture in Brazil. Am. J. Med. Genet. 184, 928–938. doi:10.1002/ajmg.c.31853
Probst, C. M., Bompeixe, E. P., Pereira, N. F., de O Dalalio, M. M., Visentainer, J. E., Tsuneto, L. T., et al. (2000). HLA Polymorphism and Evaluation of European, African, and Amerindian Contribution to the white and Mulatto Populations from Paraná, Brazil. Hum. Biol. 72, 597–617.
R Development Core Team, R. (2011). R: A Language and Environment for Statistical Computing. R. Found. Stat. Comput. 1, 409. doi:10.1007/978-3-540-74686-7
Rodriguez-Rius, A., Lopez, S., Martinez-Perez, A., Souto, J. C., and Soria, J. M. (2020). Identification of a Plasma MicroRNA Profile Associated with Venous Thrombosis. Atvb 40, 1392–1399. doi:10.1161/ATVBAHA.120.314092
Ryu, S., Atzmon, G., Barzilai, N., Raghavachari, N., and Suh, Y. (2016). Genetic Landscape of APOE in Human Longevity Revealed by High-Throughput Sequencing. Mech. Ageing Development 155, 7–9. doi:10.1016/j.mad.2016.02.010
Salviano-Silva, A., Lobo-Alves, S., Almeida, R., Malheiros, D., and Petzl-Erler, M. (2018). Besides Pathology: Long Non-coding RNA in Cell and Tissue Homeostasis. ncRNA 4, 3–37. doi:10.3390/ncrna4010003
Sartori, M., Mendes, T., Desai, S., Lasorsa, A., Herledan, A., Malmanche, N., et al. (2019). BIN1 Recovers Tauopathy-Induced Long-Term Memory Deficits in Mice and Interacts with Tau through Thr348 Phosphorylation. Acta Neuropathol. 138, 631–652. doi:10.1007/s00401-019-02017-9
Simón-Sánchez, J., Schulte, C., Bras, J. M., Sharma, M., Gibbs, J. R., Berg, D., et al. (2009). Genome-wide Association Study Reveals Genetic Risk Underlying Parkinson's Disease. Nat. Genet. 41, 1308–1312. doi:10.1038/ng.487
Soerensen, M., Dato, S., Tan, Q., Thinggaard, M., Kleindorp, R., Beekman, M., et al. (2013). Evidence from Case-Control and Longitudinal Studies Supports Associations of Genetic Variation in APOE, CETP, and IL6 with Human Longevity. Age 35, 487–500. doi:10.1007/s11357-011-9373-7
Tan, M.-S., Yu, J.-T., and Tan, L. (2013). Bridging Integrator 1 (BIN1): Form, Function, and Alzheimer's Disease. Trends Mol. Med. 19, 594–603. doi:10.1016/j.molmed.2013.06.004
Thomas, S., Hoxha, K., Tran, A., and Prendergast, G. C. (2019). Bin1 Antibody Lowers the Expression of Phosphorylated Tau in Alzheimer's Disease. J. Cel. Biochem. 120, 18320–18331. doi:10.1002/jcb.29142
van Scheppingen, J., Mills, J. D., Zimmer, T. S., Broekaart, D. W. M., Iori, V., Bongaarts, A., et al. (2018). miR147b: A Novel Key Regulator of Interleukin 1 Beta-Mediated Inflammation in Human Astrocytes. Glia 66, 1082–1097. doi:10.1002/glia.23302
Yamazaki, Y., Zhao, N., Caulfield, T. R., Liu, C.-C., and Bu, G. (2019). Apolipoprotein E and Alzheimer Disease: Pathobiology and Targeting Strategies. Nat. Rev. Neurol. 15, 501–518. doi:10.1038/s41582-019-0228-7
Yu, J.-T., Tan, L., and Hardy, J. (2014). Apolipoprotein E in Alzheimer's Disease: An Update. Annu. Rev. Neurosci. 37, 79–100. doi:10.1146/annurev-neuro-071013-014300
Zhang, C., and Pierce, B. L. (2014). Genetic Susceptibility to Accelerated Cognitive Decline in the US Health and Retirement Study. Neurobiol. Aging 35, e11–1512. e18. doi:10.1016/j.neurobiolaging.2013.12.021
Zhu, Z., Lin, Y., Li, X., Driver, J. A., and Liang, L. (2019). Shared Genetic Architecture between Metabolic Traits and Alzheimer's Disease: a Large-Scale Genome-wide Cross-Trait Analysis. Hum. Genet. 138, 271–285. doi:10.1007/s00439-019-01988-9
Zhu, Z., Yang, Y., Xiao, Z., Zhao, Q., Wu, W., Liang, X., et al. (2020). TOMM40 and APOE Variants Synergistically Increase the Risk of Alzheimer's Disease in a Chinese Population. Aging Clin. Exp. Res. doi:10.1007/s40520-020-01661-6
Keywords: Alzheimer’s disease, GWAS, APOE, BIN1, CELF1, lncRNA, miRNA
Citation: Kretzschmar GC, Alencar NM, da Silva SSL, Sulzbach CD, Meissner CG, Petzl-Erler ML, Souza RLR and Boldt ABW (2021) GWAS-Top Polymorphisms Associated With Late-Onset Alzheimer Disease in Brazil: Pointing Out Possible New Culprits Among Non-Coding RNAs. Front. Mol. Biosci. 8:632314. doi: 10.3389/fmolb.2021.632314
Received: 23 November 2020; Accepted: 31 May 2021;
Published: 05 July 2021.
Edited by:
Gennaro Pagano, King’s College London, United KingdomReviewed by:
Vicente de Paulo Martins, University of Brasilia, BrazilWan-Ping Lee, University of Pennsylvania, United States
Copyright © 2021 Kretzschmar, Alencar, da Silva, Sulzbach, Meissner, Petzl-Erler, Souza and Boldt. This is an open-access article distributed under the terms of the Creative Commons Attribution License (CC BY). The use, distribution or reproduction in other forums is permitted, provided the original author(s) and the copyright owner(s) are credited and that the original publication in this journal is cited, in accordance with accepted academic practice. No use, distribution or reproduction is permitted which does not comply with these terms.
*Correspondence: Angelica Beate Winter Boldt, YW5nZWxpY2Fib2xkdEBnbWFpbC5jb20=