- 1The First Clinical Medical College, Zhejiang Chinese Medical University, Hangzhou, China
- 2Zhejiang Provincial Hospital of Traditional Chinese Medicine, Hangzhou, China
Background: FCGR1A encodes a protein that plays an important role in the immune response. The prognostic impact and immune infiltration of FCGR1A in heterogeneous cancers remain unclear.
Methods: Differential expression of FCGR1A between tumor and normal tissues and the discrepancies in overall survival (OS) among diverse cancer types were performed by Gene Expression Profiling Interactive Analysis. The correlation between FCGR1A and immune cells or gene marker sets of immune infiltrates was analyzed via Tumor Immune Estimation Resource (TIMER). Gene Ontology (GO), Kyoto Encyclopedia of Genes and Genomes (KEGG) analysis, and protein-to-protein interaction (PPI) network were used to explore the function and related genes of FCGR1A. The relationships among these genes were further analyzed by TIMER.
Results: FCGR1A is highly expressed in various cancer types. FCGR1A was significantly correlated with the OS of cervical and endocervical cancer (CESC), cholangiocarcinoma (CHOL), kidney renal clear cell carcinoma (KIRC), and skin cutaneous melanoma (SKCM) (P < 0.05). High expression of FCGR1A meant a better prognosis besides KIRC. FCGR1A showed significant differences at different stages of KIRC and SKCM (P < 0.05). Furthermore, FCGR1A was notably associated with infiltrating levels of CD4+ T cells, CD8+ T cells, B cells, macrophages, neutrophils, and dendritic cells in the four cancers (P < 0.05). FCGR1A also showed close relevance with different immune gene markers. The copy number variation of FCGR1A significantly influenced the abundance of immune infiltration in KIRC and SKCM. GO, KEGG analysis, and PPI network analysis revealed that FCGR1A is involved in many pathophysiological processes and was most related to FCGR3A. And this gene indicated highly significant positive correlations with FCGR1A in four cancers.
Conclusion: FCGR1A may be a potential prognostic biomarker and related to immune infiltration levels in diverse cancers, especially in CESC, CHOL, KIRC, and SKCM. Besides, FCGR1A may be involved in the activation, regulation, or induction of immune cells and diverse physiological and pathological processes.
Introduction
FCGR1A (CD64) is a 72-kDa transmembrane glycoprotein with CD32 and CD16 receptors (Kiyoshi et al., 2015; Lu and Sun, 2015). It encodes a protein that plays an important role in both innate and adaptive immune response. The protein is a high-affinity Fc-gamma receptor. FCGR1A is one of three related gene family members located on chromosome 1 and often expressed on the surface of monocytes, macrophages, and dendritic cells (DCs) (Hulett and Hogarth, 1998). However, CD64 is rarely expressed on the surface of neutrophils (PMN) in normal condition. When the body is infected, CD64 expression on the surface of neutrophils can be rapidly increased under the stimulation of bacterial lipopolysaccharide, interleukin-12, interferon-γ, and granulocyte colony-stimulating factor (Mangalam and Yadav, 2019). Neutrophils are often the first immune cells to reach sites of infection, and their main function is to phagocytose and destroy invading microorganisms or foreign material. Increased CD64 expression is one of the markers of PMN activation and can be used as a good diagnostic indicator of infectious diseases (Cid et al., 2010). Previous studies have reported that FCGR1A (CD64) is of great significance for diagnosis of leukemia (Kern et al., 2006). Acute myelomonocytic leukemia (M4) and acute monocytic leukemia (M5) are both subtypes of AML, which significantly correlated with the expression of CD64 (Thepen et al., 2009).
Cervical cancer is the fourth most common cancer among women worldwide, with a high mortality rate, especially in developing countries (Bray et al., 2018). High-risk types of human papillomavirus (HPV) infections are strongly associated with a high incidence of cervical cancers; thus, HPV vaccination and screening are necessary (Crosbie et al., 2013). Immunotherapy brings hope for women with recurrent and metastatic cervical cancer (Cohen et al., 2019). Cholangiocarcinoma (CHOL) is an epithelial cell malignancy arising from diverse anatomic locations within the biliary tree, which is classified as intrahepatic, perihilar, and distal CHOL based on anatomical location (Razumilava and Gores, 2014). CHOL accounts for approximately 3% of all gastrointestinal malignancies, and the diagnosis and treatment remain extremely difficult (Khan et al., 2019). Several studies have pointed out that M2 macrophage polarization correlates with poor prognosis and metastasis of CHOL (Hasita et al., 2010; Thanee et al., 2015; Kaneda et al., 2016). Tumor-associated macrophages (TAMs) and tumor-associated neutrophil infiltration are positively associated with poor clinical outcomes, but they have not yet been considered as a therapeutic target for CHOL (Shen et al., 2014; Lee et al., 2016; Tan et al., 2016). Kidney renal clear cell carcinoma (KIRC) is the most common subtype of renal cell carcinoma (80%), and nearly 25% of patients were diagnosed at an advanced stage with lymphatic or distant metastasis (Sinha et al., 2017; Zhang B. et al., 2019). Several reports have indicated that high expression of CTLA4, LAG3, and TIGIT is related to a worse overall survival (OS), whereas high expression of TIM-3 is associated with better prognosis (Zhang S. et al., 2019). Programmed death-1 (PD-1) inhibitors (nivolumab) have been approved by the U.S. Food and Drug Administration (FDA) for the treatment of advanced RCC. CTLA-4 (ipilimumab) and PD-1 ligand inhibitors (avelumab) are currently under study (Barata and Rini, 2017). Skin cutaneous melanoma (SKCM) is one of the most aggressive cancers, accounting for 4% of all skin cancer types but 75% of skin cancer–related deaths because of its high metastatic potential (Shenenberger, 2012). The checkpoint inhibitors nivolumab (Olbryt, 2019) and pembrolizumab (Bardhan et al., 2016) have been approved by the FDA for advanced melanoma patients and have yielded a superior overall response rate (Olbryt, 2019).
In this study, we comprehensively analyzed the relationship between the expression of FCGR1A and prognosis of various cancer types using the Gene Expression Profiling Interactive Analysis (GEPIA) database. Besides, Tumor Immune Estimation Resource (TIMER) was utilized to study the correlation between FCGR1A and tumor-infiltrating immune cells. We also elaborated the important role of FCGR1A in cervical and endocervical cancer (CESC), CHOL, KIRC, and SKCM, as well as revealing a potential relationship among FCGR1A, tumor–immune interactions, and neighboring genes.
Materials and Methods
GEPIA Analysis
The expression level of the FCGR1A gene in distinct cancer types was analyzed via the GEPIA1. GEPIA is an open web that contains 9,736 tumor and 8,587 normal samples from The Cancer Genome Atlas (TCGA) and GTEx projects, which mainly including 33 cancer types (Tang et al., 2017). This web focused on the analysis of RNA sequence expression. We utilized GEPIA to plot survival curves with different FCGR1A expression in 33 cancer types, and survival differences were compared with the log-rank test. The primary endpoints of the study were OS and disease-free survival (DFS). Expression differences of FCGR1A between normal and tumor tissues were graphically visualized using box plots. Meanwhile, the effect of FCGR1A expression for diverse pathological stages was presented as violin plots.
TIMER Database Analysis
Tumor immune estimation resource is a public resource dedicated to tumor immune infiltrates across 32 cancer types incorporating 10,897 samples from TCGA2 (Li et al., 2017). This web server provides a variety of immune deconvolution methods to estimate the abundance of immune infiltration and explore tumor immunity or genomic characteristic comprehensively (Li et al., 2016). Differential expression levels of FCGR1A in different cancer types were shown by the box plots via TIMER. Cancers with significant differences in FCGR1A expression would be selected for further analysis. The association between FCGR1A expression and the abundance of immune infiltrates, including CD4+ T cells, CD8+ T cells, B cell, macrophages, neutrophils, and DCs, was analyzed via gene modules. Furthermore, associations between FCGR1A and gene markers of tumor-infiltrating immune cells were also explored (Danaher et al., 2017; Siemers et al., 2017). The gene markers mainly included T cells, CD8+ T cells, B cells, monocyte, TAMs, neutrophils, natural killer (NK) cells, DCs, T-helper 1 (TH1) cells, regulatory T lymphocytes (Tregs), and exhausted T cells. Besides, the relationship between the copy number variation (CNV) of FCGR1A and tumor immune infiltrating level can also be analyzed easily. FCGR1A and relevant marker genes were placed on x- and y-axis, respectively, to depict scatter plots and obtain correlation coefficient as well as P values. The expression level was exhibited with log2 TPM.
Related Gene Identification
Related genes with FCGR1A expression were conducted by STRING database3. STRING database is an online software that could perform a synthetic analysis of the direct or indirect associations among selected genes. Gene Ontology (GO) function and Kyoto Encyclopedia of Genes and Genomes (KEGG) pathway enrichment analyses of FCGR1A were performed using the STRING, and related results were obtained. The protein-to-protein interaction (PPI) networks were also constructed using the protein query function in STRING. We further analyzed the potential relationships between FCGR1A and the most related genes via TIMER database.
Statistical Analysis
Survival curves were depicted by GEPIA. The results of GEPIA, TIMER, and STRING were displayed with hazard ratio (HR), P values, correlation score, or false discovery rate. The correlation of FCGR1A expression was assessed by Spearman correlation and statistical significance. In addition, the strength of the correlation should follow the following criteria: 0.00–0.19 (very weak or none), 0.20–0.39 (weak), 0.4–0.59 (moderate), 0.6–0.79 (strong), and 0.8–1.0 (very strong). P < 0.05 was regarded as statistically significant.
Results
The FCGR1A Expression Levels in Different Cancer Types
To determine the differential expression of FCGR1A in various cancer locations, we used RNA-seq data from TCGA database to explore the relationship between prognosis and FCGR1A expression in multiple cancer types via GEPIA. Results of FCGR1A expression between tumor and normal tissues are shown in Figure 1A. To obtain more comprehensive and accurate conclusion, we also analyzed FCGR1A using the “general” module in GEPIA, and the results were exhibited in Figure 1B. Together with the above results, FCGR1A expression levels were significantly higher in bladder urothelial carcinoma (BLCA), breast invasive carcinoma (BRCA), CESC, CHOL, colon adenocarcinoma (COAD), esophageal carcinoma (ESCA), glioblastoma multiforme (GBM), head and neck cancer (HNSC), KIRC, kidney renal papillary cell carcinoma (KIRP), acute myeloid leukemia (LAML), lower-grade glioma (LGG), ovarian serous cystadenocarcinoma (OV), pancreatic adenocarcinoma (PAAD), prostate adenocarcinoma (PRAD), rectum adenocarcinoma (READ), SKCM, stomach adenocarcinoma (STAD), testicular germ cell tumor (TGCT), thyroid carcinoma (THCA), uterine corpus endometrial carcinoma (UCEC), and uterine carcinosarcoma (UCS). Conversely, FCGR1A had markedly lower expression in lung adenocarcinoma (LUAD), lung squamous cell carcinoma (LUSC), and thymoma (THYM).
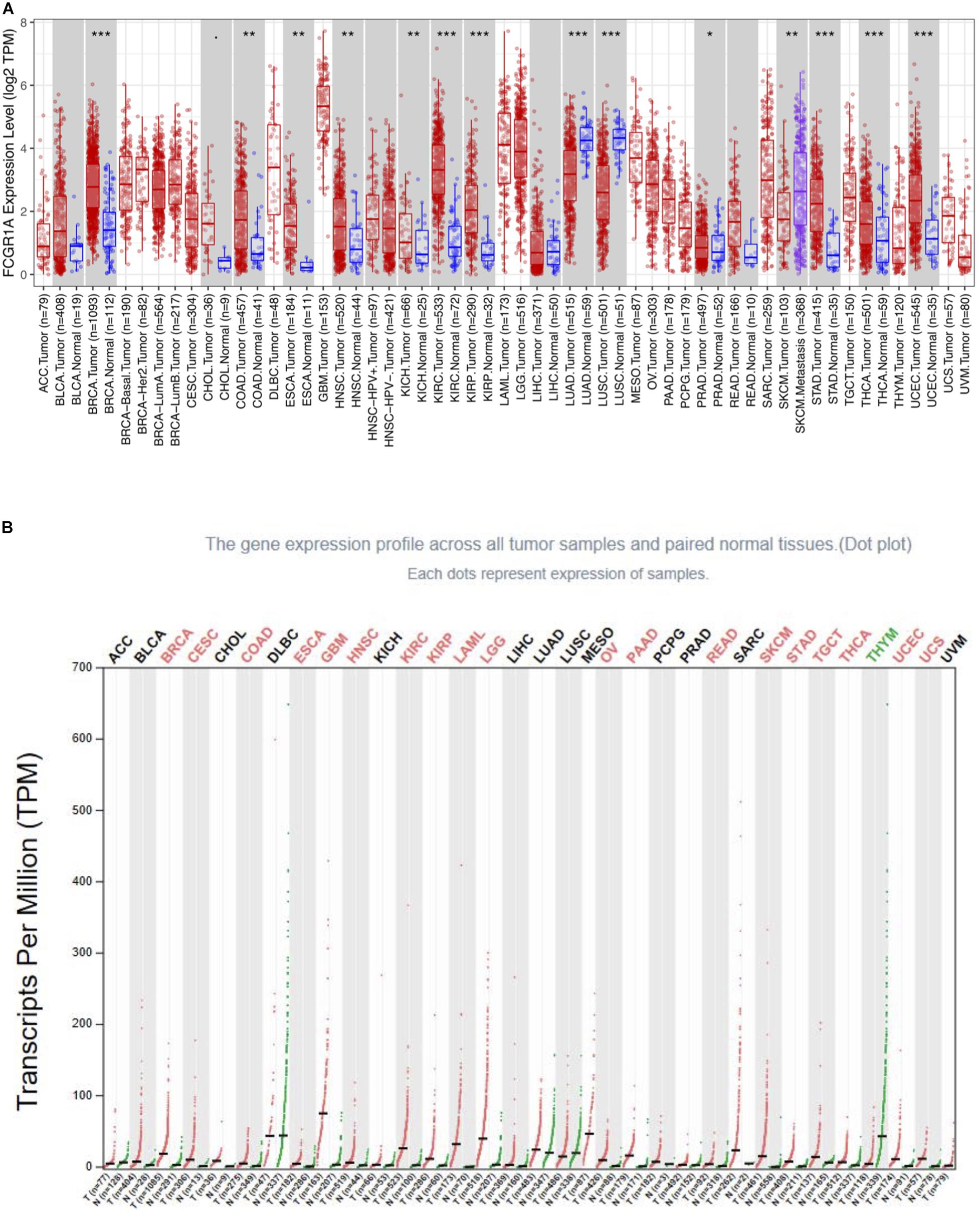
Figure 1. FCGR1A expression in different cancers types. (A) FCGR1A expression levels in different cancer types from TCGA database were performed by TIMER (*P < 0.05, **P < 0.01, ***P < 0.001). (B) Increased or decreased FCGR1A expression of different cancers compared with normal tissues in GEPIA.
A Potential Prognostic Biomarker for CESC, CHOL, KIRC, and SKCM: FCGR1A
Survival analysis of 33 cancer types mentioned above with different FCGR1A expression was performed using GEPIA. The results showed that FCGR1A was significantly associated with prognosis in four cancer types, which were CESC, CHOL, KIRC, and SKCM, respectively (Figure 2). Based on these results, box plots indicated significant differences in FCGR1A expression levels between the tumor and normal tissues among these four cancer types (Figure 3). In addition, high FCGR1A expression exhibited superior OS in CESC (HR = 0.55, p = 0.012), CHOL (HR = 0.3, p = 0.016), and SKCM (HR = 0.53, p = 3.2e-06), and also showed better DFS in CHOL (HR = 0.29, p = 0.010). However, low expression of FCGR1A in KIRC was more beneficial to survival (HR = 1.9, p = 5.3e-05). FCGR1A expression levels at different stages of the four aforementioned cancer types were also analyzed. Significant differences were observed in the FCGR1A expression levels between different histological stages in KIRC (P = 4.76e-10) and SKCM (P = 0.00141). However, no significant differences were detected in CESC and CHOL (Figure 4). Thus, these results demonstrated that FCGR1A expression was linked to the prognosis of CESC, CHOL, KIRC, and SKCM. And the effect of different FCGR1A expression on four tumor locations varied.
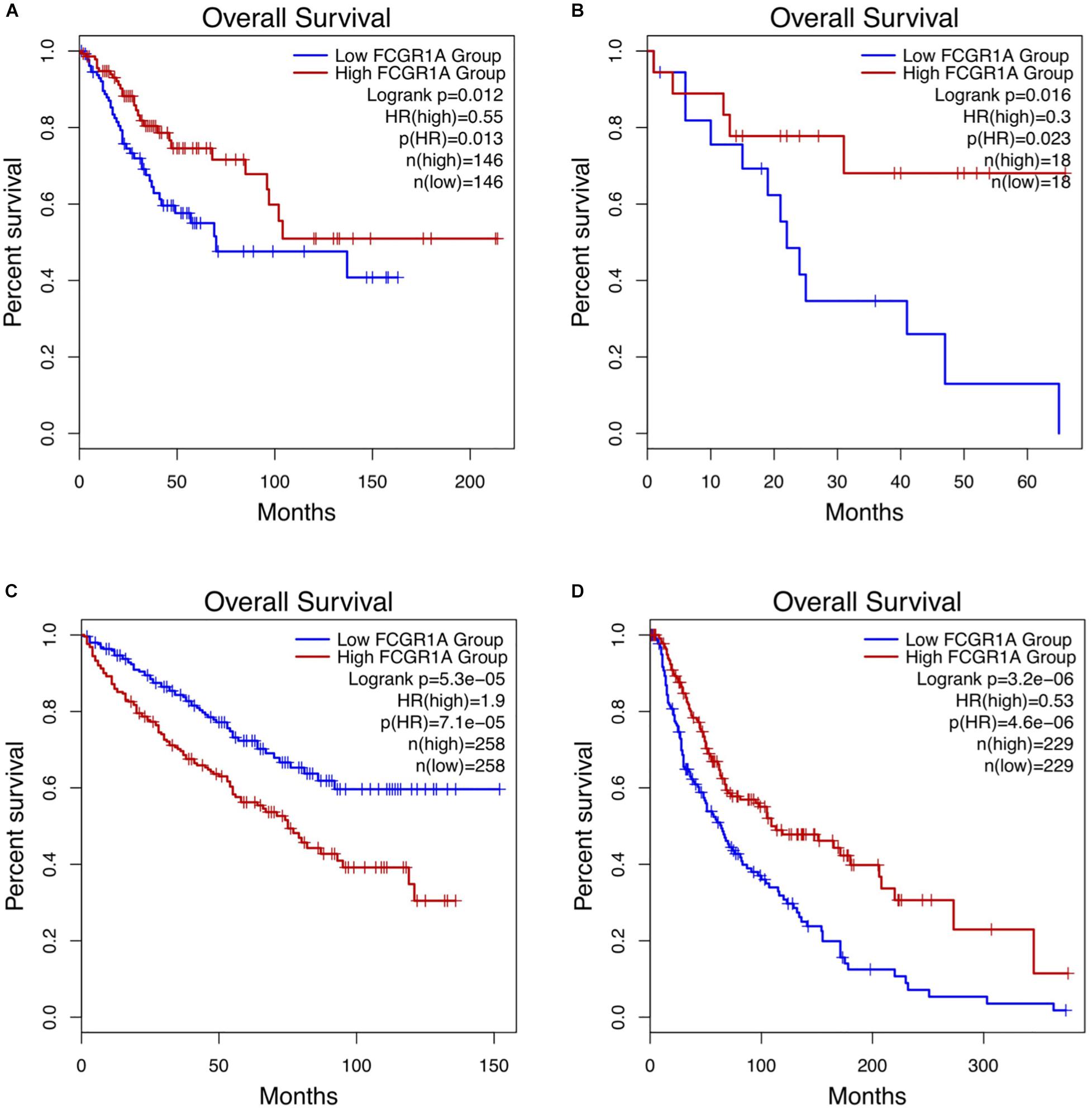
Figure 2. Kaplan–Meier survival curves comparing the high and low expression of FCGR1A in four cancer types in GEPIA. (A) CESC, (B) CHOL, (C) KIRC, and (D) SKCM.
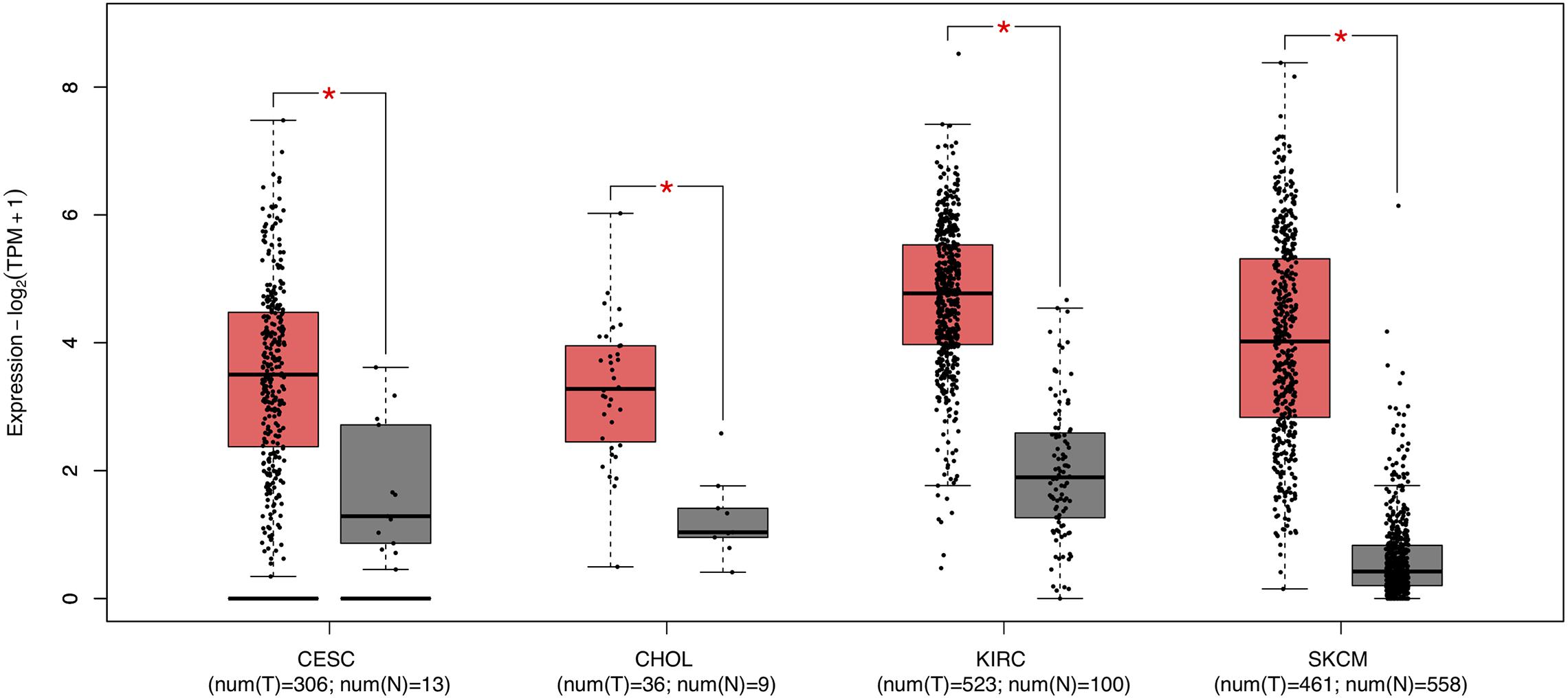
Figure 3. Box plots of FCGR1A expression between tumor and normal tissue in four cancer types. *P < 0.05.
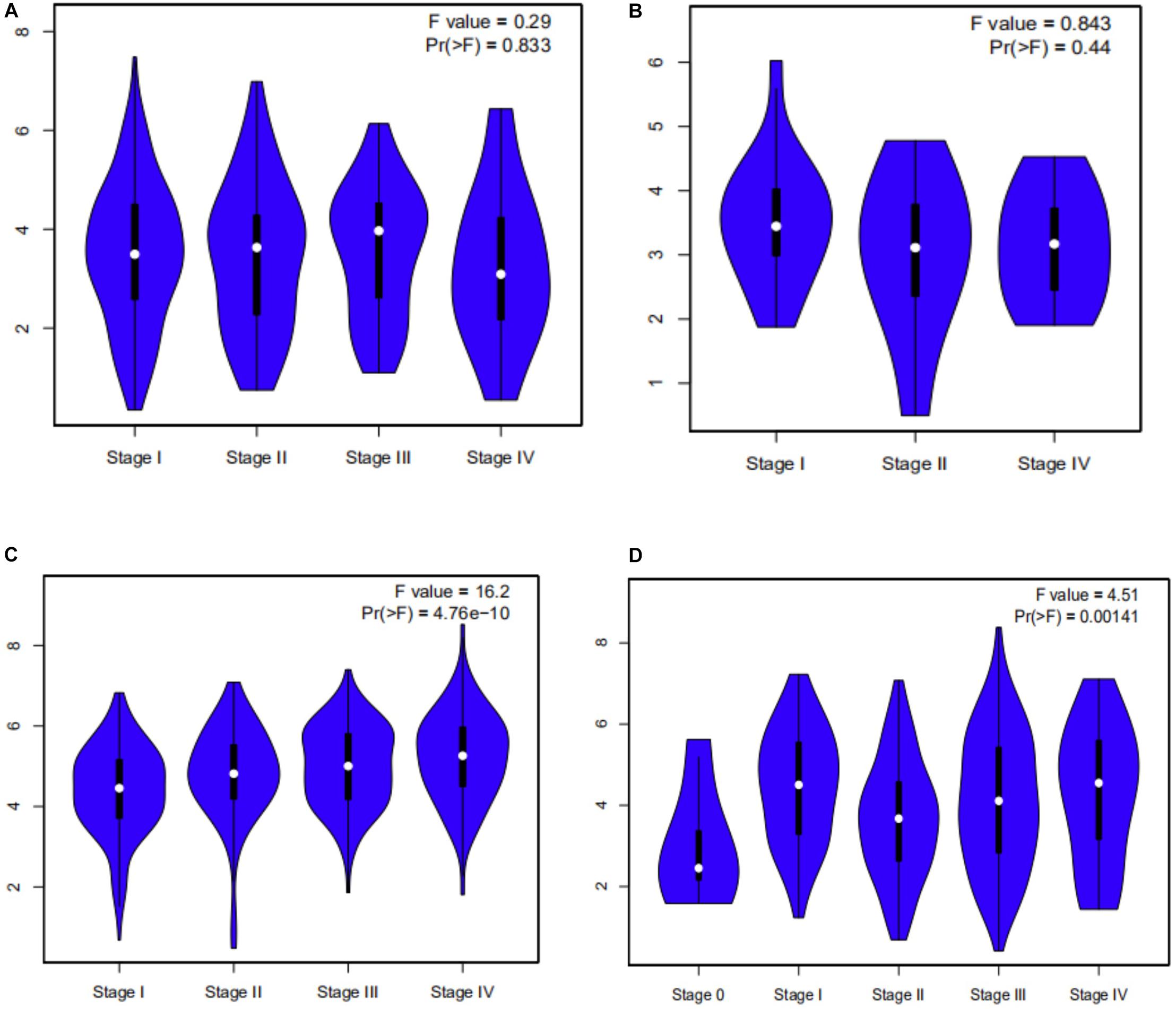
Figure 4. Violin plots pf FCGR1A expression in different stages of four cancers. (A) CESC, (B) CHOL, (C) KIRC, and (D) SKCM.
FCGR1A Expression and Somatic CNV Related With Immune Infiltration Level in CESC, CHOL, KIRC, and SKCM
To understand the correlation between FCGR1A and different infiltrated immune cells including CD4+ T cells, CD8+ T cells, B cells, macrophages, neutrophils, and DCs, TIMER was used to identify the relationship in CESC, CHOL, KIRC, and SKCM (Figure 5). All immune cells were significantly associated with FCGR1A expression levels in the four cancers (P < 0.05). However, relevant between FCGR1A and diverse immune cells varied substantially in different cancer types. FCGR1A had the highest correlations with DCs among CESC, KIRC, and SKCM, which were 0.682, 0.704, and 0.754, respectively. In CHOL, FCGR1A was more closely related to neutrophils (cor = 0.778). We also analyzed the correlation between FCGR1A and immune marker genes of various immune cells. The results showed that FCGR1A was notably related to most immune marker genes in CESC, CHOL, KIRC, and SKCM after purity adjustment (Table 1). And FCGR1A was strongly correlated with immune marker genes of some immune cells, which mainly concentrated in T cells, monocytes, M2 macrophages, and DCs. More specifically, immune marker genes included CD3E, CD2, CD86, CD115, CD163, VSIG4, MS4A4A, HLA-DPB1, HLA-DRA, HLA-DPA1, and ITGAX (cor ≥ 0.6). We also validated the relationship between these immune marker genes and FCGR1A expression in GEPIA and obtained similar results (Table 2). In addition, the strong correlation between FCGR1A and immune marker genes predominantly focused on CESC, KIRC, and SKCM including T cell, CD8+ T cell, monocyte, TAM, M2 macrophage, DC, TH1, and T cell exhaustion. Particularly, TIM-3 of the T cell exhaustion was strongly associated with CESC (cor = 0.887) and SKCM (cor = 0.934). CD86 also showed a close relation with four cancers, especially in SKCM (cor = 0.860). Nevertheless, it indicated very weak or even no correlation with four cancers in B cells, M1 macrophages, and NK cells.
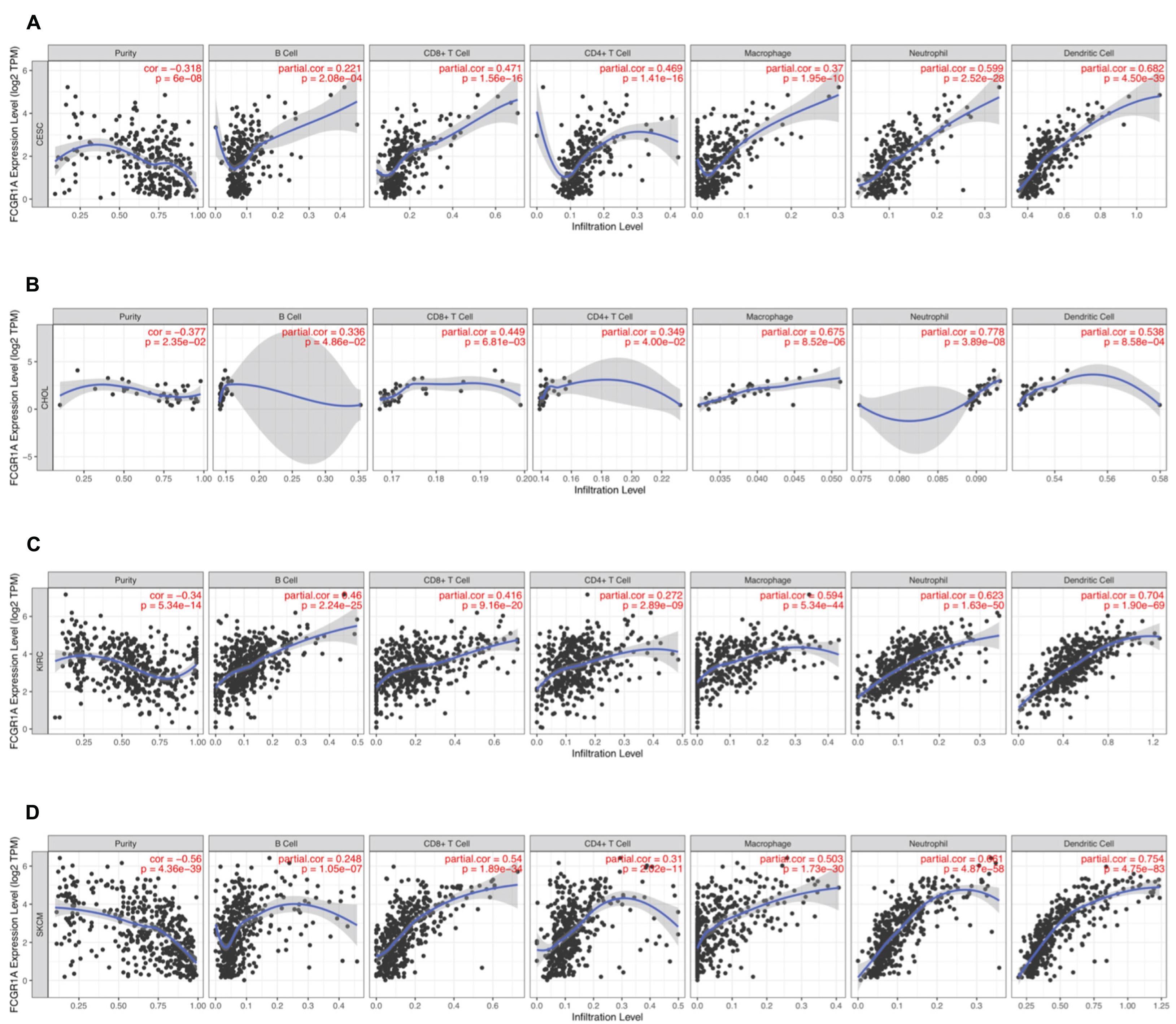
Figure 5. Correlation of FCGR1A expression with immune infiltration level in CESC, CHOL, KIRC, and SKCM. (A) CESC, (B) CHOL, (C) KIRC, and (D) SKCM.
Relevance between somatic CNV and immune infiltration using the SNCA module in TIMER differed among four cancers. Studies showed that arm-level deletion in KIRC was significantly associated with the infiltration of B cells, CD8+ T cells, CD4+ T cells, macrophages, neutrophils, and DCs (P < 0.05). Moreover, high amplification was related to the level of macrophage infiltration (P < 0.05). In SKCM, arm-level gain was prominently correlated with six immune cells, whereas arm-level deletion was related only to B cells, CD4+ T cells, macrophages, and DCs (P < 0.05). No association was observed between CNV and immune cell infiltration in the other two cancer types (P > 0.05) (Figure 6).
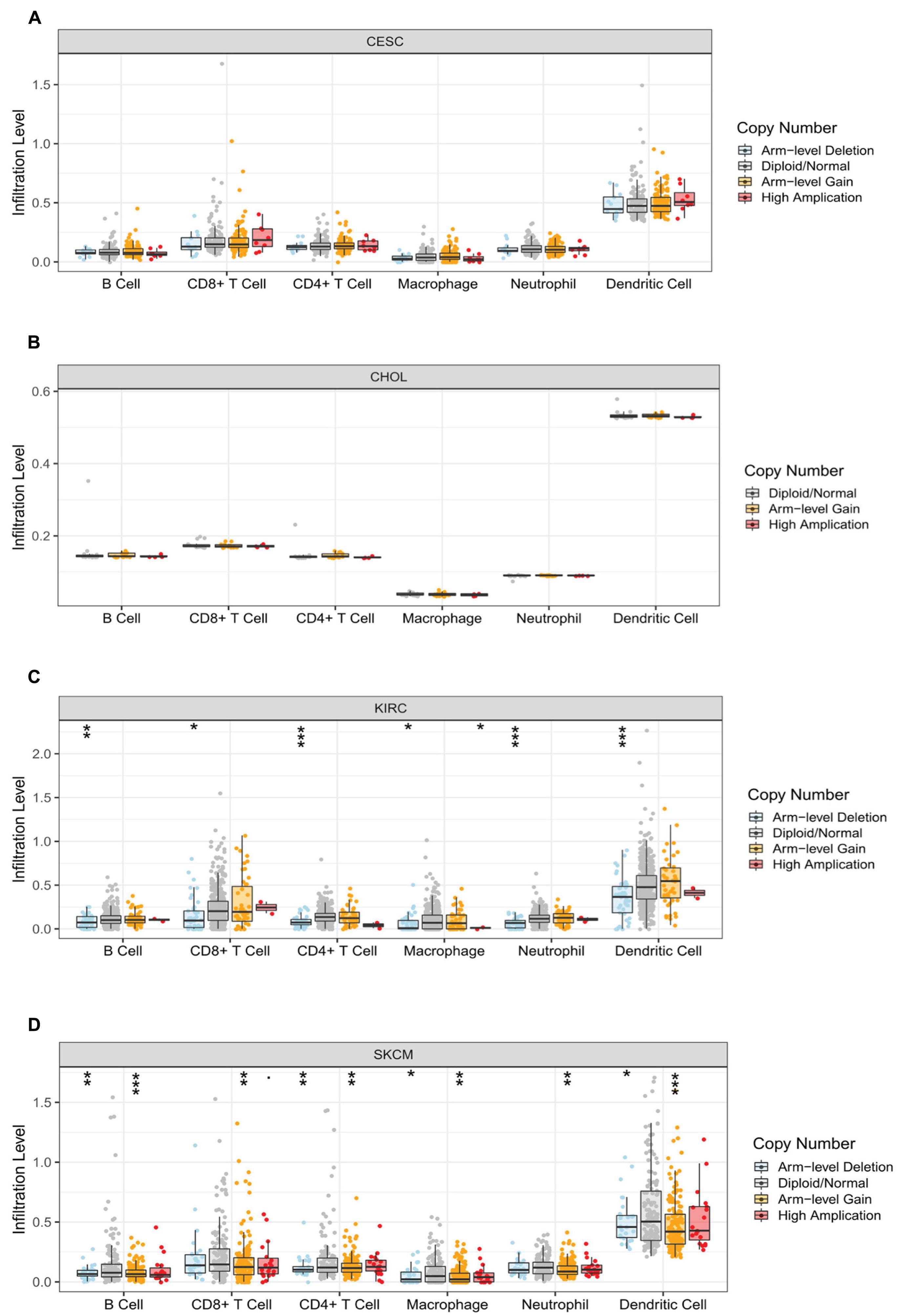
Figure 6. Correlation between somatic copy number variation and immune infiltration levels of six immune cells in four cancers. (A) CESC, (B) CHOL, (C) KIRC, and (D) SKCM (*P < 0.05, **P < 0.01, ***P < 0.001).
Function Analysis of the PPI Network
STRING was utilized to construct the PPI network, and GO and KEGG analyses were performed to identify the enrichment of FCGR1A in GO terms and metabolic pathways (Table 3). The GO enrichment analysis consisted of three parts: GO biological process (GO-BP), GO molecular function (GO-MF), and GO cellular component (GO-CC). GO-BP included 115 GO-terms, the most important of which were Fc receptor signaling pathway and immune response–regulating cell surface receptor signaling pathway. GO-MF and GO-CC contained 17 and 21 GO terms, respectively. GO-BF contained 17 items, and FCGR1A was largely involved in phosphotyrosine residue binding, immunoglobulin binding, and calcium ion binding. Meanwhile, FCGR1A was enriched for 21 GO-CC terms, and plasma membrane, whole membrane, and cytoplasmic vesicle were the top three categories. Eighteen items were included in the KEGG pathways analysis. The results indicated that FCGR1A was predominantly associated with Fc gamma R–mediated phagocytosis, osteoclast differentiation, and NK cell–mediated cytotoxicity. The PPI network displayed intricate relationship between FCGR1A and other genes in Figure 7. FCGR3A, SYK, and HCK were top three significant genes related to the FCGR1A according to the correlation score (Table 4). The relationship between FCGR3A and FCGR1A displayed highly significant positive correlation in four cancers (Supplementary Figure 1).
Discussion
FCGR1A (CD64) is the single high-affinity receptor of immunoglobulin G (IgG) in human. The expression of FCGR1A is closely related to immune inflammation. Although there are few extensive and systematical studies about CD64, its high sensitivity and specificity for the diagnosis of sepsis and systemic infection had been demonstrated, with a sensitivity of greater than 90% and specificity of 90–100% in adults and children (Hoffmann, 2009). Besides, elevated CD64 had been reported as a potential biomarker for the diagnosis of PFAPA (periodic fever, aphthous stomatitis, pharyngitis, and adenitis) syndrome (Yamazaki et al., 2014). FCGR1A is the encoding gene of CD64, and the up-regulation of FCGR1A leads to high expression of CD64. CD64 plays an important role in resistance to pathogen invasion through antibody-dependent cell-mediated cytotoxicity (ADCC), cell phagocytosis, and clearance of immune complexes. In normal conditions, CD64 is mainly expressed in monocytes, macrophages, and DCs but lower expression in neutrophils and lymphocytes. When the body or cells are stimulated by endotoxin or immune factors, the expression level of CD64 in the neutrophils increases, and FCGR1A is up-regulated. Here, we studied the association between FCGR1A and the prognosis of different cancers. High expression of FCGR1A exhibited a superior survival in CESC, CHOL, and SKCM, but associated with a poor prognosis in KIRC. Moreover, our research showed that immune infiltration levels and various immune marker genes were closely related to the FCGR1A expression levels. Thus, we can infer that FCGR1A can be regarded as a potential biomarker of cancers and play a role in tumor immunology.
We explored the expression levels of FCGR1A in 33 cancer types using TCGA data in GEPIA. The significant differential expression of FCGR1A was observed in many cancer types. In contrast to the normal tissue, FCGR1A expressed highly in BLCA, BRCA, CESC, CHOL, COAD, ESCA, GBM, HNSC, KIRC, KIRP, LAML LGG, OV, PAAD, PRAD, READ, SKCM, STAD, TGCT, THCA, UCEC, and UCS, but lowly in LUAD, LUSC, and THYM. Moreover, FCGR1A expression levels were significantly correlated with the OS of CESC, CHOL, KIRC, and SKCM and had notable differences between tumor and normal tissues in these four cancers. Besides, FCGR1A expression in KIRC and SKCM showed conspicuous discrepancies at different stages. Taken together with the above results, FCGR1A was strongly confirmed as a prognostic biomarker in CESC, CHOL, KIRC, and SKCM.
This study also indicated that FCGR1A was associated with multiple levels of immune infiltration in cancers. FCGR1A had the strongest correlation with DCs among six tumor-infiltrating immune cells in CESC, KIRC, and SKCM. It had higher relevancy with neutrophils in CHOL as well. Furthermore, significant positive relevance between FCGR1A expression and six immune cells was discovered in four cancers. CD3E, CD2, CD86, CD163, VSIG4, MS4A4A, HLA-DPB1, HLA-DRA, HLA-DPA1, and TIM-3 were found to be mainly gathering in T cells, monocytes, macrophages, and DCs were significantly correlated with FCGR1A. Similar results were validated in GEPIA. Therefore, the association of FCGR1A with diverse immune cell marker genes suggested its role in regulating tumor immunology in CESC, CHOL, KIRC, and SKCM. M1 macrophage gene markers (such as NOS2 and IRF5) had weak or no correlation with FCGR1A expression, while M2 macrophage markers CD163, VSIG4, and MS4A4A had strong correlations. These consequences indicated the potential regulatory role of FCGR1A in the polarization of TAMs. The increased expression of FCGR1A was positively correlated with the expression of Tregs and T cell exhaustion markers (FOXP3, CCR8, PD-1, Tim-3, and LAG3), suggesting that FCGR1A may play a role in the activation of Tregs and induction of T cell exhaustion. The FCGR1A was moderately or strongly associated with most immune marker genes in DCs, which can induce immune memory responses in cancer and promote anti-tumor immunity. Thus, the relationship between FCGR1A expression and the infiltration level of DCs may predict the prognosis of the disease and the response to therapies. TIM-3 is a vital surface protein on T cell exhaustion (Huang et al., 2015) and is highly correlated with FCGR1A expression in CESC and SKCM. Literature had reported that patients with high expression of TIM-3 had high metastatic potential, advanced cancer grades, and shorter OS than those with low expression in cervical cancer (Cao et al., 2016). And some studies had pointed out that TIM-3 is an immunomodulatory molecule in melanoma cells (Wiener et al., 2007). Besides, FCGR1A was positively correlated with T helper cells (such as TH1) and may be involved in the regulatory role of T cells. Taken together, FCGR1A plays a crucial role in the activation and regulation of immune cells in CESC, CHOL, KIRC, and SKCM based on the above evidence.
To have a better understanding of FCGR1A, GO enrichment and KEGG pathway analysis was carried out. FCGR1A was involved in various biological process, molecular function, and cellular component, which mainly includes Fc receptor signaling pathway, immune response–regulating cell surface receptor signaling pathway, phosphotyrosine residue binding, immunoglobulin binding, plasma membrane, whole membrane, etc. KEGG analysis was used to identify significant signaling pathways. Among its related pathways, Fc gamma R–mediated phagocytosis and osteoclast differentiation were the most important. According to the PPI network and correlation score, FCGR3A, SYK, and HCK were the most relevant neighboring genes. FCGR3A (low-affinity immunoglobulin gamma Fc region receptor III-A) was the receptor for the Fc region of IgG and bound complexed or aggregated IgG and monomeric IgG. It mainly mediated ADCC and other antibody-dependent responses (Mahaweni et al., 2018). SYK was a non-receptor tyrosine kinase, which mediates signal transduction downstream of transmembrane receptors including classical immunoreceptors (Mócsai et al., 2010). HCK was found in hematopoietic cells that transmitted signal from cell surface receptors and regulated innate immune responses. It also acted downstream of receptors that bound the Fc region of immunoglobulins, such as FCGR1A, FCGR2A, CSF3R, etc. (Yang et al., 2016). These three genes were all demonstrated to be associated with various cancer types or drug responses (Fueyo et al., 2018; Magnes et al., 2018; Roseweir et al., 2019). Our findings also confirmed that FCGR3A had highly significant positive associations with FCGR1A in four cancers. This evidence may help us infer the correlation between FCGR1A and cancers. In addition, we sought to investigate the relationship between FCGR1A and some genes that were confirmed to be related to cancers. Here, we took CASS4 (HEPL) as an example. CASS4 (Cas scaffold protein family member 4) was a protein coding gene and played a role in tyrosine kinase-base signaling related to cell adhesion and cell spreading, as well as regulating PTK2/FAK1 activity and focal adhesion integrity (Singh et al., 2008). The published research showed that CASS4 overexpression increased cell spreading and FAK activation. And it may be most in connection with the normal function of lung and hematopoietic system. We explored the correlation between FCGR1A and CASS4 in various cancer types through TIMER database. The results indicated that FCGR1A and CASS4 showed positive correlations in all cancer types including the four cancers we studied (P < 0.05, ρ > 0) (Supplementary Figure 2). Meanwhile, genemania4 was used to construct the PPI network (Supplementary Figure 3). Although there was no direct correlation between CASS4 and FCGR1A, these two genes were both associated with CD68, CCR1, CD300C, LILRA5, and PLEKHS1. The underlying mechanisms for gene-specific interplay among these two genes may be worthy of further investigation.
Several studies on FCGR1A (CD64) mainly focused on the diagnosis and distinction of infectious diseases, but rarely on cancer. However, an experimental study published in 2013 pointed out that FcRI (CD64) could mediate the protective effect of anti-tumor antibodies on melanoma metastasis and may also have a protective effect on solid tumors (Mancardi et al., 2013). In addition, CD64 is often used in combination with C-reactive protein (CRP), procalcitonin (PCT), and other inflammatory markers for diagnosis (Yeh et al., 2019). Multiple studies have demonstrated the predictive value of CRP in cancer outcomes. They showed that CRP was generally 1.3-fold higher than normal level in all cancer types. In lung cancer, CRP displayed more than 2-fold higher than normal value and increased approximately 80% risk of early mortality (Allin and Nordestgaard, 2011). Moreover, elevated CRP at diagnosis was associated with poor prognosis in breast cancer (Allin et al., 2011). A study proposed that PCT was closely related to the diagnosis and outcome of lung cancer (Pardo-Cabello and Manzano-Gamero, 2019). Therefore, infectious indicators are also of great value in cancer diagnosis and prognosis prediction and might be a novel promising biomarker. However, at present, insufficient information is available about FCGR1A; further experimental and clinical studies should be conducted to validate our findings.
Conclusion
In summary, high FCGR1A expression leads to different outcomes in diverse cancers. It is associated with better prognosis in CESC, CHOL, and SKCM besides CHOL. Meanwhile, FCGR1A is positively or even strongly correlated with immune cells and various immune cell marker genes in four cancer types, such as CD2, CD3E, CD86, CD163, VSIG4, MS4A4A, HLA-DPB1, HLA-DRA, HLA-DPA1, ITGAX, and TIM-3 in T cell, monocyte, M2 macrophage, DC, TH1, and T cell exhaustion. Thus, FCGR1A may contribute to the activation and regulation of the aforementioned immune cells to varying degrees. FCGR1A may play an important role in immune cell infiltration and serve as a prognostic biomarker for four cancers, especially in SKCM. Also, FCGR1A is involved in many pathophysiological processes and is closely related to FCGR3A, SYK, and HCK in various cancers. It seems that many questions need to be addressed by future research.
Data Availability Statement
Publicly available datasets were analyzed in this study. This data can be found here: http://gepia2.cancer-pku.cn/#general and https://cistrome.shinyapps.io/timer/.
Author Contributions
J-lX: conceptualization, formal analysis and investigation, and writing-original draft preparation. YG: conceptualization, methodology, writing-review and editing, and supervision. Both authors contributed to the article and approved the submitted version.
Funding
This study was supported by the National Natural Science Foundation of China (Grant No: 81973805); Zhejiang Provincial TCM Science and Technology Project (Grant No: 2015ZA088); and Zhejiang Provincial Project for the Key Discipline of Traditional Chinese Medicine (YG, No: 2017-XK-A09, http://www.zjwjw.gov.cn/).
Conflict of Interest
The authors declare that the research was conducted in the absence of any commercial or financial relationships that could be construed as a potential conflict of interest.
Acknowledgments
This manuscript has been released as a pre-print at Research Square (Xu and Guo, 2020).
Supplementary Material
The Supplementary Material for this article can be found online at: https://www.frontiersin.org/articles/10.3389/fmolb.2020.581615/full#supplementary-material
Footnotes
- ^ http://gepia.cancer-pku.cn/index.html
- ^ https://cistrome.shinyapps.io/timer/
- ^ https://string-db.org/
- ^ http://genemania.org
References
Allin, K. H., and Nordestgaard, B. G. (2011). Elevated C-reactive protein in the diagnosis, prognosis, and cause of cancer. Crit. Rev. Clin. Lab. Sci. 48, 155–170. doi: 10.3109/10408363.2011.599831
Allin, K. H., Nordestgaard, B. G., Flyger, H., and Bojesen, S. E. (2011). Elevated pre-treatment levels of plasma C-reactive protein are associated with poor prognosis after breast cancer: a cohort study. Breast Cancer Res. 13:R55. doi: 10.1186/bcr2891
Barata, P. C., and Rini, B. I. (2017). Treatment of renal cell carcinoma: current status and future directions. CA Cancer J. Clin. 67, 507–524. doi: 10.3322/caac.21411
Bardhan, K., Anagnostou, T., and Boussiotis, V. A. (2016). The PD1:PD-L1/2 pathway from discovery to clinical implementation. Front. Immunol. 7:550. doi: 10.3389/fimmu.2016.00550
Bray, F., Ferlay, J., Soerjomataram, I., Siegel, R. L., Torre, L. A., and Jemal, A. (2018). Global cancer statistics 2018: GLOBOCAN estimates of incidence and mortality worldwide for 36 cancers in 185 countries. CA Cancer J. Clin. 68, 394–424. doi: 10.3322/caac.21492
Cao, Y., Zhou, X., Huang, X., Li, Q., Gao, L., Jiang, L., et al. (2016). Correction: tim-3 expression in cervical cancer promotes tumor metastasis. PLoS One 11:e0152830. doi: 10.1371/journal.pone.0152830
Cid, J., Aguinaco, R., Sánchez, R., García-Pardo, G., and Llorente, A. (2010). Neutrophil CD64 expression as marker of bacterial infection: a systematic review and meta-analysis. J. Infect. 60, 313–319. doi: 10.1016/j.jinf.2010.02.013
Cohen, P. A., Jhingran, A., Oaknin, A., and Denny, L. (2019). Cervical cancer. Lancet 393, 169–182. doi: 10.1016/S0140-6736(18)32470-X
Crosbie, E. J., Einstein, M. H., Franceschi, S., and Kitchener, H. C. (2013). Human papillomavirus and cervical cancer. Lancet 382, 889–899. doi: 10.1016/S0140-6736(13)60022-7
Danaher, P., Warren, S., Dennis, L., D’Amico, L., White, A., Disis, M. L., et al. (2017). Gene expression markers of tumor infiltrating leukocytes. J. Immunother. Cancer 5:18. doi: 10.1186/s40425-017-0215-8
Fueyo, J., Alonso, M. M., Parker Kerrigan, B. C., and Gomez-Manzano, C. (2018). Linking inflammation and cancer: the unexpected SYK world. Neuro. Oncol. 20, 582–583. doi: 10.1093/neuonc/noy036
Hasita, H., Komohara, Y., Okabe, H., Masuda, T., Ohnishi, K., Lei, X. F., et al. (2010). Significance of alternatively activated macrophages in patients with intrahepatic cholangiocarcinoma. Cancer Sci. 101, 1913–1919. doi: 10.1111/j.1349-7006.2010.01614.x
Hoffmann, J. J. (2009). Neutrophil CD64: a diagnostic marker for infection and sepsis. Clin. Chem. Lab. Med. 47, 903–916. doi: 10.1515/CCLM.2009.224
Huang, Y. H., Zhu, C., Kondo, Y., Anderson, A. C., Gandhi, A., Russell, A., et al. (2015). CEACAM1 regulates TIM-3-mediated tolerance and exhaustion. Nature 517, 386–390. doi: 10.1038/nature13848
Hulett, M. D., and Hogarth, P. M. (1998). The second and third extracellular domains of FcgammaRI (CD64) confer the unique high affinity binding of IgG2a. Mol. Immunol. 35, 989–996. doi: 10.1016/s0161-5890(98)00069-8
Kaneda, M. M., Cappello, P., Nguyen, A. V., Ralainirina, N., Hardamon, C. R., Foubert, P., et al. (2016). Macrophage PI3Kγ drives pancreatic ductal adenocarcinoma progression. Cancer Discov. 6, 870–885. doi: 10.1158/2159-8290.CD-15-1346
Kern, W., Kohlmann, A., Schoch, C., Schnittger, S., and Haferlach, T. (2006). Comparison of mRNA abundance quantified by gene expression profiling and percentage of positive cells using immunophenotyping for diagnostic antigens in acute and chronic leukemias. Cancer 107, 2401–2407. doi: 10.1002/cncr.22251
Khan, S. A., Tavolari, S., and Brandi, G. (2019). Cholangiocarcinoma: epidemiology and risk factors. Liver Int. 39(Suppl. 1), 19–31. doi: 10.1111/liv.14095
Kiyoshi, M., Caaveiro, J. M., Kawai, T., Tashiro, S., Ide, T., Asaoka, Y., et al. (2015). Structural basis for binding of human IgG1 to its high-affinity human receptor FcγRI. Nat. Commun. 6:6866. doi: 10.1038/ncomms7866
Lee, B. S., Lee, S. H., Son, J. H., Jang, D. K., Chung, K. H., Lee, Y. S., et al. (2016). Neutrophil-lymphocyte ratio predicts survival in patients with advanced cholangiocarcinoma on chemotherapy. Cancer Immunol. Immunother. 65, 141–150. doi: 10.1007/s00262-015-1780-7
Li, B., Severson, E., Pignon, J. C., Zhao, H., Li, T., Novak, J., et al. (2016). Comprehensive analyses of tumor immunity: implications for cancer immunotherapy. Genome Biol. 17:174. doi: 10.1186/s13059-016-1028-7
Li, T., Fan, J., Wang, B., Traugh, N., Chen, Q., Liu, J. S., et al. (2017). TIMER: a web server for comprehensive analysis of tumor-infiltrating immune cells. Cancer Res. 77, e108–e110. doi: 10.1158/0008-5472.CAN-17-0307
Lu, J., and Sun, P. D. (2015). Structural mechanism of high affinity FcγRI recognition of immunoglobulin G. Immunol. Rev. 268, 192–200. doi: 10.1111/imr.12346
Magnes, T., Melchardt, T., Hufnagl, C., Weiss, L., Mittermair, C., Neureiter, D., et al. (2018). The influence of FCGR2A and FCGR3A polymorphisms on the survival of patients with recurrent or metastatic squamous cell head and neck cancer treated with cetuximab. Pharmacogenom. J. 18, 474–479. doi: 10.1038/tpj.2017.37
Mahaweni, N. M., Olieslagers, T. I., Rivas, I. O., Molenbroeck, S. J. J., Groeneweg, M., Bos, G. M. J., et al. (2018). A comprehensive overview of FCGR3A gene variability by full-length gene sequencing including the identification of V158F polymorphism. Sci. Rep. 8:15983. doi: 10.1038/s41598-018-34258-1
Mancardi, D. A., Albanesi, M., Jönsson, F., Iannascoli, B., Van Rooijen, N., Kang, X., et al. (2013). The high-affinity human IgG receptor FcγRI (CD64) promotes IgG-mediated inflammation, anaphylaxis, and antitumor immunotherapy. Blood 121, 1563–1573. doi: 10.1182/blood-2012-07-442541
Mangalam, A. K., and Yadav, R. (2019). Utility of CD64 expression on neutrophils as a marker to differentiate infectious versus noninfectious disease flares in autoimmune disorders. Indian J. Rheumatol. 14, 9–11. doi: 10.4103/0973-3698.254192
Mócsai, A., Ruland, J., and Tybulewicz, V. L. (2010). The SYK tyrosine kinase: a crucial player in diverse biological functions. Nat. Rev. Immunol. 10, 387–402. doi: 10.1038/nri2765
Olbryt, M. (2019). Molecular background of skin melanoma development and progression: therapeutic implications. Postepy Dermatol. Alergol. 36, 129–138. doi: 10.5114/ada.2019.84590
Pardo-Cabello, A. J., and Manzano-Gamero, V. (2019). Small cell lung cancer elevates procalcitonin levels in the absence of infection. Lung Cancer 134, 272–273. doi: 10.1016/j.lungcan.2019.06.018
Razumilava, N., and Gores, G. J. (2014). Cholangiocarcinoma. Lancet 383, 2168–2179. doi: 10.1016/S0140-6736(13)61903-0
Roseweir, A. K., Powell, A. G. M. T., Horstman, S. L., Inthagard, J., Park, J. H., McMillan, D. C., et al. (2019). Src family kinases, HCK and FGR, associate with local inflammation and tumour progression in colorectal cancer. Cell Signal. 56, 15–22. doi: 10.1016/j.cellsig.2019.01.007
Shen, M., Hu, P., Donskov, F., Wang, G., Liu, Q., and Du, J. (2014). Tumor-associated neutrophils as a new prognostic factor in cancer: a systematic review and meta-analysis. PLoS One 9:e98259. doi: 10.1371/journal.pone.0098259
Shenenberger, D. W. (2012). Cutaneous malignant melanoma: a primary care perspective. Am. Fam. Phys. 85, 161–168.
Siemers, N. O., Holloway, J. L., Chang, H., Chasalow, S. D., Ross-MacDonald, P. B., Voliva, C. F., et al. (2017). Genome-wide association analysis identifies genetic correlates of immune infiltrates in solid tumors. PLoS One 12:e0179726. doi: 10.1371/journal.pone.0179726
Singh, M. K., Dadke, D., Nicolas, E., Serebriiskii, I. G., Apostolou, S., Canutescu, A., et al. (2008). A novel Cas family member, HEPL, regulates FAK and cell spreading. Mol. Biol. Cell 19, 1627–1636. doi: 10.1091/mbc.e07-09-0953
Sinha, R., Winer, A. G., Chevinsky, M., Jakubowski, C., Chen, Y. B., Dong, Y., et al. (2017). Analysis of renal cancer cell lines from two major resources enables genomics-guided cell line selection. Nat. Commun. 8:15165. doi: 10.1038/ncomms15165
Tan, D. W., Fu, Y., Su, Q., Guan, M. J., Kong, P., Wang, S. Q., et al. (2016). Prognostic significance of neutrophil to lymphocyte ratio in oncologic outcomes of cholangiocarcinoma: a meta-analysis. Sci. Rep. 6:33789. doi: 10.1038/srep33789
Tang, Z., Li, C., Kang, B., Gao, G., Li, C., and Zhang, Z. (2017). GEPIA: a web server for cancer and normal gene expression profiling and interactive analyses. Nucleic Acids Res. 45, W98–W102. doi: 10.1093/nar/gkx247
Thanee, M., Loilome, W., Techasen, A., Namwat, N., Boonmars, T., Pairojkul, C., et al. (2015). Quantitative changes in tumor-associated M2 macrophages characterize cholangiocarcinoma and their association with metastasis. Asian Pac. J. Cancer Prev. 16, 3043–3050. doi: 10.7314/apjcp.2015.16.7.3043
Thepen, T., Huhn, M., Melmer, G., Tur, M. K., and Barth, S. (2009). Fcgamma receptor 1 (CD64), a target beyond cancer. Curr. Pharm. Des. 15, 2712–2718. doi: 10.2174/138161209788923967
Wiener, Z., Kohalmi, B., Pocza, P., Jeager, J., Tolgyesi, G., Toth, S., et al. (2007). TIM-3 is expressed in melanoma cells and is upregulated in TGF-beta stimulated mast cells. J. Invest. Dermatol. 127, 906–914. doi: 10.1038/sj.jid.5700616
Xu, J., and Guo, Y. (2020). FCGR1A serves as a novel biomarker and correlates with immune infiltration in four cancer types. Research Square [Preprint], doi: 10.21203/rs.3.rs-38062/v1
Yamazaki, T., Hokibara, S., Shigemura, T., Kobayashi, N., Honda, K., Umeda, Y., et al. (2014). Markedly elevated CD64 expressions on Neutrophils and Monocytes are useful for diagnosis of periodic fever, Aphthous stomatitis, Pharyngitis, and cervical adenitis (PFAPA) syndrome during flares. Clin. Rheumatol. 33, 677–683. doi: 10.1007/s10067-014-2542-1
Yang, G., Buhrlage, S. J., Tan, L., Liu, X., Chen, J., Xu, L., et al. (2016). HCK is a survival determinant transactivated by mutated MYD88, and a direct target of ibrutinib. Blood 127, 3237–3252. doi: 10.1182/blood-2016-01-695098
Yeh, C. F., Wu, C. C., Liu, S. H., and Chen, K. F. (2019). Comparison of the accuracy of neutrophil CD64, procalcitonin, and C-reactive protein for sepsis identification: a systematic review and meta-analysis. Ann. Intensive Care 9:5. doi: 10.1186/s13613-018-0479-2
Zhang, B., Wu, Q., Wang, Z., Xu, R., Hu, X., Sun, Y., et al. (2019). The promising novel biomarkers and candidate small molecule drugs in kidney renal clear cell carcinoma: evidence from bioinformatics analysis of high-throughput data. Mol. Genet. Genom. Med. 7:e607. doi: 10.1002/mgg3.607
Keywords: biomarker, prognosis, tumor-infiltrating, timer, GEPIA, FCGR1A
Citation: Xu J-l and Guo Y (2020) FCGR1A Serves as a Novel Biomarker and Correlates With Immune Infiltration in Four Cancer Types. Front. Mol. Biosci. 7:581615. doi: 10.3389/fmolb.2020.581615
Received: 23 July 2020; Accepted: 03 November 2020;
Published: 03 December 2020.
Edited by:
Adriana Albini, MultiMedica (IRCCS), ItalyReviewed by:
Mahendra K. Singh, University of Miami, United StatesRamya Sivakumar, University of Washington, United States
Copyright © 2020 Xu and Guo. This is an open-access article distributed under the terms of the Creative Commons Attribution License (CC BY). The use, distribution or reproduction in other forums is permitted, provided the original author(s) and the copyright owner(s) are credited and that the original publication in this journal is cited, in accordance with accepted academic practice. No use, distribution or reproduction is permitted which does not comply with these terms.
*Correspondence: Yong Guo, guoyong1047@gmail.com; orcid.org/0000-0002-0202-3359