- 1Hubei Key Laboratory of Biological Resources Protection and Utilization, Hubei Minzu University, Enshi, China
- 2College of Biological and Food Engineering, Hubei Minzu University, Enshi, China
- 3College of Life Science, Baicheng Normal University, Baicheng, China
The mining industry in China plays a pivotal role in economic development but also leads to severe environmental issues, particularly heavy metal pollution in soils. Heavy metal pollution significantly impacts soil microbial communities due to its persistence and long-term residual effects. We assessed changes in microbial diversity, community structure, and assembly mechanisms in selenium-impacted soils. This study investigates the impacts of selenium (Se) and other heavy metals on soil microbial communities in selenium-rich mining areas using full-length 16S rRNA gene sequencing. Our results showed that Se and other heavy metal contamination significantly altered microbial community composition, favoring metal-tolerant phyla such as Proteobacteria, Actinobacteriota and Firmicutes, while reducing the abundance of sensitive groups like Acidobacteriota and Chloroflexi. Microbial diversity decreased as Se and other heavy metal concentrations increased. Mantel test analysis revealed that soil total potassium (TK), soil organic carbon, total nitrogen, and several other metals, including zinc, niobium, titanium (Ti), manganese, rubidium, barium, potassium, cobalt, gallium (Ga), Se, chromium (Cr), vanadium, and copper were significantly and positively correlated with microbial community composition across all soil samples. Random forest analysis showed that soil TK and multiple elements [Cr, Ti, nickel (Ni), Ga and Se] were the most important predictors of bacterial diversity, emphasizing the role of multiple elements in shaping microbial communities. Co-occurrence network analysis revealed that Se and other heavy metal contamination reduced network complexity and stability, with high Se-contaminated soils exhibiting fragmented microbial networks. Community assembly was primarily driven by drift in control soils, whereas dispersal limitation became more prominent in Se-contaminated soils due to heavy metal toxicity. These findings highlight the ecological consequences of heavy metal pollution on microbial communities and offer valuable insights for effective soil management and remediation strategies.
1 Introduction
In China, the mining industry plays a crucial role in socio-economic development, but also causes severe ecological and environmental challenges, particularly heavy metal pollution in soils (Marimuthu et al., 2021; Wen et al., 2024). Among these pollutants, selenium (Se) is of particular concern due to its dual nature—it is an essential micronutrient at trace levels but becomes toxic at higher concentrations, posing significant risks to soil ecosystems, water quality, and agricultural productivity by disrupting microbial functions, soil fertility, and plant growth (Banerjee et al., 2022; Ma et al., 2024). Se contamination in mining regions is exacerbated by the release of Se-rich tailings and wastewater, which can infiltrate adjacent soils, altering their physicochemical properties and harming the microbial communities that are crucial for ecosystem stability (Rosenfeld et al., 2018; Peng et al., 2024). The impact of Se on soil microorganisms is especially critical, as these organisms play essential roles in nutrient cycling and maintaining ecological balance (Yang R. et al., 2022). When exposed to elevated Se levels, soil microbial communities may undergo significant shifts, with certain taxa either adapting to or being suppressed by the contaminant. These changes can have profound effects on microbial biodiversity, community structure, and soil functions such as carbon and nitrogen cycling (Rijk, 2023).
Soil microorganisms, which are primary drivers of nutrient cycling and ecosystem stability, are highly susceptible to heavy metal contamination (Tang et al., 2019). Their high surface-area-to-volume ratio, coupled with their physiological diversity, makes microbial communities both sensitive indicators and key mediators of soil responses to metal stress (Ma et al., 2022; Sarkar et al., 2022). Heavy metal contamination significantly alters microbial biomass, community structure, and diversity, frequently favoring metal-tolerant groups such as Proteobacteria and Firmicutes, while suppressing metal-sensitive taxa, including members of Actinobacteria and Acidobacteria (Zhao et al., 2019; Hemmat-Jou et al., 2021). These shifts disrupt critical ecosystem functions, including carbon and nitrogen cycling, and impair the soil’s capacity to support plant growth (Zhou et al., 2016; Rijk and Ekblad, 2020). Although there is substantial research on the effects of heavy metals like cadmium (Cd) and arsenic (As) on microbial communities (Olaniran et al., 2013), the specific impact of Se contamination and its interaction with microorganisms in mining environments remains incompletely understood. Selenium’s behavior in soil is influenced by various factors, including its chemical form and the presence of other contaminants, making it complex to investigate how microbial communities respond to varying Se contamination levels (Rosenfeld et al., 2018). High-throughput sequencing technologies and metagenomics offer powerful tools to explore these interactions in detail, enabling a deeper understanding of how microbial communities adapt and function under Se and other heavy metal stress (Xiao et al., 2019; Yan et al., 2020).
The assembly processes of microbial communities are key determinants of microbial diversity and composition, especially under heavy metal stress (Chen et al., 2024; Sun et al., 2024). These processes are governed by two principal ecological mechanisms: deterministic processes, driven by environmental filtering and niche selection, and stochastic processes, which include dispersal limitation, mass effects, and random demographics (Caruso et al., 2011; Chase and Myers, 2011; Dini-Andreote et al., 2015). In heavy metal-contaminated soils, deterministic processes often dominate due to the strong selective pressures exerted by metal toxicity and altered soil chemistry, such as reduced pH or elevated ionic strength (Zhang et al., 2022; Sun et al., 2024). These pressures favor microbial taxa with specialized traits, such as efflux pumps and enzymatic detoxification pathways, which provide resistance to metals (Silver, 1996; Sharma et al., 2024). Conversely, in less polluted or more heterogeneous environments, stochastic processes play a more significant role, as random factors influence microbial distribution (Zhang et al., 2022; Liu et al., 2024). Although deterministic processes are generally regarded as the primary drivers in heavily polluted environments, the relative contributions of deterministic and stochastic factors along Se and other heavy metal contamination remain poorly quantified. In addition to assembly mechanisms, microbial interactions within communities are critical to their resilience and functionality in stressed environments (Nizamani et al., 2024). Under heavy metal stress, cooperative interactions, such as nutrient sharing and mutual detoxification, often emerge as survival strategies, leading to the formation of tightly connected microbial networks (Sarsan et al., 2021). Advanced network analysis methods, including those using random matrix theory (RMT)-based molecular ecological network (MEN) analysis, offer robust frameworks for quantifying these interactions and assessing microbial community stability (Deng et al., 2012). Recent studies have shown that the complexity and connectivity of microbial networks are positively correlated with ecosystem resilience, suggesting that such networks play a pivotal role in maintaining functionality under heavy metal stress (Chen et al., 2021; Wu et al., 2024). However, the links between microbial interaction networks and community assembly processes in Se-contaminated soils remain underexplored.
To address these research gaps, this study aims to systematically investigate the effects of Se and other heavy metal pollution on soil microbial communities. Specifically, we focus on: (1) characterizing shifts in microbial community structure and diversity along gradients of Se and other heavy metal contamination; (2) quantifying the relative contributions of deterministic and stochastic processes to microbial community assembly under varying Se and other heavy metal contamination; and (3) elucidating the role of microbial interactions in maintaining community stability and functionality. By integrating high-throughput sequencing and advanced ecological modeling, this study provides comprehensive insights into the interplay between Se, other heavy metal pollution, and soil microbial ecology, contributing to the development of sustainable soil management and remediation practices.
2 Materials and methods
2.1 Soil collection and treatments
Soil samples were collected from Enshi, Hubei Province, China, which is known as the only Se mine in the world (Zhu and Zheng, 2001). Samples were collected from two different contamination levels (30°10′N, 109°46′E, elevation 1,600 m): a selenium-rich carbonaceous siliceous rock area (HSe), which is heavily contaminated by Se, and a relatively less contaminated area located 100 m away from the selenium-rich site (LSe). A non-contaminated region with similar soil parent material similar to that of the contaminated regions was selected as the control (Control) without Se contamination. Ten independent sampling points were randomly selected within each region (HSe, LSe, Control), with each sample representing an independent biological replicate. The surface soil was collected from the top 0–20 cm layer. At each sampling point, the soil was collected using a clean soil auger, and composite samples were created by mixing soil from five sub-sampling points within a 1-meter radius around each random point. Each composite sample represented one biological replicate. The samples were then divided into two subsamples: one for measuring soil indicators and the other stored at −80°C for subsequent high-throughput sequencing analysis. Soil moisture content was measured before the soil samples were subjected to natural air-drying. Freshly collected soil samples were weighed immediately after collection to determine their moisture content. Soil moisture content was determined using the drying and combustion method.
After natural air-drying, the soil samples were sieved through a 2 mm mesh to homogenize them, removing fine roots and stones. Soil pH was measured using a pH meter with a soil/water ratio of 5 g/25 mL. Soil organic carbon (SOC) and total nitrogen (TN) were determined using an elemental analyzer (Elementar, Hesse, Germany). Total potassium (TK) content was measured using the NaOH fusion-flame photometry method. Available potassium (AK) was extracted with 1 mol/L ammonium acetate and determined by flame photometry. Total phosphorus (TP) was determined using sulfuric acid-perchloric acid digestion followed by atomic spectroscopy. Available phosphorus (AP) was extracted with 0.5 mol/L NaHCO₃ and analyzed using the molybdenum-antimony anti-spectrophotometric method (Olsen method). Alkali-hydrolyzed nitrogen (AN) was determined using the alkali diffusion method. Soil metal elements contents were determined by inductively coupled plasma mass spectrometry (ICP-MS). Statistical analysis was performed using one-way analysis of variance (ANOVA) to determine the significant differences among the different pollution areas, with a significance level set at p < 0.05.
2.2 DNA extraction, library construction, and sequencing
A total of 30 soil samples were collected, and high-quality genomic DNA was extracted from 0.1 g of soil using a commercially available soil DNA extraction kit (Power Soil DNA Isolation Kit, Qiagen) following the manufacturer’s protocol. The purity, concentration, and integrity of the extracted DNA were assessed using a Nanodrop spectrophotometer (Thermo Fisher Scientific, Waltham, MA, United States), and the fragment size distribution was examined by agarose gel electrophoresis. The full-length 16S rRNA gene was amplified using specific primers (27F 5′-AGRGTTTGATYNTGGCTCAG-3′, 1492R 5′-TASGGHTACCTTGTTASGACTT-3′). The PCR products were precisely quantified using a Qubit fluorometer (Thermo Fisher Scientific, Waltham, MA, United States), and the fragment size distribution of the amplicons was verified by agarose gel electrophoresis. The amplified products then underwent end repair and dA-tailing. Sequencing adapters were ligated to the ends of the repaired products, followed by purification of the constructed libraries using magnetic beads. The final libraries were loaded onto an R10.4 Flow Cell, and sequencing was performed on a PromethION P48 sequencing platform (Oxford Nanopore Technologies, Oxford, United Kingdom).
2.3 Data process and statistical analyses
The raw sequencing data were processed using Guppy base-calling software version 5.0.16 (Oxford Nanopore, Oxford, United Kingdom) with default parameters to generate high-quality reads. Adapter and barcode sequences were trimmed using Porechop (version 0.2.3). Quality filtering was performed using NanoFilt (version 2.7.1) to exclude sequences with a quality score below 10, and Cutadapt (version 3.5) was employed to remove length discrepancies and obtain clean, primer-free sequences. Chimeric sequences were identified and removed with Minimap2 (version 2.17) and yacrd (version 0.6.2). Finally, taxonomic assignment of ASVs was performed using QIIME2 (version 2022.3) based on the SILVA 138 release.
Alpha diversity indices, including abundance-based coverage estimators (ACE), Shannon, Chao1, and Simpson, were calculated to evaluate microbial community richness and diversity. Beta diversity metrics, such as Bray–Curtis dissimilarity, were computed to assess the differences of community composition. The relationships between examined soil variables and the dominant bacterial phyla or genus were displayed with a Spearman correlation heatmap, and visualized with the R package ggplot2. Principal component analysis (PCA) was performed to visualize microbial community variations among samples. Additionally, three non-parametric methods—Adonis, ANOSIM, and MRPP—were employed to analyze overall bacterial community structure. Redundancy analysis (RDA) was conducted to investigate the relationships between soil bacterial communities and environmental factors, with significant variables identified via the envfit function in the vegan package using 999 permutations. The Mantel test was employed to evaluate correlations between soil physicochemical properties and bacterial community structure, and the results were visualized with heatmaps generated using the pheatmap package. The relative abundance-weighted community degree (RACD) was calculated to examine the variations of diazotrophic community associations by different components, as follows: ∑N × RA, where N is the number of ASVs, and RA is the relative abundance of the ASVs (Sun et al., 2020). A random forest model was applied to identify the importance of soil variables in predicting the microbial diversity indices. Predictor importance was assessed based on the percentage increase in mean squared error (MSE), and the significance of each predictor was validated using the R package “randomForest” (R version 4.3.3).
2.4 Co-occurrence network analysis and community assembly
A co-occurrence network analysis was conducted using the phylogenetic molecular ecological network analysis pipeline (pMENA) to explore relationships between different microbial taxa (Deng et al., 2012). Amplicon sequencing variants (ASVs) only present in over eight samples were retained for subsequent analysis. Low-abundance taxa were filtered out from the ASV table to reduce interference. Network topological properties, including node degree, betweenness centrality, average clustering coefficient and modularity, were calculated to identify key taxa and network modules. The networks were visualized using Gephi software (Bastian et al., 2009). The vulnerability and robustness of microbial networks were calculated as described in Yuan et al. (2021). Based on differences in ASV abundance within modules in the co-occurrence network diagram, the within-module connectivity (Zi) and among-module connectivity (Pi) for each node were calculated, and keystone taxa were identified within the modules (Shi et al., 2016). Nodes were categorized into four distinct types based on their calculated Zi and Pi values: module hubs (Zi > 2.5), network hubs (Zi > 2.5 and Pi > 0.62), connectors, and peripherals (Zi < 2.5 and Pi < 0.62) (Zhou et al., 2010).
To elucidate the influence of heavy metals on bacterial community assembly, four ecological models were employed: the normalized stochasticity ratio (NST), Sloan neutral community model (NCM), β-nearest taxon index (βNTI), and infer community assembly mechanisms by phylogenetic-bin-based null model analysis (iCAMP). Each model offers unique insights into the underlying processes shaping microbial communities. NST was used to quantify the balance between stochastic and deterministic processes, with values below 50% indicating deterministic dominance and those above 50% reflecting stochasticity (Ning et al., 2019). The Sloan NCM provided a probabilistic framework to evaluate the role of neutral processes in microbial community assembly, taking into account factors such as dispersal limitation and ecological drift (Sloan et al., 2006). βNTI was applied to assess the phylogenetic turnover between communities, enabling the identification of deterministic processes such as homogeneous or heterogeneous selection (Stegen et al., 2012). Finally, iCAMP integrated phylogenetic and ecological information to dissect community assembly into specific deterministic processes (e.g., homogeneous and heterogeneous selection) and stochastic processes (e.g., dispersal limitation, homogenizing dispersal, and drift) based on βNRI and RC metrics (Ning et al., 2020). Together, these models provided a comprehensive framework to investigate how elemental variations influence bacterial community assembly through deterministic and stochastic processes.
2.5 Data availability
All the bacterial raw sequences have been deposited to GenBank Short Read Archive (PRJNA1153714).
3 Results
3.1 Differences in soil physicochemical properties and enzyme activities among different pollution areas
The contents of elements in soils are shown in Table 1. Soil water content, pH, AK, and AP did not show significant difference among regions (p > 0.05). TN, TK, and SOC were significantly higher in selenium-contaminated soils (LSe and HSe) than in Control, while AN was the lowest in HSe soils (p < 0.05). Soil K, Mn, Ba, Ti, Co, Ga, and Rb contents were significantly higher in Control soils than in LSe and HSe soils (p < 0.05). In addition, the contents of Se, Cr, Ni, Cu, and V were significantly higher in Se-contaminated than in Control soil (p < 0.05), with the most significant changes observed in HSe soils, where the increase ranged from 2 to 74 times. However, the contents of other elements, including K, Ce, P, Zn, Na, Mg, Ca, Fe, La, Li, Be, Ti, Cr, Co, Ni, Sr, Mo, Pb, V, Sc, Th, Nb, and As did not show significant difference among regions (p > 0.05).
3.2 Effects of different pollution areas on soil bacterial community composition and α-diversity
After filtering out non-target sequences (including chloroplast, mitochondrial, archaeal, and unassigned sequences), a total of 2,105,317 high-quality bacterial sequences were obtained from 30 samples, with an average of 70,177 sequences per sample. As shown in Figure 1A, 5,027 bacterial ASVs were identified across all samples. All alpha diversity indexes, including ACE, Shannon, Chao1 and Simpson were significantly lower in HSe group compared to the LSe and Control groups (p < 0.05) (Figure 1A).
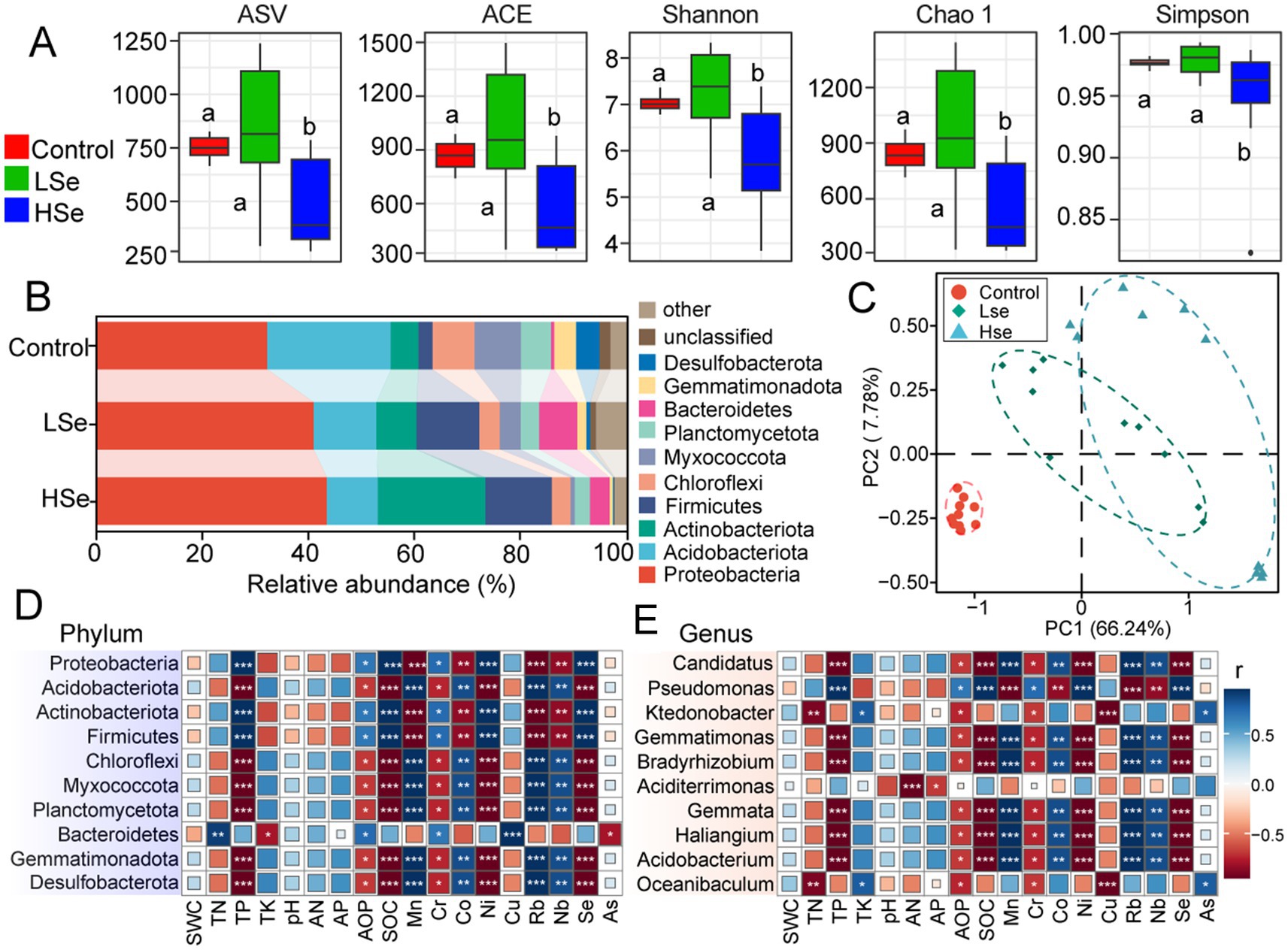
Figure 1. Alpha- and beta-diversity indices of microbial communities in Se-contaminated soils. (A) Alpha- diversity indices. (B) Relative abundance of microbial phyla across different soil samples. (C) Correlation analysis between soil physicochemical properties and relative abundance. Correlation analysis of microbial phyla (D) and genus (E) with soil physicochemical properties in Se-contaminated soils. ***p < 0.001, **p < 0.01, and *p < 0.05.
The effects of varying Se concentrations and heavy metals on soil microbial communities were primarily reflected in the community composition at the phylum level (Figure 1B). Proteobacteria, Acidobacteriota, Actinobacteriota, and Firmicutes were the four most abundant phyla. In the Control group, Proteobacteria, Actinobacteriota, and Firmicutes exhibited the lowest relative abundances, but their relative abundances increased with higher Se concentrations. Specifically, Proteobacteria increased from 32.05 to 43.30%, Actinobacteriota increased from 5.21 to 20.29%, and Firmicutes from 2.66 to 12.56%. Interestingly, Acidobacteriota showed the opposite trend, decreasing from 23.32 to 9.64%. Apart from these phyla, most other phyla within the top 10 also exhibited a decline in relative abundance. For example, Chloroflexi decreased from 7.95 to 3.51%, Myxococcota from 8.78 to 0.83%, Planctomycetota from 5.65 to 2.84%, Gemmatimonadota from 4.12 to 3.66%, and Thermodesulfobacteriota from 4.44 to 0.17%. The PCA plot demonstrated clear clustering of microbial communities between the Se-contaminated (LSe and HSe) and the Control soils, with the first two principal components explaining 66.24 and 7.78% of the total variance, respectively (Figure 1C). These results indicate distinct microbial community compositions under varying levels of Se and other heavy metal exposure. This was further confirmed by statistical analyses, including ANOSIM (p = 0.009), MRPP (p = 0.001), and Adonis (p = 0.001), all of which demonstrated significant differences between groups (p < 0.05) (Table 2).
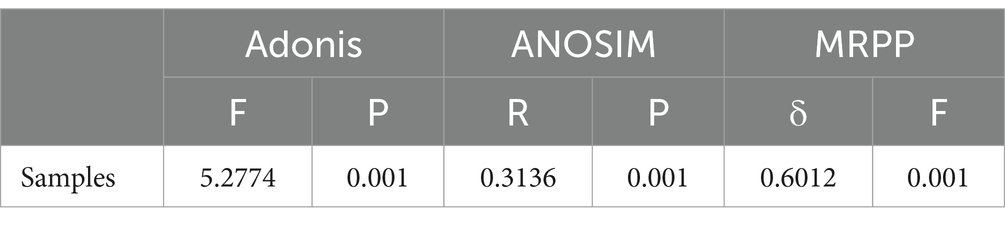
Table 2. Significance tests of the effects of different samples on the bacterial community with three different statistical approaches.
Correlation analysis revealed variations in the adaptability and ecological functions of microbial communities under specific environmental conditions (Figures 1D,E). At the phylum level (Figure 1D), Proteobacteria showed a significant positive correlation with SOC and Se, while Acidobacteriota exhibited a significant negative correlation with the same variables. Actinobacteriota demonstrated positive correlations with heavy metals such as Mn, Cr, and Cu, indicating potential heavy metal tolerance. In contrast, Gemmatimonadota showed negative correlations with multiple environmental variables, suggesting growth limitations. At the genus level (Figure 1E), Candidatus displayed significant negative correlations with AP and Se, while Pseudomonas had strong positive correlations with various environmental variables, particularly heavy metals like Cr and Cu. Ktedonobacter showed a positive correlation with Se and As, whereas Gemmatimonas exhibited negative correlations with AP and SOC, indicating unfavorable conditions for its growth.
3.3 Relationship between environmental variables and microbial communities in different pollution areas
The microbial community structure was significantly influenced by environmental variables, as revealed through various multivariate analyses. The RDA plot identified the major environmental factors shaping microbial community composition. Soil Se, TN, SOC, and TP were the primary drivers of microbial variation and were correlated with specific microbial taxa (Figure 2A). For instance, Proteobacteria, Actinobacteriota, Firmicutes, and Bacteroidetes showed strong associations with elevated Se and heavy metal concentrations, while other phyla were more closely linked to TK gradients. Mantel test analysis was conducted to explore the potential abiotic factors driving variations in the soil microbial community across different Se-contamination levels. The analysis revealed that TK, SOC, TN, Zn, Nb, Ti, Mn, Rb, Ba, K, Co, Ga, Se, Cr, V, and Cu were significantly and positively correlated with microbial community composition across all groups (Figure 2B). Additionally, heavy metals such as Cr and Cu showed moderate positive correlations with the microbial community, indicating their potential role in shaping community structure. In contrast, certain environmental variables, such as K and Ba, displayed weaker or no significant correlations with microbial community composition. Notably, the strength of these correlations varied between LSe and HSe soils, suggesting that the impact of environmental factors on microbial communities is influenced by the degree of Se contamination. These findings highlight the complex interactions between biotic and abiotic factors in shaping soil microbial communities under Se contamination.
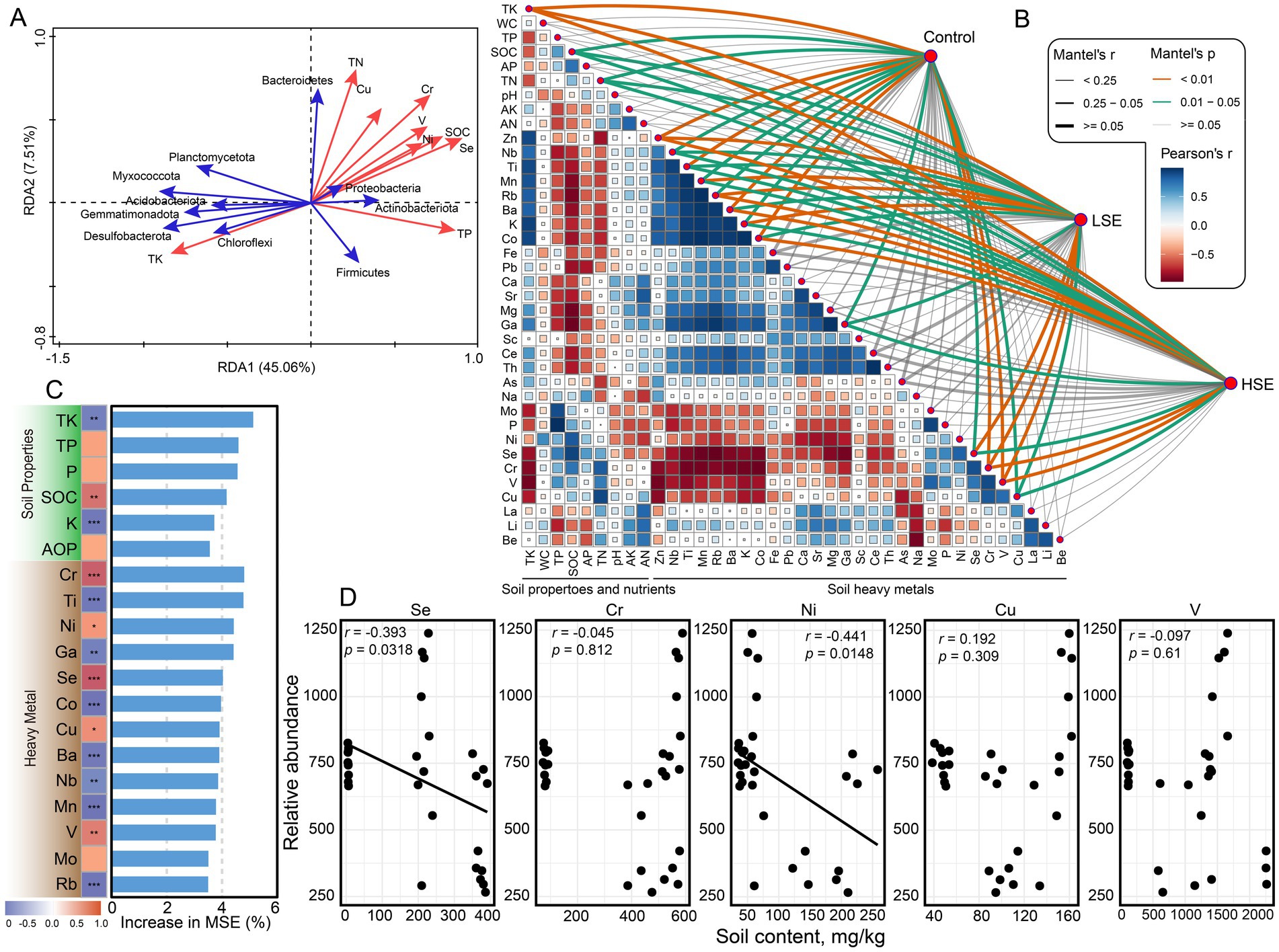
Figure 2. Effect of heavy metals and soil properties on bacterial community diversity. (A) Redundancy analysis (RDA) showing the relationships between soil properties, heavy metals, and microbial community compositions. Red arrows represent soil properties and heavy metals, while blue arrows represent microbial taxa. (B) Mantel test results visualizing the correlations between soil properties, heavy metals, and microbial communities, with Pearson’s correlation coefficients color-coded. Line thickness indicates the strength of Mantel’s r, and significance levels are indicated by line type. (C) Random forest analysis ranking the importance of soil properties and heavy metals in influencing microbial community compositions, with the percentage increase in mean squared error (MSE) shown. (D) Pearson correlation between soil heavy metal (Se, Cr, Ni, Cu, and V) contents and relative abundance of bacteria. **Asterisks indicate significance levels: ***p < 0.05, **p < 0.01, and ***p < 0.001.
Random forest analysis identified TK as the most critical soil property influencing microbial community structure, with TK showing the highest increase in mean squared error (MSE) (Figure 2C). Multiple heavy metal elements (Cr, Ti, Ni, Ga, and Se) were the most influential factors, further highlighting the combined importance of soil fertility and heavy metal contamination in shaping microbial community dynamics. Pearson correlation analysis demonstrated a significant negative relationship between bacterial relative abundance and soil Se and Ni concentrations (p < 0.05) (Figure 2D). At the phylum level, Proteobacteria, Actinobacteriota, and Bacteroidetes exhibited positive correlations with the five heavy metal elements (Supplementary Table S1). Additionally, analysis of soil Se content and the top 20 most abundant genera revealed that Pseudomonas, Staphylococcus, Candidatus Solibacter, and Serratia were positively correlated with the majority of these heavy metals (Supplementary Table S1). Together, these analyses underscore the complex interplay between heavy metals and soil properties in shaping microbial community structure.
3.4 Microbial co-occurrence networks in different pollution areas
The bacterial co-occurrence network results demonstrated significant shifts in the microbial co-occurrence network structures under Se-contamination soils. In the Control group, the network exhibited a more modular structure with higher total nodes (257) and links (360), as well as a lower degree centralization (CD = 0.052) and higher average clustering coefficient (avgCC = 0.161) (Table 3), indicating a stable and well-connected network. The presence of keystone ASVs, such as Myxococcota, Proteobacteria, Armatimonadota, and Firmicutes, in the Control group module hubs highlights a diverse and functional microbial community (Figure 3E and Table 4). However, under the LSe group, the microbial network displayed increased total links (551) and average degree (4.322), along with reduced modularity (0.607), suggesting a denser but less modular network with increased connectivity. Keystone taxa in the LSe group predominantly belonged to Proteobacteria and Nitrospirota, which were identified as both connectors and module hubs, indicating their critical role in maintaining microbial interactions and network stability under low Se stress.
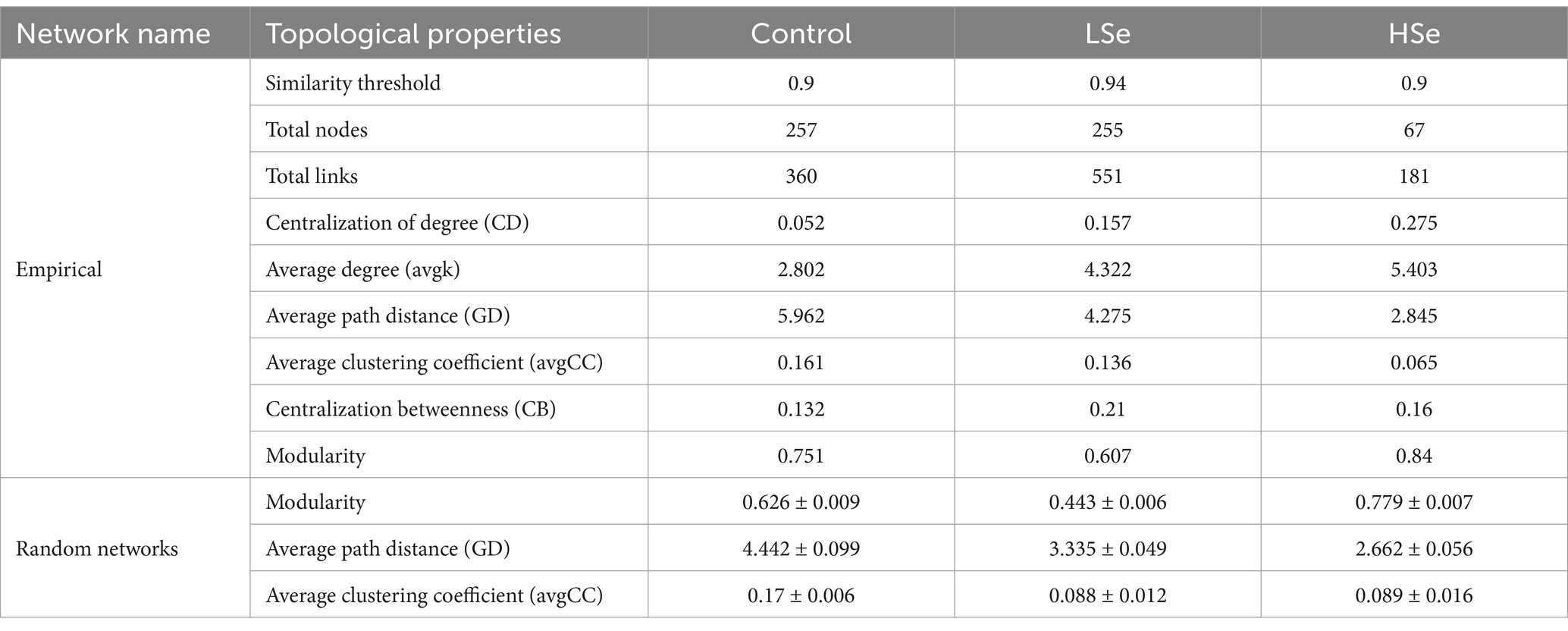
Table 3. Topological properties of empirical networks of different bacterial communities and their associated random ecological networks.
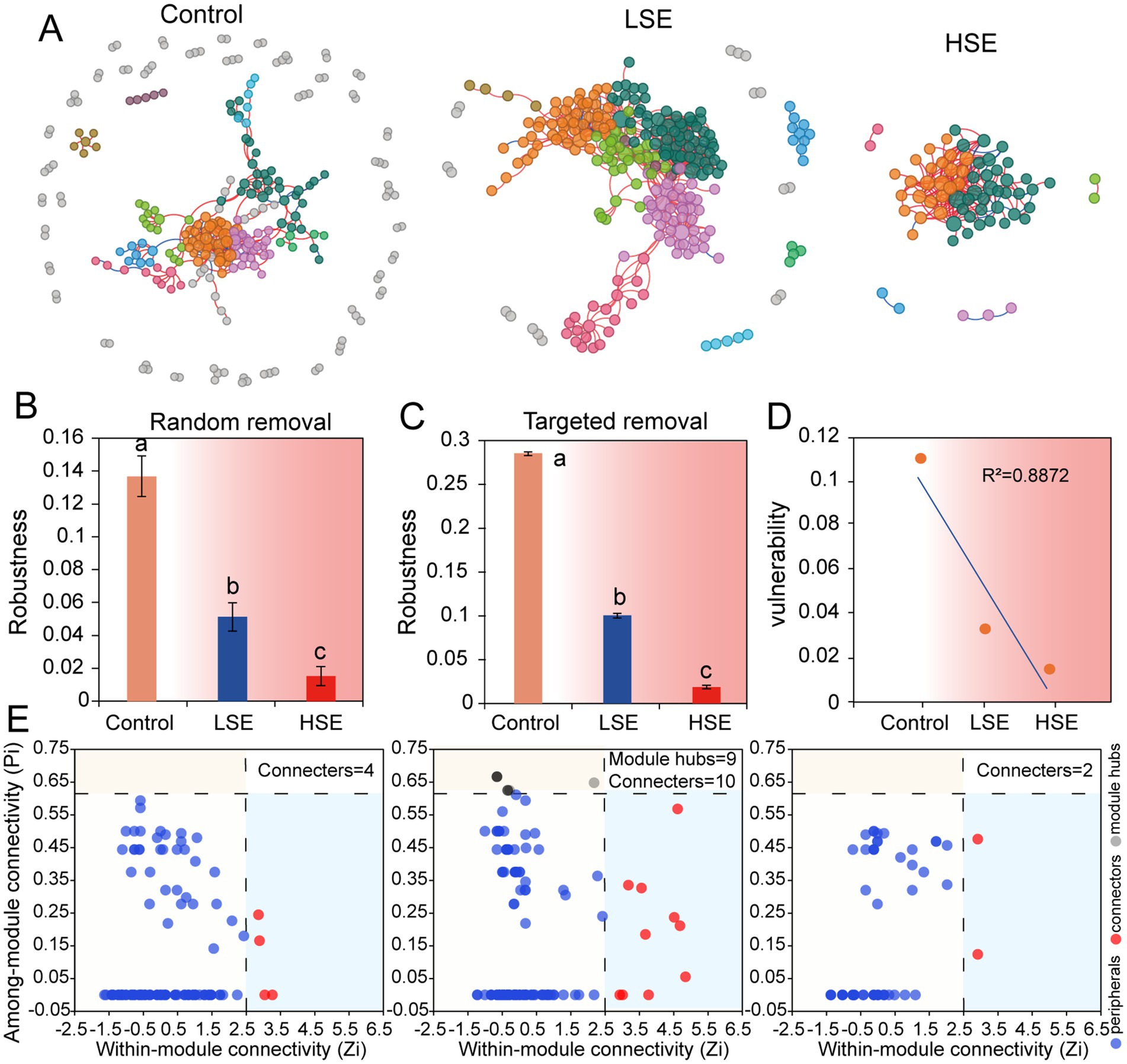
Figure 3. Co-occurrence network analysis of soil microbial community based on Pearson’s correlation analysis. (A) Blue and red lines represent significant negative and positive correlations, respectively. The nodes indicate the ASVs, and the color of node represents the model hub. Robustness is calculated as the proportion of remaining species in the community after randomly removing 50% of the nodes (B) or targeted hubs (C), while vulnerability (D) is determined by the highest node vulnerability in each network. (E) Putative keystone taxa in the different networks based on Pi and Zi. The solid circle represents an ASV.
In contrast, high Se exposure drastically reduced network complexity (Figure 3A), with total nodes decreasing to 67 and links to 181, accompanied by increased degree centralization (CD = 0.275) and a lower clustering coefficient (avgCC = 0.065). The reduced average path distance (2.845) and higher modularity (0.84) in HSe reflect a fragmented and simplified network structure, suggesting a breakdown in microbial interactions and niche differentiation under high Se and other heavy metal stress. Keystone ASVs in the HSe group were primarily from Acidobacteriota, highlighting the resilience of specific taxa in highly stressed environments (Figure 3E and Table 4). Random and targeted node removal analyses further revealed that the robustness of microbial networks decreased significantly with increasing Se and other heavy metal contamination (Figures 3B,C), with HSe networks exhibiting the highest vulnerability (p < 0.05) (Figure 3D). Collectively, these findings demonstrate that selenium contamination disrupts microbial co-occurrence patterns, reduces network complexity, and alters keystone taxa affiliation, with high Se and other heavy metal stress leading to significant microbial community fragmentation and functional instability.
3.5 Ecological processes governing bacterial community assembly in different pollution areas
To clarify the influence of heavy metals on bacterial community assembly, four ecological models were employed to dissect the relative roles of deterministic and stochastic processes. First, niche breadth and the normalized stochasticity ratio (NST) were analyzed to assess community adaptability and the balance between stochasticity and determinism. The Control group displayed the broadest niche breadth, reflecting high adaptability to environmental conditions (Figure 4A). However, with increasing Se and other heavy metal concentrations, especially in the HSe group, niche breadth significantly decreased (p < 0.05). This decline indicates that elevated stress reduced species adaptability, leading to a drop in microbial diversity and a shift in the dominance of stochastic and deterministic processes. NST values calculated using Jaccard, Bray–Curtis, and weighted UniFrac metrics revealed that stochastic processes controlled the majority of bacterial community assembly in all groups (89.87, 73.10, and 66.79% for Control, LSe, and HSe, respectively) (Figure 4B). While stochastic processes prevailed in the Control and LSe groups, a notable decline in NST in the HSe group suggested a growing contribution of deterministic processes. This pattern likely results from increased environmental pressure, which favors the survival of specific taxa, causing a shift toward more deterministic community assembly.
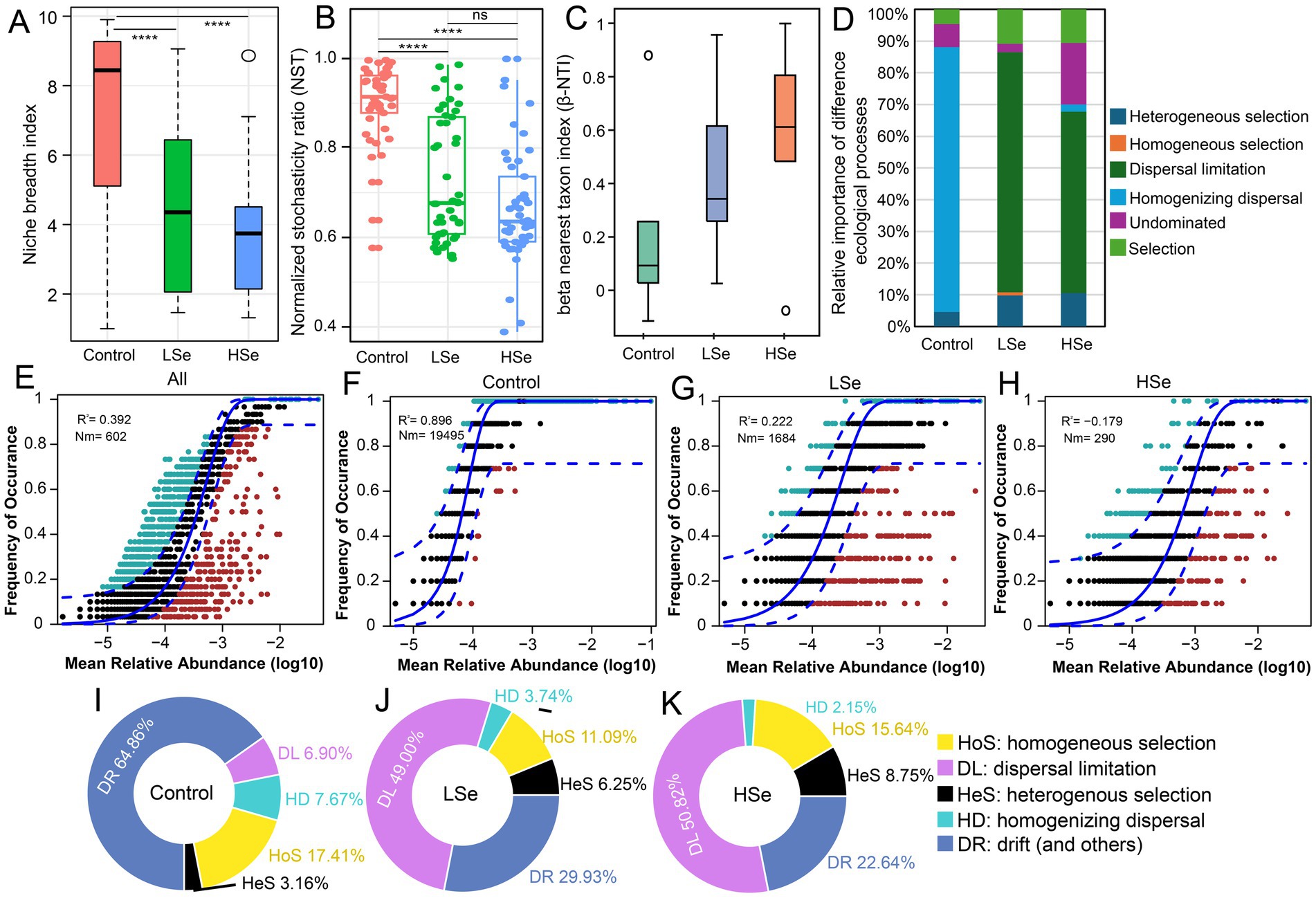
Figure 4. Impact of selenium contamination on bacterial community assembly processes. (A) Niche breadth across different samples. (B) Normalized stochasticity ratio (NST) based on Jaccard distances. (C) Distribution of beta nearest taxon index (β-NTI) among different samples. (D) Relative importance of different ecological processes, including heterogeneous selection, homogeneous selection, dispersal limitation, homogenizing dispersal, and drift, highlighting increased deterministic processes (heterogeneous selection). (E–H) Sloan neutral community model (NCM) fitting for different soils. (I–K) The relative contributions of stochastic and deterministic processes based on the infer community assembly mechanisms by phylogenetic-bin-based null model analysis (iCAMP).
To better identify the ecological drivers, βNTI values were used to assess community assembly mechanisms. βNTI values between −2 and 2 indicated that stochastic processes predominated across all samples (Figure 4C). However, a closer analysis revealed that in the Control group, bacterial communities were primarily regulated by homogenizing dispersal, while in the LSe and HSe groups, dispersal limitation became the key stochastic factor influencing community assembly (Figure 4D). To further investigate the role of stochastic processes, we applied the Sloan neutral community model (NCM). The R2 values of 0.896, 0.222, and −0.179 for the Control, LSe, and HSe groups (Figures 4E–H) highlighted that stochasticity significantly influenced bacterial community assembly in the absence of Se and other heavy metal stress but decreased with Se contamination. The m value, reflecting microbial dispersal ability, was substantially higher in the Control group (0.971) compared to Se-polluted soils (0.014–0.084) (Supplementary Table S2), suggesting that Se and other heavy metal contamination severely constrains microbial dispersal.
iCAMP analysis provided additional insights into the contributions of deterministic and stochastic processes (Figures 4I–K). In the Control group, stochastic processes (sum of DR, DL, and HD), including drift and homogenizing dispersal, explained the majority of bacterial community variation (72.53%), whereas deterministic processes accounted for 27.47% (Figure 4I). In contrast, stochastic processes dominated in the HSe group, explaining 76.21% of community variation, while deterministic processes contributed only 24.79% (Figure 4K). This shift highlights the increasing impact of selection pressure and dispersal constraints under Se pollution, as also supported by βNTI results. Notably, drift contributions declined significantly as heavy metal content increased (from 64.86 to 22.64%), while dispersal limitation rose sharply (from 6.9 to 50.82%). Overall, dispersal limitation (49–50.82%) emerged as the most significant stochastic factor driving bacterial community assembly under Se and other heavy metal stress, followed by drift (22.64–29.93%).
4 Discussion
4.1 Interactions between selenium, other heavy metals, and soil properties
In previous studies, conflicting results have emerged regarding the relationship between soil Se content and other elements such as Cu, Ni, Cd, Pb, and As. For instance, Jiang (2011) reported a negative correlation between Se and these elements, while Guo (2011) observed a positive correlation, findings that were further supported by Li et al. (2023). In our study, Se showed a negative correlation with As and Pb but a positive correlation with Cu and Ni. These differing correlations can be attributed to several factors, including the chemical properties of Se and the presence of other contaminants in the soil. Se exists in different forms (e.g., selenate, selenite, and elemental Se), each of which interacts differently with other elements depending on its concentration and speciation (Fernández-Martínez and Charlet, 2009). Additionally, other heavy metals or organic matter can influence how Se interacts with these elements, affecting the overall correlation patterns (Xiao, 2014). Plant species may also play a role in shaping these correlations. Certain plants, particularly Se-hyperaccumulator plants (Cardamine enshiensis), can alter the mobility and bioavailability of Se and other heavy metals through processes such as ion exchange, root exudation, and metal uptake (Kumar et al., 2017; Li et al., 2018). These plants, known for their ability to absorb high concentrations of metals, can influence the solubility of Se and its interactions with other metals like Cu, Ni, and Pb (El Mehdawi and Pilon-Smits, 2012).
Another significant issue associated with Se is its potential to exacerbate heavy metal pollution in soil. The observed coexistence relationships between Se and Cr, Ni, and Cu in Se-rich soils suggest that Se may enhance the mobility or bioavailability of these metals. For instance, selenate ions can form stable complexes with metal ions, increasing their solubility and transport in soil (Lenz and Lens, 2009; Torres et al., 2010). This effect is particularly relevant in soils with high Se content, where heavy metals may exceed regulatory thresholds due to increased mobilization.
Microbial activity also plays a critical role in modulating the effects of heavy metals on soil properties. Microbes can reduce selenate and selenite to elemental Se or volatilize it as dimethyl selenide, which can influence the mobility and bioavailability of Se (Jiang et al., 2024). Furthermore, the influence of Se on microbial and plant communities can indirectly affect heavy metal dynamics. For example, appropriate Se supplementation has been shown to reduce heavy metal accumulation in crops by modulating plant uptake pathways (Zhu et al., 2022). Additionally, microbial activity can affect soil pH and redox potential, which in turn influences the solubility of metal ions (Husson, 2013). This complex interplay between microbial activity, metal contamination, and soil properties underscores the difficulty in predicting the specific impact of Se and other heavy metals on soil physicochemical characteristics.
4.2 Effects of Se and other heavy metals on soil microbial communities
Heavy metal contamination significantly affects soil microbial communities due to its persistence and slow degradation in the environment. Se, as both an essential trace element and a potential contaminant, exerts a more complex effect on soil bacterial communities and their diversity (Kushwaha et al., 2022). At low concentrations, Se can promote microbial activity by participating in microbial metabolism (Jiang et al., 2024). However, at high concentrations, its toxicity inhibits microbial growth, disrupts cellular processes, and damages enzyme functions, leading to a decline in microbial diversity (Kushwaha et al., 2022). In this study, Se and other heavy metal concentration was closely associated with changes in bacterial community composition, with significant shifts observed in the relative abundance of dominant bacterial phyla and genera (Figures 1, 2). Certain selenium-tolerant bacteria, such as Proteobacteria and Actinobacteriota, increased in abundance in Se-contaminated soils, possibly due to their ability to reduce selenates (SeO₄2−) or selenites (SeO₃2−) to less toxic elemental Se (Se0) or volatile Se compounds (Shi et al., 2021). These microbes may play a key role in the biogeochemical cycling of Se. Researchers also found that Se was positively correlated with the growth of nitrogen-fixing bacteria, such as Rhizobiaceae and Frankia, and nitrifying bacteria like Proteobacteria, Firmicutes, and Chloroflexi (Lei et al., 2022; Tang et al., 2024), while Se inhibits the growth of Ascomycota (Yang X. et al., 2022). Moreover, the interaction between Se and other soil properties and pollutants plays an important role in regulating microbial communities. For example, Se can form complexes with other heavy metals (such as Cu and Ni), altering their bioavailability and potentially enhancing their toxicity to microbes or plants (Feng et al., 2021). This phenomenon may explain the observed changes in microbial community composition in soils co-contaminated with Se and heavy metals. Additionally, selenium’s regulation of soil redox potential and pH may indirectly influence microbial communities by altering nutrient and pollutant availability (Kushwaha et al., 2022).
4.3 Microbial co-occurrence network structure in response to Se and other heavy metal contamination
In nature, microbes do not exist in isolation but rather interact and adapt together to environmental pressures (Chun et al., 2021). Soil microbial networks can reveal these interactions and reflect the community’s response to environmental changes (Zhou et al., 2010). In this study, the microbial network exhibited higher connectivity and lower modularity under low Se and other heavy metal contamination conditions, suggesting denser interactions and less differentiation between microbial groups (Lurgi et al., 2019). This may be due to the selective pressure of low Se and other heavy metal concentrations, which promotes broader interactions among microbial communities (Sun et al., 2022). This phenomenon indicates that low Se and other heavy metal contamination may enhance the synergistic effects between different microbial populations, thus aiding community adaptation and stability. In contrast, the complexity of the microbial network drastically decreased under high Se and other heavy metal exposure. This indicates an increase in microbial interactions or niche-sharing within microbial community due to its smaller, more tightly connected structure (Sun et al., 2022). The toxic effects of high Se and other heavy metal concentrations likely imposed selective pressure, allowing only Se-tolerant species to thrive (Colpaert et al., 2011; Chun et al., 2021). These resilient species became the “hubs” of the network, but their dominance led to reduced diversity and ecological function within the community, causing fragmentation and loss of network functionality (Chun et al., 2021). This change highlights how high Se and other heavy metal concentrations disrupt microbial community dynamics, pushing certain taxa to the core while inhibiting others from growing.
Interestingly, despite the significant reduction in network complexity under high Se and other heavy metal concentrations, microbial community stability and adaptability were enhanced under low Se and other heavy metal contamination. A potential explanation for these observations is that low Se and other heavy metal contamination may promote cooperation and nutrient interactions among microbial communities, while high Se and other heavy metal contamination may limit microbial diversity and interactions, leading to a decline in community stability and resilience (Zaefarian et al., 2011). However, it is important to note that species associations based on correlation analysis may not fully reflect the true interactions among microbes, and some of the negative/positive correlations may be incidental or influenced by environmental factors. Therefore, caution is needed when interpreting these results in microbial community network analysis (Jiao et al., 2021). Nevertheless, negative/positive correlation information remains crucial for identifying potential species relationships within microbial communities, especially in complex environmental conditions, and provides valuable insights for understanding microbial interaction patterns (Jin et al., 2022).
4.4 Effects of Se and other heavy metals on microbial community assembly
Se and heavy metal contamination significantly altered the balance between stochastic and deterministic processes in microbial community assembly. This shift is likely due to the significant disturbance of the soil microenvironment caused by high Se and other heavy metal concentrations, such as enhanced toxicity and altered resource allocation, leading to selective enrichment of specific functional groups and exclusion of sensitive ones (Guo et al., 2023). Under low Se and other heavy metal contamination, stochastic processes remained dominant, with homogenizing dispersal promoting community stability (Howeth and Leibold, 2010). This may offer opportunities for microbial coexistence under environmental stress. In contrast, under high Se and other heavy metal contamination, environmental selection effects were clearly intensified, characterized by narrowed ecological niches, limited dispersal capacity, and dominant deterministic processes. This pattern is closely linked to the inhibitory effects of Se and other heavy metal toxicity on microbial diversity and biomass. Additionally, the enhancement of dispersal limitation, as a major stochastic process, likely reflects local adaptation of microorganisms in high-pollution environments (Dai et al., 2023). Tolerant groups, such as Proteobacteria and Actinobacteriota, were preferentially enriched, likely due to their ability to reduce selenate and selenite (Kang et al., 2024), gaining an advantage in niche competition (Jing et al., 2024). These results reveal the complex role of Se and other heavy metal contamination in microbial community dynamics: on one hand, deterministic processes driven by selective pressure enhance the regulation of community structure, while on the other hand, dispersal limitation (as the dominant stochastic process) significantly affects community stability and resilience. Under high Se and other heavy metal contamination, increased environmental stress favors the survival of specific microbial groups, while the influence of random drift on the community is reduced. Moreover, dispersal limitation becomes a key factor in shaping community assembly, especially in high Se and other heavy metal environments, suggesting that microbial distribution is constrained, which may impact the recovery capacity and ecological functions of microbial communities. Such changes in assembly mechanisms may further affect the functional stability and nutrient cycling efficiency of soil ecosystems, which is particularly significant in agricultural soils and pollution remediation scenarios.
5 Conclusion
In summary, selenium and heavy metal contamination exerts complex and significant effects on soil microbial communities, influencing both community structure and function. Our findings highlight the dominance of stochastic processes in microbial community assembly, especially in less contaminated environments, while deterministic processes play a more prominent role in selenium-contaminated soils due to heavy metal toxicity. These insights enrich our understanding of the ecological consequences of selenium and heavy metal pollution and provide a theoretical foundation for developing microbiological-based soil remediation strategies aimed at restoring ecosystem balance and functionality.
Data availability statement
The datasets presented in this study can be found in online repositories. The names of the repository/repositories and accession number(s) can be found in the article/Supplementary material.
Author contributions
ZW: Formal analysis, Writing – original draft, Writing – review & editing. GD: Data curation, Formal analysis, Investigation, Visualization, Writing – review & editing. CH: Data curation, Writing – review & editing. XH: Methodology, Writing – review & editing. XZ: Software, Writing – review & editing. ZF: Software, Writing – review & editing. YZ: Conceptualization, Writing – review & editing. MP: Conceptualization, Supervision, Writing – original draft, Writing – review & editing.
Funding
The author(s) declare that financial support was received for the research and/or publication of this article. This study was financially supported by the Natural Science Foundation of Hubei Province (2023AFB484), and the Open Fund of Hubei Key Laboratory of Biological Resources Protection and Utilization (Hubei Minzu University) (KYPT012305), the Open Fund of Hubei Engineering Research Center of Selenium Food Nutrition and Health Intelligent Technology (Hubei Minzu University) (No. PT082301).
Conflict of interest
The authors declare that the research was conducted in the absence of any commercial or financial relationships that could be construed as a potential conflict of interest.
Generative AI statement
The author(s) declare that no Gen AI was used in the creation of this manuscript.
Publisher’s note
All claims expressed in this article are solely those of the authors and do not necessarily represent those of their affiliated organizations, or those of the publisher, the editors and the reviewers. Any product that may be evaluated in this article, or claim that may be made by its manufacturer, is not guaranteed or endorsed by the publisher.
Supplementary material
The Supplementary material for this article can be found online at: https://www.frontiersin.org/articles/10.3389/fmicb.2025.1561678/full#supplementary-material
References
Banerjee, M., Chakravarty, D., Kalwani, P., and Ballal, A. (2022). Voyage of selenium from environment to life: beneficial or toxic? J. Biochem. Mol. Toxicol. 36:e23195. doi: 10.1002/jbt.23195
Bastian, M., Heymann, S., and Jacomy, M. (2009). Gephi: an open source software for exploring and manipulating networks. Proceedings of the International AAAI Conference on Web and Social Media.
Caruso, T., Chan, Y., Lacap, D. C., Lau, M. C., McKay, C. P., and Pointing, S. B. (2011). Stochastic and deterministic processes interact in the assembly of desert microbial communities on a global scale. ISME J. 5, 1406–1413. doi: 10.1038/ismej.2011.21
Chase, J. M., and Myers, J. A. (2011). Disentangling the importance of ecological niches from stochastic processes across scales. Philos. Trans. R. Soc. B 366, 2351–2363. doi: 10.1098/rstb.2011.0063
Chen, Y., Tao, S., Ma, J., Qu, Y., Sun, Y., Wang, M., et al. (2024). New insights into assembly processes and driving factors of urban soil microbial community under environmental stress in Beijing. Sci. Total Environ. 947:174551. doi: 10.1016/j.scitotenv.2024.174551
Chen, Z.-J., Tian, W., Li, Y.-J., Sun, L.-N., Chen, Y., Zhang, H., et al. (2021). Responses of rhizosphere bacterial communities, their functions and their network interactions to Cd stress under phytostabilization by Miscanthus spp. Environ. Pollut. 287:117663. doi: 10.1016/j.envpol.2021.117663
Chun, S.-J., Kim, Y.-J., Cui, Y., and Nam, K.-H. (2021). Ecological network analysis reveals distinctive microbial modules associated with heavy metal contamination of abandoned mine soils in Korea. Environ. Pollut. 289:117851. doi: 10.1016/j.envpol.2021.117851
Colpaert, J. V., Wevers, J. H., Krznaric, E., and Adriaensen, K. (2011). How metal-tolerant ecotypes of ectomycorrhizal fungi protect plants from heavy metal pollution. Ann. For. Sci. 68, 17–24. doi: 10.1007/s13595-010-0003-9
Dai, T., Su, Z., Zeng, Y., Bao, Y., Zheng, Y., Guo, H., et al. (2023). Wastewater treatment plant effluent discharge decreases bacterial community diversity and network complexity in urbanized coastal sediment. Environ. Pollut. 322:121122. doi: 10.1016/j.envpol.2023.121122
Deng, Y., Jiang, Y.-H., Yang, Y., He, Z., Luo, F., and Zhou, J. (2012). Molecular ecological network analyses. BMC Bioinformatics 13:113. doi: 10.1186/1471-2105-13-113
Dini-Andreote, F., Stegen, J. C., van Elsas, J. D., and Salles, J. F. (2015). Disentangling mechanisms that mediate the balance between stochastic and deterministic processes in microbial succession. Proc. Natl. Acad. Sci. U.S.A. 112, E1326–E1332. doi: 10.1073/pnas.1414261112
El Mehdawi, A., and Pilon-Smits, E. (2012). Ecological aspects of plant selenium hyperaccumulation. Plant Biol. 14, 1–10. doi: 10.1111/j.1438-8677.2011.00535.x
Feng, R., Wang, L., Yang, J., Zhao, P., Zhu, Y., Li, Y., et al. (2021). Underlying mechanisms responsible for restriction of uptake and translocation of heavy metals (metalloids) by selenium via root application in plants. J. Hazard. Mater. 402:123570. doi: 10.1016/j.jhazmat.2020.123570
Fernández-Martínez, A., and Charlet, L. (2009). Selenium environmental cycling and bioavailability: a structural chemist point of view. Rev. Environ. Sci. Biotechnol. 8, 81–110. doi: 10.1007/s11157-009-9145-3
Guo, Y. (2011). “Geochemistry of selenium in Enshi area and experimental study ofselenium-enriched crop cultivation” in Doctoral dissertation (Wuhan: China University of Geosciences).
Guo, R., Chen, Q., Wang, F., Fang, M., Li, L., Niu, H., et al. (2023). Selenium-contaminated water: recent advances in material function and adsorption performance. J. Environ. Chem. Eng. 11:110468. doi: 10.1016/j.jece.2023.110468
Hemmat-Jou, M. H., Safari-Sinegani, A. A., Che, R., Mirzaie-Asl, A., Tahmourespour, A., and Tahmasbian, I. (2021). Toxic trace element resistance genes and systems identified using the shotgun metagenomics approach in an Iranian mine soil. Environ. Sci. Pollut. Res. 28, 4845–4856. doi: 10.1007/s11356-020-10824-x
Howeth, J. G., and Leibold, M. A. (2010). Species dispersal rates alter diversity and ecosystem stability in pond metacommunities. Ecology 91, 2727–2741. doi: 10.1890/09-1004.1
Husson, O. (2013). Redox potential (Eh) and pH as drivers of soil/plant/microorganism systems: a transdisciplinary overview pointing to integrative opportunities for agronomy. Plant Soil 362, 389–417. doi: 10.1007/s11104-012-1429-7
Jiang, L. (2011). “WanYuan selenium-rich soil environmental geochemical characteristics of selenium” in Master thesis (Chengdu: Chengdu University of Technology).
Jiang, Z., Wang, Z., Zhao, Y., and Peng, M. (2024). Unveiling the vital role of soil microorganisms in selenium cycling: a review. Front. Microbiol. 15:1448539. doi: 10.3389/fmicb.2024.1448539
Jiao, S., Peng, Z., Qi, J., Gao, J., and Wei, G. (2021). Linking bacterial-fungal relationships to microbial diversity and soil nutrient cycling. mSystems 6:e01052. doi: 10.1128/msystems.01052-01020
Jin, X., Wang, Z., Wu, F., Li, X., and Zhou, X. (2022). Litter mixing alters microbial decomposer community to accelerate tomato root litter decomposition. Microbiol. Spectr. 10:e00186. doi: 10.1128/spectrum.00186-22
Jing, Z., Li, Q., Lu, J., Ma, J., Ye, F., Tu, S., et al. (2024). Revealing microbial community assembly patterns and succession process in the blackening process of black-odor water. Environ. Pollut. 352:124129. doi: 10.1016/j.envpol.2024.124129
Kang, Y., Ming, J., Fu, W., Long, L., Wen, X., Zhang, Q., et al. (2024). Selenium fertilizer improves microbial community structure and diversity of rhizospheric soil and selenium accumulation in tomato plants. Commun. Soil Sci. Plant Anal. 55, 1430–1444. doi: 10.1080/00103624.2024.2315931
Kumar, S. S., Kadier, A., Malyan, S. K., Ahmad, A., and Bishnoi, N. R. (2017). “Phytoremediation and rhizoremediation: uptake, mobilization and sequestration of heavy metals by plants” in Plant-microbe interactions in agro-ecological perspectives (Singapore: Springer), 367–394.
Kushwaha, A., Goswami, L., Lee, J., Sonne, C., Brown, R. J., and Kim, K.-H. (2022). Selenium in soil-microbe-plant systems: sources, distribution, toxicity, tolerance, and detoxification. Crit. Rev. Environ. Sci. Technol. 52, 2383–2420. doi: 10.1080/10643389.2021.1883187
Lei, Z., Li, Q., Tang, Y., Zhang, H., Han, C., Wang, X., et al. (2022). Selenium enhanced nitrogen accumulation in legumes in soil with rhizobia bacteria. J. Clean. Prod. 380:134960. doi: 10.1016/j.jclepro.2022.134960
Lenz, M., and Lens, P. N. (2009). The essential toxin: the changing perception of selenium in environmental sciences. Sci. Total Environ. 407, 3620–3633. doi: 10.1016/j.scitotenv.2008.07.056
Li, J.-T., Gurajala, H. K., Wu, L.-H., van der Ent, A., Qiu, R.-L., Baker, A. J., et al. (2018). Hyperaccumulator plants from China: a synthesis of the current state of knowledge. Environ. Sci. Technol. 52, 11980–11994. doi: 10.1021/acs.est.8b01060
Li, H., Zhang, H., and Lu, C. (2023). Chemical characteristics and environmental ecology of selenium-rich soil in Naore Village of Ziyang County, Shaanxi Province. J. Shandong Agric. Univ. 54, 406–412.
Liu, S., Shi, Y., Chen, J., Zhang, Z., Cao, H., Li, W., et al. (2024). Interspecific barrier effect driven by heavy metals makes soil bacterial functional assembly more stochastic. Environ. Res. 253:119153. doi: 10.1016/j.envres.2024.119153
Lurgi, M., Thomas, T., Wemheuer, B., Webster, N. S., and Montoya, J. M. (2019). Modularity and predicted functions of the global sponge-microbiome network. Nat. Commun. 10:992. doi: 10.1038/s41467-019-08925-4
Ma, Y., Guo, F., Zhu, H., Wu, Y., Guo, B., Yang, J., et al. (2024). Risk assessment and impact prediction of associated heavy metal pollution in selenium-rich farmland. Sci. Total Environ. 950:175321. doi: 10.1016/j.scitotenv.2024.175321
Ma, S., Qiao, L., Liu, X., Zhang, S., Zhang, L., Qiu, Z., et al. (2022). Microbial community succession in soils under long-term heavy metal stress from community diversity-structure to KEGG function pathways. Environ. Res. 214:113822. doi: 10.1016/j.envres.2022.113822
Marimuthu, R., Sankaranarayanan, B., Ali, S. M., de Sousa Jabbour, A. B. L., and Karuppiah, K. (2021). Assessment of key socio-economic and environmental challenges in the mining industry: implications for resource policies in emerging economies. Sustain. Prod. Consum. 27, 814–830. doi: 10.1016/j.spc.2021.02.005
Ning, D., Deng, Y., Tiedje, J. M., and Zhou, J. (2019). A general framework for quantitatively assessing ecological stochasticity. Proc. Natl. Acad. Sci. U.S.A. 116, 16892–16898. doi: 10.1073/pnas.1904623116
Ning, D., Yuan, M., Wu, L., Zhang, Y., Guo, X., Zhou, X., et al. (2020). A quantitative framework reveals ecological drivers of grassland microbial community assembly in response to warming. Nat. Commun. 11:4717. doi: 10.1038/s41467-020-18560-z
Nizamani, M. M., Hughes, A. C., Qureshi, S., Zhang, Q., Tarafder, E., Das, D., et al. (2024). Microbial biodiversity and plant functional trait interactions in multifunctional ecosystems. Appl. Soil Ecol. 201:105515. doi: 10.1016/j.apsoil.2024.105515
Olaniran, A. O., Balgobind, A., and Pillay, B. (2013). Bioavailability of heavy metals in soil: impact on microbial biodegradation of organic compounds and possible improvement strategies. Int. J. Mol. Sci. 14, 10197–10228. doi: 10.3390/ijms140510197
Peng, M., Deng, G., Hu, C., Hou, X., and Wang, Z. (2024). Bioremediation potential of Rhodococcus qingshengii PM1 in sodium selenite-contaminated soil and its impact on microbial community assembly. Microorganisms 12:2458. doi: 10.3390/microorganisms12122458
Rijk, I. (2023). Effects of heavy metal contamination on carbon and nitrogen cycling: an ecological approach to assess risks to soil functions. Örebro: Örebro University.
Rijk, I. J., and Ekblad, A. (2020). Carbon and nitrogen cycling in a lead polluted grassland evaluated using stable isotopes (δ13C and δ15N) and microbial, plant and soil parameters. Plant Soil 449, 249–266. doi: 10.1007/s11104-020-04467-7
Rosenfeld, C. E., James, B. R., and Santelli, C. M. (2018). Persistent bacterial and fungal community shifts exhibited in selenium-contaminated reclaimed mine soils. Appl. Environ. Microbiol. 84:e01394. doi: 10.1128/AEM.01394-18
Sarkar, S., Kumar, R., Kumar, A., Kumar, U., Singh, D. K., Mondal, S., et al. (2022). “Role of soil microbes to assess soil health” in Structure and functions of pedosphere (Singapore: Springer), 339–363.
Sarsan, S., Pandiyan, A., Rodhe, A. V., and Jagavati, S. (2021). “Synergistic interactions among microbial communities” in Microbes in microbial communities: ecological and applied perspectives (Singapore: Springer), 1–37.
Sharma, M., Sharma, S., Paavan,, Gupta, M., Goyal, S., Talukder, D., et al. (2024). Mechanisms of microbial resistance against cadmium—a review. J. Environ. Health Sci. Eng. 22, 13–30. doi: 10.1007/s40201-023-00887-6
Shi, L.-D., Lv, P.-L., McIlroy, S. J., Wang, Z., Dong, X.-L., Kouris, A., et al. (2021). Methane-dependent selenate reduction by a bacterial consortium. ISME J. 15, 3683–3692. doi: 10.1038/s41396-021-01044-3
Shi, S., Nuccio, E. E., Shi, Z. J., He, Z., Zhou, J., and Firestone, M. K. (2016). The interconnected rhizosphere: high network complexity dominates rhizosphere assemblages. Ecol. Lett. 19, 926–936. doi: 10.1111/ele.12630
Silver, S. (1996). Bacterial resistances to toxic metal ions-a review. Gene 179, 9–19. doi: 10.1016/S0378-1119(96)00323-X
Sloan, W. T., Lunn, M., Woodcock, S., Head, I. M., Nee, S., and Curtis, T. P. (2006). Quantifying the roles of immigration and chance in shaping prokaryote community structure. Environ. Microbiol. 8, 732–740. doi: 10.1111/j.1462-2920.2005.00956.x
Stegen, J. C., Lin, X., Konopka, A. E., and Fredrickson, J. K. (2012). Stochastic and deterministic assembly processes in subsurface microbial communities. ISME J. 6, 1653–1664. doi: 10.1038/ismej.2012.22
Sun, R., Chen, Y., Han, W., Dong, W., Zhang, Y., Hu, C., et al. (2020). Different contribution of species sorting and exogenous species immigration from manure to soil fungal diversity and community assemblage under long-term fertilization. Soil Biol. Biochem. 151:108049. doi: 10.1016/j.soilbio.2020.108049
Sun, T., Li, G., Mazarji, M., Delaplace, P., Yang, X., Zhang, J., et al. (2024). Heavy metals drive microbial community assembly process in farmland with long-term biosolids application. J. Hazard. Mater. 468:133845. doi: 10.1016/j.jhazmat.2024.133845
Sun, C., Wu, P., Wang, G., and Kong, X. (2022). Heavy metal pollution decreases the stability of microbial co-occurrence networks in the rhizosphere of native plants. Front. Environ. Sci. 10:979922. doi: 10.3389/fenvs.2022.979922
Tang, J., Zhang, J., Ren, L., Zhou, Y., Gao, J., Luo, L., et al. (2019). Diagnosis of soil contamination using microbiological indices: a review on heavy metal pollution. J. Environ. Manag. 242, 121–130. doi: 10.1016/j.jenvman.2019.04.061
Tang, Y., Zhou, Y., Wang, P., Ge, L., Lou, W., Yan, X., et al. (2024). Selenium-mediated shaping of citrus rhizobiome for promotion in root growth and soil phosphorus activation. J. Agric. Food Chem. 72, 16624–16637. doi: 10.1021/acs.jafc.4c02761
Torres, J., Pintos, V., Domínguez, S., Kremer, C., and Kremer, E. (2010). Selenite and selenate speciation in natural waters: interaction with divalent metal ions. J. Solut. Chem. 39, 1–10. doi: 10.1007/s10953-009-9491-3
Wen, P., Feng, S.-w., Liang, J.-L., Jia, P., Liao, B., Shu, W.-s., et al. (2024). Heavy metal pollution in farmland soils surrounding mining areas in China and the response of soil microbial communities. Soil Secur. 17:100173. doi: 10.1016/j.soisec.2024.100173
Wu, S., Zou, H., Li, N., Yan, X., and Liu, W. (2024). Heavy metal stress strengthens the stability and complexity of microbial molecular ecological networks in Sanyang wetland sediments. J. Oceanol. Limnol., 43, 103–118. doi: 10.1007/s00343-024-4028-6
Xiao, Y. (2014). “Heavy metal’s migration and transformation of crop in Se-rich soil and effected by Se” in Master thesis (Haikou: Hainan University).
Xiao, S., Zhang, Q., Chen, X., Dong, F., Chen, H., Liu, M., et al. (2019). Speciation distribution of heavy metals in uranium mining impacted soils and impact on bacterial community revealed by high-throughput sequencing. Front. Microbiol. 10:1867. doi: 10.3389/fmicb.2019.01867
Yan, C., Wang, F., Geng, H., Liu, H., Pu, S., Tian, Z., et al. (2020). Integrating high-throughput sequencing and metagenome analysis to reveal the characteristic and resistance mechanism of microbial community in metal contaminated sediments. Sci. Total Environ. 707:136116. doi: 10.1016/j.scitotenv.2019.136116
Yang, X., Li, Y., Ma, J., Wu, F., Wang, L., Sun, L., et al. (2022). Comparative physiological and soil microbial community structural analysis revealed that selenium alleviates cadmium stress in Perilla frutescens. Front. Plant Sci. 13:1022935. doi: 10.3389/fpls.2022.1022935
Yang, R., Luo, L., Zhu, M., Zan, S., Guo, F., He, Y., et al. (2022). Selenium (Se), mercury (Hg) and physicochemical properties co-mediate the bacterial communities in a typical collapsed Lake receiving Se-and Hg-containing mine water. Water Air Soil Pollut. 233:403. doi: 10.1007/s11270-022-05870-9
Yuan, M. M., Guo, X., Wu, L., Zhang, Y., Xiao, N., Ning, D., et al. (2021). Climate warming enhances microbial network complexity and stability. Nat. Clim. Chang. 11, 343–348. doi: 10.1038/s41558-021-00989-9
Zaefarian, F., Rezvani, M., Rejali, F., Ardakani, M. R., and Noormohammadi, G. (2011). Effect of heavy metals and arbuscular mycorrhizal fungal on growth and nutrients (N, P, K, Zn, Cu, and Fe) accumulation of alfalfa (Medicago Sativa L.). Am. Eurasian J. Agric. Environ. Sci. 11, 346–352.
Zhang, M., Zhang, T., Zhou, L., Lou, W., Zeng, W., Liu, T., et al. (2022). Soil microbial community assembly model in response to heavy metal pollution. Environ. Res. 213:113576. doi: 10.1016/j.envres.2022.113576
Zhao, X., Huang, J., Lu, J., and Sun, Y. (2019). Study on the influence of soil microbial community on the long-term heavy metal pollution of different land use types and depth layers in mine. Ecotoxicol. Environ. Saf. 170, 218–226. doi: 10.1016/j.ecoenv.2018.11.136
Zhou, J., Deng, Y., Luo, F., He, Z., Tu, Q., and Zhi, X. (2010). Functional molecular ecological networks. mBio 1:e00169. doi: 10.1128/mBio.00169-10
Zhou, T., Li, L., Zhang, X., Zheng, J., Zheng, J., Joseph, S., et al. (2016). Changes in organic carbon and nitrogen in soil with metal pollution by Cd, Cu, Pb and Zn: a meta-analysis. Eur. J. Soil Sci. 67, 237–246. doi: 10.1111/ejss.12327
Zhu, Y., Dong, Y., Zhu, N., and Jin, H. (2022). Foliar application of biosynthetic nano-selenium alleviates the toxicity of Cd, Pb, and Hg in Brassica chinensis by inhibiting heavy metal adsorption and improving antioxidant system in plant. Ecotoxicol. Environ. Saf. 240:113681. doi: 10.1016/j.ecoenv.2022.113681
Keywords: selenium, heavy metal, bacterial diversity, community assembly, stochastic and deterministic processes
Citation: Wang Z, Deng G, Hu C, Hou X, Zhang X, Fan Z, Zhao Y and Peng M (2025) Microbial diversity and community assembly in heavy metal-contaminated soils: insights from selenium-impacted mining areas. Front. Microbiol. 16:1561678. doi: 10.3389/fmicb.2025.1561678
Edited by:
Enzong Xiao, Guangzhou University, ChinaCopyright © 2025 Wang, Deng, Hu, Hou, Zhang, Fan, Zhao and Peng. This is an open-access article distributed under the terms of the Creative Commons Attribution License (CC BY). The use, distribution or reproduction in other forums is permitted, provided the original author(s) and the copyright owner(s) are credited and that the original publication in this journal is cited, in accordance with accepted academic practice. No use, distribution or reproduction is permitted which does not comply with these terms.
*Correspondence: Yong Zhao, emhhb195b25nOTBAMTI2LmNvbQ==; Mu Peng, cGVuZ211MTAyNUBob3RtYWlsLmNvbQ==