- 1Department of Pharmacy, The Second Affiliated Hospital of Chongqing Medical University, Chongqing, China
- 2School of Pharmaceutical Sciences, Chongqing Key Laboratory of Natural Product Synthesis and Drug Research, Chongqing University, Chongqing, China
Therapeutics targeting cytokines such as the oncostatin M (OSM)-mediated inflammation represent a potential strategy for the treatment of inflammatory bowel disease (IBD). Despite the investigation of the specific role of the interactions between OSM and the receptor (OSMR) in IBD pathogenesis, the 3D structure of the OSM–OSMR complex remains elusive. In this work, the interaction mode between OSM and OSMR at atomic level was predicted by computational simulation approach. The interaction domain of the OSMR was built with the homology modeling method. The near-native structure of the OSM–OSMR complex was obtained by docking, and long-time scale molecular dynamics (MD) simulation in an explicit solvent was further performed to sample the conformations when OSM binds to the OSMR. After getting the equilibrated states of the simulation system, per-residue energy contribution was calculated to characterize the important residues for the OSM–OSMR complex formation. Based on these important residues, eight residues (OSM: Arg100, Leu103, Phe160, and Gln161; OSMR: Tyr214, Ser223, Asp262, and Trp267) were identified as the “hot spots” through computational alanine mutagenesis analysis and verified by additional MD simulation of R100A (one of the identified “hotspots”) mutant. Moreover, six cavities were detected at the OSM–OSMR interface through the FTMap analysis, and they were suggested as important binding sites. The predicted 3D structure of the OSM–OSMR complex and the identified “hot spots” constituting the core of the binding interface provide helpful information in understanding the OSM–OSMR interactions, and the detected sites serve as promising targets in designing small molecules to block the interactions.
Introduction
Inflammatory bowel diseases (IBDs) are complex chronic inflammatory conditions of the gastrointestinal tract that are driven by perturbed signal pathways of cytokines such as tumor necrosis factor (TNF)-α and IL-6 (Neurath, 2014). Nowadays, anti-TNF antibodies (such as infliximab and golimumab) are mainstay therapies for IBD (Choi et al., 2017). However, there are still more than 40% of patients who are non-responsive to anti-TNF agents, making the discovery of alternative therapeutic targets a priority (Kim et al., 2017). One of those potential targets, oncostatin M (OSM)-mediated inflammation, has gained a lot of interest (Verstockt et al., 2019). It is found that high pretreatment expression of OSM is strongly associated with failure of anti-TNF therapy of patients with IBD, which revealed the role of the receptor (OSMR) as part of a unique pathway that contributes to the chronicity of intestinal inflammation (West et al., 2017).
OSM belongs to the IL-6 family, and the activation of the OSM signal pathway is highly determined by the high affinity of OSM to the receptor (OSMR) (Adrian-Segarra et al., 2018a,b). The crystal structure of OSM reveals that the protein comprises four α helices ranging from 15 to 22 amino acids in length (termed A, B, C, and D) and linked by polypeptide loops (Figure 1A) (Deller et al., 2000). The OSMR is a member of the IL-6 receptor family that transduces signaling events of OSM (Yu et al., 2019). Currently, available antibodies, such as GSK315234 and GSK2330811, have already been proven to affect the OSM signal (Verstockt et al., 2019). Although neutralizing OSM antibodies are being developed and should be considered as a novel proof-of-concept trial in IBD patients (West et al., 2017), these developed biological medicines are large, complex, and relatively fragile molecules, which make them difficult and expensive to produce and administer on a large scale (Monaco et al., 2015).
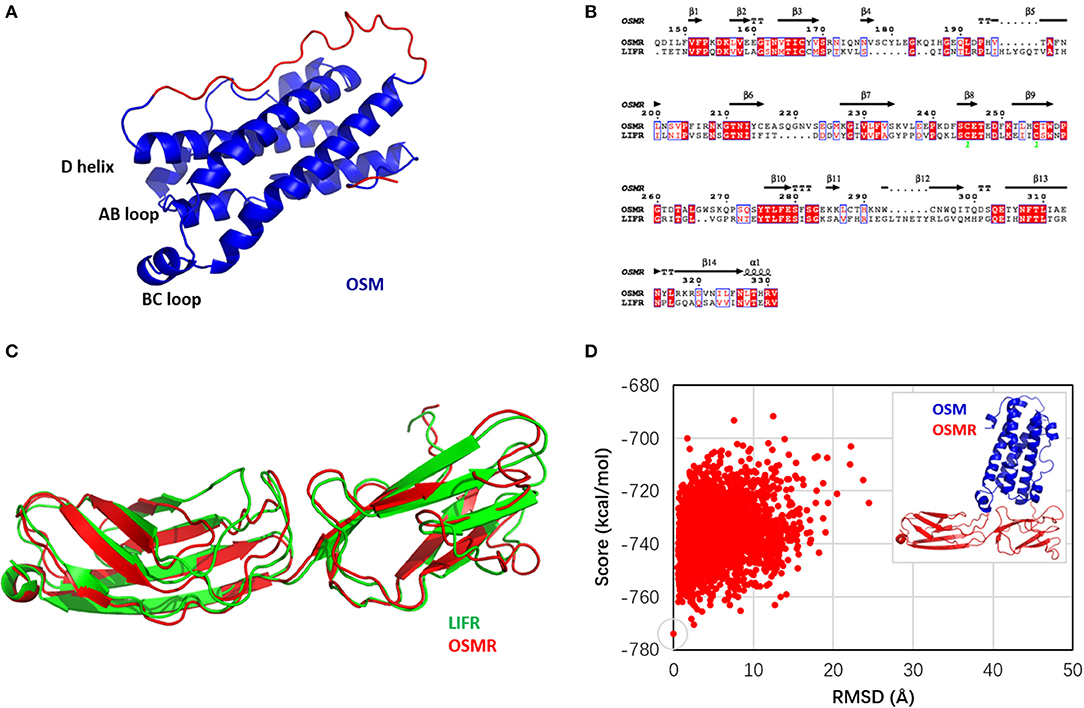
Figure 1. (A) Structure of oncostatin M (OSM); the modeled fragments are colored in red. (B) Sequence alignment between oncostatin M receptor (OSMR) and leukemia inhibitory factor receptor (LIFR). (C) Structural alignment of OSMR homology model (red) and LIFR crystal structure (green). (D) Docking funnel of OSM and OSMR. Inset: the top scoring conformation as near-native OSM–OSMR structure.
In recent years, development of small molecule modulators targeting protein–protein interactions (PPIs) has emerged as a promising therapeutic intervention in complex diseases (Nero et al., 2014; Nim et al., 2016; Weng et al., 2019). In selecting biologically relevant protein–protein interfaces, the availability of computer-aided drug design (CADD) approach has led to the discovery of small molecules either stabilizing or disrupting the biological processes (Arkin et al., 2014; Laraia et al., 2015). The critical role for OSM in antipathogen immunity has not been described, and targeting OSM–OSMR may offer inhibition of the inflammatory pathology while preserving protective immunity (Verstockt et al., 2019). These hypotheses stimulate the idea of identification of small molecular inhibitors against the OSM–OSMR interface, which might provide safer and more broadly effective alternatives to conventional antibodies targeting monomeric macromolecules. To discover ligands specifically disrupting the OSM–OSMR interface, the information of the protein–protein interactions is needed. Unfortunately, the 3D structure of the OSM–OSMR complex remains elusive (Kim et al., 2017). It is of paramount importance to understand the details of the OSM and OSMR complex formation as well as the potential binding site between the protein–protein interface.
In this work, molecular simulation approaches aimed at filling the aforementioned gap were performed to accelerate the discovery of small molecules targeting OSM–OSMR. Starting from the crystal structure of OSM (Deller et al., 2000) and the model of the OSMR [a protein-binding region was built using the leukemia inhibitory factor receptor (LIFR) crystal structure (Huyton et al., 2007) as a template], the near-native conformation of the OSM–OSMR complex was obtained through protein–protein docking. The docking conformation was further sampled through long-time scale (1 μs) molecular dynamics (MD) simulation to get the equilibrated binding states. Based on the simulation trajectory, per-residue binding free energy decomposition (Tu et al., 2018; Wang et al., 2019) and computational alanine scanning (CAS) (Huo et al., 2002) analysis were carried out to identify the protein–protein interface “hotspots.” Using one of the identified “hotspots” (Arg100) as an example, an additional 500 ns of MD simulation was performed to investigate the stability of the R100A mutant complex. Finally, the “hotspots” were mapped to the seven binding sites located at the OSM–OSMR interface detected using FTMap (Kozakov et al., 2011), and three of them were suggested as important target sites for future designs of small molecular modulators in the OSM–OSMR interaction.
Materials and Methods
Structure Preparation
Construction of OSM Missing Loop
The crystal structure and sequence of OSM were obtained from the PDB database (PDB code: 1EVS) (Deller et al., 2000). Residues from 1 to 3 and 135 to 155 (highlighted in red color in Table S1) were missing in the resolved crystal structure. The coordinates of the missing fragments of the OSM structure were constructed using the optimization-based approach (Fiser et al., 2000) in Modeler (Webb and Sali, 2016).
Homology Modeling of OSMR
The full-length sequence of the OSMR was obtained from the NCBI database (GenBank: AAI25210.1) (Strausberg et al., 2002). Then the sequence of the OSMR was submitted to search a template structure with the BLAST algorithm (Schaffer et al., 2001). Searching result showed that the sequence identity between the OSMR and LIFR was higher than 30%, especially in the protein-binding domain (57%). Therefore, based on the crystal structure (PDB code: 2Q7N) (Huyton et al., 2007) of the LIFR (residues from 201 to 383), 10 homology models of the OSMR protein-binding domain was constructed using Modeler (Webb and Sali, 2016).
Protein–Protein Docking
OSM–OSMR docking was performed using the protein docking module of the latest version of Rosetta (Alford et al., 2017). Before docking, the PDB structures of OSM and OSMR were first formed through the script of clean_pdb.py. The formed structures of the two proteins were refined by running the Rosetta relax protocol, and the PDB files consisting of refined OSM and OSMR were generated. Then, according to the knowledge of the residues of OSM for OSMR binding detected by site mutagenesis studies (Adrian-Segarra et al., 2018b), the generated two complexes were loaded into PyMOL (Schrödinger, 2010) and with OSM reoriented to contact with the OSMR. To ensure low-energy starting side-chain conformations for docking, further prepacking of the OSM and OSMR complexes were conducted. Finally, 10,000 poses were calculated for the OSM–OSMR interactions using the Monte Carlo (MC) refinement method (Gray et al., 2003), with the pre-packed conformation as a starting point.
Docking Funnel Analysis
With InterfaceAnalyzer mover in RosettaScripts (Fleishman et al., 2011), the RMSD was calculated from the heavy atoms of the interface residues (Table S2) using each pose of the top five scorers as a reference structure (Chaudhury et al., 2011). The docking funnel was then identified through plotting total_score against RMSD. Finally, the top scoring structure with the lowest RMSD was selected as the successful pose of the OSM–OSMR complex.
Molecular Dynamics Simulation
Molecular dynamics (MD) simulation was performed with GPU-accelerated PMEMD in AMBER14 (Babin et al., 2014). The selected near-native structure of OSM–OSMR from Rosetta docking was used as the initial conformation for MD simulation. The LEaP (Wang et al., 2006) was applied to assign AMBERff14SB force field parameters (Maier et al., 2015) for the two proteins, and two disulfide bonds in OSM and one disulfide bonds in the OSMR were identified and added. The complex was immersed into a rectangular periodic box of TIP3P (Hornak et al., 2006) water molecules, and the system was neutralized with two chloride ions. The distance between any protein atom and the edge of the box was set to 10 Å, and the prepared system contains 86,446 atoms per periodic cell. Starting from the representative snapshot of wild type OSM–OSMR, additional MD simulation was performed on the R100A complex using the same setup.
MM/GBSA Binding Free Energy
The binding free energy (ΔGtol) between OSM and OSMR was estimated by the end-point molecular mechanics generalized Born surface area (MM/GBSA) approach (Kollman et al., 2000) as below:
where ΔEvdW and ΔEele are the van der Waals and electrostatic interaction energies, and ΔGpol and ΔGnonpol are the polar and non-polar solvent energies, respectively. ΔEvdW and ΔEele were calculated using AMBER ff14SB (Maier et al., 2015) in the gas phase. ΔGpol was calculated by solving the GB equation (Onufriev et al., 2004) with the dielectric constants of solute and solvent set to 1 and 80, respectively. ΔGnonpol was calculated by ΔGnonpol = γ × SASA, where γ = 0.0072, and SASA is referred to the solvent-accessible area and determined using a water probe radius of 1.4 Å (Sitkoff et al., 1994).
To further analyze the energy contribution between OSM and OSMR at a per-residue basis (), the total binding free energy was decomposed by:
The definition of each term in Equation (2) is similar as in Equation (1), except that SASA was computed by recursively approximating a sphere around an atom, starting from an icosahedron (ICOSA) (Babin et al., 2014).
Computational Alanine Scanning Mutagenesis
Computational alanine scanning (CAS) mutagenesis was widely used to characterize the “hotspots” associated to protein–protein interactions (Huo et al., 2002). The whole process included the generation of mutated snapshots, and the binding free energy difference (ΔΔGcalc) between the wild type (WT) and mutant (MUT) complex is calculated below
where GWT andGMUT refer to the MM/GBSA binding free energy of the WT and MUT complexes, respectively. Snapshot(s) of the WT of OSM-OSM and LIF-LIFR complex were collected from the last 500-ns trajectory and the crystal structure 2Q7N (Huyton et al., 2007), respectively. Alanine mutation was generated by truncating the selected mutation residue at Cγ and by replacing Cγ with a hydrogen atom at a 1.09-Å distance from Cß along the direction of the Cγ-Cß bond (Huo et al., 2002).
Detection of Druggable Binding Sites
Based on the representative snapshot of the OSM–OSMR structure derived from the long-time simulation and the crystal structure of LIF–LIFR (Huyton et al., 2007), FTMap (Kozakov et al., 2011) was employed to detect the druggable binding site in the protein–protein interaction complexes. FTMap uses a fragment-based mapping algorithm that implements an efficient fast Fourier transform (FFT) correlation approach to search a global protein surface for potential druggable binding sites. The fragments include 16 small organic probe molecules (benzene, cyclohexane, ethane, ethanol, isopropanol, isobutanol, acetone, acetaldehyde, dimethyl ether, acetonitrile, urea, methylamine, phenol, benzaldehyde, acetamide, and N, N-dimethylformamide) of varying sizes, shapes, and polarities (Kozakov et al., 2015).
Results and Discussion
Modeled Structures of OSM and OSMR
The missing structures of OSM (Table S1), including the N-terminal fragment (1–3, AAI) and loop (135–155, SDTAEPTKAGRGASQPPTPTP), were built and refined using Modeler (Webb and Sali, 2016) because sequence identity between the loops of OSM and LIF (SKYHVGHVDVTYGPDTSGKDV) was only 10.3%. In addition, structural alignment indicated that the conformations of the two terminals that link the loops in the crystal structures of 1EVS and 2Q7N was significantly different (Figure S1). Therefore, the missing loop of OSM was predicted based on its own crystal structure 1EVS. Homology modeling approach in Modeler (Webb and Sali, 2016) was applied to provide the 3D structure of the OSMR binding domain (146–331) using the LIFR crystal structure (PDB code: 2Q7N) (Huyton et al., 2007) as a template. Figure 1B shows that the sequence identity between the OSMR and LIFR binding domain was 57%. As a result, 10 models were predicted for OSM and OSMR, respectively, and the model for each of them (Figures 1A,C) was selected by picking the structure with the best DOPE assessment score considering the Lennard–Jones potential and GBSA implicit solvent interaction (Shen and Sali, 2006).
Prediction of OSM–OSMR Interaction Profiles
The Near-Native Conformation of OSM–OSMR Complex
To predict the OSM–OSMR binding funnel, RosettaDock was used to sample 10,000 poses from the starting position. The starting position was estimated according to the knowledge of binding site residues identified by site mutagenesis studies (Adrian-Segarra et al., 2018b), as the presence of a docking funnel is considered to be the most robust measure of success in a docking simulation (Chaudhury et al., 2011). Here, the top five scorers of the OSM–OSMR complex were used as references to plot the docking score of all 10,000 poses as a function of RMSD (Figure S2). One of the top five structures presenting the most reasonable docking funnel, in which the near-native conformations consistently have better scores than the non-native conformations (Chaudhury et al., 2011), is shown in Figure 1D. Therefore, the top scoring structures with the lowest RMSD in Figure 1D was selected as the initial conformation of OSM–OSMR for further studies. In addition, given that the structure of OSM is very similar to that of LIF, and the OSMR is modeled using the LIFR as the template, the structure of the OSM–OSMR complex was modeled based on the crystal structure of the LIF–LIFR complex. The calculated RMSD between the template-based and docking structures of the OSM–OSMR was 3.37 Å, suggesting that the two modeled structures are very similar with each other (Figure S3A). However, several spatial clashes were found between the interface of OSM and OSMR in the template-based OSM–OSMR complex (Figure S3B). As a result, it is proposed that the docking pose of the OSM–OSMR complex is more suitable for further investigation.
The Simulated Equilibration States of OSM–OSMR Complex
Starting from the docking conformation, 1 μs of all-atom MD simulation was performed for OSM–OSMR in explicit water. The time evolution of the RMSD of the Cα atom of proteins with respect to the initial coordinates of the docking pose is shown in Figure S4A. The RMSD values of OSM (~4 Å) and OSMR (~5 Å) showed that the two protein partners underwent conformation changes over the course of the simulation. In addition, compared with OSM and OSMR, the higher RMSD of the complex (~6 Å) suggested that the rotation of the two-partner orientation occurred. The extended root mean square fluctuation (RMSF) analysis of the protein residues indicated that the loop residues (135–155) in OSM were more flexible during the simulation; however, the interface residues in both OSM and OSMR were stabilized due to the non-bond interactions (Huang et al., 2019) between the two proteins (Figures S4B,C). Compared with the RMSF analysis of the OSM residues (Figure S4B) with the plot of B-factor of the LIF residues (12–180) in the crystal structure 2Q7N (Figure S5) indicated that OSM shares a similar structural fluctuation with LIF, especially in the loop region (135–155).
The Thermodynamics Properties of OSM–OSMR Complex
To characterize the thermodynamics properties between OSM and OSMR interaction, the snapshots derived from the last 500-ns equilibrated trajectory were used to estimate the MM/GBSA (Kollman et al., 2000) binding free energy. The decomposed energy terms of the total binding free energy (Gtol) indicated that electrostatic interaction energy (Eele, −338.29 ± 44.73 kcal/mol), van der Waals interaction energy (EvdW, −82.02 ± 7.17 kcal/mol), and non-polar solvent energy (Gnonpol, −11.74 ± 1.08 kcal/mol) play important roles in the formation of the protein–protein complex, whereas polar solvent energies (Gpolar, 383.11 ± 43.06 kcal/mol) were unfavored for the interaction.
In addition, per-residue energy decomposition analysis was performed to identify the important residues for the OSM–OSMR complex formation. The residues with an absolute energy contribution of more than 0.5 kcal/mol are listed in Table S3. The chart of the per-residue interaction energy and the interaction mode between OSM and OSMR are further shown in Figure 2. The per-residue energy decomposition analysis successfully predicted five residues in OSM (AB loop: Gly39, Leu40, Lys44, and Leu45; D helix: Phe160) reported by experiments, which played specific roles in activating OSMR signaling (Adrian-Segarra et al., 2018a,b). In addition, seven new residues (Arg36, Asp41, Val42, Arg100, Leu103, Gln161, and Leu164) in OSM were predicted as the important ones that contribute to the protein–protein interaction. Moreover, the 18 residues (Cys179, Leu181, Phe205, Ile206, Asn208, Lys209, Gly210, Tyr214, Glu216, Gln219, Gly220, Asn221, Val222, Ser223, Asp262, Ala264, Leu265, and Trp267) characterized in the OSMR were informative in experimentally verifying these residues, which may play an important role in OSM and OSMR interaction (Huang et al., 2019).
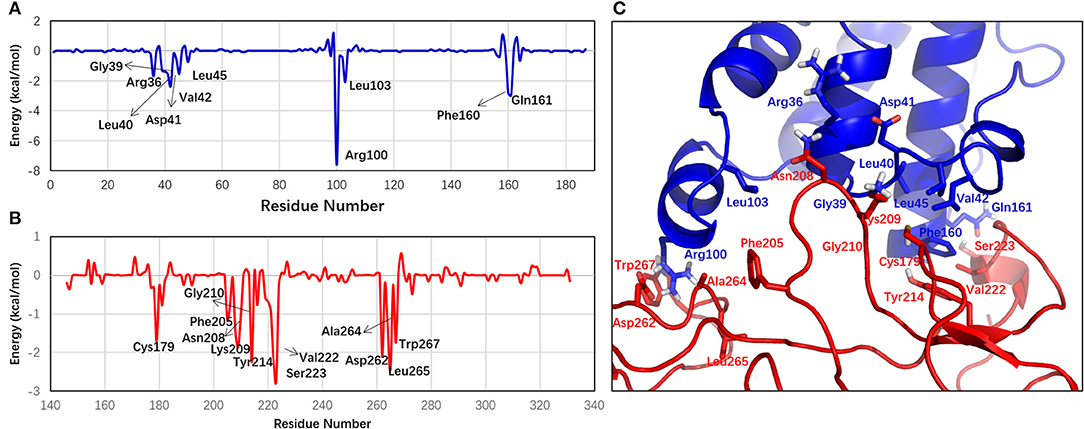
Figure 2. Per-residue energy profiles in (A) OSM and (B) OSMR contribute to the formation the complex. (C) The cartoon representation of the interaction mode of OSM–OSMR interface. Only the important residues (the absolute energy contribution ≥1 kcal/mol) are labeled.
“Hot Spots” Located at OSM–OSMR Interface
In the context of protein–protein interaction, residues that made major contribution to the binding of free energy were termed as “hot spots,” which can be determined by alanine scanning mutagenesis (Zerbe et al., 2012). These “hot-spots” are highly interesting since the protein–protein interaction could be disrupted by targeting them (Grosdidier and Fernandez-Recio, 2008). Herein, to find the “hot spots” located at the OSM–OSMR interface, the computational alanine scanning (CAS) mutagenesis calculation was conducted on the residues with an absolute energy contribution of more than 1 kcal/mol identified by the per-residue energy decomposition analysis (Yang et al., 2018; Du et al., 2020). There were eight “hot spots” (OSM: Arg100, Leu103, Phe160, and Gln161; OSMR: Tyr214, Ser223, Asp262, and Trp267) with a relative binding free energy (G) of more than 2 kcal/mol (Figure 3A) (Moreira et al., 2007; Tu et al., 2018). Figure 3B clearly shows that some important non-bond interactions formed among those “hot spots,” such as the hydrogen bonds between Arg100 and Asp262, Gln161, and Ser223, and the π-π interaction between Phe160 and Tyr214. Among them, Phe160 was found to play an important role in OSM–OSMR recognition (Adrian-Segarra et al., 2018a,b). In addition, the other predicted “hot spots,” especially R100 and D262, were predicted to have a ΔΔG larger than 8 kcal/mol, which might be very useful for further theoretical and experimental studies. To investigate the stability of the mutant, using R100A complex as an example, an additional MD simulation (500 ns) was performed starting from the representative snapshot of wild-type OSM–OSMR. The calculated RMSD values of the OSM–OSMR complex are shown in Figure S4D. It is noted that RMSD significantly increased by around 470 ns for the R100A (~8 Å) complex. In addition, snapshots with the largest RMSD value during the simulation were extracted and shown in Figure 3C. Compared with the equilibrated state conformation of the wild-type OSM–OSMR, significant conformational change near the mutation site occurred in the R100A complex.
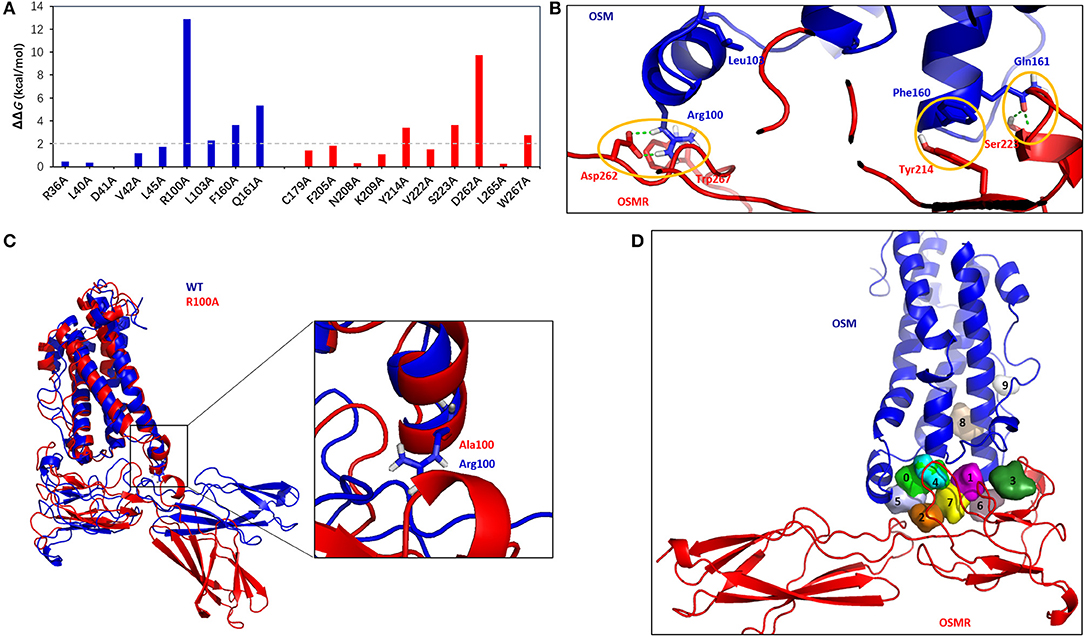
Figure 3. “Hot spots” and potential binding sites located at the OSM–OSMR interface. (A) Computational alanine scanning calculation of the 19 residues with absolute energy contribution of more than 1 kcal/mol identified in per-residue energy decomposition analysis. (B) Interactions of the eight “hot spots” located at the OSM–OSMR interface. The hydrogen bonds are displayed as green dashes. (C) Comparison of the equilibrated state conformation of wild-type OSM–OSMR with the snapshot of R100A mutant after 470-ns molecular dynamics (MD) simulation. (D) Potential binding sites in the OSM–OSMR complex identified through FTMap analysis. The detected 10 sites are labeled (0–9) and shown as surface with different colors in the structure.
Moreover, using the crystal structure of the LIF–LIFR complex (PDB code 2Q7N), CAS analysis was performed on residues (Pro51, Phe52, Pro53, Leu56, Pro106, Leu109, Phe156, Gln157, Ile234, Val258, Asn261, Ser262, Ile267, Ile310, and Leu313) corresponding to the residues (Arg39, Asp41, Val42, Leu45, Arg100, Leu103, Phe160, Gln161, Phe205, Asn208, Lys209, Tyr214, Asp262, and Leu265) located at same position in the OSM–OSMR interface. It is found that Pro106, Phe156 in LIF, and Ile267 in LIFR (Figure S6), corresponding to Arg100, Phe160 in OSM, and Tyr214 in OSMR could be regarded as common “hot-spot” residues for both the OSM–OSMR and LIF-LIFR complexes. In the meanwhile, alanine mutations of other residues have little effect in the interaction energy of the LIF–LIFR complex, suggesting that the interface of the LIF–LIFR complex is significantly different from that of the predicted OSM–OSMR.
Detection of Druggable Sites in OSM–OSMR Interface
Through FTMap (Kozakov et al., 2011) analysis of the MD simulation-derived structure of the OSM–OSMR complex, 10 potential druggable binding sites were detected from fragment-based searching of the global protein surface (Figure 3D), indicating that the conformation of the residues in the recognition interface is very flexible. To further verify the feasibility of the predicted OSM–OSMR model for potential binding sites analysis, the crystal structure of the LIF–LIFR complex was submitted for FTMap analysis using the same approach. The result showed that a total of 14 potential binding sites were detected, and the location of the position is similar with that of the OSM–OSMR complex (Figure S7). Therefore, it could be concluded that the predicted OSM–OSMR model is feasible for binding site prediction analysis. For the OSM–OSMR complex, the protein residues that interacted with bound fragments (within 4 Å) in the binding sites are summarized in Table 1. As shown in Table 1, six of the 10 sites (sites 0, 1, 3–6) were located at the interface of the OSM and OSMR interaction. Sites 2 and 7 were located in the OSMR (Table 1), and sites 8 and 9 were found in OSM (Table 1 and Figure 3D).
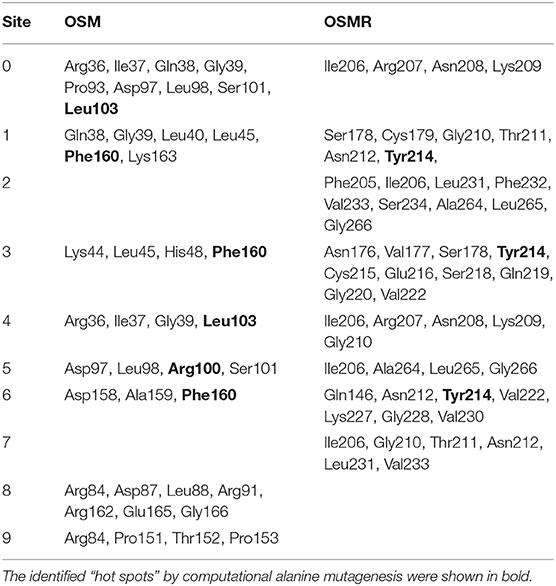
Table 1. List of key interacting residues within 4 Å of the bound probe molecules in the detected potential binding sites rendered as spheres in Figure 3D.
To further evaluate the druggability of the detected binding sites in the OSM–OSMR complex, the identified eight “hot spots” were mapped to the protein residues summarized in Table 1. Interestingly, two common “hot spots” (OSM: Phe160, OSMR: Tyr214) were found in sites 1, 3, and 6. However, only one hot spot (OSM: Arg100 or Leu103) was found in sites 0, 4, and 5, and no “hot spot” was found in sites 2, 7, 8, and 9. This could be understand through the relationship between “hot spots” and ligand binding “hot spots” in the protein–protein interface, in which additional topological requirements were needed in a “hot spot” for small molecule binding (Zerbe et al., 2012). Therefore, sites 1, 3, and 6 were important target sites for designing inhibitors that may inhibit the protein–protein interaction between OSM and OSMR. In addition, as the binding site analysis was performed on the global protein surface, the predicted sites 2, 7 in OSMR and sites 8, 9 in OSM, especially the latter two sites (Figure 3D), which are located far away from the interface, could be regarded as potential allosteric sites.
Conclusion
Targeting the OSM and OSMR pathway represents a potential strategy for the treatment of IBD. In this work, the interaction between OSM and OSMR was investigated by employing computational simulation techniques including homology modeling, protein–protein docking, and long-time scale MD simulation. Post-analysis of the equilibrated simulation trajectory characterized seven new residues in OSM and 18 residues characterized in the OSMR as the important ones contributing to the protein–protein interaction. Based on these important residues, computational alanine scanning and FTMap analysis detected eight “hot spots” and six potential binding sites located at the OSM–OSMR interface. It is interesting to note that, compared with the equilibrated state conformation, significant conformational change near the mutation site occurred in the R100A (one of the identified “hot spots”) complex during MD simulation. Further mapping of the eight “hot spots” in the detected binding sites suggested that sites 1, 3, and 6 were important target sites, which may be used for designing inhibitors to block OSM and OSMR interaction.
Data Availability Statement
All datasets generated for this study are included in the article/Supplementary Material.
Author Contributions
QD and WX designed the experiments and performed computational simulations. QD, YQ, and WX analyzed the data and wrote the paper.
Funding
This research was funded by the Fundamental Research Funds for Central Universities (2019CDYGYB005), Technology Innovation and Application Demonstration Project of Chongqing (cstc2018jscx-msybX0287), and the Young Talents Nursery Foundation of the Second Affiliated Hospital of Chongqing Medical University.
Conflict of Interest
The authors declare that the research was conducted in the absence of any commercial or financial relationships that could be construed as a potential conflict of interest.
Supplementary Material
The Supplementary Material for this article can be found online at: https://www.frontiersin.org/articles/10.3389/fmolb.2020.00029/full#supplementary-material
References
Adrian-Segarra, J. M., Schindler, N., Gajawada, P., Lorchner, H., Braun, T., and Poling, J. (2018a). The AB loop and D-helix in binding site III of human Oncostatin M (OSM) are required for OSM receptor activation. J. Biol. Chem. 293, 7017–7029. doi: 10.1074/jbc.RA118.001920
Adrian-Segarra, J. M., Sreenivasan, K., Gajawada, P., Lorchner, H., Braun, T., and Poling, J. (2018b). The AB loop of oncostatin M (OSM) determines species-specific signaling in humans and mice. J. Biol. Chem. 293, 20181–20199. doi: 10.1074/jbc.RA118.004375
Alford, R. F., Leaver-Fay, A., Jeliazkov, J. R., O'Meara, M. J., DiMaio, F. P., Park, H., et al. (2017). The Rosetta all-atom energy function for macromolecular modeling and design. J. Chem. Theory Comput. 13, 3031–3048. doi: 10.1021/acs.jctc.7b00125
Arkin, M. R., Tang, Y., and Wells, J. A. (2014). Small-molecule inhibitors of protein-protein interactions: progressing toward the reality. Chem. Biol. 21, 1102–1114. doi: 10.1016/j.chembiol.2014.09.001
Babin, V., Berryman, J. T., Betz, R. M., Cai, Q., Cerutti, D. S., Cheatham, T., et al. (2014). AMBER, version 14. California, SF: University of California.
Chaudhury, S., Berrondo, M., Weitzner, B. D., Muthu, P., Bergman, H., and Gray, J. J. (2011). Benchmarking, and analysis of protein docking performance in Rosetta v3.2. PLoS ONE 6:e22477. doi: 10.1371/journal.pone.0022477
Choi, S. Y., Kang, B., Lee, J. H., and Choe, Y. H. (2017). Clinical use of measuring trough levels and antibodies against infliximab in patients with pediatric inflammatory bowel disease. Gut Liver 11, 55–61. doi: 10.5009/gnl16041
Deller, M. C., Hudson, K. R., Ikemizu, S., Bravo, J., Jones, E. Y., and Heath, J. K. (2000). Crystal structure and functional dissection of the cytostatic cytokine oncostatin M. Structure 8, 863–874. doi: 10.1016/S0969-2126(00)00176-3
Du, Q., Qian, Y., Yao, X., and Xue, W. (2020). Elucidating the tight-binding mechanism of two oral anticoagulants to factor Xa by using induced-fit docking and molecular dynamics simulation. J. Biomol. Struct. Dyn. 38, 625–633. doi: 10.1080/07391102.2019.1583605
Fiser, A., Do, R. K., and Sali, A. (2000). Modeling of loops in protein structures. Protein Sci. 9, 1753–1773. doi: 10.1110/ps.9.9.1753
Fleishman, S. J., Leaver-Fay, A., Corn, J. E., Strauch, E. M., Khare, S. D., Koga, N., et al. (2011). RosettaScripts: a scripting language interface to the Rosetta macromolecular modeling suite. PLoS ONE 6:e20161. doi: 10.1371/journal.pone.0020161
Gray, J. J., Moughon, S., Wang, C., Schueler-Furman, O., Kuhlman, B., Rohl, C. A., et al. (2003). Protein-protein docking with simultaneous optimization of rigid-body displacement and side-chain conformations. J. Mol. Biol. 331, 281–299. doi: 10.1016/S0022-2836(03)00670-3
Grosdidier, S., and Fernandez-Recio, J. (2008). Identification of hot-spot residues in protein-protein interactions by computational docking. BMC Bioinformatics 9:447. doi: 10.1186/1471-2105-9-447
Hornak, V., Abel, R., Okur, A., Strockbine, B., Roitberg, A., and Simmerling, C. (2006). Comparison of multiple Amber force fields and development of improved protein backbone parameters. Proteins 65, 712–725. doi: 10.1002/prot.21123
Huang, D., Qi, Y., Song, J., and Zhang, J. Z. H. (2019). Calculation of hot spots for protein-protein interaction in p53/PMI-MDM2/MDMX complexes. J. Comput. Chem. 40, 1045–1056. doi: 10.1002/jcc.25592
Huo, S., Massova, I., and Kollman, P. A. (2002). Computational alanine scanning of the 1:1 human growth hormone-receptor complex. J. Comput. Chem. 23, 15–27. doi: 10.1002/jcc.1153
Huyton, T., Zhang, J. G., Luo, C. S., Lou, M. Z., Hilton, D. J., Nicola, N. A., et al. (2007). An unusual cytokine:Ig-domain interaction revealed in the crystal structure of leukemia inhibitory factor (LIF) in complex with the LIF receptor. Proc. Natl. Acad. Sci. U.S.A. 104, 12737–12742. doi: 10.1073/pnas.0705577104
Kim, W. M., Kaser, A., and Blumberg, R. S. (2017). A role for oncostatin M in inflammatory bowel disease. Nat. Med. 23, 535–536. doi: 10.1038/nm.4338
Kollman, P. A., Massova, I., Reyes, C., Kuhn, B., Huo, S., Chong, L., et al. (2000). Calculating structures and free energies of complex molecules: combining molecular mechanics and continuum models. Acc. Chem. Res. 33, 889–897. doi: 10.1021/ar000033j
Kozakov, D., Grove, L. E., Hall, D. R., Bohnuud, T., Mottarella, S. E., Luo, L., et al. (2015). The FTMap family of web servers for determining and characterizing ligand-binding hot spots of proteins. Nat. Protoc. 10, 733–755. doi: 10.1038/nprot.2015.043
Kozakov, D., Hall, D. R., Chuang, G. Y., Cencic, R., Brenke, R., Grove, L. E., et al. (2011). Structural conservation of druggable hot spots in protein-protein interfaces. Proc. Natl. Acad. Sci. U.S.A. 108, 13528–13533. doi: 10.1073/pnas.1101835108
Laraia, L., McKenzie, G., Spring, D. R., Venkitaraman, A. R., and Huggins, D. J. (2015). Overcoming chemical, biological, and computational challenges in the development of inhibitors targeting protein-protein interactions. Chem. Biol. 22, 689–703. doi: 10.1016/j.chembiol.2015.04.019
Maier, J. A., Martinez, C., Kasavajhala, K., Wickstrom, L., Hauser, K. E., and Simmerling, C. (2015). ff14SB: improving the accuracy of protein side chain and backbone parameters from ff99SB. J. Chem. Theory Comput. 11, 3696–3713. doi: 10.1021/acs.jctc.5b00255
Monaco, C., Nanchahal, J., Taylor, P., and Feldmann, M. (2015). Anti-TNF therapy: past, present and future. Int. Immunol. 27, 55–62. doi: 10.1093/intimm/dxu102
Moreira, I. S., Fernandes, P. A., and Ramos, M. J. (2007). Computational alanine scanning mutagenesis–an improved methodological approach. J. Comput. Chem. 28, 644–654. doi: 10.1002/jcc.20566
Nero, T. L., Morton, C. J., Holien, J. K., Wielens, J., and Parker, M. W. (2014). Oncogenic protein interfaces: small molecules, big challenges. Nat. Rev. Cancer 14, 248–262. doi: 10.1038/nrc3690
Neurath, M. F. (2014). Cytokines in inflammatory bowel disease. Nat. Rev. Immunol. 14, 329–342. doi: 10.1038/nri3661
Nim, S., Jeon, J., Corbi-Verge, C., Seo, M. H., Ivarsson, Y., Moffat, J., et al. (2016). Pooled screening for antiproliferative inhibitors of protein-protein interactions. Nat. Chem. Biol. 12, 275–281. doi: 10.1038/nchembio.2026
Onufriev, A., Bashford, D., and Case, D. A. (2004). Exploring protein native states and large-scale conformational changes with a modified generalized born model. Proteins 55, 383–394. doi: 10.1002/prot.20033
Schaffer, A. A., Aravind, L., Madden, T. L., Shavirin, S., Spouge, J. L., Wolf, Y. I., et al. (2001). Improving the accuracy of PSI-BLAST protein database searches with composition-based statistics and other refinements. Nucleic Acids Res. 29, 2994–3005. doi: 10.1093/nar/29.14.2994
Schrödinger, L. (2010). The PyMOL Molecular Graphics System, version 1.3, New York, NY: Schrödinger, LLC.
Shen, M. Y., and Sali, A. (2006). Statistical potential for assessment and prediction of protein structures. Protein Sci. 15, 2507–2524. doi: 10.1110/ps.062416606
Sitkoff, D., Sharp, K. A., and Honig, B. (1994). Accurate calculation of hydration free energies using macroscopic solvent models. J. Phys. Chem. 98, 1978–1988. doi: 10.1021/j100058a043
Strausberg, R. L., Feingold, E. A., Grouse, L. H., Derge, J. G., Klausner, R. D., Collins, F. S., et al. (2002). Generation and initial analysis of more than 15,000 full-length human and mouse cDNA sequences. Proc. Natl. Acad. Sci. U.S.A. 99, 16899–16903. doi: 10.1073/pnas.242603899
Tu, G., Fu, T., Yang, F., Yao, L., Xue, W., and Zhu, F. (2018). Prediction of GluN2B-CT1290-1310/DAPK1 interaction by protein(-)peptide docking and molecular dynamics simulation. Molecules 23:3018. doi: 10.3390/molecules23113018
Verstockt, S., Verstockt, B., and Vermeire, S. (2019). Oncostatin M as a new diagnostic, prognostic and therapeutic target in inflammatory bowel disease (IBD). Expert Opin. Ther. Targets 23, 943–954. doi: 10.1080/14728222.2019.1677608
Wang, E., Sun, H., Wang, J., Wang, Z., Liu, H., Zhang, J. Z. H., et al. (2019). End-point binding free energy calculation with MM/PBSA and MM/GBSA: strategies and applications in drug design. Chem. Rev. 119, 9478–9508. doi: 10.1021/acs.chemrev.9b00055
Wang, J., Wang, W., Kollman, P. A., and Case, D. A. (2006). Automatic atom type and bond type perception in molecular mechanical calculations. J. Mol. Graph. Model. 25, 247–260. doi: 10.1016/j.jmgm.2005.12.005
Webb, B., and Sali, A. (2016). Comparative protein structure modeling using MODELER. Curr. Protoc. Bioinformatics 54, 5.6.1–5.6.37. doi: 10.1002/cpbi.3
Weng, G., Wang, E., Wang, Z., Liu, H., Zhu, F., Li, D., et al. (2019). HawkDock: a web server to predict and analyze the protein-protein complex based on computational docking and MM/GBSA. Nucleic Acids Res. 47, W322–W330. doi: 10.1093/nar/gkz397
West, N. R., Hegazy, A. N., Owens, B. M. J., Bullers, S. J., Linggi, B., Buonocore, S., et al. (2017). Oncostatin M drives intestinal inflammation and predicts response to tumor necrosis factor-neutralizing therapy in patients with inflammatory bowel disease. Nat. Med. 23, 579–589. doi: 10.1038/nm.4307
Yang, F., Zheng, G., Fu, T., Li, X., Tu, G., Li, Y. H., et al. (2018). Prediction of the binding mode and resistance profile for a dual-target pyrrolyl diketo acid scaffold against HIV-1 integrase and reverse-transcriptase-associated ribonuclease H. Phys. Chem. Chem. Phys. 20, 23873–23884. doi: 10.1039/C8CP01843J
Yu, Z., Li, Z., Wang, C., Pan, T., Chang, X., Wang, X., et al. (2019). Oncostatin M receptor, positively regulated by SP1, promotes gastric cancer growth and metastasis upon treatment with Oncostatin M. Gastric Cancer 22, 955–966. doi: 10.1007/s10120-019-00934-y
Keywords: inflammatory bowel disease, oncostatin M and oncostatin M Receptor, protein-protein docking, molecular dynamics simulation, binding sites prediction
Citation: Du Q, Qian Y and Xue W (2020) Molecular Simulation of Oncostatin M and Receptor (OSM–OSMR) Interaction as a Potential Therapeutic Target for Inflammatory Bowel Disease. Front. Mol. Biosci. 7:29. doi: 10.3389/fmolb.2020.00029
Received: 24 December 2019; Accepted: 11 February 2020;
Published: 04 March 2020.
Edited by:
Guang Hu, Soochow University, ChinaReviewed by:
Fei Ye, Zhejiang Sci-Tech University, ChinaYong Wang, University of Copenhagen, Denmark
Copyright © 2020 Du, Qian and Xue. This is an open-access article distributed under the terms of the Creative Commons Attribution License (CC BY). The use, distribution or reproduction in other forums is permitted, provided the original author(s) and the copyright owner(s) are credited and that the original publication in this journal is cited, in accordance with accepted academic practice. No use, distribution or reproduction is permitted which does not comply with these terms.
*Correspondence: Yan Qian, Y3FxaWFueWFuJiN4MDAwNDA7aG9zcGl0YWwuY3FtdS5lZHUuY24=; Weiwei Xue, eHVld3cmI3gwMDA0MDtjcXUuZWR1LmNu