- Xinjiang Key Laboratory for Ecological Adaptation and Evolution of Extreme Environment Biology, College of Life Sciences, Xinjiang Agricultural University, Ürümqi, China
The gut microbiome was involved in a variety of physiological processes and played a key role in host environmental adaptation. However, the mechanisms of their response to altitudinal environmental changes remain unclear. In this study, we used 16S rRNA sequencing and LC-MS metabolomics to investigate the changes in the gut microbiome and metabolism of the Yarkand toad-headed agama (Phrynocephalus axillaris) at different altitudes (−80 m to 2000 m). The results demonstrated that Firmicutes, Bacteroidetes, and Proteobacteria were the dominant phylum, Lachnospiraceae and Oscillospiraceae were the most abundant family, and the low-altitude populations had higher richness than high-altitude populations; Akkermansiaceae appeared to be enriched in high-altitude populations and the relative abundance tended to increase with altitude. The gut microbiome of three populations of P. axillaris at different altitudes was clustered into two different enterotypes, low-altitude populations and high-altitude populations shared an enterotype dominated by Akkermansia, Kineothrix, Phocaeicola; intermediate-altitude populations had an enterotype dominated by Mesorhizobium, Bradyrhizobium. Metabolites involved in amino acid and lipid metabolism differed significantly at different altitudes. The above results suggest that gut microbiome plasticity drives the extensive adaptation of P. axillaris to multi-stress caused by different altitudes. With global warming, recognizing the adaptive capacity of wide-ranging species to altitude can help plan future conservation strategies.
1 Introduction
The impact of global climate change on biodiversity is a focus of concern due to the rapid loss of biodiversity (Ceballos et al., 2017). Revealing the long-term adaptive and phenotypic plasticity of organisms to environmental change is extremely challenging due to the interaction of multiple biotic and abiotic factors that generate complex biological responses (Serén et al., 2023). Understanding plasticity responses to environmental pressures focuses on widely distributed species, especially with extensive altitudinal gradients. Altitude is a complex ecological factor that encompasses a wide range of potential stressors, (temperature, oxygen pressure, and ultraviolet radiation), it has a profound effect on the phenotype, genotype, and geographic distribution of animals. Altitudinal gradients serve as powerful “natural experiments” for testing ecological and evolutionary adaptations (Körner, 2007). Plasticity buffers environmental changes along altitudinal gradients and ultimately evolves specific strategies in genetics, physiology, morphology, and behavior to adapt to local environments (Beldade et al., 2011; Enriquez-Urzelai et al., 2020; Grether, 2005). Ectotherms are more vulnerable to environmental changes than endotherms due to their strong dependence on external conditions for regulating body temperature (Paaijmans et al., 2013). However, several studies have demonstrated that ectotherms exhibit remarkable adaptability to varying altitudes, with significant plasticity in response to environmental changes, including gene expression, metabolic rate regulation, growth, and reproductive strategies (Niu et al., 2022; Yang et al., 2019; Zhang et al., 2023). The changes in gut microbiome at different altitudes and their adaptive significance remain poorly studied (Chen et al., 2022).
The gut microbiome plays an important role in various animal physiological activities, including health, growth, development, and behavior. It can even influence the nervous system through the secretion of metabolites (Ling et al., 2018; Maritan et al., 2024; Pascale et al., 2018; Strati et al., 2017; Sun et al., 2024). Consequently, the host and its gut microbiome coexist in a dynamic mutualistic relationship (Karl et al., 2018). Understanding the origins of the gut microbiome is critical for the identification and interpretation of potential fitness-related traits for the host. The presence of recurrent microbial compositional patterns in the gut microbiome is characterized by differences in the abundance of signature taxa, referred to as enterotypes (Wang et al., 2014). Enterotype was first reported in humans and later extended to other mammalian hosts (Arumugam et al., 2011; Costea et al., 2018; Couch et al., 2021; Hicks et al., 2018). That enterotype can serve as a valuable tool for studying gut microbiome from different habitats and taxa and their effects on the host. On the other hand, metabolomics analyses provide opportunities to assess metabolic regulatory mechanisms and discover new biomarkers of animal responses to environmental stresses (Lankadurai et al., 2013). Fecal metabolomics analysis can identify most of the metabolites present in a sample that reflects the net result of nutrient ingestion, digestion, and absorption by both the gut microbiome and the gut (Karu et al., 2018). Therefore, fecal metabolomics is widely considered a key tool for studying the relationship between hosts and their gut microbiome (Jonas et al., 2018).
Reptiles are highly diverse ectothermic vertebrates, of which the Sauria (lizards) contain 7458 species.1 In recent years, the studies of the gut microbiome in lizards have attracted considerable attention. Many factors can affect the gut microbiome of lizards, as demonstrated by studies of several species including (Shinisaurus crocodilurus), Takydromus septentrionalis, Japalura sensu lato, Teratoscincus roborowskii, Sceloporus grammicus, Eremias multiocellata, and Phrynocephalus vlangalii. These factors include captive conditions (Tang et al., 2020; Zhou et al., 2020), gut and oral compartments (Tian et al., 2022), temperature changes (Yang et al., 2024), diet (Gao et al., 2023; Montoya-Ciriaco et al., 2020), urbanization (Littleford-Colquhoun et al., 2019), and altitudes (Zhang et al., 2018).
Phrynocephalus axillaris is a representative and dominant species in the Northwest Desert Region in China, with prominent desert adaptive ability and a wide altitudinal distribution from Aiding Lake in the Turpan Basin, which is 155 m below sea level, to the Altun-Kunlun Mountains, which is 3,000 m above sea level (Zhao and Zhao, 1999), and the altitudinal gradient of more than 3000 m means that the species needs to cope with different ecological environments. So it is an ideal mode to reveal the impact of altitude changes on gut microbiome. Located in eastern Xinjiang, the Turpan Basin is the second deepest lowland in the world (Fang et al., 2010). And it is a typical arid inland basin characterized by an extremely dry environment, low average annual precipitation, low relative atmospheric humidity, water scarcity and fragile ecosystems, which has resulted in a desert plant community characterized by more Poaceae species and annual herbaceous plants (Tu et al., 2024; Domrös et al., 1992). Altun-Kunlun Mountain is located northward toward the Taklamakan Desert, with an arid climate, low annual precipitation, and an alpine steppe, mainly in a desert environment (Chen and Li, 2023). Current studies on P. axillaris have involved age and growth (Ran et al., 2023), genetic diversity analysis (Xie et al., 2023), phylogeographic patterns, mitochondrial genome (Chen et al., 2019; Zhang et al., 2010), and sexual dimorphism (Cao et al., 2020). However, the effects of different altitude habitats on the gut microbiome of P. axillaris and their ecological adaptation mechanisms are not clear.
Therefore, we used 16S rRNA sequencing and LC-MS metabolomics to investigate the altitude-adaption of gut microbiome and metabolites in widely distributed P. axillaris. We aim to understand the co-evolution of the lizard and its gut microbiome, and thus reveal its complex environmental adaptation mechanisms. Our speculations are as follows: (i) Dominant gut microbiome remains relatively stable, and signature taxa show plasticity to respond to different altitudes. (ii) Low temperatures, hypoxia, and strong UV are present at high altitude, leading to an increase in the abundance of probiotics that enhance energy metabolism; and extremely high temperatures at low altitude lead to an enrichment of probiotics that are associated with thermo-tolerance and anti-inflammatory effects. (iii) Decreased fitness of populations at low and high altitude resulted in a similar enterotype associated with stress response, which is different from that of intermediate altitude populations.
2 Materials and methods
2.1 Fecal sample collection and processing
Individuals of P. axillaris were captured from Turpan City, Yuli County, Qiemo County between June 2023 and August 2023, which are positioned at low altitudes (LA, <500 m, N = 9, −44 m, −25 m, 369 m), intermediate altitudes (IA, 500–1500 m, N = 5, 861 m, 886 m, 1109 m), and high altitudes (HA, >1500 m, N = 8, 1892 m) (Supplementary Appendix Table 1). After capture, P. axillaris were recorded for sex and weight. Subsequently, the lizards were individually maintained in 21 × 14 × 13 cm (L × W × H) plastic boxes, and fecal sampling was used to check for excretion every 1 h to ensure that fresh fecal samples were collected. Fecal samples were collected under aseptic conditions using sterile tweezers and completed fresh fecal samples were collected into sterile cryopreservation tubes, labeled, and stored in liquid nitrogen (Gao et al., 2023). Samples of feces should be collected without providing food to guarantee that they accurately represent the gut microbiota in the field. We collected 22 fecal pellets from 9 samples in LA, 15 fecal pellets from 5 samples in IA, and 32 fecal pellets from 8 samples in HA. After the experiment, all the animals were in good physiological condition and released at the original collection site, and the study was approved by the Animal Welfare and Ethics Committee of Xinjiang Agricultural University (2023014).
2.2 DNA extraction sequencing
Microbial DNA was extracted from fecal samples of individuals from different altitude groups by referring to the E.Z.N.A.® Soil DNA Kit (Omega Bio-Tek, Norcross, GA, U.S.) according to the manufacturer’s protocols. To test the quality of the DNA, agarose gel electrophoresis was conducted. The 16S rRNA gene was amplified using the forward primer 27F (5′-AGRGTTYGATYMTGGCTCAG-3′) and the reverse primer 1492R (5′-RGYTACCTTGTTACGACTT-3′) (Callahan et al., 2019). The PCR amplification was performed in a total volume of 20 μL, including: 4 μL 5× FastPfu Buffer, 2 μL 2.5 mM dNTPs, 0.8 μL of each primer (5 μM), 0.4 μL FastPfu Polymerase, and 10ng template DNA. The reactions were performed on GeneAmp®9700 with the following amplification conditions: initial denaturation at 95°C for 5 min; followed by 27 cycles of denaturation at 95°C for 30 s, annealing at 55°C for 30 s, elongation at 72°C for 45 s; and final extension at 72°C for 10 min. Amplicons were extracted from 2% agarose gels and purified using the AxyPrep DNA Gel Extraction Kit (Axygen Biosciences, Union City, CA, U.S.) according to the manufacturer’s instructions.
SMRTbell libraries were prepared from the amplified DNA by blunt ligation according to the manufacturer’s instructions (Pacific Biosciences). Purified SMRTbell libraries from the Zymo and HMP mock communities were sequenced on dedicated PacBio Sequel II 8M cells using the Sequencing Kit 2.0 chemistry. Purified SMRTbell libraries from the pooled and barcoded samples were sequenced on a single PacBio Sequel II cell.
2.3 Data processing and analysis
PacBio raw reads were processed using the SMRT Link Analysis software version 9.0 to obtain demultiplexed circular consensus sequence (CCS) reads. Raw reads were processed through SMRT Portal to filter sequences for length and quality. OTUs were clustered according to a 98.65% similarity threshold using UPARSE (version 7.1),2 and chimeric sequences were identified and removed using UCHIME. The phylogenetic affiliation of each 16S rRNA gene sequence was analyzed by RDP Classifier3 against the silva (SSU132) 16S rRNA database using a confidence threshold of 70% (Uparse, 2013). Species taxonomic annotation was performed for each sequence using RDP Classifier (see text footnote 3, version 2.2), chimera sequences were removed with the UCHIME algorithm. These effective tags were clustered into operational taxonomic units (OTUs) based on a sequence similarity threshold of 97% using UPARSE (version 7.0) (Amato et al., 2013; Wang et al., 2007).
The rarefaction analysis is based on Mothur verson.1.30.1 (Schloss et al., 2011) was conducted to reveal the diversity indices, including the Chao1, ACE, Shannon and Simpson diversity indices. The beta diversity analysis was performed using Bray-Curtis to compare the results of the principal component analysis (PCoA) using the community ecology package, R-forge (Vegan 2.0 package was used to generate a PCoA figure) (Dixon, 2003). Adonis to assess significant differences in microbiological structural differences across groups (Kelly et al., 2015), One-way analysis of variance (ANOVA) tests were performed to assess the statistically significant difference of diversity indices among samples. Relative abundance of gut microbiome at different taxonomic levels was tested using the Kruskal-Wallis H test. Differences were considered significant at P < 0.05. For identification of biomarkers for highly dimensional colonic bacteria, LEfSe (linear discriminant analysis effect size) analysis was done (Segata et al., 2011), and followed by LDA > 3.5, P < 0.05 analysis to screen for differential. The enterotypes were obtained from the relative abundance profiles at the genus level using Jensen-Shannon divergence (JSD) and partitioning around medoid (PAM) clustering in R (version R-3.4.3). JSD is based on the abundance method and is suitable for revealing variations in abundance taxa. To identify genus taxa contributing to enterotype, we applied the SIMPER method (Guo et al., 2021; Lee et al., 2020). Phylogenetic Investigation of Communities by Reconstruction of Unobserved States (PICRUSt2)4 program based on the Kyoto Encyclopedia of Genes and Genomes (KEGG) database was used to predict the functional alteration of the microbiome in different samples (Douglas et al., 2019). Significant differences in functional pathways were assessed using one-way ANOVA or Kruskal-Wallis H tests, P < 0.05.
2.4 LC-MS metabolomics detection and analysis
Fecal samples (100 mg) were individually ground with liquid nitrogen, and the homogenate was resuspended with prechilled 80% methanol and 0.1% formic acid by well vortex, incubated at 4°C for 5 min and centrifuged at 15000 rpm for 5 min. Some of supernatant was diluted to final concentration containing 53% methanol by LC-MS grade water. The samples were transferred to a fresh Eppendorf tube and then were centrifuged at 15000 g, 4°C for 10 min. Finally, the supernatant was injected into the LC-MS/MS system analysis (Want et al., 2006). Liquid sample (100 μL) and prechilled methanol (400 μL) were mixed by well vortexing (Barri and Dragsted, 2013). Cell samples and 4 times prechilled 80% methanol were mixed by well vortexing and then sonicated for 6 min. Repeating this step once again and then operating the same steps as above (Yuan et al., 2012). UHPLC-MS/MS analyses were performed using a Vanquish UHPLC system (Thermo Fisher, Germany) coupled with an Orbitrap Q Exactive™ HF mass spectrometer (Thermo Fisher, Germany).
2.5 LC-MS metabolomics data processing and analysis
The raw data files generated by UHPLC-MS/MS were processed using Compound Discoverer 3.1 (CD3.1, Thermo Fisher) to perform peak alignment, peak picking, and quantitation for each metabolite. Peaks with a signal-to-noise ratio (S/N) greater than 50 were considered and normalized to the intensity of the total spectral intensity. After that, peak intensities were normalized to the total spectral intensity. The normalized data was used to predict the formula based on additive ions, molecular ion peaks, and fragment ions. And then peaks were matched with the mzCloud,5 mzVault, and MassList databases to obtain accurate and relative quantitative results (Sapozhnikova and Nuñez, 2022). Statistical analyses were performed with the statistical software R (version R-3.4.3).
The KEGG database6 was used to annotate these metabolites for annotation (Gil de la Fuente et al., 2017). This was followed by Principal Component Analysis (PCA), Partial Least Squares Regression Analysis (PLS-DA) and Orthogonal Partial Least Squares Discriminant Analysis (OPLS-DA) to visualize the samples for clustering (Szymanska et al., 2011). We used one-way analysis of variance (t-test) to calculate statistical significance (P-value). VIP > 1 (VIP: projected variable importance), P-value < 0.05 and FC > 1 (FC: fold change) were considered as differential metabolites. Based on the log2FC, P-value of metabolites, volcano plots and bi-directional bar charts were used to screen for significantly different metabolites (Ahluwalia et al., 2021; Li et al., 2014).
3 Results
3.1 Composition of gut microbiome at different altitudes
Using these 22 samples, we identified 36036 OTUs based on 98.65% nucleotide sequence identification total reads. A total of 1460 OTUs were shared among samples from the three populations of P. axillaris at different altitudes. The unique OTUs from HA, IA, and LA were 12085, 4040, and 13539, respectively (Supplementary Appendix Figure 1A).
3.2 The community structure of the gut microbiome at different altitudes
At the phylum level, the dominant microbiota were Firmicutes (42.01 ± 2.01%), Bacteroidetes (29.21 ± 1.83%), Proteobacteria (14.86 ± 1.77%), and Verrucomicrobia (11.98 ± 1.75%). At the family level, the dominant microbiota were Lachnospiraceae (17.47 ± 2.34%), Oscillospiraceae (12.83 ± 1.16%), Akkermansiaceae (11.96 ± 1.75%), Bacteroidaceae (10.70 ± 1.12%). At the genus level, the dominant microbiota were Akkermansia (11.96 ± 1.75%), Odoribacter (7.58 ± 1.11%), Bacteroides (6.25 ± 0.69%), and Parabacteroides (4.79 ± 0.70%) (Figure 1).
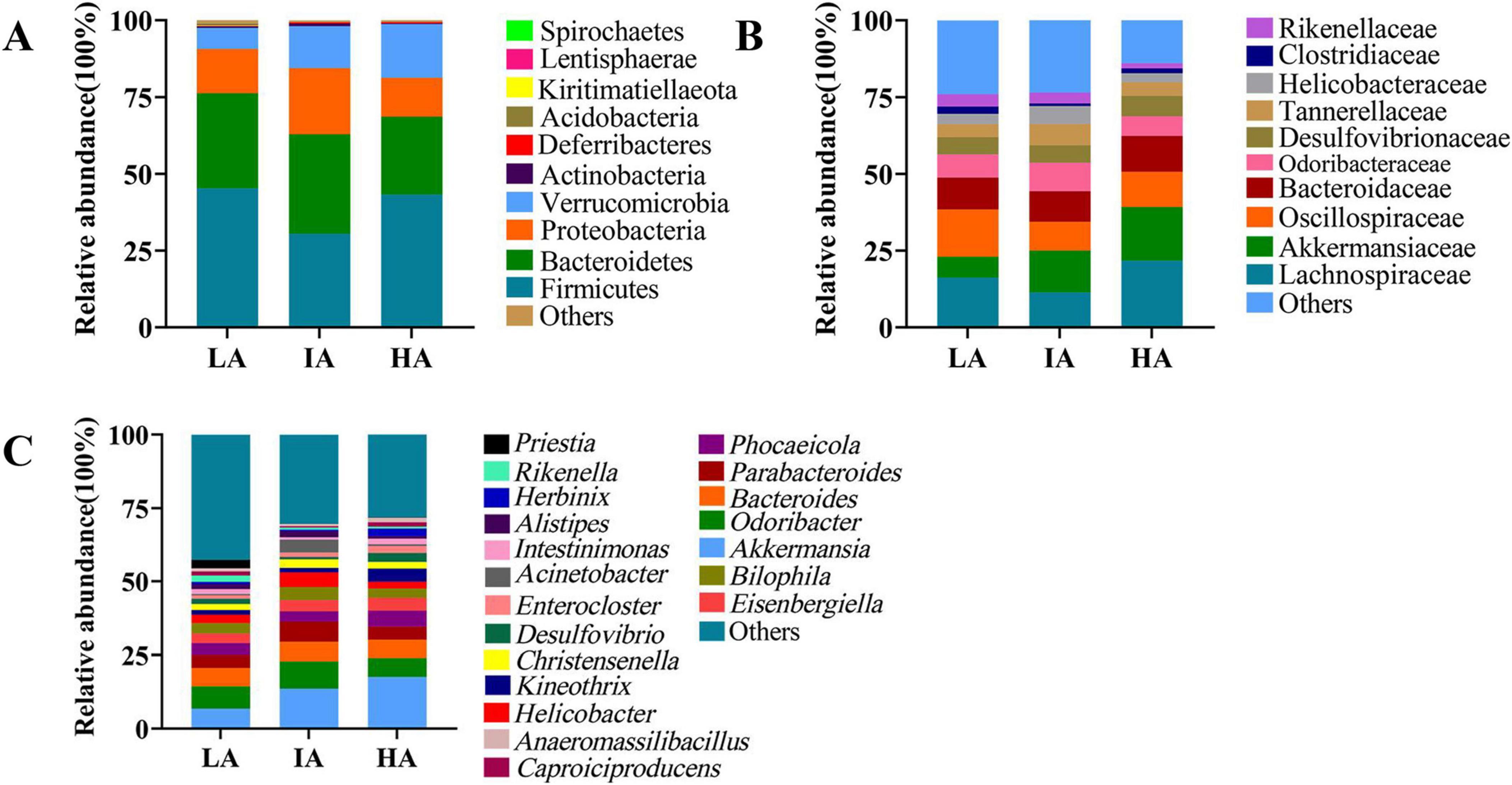
Figure 1. The relative abundance of the gut microbiome at the phylum (A), family (B), and genus (C) levels in three populations of Phrynocephalus axillaris at different altitudes. Different colors in the figures indicate the different microbes composition, and details are shown on the right sides of each figure, respectively.
3.3 Alpha diversity of gut microbiome at different altitudes
Alpha diversity for different altitude groups were compared based on one-way ANOVA test at the phylum level, and the results showed that the community richness (Chao 1 index, ACE index) at LA was higher than that of HA (P = 0.009 < 0.01, P = 0.042 < 0.05) (Figures 2A, B). The community diversity (Shannon index, Simpson index) was not significantly different (Figures 2C, D), showing a gradual decrease in the abundance of the gut microbiome with increasing altitude.
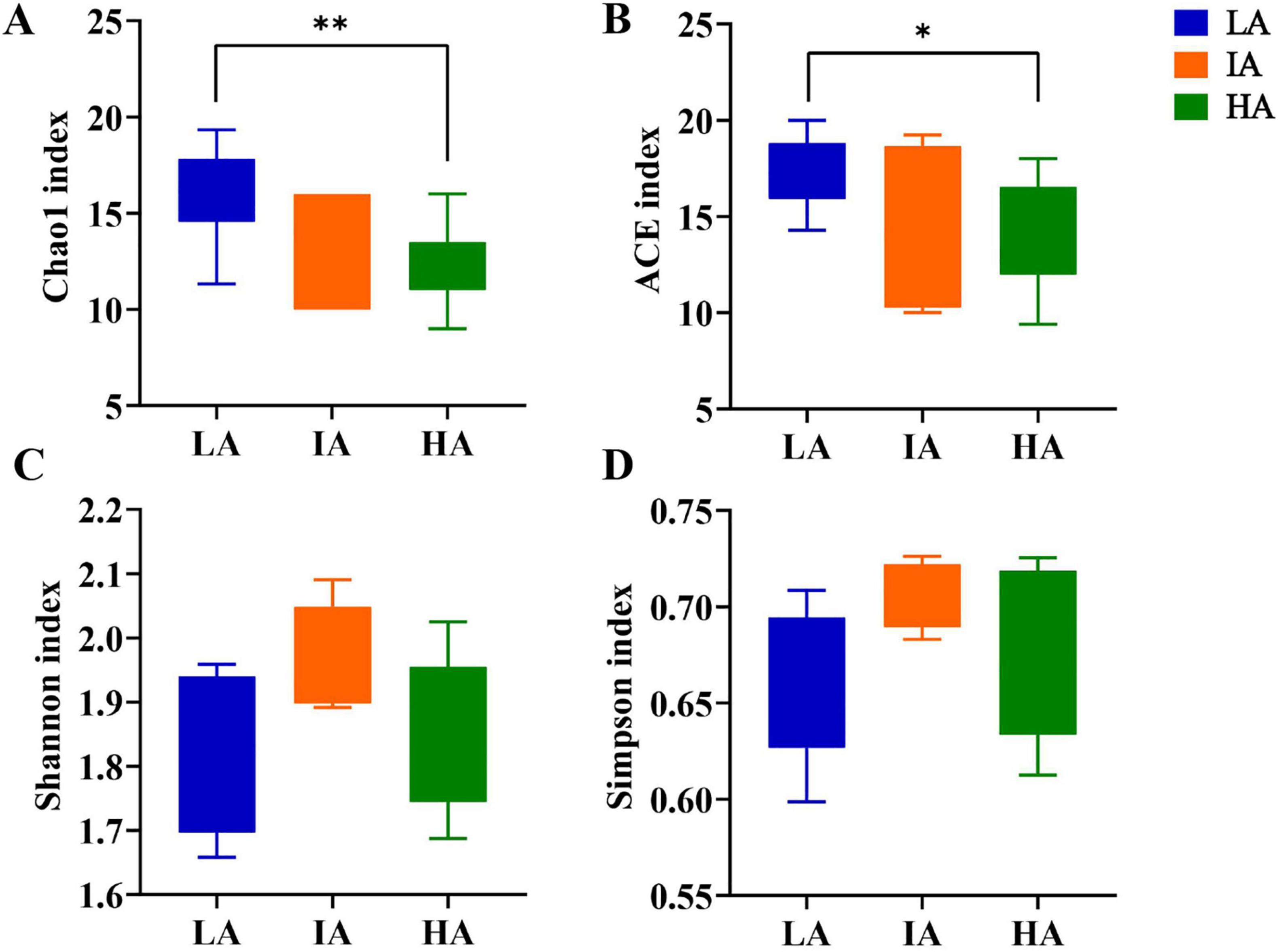
Figure 2. Boxplots of phylum level gut microbiome alpha-diversity of the three populations of Phrynocephalus axillaris at different altitudes. (A) Chao1 index; (B) ACE index; (C) Shannon index; (D) Simpson index (P < 0.05 indicated by*, P < 0.01 indicated by**).
At the OTU level, principal coordinate analysis (PCoA) based on Bray-Curtis distance found that there was a significant separation among three elevation populations of P. axillaris, and the Adonis test also showed that there was a significant difference among them (Adonis test, R2 = 0.1474, P = 0.001; Supplementary Appendix Figure 1B).
3.4 Differences in the gut microbiome composition at different altitudes
Based on Kruskal-Wallis H, at the phylum level, the relative abundance of Verrucomicrobia differed significantly among the three populations, HA was higher than LA (P = 0.005 < 0.01). Further, at the family level, compared with LA, the relative abundance of Akkermansiaceae was significantly increased (P = 0.005 < 0.01) at HA. Compared with LA, the relative abundance of Clostriiaceae was significantly decreased (P = 0.005 < 0.01) at IA. At the genus level, compared with LA, the relative abundance of Akkermansia was significantly increased (P = 0.005 < 0.01) at HA (P = 0.005 < 0.01) (Figure 3). Among them, the relative abundance of Verrucomicrobia, Akkermansia, and Akkermansia increased with altitude.
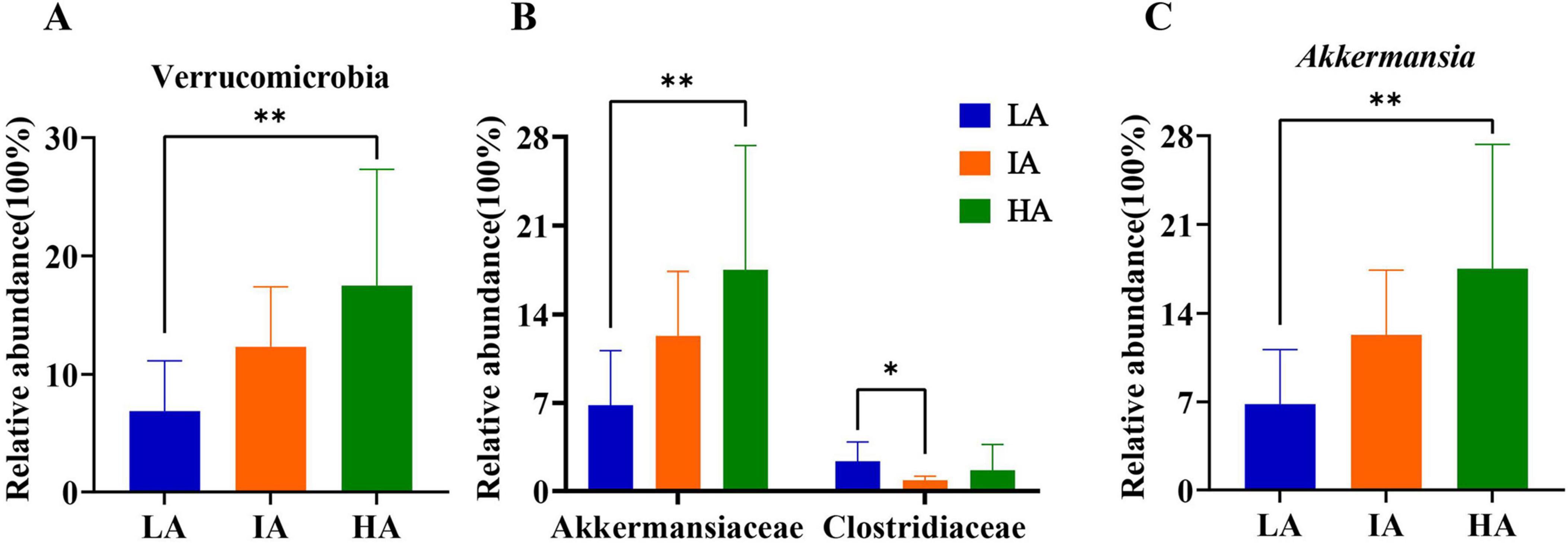
Figure 3. Differences in the relative abundance of microbiome at phyla (A), family (B), and genus (C) levels in three populations of Phrynocephalus axillaris at different altitudes. P < 0.05 indicated by*, P < 0.01 indicated by**.
LEfSe analysis shows that the abundance of f_Dysgonomonadaceae and f_Clostridiaceae were higher enrichment at LA, Akkermansiaceae was higher enrichment at HA (Figure 4A).
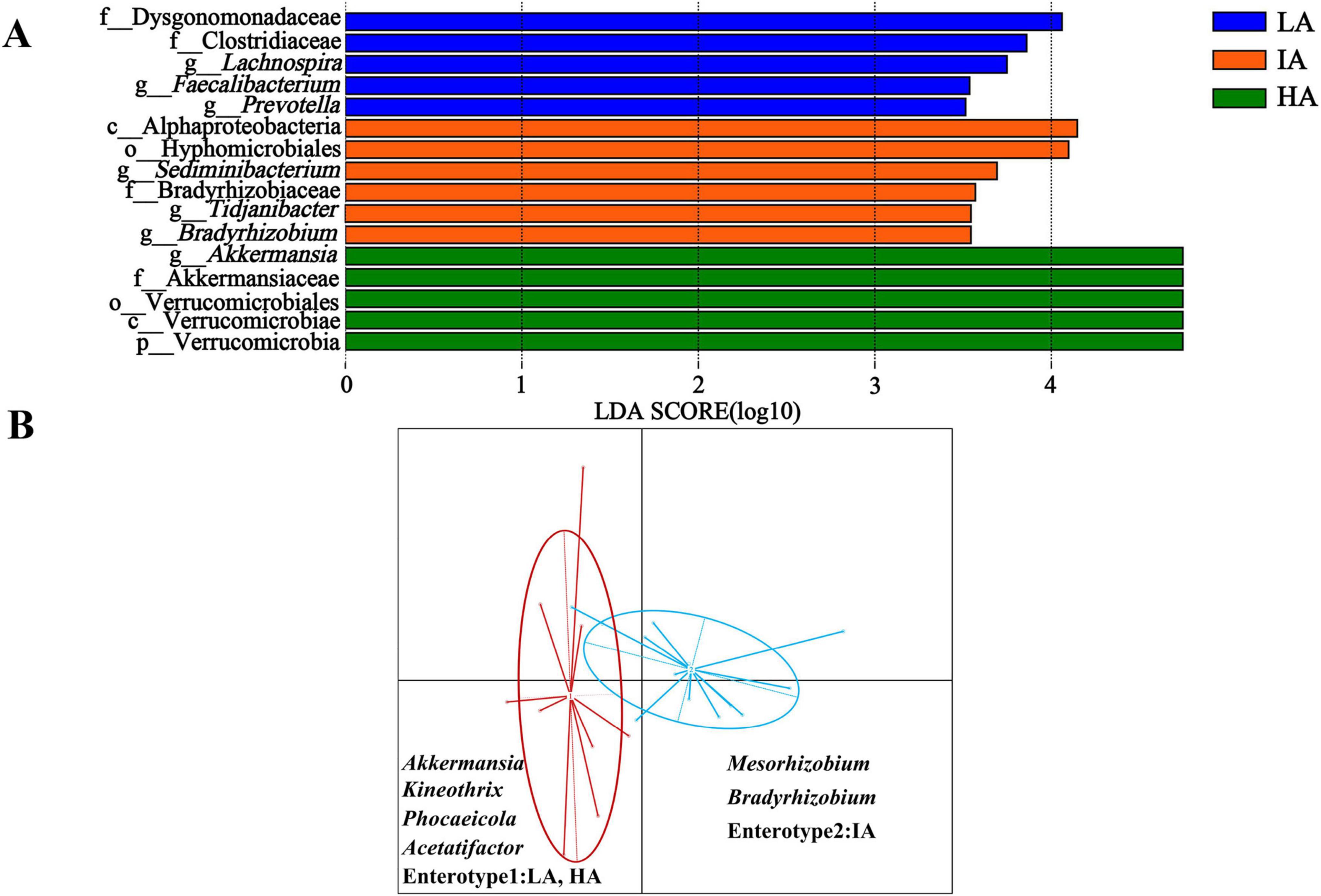
Figure 4. Differences in gut microbiome taxonomic composition among three elevation populations of Phrynocephalus axillaris at different altitudes. (A) Differences in gut microbiome determined by linear discriminate analysis of effect size (LEfSe) among three populations of P. axillaris at different altitudes. (B) Gut microbiome enterotype associated with elevation using Bray-Curtis dissimilarity of P. axillaris. The highlighted taxa were significantly enriched in the group that corresponds to each color (P < 0.05). Linear discriminatory analysis (LDA) scores >3.5.
Two distinct enterotypes were formed from 22 samples. Each enterotype was driven by the variation of its representative genera level: Akkermansia, Kineothrix, and Phocaeicola in enterotype 1, occurring at LA and HA. Mesorhizobium and Bradyrhizobium in enterotype 2, occurring at IA (Figure 4B).
3.5 Functional prediction of gut microbiome at different altitudes
KEGG analysis showed that the predicted functions in gut microbiota were mainly involved in Metabolism (67.98% ± 0.23%), Genetic Information Processing (16.35% ± 0.20%), Environmental Information Processing (9.33% ± 0.20%), Cellular Processes (4.08% ± 0.09%), Organismal Systems (1.19% ± 0.01%), and Human Diseases (1.09% ± 0.03%) at the first level. Based on one-way ANOVA tests, significant differences in the metabolic pathways of Human Diseases among the three populations (Figure 5A). In the differential analysis of the KEGG metabolic pathways at the second level, IA showed significantly higher than HA such as Amino acid metabolism, Cell growth and death, Neurodegenerative disease, Cancer:specific types, Cancer: overview, Cardiovascular disease, and Infectious disease: parasitic. The metabolic pathways of Amino acid metabolism, Metabolism of terpenoids and polyketides of LA were significantly higher than HA (Figure 5B).
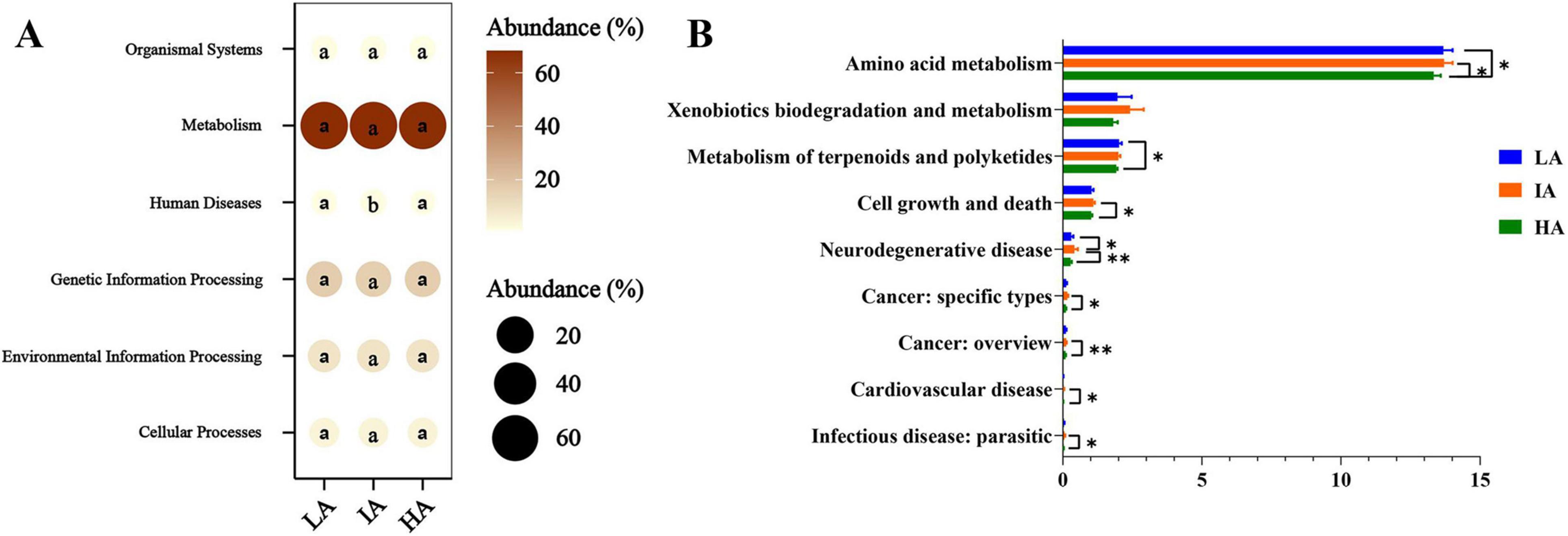
Figure 5. Different functions of gut microbiome of three populations of Phrynocephalus axillaris at different altitudes. The microbial functions were predicted using PICRUSt2 at the first (A) and the second (B) level of the KEGG pathway and were expressed as relative abundances. P < 0.05 indicated by*, P < 0.01 indicated by**.
3.6 Fecal metabolic analysis and screening
There were 1649 metabolites obtained by LC-MS metabolic analysis among three populations of P. axillaris at different altitudes. The PCA score plot showed significant differences in metabolites at different altitudes (PC1: 20.2%, PC2: 11.7%) (Figure 6A). The PLS-DA model was efficient and reliable with a low risk of overfitting (Q2 = 0.88, R2 = 0.97, P = 0.03 < 0.05). The metabolic curves were clearly different at different altitudes, which indicates obvious differences in fecal metabolites of three populations of P. axillaris at different altitudes (Figure 6B). VIP analysis of fecal metabolites from three populations identified the top 10 key metabolites (Figure 6C). The five metabolites, including Tropine, Pilocarpine, 4′-(Imidazol-1-yl)acetophenone, Ergothioneine, and 5-Phenylvaleric Acid tend to be higher with increasing altitude (Figure 6D).
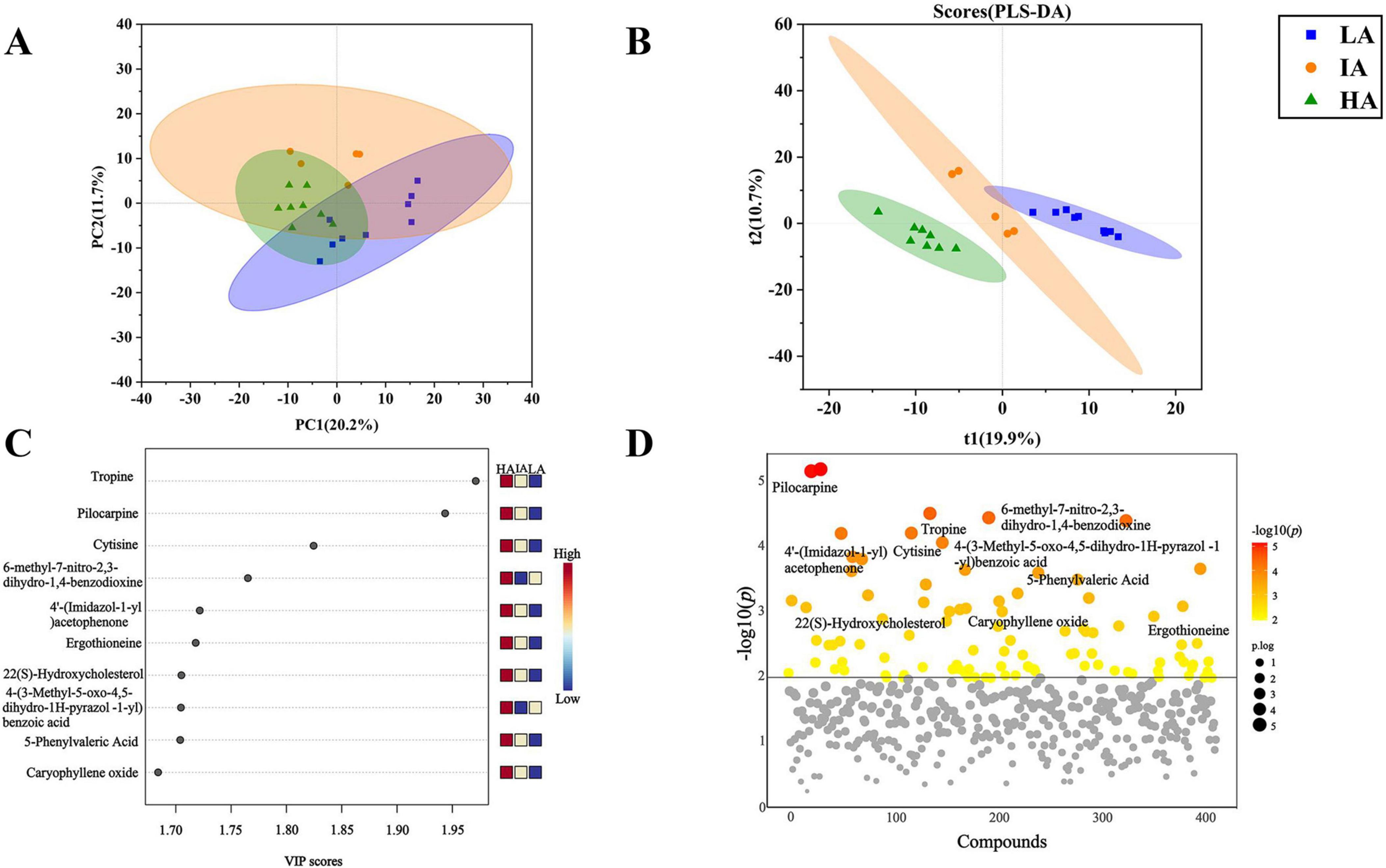
Figure 6. Principal coordinate analysis (PCA) (A) and PLS-DA analysis diagram (B) based on metabolomics of the three populations of Phrynocephalus axillaris at different altitudes. Metabolites variable importance of projection (VIP) analysis (C) and ANOVA test of metabolites (VIP > 1) (D) in fecal sample from three populations of P. axillaris at different altitudes, metabolites above the solid line had significant differences.
The OPLS-DA analysis was performed on the pairwise metabolite data with par scaling, consisting of HA, IA, and LA (Supplementary Appendix Figure 2). Between the two sample groups of LA and IA, LA and HA, and IA and HA (Q2 = 0.788, R2Y = 0.99; Q2 = 0.779, R2Y = 0.999; Q2 = 0.747, R2Y = 0.99), indicated an excellent model and the metabolic curves were clearly different. Differential metabolites were screened based on the criteria of log2FC ≥ 1, OPLS-DA_VIP ≥ 1, P ≤ 0.05 to get the top 5 metabolites in each group (Supplementary Appendix Figure 3). Compared with IA, the level of L-arginine, 3-Methyl-2-oxobutanoic acid showed significant decrease at LA, which they were mainly involved in the metabolism of amino acids.
The absolute values of log2FC (Fold Change, FC) were sorted to get the top 10 metabolites in each group (Supplementary Appendix Figure 4). As compared to IA, Prostaglandin D3, 19(R)-Hydroxy-prostaglandin E2, Cannabigerolic acid, isoleucine, 5-Methyluridine were found to be significantly higher at LA. As compared to HA, the metabolites of Glycolithocholic acid and N-Acetyl-L-methionine increased significantly at IA.
4 Discussion
Understanding how ectotherms respond and eventually adapt to different altitudes is of great relevance in evolutionary biology, not only to better interpret how they thrive under conditions of increasing environmental harshness but also to predict how endangered species can respond to rapid climate change (Serén et al., 2023). The gut microbiome is highly relevant as it might be related to ecophysiological adaptations of ectotherms to environmental changes in the context of elevation change (Bestion et al., 2017). P. axillaris is distributed from high altitude mountain environments to the low altitudual Turpan Basin due to its exceptional wide-ranging adaptations (Arzigul, 2021). Their unique environments are characterized by harsh climates, with high altitude being characterized by low temperatures, low oxygen, strong UV, and low altitude being characterized by high temperatures and drought. Altitudinal changes affect the diversity, structure, and stability of the gut microbiome, which has evolved unique physiological adaptations to different environments (Du et al., 2022). The gut microbiome of the three populations of P. axillaris at different altitudes was significantly distinct in terms of diversity, composition, and function, reflecting the plasticity of the gut microbiome that facilitates adaptation to different altitudes.
4.1 Dominant gut microbiome at different altitudes
The dominant gut microbiome remained relatively stable despite changes with different elevations. The dominan gut microbiome of P. axillaris was dominated by Firmicutes and Bacteroidetes, consistent with previous descriptions of vertebrate (including lizard) (Ibáñez et al., 2021; Youngblut et al., 2019), demonstrating the functional importance of microbiome and ecological significance. Firmicutes and Bacteroidetes are involved in the metabolism of carbohydrates, short-chain fatty acids, and vitamins (Lapébie et al., 2019; Lara et al., 2017), and play an important role in the regulation of host metabolism, and the maintenance of gut microbiome stability. The higher abundance of Lachnospiraceae and Oscillospiraceae, which are abundant in the gut of mammals, particularly humans and ruminants, has been shown to be associated with the production of butyrate, which has an important role in the maintenance of colonic epithelial tissue (Duncan et al., 2002; Zhang et al., 2019). In addition, Lachnospiraceae are involved in carbohydrate metabolism, fatty acid synthesis and degradation, branched-chain amino acid biosynthesis, purine and urea metabolism, and folate biosynthesis (Lin et al., 2024). The abundance of Oscillospira increased in response to prolonged fasting in lizards and other vertebrates as well, probably promoting the degradation of some glycans of the host (Buglione et al., 2022). Wildlife are more likely to face unpredictable environments, such as food or water shortages, rapid changes in temperature, etc., and the nutrient compensation of gut microbiome under adverse conditions is crucial for animal survival.
4.2 Potential adaptation of the gut microbiome to high altitude
The Chao1 and ACE richness index of the gut microbiome of P. axillaris decreased with increasing altitude. The decrease in Alpha diversity of gut microbiome in the high altitude population of P. vlangalii was considered to be an important manifestation of hypoxic acclimatization (Zhang et al., 2018). It is worth noting that the elevation range of P. vlangalii was 2900–4250 m, all of which were in the high-elevation interval, whereas P. axillaris covered the range of very low to intermediate-high elevations.
The relative abundance of Verrucomicrobia, which is the fourth most abundant gut microbiome in P. axillaris, increased gradually with elevation and was enriched in HA. This was caused by the increase in the relative abundance of Akkermansiaceae, Akkermansia. Akkermansiaceae are mucus-degrading bacteria living in the mucus layer of the gut (Belzer and De Vos, 2012), and their ability to adhere to the mucus layer is considered to be a typical characteristic of probiotics. It has been found that Akkermansiaceae may be one of the immunomodulators that are negatively associated with human diseases such as obesity, diabetes, inflammation, and metabolic disorders (Roopchand et al., 2015; Zhang et al., 2019), and potential bio-indicators for cancer patients (Huang et al., 2021; Kim et al., 2021). It has been found that Akkermansiaceae (Akkermansia muciniphila) can adhere to the gut mucosal interface between the lumen and host cells to protect epithelial cells from pathogenic microbes. Potential probiotics enriched at high altitude are likely to help P. axillaris improve their metabolic capacity and maintain host health to adapt to the harsh, cold, and hypoxic high altitude environment, where they are highly competitive on a restricted diet of low calorie and nutrient levels at high altitude (Derrien et al., 2004).
The results of PICRUSt2 showed significant differences in metabolic pathways such as Amino acid metabolism, and the relative abundance at IA was significantly higher than at HA, which suggests that high-altitude environments alter the gut microbiome, leading to changes in physiological and metabolic functions. It has been shown that the low-temperature, low-oxygen environment at high altitude threatens biological function and survival, and the body’s self-protection produces an adaptive response to reduce energy demand (Murray and Montgomery, 2014). Metabolically, Glycolithocholic acid and N-Acetyl-L-methionine were significantly decreased in the HA compared to IA. Glycolithocholic acid is a secondary bile acid metabolite that plays an important role in the host and in disease (Cai et al., 2024), and N-Acetyl-L-methionine has antioxidant effects on fats and proteins (Liu Y. et al., 2024). It has been shown that the major oxidative factors UVR, temperature, and oxygen pressure decrease with altitude, resulting in a less oxidative environment at high altitude (Costantini, 2014), and therefore less oxidative damage in high-altitude lizard populations (Reguera et al., 2015), with down-regulation of the relevant metabolites involved.
4.3 Potential adaptations of the gut microbiome to low altitude
LEfSe analysis showed that f_Dysgonomonadaceae, f_Clostridiaceae, g_Lachnospira, g_Faecalibacterium, and g_Prevotella appeared to be enriched in P. axillaris at LA. Among them, f_Dysgonomonadaceae and f_Clostridiaceae are involved in the digestion and degradation of polysaccharides in food as thermo-tolerant bacteria (Appert et al., 2020; Jiang et al., 2017; Murakami et al., 2018). g_Lachnospira, g_ Faecalibacterium are associated with intestinal diseases (Vacca et al., 2020). Organisms inhabiting extreme environments have evolved extreme environmental adaptations in order to avoid extinction (Berihulay et al., 2019). The enrichment of the gut microbiome at low altitude Turpan Basin with the extremely dry and hot climate, reveals the effect of heat stress on the intestinal mucosal barrier (Koch et al., 2024; Wen et al., 2021).
L-arginine and 3-methyl-2-oxobutanoic acid are involved in the absorption and utilization of proteins high-regulated in IA, compared with LA (Hallen et al., 2013). Prostaglandin D3 and 19(R)-Hydroxy-prostaglandin E2, which are involved in lipid metabolism (Sam et al., 2012), are high-regulated in LA, compared with IA. A related study demonstrated that the intestinal symbiotic bacterium Klebsiella michiganensis modulates L-arginine levels, thereby enhancing resistance to low-temperature stress in the orange fruit fly (Bactrocera dorsalis) (Raza et al., 2020). The intermediate elevations P. axillaris appeared to have followed a similar metabolic pattern over a long evolutionary period. Temperatures can alter the digestive energy of lizards and differentially affect the utilization of proteins and lipids in the insect-dominated diet (Belhadj Slimen et al., 2016; Plasman et al., 2019), with elevated temperatures increasing the lipid metabolism of lizards (Schramm et al., 2018; Wang et al., 2019), and protein synthesis, and degradation metabolism decreases (Ríus, 2019). The series of metabolite changes may be due to the effects of the extremely high environmental temperatures in the Turpan Basin.
4.4 Similar response of gut microbiome to different stresses at low and high altitude
A study of Sceloporus grammicus across different altitudes revealed no significant correlation between gut microbiome diversity and elevation (Montoya-Ciriaco et al., 2020). The gut microbiome shaping was associated with changes in diet, met-barcoding based dietary studies of P. axillaris found no significant differences in invertebrate diversity in the diets of the LA, IA, and HA (unpublished data), which seems to indicate that there is no a strong correlation between the shaping of gut microbiome and diet in this species, a pattern that is also frequently observed in studies on mammals (Guo et al., 2021). This pattern places greater emphasis on the nutritional convergence of gut microbiome with food, thereby increasing ecological competitiveness and limiting colonization by exogenous microbes (Hernandez-Baixauli et al., 2024).
Gastrointestinal parasites affect the immune system and health of the host (Barelli et al., 2019). However, host-parasite interactions are complex, and the gut microbiome may be a source of parasitic infection (Gouba and Drancourt, 2015). A met-barcoding based study of the parasites of P. axillaris found no significant differences in parasite abundance in the gut among the LA, IA, and HA (unpublished data), suggesting that the pattern of altitudinal changes in parasites may be complex and related to the lizard’s own tolerance and other natural environmental factors.
Lizard populations are exposed to extreme heat and cold for longer periods of time in the LA and HA, which promotes prolonged periods of inactivity and limits foraging time of lizards, leading to nutritional stress (Zamora-Camacho et al., 2013). Additionally, higher altitude populations grow slower, store less lipids, are smaller, produce fewer offspring (Hodkinson, 2005), and will have reduced fecundity, while at lower altitudes molecular pathways associated with injury will be upregulated due to high temperatures (e.g., cellular stress response), leading to increased population mortality (Schaefer, 2014) and reduced survival. These gradient changes in fecundity and survival combine to result in a sharp decline in the fitness of populations at low and high elevations, while peak fecundity and survival are reached at intermediate elevations (Buckley et al., 2021; Serén et al., 2023). On the other hand, organisms will evolve strategies to adapt to low and high altitude environments, where metabolic enzymes will be selected to up-regulate energy production rates in response to shorter growing seasons (Marden, 2013; Seebacher, 2018) and to enhance fecundity at high altitudes, and at low altitudes, where extreme environmental conditions (e.g., heatwaves) will be mediated through mechanisms such as heat-shock protein expression and anti-oxidative stress resistance leading to animal selection for enhanced heat tolerance (MacMillan, 2019).
The gut microbiome regulates host responses to the external environment, and interactions between host and gut microbiome influence animal physiological performance and adaptations. The gut microbiome of P. axillaris produces similar adaptations to different stressful environments at low and high altitudes, forming 2 different enterotypes, enterotype 1 is found in LA and HA, with representative genera Akkermansia, Kineothrix, and Phocaeicola, and enterotype 2 is found in IA, with representative genera Mesorhizobium, Bradyrhizobium. Enterotype 1 is associated with immune stress. Akkermansia metabolizes nutrients to provide energy for growth (Chelakkot et al., 2018; Hänninen et al., 2018) and can degrade gut mucin to produce short-chain fatty acids such as acetate, propionate, etc. Kineothrix ferments sugar to produce butyrate (Haas and Blanchard, 2017). Phocaeicola can degrade complex polysaccharides into short-chain fatty acids that are involved in the synthesis of vitamins and other bioactive compounds (Lück and Deppenmeier, 2022). Short-chain fatty acid production maintains gut immune status and plays an important role in metabolism, inflammation, and disease (Feng et al., 2018; Rooks and Garrett, 2016; Shealy et al., 2021). Mesorhizobium and Bradyrhizobium in enterotype 2, are not typical in the animal gut, but are considered environmentally beneficial microorganisms and are mainly found in soil (Favero et al., 2021; Laranjo et al., 2014). Mesorhizobium produces rhizobial proteins that provide metabolic and stress-related pathways for plants (Diouf et al., 2015), and associate host interactions and disease progression as an environmentally carrying symbiotic bacterium (Ebenezer et al., 2019). Bradyrhizobium also has the ability to maintain general cellular functions, such as amino acid transport and metabolism, energy production and conversion (Zhong et al., 2024), shows strong positive correlations with another gut microbiome in insects and plays an important role in cold and hot environments (Mazzucco and Schlötterer, 2021). However, the role of enterotype2 dominated by Mesorhizobium and Bradyrhizobium in animal gut is understudied, and should be requires further investigation.
Altitude changes are inevitably accompanied by changes in a variety of environmental factors, such as, temperature, humidity, oxygen content, etc., and the effects of altitude change on organisms are complex (Liu D. et al., 2024). In a study of Sceloporus grammicus, it was shown that the abundance of Akkermansia (Verrucomicrobia) increased significantly at high altitudes with low oxygen partial pressure and low temperatures (Montoya-Ciriaco et al., 2020). However, this change may not be directly due to altitude, but rather to variations in food composition in different altitudinal contexts. Recently studies have focused on how high-altitude environments with low oxygen levels, low temperatures, and intense radiation affect animal reproduction and survival, influencing not only species evolution and physiological adaptation but also the composition of animal gut microbiota (Bai et al., 2022; Wang et al., 2022). Therefore, multiple variable factors in a complex altitudinal context can jointly determine animal gut microbes. The shaping of gut microorganisms in P. axillaris by changes in altitude should also be a joint effect of multiple environmental factors. In the future, the degree of contribution of a particular factor to the formation of gut microbiome in this species should be evaluated under the premise of strictly controlling variation environmental factors.
5 Conclusion
Our study shows that P. axillaris has significant differences in gut microbiome composition, abundance, functional pathways, and metabolites among populations at different altitudes. Such differences may be an adaptation to stressors at different altitudes. The dominant gut microbiome of Firmicutes, Bacteroidetes, Lachnospiraceae, and Oscillospiraceae remained relatively stable across different altitudes, this stable may be a key factor in the strong adaptive ability of P. axillaris. Akkermansia was enriched in high-altitude populations likely in response to low temperature and hypoxic conditions. The significant enrichment of heat-resistant microbiome in low-altitude populations may be an adaptation to high temperature. The plasticity of specific microbiome in the gut may be the main way for the species to flexibly respond to environmental changes. Under similar ecological stresses, low and high-altitude populations shared an enterotype dominated by Akkermansia, Kineothrix, Phocaeicola. Additionally, most metabolite profiles demonstrated the adaptation of gut microbiota to environments at different altitudes.
In the face of rising global temperatures, lizards have limited foraging time, morphological, physiological and behavioral functions may be sub-optimal. On the one hand, P. axillaris, as a widely distributed species, with the stability of its dominant microbiome and the plasticity of its signature taxa, is highly adaptable to cope with changes in low and high altitude environments. On the other hand, with global warming, the species approach a heat-tolerance threshold at low altitude, leading to a decline in biological performance and adaptability. In conclusion, understanding the adaptability of wide-ranging species to different environments can help to respond to global warming.
Data availability statement
The datasets presented in this study can be found in online repositories. The names of the repository/repositories and accession number(s) can be found below: https://www.ncbi.nlm.nih.gov/, PRJNA1160045.
Ethics statement
The animal study was approved by Animal Welfare and Ethics Committee of Xinjiang Agricultural University, Urumqi, Xinjiang, China (2023014). The study was conducted in accordance with the local legislation and institutional requirements.
Author contributions
JD: Conceptualization, Data curation, Formal analysis, Investigation, Methodology, Project administration, Software, Supervision, Validation, Visualization, Writing – original draft, Writing – review and editing. PZ: Conceptualization, Formal analysis, Investigation, Methodology, Software, Supervision, Visualization, Writing – review and editing. WG: Conceptualization, Formal analysis, Investigation, Methodology, Supervision, Visualization, Writing – review and editing. QL: Conceptualization, Formal analysis, Investigation, Supervision, Visualization, Writing – review and editing. LL: Conceptualization, Formal analysis, Investigation, Supervision, Visualization, Writing – review and editing. LS: Conceptualization, Data curation, Formal analysis, Funding acquisition, Investigation, Methodology, Project administration, Resources, Software, Supervision, Validation, Visualization, Writing – original draft, Writing – review and editing.
Funding
The author(s) declare that financial support was received for the research, authorship, and/or publication of this article. This study was supported by the Third Xinjiang Scientific Expedition Program (grant no. 2022xjkk1200).
Acknowledgments
We thank Dongliu Meng and Jiang miao Ran for their assistance in this study.
Conflict of interest
The authors declare that the research was conducted in the absence of any commercial or financial relationships that could be construed as a potential conflict of interest.
Generative AI statement
The authors declare that no Generative AI was used in the creation of this manuscript.
Publisher’s note
All claims expressed in this article are solely those of the authors and do not necessarily represent those of their affiliated organizations, or those of the publisher, the editors and the reviewers. Any product that may be evaluated in this article, or claim that may be made by its manufacturer, is not guaranteed or endorsed by the publisher.
Supplementary material
The Supplementary Material for this article can be found online at: https://www.frontiersin.org/articles/10.3389/fmicb.2024.1501684/full#supplementary-material
Supplementary Appendix Figure 1 | Differences in gut microbiome taxonomic composition of three populations of Phrynocephalus axillaris at different altitudes. (A) Venn diagram showing the unique and shared OTUs levels among three elevation populations of P. axillaris. (B) Principal coordinate analysis (PCoA) plot of the OTUs level gut microbiome Beta-diversity of the three elevation populations of P. axillaris.
Supplementary Appendix Figure 2 | OPLS-D analysis of the metabolic samples of three populations of Phrynocephalus axillaris at different altitudes. (A) LA vs IA, (B) LA vs HA, (C) IA vs HA.
Supplementary Appendix Figure 3 | Annotation and screening of metabolites of three populations of Phrynocephalus axillaris at different altitudes. (A) LA vs IA, (B) LA vs HA, (C) IA vs HA. The volcanic map was used for statistical analysis of differential metabolites in fecal, and the horizontal coordinate indicated the quantitative value of differential metabolites to calculate the corresponding ratio. The ordinate represents the P-value of the metabolite in the T-test between the groups. The volcano plot of the metabolites, red points represent up-regulated metabolites, green point represented down-regulated metabolites and gray points represent have no significant differences metabolites. The top 5 differential metabolites were selected and labeled.
Supplementary Appendix Figure 4 | The bidirectional bar chart was used for statistical analysis of the multiple changes of fecal differential metabolites of three populations of Phrynocephalus axillaris at different altitudes, with the horizontal coordinate representing log2 (FC) and the vertical coordinate representing the names of the top ten differential metabolites in different altitude groups. (A) LA vs IA, (B) LA vs HA, (C) IA vs HA. Dark blue represented down-regulated metabolites and light blue represent up-regulated metabolites.
Footnotes
- ^ http://reptile-database.org/
- ^ http://drive5.com/uparse/
- ^ https://ngdc.cncb.ac.cn/databasecommons/database/id/237
- ^ https://github.com/picrust/picrust2
- ^ https://www.mzcloud.org/
- ^ https://www.genome.jp/kegg/pathway.html
References
Ahluwalia, B., Iribarren, C., Magnusson, M., Sundin, J., Clevers, E., Savolainen, O., et al. (2021). A distinct faecal microbiota and metabolite profile linked to bowel habits in patients with irritable bowel syndrome. Cells 10:1459. doi: 10.3390/cells10061459
Amato, K., Yeoman, C., Kent, A., Righini, N., Carbonero, F., Estrada, A., et al. (2013). Habitat degradation impacts black howler monkey (Alouatta pigra) gastrointestinal microbiomes. ISME J. 7, 1344–1353. doi: 10.1038/ismej.2013.16
Appert, O., Garcia, A., Frei, R., Roduit, C., Constancias, F., Neuzil-Bunesova, V., et al. (2020). Initial butyrate producers during infant gut microbiota development are endospore formers. Environ. Microbiol. 22, 3909–3921. doi: 10.1111/1462-2920.15167
Arumugam, M., Raes, J., Pelletier, E., Le Paslier, D., Yamada, T., Mende, D., et al. (2011). Enterotypes of the human gut microbiome. Nature 473, 174–180.
Arzigul, Y. (2021). Dimorphism and geographic variations of morphological characteristics of Phrynocephalus axillaris. Xinjiang: Xinjiang University.
Bai, X., Liu, G., Yang, J., Zhu, J., Wang, Q., Zhou, Y., et al. (2022). Changes in the gut microbiota of rats in high-altitude hypoxic environments. Microbiol. Spectr. 10, 1626–1622.
Barelli, C., Gonzalez-Astudillo, V., Mundry, R., Rovero, F., Hauffe, H., and Gillespie, T. (2019). Altitude and human disturbance are associated with helminth diversity in an endangered primate. Procolobus gordonorum. PLoS One 14:e0225142. doi: 10.1371/journal.pone.0225142
Barri, T., and Dragsted, L. O. (2013). UPLC-ESI-QTOF/MS and multivariate data analysis for blood plasma and serum metabolomics: Effect of experimental artefacts and anticoagulant. Anal. Chim. Acta 768, 118–128. doi: 10.1016/j.aca.2013.01.015
Beldade, P., Mateus, A., and Keller, R. (2011). Evolution and molecular mechanisms of adaptive developmental plasticity. Mol. Ecol. 20, 1347–1363.
Belhadj Slimen, I., Najar, T., Ghram, A., and Abdrrabba, M. (2016). Heat stress effects on livestock: Molecular, cellular and metabolic aspects, a review. J. Anim. Physiol. Anim. Nutr. 100, 401–412.
Belzer, C., and De Vos, W. (2012). Microbes inside-from diversity to function: The case of Akkermansia. ISME J. 6, 1449–1458. doi: 10.1038/ismej.2012.6
Berihulay, H., Abied, A., He, X., Jiang, L., and Ma, Y. (2019). Adaptation mechanisms of small ruminants to environmental heat stress. Animals 9:75.
Bestion, E., Jacob, S., Zinger, L., Gesu, L., and Cote, J. (2017). Climate warming reduces gut microbiota diversity in a vertebrate ectotherm. Nat. Ecol. Evol. 1:161. doi: 10.1038/s41559-017-0161
Buckley, L., Schoville, S., and Williams, C. (2021). Shifts in the relative fitness contributions of fecundity and survival in variable and changing environments. J. Exp. Biol. 224(Suppl._1):jeb228031. doi: 10.1242/jeb.228031
Buglione, M., Ricca, E., Petrelli, S., Baccigalupi, L., Troiano, C., Saggese, A., et al. (2022). Gut microbiota plasticity in insular lizards under reversed island syndrome. Sci. Rep. 12:12682. doi: 10.1038/s41598-022-16955-0
Cai, X., Li, Z., Yao, Y., Zheng, Y., Zhang, M., and Ye, Y. (2024). Glycolithocholic acid increases the frequency of circulating tregs through constitutive androstane receptor to alleviate postmenopausal osteoporosis. Biochem. Pharmacol. 219:115951. doi: 10.1016/j.bcp.2023.115951
Callahan, B., Wong, J., Heiner, C., Oh, S., Theriot, C., Gulati, A., et al. (2019). High-throughput amplicon sequencing of the full-length 16S rRNA gene with single-nucleotide resolution. Nucleic Acids Res. 47:e103. doi: 10.1093/nar/gkz569
Cao, L., Zhou, T., and Li, J. (2020). Sexual dimorphism in body size of Phrynocephalus axillaris in Tarim Basin, Southern Xinjiang China. Chin. J. Wildlife 41, 182–188.
Ceballos, G., Ehrlich, P., and Dirzo, R. (2017). Biological annihilation via the ongoing sixth mass extinction signaled by vertebrate population losses and declines. Proc. Natl. Acad. Sci. 114, e6089–e6096. doi: 10.1073/pnas.1704949114
Chelakkot, C., Choi, Y., Kim, D., Park, H., Ghim, J., Kwon, Y., et al. (2018). Akkermansia muciniphila-derived extracellular vesicles influence gut permeability through the regulation of tight junctions. Exp. Mol. Med. 50:e450. doi: 10.1038/emm.2017.282
Chen, C., and Li, G. (2023). Temporal and spatial variation of grassland NDVI in kunlun mountains, altun mountains and its responses to temperature and precipitation from 1981 through 2020. Chin. J. Grassl. 45, 13–25.
Chen, D., Li, J., and Guo, X. (2019). Next-generation sequencing yields a nearly complete mitochondrial genome of the Yarkand toad-headed agama (Phrynocephalus axillaris) from the Turpan Depression. Mitochondrial DNA Part B. 4, 1198–1199.
Chen, J., Zhang, L., Zhao, R., Wu, H., Lin, L., Li, P., et al. (2022). Gut microbiota differs between two cold-climate lizards distributed in thermally different regions. BMC Ecol. Evol. 22:120. doi: 10.1186/s12862-022-02077-8
Costantini, D. (2014). Oxidative stress and hormesis in evolutionary ecology and physiology. A Marriage Between Mechanistic and Evolutionary Approaches. Berlin: Springer, 362.
Costea, P., Hildebrand, F., Arumugam, M., Bäckhed, F., Blaser, M., Bushman, F., et al. (2018). Enterotypes in the landscape of gut microbial community composition. Nat. Microbiol. 3, 8–16.
Couch, C., Stagaman, K., Spaan, R., Combrink, H., Sharpton, T., Beechler, B., et al. (2021). Diet and gut microbiome enterotype are associated at the population level in African buffalo. Nat. Commun. 12:2267. doi: 10.1038/s41467-021-22510-8
Derrien, M., Vaughan, E., Plugge, C., and de Vos, W. (2004). Akkermansia muciniphila gen. nov., sp. nov., a human intestinal mucin-degrading bacterium. Int. J. Syst. Evol. Microbiol. 54, 1469–1476. doi: 10.1099/ijs.0.02873-0
Diouf, F., Diouf, D., Klonowska, A., Le Quere, A., Bakhoum, N., Fall, D., et al. (2015). Genetic and genomic diversity studies of Acacia symbionts in Senegal reveal new species of Mesorhizobium with a putative geographical pattern. PLoS One 10:e0117667. doi: 10.1371/journal.pone.0117667
Dixon, P. (2003). VEGAN a package of R functions for community ecology. J. Vegetation Sci. 14, 927–930.
Domrös, M., Peng, G., and Zhang, S. (1992). The characteristics of the desert climate at Turpan, China. Erdkunde 46, 217–233.
Douglas, G., Maffei, V., Zaneveld, J., Yurgel, S., and Langille, M. (2019). PICRUSt2: An improved and extensible approach for metagenome inference. BioRxiv [Preprint]. doi: 10.1101/672295
Du, X., Li, F., Kong, F., Cui, Z., Li, D., Wang, Y., et al. (2022). Altitude-adaption of gut microbiota in Tibetan chicken. Poultry Sci. 101:101998. doi: 10.1016/j.psj.2022.101998
Duncan, S., Hold, G., Harmsen, H., Stewart, C., and Flint, H. (2002). Growth requirements and fermentation products of Fusobacterium prausnitzii, and a proposal to reclassify it as Faecalibacterium prausnitzii gen. nov., comb. nov. Int. J. Syst. Evol. Microbiol. 52, 2141–2146. doi: 10.1099/00207713-52-6-2141
Ebenezer, K., Chidambaram Tamilselvan, C., and Rekha Manivanan, R. (2019). Molecular characterization and distance tree identification of unique environmental carryover proteobacteria Mesorhizobium sp. in the midgut of Anopheles stephensi. Indian J. Mosquito Res. 6, 53–56.
Enriquez-Urzelai, U., Tingley, R., Kearney, M. R., Sacco, M., Palacio, A. S., Tejedo, M., et al. (2020). The roles of acclimation and behaviour in buffering climate change impacts along elevational gradients. J. Anim. Ecol. 89, 1722–1734. doi: 10.1111/1365-2656.13222
Fang, S., Pei, H., Liu, Z., Beven, K., and Wei, Z. (2010). Water resources assessment and regional virtual water potential in the Turpan Basin, China. Water Resour. Manag. 24, 3321–3332.
Favero, V., Carvalho, R., Motta, V., Leite, A., Coelho, M., Xavier, G., et al. (2021). Bradyrhizobium as the only rhizobial inhabitant of mung bean (Vigna radiata) nodules in tropical soils: A strategy based on microbiome for improving biological nitrogen fixation using bio-products. Front. Plant Sci. 11:602645. doi: 10.3389/fpls.2020.602645
Feng, W., Ao, H., and Peng, C. (2018). Gut microbiota, short-chain fatty acids, and herbal medicines. Front. Pharmacol. 9:1354. doi: 10.3389/fphar.2018.01354
Gao, W., Yang, Y., and Shi, L. (2023). Seasonal dietary shifts alter the gut microbiota of a frugivorous lizardTeratoscincus roborowskii(Squamata, Sphaerodactylidae). Ecol. Evol. 13:e10363. doi: 10.1002/ece3.10363
Gil de la Fuente, A., Grace Armitage, E., Otero, A., Barbas, C., and Godzien, J. (2017). Differentiating signals to make biological sense–A guide through databases for MS-based non-targeted metabolomics. Electrophoresis 38, 2242–2256. doi: 10.1002/elps.201700070
Gouba, N., and Drancourt, M. (2015). Digestive tract mycobiota: A source of infection. Medecine Maladies Infectieuses. 45, 9–16.
Grether, G. (2005). Environmental change, phenotypic plasticity, and genetic compensation. Am. Nat. 166, E115–E123.
Guo, N., Wu, Q., Shi, F., Niu, J., Zhang, T., Degen, A., et al. (2021). Seasonal dynamics of diet–gut microbiota interaction in adaptation of yaks to life at high altitude. npj Biofilms Microbiomes 7:38. doi: 10.1038/s41522-021-00207-6
Haas, K., and Blanchard, J. (2017). Kineothrix alysoides, gen. nov., sp. nov., a saccharolytic butyrate-producer within the family Lachnospiraceae. Int. J Syst. Evol. Microbiol. 67, 402–410. doi: 10.1099/ijsem.0.001643
Hallen, A., Jamie, J., and Cooper, A. (2013). Lysine metabolism in mammalian brain: An update on the importance of recent discoveries. Amino Acids 45, 1249–1272. doi: 10.1007/s00726-013-1590-1
Hänninen, A., Toivonen, R., Pöysti, S., Belzer, C., Plovier, H., Ouwerkerk, J., et al. (2018). Akkermansia muciniphila induces gut microbiota remodelling and controls islet autoimmunity in NOD mice. Gut 67, 1445–1453. doi: 10.1136/gutjnl-2017-314508
Hernandez-Baixauli, J., Chomiciute, G., Tracey, H., Mora, I., Cortés-Espinar, A., Ávila-Román, J., et al. (2024). Exploring metabolic and gut microbiome responses to paraquat administration in male wistar rats: Implications for oxidative stress. Antioxidants 13:67. doi: 10.3390/antiox13010067
Hicks, A., Lee, K., Couto-Rodriguez, M., Patel, J., Sinha, R., Guo, C., et al. (2018). Gut microbiomes of wild great apes fluctuate seasonally in response to diet. Nat. Commun. 9:1786. doi: 10.1038/s41467-018-04204-w
Hodkinson, I. (2005). Terrestrial insects along elevation gradients: Species and community responses to altitude. Biol. Rev. 80, 489–513.
Huang, P., Yang, Y., Wang, C., Hsiao, P., Chiang, H., and Chen, T. (2021). Increase in Akkermansiaceaein gut microbiota of prostate cancer-bearing mice. Int. J. Mol. Sci. 22:9626.
Ibáñez, A., Bletz, M., Quezada, G., Geffers, R., Jarek, M., Vences, M., et al. (2021). No impact of a short-term climatic “El Niño” fluctuation on gut microbial diversity in populations of the Galápagos marine iguana (Amblyrhynchus cristatus). Sci. Nat. 108, 1–15.
Jiang, H., Ma, J., Li, J., Zhang, X., Li, L., He, N., et al. (2017). Diets alter the gut microbiome of crocodile lizards. Front. Microbiol. 8:2073. doi: 10.3389/fmicb.2017.02073
Jonas, Z., Jackson, M., Gabi, K., Massimo, M., Tao, L., Amalio, T., et al. (2018). The fecal metabolome as a functional readout of the gut microbiome. Nat. Genet. 50, 790–795.
Karl, J., Berryman, C., Young, A., Radcliffe, P., Branck, T., Pantoja-Feliciano, I., et al. (2018). Associations between the gut microbiota and host responses to high altitude. Am. J. Physiol. Gastrointestinal Liver Physiol. 315, G1003–G1015.
Karu, N., Deng, L., Slae, M., Guo, A., Sajed, T., Huynh, H., et al. (2018). A review on human fecal metabolomics: Methods, applications and the human fecal metabolome database. Anal. Chim. Acta 1030, 1–24.
Kelly, B., Gross, R., Bittinger, K., Sherrill-Mix, S., Lewis, J., Collman, R., et al. (2015). Power and sample-size estimation for microbiome studies using pairwise distances and PERMANOVA. Bioinformatics 31, 2461–2468. doi: 10.1093/bioinformatics/btv183
Kim, J., Han, K., Han, Y., Kang, N., Shin, T., Park, H., et al. (2021). Microbiome markers of pancreatic cancer based on bacteria-derived extracellular vesicles acquired from blood samples: A retrospective propensity score matching analysis. Biology 10:219. doi: 10.3390/biology10030219
Koch, F., Reyer, H., Görs, S., Hansen, C., Wimmers, K., and Kuhla, B. (2024). Heat stress and feeding effects on the mucosa-associated and digesta microbiome and their relationship to plasma and digesta fluid metabolites in the jejunum of dairy cows. J. Dairy Sci. 107, 5162–5177. doi: 10.3168/jds.2023-24242
Lankadurai, B., Nagato, E., and Simpson, M. (2013). Environmental metabolomics: An emerging approach to study organism responses to environmental stressors. Environ. Rev. 21, 180–205. doi: 10.1016/j.ecoenv.2024.117319
Lapébie, P., Lombard, V., Drula, E., Terrapon, N., and Henrissat, B. (2019). Bacteroidetes use thousands of enzyme combinations to break down glycans. Nat. Commun. 10:2043. doi: 10.1038/s41467-019-10068-5
Lara, C., Romina, M., Barbara, F., and Nicolò, M. (2017). Impact of Omega-3 fatty acids on the gut microbiota. Int. J. Mol. Sci. 18:2645.
Laranjo, M., Alexandre, A., and Oliveira, S. (2014). Legume growth-promoting rhizobia: An overview on the Mesorhizobium genus. Microbiol. Res. 169, 2–17. doi: 10.1016/j.micres.2013.09.012
Lee, S., Yoon, S., Jung, Y., Kim, N., Min, U., Chun, J., et al. (2020). Emotional well-being and gut microbiome profiles by enterotype. Sci. Rep. 10:20736.
Li, W., Freudenberg, J., Suh, Y., and Yang, Y. (2014). Using volcano plots and regularized-chi statistics in genetic association studies. Comput. Biol. Chem. 48, 77–83. doi: 10.1016/j.compbiolchem.2013.02.003
Lin, X., Hu, T., Wu, Z., Li, L., Wang, Y., Wen, D., et al. (2024). Isolation of potentially novel species expands the genomic and functional diversity of Lachnospiraceae. iMeta 3:e174.
Ling, Z., Yufen, H., Lin, L., Wei, Y., Tao, H., Zesi, L., et al. (2018). Saturated long-chain fatty acid-producing bacteria contribute to enhanced colonic motility in rats. Microbiome 6:107. doi: 10.1186/s40168-018-0492-6
Littleford-Colquhoun, B. L., Weyrich, L. S., Jackson, N., and Frere, C. H. (2019). City life alters the gut microbiome and stable isotope profiling of the eastern water dragon (Intellagama lesueurii). Mol. Ecol. 28, 4592–4607. doi: 10.1111/mec.15240
Liu, D., Chen, D., Xiao, J., Wang, W., Zhang, L., Peng, H., et al. (2024). High-altitude-induced alterations in intestinal microbiota. Front. Microbiol. 15:1369627. doi: 10.3389/fmicb.2024.1369627
Liu, Y., Chen, W., Zhang, S., Zhu, X., Wu, H., Meng, Q., et al. (2024). N-acetyl-l-methionine dietary supplementation improves meat quality by oxidative stability of finishing Angus heifers. Meat Sci. 214:109499. doi: 10.1016/j.meatsci.2024.109499
Lück, R., and Deppenmeier, U. (2022). Genetic tools for the redirection of the central carbon flow towards the production of lactate in the human gut bacterium Phocaeicola (Bacteroides) vulgatus. Appl. Microbiol. Biotechnol. 106, 1211–1225. doi: 10.1007/s00253-022-11777-6
MacMillan, H. (2019). Dissecting cause from consequence: A systematic approach to thermal limits. J. Exp. Biol. 222:jeb191593.
Marden, J. H. (2013). Nature’s inordinate fondness for metabolic enzymes: Why metabolic enzyme loci are so frequently targets of selection. Mol. Ecol. 22, 5743–5764. doi: 10.1111/mec.12534
Maritan, E., Quagliariello, A., Frago, E., Patarnello, T., and Martino, M. (2024). The role of animal hosts in shaping gut microbiome variation. Philos. Trans. R. Soc. B. 379:20230071.
Mazzucco, R., and Schlötterer, C. (2021). Long-term gut microbiome dynamics in Drosophila melanogaster reveal environment-specific associations between bacterial taxa at the family level. Proc. R. Soc. B. 288:20212193. doi: 10.1098/rspb.2021.2193
Montoya-Ciriaco, N., Gómez-Acata, S., Muñoz-Arenas, L., Dendooven, L., Estrada-Torres, A., Díaz de la Vega-Pérez, A. H., et al. (2020). Dietary effects on gut microbiota of the mesquite lizard Sceloporus grammicus (Wiegmann, 1828) across different altitudes. Microbiome 8, 1–19. doi: 10.1186/s40168-020-0783-6
Murakami, T., Segawa, T., Takeuchi, N., Barcaza Sepúlveda, G., Labarca, P., Kohshima, S., et al. (2018). Metagenomic analyses highlight the symbiotic association between the glacier stonefly Andiperla willinki and its bacterial gut community. Environ. Microbiol. 20, 4170–4183. doi: 10.1111/1462-2920.14420
Murray, A., and Montgomery, H. (2014). How wasting is saving: Weight loss at altitude might result from an evolutionary adaptation. Bioessays 36, 721–729. doi: 10.1002/bies.201400042
Niu, Y., Zhang, X., Xu, T., Li, X., Zhang, H., Wu, A., et al. (2022). Physiological and biochemical adaptations to high altitude in Tibetan frogs. Nanorana parkeri. Front. Physiol. 13:942037. doi: 10.3389/fphys.2022.942037
Paaijmans, K., Heinig, R., Seliga, R., Blanford, J., Blanford, S., Murdock, C., et al. (2013). Temperature variation makes ectotherms more sensitive to climate change. Glob. Change Biol. 19, 2373–2380.
Pascale, A., Marchesi, N., Marelli, C., Coppola, A., Luzi, L., Govoni, S., et al. (2018). Microbiota and metabolic diseases. Endocrine 61, 357–371. doi: 10.1016/j.molmet.2016.03.004
Plasman, M., McCue, M., Reynoso, V., Terblanche, J., and Clusella-Trullas, S. (2019). Environmental temperature alters the overall digestive energetics and differentially affects dietary protein and lipid use in a lizard. J. Exp. Biol. 222:jeb194480. doi: 10.1242/jeb.194480
Ran, J., Li, Y., Yuan, C., and Shi, L. (2023). Age and growth of Phrynocephalus axillaris (Agamidae) by skeletochronology. Chin. J. Ecol. 337, 491–500.
Raza, M., Wang, Y., Cai, Z., Bai, S., Yao, Z., Awan, U., et al. (2020). Gut microbiota promotes host resistance to low-temperature stress by stimulating its arginine and proline metabolism pathway in adult Bactrocera dorsalis. PLoS Pathogens 16:1008441. doi: 10.1371/journal.ppat.1008441
Reguera, S., Zamora-Camacho, F., Melero, E., Garcia-Mesa, S., Trenzado, C., Cabrerizo, M., et al. (2015). Ultraviolet radiation does not increase oxidative stress in the lizard Psammodromus algirus along an elevational gradient. Comp. Biochem. Physiol. Part A: Mol. Integr. Physiol. 183, 20–26.
Ríus, A. (2019). Invited review: Adaptations of protein and amino acid metabolism to heat stress in dairy cows and other livestock species. Appl. Anim. Sci. 35, 39–48.
Rooks, M., and Garrett, W. (2016). Gut microbiota, metabolites and host immunity. Nat. Rev. Immunol. 16, 341–352.
Roopchand, D., Carmody, R., Kuhn, P., Moskal, K., Rojas-Silva, P., Turnbaugh, P., et al. (2015). Dietary polyphenols promote growth of the gut bacterium Akkermansia muciniphila and attenuate high-fat diet–induced metabolic syndrome. Diabetes 64, 2847–2858. doi: 10.2337/db14-1916
Sam, V., Mojgan, M., Vidya, V., Yew, T., Martin, D., Tapani, S., et al. (2012). Lipocalin prostaglandin D synthase and PPARγ2 coordinate to regulate carbohydrate and lipid metabolism in vivo. PLoS One 7:e39512. doi: 10.1371/journal.pone.0039512
Sapozhnikova, Y., and Nuñez, A. (2022). Non-targeted analysis with liquid chromatography-high resolution mass spectrometry for the identification of food packaging migrants. J. Chromatogr. A. 1676, 463215.
Schaefer, L. (2014). Complexity of danger: The diverse nature of damage-associated molecular patterns. J. Biol. Chem. 289, 35237–35245.
Schloss, P., Gevers, D., and Westcott, S. (2011). Reducing the effects of PCR amplification and sequencing artifacts on 16S rRNA-based studies. PLoS One 6:e27310. doi: 10.1371/journal.pone.0027310
Schramm, B., Gudowska, A., Antoł, A., Labecka, A., Bauchinger, U., Kozłowski, J., et al. (2018). Effects of fat and exoskeletal mass on the mass scaling of metabolism in Carabidae beetles. J. Insect Physiol. 106, 232–238. doi: 10.1016/j.jinsphys.2017.10.002
Seebacher, F. (2018). The evolution of metabolic regulation in animals. Comp. Biochem. Physiol. Part B: Biochem. Mol. Biol. 224, 195–203.
Segata, N., Izard, J., Waldron, L., Gevers, D., Miropolsky, L., Garrett, W., et al. (2011). Metagenomic biomarker discovery and explanation. Genome Biol. 12, 1–18.
Serén, N., Megía-Palma, R., Simèiè, T., Krofel, M., Guarino, F. M., Pinho, C., et al. (2023). Functional responses in a lizard along a 3.5-km altitudinal gradient. J. Biogeogr. 50, 2042–2056.
Shealy, N., Yoo, W., and Byndloss, M. (2021). Colonization resistance: Metabolic warfare as a strategy against pathogenic Enterobacteriaceae. Curr. Opin. Microbiol. 64, 82–90. doi: 10.1016/j.mib.2021.09.014
Strati, F., Cavalieri, D., Albanese, D., De Felice, C., Donati, C., Hayek, J., et al. (2017). New evidences on the altered gut microbiota in autism spectrum disorders. Microbiome 5:24.
Sun, H., Chen, F., Zheng, W., Huang, Y., Peng, H., Hao, H., et al. (2024). Impact of captivity and natural habitats on gut microbiome in Epinephelus akaara across seasons. BMC Microbiol. 24:239. doi: 10.1186/s12866-024-03398-y
Szymañska, E., Saccenti, E., Smilde, A., and Westerhuis, J. Double-check: Validation of diagnostic statistics for PLS-DA models in metabolomics studies. Metabolomics 2011, 3–16. doi: 10.1007/s11306-011-0330-3
Tang, G., Liang, X., Yang, M., Wang, T., Chen, J., Du, W., et al. (2020). Captivity influences gut microbiota in crocodile lizards (Shinisaurus crocodilurus). Front. Microbiol. 11:550. doi: 10.3389/fmicb.2020.00550
Tian, Z., Pu, H., Cai, D., Luo, G., Zhao, L., Li, K., et al. (2022). Characterization of the bacterial microbiota in different gut and oral compartments of splendid japalure (Japalura sensu lato). BMC Veterinary Res. 18:205. doi: 10.1186/s12917-022-03300-w
Tu, W., Lu, W., Gu, J., and Lou, A. R. (2024). The species diversity and phylogenetic structure patterns of desert plant communities in the Turpan-Hami Region, Xinjiang. Glob. Ecol. Conserv. 55:e03239.
Uparse, R. (2013). Highly accurate OTU sequences from microbial amplicon reads. Nat. Methods 10, 996–998. doi: 10.1038/nmeth.2604
Vacca, M., Celano, G., Calabrese, F., Portincasa, P., and Angelis, M. (2020). The controversial role of human gut lachnospiraceae. Microorganisms 8:573.
Wang, J., Linnenbrink, M., Künzel, S., Fernandes, R., Nadeau, M., Rosenstiel, P., et al. (2014). Dietary history contributes to enterotype-like clustering and functional metagenomic content in the intestinal microbiome of wild mice. Proc. Natl. Acad. Sci. 111, E2703–E2710. doi: 10.1073/pnas.1402342111
Wang, Q., Garrity, G., Tiedje, J., and Cole, J. (2007). Naive Bayesian classifier for rapid assignment of rRNA sequences into the new bacterial taxonomy. Appl. Environ. Microbiol. 73, 5261–5267. doi: 10.1128/AEM.00062-07
Wang, X., Li, S., Li, L., Zhang, F., and Sun, B. (2019). Thermal-physiological strategies underlying the sympatric occurrence of three desert lizard species. Asian Herpetol. Res. 10, 190–196.
Wang, X., Wu, X., Shang, Y., Gao, Y., Li, Y., Wei, Q., et al. (2022). High-altitude drives the convergent evolution of alpha diversity and Indicator microbiota in the gut microbiomes of ungulates. Front. Microbiol. 13:953234. doi: 10.3389/fmicb.2022.953234
Want, E., O”Maille, G., Smith, C. A., Brandon, T. R., Uritboonthai, W., and Qin, C. (2006). Solvent-dependent metabolite distribution, clustering, and protein extraction for serum profiling with mass spectrometry. Anal. Chem. 78, 743–752. doi: 10.1021/ac051312t
Wen, C., Li, S., Wang, J., Zhu, Y., Zong, X., Wang, Y., et al. (2021). Heat stress alters the intestinal microbiota and metabolomic profiles in mice. Front. Microbiol. 12:706772. doi: 10.3389/fmicb.2021.706772
Xie, H., Jiang, L., and Zhao, W. (2023). Genetic diversity analysis of Phrynocephalus axillaris based on nuclear gene and microsatellite. J. Anhui Agric. Sci. 51, 81–87.
Yang, C., Tingting, F., Lan, X., Zhang, Y., Nneji, L., Murphy, R., et al. (2019). Comparative skin histology of frogs reveals high-elevation adaptation of the Tibetan Nanorana parkeri. Asian Herpetol. Res. 7, 79–85.
Yang, J., Liu, W., Han, X., Hao, X., Yao, Q., and Du, W. (2024). Gut microbiota modulation enhances the immune capacity of lizards under climate warming. Microbiome 12:37. doi: 10.1186/s40168-023-01736-2
Youngblut, N., Reischer, G., Walters, W., Schuster, N., and Farnleitner, A. (2019). Host diet and evolutionary history explain different aspects of gut microbiome diversity among vertebrate clades. Nat. Commun. 10:2200. doi: 10.1038/s41467-019-10191-3
Yuan, M., Breitkopf, S., Yang, X., and Asara, J. (2012). A positive/negative ion-switching, targeted mass spectrometry-based metabolomics platform for bodily fluids, cells, and fresh and fixed tissue. Nat. Protoc. 7, 872–881. doi: 10.1038/nprot.2012.024
Zamora-Camacho, F., Reguera, S., Moreno-Rueda, G., and Pleguezuelos, J. (2013). Patterns of seasonal activity in a Mediterranean lizard along a 2200 m altitudinal gradient. J. Thermal Biol. 38, 64–69.
Zhang, Q., Xia, L., He, J., Wu, Y., Fu, J., and Yang, Q. (2010). Comparison of phylogeographic structure and population history of two Phrynocephalus species in the Tarim Basin and adjacent areas. Mol. Phylogenet. Evol. 57, 1091–1104. doi: 10.1016/j.ympev.2010.10.003
Zhang, T., Li, Q., Cheng, L., Buch, H., and Zhang, F. (2019). Akkermansia muciniphila is a promising probiotic. Microb. Biotechnol. 12, 1109–1125.
Zhang, W., Li, N., Tang, X., Liu, N., and Zhao, W. (2018). Changes in intestinal microbiota across an altitudinal gradient in the lizard Phrynocephalus vlangalii. Ecol. Evol. 8, 4695–4703. doi: 10.1002/ece3.4029
Zhang, X., Men, S., Jia, L., Tang, X., Storey, K., Niu, Y., et al. (2023). Comparative metabolomics analysis reveals high-altitude adaptations in a toad-headed viviparous lizard, Phrynocephalus vlangalii. Front. Zool. 20:35. doi: 10.1186/s12983-023-00513-z
Zhao, E., and Zhao, K. (1999). Fauna Sinica, Reptilian volume2, Squamata. Beijing Lacertilia: Beijing Science Press.
Zhong, C., Hu, G., Hu, C., Xu, C., Zhang, Z., and Ning, K. (2024). Comparative genomics analysis reveals genetic characteristics and nitrogen fixation profile of Bradyrhizobium. iScience 27:108948. doi: 10.1016/j.isci.2024.108948
Keywords: Phrynocephalus axillaris, altitude gradients, gut microbiome, 16S rRNA, LC-MS metabolomics, plasticity
Citation: Du J, Zheng P, Gao W, Liang Q, Leng L and Shi L (2025) All roads lead to Rome: the plasticity of gut microbiome drives the extensive adaptation of the Yarkand toad-headed agama (Phrynocephalus axillaris) to different altitudes. Front. Microbiol. 15:1501684. doi: 10.3389/fmicb.2024.1501684
Received: 25 September 2024; Accepted: 24 December 2024;
Published: 08 January 2025.
Edited by:
Huan Li, Lanzhou University, ChinaReviewed by:
Ferhat Matur, Dokuz Eylül University, TürkiyeYang Wang, Hebei Normal University, China
Efe Sezgin, Izmir Institute of Technology, Türkiye
Xiaoliang Hu, Yibin University, China
Copyright © 2025 Du, Zheng, Gao, Liang, Leng and Shi. This is an open-access article distributed under the terms of the Creative Commons Attribution License (CC BY). The use, distribution or reproduction in other forums is permitted, provided the original author(s) and the copyright owner(s) are credited and that the original publication in this journal is cited, in accordance with accepted academic practice. No use, distribution or reproduction is permitted which does not comply with these terms.
*Correspondence: Lei Shi, bGVpc0B4amF1LmVkdS5jbg==