- 1Department of Pathology, Affiliated Hangzhou First People’s Hospital, Zhejiang University School of Medicine, Hangzhou, China
- 2Department of Surgery, The Second Affiliated Hospital, Zhejiang University School of Medicine, Hangzhou, China
We aimed to explore the tumor mutational burden (TMB) and immune infiltration in HCC and investigate new biomarkers for immunotherapy. Transcriptome and gene mutation data were downloaded from the GDC portal, including 374 HCC samples and 50 matched normal samples. Furthermore, we divided the samples into high and low TMB groups, and analyzed the differential genes between them with GO, KEGG, and GSEA. Cibersort was used to assess the immune cell infiltration in the samples. Finally, univariate and multivariate Cox regression analyses were performed to identify differential genes related to TMB and immune infiltration, and a risk prediction model was constructed. We found 10 frequently mutated genes, including TP53, TTN, CTNNB1, MUC16, ALB, PCLO, MUC, APOB, RYR2, and ABCA. Pathway analysis indicated that these TMB-related differential genes were mainly enriched in PI3K-AKT. Cibersort analysis showed that memory B cells (p = 0.02), CD8+ T cells (p = 0.09), CD4+ memory activated T cells (p = 0.07), and neutrophils (p = 0.06) demonstrated a difference in immune infiltration between high and low TMB groups. On multivariate analysis, GABRA3 (p = 0.05), CECR7 (p < 0.001), TRIM16 (p = 0.003), and IL7R (p = 0.04) were associated with TMB and immune infiltration. The risk prediction model had an area under the curve (AUC) of 0.69, suggesting that patients with low risk had better survival outcomes. Our study demonstrated for the first time that CECR7, GABRA3, IL7R, and TRIM16L were associated with TMB and promoted antitumor immunity in HCC.
Introduction
Hepatocellular carcinoma (HCC) is the seventh most common cancer worldwide and the fourth leading cause of cancer-related deaths (Yang et al., 2019). Despite the efforts made by the developing countries in the past few years, 80% of HCC cases are from these countries (Yang et al., 2019). HCC is the main type of primary liver cancer, accounting for more than 80% of the cases (El-Serag and Rudolph, 2007). In America, the incidence of HCC increased in each consecutive birth cohort through 1959, predominantly in Asian Americans. The incidence of HCC dropped significantly from 1960 to 1969 birth cohorts, and it was expected that the incidence would drop further from 2013 to 2020, with about 1.59% decline in men and 2.20% decline in women. However, the epidemiological data based on the population cancer register show that the incidence of HCC has increased 4-fold in the past 40 years, and this increase in the incidence will continue in the future (Petrick et al., 2016; Massarweh and El-Serag, 2017; Singal et al., 2020). The common treatment methods for HCC include surgery, radiotherapy, chemotherapy, and immunotherapy. Despite the various treatment methods, the overall survival rate of HCC is low and the mortality rate is high. The 10-year survival rate is approximately 10%, and it is accompanied by high recurrence and metastasis (Siegel et al., 2016).
In recent years, immunotherapy has become a common treatment for metastatic and aggressive tumors (Thyer et al., 2013; Tian et al., 2019). Tumor immunotherapy principally refers to a treatment that uses the body’s own immune system to attack the cancer cells. Immunotherapy mainly covers tumor vaccines, biological therapy, CAR-T cells, and immune checkpoint inhibitors (PD-1, CTLA-4) (Prieto et al., 2015). Kim et al. reported that inhibiting PD-1/PD-L1 increased the infiltration of CD8+ T cells resulting in the killing of the tumor cells (Kim et al., 2018). PD-1 checkpoint inhibitors such as atezolizumab and nivolumab have been shown to significantly improve the clinical symptoms of patients and prolong survival time in non-small cell lung cancer (Chardin et al., 2020), renal cell carcinoma (Buchbinder et al., 2019), breast cancer (Szekely et al., 2018), and melanoma (Luke et al., 2017). However, Chiu’s study demonstrated that upregulation of PVRL1 inhibited the cytotoxic T cell response through the T-cell immunoglobulin and ITIM domain (TIGIT), thus mediating resistance to PD1 inhibitors in HCC (Chiu et al., 2020). Immunotherapy is only effective in about one-fifth of the patients, and most patients are unable to benefit from it.
Tumor mutation burden (TMB) is a recent biomarker that is used to predict the effect of immunotherapy. This refers to the total number of mutations per megabase in the genome. Generally speaking, the higher the TMB, the greater the difference in the tumor tissue, and the higher the patients benefit from immunotherapy (Chan et al., 2019). Previous studies have indicated that a high TMB predicts a better prognosis in melanoma and non-small cell lung cancer (Chen et al., 2019a; Chen et al., 2019b). However, research on TMB and immune infiltration in hepatocellular carcinoma remains inconclusive.
In this study, we collected somatic mutation data and transcriptome data from The Cancer Genome Atlas (TCGA) database. We aimed to explore the TMB combined with immune infiltrates in HCC. Our study demonstrated that CECR7, GABRA3, IL7R, and TRIM16L were associated with TMB and promoted antitumor immunity in HCC.
Materials and Methods
Data Acquisition and Processing
Transcriptome data, somatic mutation data, and clinical information of 374 HCC samples and 50 matched normal or adjacent tissue samples were obtained from the GDC portal (https://portal.gdc.cancer.gov/). In addition, the clinical data of HCC patients (n = 376) comprising age, sex, survival time, survival status, grade, stage, and the American Joint Committee on Cancer (AJCC-TNM) staging were collected (Table 1).
Classification of Clinical Data Based on the Tumor Mutation Burden
We calculated TMB by the total number of mutations per megabase of the genome. We processed the TMB and raw transcriptome data obtained from the GDC portal using the R software (https://www.r-project.org/) and Excel “vlookup” to obtain the TMB scores of each sample. According to the median of the TMB scores (median = 2.45), we divided the HCC samples into high and low TMB groups. In addition, We analyzed the relationship between TMB scores and clinical characteristics (Supplementary Figure S1).
Multi-Omics Analysis
The somatic mutation data processed by Varscan were downloaded from TCGA, and the “maftools” package (http://bioconductor.org/) was used to visualize the TMB information (Koboldt et al., 2012). Based on the Java working environment, strawberry-perl (https://www.perl.org/) was used to run the perl script to extract the genome mutation information of the 364 HCC patients.
Using the R language, we merged the mutation information of HCC patients with the clinical survival data. According to the median value of the TMB scores, samples were classified into high and low TMB groups. Gene ontology (GO) and gene set enrichment analysis (GSEA) were performed as previously described (Subramanian et al., 2005). A p-value < 0.05 and
Uni and multivariate Cox regression analyses were conducted to assess the immune-related genes. Based on the results of the multivariate regression analysis, we constructed a risk prediction model and divided the patients into high-risk and low-risk groups according to the risk scores (TMBRS). A receiver operating characteristic (ROC) curve was used to test the sensitivity and specificity of this model. Finally, an analysis of immune cell infiltration with survival outcomes was conducted using the Kaplan–Meier method.
Statistical Analysis
All statistical analyses were performed using R, including the Kaplan-Meier survival analysis and uni- and multivariate Cox regression analyses. For all comparisons, p < 0.05 was considered to indicate statistical significance.
Results
Landscape of Frequent Gene Mutations in Hepatocellular Carcinoma
We obtained the mutation data of 364 HCC samples processed by “Varsan” from the GDC portal and the “maftools” package was used to visualize the data. Among somatic mutations, missense mutations were the most common type (Figure 1A), single nucleotide polymorphisms and C > T mutations were the most common (Figures 1B,C). The tumor mutation burden of each sample was shown using various colors in box plots (Figures 1D,E). A waterfall plot displays the comprehensive mutation data of each sample . In addition, we selected the 10 most frequently mutated genes in the American HCC’s samples obtained from the TCGA cohort, including TP53 (30%), TTN (24%), CTNNB1 (25%), MUC16 (14%), ALB (13%), PCLO (10%), MUC (9%), APOB (9%), RYR2 (9%), and ABCA (8%) (Figures 1F,G). Furthermore, we performed co-expression analysis of these mutant genes (Figure 1H).
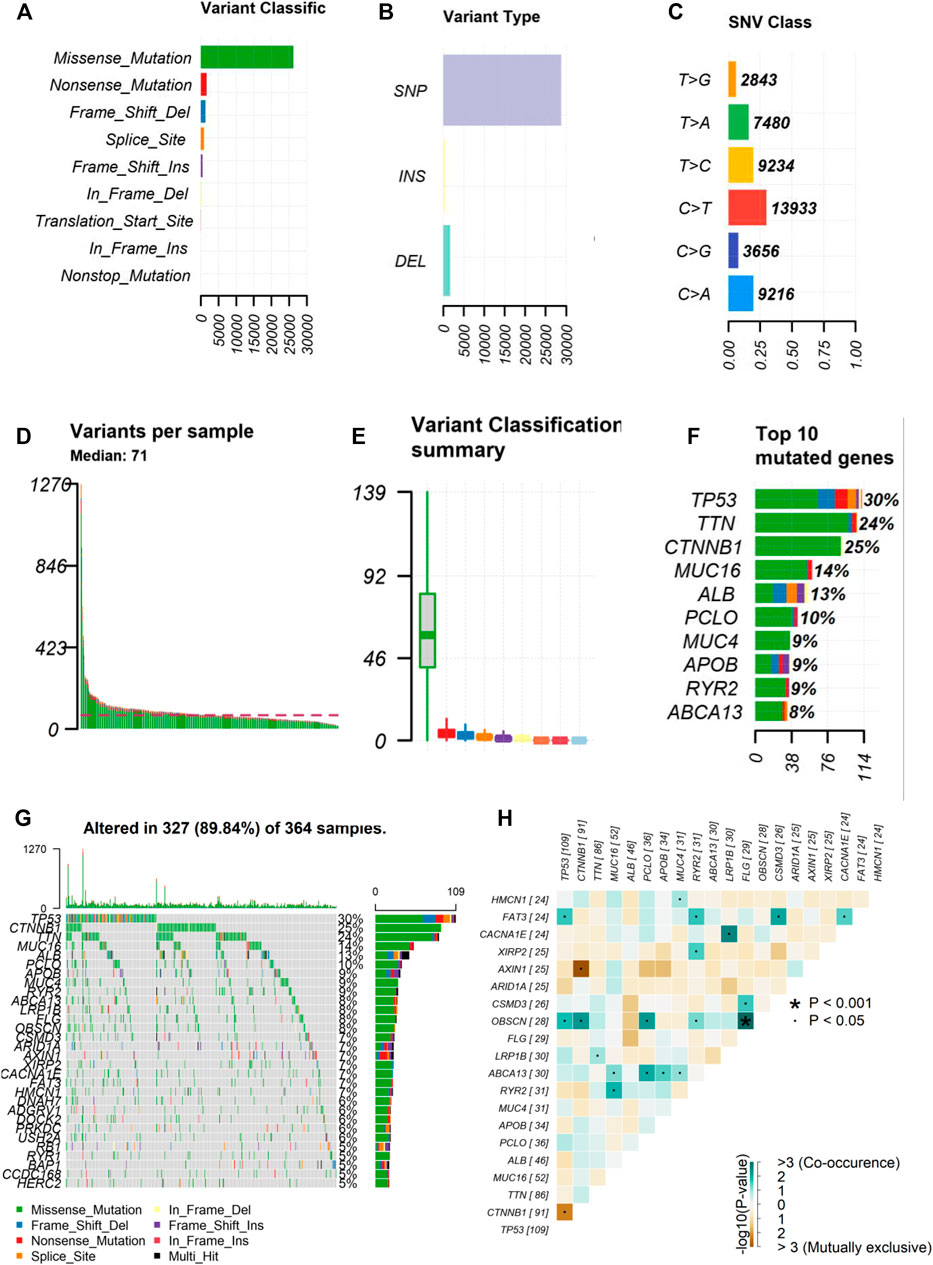
FIGURE 1. Landscape of frequently gene mutation in HCC. (A–C) Statistical calculations of mutation types based on different categories, where missense mutation, SNP, C > T mutation were the most (D,E) Display of TMB in each HCC sample (F) The top 10 mutant genes in HCC, including TP53, TTN, CTNNB1, MUC16, ALB, PCLO, MUC, APOB, RYR2, ABCA. (G) Landscape of mutation information of each HCC sample in waterfall plot. Each column represents a sample (H) Co-expression of mutant genes in HCC. HCC, hepatocellular carcinoma; SNP, single nucleotide polymorphism. *p < 0.001, ˙p < 0.05.
Gene Ontology and KEGG Analysis of Gene Expression in the Two TMB Groups
Differentially expressed genes were obtained through R software (Supplementary Figure S2). The “Limma” package was used to analyze the differentially expressed genes in the two TMB groups, and 375 differentially expressed genes were obtained. Differential genes were identified by log (fold change) > 1 and p < 0.05. For the biological processes, we used Gene Ontology to enrich the differential genes, including biological process (BP), cellular component (CC), and molecular function (MF). With regard to BP, these differential genes were mainly enriched in the extracellular matrix organization. With regard to CC and MF, the differential genes were predominantly enriched in the collagen-containing extracellular matrix and matrix structural constituent, respectively (Figures 2A,B). Furthermore, we used KEGG to conduct pathway analysis on these differentially expressed genes, and found that the TMB-related differential genes were mainly enriched in the PI3K-Akt signaling pathway, a pathway that has been clearly reported to be related to cancer (Revathidevi and Munirajan, 2019). In addition, the KEGG analysis revealed that they were also significantly enriched in cytokine-cytokine receptor interaction and focal adhesion pathway (Figures 2C,D; Table 2).
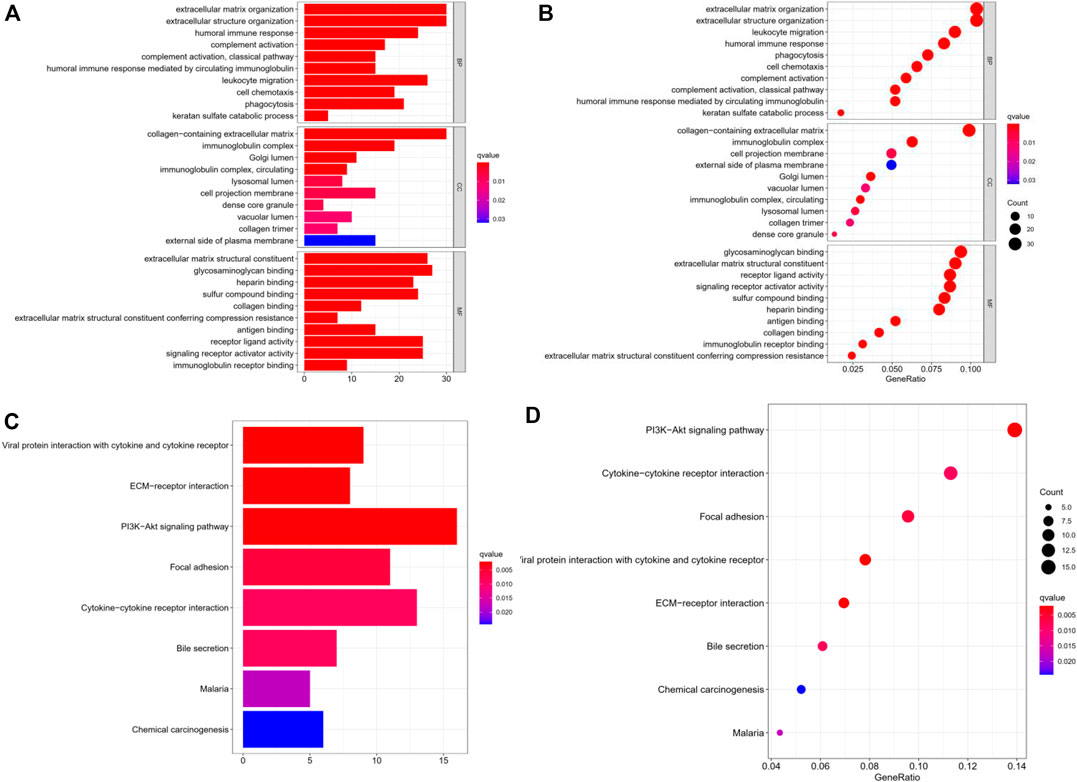
FIGURE 2. Gene ontology and KEGG analysis of the gene expression of the two TMB groups. (A,B) GO enriched analysis of differential genes in BP, CC, MP. (C,D) KEGG analysis with these differential genes were enriched in PI3K-AKt, cytokine-cytokine receptor interaction and focal adhesion axis. TMB: tumor mutation burden; BP: biological process; CC, cellular component; MP, molecular function; KEGG, kyoto encyclopedia of genes and genomes.
Gene Set Enrichment Analysis with High and Low TMB Groups
GSEA was used to analyze the TCGA cohort. We found that in the high TMB group, the differentially expressed genes were mainly enriched in the proteasome, drug metabolism other enzymes, porphyrin, and chlorophyll metabolism (Figures 3A–C). In the low TMB group, the differentially expressed genes chiefly participated in ECM receptor interaction, vascular smooth muscle contraction, and ether lipid metabolism (Figures 3D–F). The top 10 differentially expressed GSEA outcomes in the high and low TMB groups are shown in Supplementary Tables S1, S2.
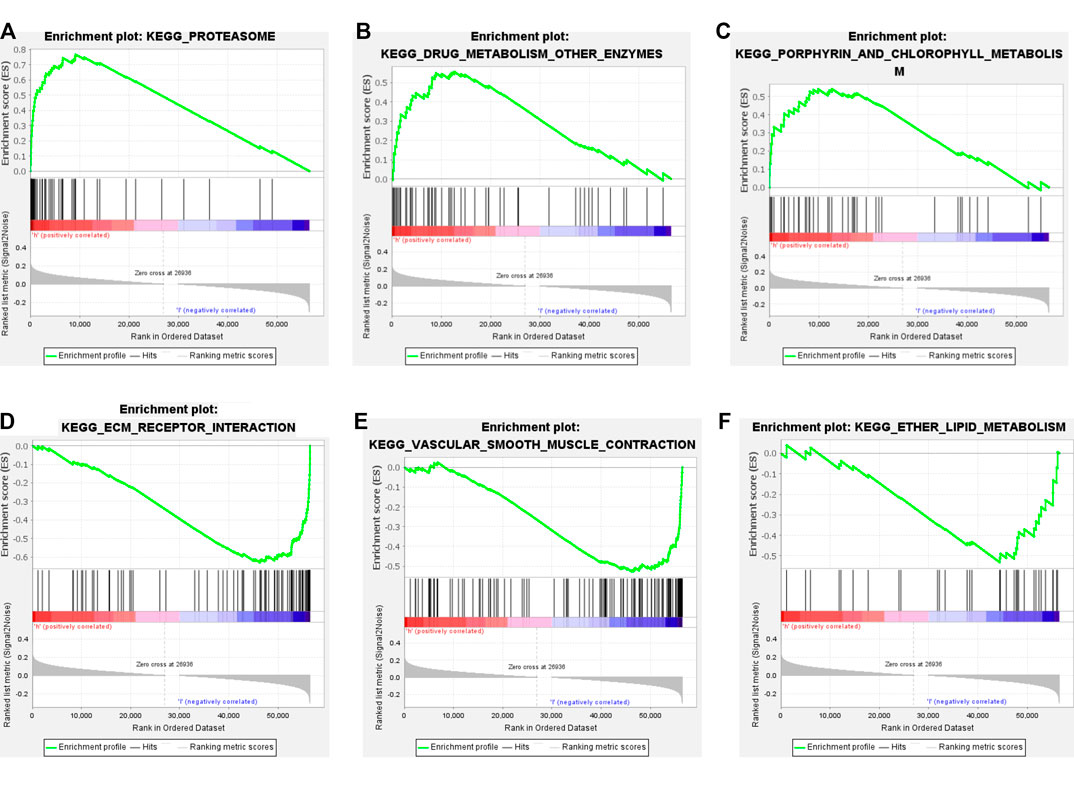
FIGURE 3. Gene set enrichment analysis GSEA with high and low TMB groups. (A–C) The top3 pathway axis enriched in high TMB groups included proteasome, drug metabolism other enzymes, porphyrin and chlorophyll metabolism. (D–F) The top3 pathway axis enriched in low TMB groups were ECM receptor interaction, vascular smooth muscle contraction, and ether lipid metabolism.
Tumor-Infiltrating Immune Cells in Hepatocellular Carcinoma
Based on the Cibersort algorithm, we analyzed the infiltration of 22 immune cells in the HCC microenvironment. As shown in Figure 4A, each column represents a sample, and different colors represent different immune cells. It was discovered that the 22 kinds of tumor-infiltrating immune cells varied significantly in each sample. Furthermore, we used the R software to process them by dividing them into high and low TMB groups, and compared the immune cell infiltration between the two groups. It was found that the memory B cells (p = 0.027) demonstrated a significantly differential expression in the high and low TMB groups. CD8+ T cells (p = 0.09), CD4+ memory activated T cells (p = 0.07) and neutrophils (p = 0.06) also showed differences in expression between the high and low TMB groups (Figure 4B).
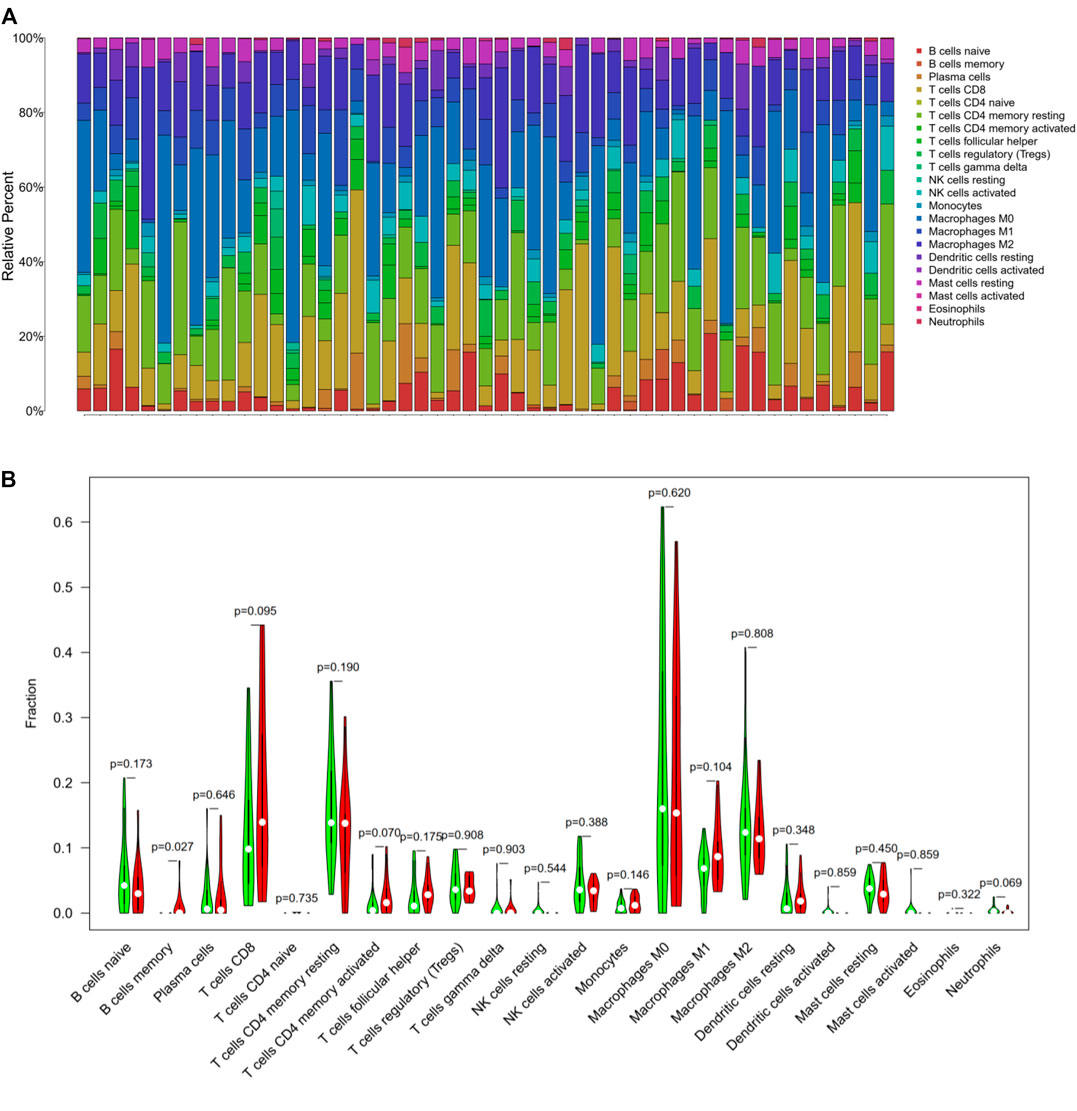
FIGURE 4. Tumor-infiltrating immune cells in hepatocellular carcinoma. (A) The stacked bar graph showed the infiltration of 22 immune cells in each sample. Each color represented a type of immune cell. (B) The Wilcoxon rank-sum test displayed that memory B cells (p = 0.02), CD8+ T cells (p = 0.09), CD4+ memory activated T cells (p = 0.07) and neutrophils (p = 0.06) had a difference in high and low TMB groups.
Identification of Significant Immune Genes for HCC Prognostication
Immune-related genes were downloaded from the database (http://www.immport.org/) and intersected with the differential genes in the sample to obtain 51 related differential immune genes (Figure 5A). Subsequently, we performed univariate Cox regression analysis using these differential immune genes, and selected genes with statistical significance for prognosis, including GABRA3, LUCAT1, MAGEA12, CECR7, STEAP4, CSAG1, TRIM16L, LINC00958, IL7R, and MAGEA3 (Table 3). Multivariate Cox regression analysis was performed as described above and the differential immune genes obtained were GABRA3, CECR7, TRIM16L, and IL7R. In addition, we performed survival analysis (Kaplan-Meier method) on these four immune genes and found that the mutations in GABRA3, CECR7, TRIM16, and IL7R were related to TMB and promoted anti-tumor immunity. The low expression of GABRA3, CECR7, and TRIM16 and the high expression of IL7R significantly prolonged the survival time of patients (Figures 5B–E; Table 4). Furthermore, the associations between GABRA3, CECR7, TRIM16, and IL7R expression, with immune cell infiltration were shown in Supplementary Figure S3.
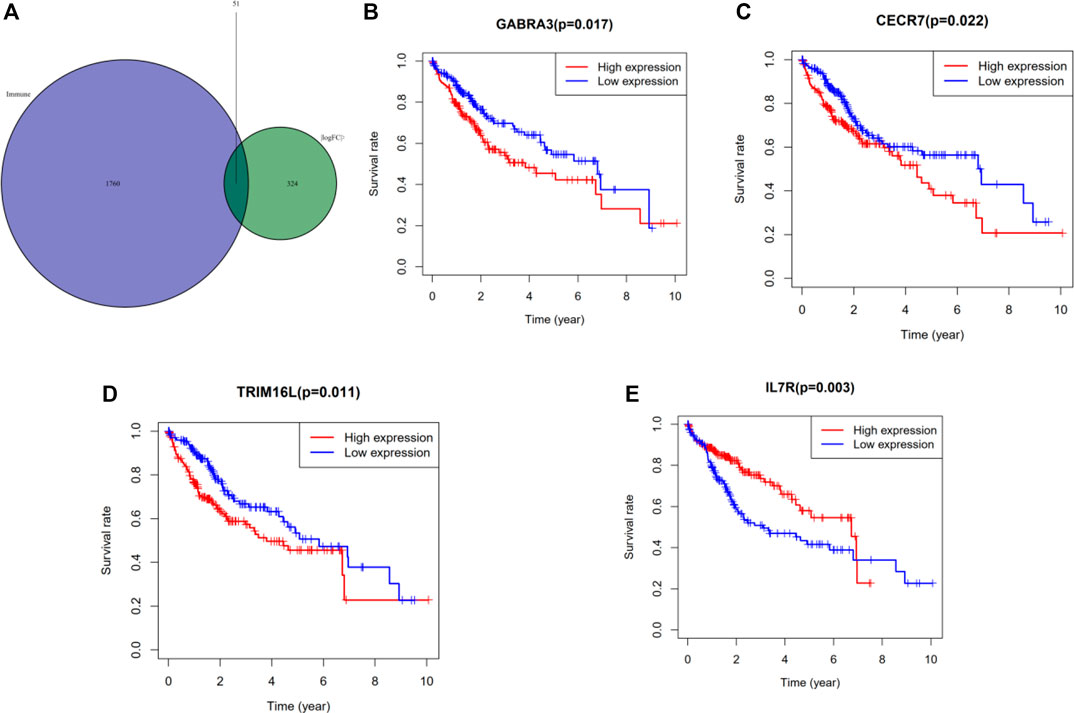
FIGURE 5. Identification of significant immune genes for HCC prognostication. (A) The Venn diagram showed that a total of 51 differential immune genes were associated with tumor mutation burden and immune infiltration. Kaplan–Meier analysis revealed that down-expression of GABRA3, CECR7, TRIM16 and up-expression of IL7R were associated with better survival outcomes and low recurrence. (B) GABRA3 (p = 0.0017) (C) CECR7 (p = 0.022) (D) TRIM16L (p = 0.011) (E) IL7R (p = 0.003)
Analysis and Evaluation of the Risk Prediction Model
Based on the multivariate Cox regression analysis, we constructed a risk prediction model and the coefficients are shown in Table 4. We divided the TCGA cohort into a high-risk group and low-risk group according to the median of the risk scores (median = 0.96), and then performed Kaplan-Meier analysis. It was found that the survival rate of the high-risk group was significantly reduced (p = 0.002, Figure 6A). Additionally, by analyzing the ROC curve of the model, we found that the established model had certain clinical significance for the prognosis of HCC (area under the curve [AUC] = 0.691, Figure 6B).
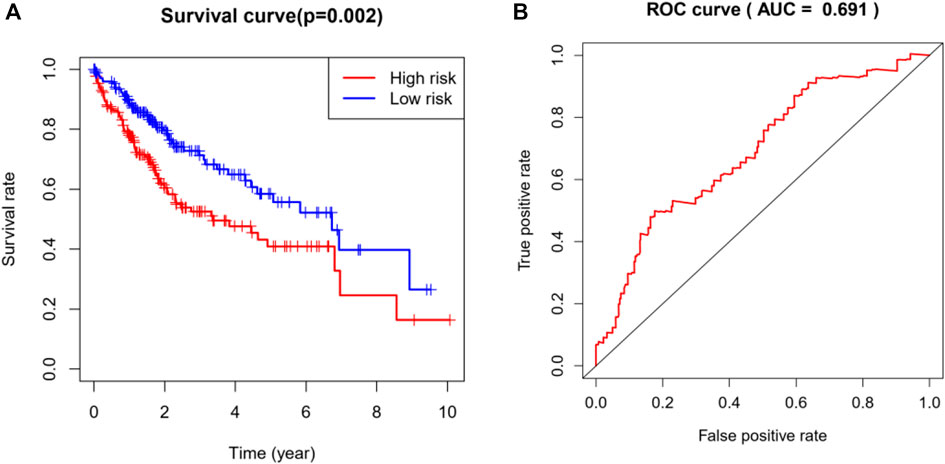
FIGURE 6. Analysis and evaluation of the risk prediction (diagnosis) models in HCC. (A) Kaplan-Meier analysis demonstrated that patients with higher risk showed worse survival rate (p = 0.002). (B) The AUC of ROC curve (AUC = 0.69) showed the predictive accuracy of TMB risk scores. AUC, area under curve; HCC, hepatocellular carcinoma; ROC, receiver operating characteristic.
Discussion
HCC is the fourth leading cause of cancer-related deaths worldwide, with approximately 750,000 new cases each year (Ferlay et al., 2015; Yang et al., 2019). Once HCC is diagnosed, it is often an aggressive or metastatic cancer, and 70% of the patients receive palliative treatment (Llovet et al., 2016; Vogel et al., 2019). The development of HCC is a complex process involving more than 160 genetic changes (Schulze et al., 2015). In recent years, immunotherapy has achieved great success in the treatment of HCC. Immunotherapy is the use of drugs to promote immune cell infiltration in the tumor microenvironment leading to the killing of the tumor cells by T or B lymphocytes. The most common first-line treatment for HCC is sorafenib, which has been proven to prolong the survival of patients with HCC for several months (Kim et al., 2017; Flynn et al., 2019). Immunotherapy mainly includes tumor vaccines, biological therapy, CAR-T cells, and immune checkpoint inhibitors (PD-1, CTLA-7). Liu et al. (2020) reported that the BET protein inhibitor JQ1 can enhance the expression of Rab8A and further upregulate the expression of PD-1, which can enhance the tumor-killing effect of CD8+ cells. In addition to the immune checkpoint inhibitors, tumor vaccines have also been widely used in the treatment of HCC. Lu et al. (2017) indicated that exosomes derived from dendritic cells stimulated the tumor immune response in HCC and killed or inhibited the tumor cells. Tumor immunotherapy has been widely reported to achieve good results in lung cancer, kidney cancer, and melanoma (Larkin et al., 2015).
Although immunotherapy has achieved great success, only a small proportion of patients benefit from it. Previous studies have revealed that patients with a high TMB had accompanying obvious immune cell infiltration and often benefited from immunotherapy. Therefore, there is an urgent need for biomarkers to predict the effect of immunotherapy. Zhu et al. found that the EP300 mutation was related to the TMB and promoted anti-tumor immunity in bladder cancer (Zhu et al., 2020). Zhang et al. (2020) reported that immune genes such as CES1 and CSAG1 could be used as biomarkers for predicting the effects of immunotherapy through a multi-omics analysis. Similarly, Pan et al. (2019) discovered that Laylin is a prognostic marker of survival and is associated with immune infiltration in gastric and colon cancer.
GABRA3 is a type of aminobutyric acid receptor and recognized as a typical oncogene. It is a subunit of ion channel GABAa receptors. Existing studies have shown that GABRA3 is significantly up-regulated in tumor tissues and can regulate tumor proliferation, invasion and metastasis through AKT-mTOR (Long et al., 2017). CECR7 is an immune-related lncRNA. Multi-omics’ study show that it can play an immunomodulatory role through CECR7-hsa-miR-429-CTLA4. Competitive endogenous immune lncRNA CECR7 binds to has-miR-429, regulating the key point of CTLA4 (Yao et al., 2017). TMRI16L and IL7R are also related to tumor immunity, which regulate tumors through the typical JAK/STAT pathway and the endogenous competitive ceRNA network (Fan et al., 2018).
In this study, we downloaded a dataset of 364 American HCC samples from the TCGA. By comparing the immune-infiltrating cells in the high and low TMB groups through the violin chart, we found that memory B cells, CD8+ T cells, and CD4+ memory activated T cells were more abundant in the high TMB group. The main limitation of this study was that we only included samples from the TCGA database and lacked clinical validation experiments. Even if the expressions of GABRA3, CECR7, TRIM16L, and IL7R are obviously changed in HCC in the United States and are associated with TMB and immune infiltration, this does not mean that this risk prediction model can provide a basis for immunotherapy in actual clinical practice. Further clinical trials are needed for verifying this risk prediction model.
In conclusion, our study proved that GABRA3, CECR7, TRIM16L, and IL7R were frequently mutated in HCC and related to TMB and immune infiltration. In addition, we built a prognostic model to evaluate the effect of immunotherapy in HCC. These results suggest novel biomarkers for immunotherapy in HCC.
Data Availability Statement
The datasets presented in this study can be found in online repositories. The names of the repository/repositories and accession number(s) can be found in the article/Supplementary Material.
Author Contributions
LY and LZ conceived and designed the study and analyzed the data. LZ drafted the article. RX reviewed the manuscript. All authors read and approved the manuscript.
Conflict of Interest
The authors declare that the research was conducted in the absence of any commercial or financial relationships that could be construed as a potential conflict of interest.
Acknowledgments
This manuscript has been released as a pre-print at Research Square (Yin et al., 2020).
Supplementary Material
The Supplementary Material for this article can be found online at: https://www.frontiersin.org/articles/10.3389/fmolb.2020.599142/full#supplementary-material.
Abbreviations
HCC, hepatocellular carcinoma; TMB, tumor mutation burden; TCGA, the cancer genome atlas; GO, gene ontology; GSEA, gene set enrichment analysis; TNM: tumor-node-metastasis; HR: hazard ratio; ECM: extracellular matrix.
References
Buchbinder, E. I., Dutcher, J. P., Daniels, G. A., Curti, B. D., Patel, S. P., Holtan, S. G., et al. (2019). Therapy with high-dose Interleukin-2 (HD IL-2) in metastatic melanoma and renal cell carcinoma following PD1 or PDL1 inhibition. J. Immunother. Cancer 7 (1), 49. doi:10.1186/s40425-019-0522-3
Chan, T. A., Yarchoan, M., Jaffee, E., Swanton, C., Quezada, S. A., Stenzinger, A., et al. (2019). Development of tumor mutation burden as an immunotherapy biomarker: utility for the oncology clinic. Ann. Oncol. 30 (1), 44–56. doi:10.1093/annonc/mdy495
Chardin, D., Paquet, M., Schiappa, R., Darcourt, J., Bailleux, C., Poudenx, M., et al. (2020). Baseline metabolic tumor volume as a strong predictive and prognostic biomarker in patients with non-small cell lung cancer treated with PD1 inhibitors: a prospective study. J. Immunother. Cancer 8 (2), e000645. doi:10.1136/jitc-2020-000645
Chen, H., Chong, W., Wu, Q., Yao, Y., Mao, M., and Wang, X. (2019a). Association of LRP1B Mutation with tumor mutation burden and outcomes in melanoma and non-small cell lung cancer patients treated with immune check-point blockades. Front. Immunol. 10, 1113. doi:10.3389/fimmu.2019.01113
Chen, Y., Liu, Q., Chen, Z., Wang, Y., Yang, W., Hu, Y., et al. (2019b). PD-L1 expression and tumor mutational burden status for prediction of response to chemotherapy and targeted therapy in non-small cell lung cancer. J. Exp. Clin. Cancer Res. 38 (1), 193. doi:10.1186/s13046-019-1192-1
Chiu, D. K., Yuen, V. W., Wing-Sum Cheu, J., Wei, L. L., Ting, V., Fehlings, M., et al. (2020). Hepatocellular carcinoma cells up-regulate PVRL1, stabilizing poliovirus receptor and inhibiting the cytotoxic T-cell response via TIGIT to mediate tumor resistance to PD1 inhibitors in mice. Gastroenterology 159 (20), 609–623. doi:10.1053/j.gastro.2020.03.074
El-Serag, H. B., and Rudolph, K. L. (2007). Hepatocellular carcinoma: epidemiology and molecular carcinogenesis. Gastroenterology 132 (7), 2557–2576. doi:10.1053/j.gastro.2007.04.061
Fan, Y., Nan, Y., Huang, J., Zhong, H., and Zhou, W. (2018). Up-regulation of inflammation-related LncRNA-IL7R predicts poor clinical outcome in patients with cervical cancer. Biosci. Rep. 38 (3), BSR20180483. doi:10.1042/BSR20180483
Ferlay, J., Soerjomataram, I., Dikshit, R., Eser, S., Mathers, C., Rebelo, M., et al. (2015). Cancer incidence and mortality worldwide: sources, methods and major patterns in GLOBOCAN 2012. Int. J. Cancer 136 (5), E359–E386. doi:10.1002/ijc.29210
Flynn, M. J., Sayed, A. A., Sharma, R., Siddique, A., and Pinato, D. J. (2019). Challenges and opportunities in the clinical development of immune checkpoint inhibitors for hepatocellular carcinoma. Hepatology 69 (5), 2258–2270. doi:10.1002/hep.30337
Kim, D. W., Talati, C., and Kim, R. (2017). Hepatocellular carcinoma (HCC): beyond sorafenib-chemotherapy. J. Gastrointest Oncol. 8 (2), 256–265. doi:10.21037/jgo.2016.09.07
Kim, H. D., Song, G. W., Park, S., Jung, M. K., Kim, M. H., Kang, H. J., et al. (2018). Association between expression level of PD1 by tumor-infiltrating CD8+ T cells and features of hepatocellular carcinoma. Gastroenterology 155 (6), 1936–1950.e17. doi:10.1053/j.gastro.2018.08.030
Koboldt, D.C., Zhang, Q., Larson, D.E., Shen, D., McLellan, M.D., Lin, L., et al. (2012). VarScan 2: somatic mutation and copy number alteration discovery in cancer by exome sequencing. Genome Res. 22 (3), 568–76. doi:10.1101/gr.129684.111
Larkin, J., Chiarion-Sileni, V., Gonzalez, R., Grob, J. J., Cowey, C. L., Lao, C. D., et al. (2015). Combined nivolumab and ipilimumab or monotherapy in untreated melanoma. N. Engl. J. Med. 373 (1), 23–34. doi:10.1056/NEJMoa1504030
Liu, C., Miao, X., Wang, Y., Wen, L., Cheng, X., Kong, D., et al. (2020). BET protein inhibition improves immunotherapy efficacy in hepatocellular carcinoma. Cancer Sci. 111, 3503. doi:10.1111/cas.1458810.1111/cas.14588
Llovet, J. M., Zucman-Rossi, J., Pikarsky, E., Sangro, B., Schwartz, M., Sherman, M., et al. (2016). Hepatocellular carcinoma. Nat. Rev. Dis. Primers 2, 16018. doi:10.1038/nrdp.2016.18
Long, M., Zhan, M., Xu, S., Yang, R., Chen, W., Zhang, S., et al. (2017). miR-92b-3p acts as a tumor suppressor by targeting Gabra3 in pancreatic cancer. Mol. Cancer. 16 (1), 167. doi:10.1186/s12943-017-0723-7
Luke, J. J., Flaherty, K. T., Ribas, A., and Long, G. V. (2017). Targeted agents and immunotherapies: optimizing outcomes in melanoma. Nat. Rev. Clin. Oncol. 14 (8), 463–482. doi:10.1038/nrclinonc.2017.43
Lu, Z., Zuo, B., Jing, R., Gao, X., Rao, Q., Liu, Z., et al. (2017). Dendritic cell-derived exosomes elicit tumor regression in autochthonous hepatocellular carcinoma mouse models. J. Hepatol. 67 (4), 739–748. doi:10.1016/j.jhep.2017.05.019
Massarweh, N.N., and El-Serag, H.B. (2017). Epidemiology of hepatocellular carcinoma and intrahepatic cholangiocarcinoma. Cancer Control 24 (3), 1073274817729245. doi:10.1177/1073274817729245
Newman, A. M., Liu, C. L., Green, M. R., Gentles, A. J., Feng, W., Xu, Y., et al. (2015). Robust enumeration of cell subsets from tissue expression profiles. Nat. Methods 12 (5), 453–7. doi:10.1038/nmeth.3337
Pan, J. H., Zhou, H., Cooper, L., Huang, J. L., Zhu, S. B., Zhao, X. X., et al. (2019). LAYN Is a prognostic biomarker and correlated with immune infiltrates in gastric and colon cancers. Front. Immunol. 10, 6. doi:10.3389/fimmu.2019.00006
Petrick, J. L., Kelly, S. P., Altekruse, S. F., McGlynn, K. A., and Rosenberg, P. S. (2016). Future of Hepatocellular Carcinoma Incidence in the United States Forecast Through 2030. J. Clin Oncol. 34 (15), 1787–1794. doi:10.1200/JCO.2015.64.7412
Prieto, J., Melero, I., and Sangro, B. (2015). Immunological landscape and immunotherapy of hepatocellular carcinoma. Nat. Rev. Gastroenterol. Hepatol. 12 (12), 681–700. doi:10.1038/nrgastro.2015.173
Schulze, K., Imbeaud, S., Letouzé, E., Alexandrov, L. B., Calderaro, J., Rebouissou, S., et al. (2015). Exome sequencing of hepatocellular carcinomas identifies new mutational signatures and potential therapeutic targets. Nat. Genet. 47 (5), 505–511. doi:10.1038/ng.3252
Revathidevi, S., and Munirajan, A. K. (2019). Akt in cancer: Mediator and more. Semin. Cancer Biol. 59, 80–91. doi:10.1016/j.semcancer.2019.06.002
Siegel, R. L., Miller, K. D., and Jemal, A. (2016). Cancer statistics, 2016. CA Cancer J. Clin. 66 (1), 7–30. doi:10.3322/caac.21332
Singal, A. G., Lampertico, P., and Nahon, P. (2020). Epidemiology and surveillance for hepatocellular carcinoma: new trends. J. Hepatol. 72 (2), 250–261. doi:10.1016/j.jhep.2019.08.025
Subramanian, A., Tamayo, P., Mootha, V. K., Mukherjee, S., Ebert, B. L., Gillette, M. A., et al. (2005). Gene set enrichment analysis: a knowledge-based approach for interpreting genome-wide expression profiles. Proc. Natl. Acad. Sci. U.S.A. 102 (43), 15545–15550. doi:10.1073/pnas.0506580102
Szekely, B., Bossuyt, V., Li, X., Wali, V. B., Patwardhan, G. A., Frederick, C., et al. (2018). Immunological differences between primary and metastatic breast cancer. Ann. Oncol. 29 (11), 2232–2239. doi:10.1093/annonc/mdy399
Tian, M., Shi, Y., Liu, W., and Fan, J. (2019). Immunotherapy of hepatocellular carcinoma: strategies for combinatorial intervention. Sci. China Life Sci. 62 (9), 1138–1143. doi:10.1007/s11427-018-9446-2
Thyer, L., Ward, E., Smith, R., Branca, J. J., Morucci, G., Gulisano, M., et al. (2013). Therapeutic effects of highly purified de-glycosylated GCMAF in the immunotherapy of patients with chronic diseases. Am. J. Reprod. Immunol. 9 (3), 78–84. doi:10.3844/ajisp.2013.78.84
Vogel, A., Cervantes, A., Chau, I., Daniele, B., Llovet, J. M., Meyer, T., et al. (2019). Hepatocellular carcinoma: ESMO clinical practice guidelines for diagnosis, treatment and follow-up. Ann. Oncol. 30 (5), 871–873. doi:10.1093/annonc/mdy510
Yang, J. D., Hainaut, P., Gores, G. J., Amadou, A., Plymoth, A., and Roberts, L. R. (2019). A global view of hepatocellular carcinoma: trends, risk, prevention and management. Nat. Rev. Gastroenterol Hepatol. 16 (10), 589–604. doi:10.1038/s41575-019-0186-y
Yao, K., Wang, Q., Jia, J., and Zhao, H. (2017). A competing endogenous RNA network identifies novel mRNA, miRNA and lncRNA markers for the prognosis of diabetic pancreatic cancer. Tumour Biol. 39 (6), 1010428317707882. doi:10.1177/1010428317707882
Yin, L., Zhou, L.Z., and Xu, R.J. (2020). Identification of tumor mutation burden and immune infiltrates in hepatocellular carcinoma based on multi-omics analysis. Research Square [Epub ahead of print]. doi:10.21203/rs.3.rs-63032/v1
Zhang, C., Shen, L., Qi, F., Wang, J., and Luo, J. (2020). Multi-omics analysis of tumor mutation burden combined with immune infiltrates in bladder urothelial carcinoma. J. Cell Physiol. 235 (4), 3849–3863. doi:10.1002/jcp.29279
Keywords: hepatocellular carcinoma, immune infiltration, tumor mutation burden, biomarkers, prognosis
Citation: Yin L, Zhou L and Xu R (2021) Identification of Tumor Mutation Burden and Immune Infiltrates in Hepatocellular Carcinoma Based on Multi-Omics Analysis. Front. Mol. Biosci. 7:599142. doi: 10.3389/fmolb.2020.599142
Received: 27 August 2020; Accepted: 21 December 2020;
Published: 16 February 2021.
Edited by:
Nikolay Mikhaylovich Borisov, Moscow Institute of Physics and Technology, RussiaCopyright © 2021 Yin, Zhou and Xu. This is an open-access article distributed under the terms of the Creative Commons Attribution License (CC BY). The use, distribution or reproduction in other forums is permitted, provided the original author(s) and the copyright owner(s) are credited and that the original publication in this journal is cited, in accordance with accepted academic practice. No use, distribution or reproduction is permitted which does not comply with these terms.
*Correspondence: Lu Yin, eWlubHVAemp1LmVkdS5jbg==
†These authors have contributed equally to this work