- 1State Key Laboratory of Organ Failure Research, School of Basic Medical Sciences, Southern Medical University, Guangzhou, China
- 2Department of Respiratory and Critical Care Medicine, Nanfang Hospital, Southern Medical University, Guangzhou, China
Radiotherapy (RT) plays an important role in the prognosis of lung adenocarcinoma (LUAD) patients, but the radioresistance (RR) of LUAD is still a challenge that needs to be overcome. The current study aimed to investigate LUAD patients with RR to illuminate the underlying mechanisms. We utilized gene set variation analysis (GSVA) and The Cancer Immunome Atlas (TCIA) database to characterize the differences in biological functions and neoantigen-coding genes between RR and radiosensitive (RS) patients. Weighted Gene co-expression network analysis (WGCNA) was used to explore the relationship between RT-related traits and hub genes in two modules, i.e., RR and RS; two representative hub genes for RR (MZB1 and DERL3) and two for RS (IFI35 and PSMD3) were found to be related to different RT-related traits. Further analysis of the hub genes with the Lung Cancer Explorer (LCE), PanglaoDB and GSVA resources revealed the differences in gene expression levels, cell types and potential functions. On this basis, the Tumor and Immune System Interaction Database (TISIDB) was used to identify the potential association between RR genes and B cell infiltration. Finally, we used the Computational Analysis of Resistance (CARE) database to identify specific gene-associated drugs for RR patients and found that GSK525762A and nilotinib might be promising candidates for RR treatment. Taken together, these results demonstrate that B cells in TME may have a significant impact on the RT and that these two drug candidates, GSK525762A and nilotinib, might be helpful for the treatment of RR patients.
Introduction
Lung cancer is one of the deadliest malignancies in the world. Eighty-five percent of lung cancer patients suffer from non-small-cell lung cancer (NSCLC) and are in the advanced stage, requiring more aggressive treatment options (Price, 2012; Kumarakulasinghe et al., 2015). Histopathologically, NSCLC can be divided into lung adenocarcinoma (LUAD) and lung squamous cell carcinoma (LUSC). LUAD is the most common subtype, accounting for more than 50% of all NSCLC cases (Kumarakulasinghe et al., 2015; Faruki et al., 2017). The interaction between immune cells and the tumor microenvironment (TME) is highly dynamic in different steps of cancer progression (Xiao et al., 2019; Deberardinis, 2020; Menzel and Black, 2020). Although the microenvironment of normal tissue can inhibit tumor growth, changes induced by tumor cells influence this microenvironment to promote tumor progression and metastasis (Wallace et al., 2013). In addition, numerous studies have shown that the tumor-infiltrating immune cells play a pivotal role in the TME (Chen et al., 2020).
Radiotherapy (RT) could not only reduce tumor burden to the minimum but also trigger the anti-tumor immunity and reprogram the TME (Liu et al., 2020). However, Radioresistance (RR) limits the efficacy of RT through TME alteration during fractionated RT (Gu et al., 2019). Several studies have shown that interactions between multiple cells in the TME are the main cause of treatment tolerance to RT (Yang et al., 2019). Nevertheless, the molecular mechanism of radioresistant LUAD is not yet well understood.
Over the past 25 years, high-throughput sequencing technologies have been used to explore the mechanism of tumorigenesis (Cancer Genome Atlas Research Network, 2014; Girard et al., 2016). On this basis, a series of immune-related prediction algorithms have been developed (Rigden and Fernandez, 2020), which provide an excellent chance to explore the TME of various tumors. To explore the mechanism of RT in LUAD and provide helpful advice for treatment, in this study, we performed integrated bioinformatic analysis of LUAD and identified functional differences among RT-treated LUAD patients after gene set variation analysis (GSVA) with The Cancer Genome Atlas (TCGA) data.
The Cancer Immunome Atlas (TCIA) was used to analyze tumor neoantigen-coding genes associated with TME differences among individual RT patients. Furthermore, we performed weighted gene co-expression network analysis (WGCNA) to identify the key modules related to clinical characteristics and ClueGO to assess the potential function of these modules. Four hub genes, including RR module genes (MZB1 and DERL3) and radiosensitive (RS) module genes (PSMD3 and IFI35), were selected for further analysis. Lung Cancer Explorer (LCE) and PanglaoDB were used to determine the gene expression and localization in different cells, and GSVA was used to study the potential biological function of the hub genes. After finding that RR was related to the response module and TME, the Tumor and Immune System Interaction Database (TISIDB) was used to evaluate the correlation between immune cell abundance and the hub genes in the LUAD samples. Finally, we analyzed the expression of the hub genes and the efficacy of drugs derived from the Computational Analysis of Resistance (CARE) database to provide potential complementary therapy for RR patients.
Materials and Methods
Data Collection
Transcriptome RNA-seq data of 513 LUAD tumor samples and the corresponding clinical data were downloaded from TCGA (release-18.0) database (https://portal.gdc.cancer.gov/) with level 3. Based on the TCGA sample phenotype, we defined the patients with complete response (CR)/partial response (PR) as RS, and those with progressive disease (PD)/stable disease (SD) as RR.
Gene Set Variation Analysis
We utilized the GSVA (Hanzelmann et al., 2013) R package version 1.34.0 to find the pathways most associated with RT and the potential function of RT-related hub genes by setting kcdf = “Poisson,” min.sz = 20, max.sz = 500. Fifty samples with RT data in Table 1 were selected for GSVA analysis, and both top 25 terms enriched in RR or RS groups were selected for heatmap display (Kolde, 2019).
Through GSVA analysis for a single gene, 513 TCGA-LUAD tumor samples were divided into a high expression group and a low expression group based on the median gene expression to reveal the gene potential function. In addition, the “Limma” R package version 3.42.2 (Ritchie et al., 2015) was used to find the different essential pathways of LUAD. Adjusted p < 0.001 was regarded as statistically significant. The gene sets of “c2.cp.kegg.v7.0.symbols.gmt,” “c2.cp.reactome.v7.0.symbols.gmt,” “c5.all.v7.0.symbols.gmt,” and “c7.all.v7.0.symbols.gmt” were downloaded from the Molecular Signature Database (MSigDB, http://software.broadinstitute.org/gsea/downloads.jsp) (Liberzon et al., 2015) as the reference.
Weighted Gene Co-Expression Network Construction
The R package Weighted Correlation Network Analysis (WGCNA) version 1.70-3 (Langfelder and Horvath, 2008) was applied to find clinical trait-related modules and hub genes among them. The adjacency matrix was transformed into a topological overlap matrix (TOM). According to the TOM-based dissimilarity measure, genes were divided into different gene modules. Here, we set the soft-thresholding power to 3 (scale-free R2 = 0.85, networkType = “signed hybrid”), the cut off to 0.25, deepSplit to 3, TOMType to unsigned, corType to pearson, and the minimal module size to 50 to identify key modules. The module with the highest and lowest correlation with RT was selected to explore its biological function through ClueGO analyses. Hub genes were defined as those with eigengene connectivity (kME) > 0.8.
Gene Function Enrichment and Pathway Analysis
We conducted Gene Ontology (GO) enrichment and Kyoto Encyclopedia of Genes and Genomes (KEGG) pathway analysis using Cytoscape version 3.7.2 (Doncheva et al., 2019) software “ClueGO” (Bindea et al., 2009) as well as the R package “clusterProfiler” version 3.14.3 (Yu et al., 2012). GO term or KEGG pathways with adjusted p < 0.05 were considered statistically significant and visualized by “ClueGO” and “Enrichplot” (Yu, 2018).
Analysis of Neoantigen-Coding Genes of RT Samples
The Cancer Immunome Atlas (https://tcia.at/home; Charoentong et al., 2017) is widely used in the query for gene expression of neoantigens and cancer germline antigens, by which we collected the neoantigen-coding genes of RR and RS samples with default parameters. We got the list of neoantigen-coding genes by choosing the “Neoantigens” tab after inputting IDs of RS and RR patients in the TCIA filter. In addition, a web tool for list comparison, jvenn (http://jvenn.toulouse.inra.fr/app/example.html; Bardou et al., 2014), was used to find different neoantigen-coding genes from the RT samples. GO and KEGG analyses were performed to study the biological functions and associated pathways of the differential neoantigen-coding genes.
Analysis of Gene Expression in Different Cell Types
To investigate the expression of hub genes in LUAD, we applied the online tool Lung Cancer Explorer (http://lce.biohpc.swmed.edu/lungcancer/metagenename.php; Cai et al., 2019), which contains 56 lung cancer datasets from the Sequence Read Archive (SRA) and Gene Expression Omnibus (GEO) databases. From this database, we collected a meta-analysis of hub gene expression. Then we performed meta-analysis on the LUAD dataset with default parameters. In addition, PanglaoDB, a single-cell gene expression resource database (https://PanglaoDB.se/index.html; Franzen et al., 2019), was used to confirm the cell type expressing hub genes.
Analysis of Gene Expression and Tumor-Infiltrating Immune Cells
To investigate the correlation between the expression of selected hub genes and the abundance of tumor-infiltrating lymphocytes (TILs), we applied the online tool TISIDB (http://cis.hku.hk/TISIDB/index.php; Ru et al., 2019), which reports 988 genes involved in antitumor immunity. Then we determined the relationship between hub gene expression and tumor-infiltrating immune cells by TISIDB analysis with selection of the tabs for lymphocytes, the LUAD dataset and antigen-presenting cells (APCs).
Hub Gene Computational Analysis of Drug Efficacy
Computational Analysis of Resistance (CARE, http://care.dfci.harvard.edu/) (Jiang et al., 2018) was utilized to evaluate the hub genes associated with drug efficacy. A positive CARE score indicates a higher expression value to be associated with drug response, while a negative score indicates drug resistance. CARE can evaluate the relationship between hub genes and drug therapy and can be used as an alternative therapy for RR. The 3D structures of drugs were downloaded from the PubChem database (http://pubchem.ncbi.nlm.nih.gov/).
Score Calculation of Tumor-Infiltrating Immune Cells
To verify the differences of tumor-infiltrated immune cells between RR and RS groups, we used CIBERSORT-ABS in an online tool TIMER2.0 (Newman et al., 2015; Li et al., 2016; Sturm et al., 2019) to calculate the scores of tumor-infiltrated immune cells by tissue transcriptional profiles. The average CIBERSORT-ABS scores of both groups are showed in a bar plot.
Results
Identification of RT-Related Gene Function and Tumor Immune Environment
Data analysis flow chart was shown in Figure 1. To find the difference between the RR and RS groups, we utilized GSVA to distinguish the biological function of the RR and RS samples (Figure 2A). Based on GSVA analysis, we found that the functional difference between RS and RR patients was associated with extracellular matrix (ECM) and DNA-related functions, which was consistent with previous reports (Ljungman, 2009; Bian et al., 2020; Haeger et al., 2020). Interestingly, two immune-related terms, immunoglobulin receptor binding and CD22-mediated BCR regulation (Figure 2A), were observed in the list of the top 25 terms enriched in the RR or RS groups, indicating that the immune microenvironment is involved in the RT response.
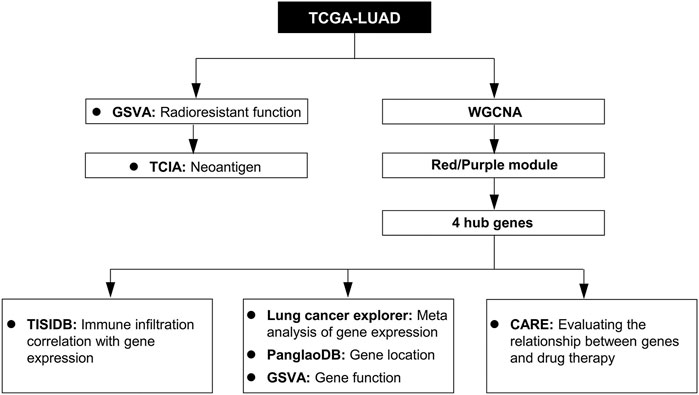
FIGURE 1. Study workflow. TCGA-LUAD, The Cancer Genome Atlas LUAD datasets; GSVA, gene set variation analysis; WGCNA, weighted gene co-expression network analysis; TCIA, The Cancer Immunome Database; TISIDB, Tumor and Immune System Interaction Database; PanglaoDB, single-cell RNA sequencing experiments from mouse and human database; CARE, Computational Analysis of Resistance.
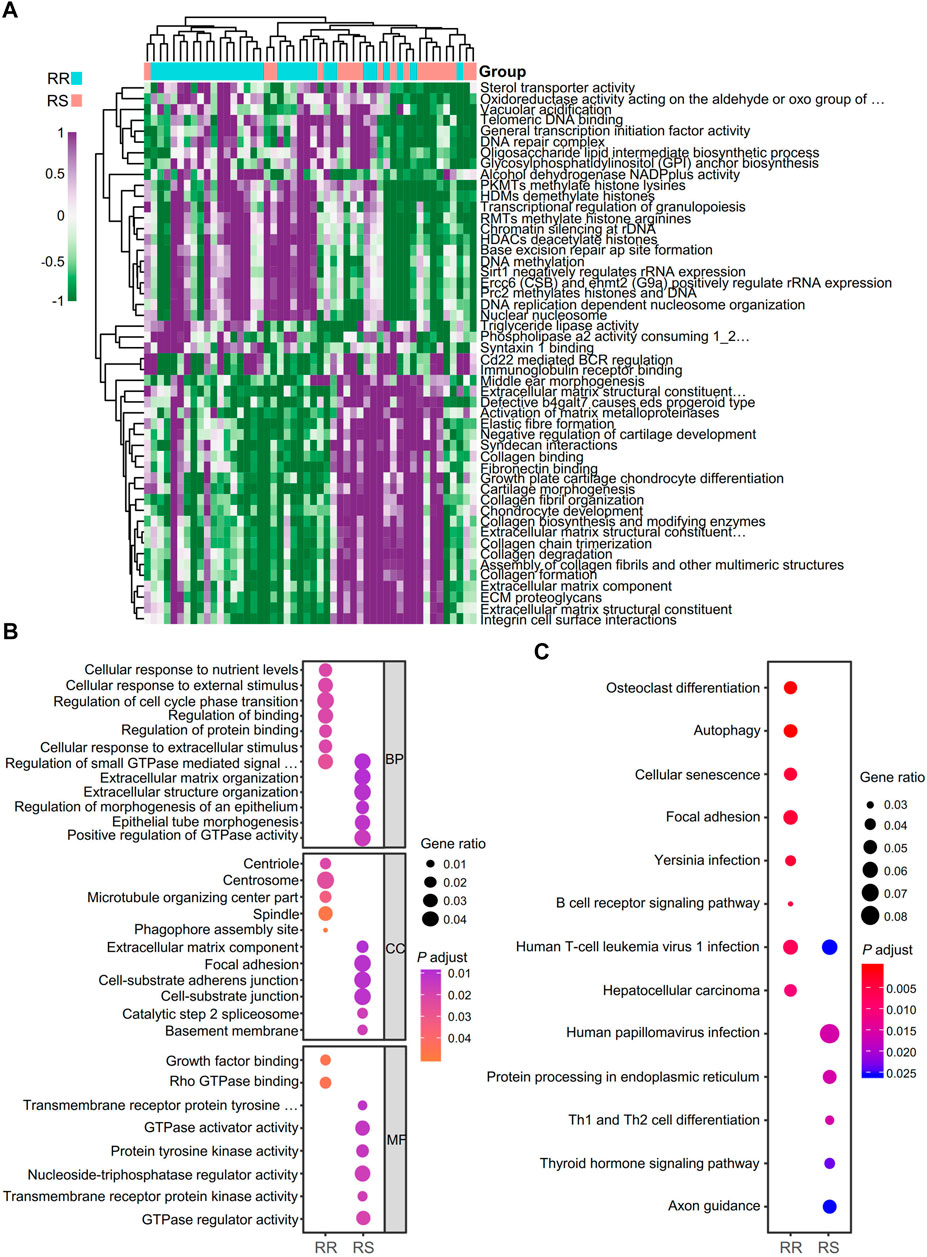
FIGURE 2. Identification of biological function and neoantigen-coding genes of RT patients. (A) Analysis of the biological functions of RT patients with GSVA. RR, radioresistant; RS, radiosensitive. (B) GO term analysis for differential neoantigen-coding genes between the RR and RS groups in the TCIA database. (C) KEGG analysis of differential neoantigen-coding genes between the RR and RS groups in the TCIA database.
To identify neoantigen-coding genes in RR and RS patients, the TCIA database was used for analysis, and we found that there were only 13.6% neoantigen-coding genes with overlap. To address the different functions of RS and RR neoantigen-coding genes, GO and KEGG analyses were performed. In GO term analysis, we observed that the RR group was mainly related to the terms of cellular response to nutrient levels, cellular response to external stimulus, regulation of small GTPase mediated signal transduction, regulation of cell cycle phase transition, regulation of binding, centriole, spindle, microtubule organizing center part, growth factor binding and Rho GTPase binding, while the RS group was closely related to the terms extracellular matrix organization, regulation of morphogenesis of an epithelium, epithelial tube morphogenesis, positive regulation of GTPase activity, focal adhesion, cell-substrate junction, catalytic step 2 spliceosome, basement membrane, protein tyrosine kinase activity, GTPase regulator activity and transmembrane receptor protein kinase activity (Figure 2B).
The results of KEGG pathway analysis demonstrated that the RR group was related to the terms osteoclast differentiation, autophagy, cellular senescence, focal adhesion and B cell receptor signaling pathway, while the RS group was mainly related to human papillomavirus infection, protein processing in the endoplasmic reticulum (ER), Th1 and Th2 cell differentiation, thyroid hormone signaling pathway and axon guidance (Figure 2C).
We used CIBERSORT-ABS in TIMER2.0 to identify the tumor-infiltrating immune cell types of RR and RS patients according to a previous study (Yi et al., 2021; Supplementary Figure S1A). The proportion of the immune cells between the RR and RS groups was different, especially the B cells, monocytes, myeloid dendritic cells and eosinophils (Supplementary Figure S1B).
Validation of RT-Related Gene Modules in the TCGA-LUAD Dataset
The data of therapeutic effect on patients who accepted either RT or chemotherapy or a combination of both treatments are shown in Table 1 as the clinical case information. To identify the key modules of RT, we performed WGCNA with the data from 126 patients (Table 1). By setting the soft-thresholding power as 3 (scale-free R2 = 0.85) and the cut height as 0.25, we identified 17 modules (Figures 3A–C). From the module-trait correlation heatmap, two modules were identified with close relation to RT. The purple module was significantly correlated with RS, and the red module was significantly correlated with RR; neither module was related to chemotherapy (Figure 3D). ClueGO was used to reveal the potential biological functions and pathways of RR and RS modules. Our results demonstrate that the RS module is related to the type I interferon pathway (Figure 3E), which is in agreement with previous reports (Burnette et al., 2011; Wilkins et al., 2019). In contrast, the RR module displays a close relation with protein assembly transport (Figure 3F).
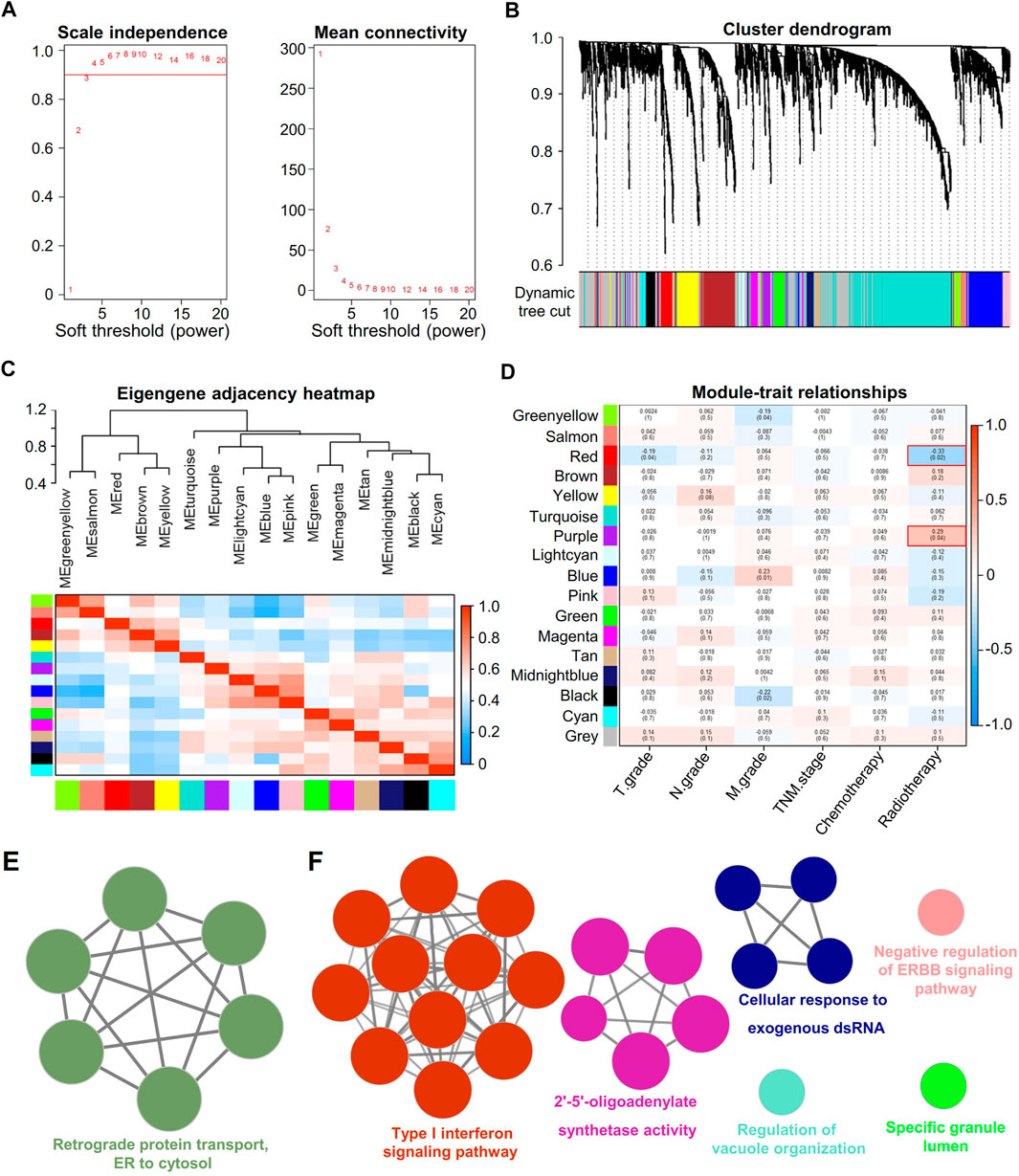
FIGURE 3. Identification of key modules correlated with clinical traits in the TCGA-LUAD dataset with WGCNA and ClueGO. (A) Soft thresholding for WGCNA analysis. (B) Clustering dendrogram of genes based on topological overlap. (C) Clustering of module eigengenes. The heatmap shows the correlation of the adjacency module. (D) Heatmap of the correlation between module eigengenes and clinical traits of RT. (E) Enrichment analysis of the red module. Each node represents an enrichment pathway. (F) Enrichment analysis of the purple module. Each node represents an enrichment pathway, and the circle color indicates different functional groups.
Identification of Hub Genes in RR and RS Modules
Genes in the RR and RS modules with kME > 0.8 are displayed in Tables 2, 3, with descending order on kME. Most of the genes in the RR module belong to the immunoglobulin family. Interestingly, some RR module genes, such as PIM2 (Guo et al., 2005) (kME = 0.953), did not belong to the immunoglobulin family but were reported to be associated with RT. Then, we selected the top two genes MZB1 (kME = 0.928) and DERL3 (kME = 0.863) other than immunoglobulin and previously reported genes as the hub genes. In the RS module, we selected hub genes with the highest kME values, including PSMD3 (kME = 0.924) and IFI35 (kME = 0.893), for further analysis.
Hub Gene Expression in Different Cells in Lung Adenocarcinoma
We performed a meta-analysis of those four genes in different datasets from the Lung Cancer Explorer, and the results are shown in Figures 4A–D. It was noted that three of the four genes, i.e., MZB1, DERL3, and PSMD3, were more highly expressed in cancer tissues than in adjacent normal lung tissues (Figures 4A–D). Then, we used PanglaoDB to explore gene expression in different cells and found that the RR module genes MZB1 and DERL3 were mainly expressed in B cells and plasma cells, while IFI35 and PSMD3 were mainly expressed in endothelial cells (Figure 4E). The results hint that the RR genes are expressed in B cells infiltrated into the TME, and the RS genes are mainly expressed in tumor tissues.
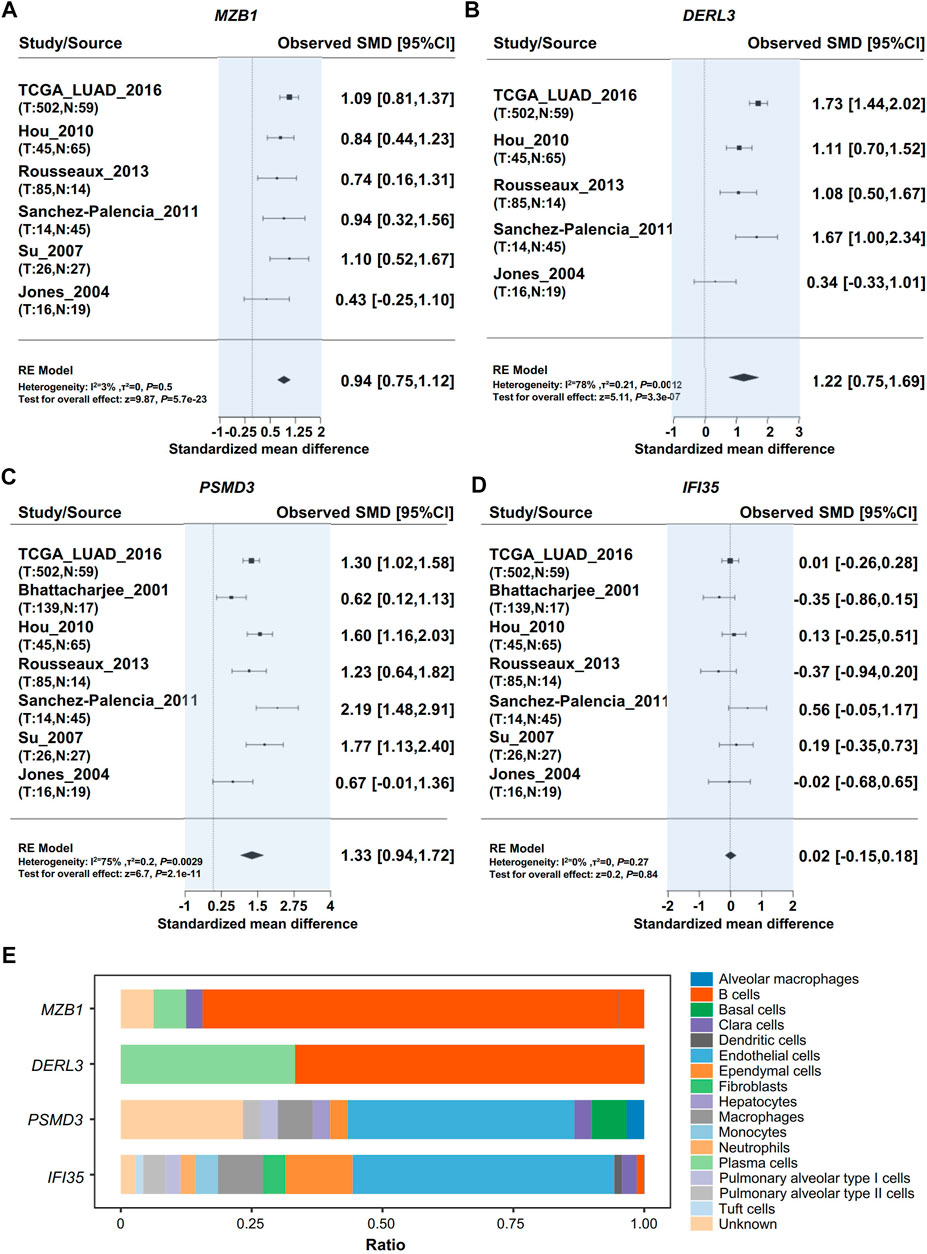
FIGURE 4. Validation of hub gene expression. Meta-analysis of Hub gene expression by using the Lung Cancer Explorer database. (A) MZB1, (B) DERL3, (C) PSMD3, (D) IFI35. (E) Analysis of cells expressing RR hub genes (MZB1, DERL3) and RS hub genes (PSMD3, IFI35) in the lung tissue with PanglaoDB.
GSVA Reveals a Close Relationship Between Hub Genes and the Immune Microenvironment
We performed GSVA to further study the potential function of these RR and RS genes in LUAD. The potential functions of the RR genes MZB1 and DERL3 were significantly enriched in terms of CD22-mediated BCR regulation and immunoglobulin receptor binding (Figures 5A,B), while the RS genes PSMD3 and IFI35 displayed a different functional category. PSMD3 shows a significant association with the proteasome accessory complex and U2 type catalytic step 2 spliceosome, and IFI35 is mainly related to the function of interferon α–β signaling and the negative regulation of type I interferon production (Figures 5C,D).
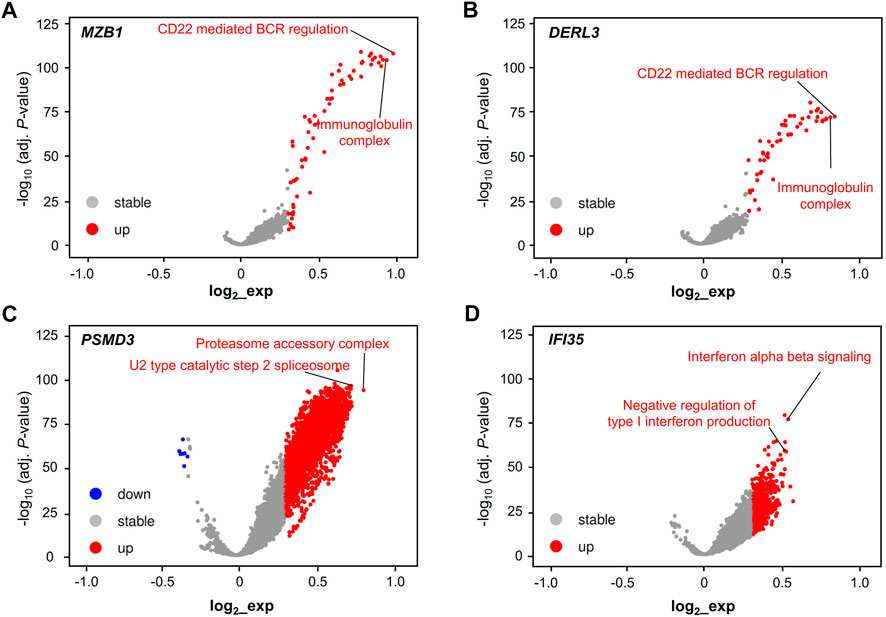
FIGURE 5. Validation of the cell type and function of hub genes. Functional volcano plot of RR hub genes MZB1 (A) and DERL3 (B) and RS hub genes PSMD3 (C) and IFI35 (D) in the TCGA-LUAD dataset. Gene set variation analysis (GSVA) and the “Limma” R package were used to find the different essential pathways of LUAD for the single hub genes, including MZB1, DERL3, PSMD3, and IFI35. Adjusted p < 0.001 and log2 (fold change) > |0.3| were regarded as statistically significant.
Correlation Between RT Hub Gene Expression and Antigen-Presenting Cells in Lung Adenocarcinoma
Tumors were engulfed in a complex microenvironment, which critically impacts disease progression and response to therapy (Quail and Joyce, 2013). We used TISIDB to explore the relationship between the expression of hub genes and the infiltration of APCs (Slatter et al., 2016). Interestingly, we found that the infiltration of APCs, as well as B cells, had a positive correlation with RR but not RS hub genes (Figure 6).
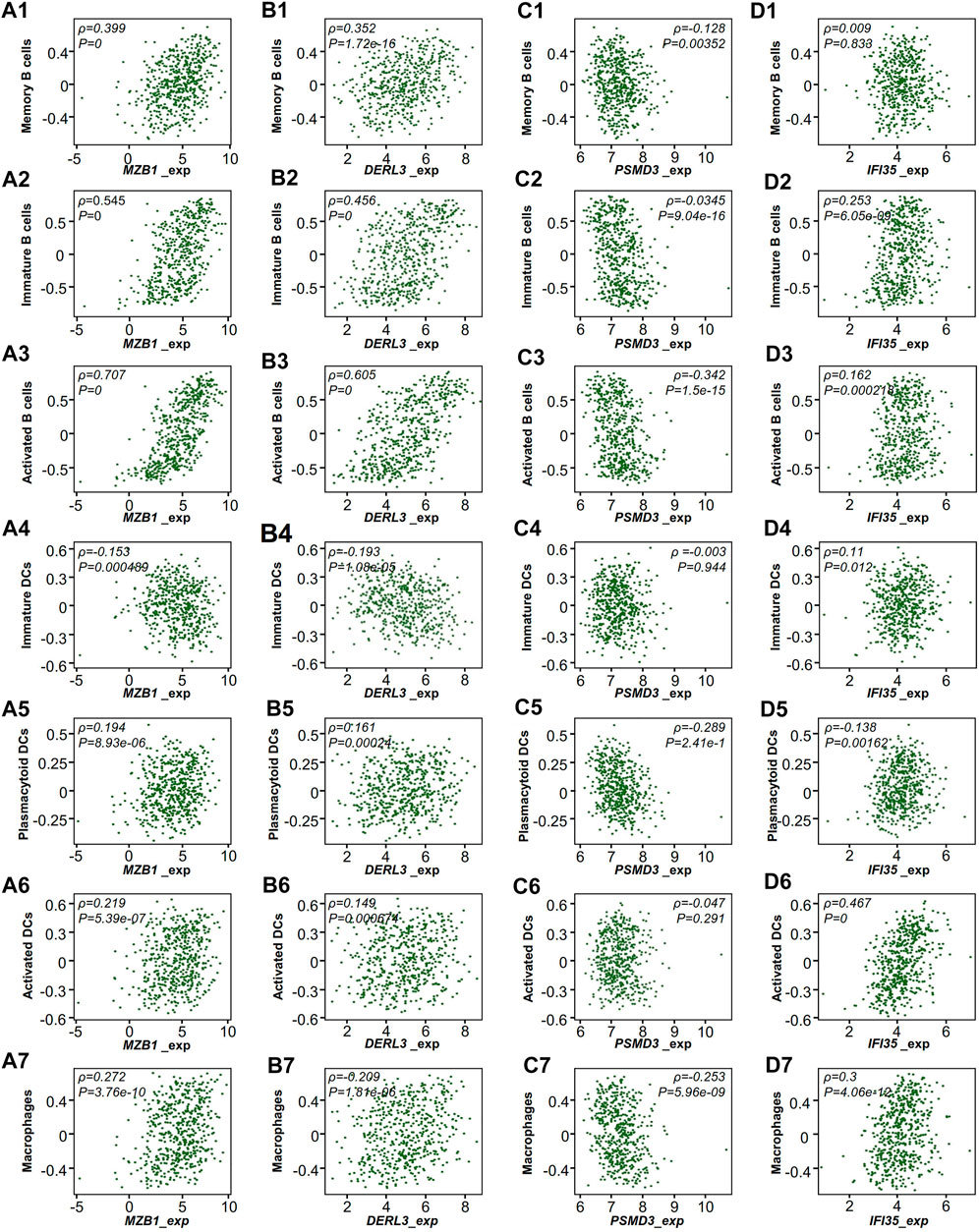
FIGURE 6. TCGA-LUAD microenvironment of RT-treated patients. Correlation of the expression of hub genes with the infiltration of immune cells from TISIDB. (A) MZB1, (B) DERL3, (C) PSMD3, (D) IFI35. Each dot represents a sample in the TCGA-LUAD dataset. ρ, Spearman correlation coefficient.
Prediction of Potential Therapy for RR Treatment
To study alternative therapy for RR patients, we used the CARE database to analyze the expression of the hub genes as well as the efficacy of drugs. Our results show that the RR hub gene expression in the databases the Broad Institute Cancer Cell Line Encyclopedia (CCLE), the Cancer Therapeutics Response Portal (CTRP) and the Genomics of Drug Sensitivity in Cancer (GDSC, also known as CGP) had a positive correlation with drug efficacy (Figures 7A,B), while the RS genes were associated with drug resistance (Figures 7C,D). Moreover, intersection was performed with the drugs positively correlated with RR genes or negatively correlated with RS genes in the CTRP and CGP databases (Figure 7E).
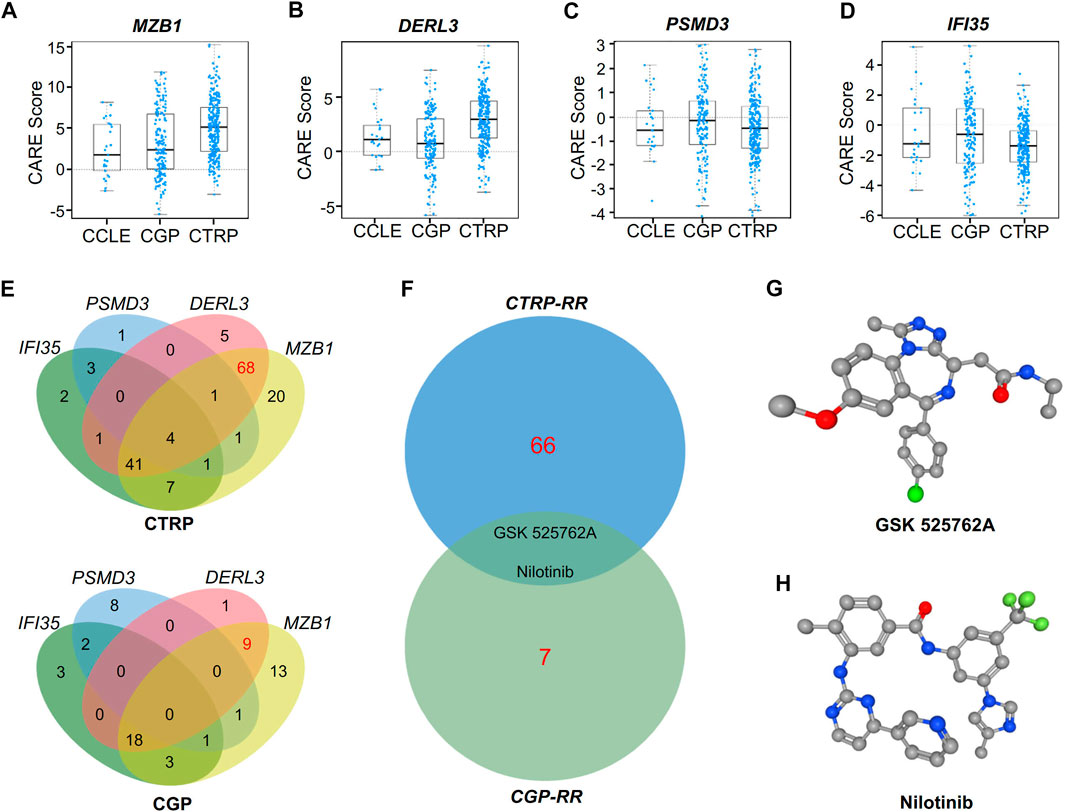
FIGURE 7. CARE analysis for hub genes in response to targeted therapy. (A–D) CARE analysis of the resistance module genes MZB1(A) and DERL3(B) and the response module genes PSMD3(C) and IFI35(D) in the databases of the Broad Institute Cancer Cell Line Encyclopedia, the CTRP and the Genomics of Drug Sensitivity in Cancer (CGP). (E) Venn diagram showing the overlap of target drugs affected by MZB1, DERL3, PSMD3, and IFI35 in the CTRP and CGP databases. (F) Venn diagram showing the potential target drugs for RR patients, i.e., GSK525762A and nilotinib. (G–H) Three-dimensional (3D) structures of GSK525762A (G) and nilotinib (H).
After exclusion of drugs targeting the RS genes, the drugs targeting both RR genes DERL3 and MZB1 in the CTRP and CGP databases were chosen for intersection analysis to find shared drugs in both databases (Figure 7F). Finally, we obtained the BRD4 inhibitor GSK525762A and the monoclonal antibody nilotinib as potential drugs for RR treatment. The three-dimensional structures of these two drugs are shown in Figures 7G,H.
Discussion
As one of mainstream cancer therapies, RT has a good effect in a variety of tumors (De Ruysscher et al., 2019; Sia et al., 2020). However, it is not so effective for several cancers, including LUAD (Li et al., 2015). In the clinic, there are many NSCLC patients who are failed to achieve a cure after surgery. In fact, 30–55% of patients with NSCLC develop recurrence and die of their disease despite curative resection (Uramoto and Tanaka, 2014). In most cases, surgery for the patients with NSCLC does not mean complete removal of cancer cells, especially for the patients received RT. RT is used as a post-surgical adjuvant therapy selectively for the patients at a risk of incomplete removal of cancer cells after surgery. Recent studies demonstrate that subsequent immune responses have a significant impact on the efficacy of RT, except for direct damage to cancer cells caused by high-energy rays (Dovedi et al., 2016; Rodriguez-Ruiz et al., 2020).
Although microarray and RNA-seq techniques have been applied in the study of LUAD, the mechanism by which RT affects the TME is still not well understood. The classical radiobiology dogma fails to consider the effect of RT on TME, however, the response of TME to RT might be significant for the success of treatment (Dai et al., 2020). To explore the factors affecting RT, we utilized GSVA to distinguish the biological functions of RR and RS tumors, indicating that the TME was involved in the RT response. Based on the association between the RT effect and the TME, we evaluated the different tumor neoantigens from RR and RS patients by GO and KEGG analysis and found that the TME significantly affected RT efficacy.
In the present study, we performed bioinformatic analysis of the gene transcriptional profiles in LUAD patients subjected to RT, which demonstrated that B cells in TME were associated with RR. B cells are the main humoral immune cells derived from hematopoietic stem cells that play an essential role in antitumor immunity. Apart from positive regulation of antitumor immune process, tumor-infiltrating B cells (TIB) can also negatively regulate antitumor immune response (Guo and Cui, 2019). In this study, we also used WGCNA to explore new hub genes associated with RT and found that the RR module was mainly composed of immunoglobulin-related genes. ClueGO analysis results demonstrated that ER to cytosol retrograde protein transport was enriched in the RR module, indicating that the RR module is involved in the term ER to cytosol retrograde protein transport. The association of TIB with RR may be related to the dysfunction of immunoglobulin generated process by B cells. In contrast, the biological function of the RS module was closely related to the type I interferon pathway, which has been reported to play an important role in the immune response of RT (Burnette et al., 2011; Feng et al., 2020). Therefore, further studies on the roles of hub genes in RR and RS modules are needed.
Several studies had reported inconsistent roles for RR hub genes, such as MZB1 and DERL3, in various malignancies. In hepatocellular carcinoma, patients with high MZB1 expression were found with a better prognosis. To the contrast of hepatocellular carcinoma, high MZB1 expression was associated with poor prognosis of patients with breast cancer. Interestingly, DERL3 expression was found to be positively correlated with MZB1 expression in the clinical specimens from breast cancer patients, suggesting DERL3 may also participate tumor progression (Watanabe et al., 2020). GSVA analysis results demonstrated that the RR genes are related to the immunoglobulin complex, indicating that they are closely related to B cells.
As for RS hub genes, high PSMD3 expression was associated with poor prognosis in the breast cancer patients (Fararjeh et al., 2019). In addition, IFI35 was reported to be involved in the regulation of radiosensitivity of colorectal cancer (CRC) cells, indicating that IFI35 might be a RT target for CRC (Hu et al., 2021). Intriguingly, the RS hub genes PSMD3 and IFI35 were found in the same GO term “positive regulation of defense response” (Subramanian et al., 2005; Watanabe et al., 2020), indicating that they are closely related to reactivity of RT.
Importantly, the GSVA result showed that the RS representative genes PSMD3 and IFI35 were associated with cellular homeostasis and immune responses, including the proteasome accessory complex and interferon α–β signaling, reflecting the characterization of tumors and immune responses induced by RT. Interestingly, we found that RS genes are mainly located in genomes 17q21 and 17q12, which indicates that the short arm of chromosome 17 contains some essential genes that influence the RT effect on LUAD.
To study alternative therapy for RR patients, we selected the efficacy of specific gene-associated drugs. We found that two RR gene-associated drugs, i.e., nilotinib and GSK525762A, were shared by CTRP and CGP databases. Intriguingly, nilotinib (Sun et al., 2019) and GSK525762A (Klingbeil et al., 2016; Zhang et al., 2018) have been reported to inhibit LUAD cell proliferation or growth. Our study provides drug candidates for the therapy of LUAD patients with RR.
In general, by integrating GSVA, WGCNA and other immune microenvironment databases, we found that the RR of LUAD might be associated with the infiltration of immune cells, especially B cells, which was in line with the finding that B cells are a key factor promoting carcinogenesis by immunosuppression (Schioppa et al., 2011; Klinker and Lundy, 2012). It is reasonable to conclude that the composition of the TME may have a significant impact on the treatment of LUAD. Furthermore, our novel findings on the expression of the hub genes and the efficacy of specific gene-associated drugs provide potential drug candidates for RR patients. However, more studies are required to reveal the effectiveness of the drugs in the treatment of LUAD.
Data Availability Statement
The original contributions presented in the study are included in the article/Supplementary Material, further inquiries can be directed to the corresponding authors.
Author Contributions
JW, QH, and YJ conceived and designed research. JW and QH analyzed data. JW, LL, and AL interpreted results. JW and HZL prepared figures. JW and QH drafted manuscript. YJ and HHL edited and revised manuscript. JW, QH, HZL, HHL, LL, AL, and YJ approved final version of manuscript.
Funding
This study was supported by the NSFC-Guangdong Joint Foundation of China (No. U1601225), Natural Science Foundation of China (No. 81971895), Guangdong Provincial Science and Technology Projects (No. 2016A020216015) and Special Support Plan for Outstanding Talents of Guangdong Province (2019JC05Y340).
Conflict of Interest
The authors declare that the research was conducted in the absence of any commercial or financial relationships that could be construed as a potential conflict of interest.
Acknowledgments
We appreciate Jie-Rong Chen and Shuai He for assistance with the bioinformatics.
Supplementary Material
The Supplementary Material for this article can be found online at: https://www.frontiersin.org/articles/10.3389/fmolb.2021.624575/full#supplementary-material
References
Bardou, P., Mariette, J., Escudié, F., Djemiel, C., and Klopp, C. (2014). Jvenn: an Interactive Venn Diagram Viewer. BMC Bioinformatics 15, 293. doi:10.1186/1471-2105-15-293
Barr Kumarakulasinghe, N., Zanwijk, N. v., and Soo, R. A. (2015). Molecular Targeted Therapy in the Treatment of Advanced Stage Non-small Cell Lung Cancer (NSCLC). Respirology 20, 370–378. doi:10.1111/resp.12490
Bian, L., Meng, Y., Zhang, M., Guo, Z., Liu, F., Zhang, W., et al. (2020). ATM Expression Is Elevated in Established Radiation-Resistant Breast Cancer Cells and Improves DNA Repair Efficiency. Int. J. Biol. Sci. 16, 1096–1106. doi:10.7150/ijbs.41246
Bindea, G., Mlecnik, B., Hackl, H., Charoentong, P., Tosolini, M., Kirilovsky, A., et al. (2009). ClueGO: a Cytoscape Plug-In to Decipher Functionally Grouped Gene Ontology and Pathway Annotation Networks. Bioinformatics 25, 1091–1093. doi:10.1093/bioinformatics/btp101
Burnette, B. C., Liang, H., Lee, Y., Chlewicki, L., Khodarev, N. N., Weichselbaum, R. R., et al. (2011). The Efficacy of Radiotherapy Relies Upon Induction of Type I Interferon-Dependent Innate and Adaptive Immunity. Cancer Res. 71, 2488–2496. doi:10.1158/0008-5472.can-10-2820
Cai, L., Lin, S., Girard, L., Zhou, Y., Yang, L., Ci, B., et al. (2019). LCE: an Open Web Portal to Explore Gene Expression and Clinical Associations in Lung Cancer. Oncogene 38, 2551–2564. doi:10.1038/s41388-018-0588-2
Cancer Genome Atlas Research Network (2014). Comprehensive Molecular Profiling of Lung Adenocarcinoma. Nature 511, 543–550. doi:10.1038/nature13385
Charoentong, P., Finotello, F., Angelova, M., Mayer, C., Efremova, M., Rieder, D., et al. (2017). Pan-Cancer Immunogenomic Analyses Reveal Genotype-Immunophenotype Relationships and Predictors of Response to Checkpoint Blockade. Cel Rep. 18, 248–262. doi:10.1016/j.celrep.2016.12.019
Chen, W., Dai, X., Chen, Y., Tian, F., Zhang, Y., Zhang, Q., et al. (2020). Significance of STAT3 in Immune Infiltration and Drug Response in Cancer. Biomolecules 10(6):834. doi:10.3390/biom10060834
Dai, Y., Zhang, Y., Yang, M., Zhou, L., Pan, H., Xiao, T., et al. (2020). Radiosensitivity-Related Genes and Clinical Characteristics of Nasopharyngeal Carcinoma. Biomed. Res. Int. 2020, 1705867. doi:10.1155/2020/1705867
De Ruysscher, D., Niedermann, G., Burnet, N. G., Siva, S., Lee, A. W. M., and Hegi-Johnson, F. (2019). Radiotherapy Toxicity. Nat. Rev. Dis. Primers 5, 13. doi:10.1038/s41572-019-0064-5
Deberardinis, R. J. (2020). Tumor Microenvironment, Metabolism, and Immunotherapy. N. Engl. J. Med. 382, 869–871. doi:10.1056/nejmcibr1914890
Doncheva, N. T., Morris, J. H., Gorodkin, J., and Jensen, L. J. (2019). Cytoscape StringApp: Network Analysis and Visualization of Proteomics Data. J. Proteome Res. 18, 623–632. doi:10.1021/acs.jproteome.8b00702
Dovedi, S. J., Lipowska-Bhalla, G., Beers, S. A., Cheadle, E. J., Mu, L., Glennie, M. J., et al. (2016). Antitumor Efficacy of Radiation Plus Immunotherapy Depends Upon Dendritic Cell Activation of Effector CD8+ T Cells. Cancer Immunol. Res. 4, 621–630. doi:10.1158/2326-6066.cir-15-0253
Fararjeh, A. S., Chen, L. C., Ho, Y. S., Cheng, T. C., Liu, Y. R., Chang, H. L., et al. (2019). Proteasome 26S Subunit, Non-ATPase 3 (PSMD3) Regulates Breast Cancer by Stabilizing HER2 From Degradation. Cancers (Basel) 11(4):527. doi:10.3390/cancers11040527
Faruki, H., Mayhew, G. M., Serody, J. S., Hayes, D. N., Perou, C. M., and Lai-Goldman, M. (2017). Lung Adenocarcinoma and Squamous Cell Carcinoma Gene Expression Subtypes Demonstrate Significant Differences in Tumor Immune Landscape. J. Thorac. Oncol. 12, 943–953. doi:10.1016/j.jtho.2017.03.010
Feng, X., Tubbs, A., Zhang, C., Tang, M., Sridharan, S., Wang, C., et al. (2020). ATR Inhibition Potentiates Ionizing Radiation-Induced Interferon Response via Cytosolic Nucleic Acid-Sensing Pathways. EMBO J. 39, e104036. doi:10.15252/embj.2019104036
Franzen, O., Gan, L. M., and Bjorkegren, J. L. M. (2019). PanglaoDB: A Web Server for Exploration of Mouse and Human Single-Cell RNA Sequencing Data. Oxford: Database
Girard, L., Rodriguez-Canales, J., Behrens, C., Thompson, D. M., Botros, I. W., Tang, H., et al. (2016). An Expression Signature as an Aid to the Histologic Classification of Non-small Cell Lung Cancer. Clin. Cancer Res. 22, 4880–4889. doi:10.1158/1078-0432.ccr-15-2900
Gu, C., Luo, J., Lu, X., Tang, Y., Ma, Y., Yun, Y., et al. (2019). REV7 Confers Radioresistance of Esophagus Squamous Cell Carcinoma by Recruiting PRDX2. Cancer Sci. 110, 962–972. doi:10.1111/cas.13946
Guo, F. F., and Cui, J. W. (2019). The Role of Tumor-Infiltrating B Cells in Tumor Immunity. J. Oncol. 2019, 2592419. doi:10.1155/2019/2592419
Guo, W.-F., Lin, R.-X., Huang, J., Zhou, Z., Yang, J., Guo, G.-Z., et al. (2005). Identification of Differentially Expressed Genes Contributing to Radioresistance in Lung Cancer Cells Using Microarray Analysis. Radiat. Res. 164, 27–35. doi:10.1667/rr3401
Haeger, A., Alexander, S., Vullings, M., Kaiser, F. M. P., Veelken, C., Flucke, U., et al. (2020). Collective Cancer Invasion Forms an Integrin-dependent Radioresistant Niche. J. Exp. Med. 217(1):e20181184. doi:10.1084/jem.20181184
Hänzelmann, S., Castelo, R., and Guinney, J. (2013). GSVA: Gene Set Variation Analysis for Microarray and RNA-Seq Data. BMC Bioinformatics 14, 7. doi:10.1186/1471-2105-14-7
Hu, Y., Wang, B., Yi, K., Lei, Q., Wang, G., and Xu, X. (2021). IFI35 Is Involved in the Regulation of the Radiosensitivity of Colorectal Cancer Cells. PREPRINT, (Version 1). doi:10.21203/rs.3.rs-459951/v1
Jiang, P., Lee, W., Li, X., Johnson, C., Liu, J. S., Brown, M., et al. (2018). Genome-Scale Signatures of Gene Interaction from Compound Screens Predict Clinical Efficacy of Targeted Cancer Therapies. Cel Syst. 6, 343–354. doi:10.1016/j.cels.2018.01.009
Klingbeil, O., Lesche, R., Gelato, K. A., Haendler, B., and Lejeune, P. (2016). Inhibition of BET Bromodomain-dependent XIAP and FLIP Expression Sensitizes KRAS-Mutated NSCLC to Pro-apoptotic Agents. Cell Death Dis 7, e2365. doi:10.1038/cddis.2016.271
Klinker, M. W., and Lundy, S. K. (2012). Multiple Mechanisms of Immune Suppression by B Lymphocytes. Mol. Med. 18, 123–137. doi:10.2119/molmed.2011.00333
Kolde, R. (2019). pheatmap: Pretty Heatmaps. R Package Version 1.0.12. https://CRAN.Rproject.org/package=pheatmap
Langfelder, P., and Horvath, S. (2008). WGCNA: an R Package for Weighted Correlation Network Analysis. BMC Bioinformatics 9, 559. doi:10.1186/1471-2105-9-559
Li, B., Severson, E., Pignon, J.-C., Zhao, H., Li, T., Novak, J., et al. (2016). Comprehensive Analyses of Tumor Immunity: Implications for Cancer Immunotherapy. Genome Biol. 17, 174. doi:10.1186/s13059-016-1028-7
Li, Y., Li, H., Peng, W., He, X.-Y., Huang, M., Qiu, D., et al. (2015). DNA-dependent Protein Kinase Catalytic Subunit Inhibitor Reverses Acquired Radioresistance in Lung Adenocarcinoma by Suppressing DNA Repair. Mol. Med. Rep. 12, 1328–1334. doi:10.3892/mmr.2015.3505
Liberzon, A., Birger, C., Thorvaldsdóttir, H., Ghandi, M., Mesirov, J. P., and Tamayo, P. (2015). The Molecular Signatures Database Hallmark Gene Set Collection. Cel Syst. 1, 417–425. doi:10.1016/j.cels.2015.12.004
Liu, Z.-L., Liu, X., Peng, H., Peng, Z.-W., Long, J.-T., Tang, D., et al. (2020). Anti-PD-1 Immunotherapy and Radiotherapy for Stage IV Intrahepatic Cholangiocarcinoma: A Case Report. Front. Med. 7, 368. doi:10.3389/fmed.2020.00368
Ljungman, M. (2009). Targeting the DNA Damage Response in Cancer. Chem. Rev. 109, 2929–2950. doi:10.1021/cr900047g
Menzel, J., and Black, J. C. (2020). Epigenetic Modulation of the Tumor Immune Microenvironment to Potentiate Immune Checkpoint Blockade Therapy. Cancer Discov. 10, 179–181. doi:10.1158/2159-8290.cd-19-1349
Newman, A. M., Liu, C. L., Green, M. R., Gentles, A. J., Feng, W., Xu, Y., et al. (2015). Robust Enumeration of Cell Subsets from Tissue Expression Profiles. Nat. Methods 12, 453–457. doi:10.1038/nmeth.3337
Price, A. (2012). Emerging Developments of Chemoradiotherapy in Stage III NSCLC. Nat. Rev. Clin. Oncol. 9, 591–598. doi:10.1038/nrclinonc.2012.135
Quail, D. F., and Joyce, J. A. (2013). Microenvironmental Regulation of Tumor Progression and Metastasis. Nat. Med. 19, 1423–1437. doi:10.1038/nm.3394
Rigden, D. J., and Fernández, X. M. (2020). The 27th Annual Nucleic Acids Research Database Issue and Molecular Biology Database Collection. Nucleic Acids Res. 48, D1–D8. doi:10.1093/nar/gkz1161
Ritchie, M. E., Phipson, B., Wu, D., Hu, Y., Law, C. W., Shi, W., et al. (2015). Limma powers Differential Expression Analyses for RNA-Sequencing and Microarray Studies. Nucleic Acids Res. 43, e47. doi:10.1093/nar/gkv007
Rodriguez-Ruiz, M. E., Vitale, I., Harrington, K. J., Melero, I., and Galluzzi, L. (2020). Immunological Impact of Cell Death Signaling Driven by Radiation on the Tumor Microenvironment. Nat. Immunol. 21, 120–134. doi:10.1038/s41590-019-0561-4
Ru, B., Wong, C. N., Tong, Y., Zhong, J. Y., Zhong, S. S. W., Wu, W. C., et al. (2019). TISIDB: an Integrated Repository portal for Tumor-Immune System Interactions. Bioinformatics 35, 4200–4202. doi:10.1093/bioinformatics/btz210
Schioppa, T., Moore, R., Thompson, R. G., Rosser, E. C., Kulbe, H., Nedospasov, S., et al. (2011). B Regulatory Cells and the Tumor-Promoting Actions of TNF- during Squamous Carcinogenesis. Proc. Natl. Acad. Sci. 108, 10662–10667. doi:10.1073/pnas.1100994108
Sia, J., Szmyd, R., Hau, E., and Gee, H. E. (2020). Molecular Mechanisms of Radiation-Induced Cancer Cell Death: A Primer. Front. Cel Dev. Biol. 8, 41. doi:10.3389/fcell.2020.00041
Slatter, T. L., Wilson, M., Tang, C., Campbell, H. G., Ward, V. K., Young, V. L., et al. (2016). Antitumor Cytotoxicity Induced by Bone-Marrow-Derived Antigen-Presenting Cells Is Facilitated by the Tumor Suppressor Protein P53 via Regulation of IL-12. Oncoimmunology 5, e1112941. doi:10.1080/2162402x.2015.1112941
Sturm, G., Finotello, F., Petitprez, F., Zhang, J. D., Baumbach, J., Fridman, W. H., et al. (2019). Comprehensive Evaluation of Transcriptome-Based Cell-type Quantification Methods for Immuno-Oncology. Bioinformatics 35, i436–i445. doi:10.1093/bioinformatics/btz363
Subramanian, A., Tamayo, P., Mootha, V. K., Mukherjee, S., Ebert, B. L., Gillette, M. A., et al. (2005). Gene Set Enrichment Analysis: a Knowledge-Based Approach for Interpreting Genome-wide Expression Profiles. Proc. Natl. Acad. Sci. 102, 15545–15550. doi:10.1073/pnas.0506580102
Sun, R., Bao, M. Y., Long, X., Yuan, Y., Wu, M. M., Li, X., et al. (2019). Metabolic geneNR4A1as a Potential Therapeutic Target for Non‐smoking Female Non‐small Cell Lung Cancer Patients. Thorac. Cancer 10, 715–727. doi:10.1111/1759-7714.12989
Uramoto, H., and Tanaka, F. (2014). Recurrence after Surgery in Patients with NSCLC. Transl Lung Cancer Res. 3, 242–249. doi:10.3978/j.issn.2218-6751.2013.12.05
Wallace, J. A., Li, F., Balakrishnan, S., Cantemir-Stone, C. Z., Pecot, T., Martin, C., et al. (2013). Ets2 in Tumor Fibroblasts Promotes Angiogenesis in Breast Cancer. PLoS One 8, e71533. doi:10.1371/journal.pone.0071533
Watanabe, M., Shibata, M., Inaishi, T., Ichikawa, T., Soeda, I., Miyajima, N., et al. (2020). MZB1 Expression Indicates Poor Prognosis in Estrogen Receptor-Positive Breast Cancer. Oncol. Lett. 20, 198. doi:10.3892/ol.2020.12059
Wilkins, A. C., Patin, E. C., Harrington, K. J., and Melcher, A. A. (2019). The Immunological Consequences of Radiation‐induced DNA Damage. J. Pathol. 247, 606–614. doi:10.1002/path.5232
Xiao, Z., Dai, Z., and Locasale, J. W. (2019). Metabolic Landscape of the Tumor Microenvironment at Single Cell Resolution. Nat. Commun. 10, 3763. doi:10.1038/s41467-019-11738-0
Yang, T., Huang, T., Zhang, D., Wang, M., Wu, B., Shang, Y., et al. (2019). TGF-β Receptor Inhibitor LY2109761 Enhances the Radiosensitivity of Gastric Cancer by Inactivating the TGF-Β/smad4 Signaling Pathway. Aging 11, 8892–8910. doi:10.18632/aging.102329
Yi, M., Li, A., Zhou, L., Chu, Q., Luo, S., and Wu, K. (2021). Immune Signature-Based Risk Stratification and Prediction of Immune Checkpoint Inhibitor's Efficacy for Lung Adenocarcinoma. Cancer Immunol. Immunother. 70(6), 1705-1719. doi:10.1007/s00262-020-02817-z
Yu, G. (2018). Enrichplot: Visualization of Functional Enrichment Result. R package version 1.0.2. https://github.com/GuangchuangYu/enrichplot
Yu, G., Wang, L.-G., Han, Y., and He, Q.-Y. (2012). clusterProfiler: an R Package for Comparing Biological Themes Among Gene Clusters. OMICS: A J. Integr. Biol. 16, 284–287. doi:10.1089/omi.2011.0118
Keywords: lung adenocarcinoma, radioresistance, immune cell infiltration, bioinformatics, drug candidate
Citation: Wang J, Han Q, Liu H, Luo H, Li L, Liu A and Jiang Y (2021) Identification of Radiotherapy-Associated Genes in Lung Adenocarcinoma by an Integrated Bioinformatics Analysis Approach. Front. Mol. Biosci. 8:624575. doi: 10.3389/fmolb.2021.624575
Received: 04 November 2020; Accepted: 31 May 2021;
Published: 15 June 2021.
Edited by:
Kamran Ghaedi, University of Isfahan, IranReviewed by:
Fei Han, Army Medical University, ChinaMing Yi, Huazhong University of Science and Technology, China
Qingzhu Jia, Army Medical University, China
Copyright © 2021 Wang, Han, Liu, Luo, Li, Liu and Jiang. This is an open-access article distributed under the terms of the Creative Commons Attribution License (CC BY). The use, distribution or reproduction in other forums is permitted, provided the original author(s) and the copyright owner(s) are credited and that the original publication in this journal is cited, in accordance with accepted academic practice. No use, distribution or reproduction is permitted which does not comply with these terms.
*Correspondence: Aihua Liu, bGFoNDcxNThAMTYzLmNvbQ==; Yong Jiang, amlhbmc0ODIzMUAxNjMuY29t
†These authors have contributed equally to this work