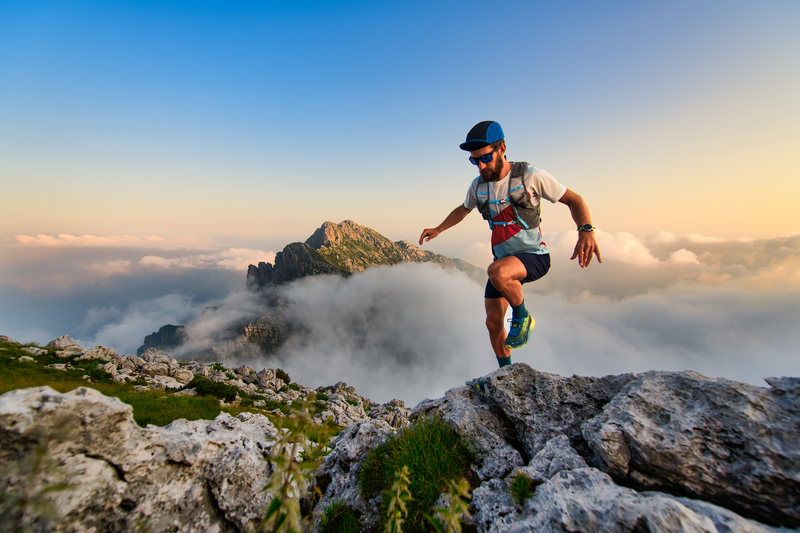
95% of researchers rate our articles as excellent or good
Learn more about the work of our research integrity team to safeguard the quality of each article we publish.
Find out more
ORIGINAL RESEARCH article
Front. Microbiol. , 26 February 2025
Sec. Antimicrobials, Resistance and Chemotherapy
Volume 16 - 2025 | https://doi.org/10.3389/fmicb.2025.1527546
This article is part of the Research Topic Global Dissemination and Evolution of Epidemic Multidrug-Resistant Gram-Negative Bacterial Pathogens: Surveillance, Diagnosis and Treatment, Volume III View all 14 articles
Introduction: Dairy farming plays a vital role in agriculture and nutrition; however, the emergence of antimicrobial resistance (AMR) among bacterial pathogens poses significant risks to public health and animal welfare. Multidrug-resistant (MDR) Escherichia coli strains are of particular concern due to their potential for zoonotic transmission and resistance to multiple antibiotics. In this study, we investigated the prevalence of AMR and analyzed the genomes of two MDR E. coli isolated from dairy cows in Shihezi City.
Methods: Fecal samples were collected from dairy cows, and E. coli strains were isolated. Antibiotic susceptibility testing was conducted using the Kirby-Bauer disk diffusion method against 14 antibiotics. Two MDR isolates (E.coli_30 and E.coli_45) were selected for whole-genome sequencing and comparative genomic analysis. The Comprehensive Antibiotic Resistance Database (CARD) was used to identify AMR genes, and virulence factors were analyzed. Phylogenetic analysis was performed to determine the evolutionary relationships of the isolates, and a pangenome analysis of 50 E. coli strains was conducted to assess genetic diversity. The presence of mobile genetic elements (MGEs), including insertion sequences (IS) and transposons, was also examined.
Results: Among the E. coli isolates, 22.9% exhibited MDR, with high resistance to imipenem and ciprofloxacin, while gentamicin and tetracycline remained the most effective antibiotics. Genomic analysis revealed key AMR genes, including mphA, qnrS1, and blaCTX-M-55 (the latter found only in E.coli_45), conferring resistance to macrolides, quinolones, and beta-lactams, respectively. Virulence genes encoding type III secretion systems (TTSS) and adhesion factors were identified, indicating pathogenic potential. Phylogenetic analysis showed that E.coli_30 and E.coli_45 originated from distinct ancestral lineages. The presence of two extended-spectrum β-lactamase (ESBL) genes in E.coli_45 was noticeable, so we studied their global and national distribution using evolutionary analysis. We found that they are endemic in E. coli, Salmonella enterica, and Klebsiella pneumoniae. Pangenome analysis revealed significant genetic diversity among E. coli strains, with unique genes related to metabolism and stress response. This indicates the bacteria’s adaptation to various environments. MGEs were identified as key contributors to genetic variability and adaptation.
Discussion: This study highlights the growing threat of MDR E. coli in dairy farms, emphasizing the critical role of MGEs in the spread of resistance genes. The genetic diversity observed suggests strong adaptive capabilities, justifying the need for continuous AMR surveillance in livestock. Effective monitoring and mitigation strategies are essential to prevent the dissemination of MDR bacteria, thereby protecting both animal and public health.
Dairy cows provide indispensable benefits to society, as they contribute to several sectors such as agriculture, nutrition, social aspect of, the economy, and the environment. Economically, dairy cows are essential because they are the largest contributors to the dairy industry as major milk producers, thus generating employment to people and supporting trade (Cabrera et al., 2008). They contribute to our nutrient intake as their milk and dairy products are rich in proteins, calcium, and vitamins (Islam et al., 2019). In agriculture, they contribute to sustainable farming through manure production and consumption of residual crops (Rotz et al., 2011). Dairy farming supports rural communities around the globe and it holds cultural significance for many people (Neethirajan, 2023). The industry is also vital to our environment, as a well-managed dairy farm can help mitigate climate change (Rotz et al., 2020).
However, alongside the benefits of dairy farming, there are several health risks posed by zoonotic pathogens. E. coli was first discovered in 1885 by Theodor Escherich and is the most extensively studied bacterium in the field of microbiology (Pakbin et al., 2021). This bacterium is a common inhabitant of the gastrointestinal tract of humans and animals, and it has been increasingly identified as a carrier of AMR genes (Yasir et al., 2020). AMR genes allow microorganisms to withstand the effects of antibiotic that once killed them or inhibit their growth. They are a significant concern in public health, veterinary medicine, and agriculture (Abdelfattah et al., 2021). A specific concern is MDR, where bacteria develop resistance to multiple antibiotic classes, and this causes difficulty in treatment.
One of the pressing issues in dairy facilities today is the interplay between drug use and drug resistance (Romeo et al., 2023; Murray et al., 2022). Bacteria that develop resistance to antibiotics have led to the inability to treat some infections. In 2019, the World Health Organization reported nearly a million deaths due to AMR, with projections suggesting that this number could rise to 20 million and incur costs that would exceed a trillion dollars if the current trends persist (Uddin et al., 2021; Watkins and Bonomo, 2016).
In addition to AMR, some E. coli pathotypes are known to be pathogenic (Liu et al., 2021) and they are capable of causing infections such as diarrhea in both humans and farm animals (Ntuli et al., 2016). Pakbin et al. (2021) discussed the virulence genes of some E. coli strains that are the leading causes of several stomach and urinary tract diseases. They also discussed how those strains cause infections by destabilizing the functions of their host cells through their virulence factors (Pakbin et al., 2021).
To address the aforementioned problems and curb AMR spread in both animals and humans, it is vital to study and monitor the emergence of AMR in our societies. In this study, we explored the phenotypic patterns of antibiotic resistance in E. coli strains isolated from the intestinal tracts of dairy cows. Additionally, we conducted a detailed genomic analysis of two MDR E. coli strains and investigated AMR genes and virulence factors, including their mechanisms. We also examined E. coli diversity using a pangenome analysis and explored the role of MGEs in the spread of AMR genes and E. coli diversity.
A cross-sectional study was conducted in Shihezi City located in the Xinjiang Autonomous Region of China. The study focused on two primary locations: the Shihezi University dairy farm that specializes in breeding dairy cows using advanced technologies and a privately owned dairy farm on the outskirts of the city. Shihezi features a semi-arid climate with extreme seasonal temperatures and fertile plains, and it relies on river irrigation that provides favorable conditions for dairy farming (Yang et al., 2021). The study population consisted exclusively of dairy cows, chosen for the isolation of E. coli from their intestinal tracts to investigate the prevalence and characteristics of the bacteria. Fresh fecal samples were collected immediately after excretion from the middle of the fecal matter to prevent environmental contamination. Samples were placed in 50-ml centrifuge tubes, chilled on ice for preservation, and transported to the laboratory for immediate processing (Lupindu, 2017).
Escherichia coli was isolated from fecal matter using a modified protocol based on the guidelines of Alcock (Ju and Willing, 2018). The isolation was performed via culture techniques on MacConkey, Eosin Methylene Blue, and Luria Bertani agar using the streaking method. Pure isolates were obtained using subculturing that was repeated three times. Colony PCR was then employed for the molecular identification of the 16S rRNA gene using universal primers 27F and 1492R to amplify all nine variable regions (Lane et al., 1985). The PCR was conducted in a 25-μl volume under specific thermal cycling conditions that included an initial denaturation at 95°C followed by 35 cycles of denaturation, annealing, and extension. Gel electrophoresis was performed to confirm the amplification and integrity of the 16S rRNA gene. After validation, 16S rRNA gene sequencing was performed. Raw sequence data were processed using BioEdit (Hall, 1999) and Trimmomatic (Bolger et al., 2014) to remove low-quality reads. This was followed by sequence alignment and analysis in MEGA11 (Tamura et al., 2021) to identify single nucleotide polymorphisms (SNPs), conserved sites, and variable regions, facilitating the identification of the target bacteria.
Based on published data regarding drugs to which E. coli is known to be susceptible (Matuschek et al., 2014; Hombach et al., 2013), we selected 14 different antibiotics for testing. Antimicrobial susceptibility testing was conducted using the Kirby-Bauer disk diffusion method, which involves placing a 6-mm filter paper disk impregnated with a specific amount of antibiotic on a culture plate pre-inoculated with a bacterial suspension (Hudzicki, 2009). Bacterial strains were initially prepared in suspensions, and the optical density was checked at 625 nm to achieve a 0.5 McFarland standard (Benkova et al., 2020). Absorbance values within the range of 0.08–0.13 were considered acceptable according to Hudzicki’s guidelines (Hudzicki, 2009).
After incubation, the diameters of the zones of inhibition around each antimicrobial disk were measured using a ruler and recorded in millimeters. These measurements were used to classify each isolate as susceptible, intermediate, or resistant to the antibiotics tested (Matuschek et al., 2014; Hombach et al., 2013).
Python scripts were used to analyze the data generated from the antimicrobial tests. The Python libraries utilized in this research included Pandas for data manipulation (McKinney, 2010); NumPy for numerical computation and array operations (Harris et al., 2020); Matplotlib for creating static, interactive, and animated visualizations (Hunter, 2007); and Seaborn for its high-level interface for drawing good and informative statistical graphics (Waskom, 2021).
Two multidrug-resistant isolates, designated as E.coli_30 and E.coli_45, were selected for genome sequencing. These two strains were chosen based on their distinctive resistance patterns observed during the susceptibility testing. E.coli_30 demonstrated resistance to all tested antibiotics except aztreonam, while E.coli_45 was resistant to all antibiotics except amikacin. This added layer of resistance compared with the other MDR isolates highlights their unique resistance profiles. This made them ideal candidates for whole-genome sequencing (WGS) to further investigate the genetic mechanisms underlying their extreme drug resistance.
DNA samples were prepared for sequencing by generating indexed libraries for each isolate. The genomic DNA of the two MDR E. coli was fragmented using sonication to an average size of 350 base pairs (bp). The fragmented DNA underwent a series of modifications, including end repair, A-tailing, and ligation with Illumina full-length adapters. This was followed by PCR amplification and purification of the resulting products. The library quality was assessed using qPCR to ensure integrity and concentration. Libraries that met the quality standards were pooled according to their effective concentrations and the desired data output. Sequencing was then performed on an Illumina platform using a paired-end 150 bp (PE150) strategy (Caporaso et al., 2012).
Quality control of raw sequencing data was conducted using FastQC v0.12.1 (Andrews, 2010) followed by de novo genome assembly using SPAdes genome assembler v3.15.5 (Bankevich et al., 2012). To detect and assemble plasmid DNA, PlasmidSpades pipeline was used, and plasmidFinder-2.1.6 (Carattoli et al., 2014) was employed to confirm the presence of known plasmid replicon sequences in the genomes. The assembled genomes were further evaluated using QUAST v5.2.0 (Gurevich et al., 2013), to ensure the selection of high-quality sequences for downstream analysis. Gene annotations were performed using Prokka v1.14.6 (Seemann, 2014) to identify coding sequences and gene structures. A graphical map of the two genomes and the plasmid was generated using nucleotide sequences of the annotated contigs (fna file) in fasta format. This was achieved using one of the CARD web tools at https://proksee.ca/tools/card (Alcock et al., 2023).
To identify and characterize antimicrobial resistance genes and associated resistance mechanisms, we utilized the Resistance Gene Identifier (RGI) tool v6.0.3 (Alcock et al., 2023; Jia et al., 2016), which analyzes the sequences against the CARD database (Alcock et al., 2020). To effectively visualize and interpret the data, we generated RGI wheels by uploading the RGI results with json extension (JSON file) to the CARD website (Jia et al., 2016).1 To identify and characterize the virulence genes, we used Abricate 1.0.1 (Seemann, 2024a), which analyzed the sequences against the Virulence Factor Database (VFDB) (Chen et al., 2016).
Evolutionary and pangenome analyses were performed to examine E. coli diversity. Several E. coli genomes were downloaded from the National Center for Biotechnology Information (NCBI) database, and 17 unpublished genomes were obtained from a colleague for multilocus sequence typing (MLST). The MLST analysis was performed using mlst 2.23.0 (Seemann, 2024b) to identify the sequence types (STs) of all the genomes intended for the phylogenetic studies and pangenome analysis. The objective was to select genomes with different STs to explore the diversity between our two isolates and other E. coli strains. The typing scheme used was the Achtman scheme, version 4 (ecoli_achtman_4), which is one of the standard MLST schemes for E. coli. All genomes selected from the MLST for evolutionary studies were annotated using prokka to generate general feature format files that are suitable for pangenome studies.
Pangenome analysis was conducted using rapid large-scale prokaryote pangenome analysis (roary 3.13.0) (Page et al., 2015). The analysis generate core genome alignments (Katoh and Standley, 2013), which were used to infer genome phylogeny based on conserved genes across all genomes selected through MLST analysis. An evolutionary tree was constructed using FastTree 2.1.11 (Price et al., 2010) that efficiently handled the large dataset and produced the phylogenetic tree. Additionally, pangenome visualizations, including pie chart, histogram, and matrix, were generated using a Python script developed by roary (Page et al., 2015).
A phylogenetic analysis was conducted for the plasmid identified in E.coli_30. The analysis was based on nucleotide similarity, as a BLASTN 2.16.0+ (Zhang et al., 2000) search was performed to identify plasmid DNA similar to our plasmid (Plasmid30). The top 50 plasmid genomes from the blast search were selected, and phylogeny was inferred using FastTree based on their core genomes. All phylogenetic trees from this study were visualized, analyzed, and interpreted using the Interactive Tree of Life: iTOL: Upload a new tree (embl.de) (Letunic and Bork, 2021).
Phylogenetic analysis was conducted for the ESBL genes, blaTEM-1 and blaCTX-M-55, identified in E.coli_45 genome. One phylogenetic tree was inferred using the protein sequences of these genes via an NCBI Blastp search, while another phylogeny for the two genes focused specifically on the endemic strains from China. E. coli genomes submitted to NCBI in the past 5 years were downloaded, and the two ESBL genes were extracted from these genomes. Multiple sequence alignment was performed using MUSCLE (Edgar, 2004), and phylogeny was inferred using IQ-TREE multicore version 2.3.0 (Minh et al., 2020; Nguyen et al., 2015). The analysis included ModelFinder selection (Kalyaanamoorthy et al., 2017), tree reconstruction, and ultrafast bootstrap with 1,000 replicates (Hoang et al., 2018). Phylogenetic relationships were inferred using Maximum Likelihood (ML), with bootstrap replication set at 1000. Evolutionary distances were estimated using ML and presented in units of base substitutions per site (scale 0.01). Default settings were used for all other parameters.
In addition to the plasmid identified in one of the genomes, other mobile genetic elements, such as IS, prophage regions, and more, were investigated using MGEfinder (Durrant et al., 2020), MobileOG-db (Brown et al., 2022), Virsorter (Roux et al., 2015), Alien Hunter (Vernikos and Parkhill, 2006), PhiGARo (Starikova et al., 2020), and ISfinder (Siguier et al., 2006). This investigation was essential, as many AMR and virulence genes are disseminated via mobile elements. Their presence and roles helped to explain the significant genetic diversity observed between our isolates.
The susceptibility test revealed that 51.1% of the isolates were susceptible to the tested antibiotics, 26% showed an intermediate response, and 22.9% were resistant (Figure 1A). By direct count, 26 out of the 53 isolates tested were multidrug-resistant. The highest level of resistance was observed against imipenem (IMP) (60%). In contrast, amikacin (AMK), ofloxacin (OFX), levofloxacin (LEV), ceftriaxone (CTR), and ciprofloxacin (CIP) demonstrated a more balanced distribution across susceptibility, intermediate, and resistance categories. Trimethoprim/sulfamethoxazole (SXT), ceftazidime (CAZ), chloramphenicol (C), aztreonam (ATM), streptomycin (S), gentamicin (GM), ampicillin (AMP), and tetracycline (TET) were the most effective drugs, with GM and TET showing the highest efficacy against over 65% of the E. coli isolates (Figures 1B,C).
Figure 1. Phenotypic analysis of antibiotic resistance in E. coli isolates. AMK, amikacin; OFX, ofloxacin; SXT, trimethoprim/sulfamethoxazole; LEV, levofloxacin;IPM, imipenem; CTR, ceftriazone; CAZ, ceftazidime; CIP, ciprofloxacin; C, chloramphenicol; ATM, aztreonam; S, streptomycin; GM, gentamicin; AMP, ampicillin; TET, tetracycline. (A) Proportion of isolates’ response across all antibiotics. (B) Antibiotics response profile for tested isolates. (C) Violin plot showing the distribution and density of resistance level across 14 antibiotics for all tested E. coli isolates. (D) Correlation matrix of antibiotics based on resistance phenotype. Values closer to 1 on the heatmap indicate strong positive correlation, while values closer to 0 suggest little to no correlation. (E) Hierarchical cluster analysis of E. coli isolates based on their median response to the antibiotics tested. The green, yellow, and red clusters represent resistant, intermediate, and susceptible isolates, respectively.
Furthermore, we calculated the correlation coefficients for all the antibiotics based on the isolates’ responses to assess potential relationships between them. Strong positive correlations were observed in the resistance profiles between certain antibiotics, such as STX and AMP, C and AMP, and TET and STX, with correlation coefficients of 0.85, 0.84, and 0.83, respectively (Figure 1D). These strong positive correlations suggest that isolates resistant to one antibiotic are often resistant to the other, possibly due to similar resistance mechanisms, such as those found within the same antibiotic class. Conversely, some antibiotics, such as GM and LEV, ATM and TET, and CAZ and TET, showed little to no correlation, indicating distinct mechanisms of action (MOA) or resistance. In some cases, we also observed negative correlations, suggesting that the isolates exhibited different MOA.
Finally, we clustered the isolates based on their median score/response to antibiotics and identified distinct response patterns (Figure 1E). Nineteen strains were classified as susceptible, 18 as intermediate, and 16 as resistant. A key observation was that isolates in the first cluster (resistant strains) exhibited resistance to multiple antibiotics. This result indicates that these strains may require special attention to manage the spread of resistance effectively. Additionally, some isolates classified as MDR negative or positive appeared to have intermediate responses due to their median scores. Finally, we also observed that some MDR isolates appeared in the susceptible category. This was because, despite being MDR, they were susceptible to most of the antibiotics tested and this resulted in a susceptible median score.
E.coli_30 and E.coli_45 were selected for genome sequencing after susceptibility testing, as they demonstrated resistance to most of the antibiotics tested, in contrast to the other isolates. After sequencing, de novo assembly of E.coli_30 (Figure 2A) revealed the number of scaffolds (75) was slightly lower than the number of contigs (84). The N50 value was significantly higher for scaffolds (344,767 bp vs. 246,478 bp), indicating better assembly continuity. The total lengths of contigs and scaffolds are similar, at approximately 5.12 million bp, with an identical GC content of 50.92%. However, the scaffolding process introduced gaps, as indicated by an increase in ‘N’s per 100 kbp (17.8 in scaffolds compared to 0.02 in contigs). Annotation of this genome revealed 4,767 genes, including 4,663 coding sequences (CDS), eight rRNA genes, 95 tRNA genes, three repeat regions, and one tmRNA.
Figure 2. (A) Genome map of E.coli_30. (B) Genome map of E.coli_45. (C) Circular map of plasmid30 (plasmid found in E.coli_30).
For the plasmid identified in E.coli_30 (Plasmid30), the total length of the assembly was approximately 218,143 bp, with the largest contig measuring 58,599 bp. The N50 value was 23,145 bp, while the N90 value was 3,131 bp for contigs and 3,150 bp for scaffolds. The GC content remained consistent at 50.58%, and no mismatches were found in the contigs. In contrast, the scaffolds exhibited 47.05 ‘N’s per 100 kbp and a total of 100 ‘N’s, indicating gaps introduced during the scaffolding process. In general, we can say that the scaffolding process slightly reduced the number of contigs, maintained the assembly’s length and continuity, and introduced a few gaps. Figure 2B shows the map of this plasmid, and it highlights various genes and elements that contribute to its functionality and antibiotic resistance.
The second genome, depicted in Figure 2C, had a total assembly length of approximately 4.99 million bp, with a GC content of 50.57%. The number of scaffolds (176) was slightly lower than the number of contigs (185), and the N50 value was higher for scaffolds (151,645 bp vs. 150,606 bp), indicating better assembly continuity. However, the scaffolding process introduced gaps, as reflected by the significant increase in ‘N’s per 100 kbp (15.83 in scaffolds compared to 0.02 in contigs). Overall, the scaffolding effectively linked contigs into longer sequences and enhanced the assembly’s utility for downstream analyses despite the expected introduction of gaps. Annotation of the scaffolds identified 5,076 genes (several were hypothetical proteins), including 4,953 CDS, eight rRNA genes, 114 tRNA genes, four repeat regions, and one tmRNA.
PlasmidFinder-2.1.6 (Carattoli et al., 2014) was used to confirm the presence of known plasmid replicon sequences within the plasmid genome identified in E.coli_30. Four plasmid replicon sequences were detected: Col440I with 94.74% identity over 114 bp in the 14th locus, IncFIB (AP001918) with 98.39% identity over 682 bp in the 7th contig, IncFII (pCoo) with 96.56% identity over 262 bp in the first contig (contig node 1), and p0111 with 98.64% identity over 885 bp in contig node 5. The presence of these plasmid replicon sequences confirmed that E.coli_30 harbors plasmid within its genome. No hits were detected for plasmid types Inc18, NT_Rep, Rep1, Rep2, Rep3, RepA_N, RepL, and Rep_trans, indicating the absence of these plasmid types in the isolate. A nucleotide BLAST search was conducted to identify the origins of these replicons and the result revealed that all the sequences were from E. coli, except for Col440I, which originated from Klebsiella pneumoniae.
RGI CARD investigations of the two genomes revealed the presence of multiple AMR genes (Figure 3), each contributing to antibiotic resistance through various mechanisms. Both E. coli genomes exhibited MDR, but the plasmid in E.coli_30 (Plasmid30) adds a layer of complexity to its resistance mechanisms. While both genomes rely heavily on efflux pumps as a primary resistance mechanism, E.coli_30 was found to have additional resistance factors from its plasmid.
Figure 3. Identification of AMR genes. (A) RGI wheel of AMR genes identified in E.coli_30. (B) RGI wheel of AMR genes in E.coli_45. (C) RGI wheel of AMR genes in Plasmid30. (D) Partial sequence alignment of E.coli_30 GlpT protein showing E448K mutation. (E) Sequence alignment showing amino acid SNP in the floR gene of plasmid30.
Both genomes contained resistance-nodulation-cell division (RND) efflux pump systems (acrA, acrB, AcrF, mdtA, mdtB, and mdtC) that confer resistance to multiple classes of antibiotics, including fluoroquinolones, cephalosporins, tetracyclines, and macrolides. The major facilitator superfamily (MFS) pumps (e.g., emrK, emrY, mdfA, and mdtM) in both genomes offer resistance to tetracyclines, phenicols, and other antibiotics. The genomes also include ATP-binding cassette (ABC) transporters like msbA and TolC that contribute to resistance to nitroimidazoles and peptide antibiotics. Plasmid30 significantly contributes to E.coli_30 resistance, particularly to macrolides, phenicols, and fluoroquinolones (Figure 3C). It carries macrolide phosphotransferase resistance gene (mphA) that is not found in E.coli_45 and is involved in antibiotic inactivation.
Although both genomes had MDR profiles, E.coli_45 displayed a broader range of efflux pump systems and additional resistance mechanisms (Table 1), such as protein overexpression and regulatory mutations (e.g., soxR, soxS, and marA). These mutations enhance antibiotic efflux and reduce β-lactam permeability. Moreover, E.coli_45 includes more entries related to aminoglycoside resistance through inactivation mechanisms (e.g., APH(3′)-IIa, and APH(3″)-Ib). Supplementary Tables S1, S2 summarize the detailed RGI findings for both genomes.
Abricate analysis of the two genomes revealed not only similarities in their pathogenic mechanisms; but also some differences in specific gene clusters (Supplementary Tables S3, S4). Both E.coli_30 and E.coli_45 rely on several core virulence factors, particularly in adhesion, nutrient acquisition, and secretion systems. For instance, both strains possess genes involved in type 1 fimbriae (fimA, fimB, and fimC), E. coli common pilus (ykgK/ecpR and yagZ/ecpA), and colonization factor antigens. Both strains also possess the curli fiber system (csgA, csgB, and csgC) that is important for biofilm formation. They share similar enterobactin siderophore systems for iron acquisition, with genes such as entA, entB, entC, fepB, fepC, fepD, fepE, and fepG. However, the salmochelin siderophore system was found only in E.coli_30 and its plasmid, giving it an advantage in nutrient acquisition over E.coli_45.
Type III secretion systems (TTSS) were detected in both genomes, with genes such as espX1, espX4, and espX5 encoding effector proteins that manipulate host cell signaling and immune defenses. However, E.coli_45 showed a slightly broader range of TTSS-related genes, such as espL1 and espR1, indicating a wider repertoire of effector proteins. Furthermore, we detected a type VI secretion system (T6SS) in E.coli_45, with genes such as tssA and hcp1/tssD1 that were absent in E.coli_30. T6SS gives E.coli_45 an advantage in bacterial competition and host interaction by enabling it to deliver toxic effectors to other bacteria or host cells.
Both strains relied on the enterobactin siderophore system for iron/nutrient acquisition. However, E.coli_45 was found to have additional enterobactin genes, such as entS, fepD, and fepE, making its siderophore system potentially more efficient than that of E.coli_30. We also identified an unknown protein related to the TraJ family that is encoded by AAA92657 in E.coli_45 and is involved in the regulation of invasin.
E.coli_30 was classified as ST3579 with alleles adk-6, fumC-29, gyrB-14, icd-16, mdh-24, purA-13, and recA-2, while E.coli_45 fell under ST1121 with a different allele combination. Some of the strains selected from NCBI—GCA_001971765.1, GCA_000010385.1, and GCA_904799825.1—were identified as ST1011, ST156, and ST23 respectively, each with distinct allele profiles. Additionally, other strains isolated from our laboratory were screened using MLST, and three distinct STs were identified and selected for comparative analysis: E.coli_zq12 (ST10), E.coli_zq44 (ST95), and E.coli_zq56 (ST2522). By including different STs, we could capture the genetic diversity among the sampled E. coli strains, providing a robust basis for evolutionary and pangenome analysis. Supplementary Table S5 presents the STs of all the E. coli strains selected for the evolutionary study.
Evolutionary relationship among the E. coli genomes was inferred using sequence alignment of all 2,755 conserved genes, generated with mafft (Katoh and Standley, 2013). The phylogenetic tree (Figure 4) has Shimodaira-Hasegawa (SH) support values ranging between 0.9 and 1.0, with most clades having SH support values of 1. This indicates that our clade groupings were robust and well supported by the data. The branch lengths in the evolutionary tree represent the level of similarity or divergence; long branches suggest genetic divergence, while short branches indicate similarity or a low level of genetic diversity. The tree scale is 0.01, representing the amount of nucleotide substitution per site.
Figure 4. Whole genome phylogeny. (A) The evolutionary tree was inferred using core genomic data. Our isolates are labeled in red, those obtained from our laboratory are labeled in blue, and isolates from NCBI are labeled in black. The red clades consists of isolates with similar STs, while the green clade contains isolates with more than one ST. (B) Plasmid30 phylogenetic tree was constructed based on nucleotide BLAST similarity. All tips labeled with numbers represent E. coli isolates. The red clade includes E. coli plasmids that are most closely related to Plasmid30.
The two genomes from this study, E.coli_30 (ST3579) and E.coli_45 (ST1121), are positioned in different clades of the phylogenetic tree, indicating that they are not closely related and have distinct evolutionary lineages. The significant genetic divergence observed between them suggests that these isolates have undergone different evolutionary pressures or might have originated from different ancestral groups.
We also observed that isolates with the same STs tend to clade together, with near-zero branch lengths in such cases, suggesting minimal genetic diversity. However, some strains with the same ST showed variation and clustered with different STs. This outcome was expected, as MLST only considers the allele combinations of six housekeeping genes, while our phylogeny was based on the concatenation of over 2,700 genes. Genetic variation can occur outside the six alleles analyzed using MLST, and this explains the observed discrepancies.
For the plasmid DNA, the top 50 plasmid DNA sequences were selected using BLASTN 2.16.0+ (Zhang et al., 2000) to infer relatedness based on similarity. The clade highlighted in red (Figure 4B) consist of E. coli plasmids that share the closest similarity to Plasmid30. A key observation here is that plasmids from different species appeared in the top 50 BLAST hits, suggesting a high degree of similarity with Plasmid30.
Roary pangenome analysis revealed the distribution of genes among the 50 different E. coli strains we analyzed. This analysis uncovered significant divergence in gene presence across the isolates, reflecting a dynamic genome structure. We observed a reduction in the core genome size, accompanied by an expansion of the pangenome. This indicates increasing genetic diversity and the presence of strain-specific genes within the different E. coli STs studied.
We identified 19,495 genes in fewer than seven strains (cloud genes), 3,034 genes present in seven to fewer than 47 strains (shell genes), 297 genes in 47 to fewer than 49 strains (soft-core genes), and 2,755 essential genes (core or housekeeping genes) in 49 to 50 strains (Figures 5A,C). Many cloud genes encode hypothetical proteins with no known function. However, among the known genes, we identified blaTEM-1, blaCTX-M-55, IS110 family transposase (IS621 and ISEc20), tyrosine recombinase (xerC), prophage tail fiber assembly protein (tfaE), Tn3 family transposase (ISEc63), IS200/IS605 family transposase (ISEc46), ISL3 family transposase (ISPst2), prophage integrase (intS), IS21 family transposase (IS100kyp), among others.
Figure 5. Pangenome analysis. (A) Pangenome pie chart showing the distribution of genes among the 50 E. coli strains analyzed. (B) Pangenome histogram showing the distribution of genes across 50 E. coli genomes. (C) Core genome phylogenetic (left) and roary matrix (right), showing the presence or absence of 25,581 gene clusters across all the 50 strains we examined.
We noted that most of the cloud genes were MGEs, and several were unique to our isolates. Some unique genes in E.coli_30 include Tn3 family transposase (TnAs1), putative DNA ligase, e14 prophage; site-specific DNA recombinase. E.coli_45 also harbors unique genes such as fertility inhibition protein, IS4 family transposase (IS4), putative transposase, CP4-44 prophage toxin of the CbtA-CbeA toxin-antitoxin system, and IS110 family transposase (ISSaen1). We identified only one common gene between the two isolates: methyl-accepting chemotaxis protein (mcpQ).
The sparsely distributed clusters in Figure 5B represent the cloud genes from the 50 genomes. The shell gene section contains numerous MGEs, virulence genes (e.g., fimD, adhesin, and fimC), AMR genes (e.g., mdtM, mdtB, and emrEe), and other accessory genes, contributing to E. coli’s genetic diversity and adaptation.
In the soft-core and core gene sections, we found genes essential for E. coli survival, including multidrug export protein (acrE), transcriptional regulatory protein (uhpA), and inner membrane protein (rclC). These core genes are normally known to form a solid block in the pangenome matrix because they were present in all the 50 genomes in the pangenome study.
One major observation from this analysis was that E. coli harbors both virulence and AMR genes in its core genome, and these are essential for survival. Additionally, the accessory genome, enriched with genes acquired from other bacterial species via MGEs, contributes to the bacteria’s evolution and adaptation.
We studied the evolutionary distribution of the two ESBL genes identified in E.coli_45 using NCBI protein BLAST (Figure 6). The top 100 protein sequences with the highest similarity to our isolates were retrieved for comparative analysis. The ML phylogeny of blaTEM provided insights into the similarities and differences between the E.coli_45 blaTEM-1 protein and those from public database. The gene is not highly conserved, as 41 amino acid SNPs were detected, and several variants with long branch lengths were observed. Other members of the blaTEM gene family exist, but none were present in this phylogeny. This study uncovered that blaTEM-1 is distributed across multiple bacterial strains, with Salmonella enterica, Klebsiella pneumoniae, and E. coli being prevalent.
Figure 6. Distribution of blaTEM and blaCTM-M genes based on top 100 NCBI protein BLAST. VSC, variant synthetic construct; DSC, derivative synthetic construct; K. pneomoniae, Klebsiella pneumoniae; S. enterica, Salmonella enterica. (A) Global spread of blaTEM-1 genes inferred using maximum likelihood. (B) Maximum likelihood tree showing the global spread of blaCTX-M gene family.
A similar analysis was conducted for the second ESBL gene (blaCTX-M-55) to examine its distribution using BLASTp. Like blaTEM, this gene is widely distributed across several microbial species, including Salmonella enterica, Klebsiella pneumoniae, and E. coli. Unlike blaTEM, variants of the blaCTX-M gene family were observed in the phylogeny, such as CTX-M-15, CTX-M-57, CTX-M-190, and CTX-M-261. More amino acid SNPs (66) were detected in the blaCTX-M comparative analysis, as more variants appeared among the top BLAST hits.
An additional evolutionary analysis was conducted to investigate the spread of these genes within China. This study focused on E. coli, Salmonella enterica, and Klebsiella pneumoniae, as our previous analysis (Figure 6) indicated that these genes were most prevalent in these three species. We examined the presence of these genes directly from the genomes of hundreds of isolates from China over the past 5 years. For the blaTEM-1 gene (Figure 7A), we detected it 92 times across E. coli, Salmonella enterica, and Klebsiella pneumoniae, with two additional variants, TEM-135 and TEM-176, also identified. Multiple occurrences of the gene within single genomes were observed, suggesting its spread through MGEs. Although other family members were detected, TEM-1 was the most prevalent, and the gene appeared highly conserved across the three bacterial species. We noted the presence of an SNP in the E.coli_45 blaTEM-1 gene and this explains its significant branch distance in the dendrogram. The gene was completely conserved across all Salmonella strains and several Klebsiella and E. coli genomes, including six strains (E.coli_zq6, E.coli_zq12, E.coli_zq40, E.coli_zq25, E.coli_zq19, and E.coli_zq26) isolated in our laboratory. The longest branch length was found in the two blaTEM-135 variants, which clustered at the bottom of the tree, showing the most genetic diversity.
Figure 7. Spread of blaTEM and blaCTM-M genes within China across three bacterial species from 2019 to 2024. (A) Maximum likelihood tree of blaTEM genes. (B) Maximum likelihood tree of blaCTX-M gene family.
We performed a similar analysis to investigate the distribution of blaCTX-M across China (Figure 7B). Comparative analysis between the three species revealed that blaCTX-M-55 was predominantly found in E. coli and Salmonella. Each gene within the blaCTX-M family formed distinct clusters, regardless of the host species. Several variants were detected, including CTX-M-15, CTX-M-65 (prevalent in Klebsiella), CTX-M-14, and CTX-M-199. These genes were found multiple times within a single genome, and this suggests their spread via MGEs. Another key observation was that blaCTX-M-55 clustered near the root of the tree. This suggests that it was the ancestral variant of the other blaCTX-M variants.
Several MGEs were identified in the two MDR E. coli genomes. MGEfinder was used to detect several insertion sequences and their insertion sites in the two genomes. Some insertions were very short sequences, approximately 20 bp, while others were larger, ranging from 1,000 to 3,000 bp. Annotation of the larger regions revealed the presence of IS110 family transposases, IS3, and several passenger genes, including invasin, electron transfer flavoprotein-ubiquinone oxidoreductase, ygcP, and fixB, among others, in E.coli_45. We also found IS110 and IS3 transposases in E.coli_30, with passenger genes including rhm1, algC, and btuD. We also found 20 inserted genes in one larger insertion site in E.coli_30.
Furthermore, the genomes were then annotated using MobileOG-db, which tracks mobile elements by organizing them into orthologous groups. We tracked a wide range of MGEs, including transposons, integrons, IS elements, phage related genes, and integrative conjugative elements, which explains the large number of MGEs found within the two genomes (Figure 8A). Among the identified MGEs, we found genes involved in critical processes such as replication, recombination, repair, integration/excision, and phage-related functions (Figures 8C,D). Notable genes include sdiA, associated with conjugation and relaxosome activity, and ruvA, ruvC, and holE_2, which play key roles in DNA repair, recombination, genome stability, and horizontal gene transfer (HGT). Additionally, phage-related genes such as dicB, essD, and quuQ_2 that contribute to lysis, lysogeny, and infection regulation, were detected. Transposon-associated genes such as tnpA and tnpB facilitate integration and excision, while stability/transfer/defense genes like hipA and cnu help maintain genetic integrity. We observed that some entries for both genomes were manually curated, while others were annotated through automated homology-based methods using several databases.
Figure 8. Mobile genetic elements. (A) Mobile elements hits identified using different tools. (B) Annotation of the contig (locus) carrying ESBL genes in E.coli_45. (C) MGEs hits in E.coli_30 using MobileOG-db. (D) MGE hits in E.coli_45 using MobileOG-db.
The phage genome annotator PhiGARo predicted prophages and other virus-like sequences in conjunction with virus orthologous groups (VOGs). We identified five prophage regions and 138 prophage genes in E.coli_30. In the second genome, we predicted 11 prophage regions and 259 genes, significantly more than those in E.coli_30. The taxonomy of these prophage genes revealed that most belonged to the Myoviridae family, a few were of unknown origin, and one was classified as Podoviridae. The prophage genes identified were associated with roles such as lysis, integration, termination, tail assembly, replication, coat formation, and portal functions. HGT regions and their insertion sites within the two genomes were predicted using Alien Hunter, while viral signals were detected using VirSorter within the two genomic sequences (Figure 8A).
Finally, we further investigated the locus harboring the ESBL genes in E.coli_45 and found that the blaTEM-1 gene was likely inserted into the genome via the Tn3 insertion sequence. Annotation of this locus revealed a Tn3 family transposase (Tn2) and a site-specific DNA recombinase (tnpR) located just a few bases upstream of the ESBL gene (Figure 8B), which likely facilitated its integration. The closest match we found using ISfinder BLAST for this locus showed the presence of a DDE recombinase (IS110 family transposase), Tn3 resolvase, and a passenger gene (blaTEM-1), and this result aligns closely with our findings.
The findings from the antibiotic resistance profiles among the E. coli isolates provided vital insights into the response of each antibiotic against the tested bacterial isolates. In this study, GEN exhibited the most efficacy against the E. coli isolates tested, while IMP and CIP, on the other hand, exhibited high levels of resistance. Shams et al. (2018) documented high levels of carbapenem-resistant E. coli, while Shariati et al. (2022) reported CIP resistance in the context of urinary tract infections and other E. coli-associated diseases.
Interestingly, the susceptibility test showed that our isolates displayed much greater levels of resistance to IMP compared to AMP, a first-generation penicillin. This situation could have occurred due to several reasons. One possibility is that when certain antibiotics begin to show reduced efficacy against bacteria, their increased consumption may result in heightened resistance. This explains why IMP-resistant bacteria are often associated with carbapenem intake, possibly corroborating our findings (Yang et al., 2018).
Another plausible reason is that the IMP-resistance isolates in our study may habor carbapenem-specific β-lactamases such as New Delhi Metallo-β-lactamase (NDM) or Klebsiella pneumoniae Carbapenemase (KPC) without ESBLs or AmpC enzymes, thus remaining susceptibility to AMP. For instance, Mojica et al. (2022) reported that some E. coli strains harboring NDM remain susceptible to some penicillins due to the specific substrate profile of NDM enzymes.
Additionally, IMP resistance could be due to alterations (mutations) in porin channels that limit the entry of IMP without affecting AMP. This phenomenon has been reported in clinical isolates where specific mutations in porin proteins confer carbapenem resistance (Poirel et al., 2020). Furthermore, efflux pumps, such as those of the RND family, which confer MDR, may have substrate specificity that includes IMP but not AMP, potentially contributing to the resistance patterns observed (Tambat et al., 2022).
The identification and characterization of AMR genes in our E. coli isolates revealed several key resistance mechanisms. The presence of pmr phosphoethanolamine transferases (e.g., marA and acrD), efflux pumps (e.g., acrAB-tolC), and β-lactamase indicate that these isolates possess vast mechanisms to evade antibiotic treatment. These findings agree with other studies that have reported the widespread presence of AMR genes in some E. coli strains, posing major problems to public health (Yang et al., 2018; Majumder et al., 2021). Efflux pumps play a crucial role in mediating bacterial resistance by expelling antibiotics from the cells. To combat this, Lu et al. (2024) developed a potential efflux pump inhibitor (EPI) derived from a seaweed compound, which displayed efficacy in reducing AMR in drug-resistant E. coli strains. Another study explored the use of a microbe-derived EPI, ethyl 4-bromopyrrole-2-carboxylate (RP1), which shows promise in combination therapies (Tambat et al., 2022). The findings from that study indicate significant reductions in minimum inhibitory concentrations and extended post-antibiotic effect, making RPI a promising candidate for enhancing existing antibiotic treatments.
We noted the presence of specific β-lactamase genes (blaTEM-1, blaCTX-M-55) in E.coli_45 (Figure 8B), making this isolate an ESBL-producing E. coli (Chen et al., 2019; Papouskova et al., 2020). The presence of ESBL enzyme in E.coli_45 indicates that this strain is resistant to most β-lactam antibiotics, posing a significant public health risk due to limited treatment options (Chen et al., 2019). A recent pharmaceutical strategy to address this issue involves the use of β-lactamase inhibitors. These inhibitors are crucial in restoring the activity of β-lactam antibiotics against bacteria that produce β-lactamase enzymes. Le Terrier et al. (2024) studied taniborbactam, a novel broad-spectrum β-lactamase inhibitor effective against metallo-β-lactamases (MBLs). Their study showed that taniborbactam exhibited significant activity against B1 subclass MBLs, including NDM and VIM (Verona integrin-encoded metallo-β-lactamase) enzymes (Le Terrier et al., 2024). However, certain variants such as NDM-9 and VIM-83 demonstrated resistance, raising concerns regarding the potential emergence of resistant strains (Le Terrier et al., 2024). Taniborbactam’s ability to inhibit a wide range of MBLs, including those resistant to traditional β-lactamase inhibitors, positions it as a critical tool in the fight against MDR pathogens.
In addition to β-lactam resistance, several other AMR genes were detected in both isolates. This underscores the critical need for monitoring and developing novel therapeutic strategies, such as bacteriophage therapy, to combat MDR (Montso et al., 2019). We also identified multiple AMR genes associated with resistance to various classes of antibiotics, including quinolones (qnr genes), sulfonamides (sul genes), and tetracyclines (tet genes). The presence of numerous variants of these genes within the MDR isolates suggests HGT as a major facilitator of AMR (Partridge et al., 2018). This finding aligns with research that highlights the role of plasmids, integrons, and transposable elements, such as IS, in spreading AMR across E. coli and other bacterial species (Zingali et al., 2020; Vinayamohan et al., 2022).
The analysis of virulence-associated genes in the test isolates revealed the presence of genes associated with adhesion (e.g., cfaB and fimH), invasion (e.g., ibeC and ompA), effector delivery systems (TTSS effectors), and metabolic factors or iron acquisition (e.g., siderophores like enterobactin and salmochelin) (Algammal et al., 2020). Notably, salmochelin siderophore virulence genes, such as iroB and iroC, were exclusively found in E.coli_30 and its plasmid. These virulence identifiers (adhesion, invasion, and nutrition) are vital for the pathogenic potential of E. coli, enabling the bacterium to colonize hosts, evade immune responses, and cause disease (Croxen et al., 2013).
Our findings are supported by previous research highlighting the wide range of virulence-associated genes found in pathogenic E. coli isolates, which significantly contribute to the bacteria’s ability to cause different types of infections (Sarowska et al., 2019; Sora et al., 2021). The identification of virulence genes, such as the TTSS effectors (e.g., espX1, espX4, and espL1) and iron uptake systems like salmochelin, in our isolates suggests their potential to cause severe infections (Radics et al., 2014; Magistro et al., 2015). These identified factors, including invasin, adhesin, and metabolic factors, facilitate bacterial invasion, intracellular survival, and nutrient acquisition, respectively, which are essential for causing infections in a host organism (Liu et al., 2022).
The presence of virulence genes in both commensal and pathogenic bacterial strains has been reported and these genes underscore the complex relationship between E. coli and its host, determining the outcome of infections (Dionisio et al., 2023).
The phylogenetic tree generated in this study revealed distinct clades, each representing different evolutionary lineages. The isolates we sequenced, E.coli_30 (ST3579) and E.coli_45 (ST1121), were placed in different clades, highlighting a major genetic divergence and suggesting that these two isolates have been through different evolutionary pressures or they may have come from different ancestral populations. What we observed here agreed with previous studies that reported on the genetic diversity within E. coli strains driven by various evolutionary factors such as mutations, HGT via MGEs, and genetic recombination (Vinayamohan et al., 2022; Touchon et al., 2009). The diverse placement of isolates with different STs in the phylogenetic tree indicate a wide genetic diversity even within smaller groups of E. coli strains. This aligned with the result of a study by Didelot et al. (2012) in which they reported on the diversity of E. coli with dynamic adaptation to many hosts and environmental conditions, leading to major genome plasticity. The 45 isolates from the NCBI database were distributed across various clades in the phylogeny, except for isolates with the same STs (mostly tend to clade together). This highlights the extensive genetic diversity present in the NCBI database for E. coli strains. The varied clustering patterns we observed in the phylogenetic tree suggest that these E. coli isolates were arranged into multiple sub-types with each strain following its unique evolutionary paths (Touchon et al., 2020).
The phylogenetic analyses of blaTEM-1 and blaCTX-M genes in E. coli, Salmonella enterica, and Klebsiella pneumoniae from China showed how widely these resistance genes have spread, thanks to MGEs. The discovery of multiple copies of these genes within individual genomes and across species highlights the important role of MGEs, such as IS and transposons, in moving these genes between bacteria. blaTEM-1 appeared to be highly conserved, meaning it has changed little across species, while blaCTX-M variants, such as CTX-M-55 and CTX-M-15, showed more diversity, forming distinct clusters that reflect different evolutionary paths. This mirrors the findings from other studies that show plasmids helping to spread resistance genes across bacterial species in China (Wu et al., 2015; Kuang et al., 2018). The presence of small mutations, or SNPs, in some of the genes suggests that they are continuing to evolve. Overall, these results emphasize how MGEs drive the spread of antibiotic resistance across bacteria, agreeing with global trends observed in similar studies (Peng et al., 2024; Jin et al., 2015; Awosile and Agbaje, 2021).
The pangenome analysis conducted in this study included both core and accessory genomes to elaborate extensively on the genetic diversity within 50 E. coli strains. The core genomes has conserved genes that are present in all the isolates, shedding knowledge on essential functions and evolutionary conserved mechanisms. The accessory genome on the other hand, consisting genes present in some but not all isolatesprovided insights into the adaptive potential of E. coli to many different environments and antibiotic treatments (Vernikos et al., 2015). The results of the pangenome analysis revealed a high proportion of unique genes in individual isolates, indicating strain-specific E. coli adaptations. Recent studies on E. coli pangenome (gene-specific) support what we reported that new genes continue to be obtained through HGT, helping in the bacteria’s adaptation and vast ecology (Hall et al., 2021). The identification of genes associated with metabolism (iron uptake), stress response, and adhesion, highlights the adaptive nature of the E. coli genome, facilitating its survival in diverse environments (Liu et al., 2022).
Our investigation into MGEs across the two genomes revealed that the results varied significantly depending on the analytical tools used. Each tool offered distinct methodologies for MGE identification and these helped illuminate different aspects of MGE diversity and behavior. MGEfinder emerged as the most reliable tool in this study, providing detailed mapping of insertion sites and accurately identifying associated insertion sequences. This tool successfully recognized IS elements, whether or not they contained inverted terminal repeats, which are essential features for many MGEs. In particular, sequences with terminal repeats, such as those from the IS3 family transposase (IS3) and ISEc17 were detected in the two genomes and across several other genomes when studying the spread of ESBL genes. These elements are widespread in E. coli genomes and often carry critical passenger genes such as blaTEM-1 and blaCTX-M that contribute to antibiotic resistance. This finding aligned with prior studies that emphasized the role of IS elements in disseminating resistance genes (Partridge et al., 2018). Additionally, MGEfinder was able to identify elements that lacked inverted terminal repeats, which are predominantly part of the IS110 family (Durrant et al., 2024). In both the two genomes, we noted the presence of IS621, an essential member of the IS110 recombinase. The integration mechanism for this family involves a recently discovered bridge RNA recombination process (Durrant et al., 2024; Hiraizumi et al., 2024), and this underscores a novel pathway for MGE mobility.
Complementary to MGEfinder, other tools such as MobileOG-db provided MGE characterization by annotating the genomes against a variety of databases. MobileOG-db matched sequences with several key databases, including pVOG, Plasmid RefSeq, GPD, and ICE. This broadened the scope of our analysis and yielded a large number of hits for MGEs in our isolate. In addition, we employed VirSorter, PhiGARo, and Alien Hunter, which further contributed to MGE detection across the genomes by targeting specific MGE types. VirSorter has been widely used in recent virome studies (Roux et al., 2015) to identify viral sequences embedded in bacterial genomes, a feature relevant to our study given the potential role of viruses in HGT. Similarly, PhiGARo and Alien Hunter have previously demonstrated effectiveness in detecting prophages and alien DNA, respectively, complementing each other by expanding the types of MGEs detected (Akhter et al., 2012).
The data presented in the study are deposited in the NCBI repository, BioProject accession number PRJNA1169417.
AB: Conceptualization, Data curation, Formal analysis, Investigation, Methodology, Resources, Software, Validation, Visualization, Writing – original draft, Writing – review & editing. SN: Methodology, Resources, Software, Writing – review & editing. QZ: Investigation, Software, Writing – review & editing. WN: Funding acquisition, Project administration, Supervision, Writing – review & editing. SH: Conceptualization, Funding acquisition, Methodology, Project administration, Resources, Software, Supervision, Validation, Writing – review & editing.
The author(s) declare that financial support was received for the research, authorship, and/or publication of this article. This work was supported financially by the Science and Technology Program of Xinjiang Production and Construction Corps (no. 2024AB050), Bingtuan Science and Technology Project (no. NYHXGG2023AA101), the Tianshan Talent Project [nos. 2022TSYCCX0125 and 2023TSYCJU0010], and the Tianshan Talent innovation team [no. 2023TSYCTD0021].
Thanks to all of the contributors to this work.
The authors declare that the research was conducted in the absence of any commercial or financial relationships that could be construed as a potential conflict of interest.
The authors declare that no Generative AI was used in the creation of this manuscript.
All claims expressed in this article are solely those of the authors and do not necessarily represent those of their affiliated organizations, or those of the publisher, the editors and the reviewers. Any product that may be evaluated in this article, or claim that may be made by its manufacturer, is not guaranteed or endorsed by the publisher.
The Supplementary material for this article can be found online at: https://www.frontiersin.org/articles/10.3389/fmicb.2025.1527546/full#supplementary-material
Abdelfattah, E. M., Ekong, P. S., Okello, E., Chamchoy, T., Karle, B. M., Black, R. A., et al. (2021). Epidemiology of antimicrobial resistance (AMR) on California dairies: descriptive and cluster analyses of AMR phenotype of fecal commensal bacteria isolated from adult cows. PeerJ 9:e11108. doi: 10.7717/peerj.11108
Akhter, S., Aziz, R. K., and Edwards, R. A. (2012). PhiSpy: a novel algorithm for finding prophages in bacterial genomes that combines similarity-and composition-based strategies. Nucleic Acids Res. 40:e126. doi: 10.1093/nar/gks406
Alcock, B. P., Huynh, W., Chalil, R., Smith, K. W., Raphenya, A. R., Wlodarski, M. A., et al. (2023). CARD 2023: expanded curation, support for machine learning, and resistome prediction at the comprehensive antibiotic resistance database. Nucleic Acids Res. 51, D690–D699. doi: 10.1093/nar/gkac920
Alcock, B. P., Raphenya, A. R., Lau, T. T. Y., Tsang, K. K., Bouchard, M., Edalatmand, A., et al. (2020). CARD 2020: antibiotic resistome surveillance with the comprehensive antibiotic resistance database. Nucleic Acids Res. 48, D517–D525. doi: 10.1093/nar/gkz935
Algammal, A. M., Hetta, H. F., Batiha, G. E., Hozzein, W. N., El Kazzaz, W. M., Hashem, H. R., et al. (2020). Virulence-determinants and antibiotic-resistance genes of MDR-E. coli isolated from secondary infections following FMD-outbreak in cattle. Sci. Rep. 10, 1–13. doi: 10.1038/s41598-020-75914-9
Andrews, S. (2010). FastQC: A quality control tool for high throughput sequence data. Cambridge, UK.
Awosile, B. B., and Agbaje, M. (2021). Genetic environments of plasmid-mediated Bla CTXM-15 beta-lactamase gene in Enterobacteriaceae from Africa. Microbiol. Res. 12, 383–394. doi: 10.3390/microbiolres12020026
Bankevich, A., Nurk, S., Antipov, D., Gurevich, A. A., Dvorkin, M., Kulikov, A. S., et al. (2012). SPAdes: a new genome assembly algorithm and its applications to single-cell sequencing. J. Comput. Biol. 19, 455–477. doi: 10.1089/cmb.2012.0021
Benkova, M., Soukup, O., and Marek, J. (2020). Antimicrobial susceptibility testing: currently used methods and devices and the near future in clinical practice. J. Appl. Microbiol. 129, 806–822. doi: 10.1111/jam.14704
Bolger, A. M., Lohse, M., and Usadel, B. (2014). Trimmomatic: a flexible trimmer for Illumina sequence data. Bioinformatics 30, 2114–2120. doi: 10.1093/bioinformatics/btu170
Brown, C. L., Mullet, J., Hindi, F., Stoll, J. E., Gupta, S., Choi, M., et al. (2022). mobileOG-db: a manually curated database of protein families mediating the life cycle of bacterial mobile genetic elements. Appl. Environ. Microbiol. 88, e00991–e00922. doi: 10.1128/aem.00991-22
Cabrera, V., Hagevoort, R., Solís, D., Kirksey, R., and Diemer, J. A. (2008). Economic impact of milk production in the state of New Mexico. J. Dairy Sci. 91, 2144–2150. doi: 10.3168/jds.2007-0791
Caporaso, J. G., Lauber, C. L., Walters, W. A., Berg-Lyons, D., Huntley, J., Fierer, N., et al. (2012). Ultra-high-throughput microbial community analysis on the Illumina HiSeq and MiSeq platforms. ISME J. 6, 1621–1624. doi: 10.1038/ismej.2012.8
Carattoli, A., Zankari, E., García-Fernández, A., Voldby Larsen, M., Lund, O., Villa, L., et al. (2014). In silico detection and typing of plasmids using PlasmidFinder and plasmid multilocus sequence typing. Antimicrob. Agents Chemother. 58, 3895–3903. doi: 10.1128/AAC.02412-14
Chen, Y., Liu, Z., Zhang, Y., Zhang, Z., Lei, L., and Xia, Z. (2019). Increasing prevalence of ESBL-producing multidrug resistance Escherichia coli from diseased pets in Beijing, China from 2012 to 2017. Front. Microbiol. 10:2852. doi: 10.3389/fmicb.2019.02852
Chen, L., Zheng, D., Liu, B., Yang, J., and Jin, Q. (2016). VFDB 2016: hierarchical and refined dataset for big data analysis—10 years on. Nucleic Acids Res. 44, D694–D697. doi: 10.1093/nar/gkv1239
Croxen, M. A., Law, R. J., Scholz, R., Keeney, K. M., Wlodarska, M., and Finlay, B. B. (2013). Recent advances in understanding enteric pathogenic Escherichia coli. Clin. Microbiol. Rev. 26, 822–880. doi: 10.1128/CMR.00022-13
Didelot, X., Bowden, R., Wilson, D. J., Peto, T. E. A., and Crook, D. W. (2012). Transforming clinical microbiology with bacterial genome sequencing. Nat. Rev. Genet. 13, 601–612. doi: 10.1038/nrg3226
Dionisio, F., Domingues, C. P. F., Rebelo, J. S., Monteiro, F., and Nogueira, T. (2023). The impact of non-pathogenic bacteria on the spread of virulence and resistance genes. Int. J. Mol. Sci. 24:1967. doi: 10.3390/ijms24031967
Durrant, M. G., Li, M. M., Siranosian, B. A., Montgomery, S. B., and Bhatt, A. S. (2020). A bioinformatic analysis of integrative mobile genetic elements highlights their role in bacterial adaptation. Cell Host Microbe 27, 140–153.e9. doi: 10.1016/j.chom.2019.10.022
Durrant, M. G., Perry, N. T., Pai, J. J., Jangid, A. R., Athukoralage, J. S., Hiraizumi, M., et al. (2024). Bridge RNAs direct programmable recombination of target and donor DNA. Nature 630, 984–993. doi: 10.1038/s41586-024-07552-4
Edgar, R. C. (2004). MUSCLE: multiple sequence alignment with high accuracy and high throughput. Nucleic Acids Res. 32, 1792–1797. doi: 10.1093/nar/gkh340
Gurevich, A., Saveliev, V., Vyahhi, N., and Tesler, G. (2013). QUAST: quality assessment tool for genome assemblies. Bioinformatics 29, 1072–1075. doi: 10.1093/bioinformatics/btt086
Hall, T. A. (1999). BioEdit: a user-friendly biological sequence alignment editor and analysis program for windows 95/98/NT. In: Nucleic acids symposium series. Oxford.
Hall, R. J., Whelan, F. J., Cummins, E. A., Connor, C., McNally, A., and McInerney, J. O. (2021). Gene-gene relationships in an Escherichia coli accessory genome are linked to function and mobility. Microb. Genom. 7:000650. doi: 10.1099/mgen.0.000650
Harris, C. R., Millman, K. J., van der Walt, S. J., Gommers, R., Virtanen, P., Cournapeau, D., et al. (2020). Array programming with NumPy. Nature 585, 357–362. doi: 10.1038/s41586-020-2649-2
Hiraizumi, M., Perry, N. T., Durrant, M. G., Soma, T., Nagahata, N., Okazaki, S., et al. (2024). Structural mechanism of bridge RNA-guided recombination. Nature 630, 994–1002. doi: 10.1038/s41586-024-07570-2
Hoang, D. T., Chernomor, O., von Haeseler, A., Minh, B. Q., and Vinh, L. S. (2018). UFBoot2: improving the ultrafast bootstrap approximation. Mol. Biol. Evol. 35, 518–522. doi: 10.1093/molbev/msx281
Hombach, M., Zbinden, R., and Böttger, E. C. (2013). Standardisation of disk diffusion results for antibiotic susceptibility testing using the sirscan automated zone reader. BMC Microbiol. 13, 225–228. doi: 10.1186/1471-2180-13-225
Hudzicki, J. (2009). Kirby-Bauer disk diffusion susceptibility test protocol, vol. 15. Washington, DC: American Society for Microbiology, 1–23.
Hunter, J. D. (2007). Matplotlib: a 2D graphics environment. Comput. Sci. Eng. 9, 90–95. doi: 10.1109/MCSE.2007.55
Islam, M., Bari, M. S., Habib, M. R., Sarkar, M. M., and Islam, M. A. (2019). Comparative economic analysis of dairy characteristics focused on repeat breeding losses in selected milk pocket areas of Bangladesh. Bangladesh J. Anim. Sci. 48, 145–154. doi: 10.3329/bjas.v48i2.46769
Jia, B., Raphenya, A. R., Alcock, B., Waglechner, N., Guo, P., Tsang, K. K., et al. (2016). CARD 2017: expansion and model-centric curation of the comprehensive antibiotic resistance database. Nucleic Acids Res. 45, D566–D573. doi: 10.1093/nar/gkw1004
Jin, Y., Shao, C., Li, J., Fan, H., Bai, Y., and Wang, Y. (2015). Outbreak of multidrug resistant NDM-1-producing Klebsiella pneumoniae from a neonatal unit in Shandong Province, China. PLoS One 10:e0119571. doi: 10.1371/journal.pone.0119571
Ju, T., and Willing, B. P. (2018). Isolation of commensal Escherichia coli strains from feces of healthy laboratory mice or rats. Bio Protoc. 8, –e2780. doi: 10.21769/BioProtoc.2780
Kalyaanamoorthy, S., Minh, B. Q., Wong, T. K. F., von Haeseler, A., and Jermiin, L. S. (2017). ModelFinder: fast model selection for accurate phylogenetic estimates. Nat. Methods 14, 587–589. doi: 10.1038/nmeth.4285
Katoh, K., and Standley, D. M. (2013). MAFFT multiple sequence alignment software version 7: improvements in performance and usability. Mol. Biol. Evol. 30, 772–780. doi: 10.1093/molbev/mst010
Kuang, D., Zhang, J., Xu, X., Shi, W., Yang, X., Su, X., et al. (2018). Increase in ceftriaxone resistance and widespread extended-spectrum β-lactamases genes among Salmonella enterica from human and nonhuman sources. Foodborne Pathog. Dis. 15, 770–775. doi: 10.1089/fpd.2018.2468
Lane, D. J., Pace, B., Olsen, G. J., Stahl, D. A., Sogin, M. L., and Pace, N. R. (1985). Rapid determination of 16S ribosomal RNA sequences for phylogenetic analyses. Proc. Natl. Acad. Sci. 82, 6955–6959. doi: 10.1073/pnas.82.20.6955
Le Terrier, C., Viguier, C., Nordmann, P., Vila, A. J., and Poirel, L. (2024). Relative inhibitory activities of the broad-spectrum β-lactamase inhibitor taniborbactam against metallo-β-lactamases. Antimicrob. Agents Chemother. 68, e00991–e00923. doi: 10.1128/aac.00991-23
Letunic, I., and Bork, P. (2021). Interactive tree of life (iTOL) v5: an online tool for phylogenetic tree display and annotation. Nucleic Acids Res. 49, W293–W296. doi: 10.1093/nar/gkab301
Liu, H., Meng, L., Dong, L., Zhang, Y., Wang, J., and Zheng, N. (2021). Prevalence, antimicrobial susceptibility, and molecular characterization of Escherichia coli isolated from raw milk in dairy herds in northern China. Front. Microbiol. 12:730656. doi: 10.3389/fmicb.2021.730656
Liu, B., Zheng, D., Zhou, S., Chen, L., and Yang, J. (2022). VFDB 2022: a general classification scheme for bacterial virulence factors. Nucleic Acids Res. 50, D912–D917. doi: 10.1093/nar/gkab1107
Lu, W.-J., Lian, Y. W., Chang, C. J., Lin, H. J., Huang, C. Y., Hsu, P. H., et al. (2024). Screening and evaluation of potential efflux pump inhibitors with a seaweed compound Diphenylmethane-scaffold against drug-resistant Escherichia coli. Antibiotics 13:628. doi: 10.3390/antibiotics13070628
Lupindu, A. M. (2017). Isolation and characterization of Escherichia coli from animals, humans, and environment. Escherichia coli-recent advances on physiology, pathogenesis and biotechnological applications. London, UK: IntechOpen Limited, 187–206.
Magistro, G., Hoffmann, C., and Schubert, S. (2015). The salmochelin receptor IroN itself, but not salmochelin-mediated iron uptake promotes biofilm formation in extraintestinal pathogenic Escherichia coli (ExPEC). Int. J. Med. Microbiol. 305, 435–445. doi: 10.1016/j.ijmm.2015.03.008
Majumder, S., Jung, D., Ronholm, J., and George, S. (2021). Prevalence and mechanisms of antibiotic resistance in Escherichia coli isolated from mastitic dairy cattle in Canada. BMC Microbiol. 21:222. doi: 10.1186/s12866-021-02280-5
Matuschek, E., Brown, D. F., and Kahlmeter, G. (2014). Development of the EUCAST disk diffusion antimicrobial susceptibility testing method and its implementation in routine microbiology laboratories. Clin. Microbiol. Infect. 20, O255–O266. doi: 10.1111/1469-0691.12373
Minh, B. Q., Schmidt, H. A., Chernomor, O., Schrempf, D., Woodhams, M. D., von Haeseler, A., et al. (2020). IQ-TREE 2: new models and efficient methods for phylogenetic inference in the genomic era. Mol. Biol. Evol. 37, 1530–1534. doi: 10.1093/molbev/msaa015
Mojica, M. F., Rossi, M. A., Vila, A. J., and Bonomo, R. A. (2022). The urgent need for metallo-β-lactamase inhibitors: an unattended global threat. Lancet Infect. Dis. 22, e28–e34. doi: 10.1016/S1473-3099(20)30868-9
Montso, P. K., Mlambo, V., and Ateba, C. N. (2019). Characterization of lytic bacteriophages infecting multidrug-resistant Shiga toxigenic atypical Escherichia coli O177 strains isolated from cattle feces. Front. Public Health 7:355. doi: 10.3389/fpubh.2019.00355
Murray, C. J., Ikuta, K. S., Sharara, F., Swetschinski, L., Aguilar, G. R., Gray, A., et al. (2022). Global burden of bacterial antimicrobial resistance in 2019: a systematic analysis. Lancet 399, 629–655. doi: 10.1016/S0140-6736(21)02724-0
Neethirajan, S. (2023). Innovative strategies for sustainable dairy farming in Canada amidst climate change. Sustain. For. 16:265. doi: 10.3390/su16010265
Nguyen, L.-T., Schmidt, H. A., von Haeseler, A., and Minh, B. Q. (2015). IQ-TREE: a fast and effective stochastic algorithm for estimating maximum-likelihood phylogenies. Mol. Biol. Evol. 32, 268–274. doi: 10.1093/molbev/msu300
Ntuli, V., Njage, P. M. K., and Buys, E. M. (2016). Characterization of Escherichia coli and other Enterobacteriaceae in producer-distributor bulk milk. J. Dairy Sci. 99, 9534–9549. doi: 10.3168/jds.2016-11403
Page, A. J., Cummins, C. A., Hunt, M., Wong, V. K., Reuter, S., Holden, M. T. G., et al. (2015). Roary: rapid large-scale prokaryote pan genome analysis. Bioinformatics 31, 3691–3693. doi: 10.1093/bioinformatics/btv421
Pakbin, B., Brück, W. M., and Rossen, J. W. (2021). Virulence factors of enteric pathogenic Escherichia coli: a review. Int. J. Mol. Sci. 22:9922. doi: 10.3390/ijms22189922
Papouskova, A., Masarikova, M., Valcek, A., Senk, D., Cejkova, D., Jahodarova, E., et al. (2020). Genomic analysis of Escherichia coli strains isolated from diseased chicken in the Czech Republic. BMC Vet. Res. 16, 1–10. doi: 10.1186/s12917-020-02407-2
Partridge, S. R., Kwong, S. M., Firth, N., and Jensen, S. O. (2018). Mobile genetic elements associated with antimicrobial resistance. Clin. Microbiol. Rev. 31:e00088. doi: 10.1128/cmr00088-17
Peng, J., Feng, J., Ji, H., Kong, X., Hong, J., Zhu, L., et al. (2024). Emergence of rarely reported extensively drug-resistant Salmonella enterica Serovar Paratyphi B among patients in East China. Antibiotics 13:519. doi: 10.3390/antibiotics13060519
Poirel, L., Palmieri, M., Brilhante, M., Masseron, A., Perreten, V., and Nordmann, P. (2020). PFM-like enzymes are a novel family of subclass B2 metallo-β-lactamases from Pseudomonas synxantha belonging to the Pseudomonas fluorescens complex. Antimicrob. Agents Chemother. 64:e01700. doi: 10.1128/aac.01700-19
Price, M. N., Dehal, P. S., and Arkin, A. P. (2010). FastTree 2–approximately maximum-likelihood trees for large alignments. PLoS One 5:e9490. doi: 10.1371/journal.pone.0009490
Radics, J., Königsmaier, L., and Marlovits, T. C. (2014). Structure of a pathogenic type 3 secretion system in action. Nat. Struct. Mol. Biol. 21, 82–87. doi: 10.1038/nsmb.2722
Romeo, L., Esposito, A., Bernacchi, A., Colazzo, D., Vassallo, A., Zaccaroni, M., et al. (2023). Application of cloning-free genome engineering to Escherichia coli. Microorganisms 11:215. doi: 10.3390/microorganisms11010215
Rotz, C., Kleinman, P. J. A., Dell, C. J., Veith, T. L., and Beegle, D. B. (2011). Environmental and economic comparisons of manure application methods in farming systems. J. Environ. Qual. 40, 438–448. doi: 10.2134/jeq2010.0063
Rotz, C. A., Stout, R. C., Holly, M. A., and Kleinman, P. J. A. (2020). Regional environmental assessment of dairy farms. J. Dairy Sci. 103, 3275–3288. doi: 10.3168/jds.2019-17388
Roux, S., Enault, F., Hurwitz, B. L., and Sullivan, M. B. (2015). VirSorter: mining viral signal from microbial genomic data. PeerJ 3:e985. doi: 10.7717/peerj.985
Sarowska, J., Futoma-Koloch, B., Jama-Kmiecik, A., Frej-Madrzak, M., Ksiazczyk, M., Bugla-Ploskonska, G., et al. (2019). Virulence factors, prevalence and potential transmission of extraintestinal pathogenic Escherichia coli isolated from different sources: recent reports. Gut Pathog. 11, 1–16. doi: 10.1186/s13099-019-0290-0
Seemann, T. (2014). Prokka: rapid prokaryotic genome annotation. Bioinformatics 30, 2068–2069. doi: 10.1093/bioinformatics/btu153
Shams, S., Hashemi, A., Esmkhani, M., Kermani, S., Shams, E., and Piccirillo, A. (2018). Imipenem resistance in clinical Escherichia coli from Qom, Iran. BMC Res. Notes 11, 1–5. doi: 10.1186/s13104-018-3406-6
Shariati, A., Arshadi, M., Khosrojerdi, M. A., Abedinzadeh, M., Ganjalishahi, M., Maleki, A., et al. (2022). The resistance mechanisms of bacteria against ciprofloxacin and new approaches for enhancing the efficacy of this antibiotic. Front. Public Health 10:1025633. doi: 10.3389/fpubh.2022.1025633
Siguier, P., Perochon, J., Lestrade, L., Mahillon, J., and Chandler, M. (2006). ISfinder: the reference Centre for bacterial insertion sequences. Nucleic Acids Res. 34, D32–D36. doi: 10.1093/nar/gkj014
Sora, V. M., Meroni, G., Martino, P. A., Soggiu, A., Bonizzi, L., and Zecconi, A. (2021). Extraintestinal pathogenic Escherichia coli: virulence factors and antibiotic resistance. Pathogens 10:1355. doi: 10.3390/pathogens10111355
Starikova, E. V., Tikhonova, P. O., Prianichnikov, N. A., Rands, C. M., Zdobnov, E. M., Ilina, E. N., et al. (2020). Phigaro: high-throughput prophage sequence annotation. Bioinformatics 36, 3882–3884. doi: 10.1093/bioinformatics/btaa250
Tambat, R., Mahey, N., Chandal, N., Verma, D. K., Jangra, M., Thakur, K. G., et al. (2022). A microbe-derived efflux pump inhibitor of the resistance-nodulation-cell division protein restores antibiotic susceptibility in Escherichia coli and Pseudomonas aeruginosa. ACS Infect. Dis. 8, 255–270. doi: 10.1021/acsinfecdis.1c00281
Tamura, K., Stecher, G., and Kumar, S. (2021). MEGA11: molecular evolutionary genetics analysis version 11. Mol. Biol. Evol. 38, 3022–3027. doi: 10.1093/molbev/msab120
Touchon, M., Hoede, C., Tenaillon, O., Barbe, V., Baeriswyl, S., Bidet, P., et al. (2009). Organised genome dynamics in the Escherichia coli species results in highly diverse adaptive paths. PLoS Genet. 5:e1000344. doi: 10.1371/journal.pgen.1000344
Touchon, M., Perrin, A., de Sousa, J. A. M., Vangchhia, B., Burn, S., O’Brien, C. L., et al. (2020). Phylogenetic background and habitat drive the genetic diversification of Escherichia coli. PLoS Genet. 16:e1008866. doi: 10.1371/journal.pgen.1008866
Uddin, T. M., Chakraborty, A. J., Khusro, A., Zidan, B. M. R. M., Mitra, S., Emran, T. B., et al. (2021). Antibiotic resistance in microbes: history, mechanisms, therapeutic strategies and future prospects. J. Infect. Public Health 14, 1750–1766. doi: 10.1016/j.jiph.2021.10.020
Vernikos, G., Medini, D., Riley, D. R., and Tettelin, H. (2015). Ten years of pan-genome analyses. Curr. Opin. Microbiol. 23, 148–154. doi: 10.1016/j.mib.2014.11.016
Vernikos, G. S., and Parkhill, J. (2006). Interpolated variable order motifs for identification of horizontally acquired DNA: revisiting the Salmonella pathogenicity islands. Bioinformatics 22, 2196–2203. doi: 10.1093/bioinformatics/btl369
Vinayamohan, P. G., Pellissery, A. J., and Venkitanarayanan, K. (2022). Role of horizontal gene transfer in the dissemination of antimicrobial resistance in food animal production. Curr. Opin. Food Sci. 47:100882. doi: 10.1016/j.cofs.2022.100882
Waskom, M. L. (2021). Seaborn: statistical data visualization. J. Open Source Softw. 6:3021. doi: 10.21105/joss.03021
Watkins, R. R., and Bonomo, R. A. (2016). Overview: global and local impact of antibiotic resistance. Infect. Dis. Clin. 30, 313–322. doi: 10.1016/j.idc.2016.02.001
Wu, H., Wang, Y., Wu, Y., Qiao, J., Li, H., Zheng, S., et al. (2015). Emergence of β-lactamases and extended-spectrum β-lactamases (ESBLs) producing Salmonella in retail raw chicken in China. Foodborne Pathog. Dis. 12, 228–234. doi: 10.1089/fpd.2014.1859
Yang, P., Chen, Y., Jiang, S., Shen, P., Lu, X., and Xiao, Y. (2018). Association between antibiotic consumption and the rate of carbapenem-resistant gram-negative bacteria from China based on 153 tertiary hospitals data in 2014. Antimicrob. Resist. Infect. Control 7, 1–7. doi: 10.1186/s13756-018-0430-1
Yang, L., Heng, T., Yang, G., Gu, X., Wang, J., and He, X. (2021). Analysis of factors influencing effective utilization coefficient of irrigation water in the Manas River basin. Water 13:189. doi: 10.3390/w13020189
Yasir, M., Farman, M., Shah, M. W., Jiman-Fatani, A. A., Othman, N. A., Almasaudi, S. B., et al. (2020). Genomic and antimicrobial resistance genes diversity in multidrug-resistant CTX-M-positive isolates of Escherichia coli at a health care facility in Jeddah. J. Infect. Public Health 13, 94–100. doi: 10.1016/j.jiph.2019.06.011
Zhang, Z., Schwartz, S., Wagner, L., and Miller, W. (2000). A greedy algorithm for aligning DNA sequences. J. Comput. Biol. 7, 203–214. doi: 10.1089/10665270050081478
Keywords: E. coli, AMR, MDR, ESBL, MGE, genomes, genes
Citation: Bello A, Ning S, Zhang Q, Ni W and Hu S (2025) Genomic analysis of multidrug-resistant Escherichia coli isolated from dairy cows in Shihezi city, Xinjiang, China. Front. Microbiol. 16:1527546. doi: 10.3389/fmicb.2025.1527546
Received: 13 November 2024; Accepted: 06 February 2025;
Published: 26 February 2025.
Edited by:
Zhi Ruan, Zhejiang University, ChinaReviewed by:
Okon Okwong Kenneth, Federal University Wukari, NigeriaCopyright © 2025 Bello, Ning, Zhang, Ni and Hu. This is an open-access article distributed under the terms of the Creative Commons Attribution License (CC BY). The use, distribution or reproduction in other forums is permitted, provided the original author(s) and the copyright owner(s) are credited and that the original publication in this journal is cited, in accordance with accepted academic practice. No use, distribution or reproduction is permitted which does not comply with these terms.
*Correspondence: Shengwei Hu, aHVzaGVuZ3dlaUAxNjMuY29t; Wei Ni, bml3ZWl3b25kZXJmdWxAc2luYS5jb20=
Disclaimer: All claims expressed in this article are solely those of the authors and do not necessarily represent those of their affiliated organizations, or those of the publisher, the editors and the reviewers. Any product that may be evaluated in this article or claim that may be made by its manufacturer is not guaranteed or endorsed by the publisher.
Research integrity at Frontiers
Learn more about the work of our research integrity team to safeguard the quality of each article we publish.