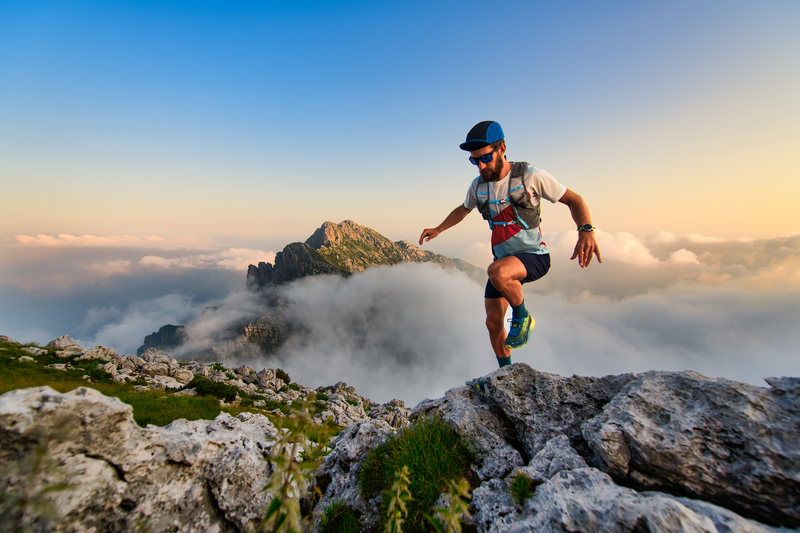
95% of researchers rate our articles as excellent or good
Learn more about the work of our research integrity team to safeguard the quality of each article we publish.
Find out more
ORIGINAL RESEARCH article
Front. Microbiol. , 25 February 2025
Sec. Infectious Agents and Disease
Volume 16 - 2025 | https://doi.org/10.3389/fmicb.2025.1531739
This article is part of the Research Topic Women in Infectious Agents and Disease: 2024 View all 10 articles
Infections due to multidrug-resistant (MDR) Escherichia coli are associated with severe morbidity and mortality, worldwide. Microbial drug resistance is a complex phenomenon which is conditioned by an interplay of several genomic, transcriptomic and proteomic factors. Here, we have conducted an integrated transcriptomics and proteomics analysis of MDR E. coli to identify genes which are differentially expressed at both mRNA and protein levels. Using RNA-Seq and SWATH-LC MS/MS it was discerned that 763 genes/proteins exhibited differential expression. Of these, 52 genes showed concordance in differential expression at both mRNA and protein levels with 41 genes exhibiting overexpression and 11 genes exhibiting under expression. Bioinformatic analysis using GO-terms, COG and KEGG functional annotations revealed that the concordantly overexpressed genes of MDR E. coli were involved primarily in biosynthesis of secondary metabolites, aminoacyl-tRNAs and ribosomes. Protein–protein interaction (PPI) network analysis of the concordantly overexpressed genes revealed 81 PPI networks and 10 hub proteins. The hub proteins (rpsI, aspS, valS, lysS, accC, topA, rpmG, rpsR, lysU, and spmB) were found to be involved in aminoacylation of tRNA and lysyl-tRNA and, translation. Further, it was discerned that three hub proteins - smpB, rpsR, and topA were non homologous to human proteins and were involved in several biological pathways directly and/or indirectly related to antibiotic stress. Also, absence of homology ensures a little cross-reactivity of their inhibitors/drugs with human proteins and undesirable side effects. Thus, these proteins might be explored as novel drug targets against both drug-resistant and -sensitive populations of E. coli.
Escherichia coli are common gut bacteria which are frequently reported from medical and veterinary clinics (Croxen et al., 2013; Nhung et al., 2015). The soaring use of antibiotics in agriculture, veterinary, poultry and clinics has increased the incidence of antimicrobial resistant bacteria, including E. coli. Several reports have indicated that E. coli have become resistant to many antibiotics of the beta-lactam, aminoglycoside, (fluoro) quinolone classes and also to the newer antibiotics like colistin, which is a matter of grave concern (Liu et al., 2016; Peng et al., 2021; Singh et al., 2022). Antimicrobial resistant infections have not only escalated the costs of medical treatment, in many cases it might lead to treatment failures (Dadgostar, 2019). According to an estimate, by the year 2050 antimicrobial resistant infections might lead to 10 million human deaths (de Kraker et al., 2016).
Antimicrobial resistance (AMR) is a complex phenomenon. Several mechanisms underlying bacterial AMR have been explained, including genetic change(s) or mutations in the target genes, horizontal acquisition of AMR genes, reduced cell wall permeability, efflux pumps etc. (Reygaert, 2018; Martinez and Baquero, 2000). Most of these mechanisms are linked with changes in the bacterial genomes and have been extensively studied using PCR-amplification of the resistance gene(s) and, more recently by whole genome sequencing. However, genomic analyses cannot predict the levels of expressed genes and proteins. Genomes express themselves via the transcriptomes and proteomes, which are the ultimate functional moieties of the cell. But their expression levels fluctuate with external stimuli like environmental stresses and drug exposure (Freiberg et al., 2004; Martínez and Rojo, 2011). Recent reports have shown that alterations in the metabolic pathways is a widely used strategy adopted by bacteria to exhibit drug tolerance, persistence and resistance (Martínez and Rojo, 2011; Bhargava and Collins, 2015; Meylan et al., 2017; Koeva et al., 2017; Goossens et al., 2020). However, the specific metabolism-related protein profiles of drug-resistant bacteria are still poorly understood. During drug exposure, bacteria might adopt alternative cellular functions and/or over- or under express various genes to overcome the drug effect. Thus, the knowledge of transcriptome and proteome of drug-resistant pathogens is necessary for a comprehensive understanding of microbial drug resistance, identification of novel drug targets and for development of new drug molecules. Studies based on elucidating the role of microbial transcriptomes and proteomes in drug resistance are quite less (Singhal et al., 2012; Chopra et al., 2013; Thai et al., 2017; Shen et al., 2021; Pan et al., 2023). Analysis of differentially expressed genes and proteins of drug-resistant bacteria can reveal novel strategies adopted by the bacteria to overcome the effects of drugs.
Thus, the aim of this study was to conduct a combined comparative transcriptomic and proteomic analyses of drug sensitive and -resistant strains of enteropathogenic E. coli to understand the mechanisms and biological pathways underlying bacterial multidrug resistance. Two strains of phylogroup D of E. coli isolated earlier by us and preserved as glycerol stocks (50% v/v) in a −80°C deep refrigerator were investigated (Bajaj et al., 2015). Molecular typing of these strains in our laboratory had revealed that these strains belonged to the same genomic clade (Bajaj et al., 2015). Also, whole genome analysis of these strains revealed that they were genetically similar as their average nucleotide identity (ANI) was 97.21% (Aswal et al., 2023). E. coli IP9 was drug-sensitive while E. coli IPE was multidrug resistant exhibiting resistance for many β-lactams, fluoroquinolones and kanamycin (Bajaj et al., 2015; Singh et al., 2022). The differentially expressed genes (DEGs) and differentially expressed proteins (DEPs) of multidrug resistant (MDR) E. coli were discerned using RNA-sequencing (RNA-Seq) and SWATH-LC MS/MS, respectively. The genes which were observed to be differentially expressed at both mRNA and protein levels were identified and their biological functions and metabolic pathways were elucidated with help of ontological analysis using GO-term enrichment, Cluster of Orthologous Genes (COG)-classification and Kyoto Encyclopaedia of Genes and Genomes (KEGG) pathway analysis. Protein–protein interaction (PPI) networks of the proteins corresponding to these genes were determined to discern their interactome, followed by identification of the hub proteins. To the best of our knowledge, this is the first study which has used an integrated proteomics, transcriptomic and bioinformatics approach to understand the mechanistic details of drug resistance and identify novel drug targets against MDR E. coli.
In this study, E. coli strains - E. coli IP9 and E. coli IPE isolated earlier from an urban river of India and preserved as glycerol stocks (50% v/v) in a −80°C deep refrigerator were investigated (Bajaj et al., 2015). Triplex-PCR based typing had revealed that these strains belonged to phylogroup D and were of the same genomic clade as revealed by REP-, ERIC- and BOX-PCR based genotypic fingerprinting (Bajaj et al., 2015). E. coli IP9 was a drug sensitive isolate while E. coli IPE was a MDR strain exhibiting resistance for many β-lactams, fluoroquinolones and kanamycin (Bajaj et al., 2015; Singh et al., 2022). The antibiotic susceptibilities of these strains were earlier determined by the Kirby-Bauer disk diffusion susceptibility test and confirmed by PCR-amplification of the relevant genes (Bajaj et al., 2015; Singh et al., 2022). Recently, whole genome sequences of these strains were determined and submitted to the NCBI genome database with accession numbers: GCA_026183935.1 for E. coli IPE and GCA_026183955.1 for E. coli IP9 (Aswal et al., 2023). The ANI of E. coli IP9 and IPE was 97.21%, indicating a significant similarity in their genomes. These strains were revived in our laboratory by overnight incubation in LB broth at 37°C, 200 rpm. The cells were harvested by centrifugation at 8000 rpm for 10 min (at 4°C) from the exponential phase (OD600 = 0.8) cultures.
Bacteria were collected by centrifugation at 4, 472 x g followed by washing the bacterial pellet with 1X PBS solution. RNA was isolated from both the strains using the Qiagen RNeasy mini kit following the manufacturer’s instructions. Briefly, a cell lysis buffer was added to the cell pellet, mixed by vigorous shaking for 10 min followed by addition of an equal volume of 100% ethanol. The samples were then transferred on a RNeasy micro column for “on-column” DNase treatment. The RNA was then eluted in 20 μL of nuclease-free water. The library preparation was performed using Illumina specific adaptors. The adaptor ligated products were barcoded and 12 cycles of PCR was performed. The PCR products were cleaned using AMPure XP beads. The final enriched library was eluted in 15 μL of 0.1X TE buffer. Sequencing was performed on a Novaseq 6,000 using 150PE chemistry. Paired end sequencing of the transcriptomes of the three biological replicates of the MDR (E. coli IPE) and drug sensitive (E. coli IP9) strains was done with a read length of 150.
Nf-core/rnaseq (ver. 3.11.2)1 module of the nf-core project was used to analyze the transcriptomics data. Nf-core/rnaseq incorporates several tools for analysis. FastQC (ver. v0.11.9) was used to determine the quality of the sequencing reads. Adapter trimming was done using Trim Galore (ver. 0.6.7). SortMeRNA (ver. 4.3.4) was used to remove ribosomal RNA reads. RSEM (ver. 1.3.1), SALMON (ver. 1.10.1) and HISTAT2 (ver. 2.2.1) were used to map the sequencing reads on the reference genome of E. coli K-12 substrain MG1655 (RefSeq assembly; accession GCF_000005845.2). Since this study involved analysis of prokaryotic RNA-Seq data, the parameters used were --featurecounts_feature_type = CDS, −-featurecounts_group_type = gene and --skip_rseqc. RNA-Seq data quality control was performed using RSeQC (ver. 3.0.1). RNA-Seq specific quality control (QC) metrics, such as sequencing depth, read distribution and coverage uniformity was performed with RSeQC (ver. 3.0.1) and feature-wise read counting was done using subread feature Counts (ver. 2.0.1). The comprehensive QC report was prepared using MultiQC (ver. 1.13).2
Analysis of the DEGs of the MDR and -sensitive strain was performed using the R language and iDEP RNA-Seq differential gene expression pipeline (release 0.96) of the DESeq2 (ver. 1.36.0). Genes were considered differentially expressed when the Benjamini–Hochberg multiple testing adjusted p-value was lesser than 0.05 (padj ≤0.05). Genes whose log2 fold change (log2FC) values were > 1.0 or < −1.0 were considered as Differentially Expressed Genes (DEGs).
Bacterial cells were washed with normal saline solution and dispersed in sonication buffer containing 50 mM Tris–HCl,10 mM MgCl2, 0.1% sodium azide, 1 mM phenylmethylsulphonyl fluoride and 1 mM; pH 7.4 (Singhal et al., 2012). Cells at a concentration of 1 g wet weight /5 mL of lysis buffer were broken down using a sonicator at 35% amplitude for 10 min at 4°C. The homogenate was centrifuged at 12,000 xg for 30 min at 4°C and the clear supernatant was overnight precipitated at -20ο C with chilled acetone. The precipitated protein was collected by centrifugation (12,000 xg, 20 min), air dried and suspended in appropriate volume of protein dissolving buffer. Protein concentration was determined using the Bradford assay (Bradford, 1976).
Equal amount of protein samples was digested with trypsin and analyzed using a Triple TOF 5600 mass spectrometer (AB Sciex, USA) equipped with Eskigent MicroLC 200 system (Eskigent, Dublin, CA) with an Eskigent C18 - reverse phase column. 1 microgram of digested proteins was desalted online using the online C18 trap column with 98% water, 2% acetonitrile and 0.1% formic acid at flow rate of 5 μL/min for 10 min. The desalted peptides were eluted on a C18 reverse phase analytical column for separation and analysis. A 120 min gradient in multiple steps (ranging from 5–50% acetonitrile in water containing 0.1% formic acid) was set for eluting the peptides from the ChromXP analytical column. The separated peptides were ionized and entered into the mass spectrometer and multiply charged molecules were fragmented using the IDA™ (information dependent data acquisition) criteria of the analyst software for library generation. In brief, 500 ng of all the samples were pooled together and run using IDA criteria of the mass spectrometer for library generation in triplicate. Mass spectrometric data for the first quadrupole was acquired in the range of 350 Da to 1,250 Da whereas 20 most abundant multiply charged peptides were fragmented in the mass range of 150 Da to 1,500 Da in the second quadrupole or collision induced dissociation cell. The accumulation time for each MS/MS experiment was 50 ms. The ionization potential for the turbo V ion source was kept at 4500 V and temperature for source was set 150°C, GS1 and GS2 were at 19 and 15 L/min, respectively. Declustering potential (DP) was set at 80 V. The resultant IDA data files were analyzed in ProteinPilot™ (Sciex software) for identification of peptides and proteins against E. coli proteome using Paragon algorithm and the pooled peptide list was used as the spectral library for SWATH analysis. Experiments were performed in technical replicates.
In SWATH™ Sciex (Sequential window acquisition of all theoretical Spectra) DIA (Data independent acquisition) acquisition method, fixed value Q1 transmission window was kept at 12 Da for the mass range of 350–1,250 Da. Total 75 sequential windows were acquired independently with an accumulation time of 62 ms, along with three technical replicates for each of the sets. Total cycle time was kept constant at <5 s. For label free quantification, peak extraction and spectral alignment was performed using PeakView® 2.2 software with the following parameters: number of peptides selected for quantitation 2, confidence of peptide identification was set as more than 95%, number of fragment ions for each peptide was set as 5, extraction ion chromatogram (XIC) peak width was fixed 30 ppm for matching the RT whereas the XIC extraction window set for matching the peptide across the different samples was set at 5 min. For statistical interpretation, MarkerView software™ (ver. 1.3. 1; AB Sciex) was used. The SWATH acquisition data was processed using SWATH™ Acquisition MicroApp (ver. 2.0) in PeakView® software.
Raw data was search processed with ProteinPilot™ using the Paragon and Progroup Algorithms for protein and peptide identification. Analysis was also done using the integrated tools in Protein Pilot at 1% false discovery rate (FDR). The identification file was used as spectral library in the quantitation experiment for SWATH/DIA. Retention time calibration was performed using the most abundant peptide across all the samples. Proteins whose log2 fold change (log2FC) values were > 0.5 or < −0.5 (padj ≤0.05) were considered as differentially expressed proteins (DEPs). Both DEGs and DEPs were visualized using ggVolcanoR.3
To elucidate the functions of DEGs and DEPs, GO-term enrichment analysis was performed with ShinyGO4 using E. coli K-12 MG1655 as the reference strain. The enrichment analysis was done at all the three levels of GO-terms: biological process (BP), cellular component (CC) and molecular function (MF). Gene sets that ranked in the top ten for BP, CC and MF were individually enriched for DEGs and DEPs. This data was used to construct GO plots, which show the number of genes and their relative enrichment significance for each enriched category.
The concordance in differentially expressed mRNA transcripts and proteins of the MDR strain was evaluated by creating a nine-quadrant correlation plot for DEGs with log2FC expression >0.1 or < −0.1 and for DEPs with log2FC expression >0.5 or < −0.5 (p ≤ 0.05). The biological functions and metabolic pathways of the concordantly differentially expressed genes were determined using the GO-term enrichment, COG classification and KEGG pathway analysis. For GO-term enrichment ShinyGO (p ≤ 0.05) was used, for COG classification COGclassifier (v1.0.5) was used and for KEGG pathway analysis Database for Annotation, Visualization and Integrated Discovery (DAVID) was used.
The protein–protein interactions (PPIs) in the concordantly differentially expressed genes were discerned using STRING database (ver.12.05) with ≥0.3 as the threshold strength. The top ten hub proteins were identified using the Maximal Clique Centrality (MCC) algorithm which is reportedly the most effective method of finding hub nodes. The hub proteins were discerned from the PPI network using cytoHubba plugin of Cytoscape ver. 3.9.1.6
The similarity of the hub proteins with the human proteins was determined using NCBI-BLAST. Hub proteins exhibiting ≥30% sequence identity and ≥ 80% coverage with human proteins, were considered as homologs of the human proteins and were removed from further analysis. The remaining hub proteins were considered as non-homologous to human proteins (NHHPs; Garg et al., 2020).
The antibiotic resistance genes of MDR E. coli were discerned using the Comprehensive Antibiotic Resistance Database (CARD; McArthur et al., 2013). A PPI network of the proteins encoded by the antibiotic resistance genes and the hub proteins was generated using the STRING database to generate their interactome (at an interaction score of 0.3). The interactome was analyzed using k-mean clustering and the clusters were enriched for biological functions at FDR < =0.05.
Analysis of RNA-Seq data of the MDR and drug-sensitive E. coli strains revealed that 4,101 genes were differentially expressed. Of these, the log2FC value of 2,127 genes was beyond the defined threshold (log2FC > 0.1 for overexpressed genes and log2FC < −0.1 for unexpressed genes; p ≤ 0.05). Hence, 2,127 genes were considered as DEGs and analysed further. Of these, 982 genes were found to be overexpressed, while 1,145 genes were found to be under expressed by the E. coli MDR strain (Figure 1A; Supplementary Table S1).
Figure 1. Volcano plots depicting differential gene/protein expressions at log2FC beyond the defined threshold; p-value ≤0.05: (A) DEGs with log2FC values beyond the range −1 to 1, (B) DEPs with log2FC values beyond the range − 0.5 to 0.5. The over- and under-expressed genes/proteins are shown in red and blue color, respectively. The plots were generated using ggvolcanoR (https://ggvolcanor.erc.monash.edu/).
Analysis of the SWATH-LC MS data of the MDR and drug-sensitive E. coli strains revealed that 770 proteins were differentially expressed. Of these, the log2FC values of 270 proteins were beyond the defined threshold (log2FC > 0.5 for upregulated proteins and log2FC < −0.5 for downregulated proteins; p ≤ 0.05) hence, only these proteins were considered as DEPs and included in further analysis. Of these, 89 proteins were found to be upregulated, while 187 proteins were found to be downregulated by the E. coli MDR strain (Figure 1B; Supplementary Table S2).
The overexpressed DEGs were found to be involved in the biological processes related to amide biosynthesis (112 proteins), peptide metabolic process (100 proteins), translation (97 proteins), peptide biosynthesis (96 proteins), ribonucleoprotein complex biogenesis (69 proteins), organelle assembly (53 proteins), ribosome assembly (48 proteins) and ribonucleoprotein complex (43 proteins). The overexpressed DEGs were located within the ribosome (59 proteins), ribonucleoprotein complex (56 proteins), ribosomal subunit (54 proteins), large ribosomal subunit (32 proteins), small ribosomal subunit (22 proteins) and exonuclease repair complex (4 proteins). The molecular functions of the overexpressed DEGs were primarily related to structural molecular activity (59 proteins), structural constituent of ribosome (56 proteins), rRNA binding (52 proteins), tRNA binding (31 proteins), aminoacyl-tRNA ligase activity (20 proteins), ribosome binding (20 proteins), translation regulator activity (18 proteins) (Supplementary Figure S1A). The under expressed DEGs were found to be involved in biological processes related to carboxylic acid catabolic process (100 proteins), carbohydrate transport (79 proteins), monocarboxylic acid catabolic process (58 proteins), response to xenobiotic stimuli (26 proteins) and primary amino acid metabolic processes (18 proteins). They were located within the cell projection (46 proteins), bacterial-type flagella (24 proteins), pilus (22 proteins), bacterial-type flagella basal body (18 proteins), and type II protein secretion system (11 proteins). Their molecular functions were primarily related to carbohydrate transmembrane transporter activity (52 proteins), protein-phosphocysteine-sugar phosphotransferase activity (18 proteins), carbohydrate cation symporter activity (15 proteins), motor activity (8 proteins) and CoA-transferase activity (7 proteins) (Supplementary Figure S1B).
Overexpressed DEPs were found to be involved in biological processes related to amide biosynthesis process (12 proteins). They were located in intracellular regions (56 proteins), cytoplasm (55 proteins) and cytosol (53 proteins). Their molecular functions were related to RNA binding (11 proteins), OB-fold nucleic acid binding domain (3 proteins), polyketide sugar unit biosynthesis (3 proteins) and tRNA synthetase class II (3 proteins) (Supplementary Figure S1C). Under expressed DEPs were involved in biological processes related to organonitrogen compound biosynthesis process (49 proteins), cellular protein metabolic process (33 proteins), small molecule biosynthesis process (31 proteins), cellular amide metabolic process (28 proteins), amide biosynthesis (23 proteins), peptide metabolic process (21 proteins), peptide biosynthesis (19 proteins), translation (19 proteins), fatty acid biosynthesis (7 proteins) and mannose transmembrane transport (3 proteins). They were located within the ribosomal subunit (11 proteins), cytosolic ribosome (11 proteins), large ribosomal unit (6 proteins), small ribosomal subunit (5 proteins), NADH dehydrogenase complex (4 proteins), respiratory chain complex (4 proteins), ATPase complex (3 proteins) and acetyl-CoA carboxylase (2 proteins). Their molecular functions were related to protein binding (51 proteins), ligase activity (18 proteins), rRNA binding (11 proteins), iron ion binding (14 proteins), structural constituent of ribosome (10 proteins), ferrous ion binding (8 proteins) and mannose transmembrane transporter (3 proteins) (Supplementary Figure S1D).
A total of 763 genes/proteins were discerned which exhibited the log2FC differential expression values within the defined thresholds (Supplementary Table S2). The Pearson’s correlation coefficient in the log2FC expression values of transcriptomics and proteomics datasets was 0.078 (p-value = 0). Of these 763 genes/proteins, 101 genes/proteins were present in 1st and 9th quadrants suggesting a non-concordance in the expression levels of mRNA and their corresponding proteins. The expression of the genes present in the 1st quadrant was upregulated at mRNA level but downregulated at the protein level. While the expression of the genes present in the 9th quadrant was upregulated at protein level but downregulated at the mRNA level. In the 2nd and the 8th quadrants, 283 genes were present which were significantly differentially expressed at the mRNA level but did not exhibit any differential expression at the protein level. In the 4th and the 6th quadrants, 120 genes were present which did not exhibit any significant differential expression at mRNA level but a significant differential expression at the protein level. In the 3rd and the 7th quadrants, 52 genes were present which exhibited a positive correlation at both transcription and translation levels (Figure 2). Thus, 52 genes were discerned in the E. coli MDR strain which were differentially expressed at both mRNA and protein levels. Of these, 41 genes were found to be overexpressed (3rd quadrant, Table 1) and 11 genes were under expressed (7th quadrant, Table 2).
Figure 2. The nine-quadrant plot showing concordance in the log2FC values of differentially expressed genes (DEGs) and differentially expressed proteins (DEPs). Genes which show positive correlation at both mRNA and protein levels are present in 3rd and 7th quadrants and are shown in blue color.
Table 1. Details of the concordantly overexpressed genes of E. coli MDR strain discerned by combined transcriptomics and proteomics.
Table 2. Details of the concordantly under-expressed genes of E. coli MDR strain discerned by combined transcriptomics and proteomics.
GO-term enrichment analysis of the 41 genes which exhibited overexpression at both mRNA and protein levels revealed that these were primarily involved in biological processes related to cellular amide metabolic biosynthesis (11 proteins), amide biosynthesis (10 proteins), ncRNA metabolic process (9 proteins), translation (8 proteins), peptide biosynthesis (8 proteins) and tRNA aminoacylation for protein translation (4 proteins). At the molecular functional level, they exhibited nucleic acid binding (16 proteins), RNA binding (12 proteins), tRNA binding (5 proteins), aminoacyl-tRNA ligase activity (4 proteins) and lysine-tRNA ligase activity (2 proteins). They were located in cytoplasm (28 proteins), non-membranous bound organelle (5 proteins) and intracellular organelle (5 proteins) (Figure 3A).
Figure 3. GO-enriched terms associated with the genes exhibiting differential expression at both mRNA and protein levels in E. coli MDR strain: (A) GO-terms associated with the 41 concordant overexpressed genes (B) GO-terms associated with the 10 concordant under-expressed genes. The X-axis shows the GO-terms and the Y-axis shows the fold enrichment values associated with each GO-term.
GO-term enrichment analysis of the 11 genes which exhibited under expression at both mRNA and protein levels revealed that these were primarily involved in biological processes related to maltodextrin transport (2 proteins), polysaccharides transport (2 proteins) and oligosaccharide transport (2 proteins). At the molecular functional level, they exhibited transition metal binding activities (5 proteins) and ferric ion binding (2 proteins). All the proteins were located on the ATP-binding cassette transporter complex (Figure 3B).
COG classification of the 41 genes which exhibited overexpression at both mRNA and protein levels revealed that they belonged to 15 functional categories. Of these, 11 genes were involved in translation, ribosomal structure and biogenesis, six genes in cell wall/membrane/envelope biogenesis, five genes in post-translational modification, turnover and chaperon, three genes in coenzyme transport and metabolism, two genes each in replication, recombination and repair, energy production and conversion, carbohydrate transport and metabolism and unknown function. One gene each was involved in transcription, signal transduction mechanism, intracellular trafficking, secretion and vesicular transport, amino acid transport and metabolism, nucleoside transport and metabolism, lipid transport and metabolism, and inorganic ion transport and metabolism (Figure 4A). The 11 genes which exhibited similarity in under expression at both mRNA and protein levels were classified into seven COG functional categories. Three genes were involved in carbohydrate transport and metabolism, two in inorganic ion transport and metabolism, one each in energy production and conversion, in amino acid transport and metabolism, coenzyme transport and metabolism, lipid transport and metabolism and general function (Figure 4B).
Figure 4. COG-based functional classification of the genes exhibiting differential expression at both mRNA and protein levels in E. coli MDR strain: (A) overexpressed genes (B) under-expressed genes.
The KEGG pathway enrichment analysis of the 41 genes which were overexpressed at both mRNA and protein levels revealed that seven of these genes were involved in biosynthesis of secondary metabolites (enrichment score 1.485704), four genes in aminoacyl-tRNA biosynthesis pathway (enrichment score 2.608108) and three genes in ribosome biosynthesis (enrichment score 2.783654) (Figure 5). The KEGG pathway enrichment analysis of the 11 genes concordantly under expressed at both mRNA and protein levels did not yield any result.
Figure 5. KEGG-enriched pathways and enrichment score associated with the concordantly overexpressed genes in E. coli MDR strain.
Analysis of the PPI network of the proteins corresponding to the 41 genes concordantly overexpressed at both transcription and translation levels revealed that they were involved in 81 PPIs (Figure 6). On the basis of the maximal clique centrality (MCC) score, ten proteins were identified as the hub proteins of these interaction networks. These were: aspS (aspartate-tRNA ligase/ aspartyl-tRNA synthetase), valS (valine-tRNA ligase/ valyl-tRNA synthetase), lysS (lysine-tRNA ligase/lysyl-tRNA synthetase), lysU (lysine-tRNA ligase/lysyl-tRNA synthetase), rpmG (large ribosomal subunit protein bL33 (50S ribosomal protein L33)), rpsI (small ribosomal subunit protein uS9/30S ribosomal protein S9), rpsR (small ribosomal subunit protein/30S ribosomal protein S18), spmB (small protein B/SsrA-binding protein), topA (DNA topoisomerase 1) and accC (biotin carboxylase). The hub proteins and their MCC scores have been detailed in Table 3. The hub proteins were found to be primarily involved in tRNA and lysyl-tRNA aminoacylation, and translation. At the molecular level they were involved in tRNA and RNA binding and were located in ribosomal subunits and cytosol. However, no PPI networks were discerned for the proteins corresponding to the concordant under expressed genes.
Figure 6. Schematic representation of the PPI networks of the concordantly overexpressed genes, identification of the hub proteins and potential drug targets against MDR E. coli.
Table 3. Details of the top ten hub proteins discerned from the PPI networks of concordantly overexpressed genes of MDR E. coli strain.
To avoid cross-reactivity of the potential inhibitors of the hub proteins with the human proteins, human homologs of the hub proteins were discerned in the human proteome using NCBI-BLAST. Of the ten potential hub proteins which could be potential drug targets, seven hub proteins (rpsI, aspS, valS, lysS, accC, rpmG and lysU) showed homology with the human proteins with identity ranging between 39 to 47% and alignment coverage ranging from 81 to 99%. Two hub proteins rpsR and smpB did not show any homology with the human proteins, while topA showed 25.28% identity and 65% alignment coverage with the human proteins (Table 3).
CARD analysis revealed 69 genes whose products were related to antibiotic resistance in MDR E. coli (Supplementary Table S3). Of these, 47 genes could be mapped by STRING on the PPI network along with the hub proteins - topA, smpB and rpsR. Three prominent clusters were observed in the interactome: cluster 1 composed of 39 proteins involved in antibiotic detoxification (acrA, acrB, acrD, acrE, acrF, acrR, acrS, baeR, cpxA, crp, emrA, emrB, emrK, emrY, evgA, evgS, gadX, hha, kdpE, leuO, marA, marR, mdfA, mdtA, mdtB, mdtC, mdtE, mdtF, mdtG, mdtH, mdtM, mdtN, mdtO, mdtP, mprA, soxR, soxS, tolC and ybiH). Cluster2 contained 7 proteins involved in lipid A biosynthesis (eptA, ugd, arnT, arnC, bacA, yojI and msbA) and cluster 3 contained 4 proteins involved in RNA binding (rpsR, smpB, topA and rsmA). The complete interactome has been depicted in Supplementary Figure S2.
An in-depth understanding of AMR is paramount for development of new antibiotics/drugs, vaccines and diagnostic tools. AMR has been mainly studied using standard microbiological growth-inhibition methods, PCR-amplification of the AMR genes, gene sequencing for mutation detection and more recently by whole genome sequencing (Boolchandani et al., 2019). Recently, several studies have indicated that bacteria withstand antibiotic stress by modifying their metabolic pathways (Richter et al., 2017; Stokes et al., 2019). However, the role of microbial metabolic pathways and other proteins in the context of bacterial drug resistance is inadequately understood. In the present study, we have used transcriptomics, proteomics and bioinformatics approaches to identify differentially expressed genes and proteins of MDR E. coli followed by extensive bioinformatics-based studies to discern novel drug targets against antibiotic resistant E. coli.
The differentially expressed genes of the MDR E. coli were inferred by integrating RNA-Seq and SWATH-LC MS/MS datasets to identify genes exhibiting concordant differential expression at both transcriptomics and proteomics levels. Thus, 763 genes/proteins were discerned whose log2FC differential expression values attained the defined thresholds. The results from both the technologies were integrated for unambiguous and technical artifact-free interpretations. The Pearson’s correlation coefficient between the log2FC expression values of transcriptomics and proteomics datasets was 0.078 (p-value = 0), which is low. This is, however, not very surprising because several studies have reported that mRNA expression levels and protein level abundance do not necessarily correlate, due to biological differences between transcription and translation processes and, also due to experimental limitations (Gygi et al., 1999; Brötz-Oesterhelt et al., 2005). Moreover, the correlation between mRNA and protein abundance was reportedly not very strong in bacteria (Gygi et al., 2000). From the biology viewpoint, differential RNA and protein turnover, post-translational modifications, proteolytic processing events and allosteric protein interactions might result in a low concordance in mRNA and protein levels. From the technological viewpoint, challenges in the experimental design and data interpretation methods might be responsible for non-concordance in mRNA and protein levels.
On the basis of the nine-quadrant correlation plot, it was discerned that 52 genes of the MDR E. coli showed a positive correlation in differential gene expression at both transcription and translation levels. Of these, 41 genes were overexpressed and 11 genes were under expressed by MDR E. coli at both mRNA and protein levels. GO-term enrichment analysis of these 41 overexpressed genes revealed that they were primarily involved in biological processes related to amide/peptide/protein biosynthesis and translation. KEGG pathway enrichment analysis of the 41 overexpressed genes revealed that they were primarily involved in biosynthesis of aminoacyl-tRNA, ribosomes and secondary metabolites. Thus, our results are similar to a recent study which reported that proteins predominantly related to metabolism, transcriptional and translational regulation, and stress response showed differential expression in drug resistant E. coli and other bacteria (Mehrotra et al., 2023). Thus, inhibitors of amide/peptide/protein biosynthesis and translation can be suitable candidates against drug resistant E. coli. However, such drug molecules which inhibit bacterial translation and related processes are available (e.g., aminoglycosides, tetracyclines macrolides etc.) but increasing instances of bacterial resistance against these antibiotics are being reported. Thus, alternative drug targets/strategies need to be discovered.
Investigating the PPIs/interactome not only helps in understanding the function(s) of individual protein(s), it also helps in characterizing the various pathways in which proteins might be involved. Analysis of the PPI networks of the proteins corresponding to the 41 concordantly overexpressed genes of the E. coli MDR strain revealed that they were involved in 81 PPI networks. Ten proteins were identified as the hub proteins of these PPI networks. These were: aspS (aspartate-tRNA ligase/ aspartyl-tRNA synthetase), valS (valine-tRNA ligase/ valyl-tRNA synthetase), lysS (lysine-tRNA ligase/lysyl-tRNA synthetase), lysU (lysine-tRNA ligase/lysyl-tRNA synthetase), rpmG (large ribosomal subunit protein bL33 (50S ribosomal protein L33)), rpsI (small ribosomal subunit protein uS9/30S ribosomal protein S9), rpsR (small ribosomal subunit protein/30S ribosomal protein S18), spmB (small protein B/SsrA-binding protein), topA (DNA topoisomerase 1) and accC (biotin carboxylase). At the functional level, the majority of the hub proteins were involved in aminoacylation of tRNA and protein-translation.
It is well known that hub proteins can interact with multiple proteins and they are critically important for the whole biological system due to their high connectivity (Higurashi et al., 2008; Ota et al., 2016). Inhibition/elimination of the function of a hub protein can influence multiple downstream pathways and biological networks which also affects the organismal fitness (He and Zhang, 2006). Thus, blocking the activity of hub proteins with appropriate inhibitors/drugs can be a useful strategy against a pathogen (Garg et al., 2020). This implies that the hub proteins discerned in this study might be explored as potential drug targets against MDR E. coli. However, if a homolog of a potential drug target is present in the host, it might lead to cross-reactivity of the inhibitor/drug with the host protein and, consequently undesirable side effects. Therefore, to avoid cross-reactivity of the potential inhibitors of the hub proteins with the host proteins, homology between the human and the hub proteins was discerned by NCBI-BLAST. Of the ten hub proteins, seven hub proteins (rpsI, aspS, valS, lysS, accC, rpmG and lysU) exhibited homology with the human proteins, their identity ranging between 39 to 47% and alignment coverage ranging between 81 to 99%. However, two hub proteins - smpB and rpsR and did not show any homology with the human proteins, while topA showed 25.28% identity and 65% alignment coverage with the human proteins. Hence, the hub proteins smpB, rpsR and topA might be explored as potential drug targets against MDR E. coli.
SmpB is reportedly a small protein (B) which along with ssrA (small stable RNA A) is required during the process of bacterial trans-translation for recycling of ribosomes stalled on defective mRNA (Li et al., 2013). In Neisseria gonorrhoeae, Mycobacterium tuberculosis, and Staphylococcus aureus trans-translation was reportedly essential for viability and virulence of bacteria (Personne and Parish, 2014; Liu et al., 2018; Aron et al., 2021). Also, disruption of the trans-translation pathway was reported to result in increased sensitivity of bacteria for antibiotics (Huang et al., 2019; Campos-Silva et al., 2021). Thus, SmpB has been proposed as a potential drug target against several bacteria (Alumasa and Keiler, 2015).
RpsR is a small ribosomal subunit (30S) protein which is an essential component of the protein biosynthetic machinery of E. coli (Shoji et al., 2011). Previous studies have reported that the expression of the gene encoding rpsR was greatly enhanced during biofilm formation in E. coli (Schembri et al., 2003; Beloin et al., 2004). A PPI network based study based on integration of differentially expressed genes of E. coli during stress conditions implicated that many RNA binding proteins (including rpsR) played an important role in bacterial response to stress (Nagar et al., 2016). In Deinococcus radiodurans, a highly upregulated expression of the ribosomal proteins rplB, rpsL, rpsR was reported in response to oxidative stress (Gao et al., 2020).
TopA is a DNA topoisomerase I enzyme which regulates global and local DNA supercoiling. DNA supercoiling affects many DNA centred processes such as, DNA replication, recombination, transcription and transposition (Drlica, 1992; Usongo and Drolet, 2014). Interestingly, the levels of DNA supercoiling are dependent on processes like transcription (Liu and Wang, 1987), nutrient availability and growth environment (Goldstein and Drlica, 1984; Balke and Gralla, 1987; Cheung et al., 2003). TopA activity has been reportedly essential for bacterial response to acid exposure, heat shock and antibiotics (Qi et al., 1996; Weinstein-Fischer et al., 2000; Stewart et al., 2005; Yang et al., 2015). Also, topA activity has been shown to be important for adaptation of E. coli for high temperature and oxidative stress (Qi et al., 1999; Tse-Dinh, 2000; Weinstein-Fischer et al., 2000).
To summarize, integrative proteo-transcriptomics revealed several genes which were differentially expressed by MDR E. coli. Bioinformatic analysis using GO-terms, COG and KEGG annotations revealed that the 41 overexpressed genes were primarily involved in tRNA aminoacylation and peptide and amide biosynthesis. Analysis of the PPI networks of the upregulated proteins revealed ten hub proteins of which, three hub proteins viz. smpB, rpsR and topA were non-homologous to human proteins. Several published reports have indicated an important role of these proteins in resistance for antibiotics and stress adaptation in E. coli. Moreover, inhibitors of these hub proteins would exhibit novel mechanisms of action hence, emergence of resistance against these seems unlikely in the near future. Further PPI network studies of the antibiotic resistance genes of MDR E. coli with the hub proteins also confirmed that smpB, rpsR and topA interacted with several antibiotic resistance genes. Since these hub proteins regulate several biological pathways of E. coli, directly and/or indirectly related to antibiotic stress, inhibitors of these proteins can be effective against both drug-resistant and -sensitive populations of E. coli. Moreover, non-homology of these proteins with the host proteins, leaves little scope for cross-reactivity with human proteins and undesirable side effects. However, further experiments including a greater number of drug-resistant and -sensitive strains of E. coli are required to understand the complex phenomenon as microbial drug resistance and explore the utility of the hub proteins smpB, rpsR and topA as novel drug targets against E. coli. Also, a judicious use of inhibitors of these novel drug-targets is warranted to minimize the possibility of resistance emergence against them, in future.
The transcriptomics datasets of this study are accessible as BioProject PRJNA1066082. E. coli IPE (MDR) and E. coli IP9 (drug sensitive) raw reads are available with SRA accessions SRR27601718 and SRR27601719, respectively. Proteomics datasets of this study are available via ProteomeXchange with identifier PXD048998. R script to generate 9 quadrant plot to integrate transcriptomics and proteomics data is available in Figshare (Kumar et al., 2024).
MA: Formal analysis, Methodology, Writing – original draft, Data curation, Investigation. NiS: Data curation, Formal analysis, Writing – review & editing. NeS: Formal analysis, Writing – review & editing, Methodology, Writing – original draft. MK: Data curation, Project administration, Resources, Supervision, Writing – review & editing.
The author(s) declare that financial support was received for the research, authorship, and/or publication of this article. The work was funded by the Indian Council of Medical Research [grant no: ISRM/12 (38)/2022], Faculty Research Programme Grant of Delhi University under Institute of Eminence (grant no: IoE/2021/12/FRP) and Department of Biotechnology (grant nos. BT/PR40180/BTIS/137/59/2023 and BT/PR40160/BTIS/137/64/2023). MA was supported by the CSIR-JRF scheme [grant number: 09/045/(1637)/2019-EMR-1] and ICMR [grant no: ISRM/12 (38)/2022]. NeS was supported by ICMR-Research Associateship [BMI/11 (29)/2022].
The authors declare that the research was conducted in the absence of any commercial or financial relationships that could be construed as a potential conflict of interest.
The author(s) declared that they were an editorial board member of Frontiers, at the time of submission. This had no impact on the peer review process and the final decision.
The authors declare that no Generative AI was used in the creation of this manuscript.
All claims expressed in this article are solely those of the authors and do not necessarily represent those of their affiliated organizations, or those of the publisher, the editors and the reviewers. Any product that may be evaluated in this article, or claim that may be made by its manufacturer, is not guaranteed or endorsed by the publisher.
The Supplementary material for this article can be found online at: https://www.frontiersin.org/articles/10.3389/fmicb.2025.1531739/full#supplementary-material
1. ^https://github.com/nf-core/rnaseq, accessed on 13 May 2023.
3. ^https://ggvolcanor.erc.monash.edu/
Alumasa, J. N., and Keiler, K. C. (2015). Clicking on trans-translation drug targets. Front. Microbiol. 6:498. doi: 10.3389/fmicb.2015.00498
Aron, Z. D., Mehrani, A., Hoffer, E. D., Connolly, K. L., Srinivas, P., Torhan, M. C., et al. (2021). Trans-translation inhibitors bind to a novel site on the ribosome and clear Neisseria gonorrhoeae in vivo. Nat. Commun. 12:1799. doi: 10.1038/s41467-021-22012-7
Aswal, M., Singhal, N., and Kumar, M. (2023). Genomic analysis of phylogroup D Escherichia coli strains using novel de-novo reference-based guided assembly. Sci Data. 10:573. doi: 10.1038/s41597-023-02444-0
Bajaj, P., Singh, N. S., Kanaujia, P. K., and Virdi, J. S. (2015). Distribution and molecular characterization of genes encoding CTX-M and AmpC β-lactamases in Escherichia coli isolated from an Indian urban aquatic environment. Sci. Total Environ. 505, 350–356. doi: 10.1016/j.scitotenv.2014.09.084
Balke, V. L., and Gralla, J. D. (1987). Changes in the linking number of supercoiled DNA accompany growth transitions in Escherichia coli. J. Bacteriol. 169, 4499–4506. doi: 10.1128/jb.169.10.4499-4506.1987
Beloin, C., Valle, J., Latour-Lambert, P., Faure, P., Kzreminski, M., Balestrino, D., et al. (2004). Global impact of mature biofilm lifestyle on Escherichia coli K-12 gene expression. Mol. Microbiol. 51, 659–674. doi: 10.1046/j.1365-2958.2003.03865.x
Bhargava, P., and Collins, J. J. (2015). Boosting bacterial metabolism to combat antibiotic resistance. Cell Metab. 21, 154–155. doi: 10.1016/j.cmet.2015.01.012
Boolchandani, M., D’Souza, A. W., and Dantas, G. (2019). Sequencing-based methods and resources to study antimicrobial resistance. Nat. Rev. Genet. 20, 356–370. doi: 10.1038/s41576-019-0108-4
Bradford, M. M. (1976). A rapid and sensitive method for the quantitation of microgram quantities of protein utilizing the principle of protein-dye binding. Anal. Biochem. 72, 248–254. doi: 10.1006/abio.1976.9999
Brötz-Oesterhelt, H., Bandow, J. E., and Labischinski, H. (2005). Bacterial proteomics and its role in antibacterial drug discovery. Mass Spectrom. Rev. 24, 549–565. doi: 10.1002/mas.20030
Campos-Silva, R., D'Urso, G., Delalande, O., Giudice, E., Macedo, A. J., and Gillet, R. (2021). Trans-translation is an appealing target for the development of new antimicrobial compounds. Microorganisms 10:3. doi: 10.3390/microorganisms10010003
Cheung, K. J., Badarinarayana, V., Selinger, D. W., Janse, D., and Church, G. M. (2003). A microarray-based antibiotic screen identifies a regulatory role for supercoiling in the osmotic stress response of Escherichia coli. Genome Res. 13, 206–215. doi: 10.1101/gr.401003
Chopra, S., Ramkissoon, K., and Anderson, D. C. (2013). A systematic quantitative proteomic examination of multidrug resistance in Acinetobacter baumannii. J. Proteome 84, 17–39. doi: 10.1016/j.jprot.2013.03.008
Croxen, M. A., Law, R. J., Scholz, R., Keeney, K. M., Wlodarska, M., and Finlay, B. B. (2013). Recent advances in understanding enteric pathogenic Escherichia coli. Clin. Microbiol. Rev. 26, 822–880. doi: 10.1128/CMR.00022-13
Dadgostar, P. (2019). Antimicrobial resistance: implications and costs. Infect Drug Resist. 12, 3903–3910. doi: 10.2147/IDR.S234610
de Kraker, M. E. A., Stewardson, A. J., and Harbarth, S. (2016). Will 10 million people die a year due to antimicrobial resistance by 2050? PLoS Med. 13:e1002184. doi: 10.1371/journal.pmed.1002184
Drlica, K. (1992). Control of bacterial DNA supercoiling. Mol. Microbiol. 6, 425–433. doi: 10.1111/j.1365-2958.1992.tb01486.x
Freiberg, C., Brötz-Oesterhelt, H., and Labischinski, H. (2004). The impact of transcriptome and proteome analyses on antibiotic drug discovery. Curr. Opin. Microbiol. 7, 451–459. doi: 10.1016/j.mib.2004.08.010
Gao, L., Zhou, Z., Chen, X., Zhang, W., Lin, M., and Chen, M. (2020). Comparative proteomics analysis reveals new features of the oxidative stress response in the Polyextremophilic bacterium Deinococcus radiodurans. Microorganisms 8:451. doi: 10.3390/microorganisms8030451
Garg, A., Singhal, N., and Kumar, M. (2020). Discerning novel drug targets for treating Mycobacterium avium ss. Paratuberculosis-associated autoimmune disorders: an in silico approach. Brief. Bioinform. 22:bbaa195. doi: 10.1093/bib/bbaa195
Goldstein, E., and Drlica, K. (1984). Regulation of bacterial DNA supercoiling: plasmid linking numbers vary with growth temperature. Proc. Natl. Acad. Sci. USA 81, 4046–4050. doi: 10.1073/pnas.81.13.4046
Goossens, S. N., Sampson, S. L., and Van Rie, A. (2020). Mechanisms of drug-induced tolerance in Mycobacterium tuberculosis. Clin. Microbiol. Rev. 34, e00141–e00120. doi: 10.1128/CMR.00141-20
Gygi, S. P., Corthals, G. L., Zhang, Y., Rochon, Y., and Aebersold, R. (2000). Evaluation of two-dimensional gel electrophoresis-based proteome analysis technology. Proc. Natl. Acad. Sci. USA 97, 9390–9395. doi: 10.1073/pnas.160270797
Gygi, S. P., Rochon, Y., Franza, B. R., and Aebersold, R. (1999). Correlation between protein and mRNA abundance in yeast. Mol. Cell. Biol. 19, 1720–1730. doi: 10.1128/MCB.19.3.1720
He, X., and Zhang, J. (2006). Why do hubs tend to be essential in protein networks? PLoS Genet. 2:e88. doi: 10.1371/journal.pgen.0020088
Higurashi, M., Ishida, T., and Kinoshita, K. (2008). Identification of transient hub proteins and the possible structural basis for their multiple interactions. Protein Sci. 17, 72–78. doi: 10.1110/ps.073196308
Huang, Y., Alumasa, J. N., Callaghan, L. T., Baugh, R. S., Rae, C. D., Keiler, K. C., et al. (2019). A small-molecule inhibitor of trans-translation synergistically interacts with cathelicidin antimicrobial peptides to impair survival of Staphylococcus aureus. Antimicrob. Agents Chemother. 63, e02362–e02318. doi: 10.1128/AAC.02362-18
Koeva, M., Gutu, A. D., Hebert, W., Wager, J. D., Yonker, L. M., O'Toole, G. A., et al. (2017). An Antipersister strategy for treatment of chronic Pseudomonas aeruginosa infections. Antimicrobial Agents Chemother. 61, e00987–e00917. doi: 10.1128/AAC.00987-17
Kumar, M., Aswal, M., Singh, N., and Singhal, N. (2024). R script to generate nine quadrant plot for integrating transcriptomics and proteomics data figshare. Available online at: https://figshare.com/articles/dataset/R_script_to_generate_nine_quadrant_plot_for_integrating_transcriptomics_and_proteomics_data/26840833/1
Li, J., Ji, L., Shi, W., Xie, J., and Zhang, Y. (2013). Trans-translation mediates tolerance to multiple antibiotics and stresses in Escherichia coli. J. Antimicrob. Chemother. 68, 2477–2481. doi: 10.1093/jac/dkt231
Liu, W., Rochat, T., Toffano-Nioche, C., Le Lam, T. N., Bouloc, P., and Morvan, C. (2018). Assessment of Bona fide sRNAs in Staphylococcus aureus. Front. Microbiol. 9:228. doi: 10.3389/fmicb.2018.00228
Liu, L. F., and Wang, J. C. (1987). Supercoiling of the DNA template during transcription. Proc. Natl. Acad. Sci. USA 84, 7024–7027. doi: 10.1073/pnas.84.20.7024
Liu, Y. Y., Wang, Y., Walsh, T. R., Yi, L. X., Zhang, R., Spencer, J., et al. (2016). Emergence of plasmid-mediated colistin resistance mechanism MCR-1 in animals and human beings in China: a microbiological and molecular biological study. Lancet Infect. Dis. 16, 161–168. doi: 10.1016/S1473-3099(15)00424-7
Martinez, J. L., and Baquero, F. (2000). Mutation frequencies and antibiotic resistance. Antimicrob. Agents Chemother. 44, 1771–1777. doi: 10.1128/AAC.44.7.1771-1777.2000
Martínez, J. L., and Rojo, F. (2011). Metabolic regulation of antibiotic resistance. FEMS Microbiol. Rev. 35, 768–789. doi: 10.1111/j.1574-6976.2011.00282.x
McArthur, A. G., Waglechner, N., Nizam, F., Yan, A., Azad, M. A., Baylay, A. J., et al. (2013). The comprehensive antibiotic resistance database. Antimicrob. Agents Chemother. 57, 3348–3357. doi: 10.1128/AAC.00419-13
Mehrotra, T., Konar, D., Pragasam, A. K., Kumar, S., Jana, P., Babele, P., et al. (2023). Antimicrobial resistance heterogeneity among multidrug-resistant gram-negative pathogens: phenotypic, genotypic, and proteomic analysis. Proc. Natl. Acad. Sci. USA 120:e2305465120. doi: 10.1073/pnas.2305465120
Meylan, S., Porter, C. B. M., Yang, J. H., Belenky, P., Gutierrez, A., Lobritz, M. A., et al. (2017). Carbon sources tune antibiotic susceptibility in Pseudomonas aeruginosa via tricarboxylic acid cycle control. Cell Chem Biol. 24, 195–206. doi: 10.1016/j.chembiol.2016.12.015
Nagar, S. D., Aggarwal, B., Joon, S., Bhatnagar, R., and Bhatnagar, S. (2016). A network biology approach to decipher stress response in Bacteria using Escherichia coli as a model. Omics 20, 310–324. doi: 10.1089/omi.2016.0028
Nhung, N. T., Cuong, N. V., Campbell, J., Hoa, N. T., Bryant, J. E., Truc, V. N., et al. (2015). High levels of antimicrobial resistance among Escherichia coli isolates from livestock farms and synanthropic rats and shrews in the Mekong Delta of Vietnam. Appl. Environ. Microbiol. 81, 812–820. doi: 10.1128/AEM.03366-14
Ota, M., Gonja, H., Koike, R., and Fukuchi, S. (2016). Multiple-localization and hub proteins. PLoS One 11:e0156455. doi: 10.1371/journal.pone.0156455
Pan, Z., Guo, J., Zhong, Y., Fan, L., and Su, Y. (2023). Gentamicin resistance to related to fatty acid metabolism based on transcriptome analysis. Can. J. Microbiol. 69, 328–338. doi: 10.1139/cjm-2023-0036
Peng, J. J., Balasubramanian, B., Ming, Y. Y., Niu, J. L., Yi, C. M., Ma, Y., et al. (2021). Identification of antimicrobial resistance genes and drug resistance analysis of Escherichia coli in the animal farm environment. J. Infect. Public Health 14, 1788–1795. doi: 10.1016/j.jiph.2021.10.025
Personne, Y., and Parish, T. (2014). Mycobacterium tuberculosis possesses an unusual tmRNA rescue system. Tuberculosis (Edinb.) 94, 34–42. doi: 10.1016/j.tube.2013.09.007
Qi, H., Menzel, R., and Tse-Dinh, Y. C. (1996). Effect of the deletion of the sigma 32-dependent promoter (P 1) of the Escherichia coli topoisomerase I gene on thermotolerance. Mol. Microbiol. 21, 703–711. doi: 10.1046/j.1365-2958.1996.241390.x
Qi, H., Menzel, R., and Tse-Dinh, Y. C. (1999). Increased thermosensitivity associated with topoisomerase I deletion and promoter mutations in Escherichia coli. FEMS Microbiol. Lett. 178, 141–146. doi: 10.1111/j.1574-6968.1999.tb13770.x
Reygaert, W. C. (2018). An overview of the antimicrobial resistance mechanisms of bacteria. AIMS Microbiol. 4, 482–501. doi: 10.3934/microbiol.2018.3.482
Richter, M. F., Drown, B. S., Riley, A. P., Garcia, A., Shirai, T., Svec, R. L., et al. (2017). Predictive compound accumulation rules yield a broad-spectrum antibiotic. Nature 545, 299–304. doi: 10.1038/nature22308
Schembri, M. A., Kjaergaard, K., and Klemm, P. (2003). Global gene expression in Escherichia coli biofilms. Mol. Microbiol. 48, 253–267. doi: 10.1046/j.1365-2958.2003.03432.x
Shen, C., Shen, Y., Zhang, H., Xu, M., He, L., and Qie, J. (2021). Comparative proteomics demonstrates altered metabolism pathways in Cotrimoxazole- resistant and amikacin-resistant isolates. Front. Microbiol. 12:773829. doi: 10.3389/fmicb.2021.773829
Shoji, S., Dambacher, C. M., Shajani, Z., Williamson, J. R., and Schultz, P. G. (2011). Systematic chromosomal deletion of bacterial ribosomal protein genes. J. Mol. Biol. 413, 751–761. doi: 10.1016/j.jmb.2011.09.004
Singh, N. S., Singhal, N., Kumar, M., and Virdi, J. S. (2022). Public health implications of plasmid-mediated quinolone and aminoglycoside resistance genes in inhabiting a major anthropogenic river of India. Epidemiol. Infect. 150, 1–21. doi: 10.1017/S095026882200053X
Singhal, N., Sharma, P., Kumar, M., Joshi, B., and Bisht, D. (2012). Analysis of intracellular expressed proteins of Mycobacterium tuberculosis clinical isolates. Proteome Sci. 10:14. doi: 10.1186/1477-5956-10-14
Stewart, N., Feng, J., Liu, X., Chaudhuri, D., Foster, J. W., Drolet, M., et al. (2005). Loss of topoisomerase I function affects the Rpo S-dependent and GAD systems of acid resistance in Escherichia coli. Microbiology 151, 2783–2791. doi: 10.1099/mic.0.28022-0
Stokes, J. M., Lopatkin, A. J., Lobritz, M. A., and Collins, J. J. (2019). Bacterial metabolism and antibiotic efficacy. Cell Metab. 30, 251–259. doi: 10.1016/j.cmet.2019.06.009
Thai, V. C., Lim, T. K., Le, K. P. U., Lin, Q., and Nguyen, T. T. H. (2017). iTRAQ-based proteome analysis of fluoroquinolone-resistant Staphylococcus aureus. J. Glob. Antimicrob. Resist. 8, 82–89. doi: 10.1016/j.jgar.2016.11.003
Tse-Dinh, Y. C. (2000). Increased sensitivity to oxidative challenges associated with top A deletion in Escherichia coli. J. Bacteriol. 182, 829–832. doi: 10.1128/JB.182.3.829-832.2000
Usongo, V., and Drolet, M. (2014). Roles of type 1A topoisomerases in genome maintenance in Escherichia coli. PLoS Genet. 10:e1004543. doi: 10.1371/journal.pgen.1004543
Weinstein-Fischer, D., Elgrably-Weiss, M., and Altuvia, S. (2000). Escherichia coli response to hydrogen peroxide: a role for DNA supercoiling, topoisomerase I and Fis. Mol. Microbiol. 35, 1413–1420. doi: 10.1046/j.1365-2958.2000.01805.x
Yang, J., Annamalai, T., Cheng, B., Banda, S., Tyagi, R., and Tse-Dinh, Y. C. (2015). Antimicrobial susceptibility and SOS-dependent increase in mutation frequency are impacted by Escherichia coli topoisomerase I C-terminal point mutation. Antimicrob. Agents Chemother. 59, 6195–6202. doi: 10.1128/AAC.00855-15
Keywords: RNA-Seq, SWATH-LC MS/MS, protein–protein interactions, multidrug resistance, drug target
Citation: Aswal M, Singh N, Singhal N and Kumar M (2025) An integrated proteo-transcriptomics approach reveals novel drug targets against multidrug resistant Escherichia coli. Front. Microbiol. 16:1531739. doi: 10.3389/fmicb.2025.1531739
Received: 20 November 2024; Accepted: 22 January 2025;
Published: 25 February 2025.
Edited by:
Alina Maria Holban, University of Bucharest, RomaniaReviewed by:
Claude Eliane Saint-Ruf, Université Paris Cité, FranceCopyright © 2025 Aswal, Singh, Singhal and Kumar. This is an open-access article distributed under the terms of the Creative Commons Attribution License (CC BY). The use, distribution or reproduction in other forums is permitted, provided the original author(s) and the copyright owner(s) are credited and that the original publication in this journal is cited, in accordance with accepted academic practice. No use, distribution or reproduction is permitted which does not comply with these terms.
*Correspondence: Neelja Singhal, bmVlbGphQHNvdXRoLmR1LmFjLmlu; Manish Kumar, bWFuaXNoQHNvdXRoLmR1LmFjLmlu
Disclaimer: All claims expressed in this article are solely those of the authors and do not necessarily represent those of their affiliated organizations, or those of the publisher, the editors and the reviewers. Any product that may be evaluated in this article or claim that may be made by its manufacturer is not guaranteed or endorsed by the publisher.
Research integrity at Frontiers
Learn more about the work of our research integrity team to safeguard the quality of each article we publish.