- 1School of Geography and Environment, Jiangxi Normal University, Nanchang, China
- 2Key Laboratory of Poyang Lake Wetland and Watershed Research, Ministry of Education, Jiangxi Normal University, Nanchang, China
- 3School of History Culture and Tourism, Longnan Normal University, Longnan, China
Bacterial communities play a crucial role in maintaining the stability of river ecosystems and driving biogeochemical cycling, exhibiting high sensitivity to environmental change. However, understanding the spatial scale effects and assembly mechanisms of riverine bacterial communities under distinct anthropogenic disturbances remains a challenge. Here, we investigated bacterial communities across three distinct watersheds [i.e., intensive urban (UW), intensive agricultural (AW), and natural (NW)] in both dry and wet seasons. We explored biogeographic patterns of bacterial communities and the influence of landscape patterns at multi-spatial scales and water chemistry on bacterial communities. Results showed that α diversity was significantly lower in UW and AW compared to NW, particularly in the dry season. A gradient of β diversity with NW > UW > AW was observed across both seasons (p < 0.05). Pseudomonadota, Bacteroidota, and Actinobacteriota were the most abundant phyla across all watersheds, with specific taxa enriched in each watershed (i.e., the class Actinobacteria was significant enrichment in UW and AW, and Clostridia in NW). The influence of landscape patterns on bacterial communities was significantly lower in human-disturbed watersheds, particularly in UW, where this influence also varied slightly from near riparian buffers to sub-watershed. Homogeneous selection and drift jointly dominated the bacterial community assembly across all watersheds, with homogeneous selection exhibiting a greater influence in UW and AW. Landscape patterns explained less variance in bacterial communities in UW and AW than in NW, and more variance was explained by water chemistry (particularly in UW). These suggest that the stronger influence of species sorting in UW and AW was driven by more allochthonous inputs of water chemistry (greater environmental stress). These findings provide a theoretical foundation for a deeper understanding of riverine bacterial community structure, spatial scale effects, and ecological management under different anthropogenic activities.
1 Introduction
Rivers, serving as primary water “sources” for industrial-agricultural production and “sinks” for nutrients and organic matter, play pivotal roles in economic and social development, public health, and environmental protection (Akasaka et al., 2010). Within these ecosystems, bacterial communities play a fundamental role in driving biogeochemical cycles, energy flow, and ecological functioning (Newton et al., 2011). Riverine bacterial communities are highly sensitive to environmental change and are shaped by a complex interplay of natural and anthropogenic factors (Staley et al., 2013; Birrer et al., 2021). The assembly of bacterial communities is influenced by local environment (local factors) and larger spatial regional environment (regional factors) (Lindström and Langenheder, 2012). Allochthonous inputs, nutrient levels (e.g., N, P, C), heavy metals (e.g., Fe, Pb, Cr), and toxic substances can exert strong selective pressures on these bacterial communities (Ajani et al., 2023; Mohapatra et al., 2023). However, stochastic processes such as dispersal limitation, spatial factors, and ecological drift also contribute significantly to shaping bacterial community structure (Wang et al., 2019; Yi et al., 2022; Zhang T. et al., 2022).
The effects of landscape patterns on riverine ecosystems exhibit discernible differences in different anthropogenic activities (Fasching et al., 2020; Zhang Y. et al., 2022). Landscape patterns include landscape compositions (i.e., the proportion of landscape types) and landscape configurations (i.e., the spatial distribution of landscape types) (Xu et al., 2021). With the rapid development of society, anthropogenic activities have significantly changed landscape patterns (urban and agricultural intensification) (Mouri et al., 2011). Intensive urban watershed is often characterized by stronger anthropogenic activities, accompanied by significant substantial industrial production, residential sewage discharge, exogenous bacterial input, and increased impervious surfaces (Samadi Todar et al., 2021; Zhang L. et al., 2021; Murphy et al., 2024). Intensive agricultural watershed often has more agricultural activities, leading to the introduction of various chemical substances (i.e., fertilizers and pesticides) into rivers during the agricultural production process (Allan, 2004; Liu et al., 2024). Intensive urban or agricultural land use leads to an imbalance in the proportion of “source” and “sink” landscapes and changes in spatial configuration, ultimately resulting in the input difference of exogenous bacteria or water chemistry (Germer et al., 2010; Hobbie et al., 2017). Water chemistry acts as a key driver of species sorting, affecting bacterial growth and competition (Guéguen et al., 2004; Gupta et al., 2023; Menéndez-Serra et al., 2023). For example, nitrogen, carbon, and organic matter in river can provide nutrients for microbial growth, while harmful substances such as heavy metals exert stress on microbial growth (Zhang J. et al., 2021, 2023; Shu et al., 2023). Additionally, water chemistry may also influence mass effects via the collective input of allochthonous bacteria (García-Armisen et al., 2014; Staley et al., 2014; Wang et al., 2019). However, the differences in the assembly mechanisms of riverine bacterial communities in intensive urban and agricultural watersheds remain poorly understood (Wang et al., 2019; Sanfilippo et al., 2021; Wu et al., 2022).
Landscape patterns exert their influence on riverine ecosystems across a range of spatial scales, with impacts observed at both local and distant reaches (Paul and Meyer, 2001). Previous studies have examined the influence of near-distance buffer zones and sub-watershed on riverine ecology, and explored whether there are patterns of distance decay across different buffer zones (Zhang et al., 2019; Wu et al., 2021). There is still no consensus on the critical scale of landscape pattern management (Laudon and Sponseller, 2018). For example, some studies emphasizing the importance of the sub-watershed scale (Wang et al., 2023; Xu et al., 2023), and others highlighting the role of riparian buffer zones (Wu and Lu, 2021; Shu et al., 2022). Whether this discrepancy arises due to inherent differences in landscape patterns in different anthropogenically disturbed watersheds remains unclear (Gao et al., 2022; Wu et al., 2022). Most of these studies only analyzed the spatial scale in a single watershed, and the conclusions are less comparable due to differences in climatic environment, watershed size, and anthropogenic disturbances (Liu et al., 2021; Zhu et al., 2022). Further comparative research is needed to elucidate potential distinctions in the spatial effects of landscape patterns on bacterial community structure within watersheds subject to different anthropogenic pressures.
This study employed a comparative analysis using high-throughput sequencing to investigate bacterial communities in three intensive watersheds characterized by distinct anthropogenic dominant disturbances [urban, agricultural, and natural (forest)] within a subtropical monsoon zone. Homogeneous selection, heterogeneous selection, and species sorting are mainly effects of the local environment, while homogenizing dispersal, dispersal limitation, and mass effects are mainly effect of the larger spatial regional (Leibold et al., 2004; Stegen et al., 2013; Zhou and Ning, 2017). So, we hypothesized that homogeneous selection and heterogeneous selection are analogous to species sorting, and homogenizing dispersal and dispersal limitation are analogous to mass effects. To disentangle these processes, we considered the unique explanatory ratio of landscape patterns as indicative of mass effects, and the unique explanatory ratio of water chemistry as indicative of species sorting (Figure 1). Our objectives of this study are: (i) to identify the spatial scale effects on riverine bacterial communities under different anthropogenic disturbances, and (ii) to elucidate the distinctions in bacterial community assembly processes and their underlying mechanisms within watersheds experiencing varying anthropogenic pressures.
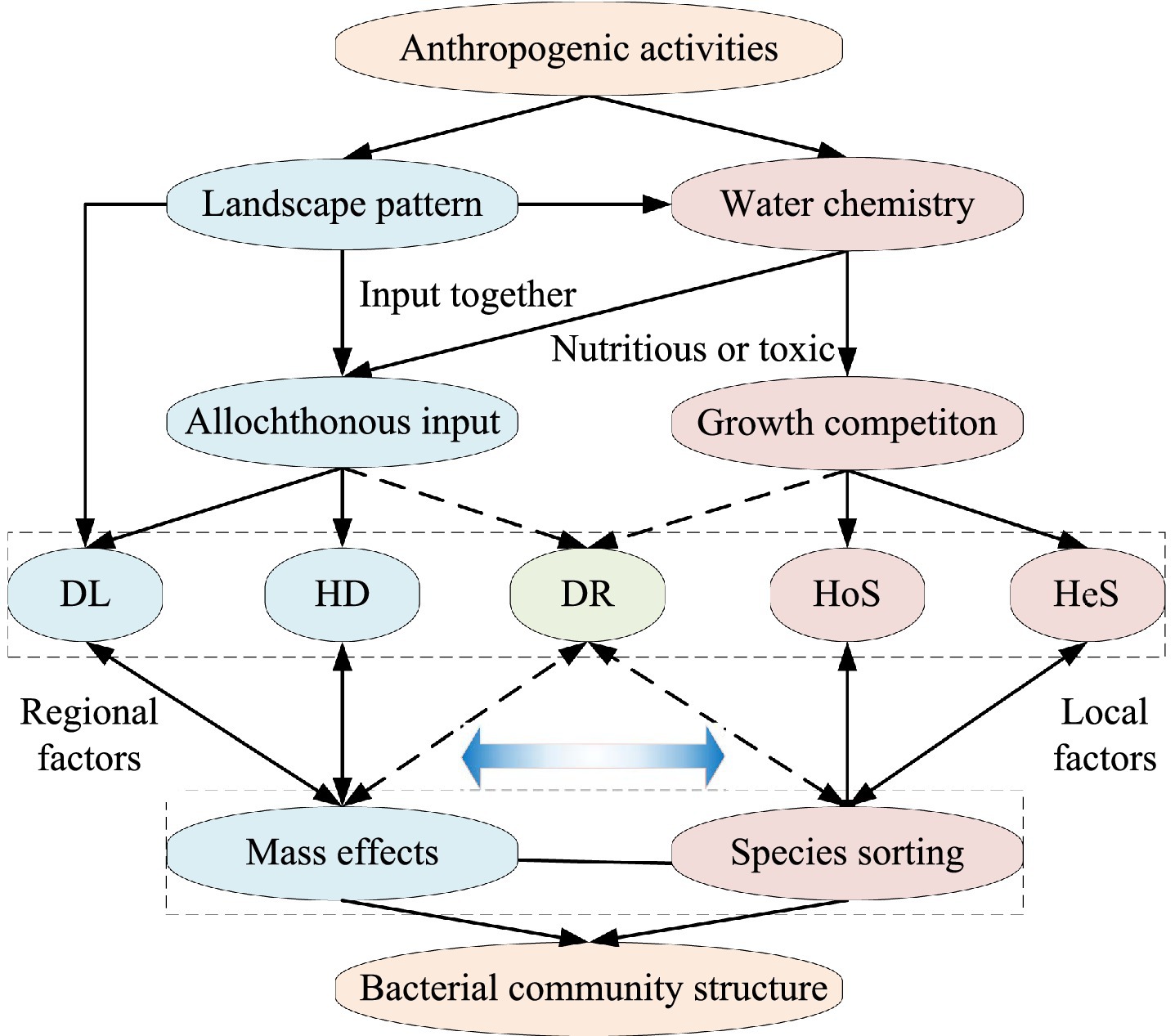
Figure 1. Schematic representation of the influence of landscape pattern and water chemistry on the bacterial community in river water disturbed by different anthropogenic activities. DL, dispersal limitation; HD, homogenizing dispersal; DR, drift; HoS, homogeneous selection; HeS, heterogeneous selection. Gradient blue arrow indicate mechanism-driven strength (Darker color means stronger). Dotted arrows indicate weaker relationships.
2 Materials and methods
2.1 Study area
The study was conducted within the Yuan River watershed, a secondary tributary of the Yangtze River located in the Poyang Lake basin, Jiangxi Province, China (Supplementary Figure S1). Three different watersheds were selected for comparison: the Nanmiao watershed (NMH), characterized by its dominant forest cover (77.74%) and serving as a natural watershed (NW); the Meng watershed (MH), with a predominance of cropland (53.29%) representing an intensive agricultural watershed (AW); and the Kongmu watershed (KMJ) with 8.94% built-up area, passing through the industrial city of Xinyu, and thus experiencing significant influence from urban life and production, constituting an urban watershed (UW) (Supplementary Figure S2; Supplementary Tables S1, S2). The study area belongs to the subtropical monsoon humid climate region, with an average annual temperature of 17.5°C and annual average precipitation of approximately 1,583 mm, primarily concentrated from April to August. The topography of the region is dominated by hills and mountains, resulting in pronounced river catchment effects.
2.2 Sample collection and processing
A total of 25 sampling sites were established across the three watersheds, with nine sites in the UW and eight sites each in the NW and AW (Supplementary Figure S1). Water samples were collected at an approximate depth of 50 cm at 25 sites located in the center of the river channel. Each sampling was conducted after a three-day period with no significant precipitation events. In January (dry season) and July (wet season) of 2022, 50 surface water samples were collected using 1 L polyethylene bottles and transported to the laboratory for analysis transported to the laboratory for analysis at the low temperature of 0~4°C. For bacterial community analysis, water samples were filtered through 0.22 μm cellulose acetate membranes (diameter 50 mm; Xingya, China) on the same day, and the filter membranes were stored at −80°C before high-throughput sequencing conducted by Shanghai Meiji Biological Company, China. Electrical conductivity (EC), water temperature (WT), pH, and dissolved oxygen (DO) were measured in situ using an HI 98360 probe (Hanna Instruments Ltd., Italy) that was calibrated before each measurement. Water samples for water chemistry measurement were filtered through a 0.45 μm pore size cellulose acetate membrane, sealed in a sampling bottle, and stored at 0~4°C. Dissolved organic carbon (DOC) was measured using a TOC analyzer (Shimadzu TOC-L CPH, Japan), while chlorine ions (Cl−) and sulfate ions (SO42−) were determined by ion chromatography system (ICS-600). The nitrate and ammonia nitrogen (NO3−-N, NH4+-N) and total phosphorus (TP), were quantified using an automatic discontinuous analyzer (Smartchem 200 Brookfield, United States) with the Environmental Monitoring method standard of the Ministry of Ecology and Environment, PRC. The concentrations of chromium (Cr), iron (Fe), manganese (Mn), copper (Cu), cadmium (Cd), and lead (Pb) were measured by inductively coupled plasma-mass spectrometry (ICP-MS, Thermo X series II, USA). The accuracy and precision of the methods and results were checked by using the certified Standard Reference Materials (SRM-1640 and SRM-1643e of National Institute of Scientific and Technology, United States).
2.3 Landscape patterns and buffer zone division
Digital elevation model (DEM) data with a 12.5 m resolution, was obtained from the NASA Earth Science Data website.1 Utilizing the ArcSWAT module within ArcGIS, sub-watershed and riparian buffers at 1,000 m, 500 m, 300 m, and 100 m scale were extracted based on rivers and sampling points (Supplementary Figure S1). Land use data for the year 2020 were obtained from the World Cover dataset published by the European Aviation Agency, featuring a grid resolution of 10 m.2 This study based on prior research (Chen et al., 2018; Zhou et al., 2020; Sanfilippo et al., 2021) that trees and shrubs were combined as “Forest,” while grasslands and sparse vegetation were combined as “Grassland.” The categories of agricultural and built-up were maintained. In aggregate, these categories encompass in excess of 97% of the total area within each watershed. The remaining categories account for a minor proportion of the total, and thus were not considered in further analysis (Supplementary Tables S1, S2; Supplementary Figure S2). Landscape configuration indices, specifically patch density (PD) and mean Euclidean nearest neighbor distance (ENN_MN), were calculated at the landscape level using Fragstats 4.2 software to quantify landscape fragmentation and connectivity (Supplementary Tables S3, S4).
2.4 DNA extraction, 16S rRNA gene amplification and sequencing
DNA was extracted from 0.5 g of well-mixed samples using the FastDNA SPIN Kit for soil (MP BIO, Qbiogene, United States) according to the manufacturer’s instructions. The bacterial V3–V4 hypervariable regions of 16S rRNA genes were amplified using a forward primer of: 338F (5′ACTCCTACGGGAGGCAGCA-3′) and a reverse primer of: 806R (5′-GGACTACHVGGGTWTCTAAT-3′) (Castrillo et al., 2017). The V3–V4 hypervariable region has been targeted via the MiSeq platform (which can produce single-end reads of 350 bp), which can allow for more accurate and cost-effective characterizations of microbiome samples (Caporaso et al., 2012; Fouhy et al., 2016). The PCR reaction was carried out in triplicate at 94°C for 5 min with an initial denaturation, followed by denaturation at 94°C for 30 s, annealing at 52°C for 30 s, extension at 72°C for 30 cycles, and a final extension step at 72°C for 10 min. The PCR products were purified, quantified, and combined for library preparation using the NEBNext® Ultra™ DNA Library Prep Kit according to the manufacturer’s recommendations. During this step, three negative controls were used to verify no contamination during DNA extraction and PCR amplification. All PCR products were sequenced using the Illumina MiSeq platform by Shanghai Meiji Biological Company, China.
2.5 Data processing and analyses
Raw sequence data were processed using the QIIME23 (Bolyen et al., 2019), followed by denoising and filtering using DADA2 (Callahan et al., 2016). Amplicon sequence variants (ASVs) were assigned based on 100% sequence similarity to the same sample sequence. α and β diversity metrics were calculated using the “vegan” package in R. Geographical distances between sampling sites were calculated based on latitude and longitude coordinates using the ‘geosphere’ package. Data normality was assessed using the Shapiro–Wilk test, and non-parametric Kruskal-Wallis tests were employed for data that did not conform to a normal distribution (p < 0.05). The “Bioenv” function (Clarke and Ainsworth, 1993) was used to select a subset of landscape patterns and water chemistry for further analysis. To reduce distribution heteroscedasticity, all environmental variables (except pH) were natural logarithm (ln) transformed before statistical analyses.
Principal coordinates analysis (PCoA) based on Bray-Curtis distances was used to visualize differences in bacterial community structure, with significance assessed using ANOSIM tests. To relate environmental variables to specific ASVs, we performed variation partitioning (“varpart” function in R) followed by canonical correspondence analysis (CCA) using the “cca” and “anova.cca” functions in the “vegan” R package. Variance partitioning analysis (VPA) (Borcard et al., 1992), as an effective method, was further used to quantify the relative contributions of landscape patterns and water chemistry to bacterial community variations. The phylogenetic normalized stochasticity ratio (pNST) was calculated using the “NST” Package. Null model analysis was carried out using the framework of Ning et al. (2020) to classify community pairs into underlying driving forces of deterministic [e.g., homogeneous selection (HoS), heterogeneous selection (HeS)] and stochastic [e.g., homogenizing dispersal (HD), dispersal limitation (DL), and “drift” (DR)]. Null model analyzed the proportion of each construction process using the big function (limit size = 24) in the iCAMP package in R (Ning et al., 2020). Linear discriminant analysis coupled with effect size (LEfSe), LDA score (log 10), was used for identifying the taxa differences among various aggregates at the genus levels (Segata et al., 2011) and visualized at ternary plots. All statistical analyses were performed in R software (v. 4.4.1).
3 Results
3.1 Diversity and composition of the bacterial community
In both dry and wet seasons, a total of 12,932 ASVs were assigned to 2,921 species, 1,396 genera, 644 families, 367 orders, 151 classes, and 52 phyla. In the dry season, α diversity, as measured by the Chao1 richness and Shannon diversity indices, was highest at site N1 (2859.40/7.28) and lowest at sites M7 (Chao1: 301.57) and M6 (Shannon: 3.65) (Supplementary Figure S3). A clear gradient in α diversity was observed among the three watersheds, with NW exhibiting the highest values, followed by UW and then AW, with significant differences among the three watersheds (p < 0.05). In contrast, in the wet season, no significant differences in α diversity were observed among the watersheds, except for a higher Shannon diversity in AW compared to UW (p < 0.05) (Figure 2). Similarly, the highest values of Chao1 richness index and Shannon diversity index (1127.91/6.43) were found at site N1, whereas the lowest Chao1 richness index (263.21) and Shannon diversity index (4.38) were found at sites K3 and N5, respectively (Supplementary Figure S3). The Chao1 richness index of the UW and AW had significant differences between the two seasons (p < 0.05). Shannon diversity showed no significant variation across any of the watersheds (p > 0.05) (Supplementary Figure S4). Spatial patterns along the river continuum were also evident in the dry season, with significantly higher Chao1 richness and Shannon diversity in upstream reaches compared to downstream reaches (p < 0.05). However, these spatial patterns disappeared in the wet season (Supplementary Figure S5).
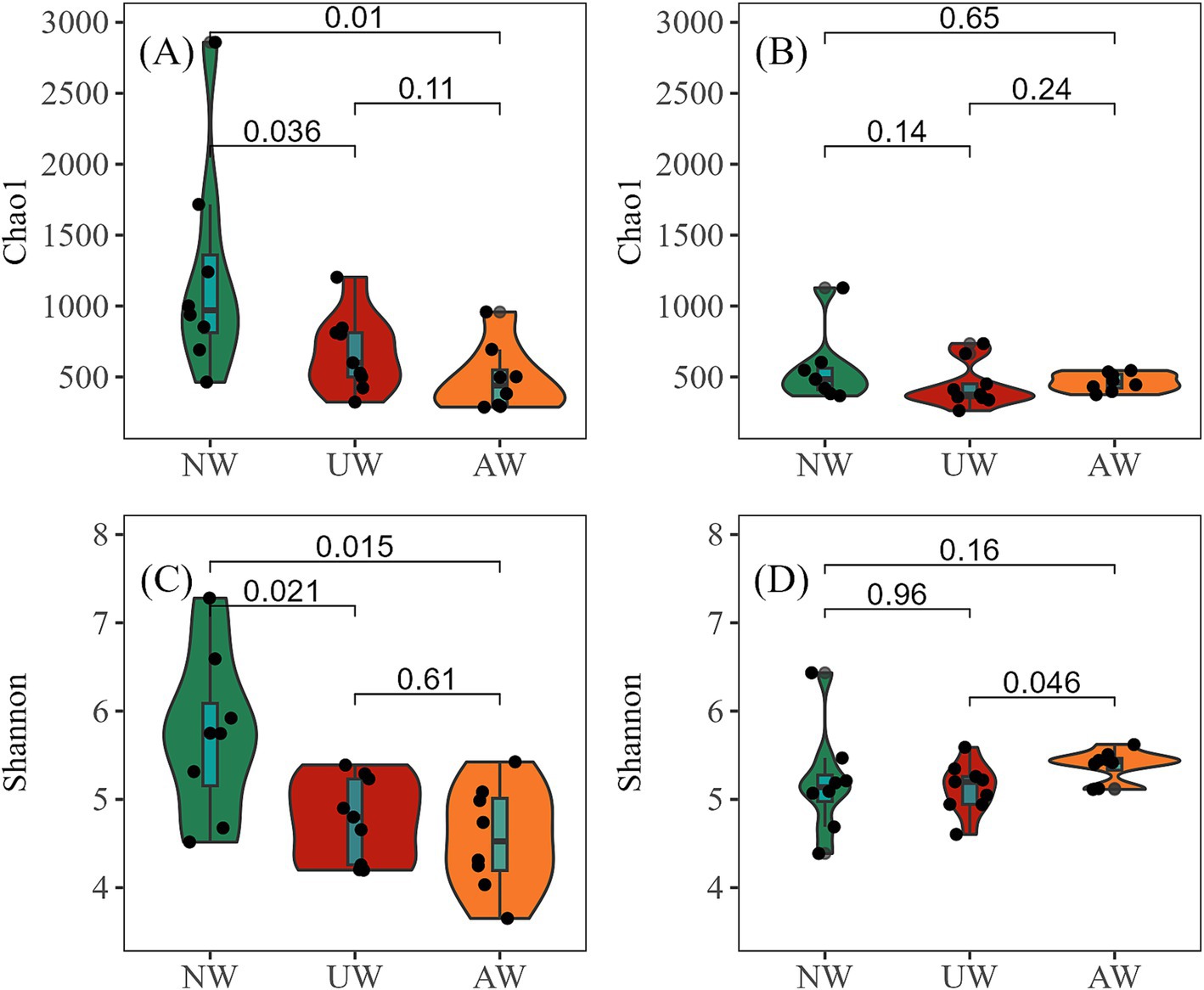
Figure 2. α diversity of bacterial communities in different watersheds (Wilcox. test). (A,B) Represent Chao1 richness in the dry and wet seasons, respectively; (C,D) represent Shannon diversity in the dry and wet seasons, respectively.
To better understand how the bacterial communities varied across space and time, we used Principal Coordinate Analysis (PCoA) and ANOSIM tests based on the relative abundance of bacteria (Figure 3). The PCoA plot separated the samples into two distinct groups based on season, a finding confirmed by the ANOSIM test (R = 0.23, p < 0.01). This indicates a significant difference in bacterial community composition between the dry and wet seasons. Furthermore, the β diversity analysis revealed significant differences among the three watersheds (AW, NW, UW) in both seasons, with even stronger separation observed in the PCoA plots and higher ANOSIM values (dry season: R = 0.36, p < 0.01; wet season: R = 0.46, p < 0.01). The distribution of sample points in the PCoA plots offered further insights. In both seasons, NW and UW samples were more scattered, suggesting greater variation in bacterial communities within these watersheds. In contrast, AW samples were more tightly clustered, particularly in the wet season, indicating greater similarity among their bacterial communities. Notably, sites N1 and K9 consistently stood out from the others in both seasons, indicating that they were significantly different from the other sites.
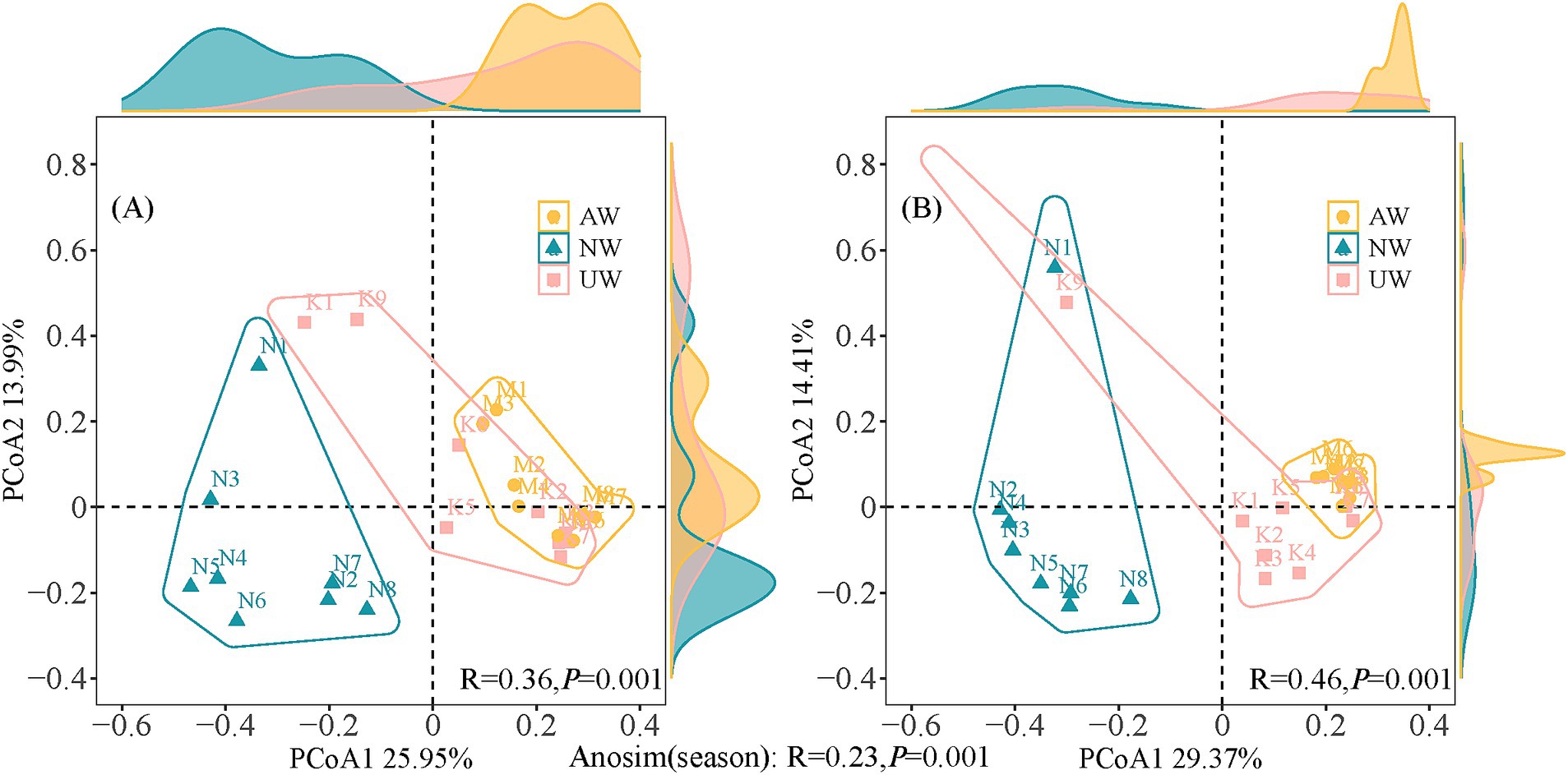
Figure 3. Principal co-ordinates analysis (PCoA) and analysis of similarity tests (ANOSIM) based on Bray-Curtis distances in the dry (A) and wet (B) seasons.
In both dry and wet seasons, Pseudomonadota was the most abundant phylum (41.46 ± 7.74%), followed by Bacteroidota (23.79 ± 11.31%), Actinobacteriota (18.73 ± 9.05%), Cyanobacteria (6.20 ± 8.41%), Bacillota (1.43 ± 1.70%), Patescibacteria (0.83 ± 1.35%), and Verrucomicrobiota (0.67 ± 0.86%) (Figure 4A). At the genus level, Limnohibatans had the highest relative abundance (9.19 ± 6.80%), followed by Flavobacterium (6.65 ± 7.67%), Pseudarcicella (5.42 ± 5.79%), hgcI_clade (5.20 ± 3.78%), unclassified_f__Comamonadaceae (5.01 ± 3.38%) (Supplementary Figure S6). The number of ASVs in each watershed was higher in the dry season than in the wet season. The order of the unique ASVs was NW (5,136/2167) > UW (1922/1156) > AW (953/599) in the dry/wet season. The total number of shared ASVs followed the order NW∩UW (935) > AW∩UW (806) > NW∩UW (658) in the dry season and AW∩UW (670) > NW∩UW (525) > NW∩UW (428) in the wet season (Supplementary Figure S7).
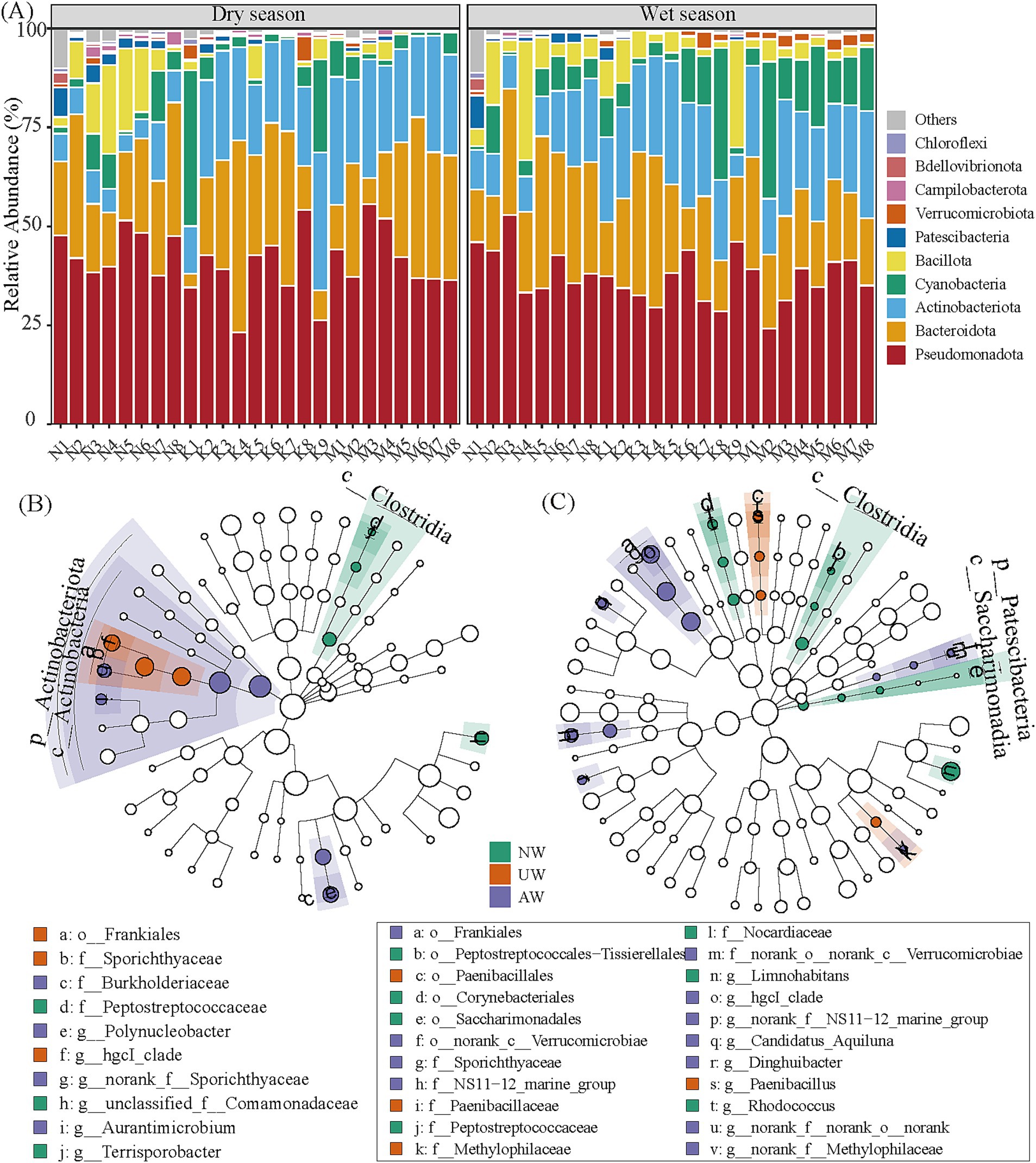
Figure 4. The relative abundances of dominant phyla in the bacterial community (A). Linear discriminant analysis (LDA) effect size (LEfSe) (LDA > 3.5, p < 0.05) to identify biomarkers in different rivers, (B,C) represent the dry season and wet seasons, respectively. The taxa with significant differences in UW, AW, and NW application are represented by red, purple, and green dots, respectively, and the taxa with no significant differences are not shown in the dendrogram. The points from the center to the outer sphere represent the level of phylum (p_), class (c_), order (o_), family (f_), and genus (g_).
To pinpoint key bacterial groups characteristic of each watershed, we employed LEfSe analysis, which identified 12 and 8 biomarkers in the dry and wet seasons, respectively (Figures 4B,C; Supplementary Table S7). In the dry season, Actinobacteriota, especially the class Actinobacteria (e.g., Frankiales and Micrococcales), thrived in both UW and AW, while Firmicutes (e.g., Clostridia and Bacilli) were enriched in NW. The wet season witnessed a shift, with Patescibacteria, Actinobacteria (Frankiales and Micrococcales), and specific orders of Bacteroidota becoming signatures of NW and AW. Meanwhile, UW was characterized by the orders Paenibacillales (class Bacilli) and Burkholderiales (class Gammaproteobacteria). These findings suggest the potential ecological roles of these bacterial groups within each watershed and across seasons.
By referencing the freshwater-specific 16S rRNA gene database (Newton et al., 2011), we classified all 12,932 identified bacterial species (ASVs) as either typical freshwater or non-freshwater bacteria. Our analysis revealed a noteworthy presence of non-freshwater bacteria, with their relative abundance ranging from 8.73 to 79.57% (average: 37.88%) in the dry season and 34.81 to 70.23% (average: 47.57%) in the wet season. This difference between seasons was statistically significant, indicating a higher proportion of non-freshwater bacteria in the wet season (Figure 5A). Among the watersheds, NW exhibited the highest relative abundance of non-freshwater bacteria in the dry season, significantly exceeding that of AW (p < 0.01) (Figure 5B). Previous studies have suggested non-freshwater bacteria primarily originate from external sources, such as the surrounding terrestrial ecosystem (Wang et al., 2019).
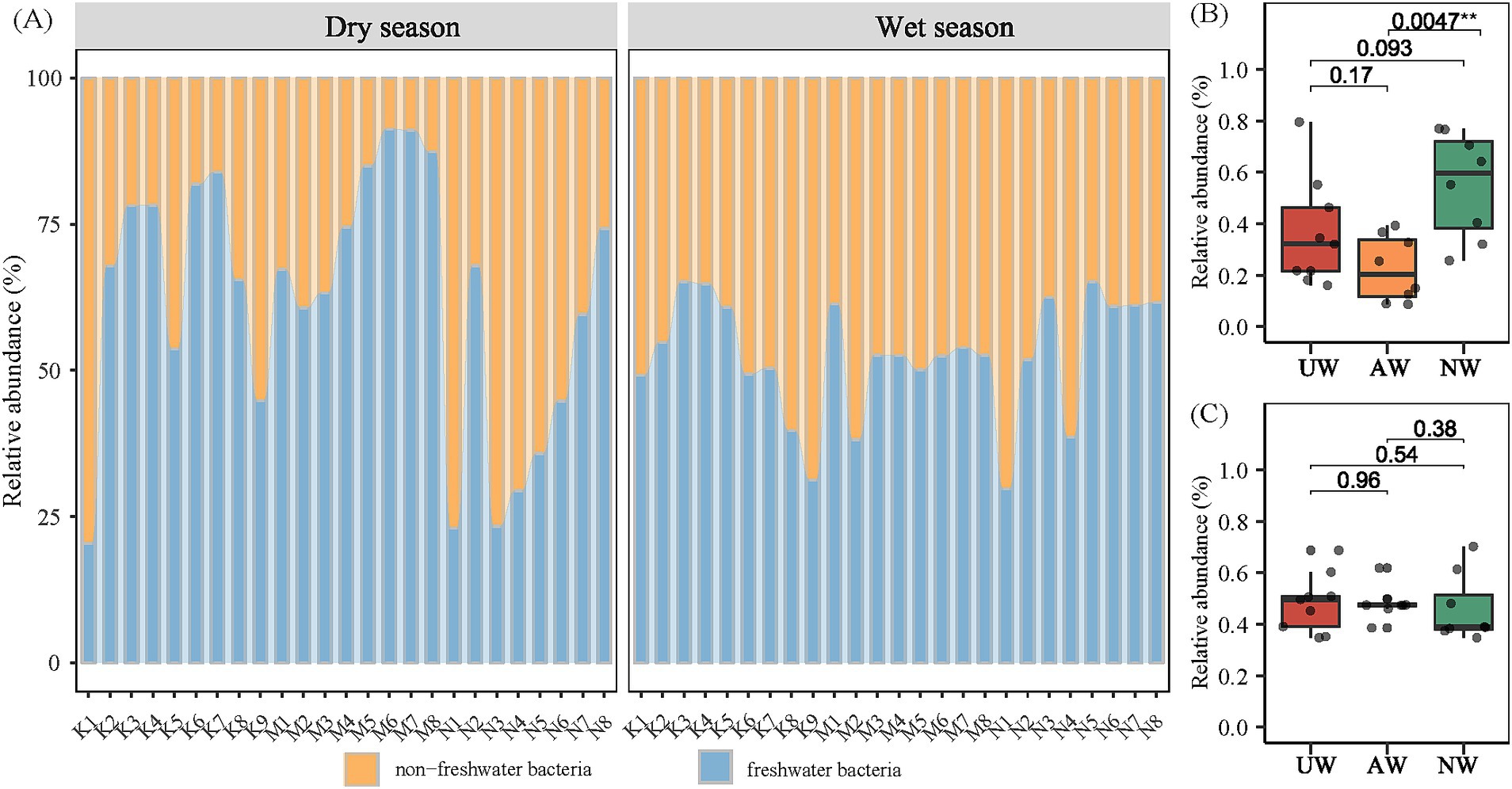
Figure 5. Proportions of sequence reads assigned to the typical freshwater lineages and non-freshwater bacteria according to the definition in the Newton freshwater database (A). Difference comparison of non-freshwater bacterial in different watersheds in the dry season (B) and the wet season (C) using Kruskal test.
3.2 Spatial scale effects on bacterial community structure
“Bioenv” analysis and the Mantel test were used to investigate the optimal spatial scale at which landscape patterns influence bacterial communities across three watersheds (Table 1). Notably, the influence of landscape patterns on bacterial community structure generally decreased from NW to AW to UW. In UW, there were slight differences in landscape patterns on bacterial communities at different spatial scales in the dry and wet seasons. For instance, the highest correlation (0.63) was observed at the 100 m riparian buffer zone in UW in the dry season, followed by the 500 m and sub-watershed (0.53). The Grassland, the Built-up, and the Built-up & ENN_MN were the most significant variables influencing the bacterial community in the 100 m riparian buffer zone, 500 m riparian buffer zone, and sub-watershed, respectively. Similar trends persisted in the wet season, with Built-up & PD and Built-up & ENN_MN being most influential at the 500 m and sub-watershed scales, respectively. In AW, correlations were slightly weaker at the sub-watershed scale than at the riparian buffer, particularly in the dry season. The 500 m riparian buffer exhibited the highest correlation (0.84), with Cropland and Grassland as the main drivers of bacterial community structure. Interestingly, in the NW and AW in the wet season, the effect on bacterial communities did not differ significantly between the riparian buffer and sub-watershed scales. Grassland, Built-up, and ENN_MN were identified as the primary factors shaping the bacterial community in the NW. The comprehensive analysis showed that the landscape patterns at the 500 m riparian buffer can better explain the variations of bacterial communities across three watersheds. Consequently, landscape pattern variables at this scale were selected for subsequent analyses.
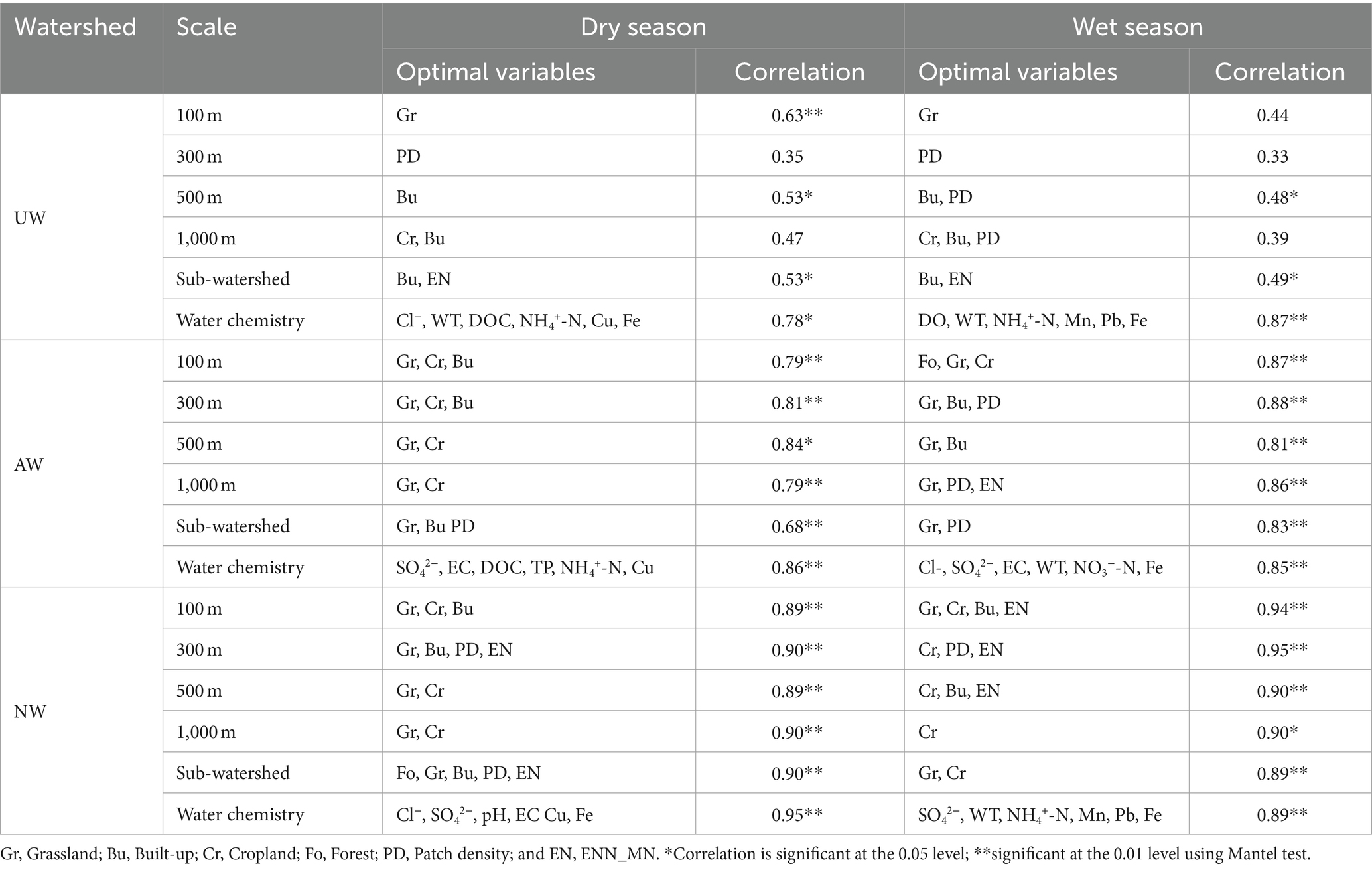
Table 1. The correlation and optimal variables of landscape patterns on the bacterial community at different spatial scales based on Bioenv.
3.3 Effects of landscape patterns and water chemistry on bacterial community structure
To evaluate the influence of landscape patterns and water chemistry on bacterial community structure, we employed linear regression models and redundancy analyses. Our results revealed distinct patterns across the three watersheds. The Euclidean distances of landscape patterns were more similar in the AW than in the NW and UW, indicating that landscape pattern structures in the AW were more similar. Moreover, a positive correlation between landscape pattern distance and Bray–Curtis dissimilarity was observed in both AW (R2 = 0.67/0.38) and NW (R2 = 0.26/0.35) in the dry/wet seasons, respectively (p < 0.05) (Figures 6A,D). A gradient of water chemistry distances was observed with UW > NW > AW in both seasons. Notably, these distances decreased significantly in the dry season compared to the wet season, particularly in the UW. In the dry season, only the NW showed a significant positive correlation between water chemistry distance and Bray–Curtis dissimilarity (R2 = 0.24, p < 0.05). However, in the wet season, both AW (R2 = 0.56) and NW (R2 = 0.48) displayed significant positive correlations (p < 0.05) (Figures 6B,E). Interestingly, the AW did not exhibit significant distance decay patterns in either season (p > 0.05) (Figures 6C,F). This further supports the observation of homogeneity in both landscape patterns and water chemistry within the AW, suggesting a more uniform environment compared to the NW and UW.
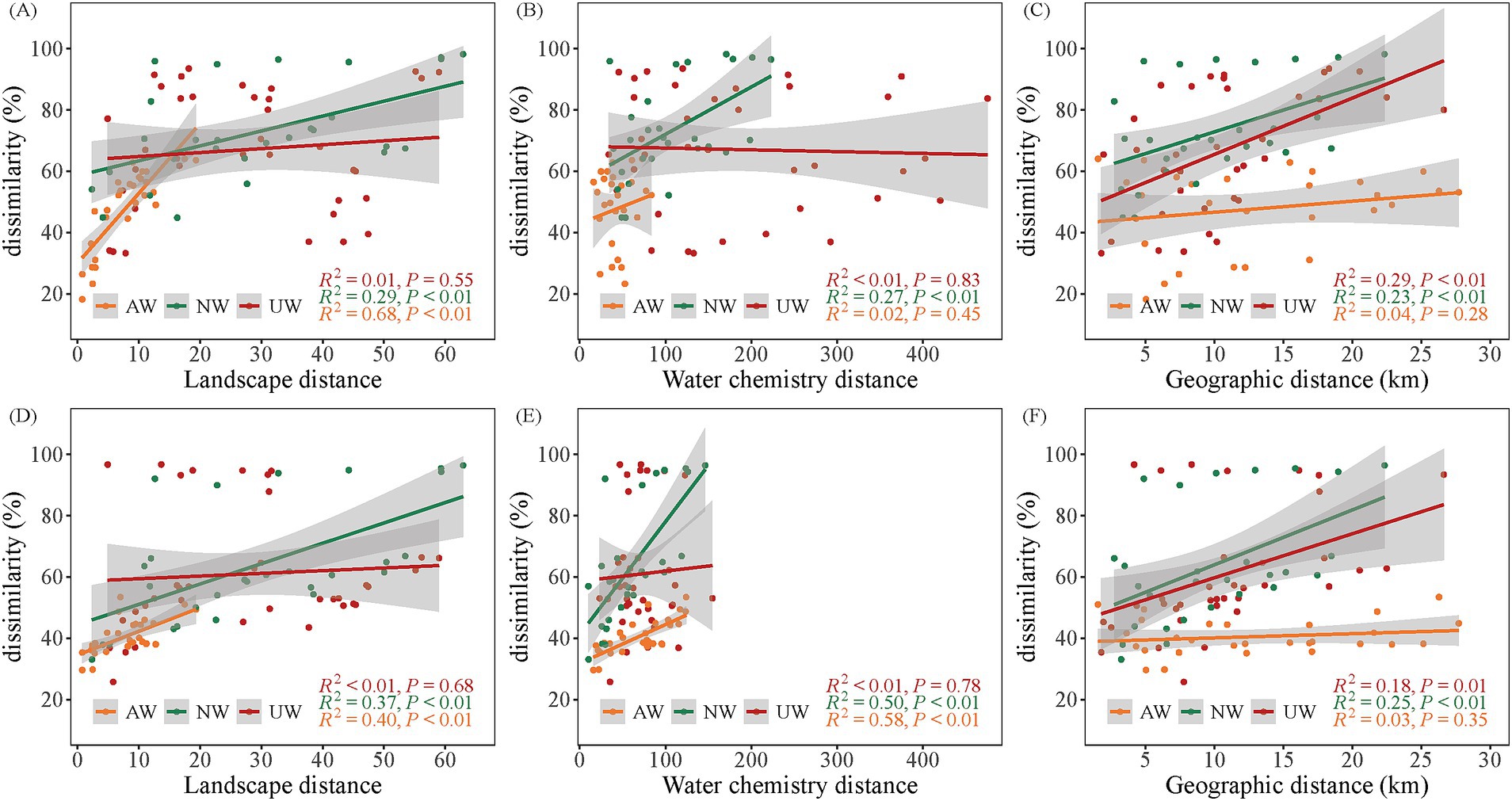
Figure 6. Relationships between β diversity (Bray–Curtis dissimilarity) and landscape patterns (Euclidean distance), water chemistry (Euclidean distance), and geographic distance. (A–C) Show linear regression relationships of landscape patterns, water chemistry and geographic distances with β diversity in the dry seasons, respectively. (D–F) Show linear regression relationships of landscape patterns, water chemistry and geographic distances with β diversity in the wet seasons, respectively.
Canonical Correspondence Analysis (CCA) revealed the influence of both landscape patterns and water chemistry on bacterial community composition across the three watersheds. The first two CCA axes explained 37.93–44.75% of the variance in community composition when considering landscape patterns (Figure 7). The highest explanatory ratio was observed in the UW in the wet season (44.75%), while the lowest was observed in the AW in the same season (37.93%) (Figures 7D1,E1). The first and second ordination axes in CCA together accounted for 38.79 ~ 45.36% of the variance in community composition in the dry and wet seasons with water chemistry, respectively. The highest and lowest explanatory rates were found in the UW (45.36%) and AW (38.79%) in the wet season (Figures 7D2,E2). In the dry season, the bacterial community was the most significant landscape pattern variable shaped by Grassland (F = 1.44, p < 0.01), Grassland (F = 2.16, p < 0.01), and Forest (F = 1.62, p < 0.01) in UW, AW, and NW, respectively. Cl− (F = 1.31, p < 0.01), SO42− (F = 1.70, p < 0.01), and Cl− (F = 1.69, p < 0.01) were the most significant water chemistry variables in UW, AW, and NW. In the wet season, the bacterial community was most significantly the landscape patterns variables shaped by Built-up (F = 1.71, p < 0.01), Grassland (F = 1.47, p < 0.01), and Forest (F = 1.64, p < 0.05) in the UW, AW, and NW. The bacterial community was most significantly the water chemistry variables influenced by DO (F = 1.75, p < 0.01) and WT (F = 1.15, p < 0.01) in the UW and AW (Supplementary Table S5). Notably, a greater number of landscape pattern variables exhibited significant correlations with bacterial communities compared to water chemistry variables in both dry and wet seasons. However, it was also observed that there was a high interpretation rate of landscape patterns for water chemistry (Supplementary Figure S8). These results highlight the complex interplay between landscape features and water chemistry in shaping bacterial communities within each watershed.
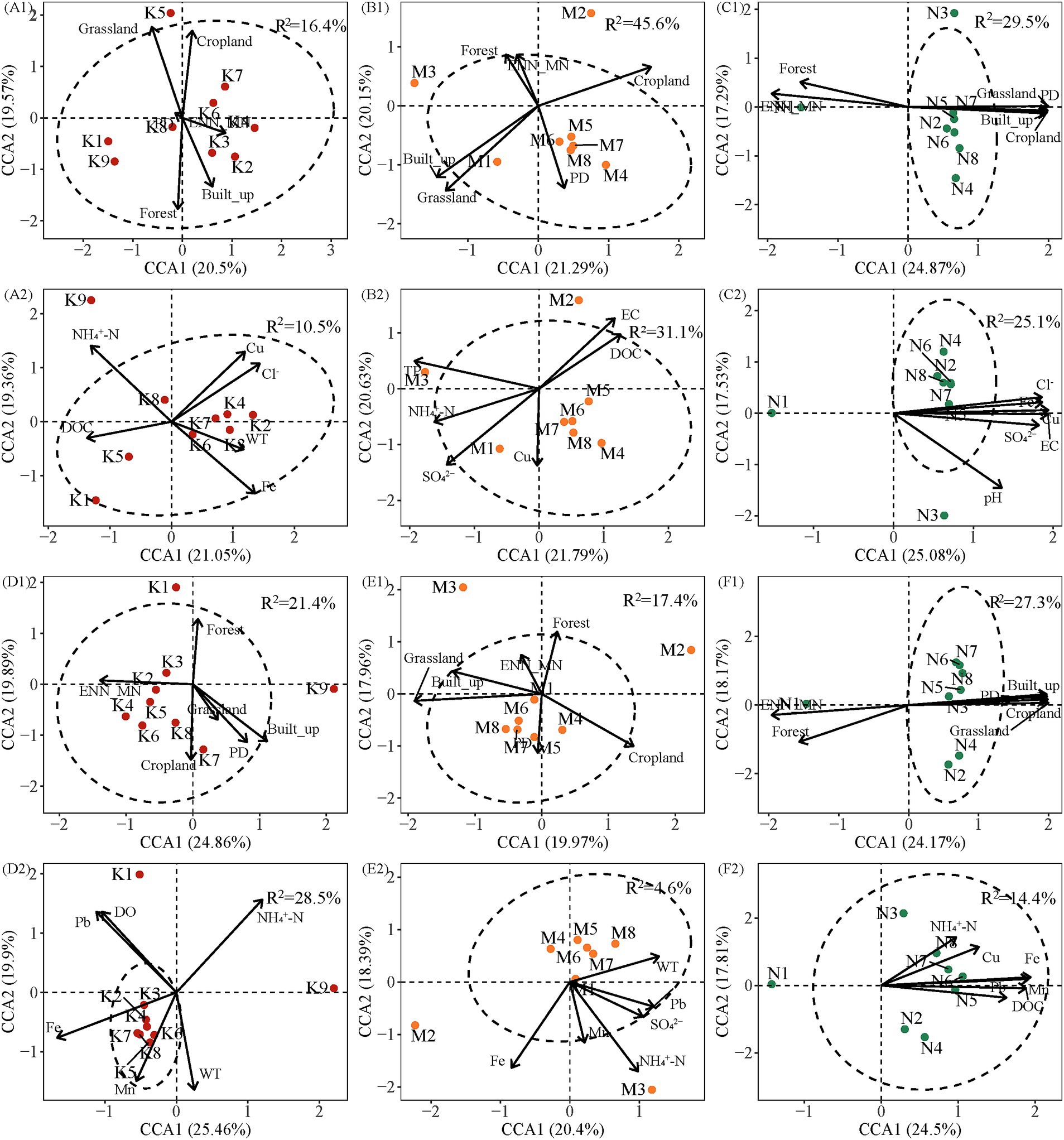
Figure 7. Canonical correspondence analysis (CCA) was performed to determine the most significant landscape patterns and water chemistry variables shaping bacterial communities in the different watersheds. Blue and red arrows indicate landscape patterns and water chemistry variables, respectively. R2 represents adjustment R2. (A1–C1) Show the relationship between landscape patterns and bacterial community in the dry season, respectively. (A2–C2) Show the relationship between water chemistry and bacterial community in the dry season, respectively. (D1–F1) Show the relationship between landscape patterns and bacterial community in the wet season, respectively. (D2–F2) Show the relationship between water chemistry and bacterial community in the wet season, respectively. Abbreviations of environmental variables are defined in the “Materials and methods” section.
3.4 Assembly mechanisms of bacterial community structure in different watershed
VPA was used to quantify the relative contributions of landscape pattern, water chemistry, and their interactions to bacterial community variation (Figure 8). Forward selection models identified the specific landscape pattern and water chemistry variables with significant effects on community structure (Supplementary Table S6). Interestingly, the explanatory ratios of water chemistry on bacterial community variation decreased as increasing anthropogenic activity, with significantly lower contributions in the UW and AW compared to the NW in both dry and wet seasons. This decrease was particularly evident in the dry season, with water chemistry explaining only 11.43 and 3.65% of the variations in AW and UW, respectively. This suggests a decreasing influence of “mass effects” on bacterial communities as human activities increase. Notably, unexplained variation in the AW was highest (76.24%) in the wet season, suggesting the presence of additional influencing factors.
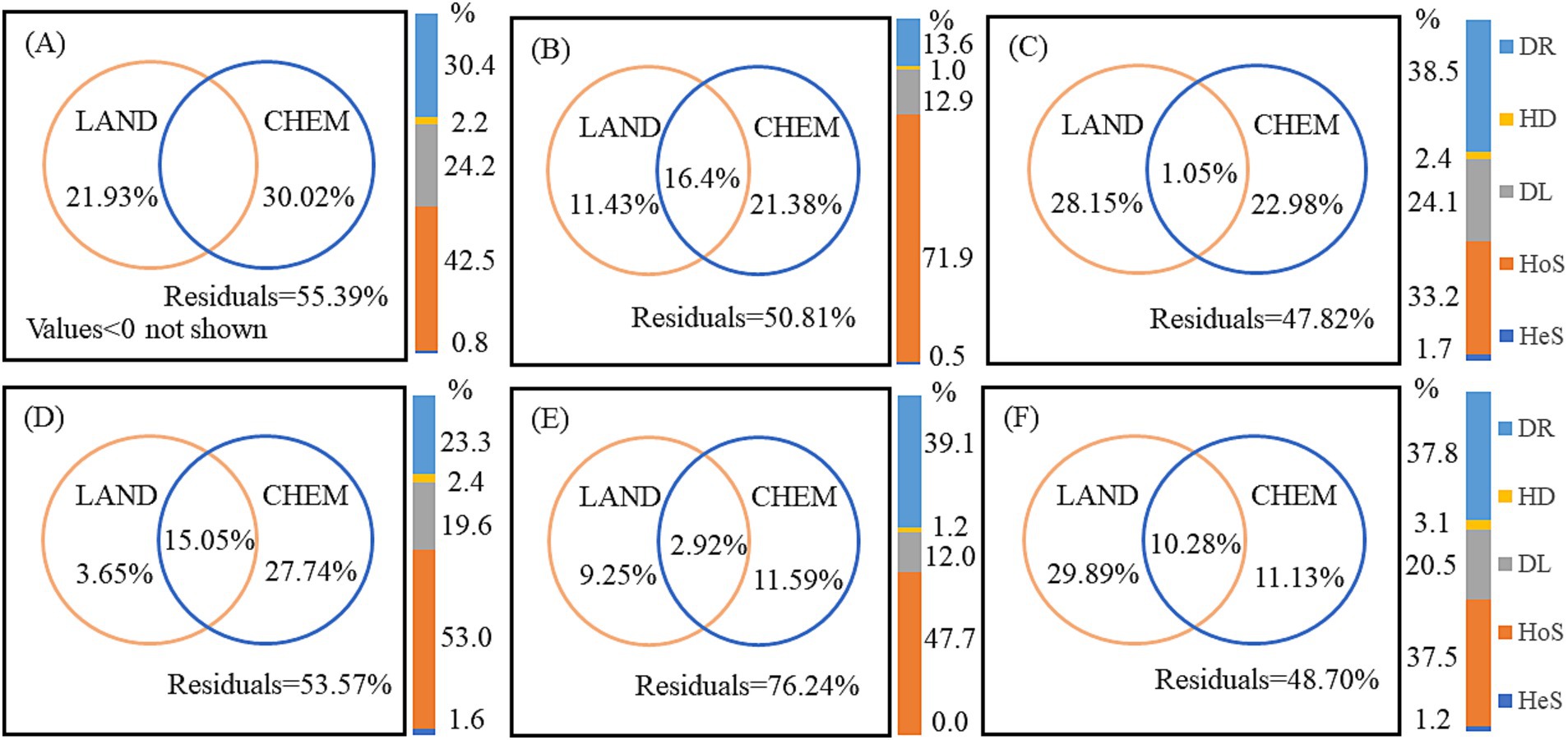
Figure 8. Variation partitioning analysis (VPA) quantified the contribution of landscape pattern (LAND) and water chemistry (CHEM) variables to bacterial community variation across watersheds and seasons. Values less than zero are not shown. Bar diagrams represent the proportion of ecological processes in the bacterial communities. DR, drift; HD, homogenizing dispersal; DL, dispersal limitation; HoS, homogeneous selection; HeS, heterogeneous selection. (A–C) Represent UW, AW, and NW in the dry season, respectively. (D–F) Represent UW, AW, and NW in the wet season, respectively.
Null model analysis revealed that deterministic processes played a greater role in shaping bacterial communities in the UW and AW compared to the NW, as indicated by the lower pNST values (<0.5) in the former, especially in the AW during the wet season (Supplementary Figure S9). This indicated that deterministic processes and stochastic processes collaborated in shaping the bacterial communities in each watershed, and deterministic process was more pronounced in the UW and AW than in the NW. Among the deterministic processes, HoS emerged as the dominant force in all watersheds, and the order of HoS was AW (71.9%) > UW (42.5%) > NW (33.2%) in the dry season, and UW (53.0%) > AW (47.7%) > NW (37.5%) in the wet season. Stochastic processes also played a role, primarily driven by DL and DR. The influence of DR was significantly reduced in UW (30.4%) and AW (13.6%) compared to NW (38.5%) in the dry season, suggesting that agricultural activities may enhance the role of HoS while reducing the influence of DR. Furthermore, DL was significantly lower in AW compared to UW and NW (Figure 8).
4 Discussion
4.1 Diversity and composition differences of bacterial community structure
Our study revealed a significant difference in microbial diversity among the three watersheds in the dry season. NW exhibited the highest Chao1 richness and Shannon diversity compared to the AW and UW in the dry season (p < 0.05). This aligns with previous research indicating that regions with intensive agriculture and industry often harbor lower microbial diversity (Ibekwe et al., 2016; Li et al., 2020). The microbiota is mainly controlled by local environmental factors, and exhibiting a spatial pattern of greater species diversity in headwater (low environment stress) than downstream (Besemer et al., 2013; Savio et al., 2015). It should be noted that the sites within NW were less influenced by pollutants. This conclusion is further supported by the fact that NW has the highest α diversity of all samples (i.e., site N1) (Supplementary Figure S3). More surface water directly flows into rivers through the upper of the impervious layer in UW compared to NW, and the rich microorganisms of the soil beneath the impermeable layer are not easily carried into the river. This might also help explain the low α diversity observed in the UW. In AW, large agricultural areas have decreased landscape heterogeneity (Figure 6), leading to a decrease in the diversity of exogenously imported bacterial communities. Furthermore, both AW and UW experience significant anthropogenic activities that introduce pollutants such as antibiotics, sewage, fertilizers, and pesticides. These stressors can negatively impact bacterial communities and contribute to lower diversity (Liu et al., 2023; Zhang et al., 2023; Zou et al., 2023). Interestingly, in the wet season, the differences in α diversity among watersheds became less pronounced, with only the Shannon diversity in AW being slightly higher than in UW (p < 0.05). This could be due to the higher temperature in the wet season promotes microbial growth (Fang et al., 2023), while increased rainfall leads to higher water levels and flow, potentially homogenizing bacterial communities and reducing diversity differences between watersheds (Chen et al., 2019). However, this is inconsistent with some of the previous conclusions. For instance, previous studies have shown that the α diversity index of river bacterial community increased significantly with the increase of human activity intensity (the proportion of built-up increased) (Zhou et al., 2020; Wu et al., 2022). These discrepancies may be due to differences in the level of anthropogenic interference, watershed scale, or the specific microbial communities studied.
Here, Pseudomonadota, Bacteroidota, Actinobacteriota, Cyanobacteria, and Bacillota are dominant phyla in the three watersheds (Figure 4), and the widespread presence of these major bacterial lineages in various riverine habitats has been suggested (Zhao et al., 2021; Wu et al., 2022; Zou et al., 2023). From the difference analysis, C_Actinobacteria was significantly enriched in the AW and UW, especially the AW. C_Actinobacteria are a highly diverse group of bacteria renowned for their remarkable metabolic versatility. They are responsible for the production of most clinically used antibiotics and a large number of other natural products with medical and agricultural applications (van Bergeijk et al., 2020). An estimated 64% of all naturally occurring antibiotics are derived from Actinobacteriota species (Hutchings et al., 2019). Their capabilities extend beyond antibiotic production, as they exhibit antipathogenic activity, enhance plant biomass production, and induce plant disease resistance. As a result, C_Actinobacteria are commonly utilized as biofertilizer inoculants, contributing to sustainable agricultural practices (Olanrewaju and Babalola, 2019; Li et al., 2022). Species sorting facilitates the growth of Actinobacteria that specialize in k-strategies (with slow growth, small size, and less susceptible to predation avoidance) (van Teeseling et al., 2015). C_Gammaproteobacteria were also significantly enriched in the AW in the dry season. This group has been identified as the core bacteria with the highest colonization potential in the gut, and was further found to be the best indicator taxon of the response to environmental concentrations of soil pollution. Furthermore, the abundance of C_Gammaproteobacteria is closely correlated with the presence of antibiotic-resistance genes (Zhang Q. et al., 2021). The NW watershed, on the other hand, showed significant enrichment of C_Clostridia and C_Bacilli (O_Erysipelotrichales). These bacteria are ubiquitous in nature, commonly found in soil samples and the human intestine. The high abundance of these groups in the NW may be related to the large number of settlements close to the riparian zone, where untreated human and poultry waste may enter the river system.
The bacterial composition showed intriguing seasonal and spatial variations. Notably, C_Bacteroidia (O_Chitinophagales) and C_Bacilli (O_Paenibacillales) in the wet season were more abundant in AW and UW, respectively. The presence of C_Bacteroidia, often found in human and animal intestines, suggests potential fecal contamination, aligning with previous research (Wéry et al., 2008; Ibekwe et al., 2016). Interestingly, soils with high NO3−-N (UW and AW) had more C_Bacteroidia and a complete loss of Firmicutes (Kandasamy et al., 2021). Thus, Bacteroidetes are detected consistently at all sites in both two seasons, suggesting that they come from anthropogenic inputs (Dubinsky et al., 2012). More runoff can carry such bacteria into the river in pipes or farther villages and towns. These microbes may be active in microbe nutrient interactions. On the other hand, C_Parcubacteria (phylum Patescibacteria) were significantly enriched in the NW, especially in the wet season. These bacteria are commonly found in groundwater, sediments, lakes, and other aquifer environments (Proctor et al., 2018; Tian et al., 2020). Their small cell size (~0.3 μm) is likely an adaptation to low-nutrient conditions, facilitating increased nutrient uptake and metabolic rates due to a higher surface-area-to-volume ratio (Savage et al., 2007; Tian et al., 2020).
4.2 Spatial scale effects of landscape pattern on bacterial community structure
The results showed that the correlations between the landscape patterns and bacterial community structure showed an obvious decrease in UW compared to NW in both the dry and wet seasons, and a slightly decrease in AW. Prior studies have demonstrated that landuse types within watersheds, such as agricultural and urban landuse are significant drivers of the structure of the aquatic biota (e.g., bacteria, zooplankton, and phytoplankton) (Uchida et al., 2021; Yang et al., 2022; Zhang Y. et al., 2022). Urbanization is the process of anthropogenic transformation of wildlands or agricultural land into built environments where human habitation and work occur (Seto et al., 2012). Urban construction, a point source of pollution (Bu et al., 2014), generates substantial industrial and domestic waste discharges, disrupting the natural link between landscape patterns and bacterial communities. Different landscape patterns alter the input of nutrients or particulate matter into rivers, which provide different nutrients or environmental stresses to bacterial communities, affecting ecological niche processes, and thus influencing bacterial community assembly (Huang and Huang, 2019; Zhao et al., 2021; Shu et al., 2022). Our analysis confirmed significantly higher concentrations of Cl−, SO₄2−, and NO₃−-N in UW compared to AW and NW (Supplementary Table S8). The extensive impervious surfaces and underground drainage networks in urban areas alter surface runoff patterns, resulting in the influx of industrial, agricultural, and domestic wastewater containing pollutants such as organic matter, heavy metals, and nutrients (Kang et al., 2010; Liu et al., 2021). The increased impervious surface area in UW further accelerates the entry of exogenous materials into the waterways (Shu et al., 2020). The influence of landscape patterns on bacterial community structure in UW was less than that in the other two watersheds (p > 0.05), which further supports this finding (Figures 6A,D). In addition, the layout of underground pipeline networks in cities makes the relationship between landscape patterns and riverine bacterial communities more complex.
Our results show that the correlations of landscape patterns on bacterial communities were similar at both the near riparian buffer scale (especially the 500 m riparian buffer scale) and the sub-watershed scale across the three watersheds, except that the correlation in the AW was slightly lower at the sub-watershed scale in the dry season. Previous studies have shown that the optimal scale of landscape patterns on bacterial communities in this region is 500 m riparian buffer in the dry season, and it is at 1000 m riparian buffer in the wet season (Shu et al., 2022). The reasons for this inconsistency are possibly due to variations in watershed area, human activities, and the choice of landscape metrics used to represent landscape patterns. Terrestrial landscapes, acting as sources of organic matter, exert both direct and indirect influences on aquatic organisms (Fasching et al., 2020). While some studies highlight the crucial role of riparian buffer zones in intercepting and processing pollutants through runoff (Shi et al., 2017; Hille et al., 2018), other studies emphasize the greater importance of landscape patterns at the sub-watershed scale for regulating water environments due to the broader spatial range of nutrient loading and retention processes. Our results suggest that managing landscape patterns at both the riparian buffer zone (particularly at 500 m) and the sub-watershed scale is equally important for maintaining a healthy riverine bacterial community. Interestingly, the effect of landscape patterns on bacterial communities remained relatively stable across seasons in AW and NW. However, in UW, this influence decreased slightly at the 100 m buffer zone from the dry to the wet season. This shift can be attributed to increased rainfall in the wet season, which introduces more pollutants from urban pipe networks into the river, thereby increasing environmental pressures on bacterial growth and diversity (Johnston and Roberts, 2009; Pokharel et al., 2020). This suggests that the effects of the environmental on river ecosystems need to be considered on a larger scale during the wet season.
4.3 Assembly mechanisms of bacterial communities
The results indicated the stronger influence of species sorting, as compared to NW, on bacterial community assembly in the intensive anthropogenic watershed. The dominance of stochastic or deterministic processes in microbial assembly depends on several variables, including nutrient levels (Chase, 2010), environmental stressors (Menéndez-Serra et al., 2023), and spatiotemporal scales (Shi et al., 2018; Fang et al., 2023). Previous research in this region has demonstrated the prevalence of either mass effects (Wang et al., 2019; Zhao et al., 2021) or species sorting (Shu et al., 2022; Wu et al., 2022) as the primary driver of community assembly. Several possible reasons contributed to this phenomenon. Firstly, distinct anthropogenic activities (i.e., urban and agricultural activities) have altered the landscape patterns, water chemistry, and structure of the riverine microbial community (Huang and Huang, 2019). Here, the cities of Xinyu (UW) are rich in iron ore and other mineral resources, which are important industrial areas in Jiangxi Province (Shu et al., 2020). However, residential areas in AW are relatively scattered, with a large number of villages and towns along the river upstream (Supplementary Figure S1). Secondly, the AW exhibited significantly smaller distances in terms of landscape patterns and water chemistry compared to other watersheds in both dry and wet seasons. Surprisingly, we did not observe a distance-decay in AW (Figure 6). Conversely, the NW displayed significantly higher levels of non-freshwater bacteria and ENN_MN (Figure 5; Supplementary Table S4). The non-freshwater populations could have been introduced via runoff or anthropogenic inputs, reflecting the influence of mass effects (Staley et al., 2013; Zhao et al., 2021). These suggest stronger mass effects in NW. Thirdly, spatial distance plays a more important role in explaining planktonic bacterial community structure than environmental factors in large-scale watersheds (He et al., 2021). However, this study focuses on small and medium-sized watersheds in the subtropical region, where the relatively gentle terrain and numerous small reservoirs increase water residence time, thereby enhancing the influence of species sorting (Read et al., 2015; Liu et al., 2018; Zhao et al., 2021).
Our results showed that homogeneous selection and dispersal limitation jointly dominated the bacterial community assembly in the three watersheds. The effect of HoS was AW (71.9%) > UW (42.5%) > NW (33.2%) in the dry season, and UW (53.0%) > AW (47.7%) > NW (37.8%) in the wet season (Figure 8). It is worth noting that the ecological prosses of the bacterial communities in AW (dry season) and UW (wet season) were dominated by deterministic process. HoS is usually considered as a leading factor under the stable state after a disturbance and it may be co-varied with physicochemical variables (Dini-Andreote et al., 2015; Li et al., 2019). In our study, the consistent environmental conditions across the watersheds likely established a stable selective pressure, resulting in HoS as the primary assembly process (Dini-Andreote et al., 2015). Given the relatively small environmental differences among the selected watersheds, HoS emerged as the primary assembly process. Local environmental factors exert a greater influence on bacterial communities than regional factors, as supported by mechanism theories. Here, water chemistry variables such as DOC, NH4+-N, Cl−, and SO42− played crucial roles in shaping the bacterial community structure (Figure 7; Supplementary Tables S5, S6). Variations in dissolved organic matter composition can differentially impact bacterial community distribution by influencing metabolic capabilities (Logue et al., 2016). For instance, Roseobacter exhibits high absorption of carbon monomers and amino acids, whereas certain Sphingomonas members thrive in low-carbon environments (Figueroa et al., 2021). Additionally, SO42− and Cl− serve as indicators of anthropogenic pollution sources (e.g., agricultural inputs, sewage leakage, and manure), while essential nutrients such as TP, TN, and NH4−-N influence the trophic state and eukaryotic microbial structure within aquatic ecosystems (Cai et al., 2022). These findings further support the increased influence of species sorting that was observed in the UW and AW.
5 Conclusion
It is important to fully understand the assembly processes of riverine bacterial communities in different anthropogenically disturbed watersheds for the ecosystem health assessments and ecological regulation of rivers. This study revealed that the influence of landscape patterns from riparian buffer zone to sub-watershed on river bacterial community was not significant in the different anthropogenically disturbed watersheds, indicating that the near riparian zone and sub-watershed scale were equally important in protecting the ecological environment of rivers. Homogenous selection leads to a more uniform bacterial community structure and lower diversity, especially in intensive agricultural watersheds. The riverine bacterial communities were mainly affected by changing local environment in intensive agriculture and urban watersheds, rather than exogenous input. These indicated that mass effects and species sorting jointly shaped bacterial community assembly, with species sorting having a greater influence on bacterial community assembly in intensive urban and agricultural watersheds. Our study provides useful and novel insights into the assembly processes of the riverine bacterial community under different anthropogenically disturbed watersheds, and provides a theoretical basis for the ecological management of river environment.
Data availability statement
The datasets presented in this study can be found in online repositories. The names of the repository/repositories and accession number(s) can be found at: https://www.ncbi.nlm.nih.gov/, PRJNA901368.
Author contributions
YS: Conceptualization, Investigation, Methodology, Visualization, Writing – original draft. PW: Funding acquisition, Supervision, Writing – review & editing. JW: Data curation, Investigation, Methodology, Writing – review & editing. MD: Formal analysis, Methodology, Writing – review & editing. HZ: Data curation, Resources, Writing – review & editing. MN: Data curation, Resources, Writing – review & editing. GH: Investigation, Resources, Writing – review & editing.
Funding
The author(s) declare that financial support was received for the research, authorship, and/or publication of this article. This work was supported by the National Natural Science Foundation of China (42167013).
Conflict of interest
The authors declare that the research was conducted in the absence of any commercial or financial relationships that could be construed as a potential conflict of interest.
Publisher’s note
All claims expressed in this article are solely those of the authors and do not necessarily represent those of their affiliated organizations, or those of the publisher, the editors and the reviewers. Any product that may be evaluated in this article, or claim that may be made by its manufacturer, is not guaranteed or endorsed by the publisher.
Supplementary material
The Supplementary material for this article can be found online at: https://www.frontiersin.org/articles/10.3389/fmicb.2024.1463549/full#supplementary-material
Footnotes
References
Ajani, P. A., Savela, H., Kahlke, T., Harrison, D., Jeffries, T., Kohli, G. S., et al. (2023). Response of planktonic microbial assemblages to disturbance in an urban sub-tropical estuary. Water Res. 243:120371. doi: 10.1016/j.watres.2023.120371
Akasaka, M., Takamura, N., Mitsuhashi, H., and Kadono, Y. (2010). Effects of land use on aquatic macrophyte diversity and water quality of ponds. Freshw. Biol. 55, 909–922. doi: 10.1111/j.1365-2427.2009.02334.x
Allan, J. D. (2004). Landscapes and Riverscapes: the influence of land use on stream ecosystems. Annu. Rev. Ecol. Evol. Syst. 35, 257–284. doi: 10.1146/annurev.ecolsys.35.120202.110122
Besemer, K., Singer, G., Quince, C., Bertuzzo, E., Sloan, W., and Battin, T. J. (2013). Headwaters are critical reservoirs of microbial diversity for fluvial networks. Proc. R. Soc. B 280:20131760. doi: 10.1098/rspb.2013.1760
Birrer, S. C., Wemheuer, F., Dafforn, K. A., Gribben, P. E., Steinberg, P. D., Simpson, S. L., et al. (2021). Legacy metal contaminants and excess nutrients in low flow estuarine Embayments Alter composition and function of benthic bacterial communities. Front. Microbiol. 12:661177. doi: 10.3389/fmicb.2021.661177
Bolyen, E., Rideout, J. R., Dillon, M., Bokulich, N., Abnet, C., al-Ghalith, G. A., et al. (2019). Reproducible, interactive, scalable and extensible microbiome data science using QIIME 2. Nat. Biotechnol. 37, 852–857. doi: 10.1038/s41587-019-0209-9
Borcard, D., Legendre, P., and Drapeau, P. (1992). Partialling out the spatial component of ecological variation. Ecology 73, 1045–1055. doi: 10.2307/1940179
Bu, H., Meng, W., Zhang, Y., and Wan, J. (2014). Relationships between land use patterns and water quality in the Taizi River basin, China. Ecol. Indic. 41, 187–197. doi: 10.1016/j.ecolind.2014.02.003
Cai, W., Huang, Q., Li, H., Cheng, H., Li, Y., and Hu, J. (2022). Longitudinal patterns of microbial communities in the water diversion Rivers of south-to-north water diversion project. Clean-Soil Air Water 50:2100303. doi: 10.1002/clen.202100303
Callahan, B. J., McMurdie, P. J., Rosen, M. J., Han, A. W., Johnson, A. J. A., and Holmes, S. P. (2016). DADA2: high-resolution sample inference from Illumina amplicon data. Nat. Methods 13, 581–583. doi: 10.1038/nmeth.3869
Caporaso, J. G., Lauber, C. L., Walters, W. A., Berg-Lyons, D., Huntley, J., Fierer, N., et al. (2012). Ultra-high-throughput microbial community analysis on the Illumina HiSeq and MiSeq platforms. ISME J. 6, 1621–1624. doi: 10.1038/ismej.2012.8
Castrillo, G., Teixeira, P. J. P. L., Paredes, S. H., Law, T. F., De Lorenzo, L., Feltcher, M. E., et al. (2017). Root microbiota drive direct integration of phosphate stress and immunity. Nature 543, 513–518. doi: 10.1038/nature21417
Chase, J. M. (2010). Stochastic community assembly causes higher biodiversity in more productive environments. Science 328, 1388–1391. doi: 10.1126/science.1187820
Chen, W., Ren, K., Isabwe, A., Chen, H., Liu, M., and Yang, J. (2019). Stochastic processes shape microeukaryotic community assembly in a subtropical river across wet and dry seasons. Microbiome 7:138. doi: 10.1186/s40168-019-0749-8
Chen, W., Wilkes, G., Khan, I. U. H., Pintar, K. D. M., Thomas, J. L., Lévesque, C. A., et al. (2018). Aquatic bacterial communities associated with land use and environmental factors in agricultural landscapes using a Metabarcoding approach. Front. Microbiol. 9:2301. doi: 10.3389/fmicb.2018.02301
Clarke, K., and Ainsworth, M. (1993). A method of linking multivariate community structure to environmental variables. Mar. Ecol. Prog. Ser. 92, 205–219. doi: 10.3354/meps092205
Dini-Andreote, F., Stegen, J. C., van Elsas, J. D., and Salles, J. F. (2015). Disentangling mechanisms that mediate the balance between stochastic and deterministic processes in microbial succession. Proc. Natl. Acad. Sci. USA 112, E1326–E1332. doi: 10.1073/pnas.1414261112
Dubinsky, E. A., Esmaili, L., Hulls, J. R., Cao, Y., Griffith, J. F., and Andersen, G. L. (2012). Application of phylogenetic microarray analysis to discriminate sources of fecal pollution. Environ. Sci. Technol. 46, 4340–4347. doi: 10.1021/es2040366
Fang, W., Fan, T., Xu, L., Wang, S., Wang, X., Lu, A., et al. (2023). Seasonal succession of microbial community co-occurrence patterns and community assembly mechanism in coal mining subsidence lakes. Front. Microbiol. 14:1098236. doi: 10.3389/fmicb.2023.1098236
Fasching, C., Akotoye, C., Bižić, M., Fonvielle, J., Ionescu, D., Mathavarajah, S., et al. (2020). Linking stream microbial community functional genes to dissolved organic matter and inorganic nutrients. Limnol. Oceanogr. 65, S71–S87. doi: 10.1002/lno.11356
Figueroa, D., Capo, E., Lindh, M. V., Rowe, O. F., Paczkowska, J., Pinhassi, J., et al. (2021). Terrestrial dissolved organic matter inflow drives temporal dynamics of the bacterial community of a subarctic estuary (northern Baltic Sea). Environ. Microbiol. 23, 4200–4213. doi: 10.1111/1462-2920.15597
Fouhy, F., Clooney, A. G., Stanton, C., Claesson, M. J., and Cotter, P. D. (2016). 16S rRNA gene sequencing of mock microbial populations- impact of DNA extraction method, primer choice and sequencing platform. BMC Microbiol. 16:123. doi: 10.1186/s12866-016-0738-z
Gao, F.-Z., He, L.-Y., Hu, L.-X., Chen, J., Yang, Y.-Y., Zou, H.-Y., et al. (2022). Anthropogenic activities and seasonal properties jointly drive the assemblage of bacterial communities in subtropical river basins. Sci. Total Environ. 806:151476. doi: 10.1016/j.scitotenv.2021.151476
García-Armisen, T., İnceoğlu, Ö., Ouattara, N. K., Anzil, A., Verbanck, M. A., Brion, N., et al. (2014). Seasonal variations and resilience of bacterial communities in a sewage polluted Urban River. PLoS One 9:e92579. doi: 10.1371/journal.pone.0092579
Germer, S., Neill, C., Krusche, A. V., and Elsenbeer, H. (2010). Influence of land-use change on near-surface hydrological processes: undisturbed forest to pasture. J. Hydrol. 380, 473–480. doi: 10.1016/j.jhydrol.2009.11.022
Guéguen, C., Gilbin, R., Pardos, M., and Dominik, J. (2004). Water toxicity and metal contamination assessment of a polluted river: the upper Vistula River (Poland). Appl. Geochem. 19, 153–162. doi: 10.1016/S0883-2927(03)00110-0
Gupta, S., Graham, D. W., Sreekrishnan, T. R., and Ahammad, S. Z. (2023). Exploring the impacts of physicochemical characteristics and heavy metals fractions on bacterial communities in four rivers. J. Environ. Manag. 325:116453. doi: 10.1016/j.jenvman.2022.116453
He, H., Pan, B., Yu, K., Zheng, X., Wu, Y., Xiao, L., et al. (2021). Determinants of bacterioplankton structures in the typically turbid Weihe River and its clear tributaries from the northern foot of the Qinling Mountains. Ecol. Indic. 121:107168. doi: 10.1016/j.ecolind.2020.107168
Hille, S., Andersen, D. K., Kronvang, B., and Baattrup-Pedersen, A. (2018). Structural and functional characteristics of buffer strip vegetation in an agricultural landscape – high potential for nutrient removal but low potential for plant biodiversity. Sci. Total Environ. 628-629, 805–814. doi: 10.1016/j.scitotenv.2018.02.117
Hobbie, S. E., Finlay, J. C., Janke, B. D., Nidzgorski, D. A., Millet, D. B., and Baker, L. A. (2017). Contrasting nitrogen and phosphorus budgets in urban watersheds and implications for managing urban water pollution. Proc. Natl. Acad. Sci. USA 114, 4177–4182. doi: 10.1073/pnas.1618536114
Huang, Y., and Huang, J. (2019). Coupled effects of land use pattern and hydrological regime on composition and diversity of riverine eukaryotic community in a coastal watershed of Southeast China. Sci. Total Environ. 660, 787–798. doi: 10.1016/j.scitotenv.2019.01.063
Hutchings, M. I., Truman, A. W., and Wilkinson, B. (2019). Antibiotics: past, present and future. Curr. Opin. Microbiol. 51, 72–80. doi: 10.1016/j.mib.2019.10.008
Ibekwe, A. M., Ma, J., and Murinda, S. E. (2016). Bacterial community composition and structure in an Urban River impacted by different pollutant sources. Sci. Total Environ. 566-567, 1176–1185. doi: 10.1016/j.scitotenv.2016.05.168
Johnston, E. L., and Roberts, D. A. (2009). Contaminants reduce the richness and evenness of marine communities: a review and meta-analysis. Environ. Pollut. 157, 1745–1752. doi: 10.1016/j.envpol.2009.02.017
Kandasamy, S., Weerasuriya, N., White, J., Patterson, G., and Lazarovits, G. (2021). “Soil’s physical and nutritional balance is essential for establishing a healthy microbiome” in Microbiome Stimulants for Crops, 381–404.
Kang, J.-H., Lee, S. W., Cho, K. H., Ki, S. J., Cha, S. M., and Kim, J. H. (2010). Linking land-use type and stream water quality using spatial data of fecal indicator bacteria and heavy metals in the Yeongsan river basin. Water Res. 44, 4143–4157. doi: 10.1016/j.watres.2010.05.009
Laudon, H., and Sponseller, R. A. (2018). How landscape organization and scale shape catchment hydrology and biogeochemistry: insights from a long-term catchment study. WIREs Water 5:e1265. doi: 10.1002/wat2.1265
Leibold, M., Holyoak, M., Mouquet, N., Amarasekare, P., Chase, J., Hoopes, M., et al. (2004). The Metacommunity concept: a framework for multi-scale community ecology. Ecol. Lett. 7, 601–613. doi: 10.1111/j.1461-0248.2004.00608.x
Li, F., Altermatt, F., Yang, J., An, S., Li, A., and Zhang, X. (2020). Human activities’ fingerprint on multitrophic biodiversity and ecosystem functions across a major river catchment in China. Glob. Change Biol. Bioenergy. 26, 6867–6879. doi: 10.1111/gcb.15357
Li, Y., Gao, Y., Zhang, W., Wang, C., Wang, P., Niu, L., et al. (2019). Homogeneous selection dominates the microbial community assembly in the sediment of the three gorges reservoir. Sci. Total Environ. 690, 50–60. doi: 10.1016/j.scitotenv.2019.07.014
Li, Y., Li, H., Han, X., Han, G., Xi, J., Liu, Y., et al. (2022). Actinobacterial biofertilizer improves the yields of different plants and alters the assembly processes of rhizosphere microbial communities. Appl. Soil Ecol. 171:104345. doi: 10.1016/j.apsoil.2021.104345
Lindström, E., and Langenheder, S. (2012). Local and regional factors influencing bacterial community assembly. Environ. Microbiol. 4, 1–9. doi: 10.1111/j.1758-2229.2011.00257.x
Liu, Y., Jiang, X., Li, D., Shen, J., An, S., and Leng, X. (2023). Intensive human land uses cause the biotic homogenization of algae and change their assembly process in a major watershed of China. Sci. Total Environ. 871:162115. doi: 10.1016/j.scitotenv.2023.162115
Liu, S., Lu, J., Adriaenssens, E. M., Wang, J., McCarthy, A. J., and Sekar, R. (2024). Industrial and agricultural land uses affected the water quality and shaped the bacterial communities in the inflow rivers of Taihu Lake. Front. Environ. Sci. 12:1340875. doi: 10.3389/fenvs.2024.1340875
Liu, S., Wang, P., Wang, C., Chen, J., Wang, X., Hu, B., et al. (2021). Ecological insights into the disturbances in bacterioplankton communities due to emerging organic pollutants from different anthropogenic activities along an urban river. Sci. Total Environ. 796:148973. doi: 10.1016/j.scitotenv.2021.148973
Liu, T., Zhang, A. N., Wang, J., Liu, S., Jiang, X., Dang, C., et al. (2018). Integrated biogeography of planktonic and sedimentary bacterial communities in the Yangtze River. Microbiome 6:16. doi: 10.1186/s40168-017-0388-x
Logue, J. B., Stedmon, C. A., Kellerman, A. M., Nielsen, N. J., Andersson, A. F., Laudon, H., et al. (2016). Experimental insights into the importance of aquatic bacterial community composition to the degradation of dissolved organic matter. ISME J. 10, 533–545. doi: 10.1038/ismej.2015.131
Menéndez-Serra, M., Ontiveros, V. J., Cáliz, J., Alonso, D., and Casamayor, E. O. (2023). Understanding stochastic and deterministic assembly processes in microbial communities along temporal, spatial and environmental scales. Mol. Ecol. 32, 1629–1638. doi: 10.1111/mec.16842
Mohapatra, M., Manu, S., Kim, J. Y., and Rastogi, G. (2023). Distinct community assembly processes and habitat specialization driving the biogeographic patterns of abundant and rare bacterioplankton in a brackish coastal lagoon. Sci. Total Environ. 879:163109. doi: 10.1016/j.scitotenv.2023.163109
Mouri, G., Takizawa, S., and Oki, T. (2011). Spatial and temporal variation in nutrient parameters in stream water in a rural-urban catchment, Shikoku, Japan: effects of land cover and human impact. J. Environ. Manag. 92, 1837–1848. doi: 10.1016/j.jenvman.2011.03.005
Murphy, S. F., Runkel, R. L., Stets, E. G., Nolan, A. J., and Repert, D. A. (2024). Urbanization and water management control stream water quality along a mountain to plains transition. Water Resour. Res. 60:e2023WR035633. doi: 10.1029/2023WR035633
Newton, R., Jones, S., Eiler, A., McMahon, K. D., and Bertilsson, S. (2011). A guide to the natural history of freshwater Lake Bacteria. Microbiol. Mol. Biol. Rev. 75, 14–49. doi: 10.1128/MMBR.00028-10
Ning, D., Yuan, M., Wu, L., Zhang, Y., Guo, X., Zhou, X., et al. (2020). A quantitative framework reveals ecological drivers of grassland microbial community assembly in response to warming. Nat. Commun. 11:4717. doi: 10.1038/s41467-020-18560-z
Olanrewaju, O. S., and Babalola, O. O. (2019). Streptomyces: implications and interactions in plant growth promotion. Appl. Microbiol. Biotechnol. 103, 1179–1188. doi: 10.1007/s00253-018-09577-y
Paul, M. J., and Meyer, J. L. (2001). Streams in the urban landscape. Annu. Rev. Ecol. Evol. Syst. 32, 333–365. doi: 10.1146/annurev.ecolsys.32.081501.114040
Pokharel, B., Wang, S.-Y. S., Meyer, J., Marahatta, S., Nepal, B., Chikamoto, Y., et al. (2020). The east–west division of changing precipitation in Nepal. Int. J. Climatol. 40, 3348–3359. doi: 10.1002/joc.6401
Proctor, C. R., Besmer, M. D., Langenegger, T., Beck, K., Walser, J.-C., Ackermann, M., et al. (2018). Phylogenetic clustering of small low nucleic acid-content bacteria across diverse freshwater ecosystems. ISME J. 12, 1344–1359. doi: 10.1038/s41396-018-0070-8
Read, D. S., Gweon, H. S., Bowes, M. J., Newbold, L., Field, D., Bailey, M., et al. (2015). Catchment-scale biogeography of riverine bacterioplankton. ISME J. 9, 516–526. doi: 10.1038/ismej.2014.166
Samadi Todar, S. A., Attarchi, S., and Osati, K. (2021). Investigation the seasonality effect on impervious surface detection from Sentinel-1 and Sentinel-2 images using Google earth engine. Adv. Space Res. 68, 1356–1365. doi: 10.1016/j.asr.2021.03.039
Sanfilippo, G. E., Homola, J. J., Ross, J., Kanefsky, J., Kimmel, J., Marsh, T. L., et al. (2021). Watershed-scale landuse is associated with temporal and spatial compositional variation in Lake Michigan tributary bacterial communities. J. Great Lakes Res. 47, 862–874. doi: 10.1016/j.jglr.2021.02.009
Savage, V. M., Allen, A. P., Brown, J. H., Gillooly, J. F., Herman, A. B., Woodruff, W. H., et al. (2007). Scaling of number, size, and metabolic rate of cells with body size in mammals. Proc. Natl. Acad. Sci. USA 104, 4718–4723. doi: 10.1073/pnas.0611235104
Savio, D., Sinclair, L., Ijaz, U., Parajka, J., Reischer, G., Stadler, P., et al. (2015). Bacterial diversity along a 2600 km river continuum. Environ. Microbiol. 17, 4994–5007. doi: 10.1111/1462-2920.12886
Segata, N., Izard, J., Waldron, L., Gevers, D., Miropolsky, L., Garrett, W. S., et al. (2011). Metagenomic biomarker discovery and explanation. Genome Biol. 12:R60. doi: 10.1186/gb-2011-12-6-r60
Seto, K. C., Güneralp, B., and Hutyra, L. R. (2012). Global forecasts of urban expansion to 2030 and direct impacts on biodiversity and carbon pools. Proc. Natl. Acad. Sci. USA 109, 16083–16088. doi: 10.1073/pnas.1211658109
Shi, Y., Li, Y., Xiang, X., Sun, R., Yang, T., He, D., et al. (2018). Spatial scale affects the relative role of stochasticity versus determinism in soil bacterial communities in wheat fields across the North China plain. Microbiome 6:27. doi: 10.1186/s40168-018-0409-4
Shi, P., Zhang, Y., Li, Z., Li, P., and Xu, G. (2017). Influence of land use and land cover patterns on seasonal water quality at multi-spatial scales. Catena 151, 182–190. doi: 10.1016/j.catena.2016.12.017
Shu, W., Li, F., Zhang, Q., Li, Z., Qiao, Y., Audet, J., et al. (2023). Pollution caused by mining reshaped the structure and function of bacterial communities in China’s largest ion-adsorption rare earth mine watershed. J. Hazard. Mater. 451:131221. doi: 10.1016/j.jhazmat.2023.131221
Shu, W., Wang, P., Xu, Q., Zeng, T., Ding, M., Zhang, H., et al. (2022). Coupled effects of landscape structures and water chemistry on bacterioplankton communities at multi-spatial scales. Sci. Total Environ. 811:151350. doi: 10.1016/j.scitotenv.2021.151350
Shu, W., Wang, P., Zhang, H., Ding, M., and Wu, B. (2020). Seasonal and spatial distribution and assembly processes of bacterioplankton communities in a subtropical urban river. FEMS Microbiol. Ecol. 96:fiaa154. doi: 10.1093/femsec/fiaa154
Shu, W., Wang, P., Zhao, J., Yu, X., and Xu, Q. (2020). Characteristics, sources and risk assessment of heavy metals in the Ganjiang River basin, China. Pol. J. Environ. Stud. 29, 1849–1868. doi: 10.15244/pjoes/109846
Staley, C., Gould, T. J., Wang, P., Phillips, J., Cotner, J. B., and Sadowsky, M. J. (2014). Bacterial community structure is indicative of chemical inputs in the upper Mississippi River. Front. Microbiol. 5:524. doi: 10.3389/fmicb.2014.00524
Staley, C., Unno, T., Gould, T. j., Jarvis, B., Phillips, J., Cotner, J. b., et al. (2013). Application of Illumina next-generation sequencing to characterize the bacterial community of the upper Mississippi River. J. Appl. Microbiol. 115, 1147–1158. doi: 10.1111/jam.12323
Stegen, J. C., Lin, X., Fredrickson, J. K., Chen, X., Kennedy, D. W., Murray, C. J., et al. (2013). Quantifying community assembly processes and identifying features that impose them. ISME J. 7, 2069–2079. doi: 10.1038/ismej.2013.93
Tian, R., Ning, D., He, Z., Zhang, P., Spencer, S. J., Gao, S., et al. (2020). Small and mighty: adaptation of superphylum Patescibacteria to groundwater environment drives their genome simplicity. Microbiome 8:51. doi: 10.1186/s40168-020-00825-w
Uchida, K., Blakey, R. V., Burger, J. R., Cooper, D. S., Niesner, C. A., and Blumstein, D. T. (2021). Urban biodiversity and the importance of scale. Trends Ecol. Evol. 36, 123–131. doi: 10.1016/j.tree.2020.10.011
Van Bergeijk, D. A., Terlouw, B. R., Medema, M. H., and van Wezel, G. P. (2020). Ecology and genomics of Actinobacteria: new concepts for natural product discovery. Nat. Rev. Microbiol. 18, 546–558. doi: 10.1038/s41579-020-0379-y
van Teeseling, M. C. F., Mesman, R. J., Kuru, E., Espaillat, A., Cava, F., Brun, Y. V., et al. (2015). Anammox Planctomycetes have a peptidoglycan cell wall. Nat. Commun. 6:6878. doi: 10.1038/ncomms7878
Wang, H., Xiong, X., Wang, K., Li, X., Hu, H., Li, Q., et al. (2023). The effects of land use on water quality of alpine rivers: a case study in Qilian Mountain, China. Sci. Total Environ. 875:162696. doi: 10.1016/j.scitotenv.2023.162696
Wang, P., Zhao, J., Xiao, H., Yang, W., and Yu, X. (2019). Bacterial community composition shaped by water chemistry and geographic distance in an anthropogenically disturbed river. Sci. Total Environ. 655, 61–69. doi: 10.1016/j.scitotenv.2018.11.234
Wéry, N., Lhoutellier, C., Ducray, F., Delgenès, J.-P., and Godon, J.-J. (2008). Behaviour of pathogenic and indicator bacteria during urban wastewater treatment and sludge composting, as revealed by quantitative PCR. Water Res. 42, 53–62. doi: 10.1016/j.watres.2007.06.048
Wu, J., Jin, Y., Hao, Y., and Lu, J. (2021). Identification of the control factors affecting water quality variation at multi-spatial scales in a headwater watershed. Environ. Sci. Pollut. Res. 28, 11129–11141. doi: 10.1007/s11356-020-11352-4
Wu, J., and Lu, J. (2021). Spatial scale effects of landscape metrics on stream water quality and their seasonal changes. Water Res. 191:116811. doi: 10.1016/j.watres.2021.116811
Wu, B., Wang, P., Devlin, A., She, Y., Zhao, J., Xia, Y., et al. (2022). Anthropogenic intensity-determined assembly and network stability of Bacterioplankton communities in the Le’an river. Front. Microbiol. 13:806036. doi: 10.3389/fmicb.2022.806036
Xu, Q., Wang, P., Shu, W., Ding, M., and Zhang, H. (2021). Influence of landscape structures on river water quality at multiple spatial scales: a case study of the Yuan river watershed, China. Ecol. Indic. 121:107226. doi: 10.1016/j.ecolind.2020.107226
Xu, Q., Yan, T., Wang, C., Hua, L., and Zhai, L. (2023). Managing landscape patterns at the riparian zone and sub-basin scale is equally important for water quality protection. Water Res. 229:119280. doi: 10.1016/j.watres.2022.119280
Yang, Y., Chen, H., Abdullah al, M., Ndayishimiye, J. C., Yang, J. R., Isabwe, A., et al. (2022). Urbanization reduces resource use efficiency of phytoplankton community by altering the environment and decreasing biodiversity. J. Environ. Sci. 112, 140–151. doi: 10.1016/j.jes.2021.05.001
Yi, M., Fang, Y., Hu, G., Liu, S., Ni, J., and Liu, T. (2022). Distinct community assembly processes underlie significant spatiotemporal dynamics of abundant and rare bacterioplankton in the Yangtze River. Front. Environ. Sci. Eng. 16:79. doi: 10.1007/s11783-021-1513-4
Zhang, J., Chen, Y., Huo, Y., Guo, J., Wan, L., Lu, Z., et al. (2021). Eutrophication increases deterministic processes and heterogeneity of co-occurrence networks of bacterioplankton metacommunity assembly at a regional scale in tropical coastal reservoirs. Water Res. 202:117460. doi: 10.1016/j.watres.2021.117460
Zhang, Y., Cheng, D., Ren, Y., Song, J., Xu, D., Chen, R., et al. (2022). Influence of land cover types and phytoplankton community on the distribution and fate of dissolved organic matter in a typical river located in the semi-arid regions of China. J. Hydrol. 610:127818. doi: 10.1016/j.jhydrol.2022.127818
Zhang, J., Li, S., Dong, R., Jiang, C., and Ni, M. (2019). Influences of land use metrics at multi-spatial scales on seasonal water quality: a case study of river systems in the three gorges reservoir area, China. J. Clean. Prod. 206, 76–85. doi: 10.1016/j.jclepro.2018.09.179
Zhang, L., Li, X., Fang, W., Cheng, Y., Cai, H., and Zhang, S. (2021). Impact of different types of anthropogenic pollution on bacterial community and metabolic genes in urban river sediments. Sci. Total Environ. 793:148475. doi: 10.1016/j.scitotenv.2021.148475
Zhang, T., Xu, S., Yan, R., Wang, R., Gao, Y., Kong, M., et al. (2022). Similar geographic patterns but distinct assembly processes of abundant and rare bacterioplankton communities in river networks of the Taihu Basin. Water Res. 211:118057. doi: 10.1016/j.watres.2022.118057
Zhang, Q., Zhang, Z., Lu, T., Yu, Y., Penuelas, J., Zhu, Y.-G., et al. (2021). Gammaproteobacteria, a core taxon in the guts of soil fauna, are potential responders to environmental concentrations of soil pollutants. Microbiome 9:196. doi: 10.1186/s40168-021-01150-6
Zhang, H., Zhou, X., Li, Z., Bartlam, M., and Wang, Y. (2023). Anthropogenic original DOM is a critical factor affecting LNA bacterial community assembly. Sci. Total Environ. 902:166169. doi: 10.1016/j.scitotenv.2023.166169
Zhao, J., Peng, W., Ding, M., Nie, M., and Huang, G. (2021). Effect of water chemistry, land use patterns, and geographic distances on the spatial distribution of Bacterioplankton communities in an Anthropogenically disturbed riverine ecosystem. Front. Microbiol. 12:633993. doi: 10.3389/fmicb.2021.633993
Zhou, L., Chen, W., Sun, J., Liu, L., and Huang, X. (2020). Spatial variation in Bacterioplankton communities in the Pearl River, South China: impacts of land use and physicochemical factors. Microorganisms 8:814. doi: 10.3390/microorganisms8060814
Zhou, J., and Ning, D. (2017). Stochastic community assembly: does it matter in microbial ecology? Microbiol. Rev. 81, e00002–e00017. doi: 10.1128/MMBR.00002-17
Zhu, X., Wang, L., Zhang, X., He, M., Wang, D., Ren, Y., et al. (2022). Effects of different types of anthropogenic disturbances and natural wetlands on water quality and microbial communities in a typical black-odor river. Ecol. Indic. 136:108613. doi: 10.1016/j.ecolind.2022.108613
Zou, K., Zhu, Y., Jiang, Y., Ma, S., Li, M., Zhao, W., et al. (2023). Distinct stochastic processes drive bacterial community assembly and co-occurrence patterns with common antibiotic resistance genes in two highly urbanised coastal ecosystems of the Pearl River estuary. J. Hazard. Mater. 459:132161. doi: 10.1016/j.jhazmat.2023.132161
Keywords: landscape pattern, bacterial community, spatial scale, assembly mechanisms, watershed
Citation: She Y, Wang P, Wen J, Ding M, Zhang H, Nie M and Huang G (2024) Riverine bacterial communities are more shaped by species sorting in intensive urban and agricultural watersheds. Front. Microbiol. 15:1463549. doi: 10.3389/fmicb.2024.1463549
Edited by:
Raju Sekar, Xi’an Jiaotong-Liverpool University, ChinaReviewed by:
Aditi Pandit, University of South Bohemia in České Budějovice, CzechiaWilliamson Gustave, University of The Bahamas, Bahamas
Copyright © 2024 She, Wang, Wen, Ding, Zhang, Nie and Huang. This is an open-access article distributed under the terms of the Creative Commons Attribution License (CC BY). The use, distribution or reproduction in other forums is permitted, provided the original author(s) and the copyright owner(s) are credited and that the original publication in this journal is cited, in accordance with accepted academic practice. No use, distribution or reproduction is permitted which does not comply with these terms.
*Correspondence: Peng Wang, d2FuZ3BlbmdqbHVAanhudS5lZHUuY24=