- 1College of Life Science, Jilin Agricultural University, Changchun, China
- 2School of Life Sciences, Central China Normal University, Wuhan, China
- 3Jilin Provincial Key Laboratory of Animal Resource Conservation and Utilization, Northeast Normal University, Changchun, China
- 4Jilin Provincial International Cooperation Key Laboratory for Biological Control of Agricultural Pests, Changchun, China
Background: The skin fungal communities of animals play a crucial role in maintaining host health and defending against pathogens. Because fungal infections can affect the skin microbiota of bats, gaining a comprehensive understanding of the characteristics of healthy bat skin fungal communities and the ecological processes driving them provides valuable insights into the interactions between pathogens and fungi.
Methods: We used Kruskal–Wallis tests and Permutational Multivariate Analysis of Variance (PERMANOVA) to clarify differences in skin fungal community structure among bat species. A Generalized Linear Model (GLM) based on a quasi-Poisson distribution and partial distance-based redundancy analysis (db-RDA) was performed to assess the influence of variables on skin fungal communities. Using community construction models to explore the ecological processes driving fungal community changes, t-tests and Wilcoxon tests were used to compare the alpha diversity and species abundance differences between the fungal structure on bat species’ skin and the environmental fungal pool.
Results: We found significant differences in the composition and diversity of skin fungal communities among bat species influenced by temperature, sampling site, and body mass index. Trophic modes and skin fungal community complexity also varied among bat species. Null model and neutral model analysis demonstrated that deterministic processes dominated the assembly of skin fungal communities, with homogeneous selection as the predominant process. Skin fungal communities on bat species were impacted by the environmental fungal reservoir, and actively selected certain amplicon sequence variants (ASVs) from the environmental reservoir to adhere to the skin.
Conclusion: In this study, we revealed the structure and the ecological process driving the skin fungal community across bat species in northern China. Overall, these results broaden our knowledge of skin fungal communities among bat species, which may be beneficial to potential strategies for the protection of bats in China.
Introduction
The skin fungal communities of bats compete with pathogens for epidermal space and nutritional resources, thereby limiting pathogen growth and contributing to the promotion of host health, reproduction, and development (Rusman et al., 2020; Nguyen and Kalan, 2022). When a pathogen upsets this balance, it impairs the host defense mechanisms and can result in death (Grimaudo et al., 2022). Host, environment and pathogens also affect host skin fungal community structure and composition (Whiting-Fawcett et al., 2024). Hence, it is paramount to elucidate the factors influencing host skin fungal community distribution patterns, as well as the selection pressures driving community assembly.
White-nose syndrome (WNS) is a fungal disease of bats caused by Pseudogymnoascus destructans (Pd), which primarily invades bat epidermal tissue during hibernation and has led to a dramatic decline in North America (Hoyt et al., 2020). However, bats in Eurasia do not exhibit significant WNS disease symptoms or mortality (Hoyt et al., 2021). It is speculated that bats have shown resistance to Pd infection in China (Hoyt et al., 2020), which may potentially be related to the roles of symbiotic skin bacterial communities and their metabolites (Li et al., 2022b). Skin fungal communities are critical in maintaining a host’s microecological balance and health due to their unique ecological niche (Harris-Tryon and Grice, 2022). Previous studies have found that bat skin mycobiomes also influence host resistance to Pd infection (Vanderwolf et al., 2021b), and may contribute more to defense against disease than resident bacteria (Martinson et al., 2017). White-nose syndrome restructures bat skin microbiomes (Ange-Stark et al., 2023). Bat skin mycobiomes in the American Southwest vary by host, environment, and feeding behavior (Kearns et al., 2023). The diversity and abundance of skin fungal assemblages on WNS-susceptible bat species is significantly lower than WNS-resistant species (Vanderwolf et al., 2021a). However, the composition and structure of skin fungal communities among bat species in China and the factors that influence them have not been reported.
Fungal community assembly mechanisms and regulatory process have always been a focus of attention in microbial ecology (Li J. et al., 2023). Fungal community assembly may be driven by multiple ecological processes at the same time, with varying relative contributions (Zhu et al., 2024). Neutral theory posits that stochastic processes are crucial to drive microbiomes to convergence or diverge (Coyte et al., 2021), while ecological niche theory suggests deterministic processes such as environmental filtering or selection and species interactions exert more substantial effects (Martinson et al., 2017). Examples of both abound; for instance, fungal pathogen infections alter the selection, spread, and migration processes of amphibian skin microbiota (Wilber et al., 2020), while microbial community assembly in termite mounds is driven by deterministic selection (Chen et al., 2021). Studies have also found that ecological processes varied considerably with host species, diet, and environment (Zhu et al., 2022b). Therefore, quantifying the community assembly processes of bat skin mycobiomes helps to comprehensively understand the structure of fungal communities.
In this study, we selected five widely-distributed bat species across five regions in northern China during the late hibernation period for skin fungal sampling, to clarify the differences in community composition and structure between bat skin and environmental samples, and to explain the processes driving skin fungal community assembly. We had three objectives: (1) to explore the differences in composition, structure, and function of skin fungal communities among bat species in China; (2) to quantify the relative importance of deterministic and stochastic processes in the assembly process of skin fungal communities; and (3) to elucidate the relationship between skin fungal communities and the environmental fungal reservoir, using the species Rhinolophus ferrumequinum and Murina leucogaster.
Materials and methods
Sample collection
We collected skin fungal and environmental samples of five bat species, including Mu. leucogaster (n = 29, MULE), Myotis ricketti (n = 15, MYRI), R. ferrumequinum (n = 64 RHFE), R. pusillus (n = 15, RHPU), and R. macrotis (n = 5, RHMA), from sites known as Bat cave (Beijing), Water channel (Henan Province), Temple cave1 (Liaoning Province), Temple cave2 (Shanxi Province), New cave and Gezi cave (Jilin Province). Environmental samples come from Water channel (n = 6), Temple cave1 (n = 6), New cave (n = 5) and Gezi cave (n = 8). Data was collected at the end of March and early April 2019 (Table 1). All sample collection processes were approved by the Laboratory Animal Welfare and Ethics Committee of Jilin Agricultural University.
Based on previous research, we used sterile polyester swabs dipped in sterile water to swab bat forearms and muzzles (5 times) for Pd detection (Hoyt et al., 2016). Skin fungal samples were collected using a similar method to obtain skin bacterial samples (Li et al., 2022a). Environmental samples were derived from bat roosting cave walls (∼5 cm) and swabs were wiped back and forth five times. These samples were preserved in 500 μL RNA later (TIANGEN, Beijing, China). The roost temperature of bats was recorded with an infrared thermometer (Fluke, Everett, WA, United States). Then, species and sex were identified, and forearm length and weight were measured for calculating the body mass index (BMI = weight/forearm length). Bats were released in caves immediately after sampling.
Molecular methods and sequencing
We extracted DNA from swab samples using the Qiagen DNeasy Blood and Tissue Kit (Qiagen, Hilden, Germany), following the manufacturer’s instructions and examined the presence of Pd using qPCR. The positive control was Pd ATCC MYA-4855 (Ingala et al., 2017). Determined infected (with Ct value) and uninfected (without Ct value) bat individuals based on the Ct values from qPCR results. Pd loads were calculated with the formula: Pd = 10ˆ [(Ct −22.04942)/−3.34789]. For skin fungal samples, DNA extraction was performed using the E.Z.N.A™ Mag-Bind Soil DNA Kit (OMEGA Bio-tek, Georgia, United States) as described in the instructions. We amplified the ITS1 gene from the ITS1 region using the universal fungal-specific primers ITS1F and ITS2R (Sun et al., 2023). The PCR reaction system and conditions were referenced from a previous research (Kearns et al., 2023). PCR products were purified, quantified, and sequenced on the Illumina MiSeq platform (2 × 300 bp) (Shanghai, China).
We amplified 20,665,935 raw sequences from 130 bat samples [average 158,968 reads/sample (range 34,243–846,865)] and 25 environmental samples with 3,134,019 raw sequences [average 125,360 reads/sample (range 62,472–250,905)]. Sequence analysis was performed using Quantitative Insights Into Microbial Ecology (QIIME) 2 (Estaki et al., 2020). The DADA2 plugin (Callahan et al., 2016) was used to correct errors, quality filtering and chimera removal, along with generation of an amplicon sequence variants (ASVs). Taxonomy was assigned to the sequence features using QIIME2’s feature-classifier classify-sklearn plugin, based on the UNITE fungal database version 8.21.
Data analysis
We compared Pd loads among species using Kruskal–Wallis tests. Skin fungal community composition was calculated for each bat species at the phylum and genus levels. The shared and unique ASVs of the five bat skin mycobiomes were determined by UpSet analysis and the use of Venn Diagrams. In order to clarify differences in skin fungal community structure among bat species, we analyzed alpha diversity indices (Shannon diversity and Observation richness) and tested their differences using Kruskal–Wallis test. A generalized linear model (GLM) based on a quasi-Poisson distribution was used to examined the effects of predictor variables on alpha diversity indices, including sites, BMI, temperature, sex, Pd load and Pd infection status. Beta diversity was calculated based on Bray–Curtis distance matrix and plotted using non-metric multidimensional scaling (NMDS) with phyloseq package (McMurdie and Holmes, 2013). Permutational multivariate analysis of variance (PERMANOVA) was implemented to assess differences in beta diversity among bat species using the adonis() function on the vegan package (Oksanen et al., 2015). Subsequently, partial distance-based redundancy analysis (db-RDA) was performed to assess the influence of variables (BMI, temperature, sex, Pd load, and Pd infection status) on skin fungal communities, while controlling for the effects of species and site variables with the anova.cca() function of the vegan package.
Null model analysis was carried out to quantify the relative contributions of ecological processes to the assembly of skin fungal communities among bat species, including drift, selection and dispersal (Zhang et al., 2022). The beta Nearest Taxon Index (βNTI) was calculated with the picante package based on phylogenetic trees (Fouquier et al., 2016) and the relative abundances of ASVs. |βNTI| ≥ 2 indicates that deterministic processes are playing a dominant role in shaping microbial communities, while smaller absolute values (|βNTI| < 2) point to a stronger influence from stochastic processes instead. βNTI was combined with the Bray–Curtis-based Raup-Crick index (RCI) to estimate the important processes shaping skin fungal communities: homogeneous selection (βNTI < −2), heterogeneous selection (βNTI > 2), homogenizing dispersal (RCI < 0.95 and |β NTI| < 2), dispersal limitation (RCI > 0.95 and |βNTI| < 2), and drift (|RCI| < 0.95 and |βNTI| < 2) (Fang et al., 2023). FUNGuild was used to predict the function of bat skin mycobiomes (Nguyen et al., 2016). Through linear discriminant analysis (LDA) size effect (LEfSe) analysis, we determined that skin fungal communities significantly differed among bat species based on LDA > 3.5, p < 0.001. Molecular Ecological Network Analysis Pipeline (MENAP) analysis was conducted to understand the structural characteristics of skin fungal communities including complexity (defined as the number of edges and nodes in the network, http://ieg4.rccc.ou.edu/MENA/). A correlation network was constructed based on Spearman correlation (p < 0.05, correlation coefficient threshold = 0.5) by selecting the top 30 ASVs at the genus level in terms of relative abundances from the results of the LEfSe analysis2 (Gao et al., 2024).
To explore the relationship between the structure of skin fungal communities of bat species and the environmental fungal reservoir, we calculated Shannon diversity and Observation richness, and compared them using t-tests and Wilcoxon tests. We then, visualized the beta diversity and performed PERMANOVA to test for significant differences between bat and environment samples. Procrustes analysis was used to assess the consistency in the composition of bat and environment samples using the Procrustes() function of the vegan package. We also conducted source tracking analysis on bat skin mycobiomes by applying the Fast Expectation–Maximization Microbial Source Tracking (FEAST) method3 (Shenhav et al., 2019), using the FEAST package to calculate the proportion of host fungal taxa derived from the potential environmental fungal reservoir (Knights et al., 2011). Neutral modeling analysis revealed the role of neutrality and deviations from neutrality in shaping skin fungal communities (Zhu et al., 2022a). The fundamental assumption of the neutral model is that the probability of detecting an ASV on skin is proportional to the relative abundance of that ASV in the environmental reservoir. Calculating the occurrence frequency of ASVs falls within the 95% confidence intervals using the Hmisc package (Tong et al., 2019), we categorized ASVs into three groups: neutrally distributed (within the predicted range), over-represented (occurring more frequent than predicted), and under-represented (occurring less frequently than predicted) (Li A. Q. et al., 2023).
Results
Pseudogymnoascus destructans infection
The Pd loads showed no significant differences among the five bat species (Kruskal–Wallis test: Chi-squared = 6.50, p = 0.062) (Supplementary Figure S1A). RHFE had the highest Pd loads (average Pd loads: −4.35) and RHPU had the lowest Pd loads (−5.97). Further analysis revealed significant differences in Pd loads of RHFE among different sites (Kruskal–Wallis: Chi-squared = 11.16, p < 0.05). RHFE had the highest Pd loads at Bat cave (−3.29), while the Pd loads at Water channel were the lowest (−5.97). Simultaneously, MYRI had the highest prevalence (93.75%), while RHPU was the lowest prevalence (28.57%) (Supplementary Figure S1B). The Pd prevalence of RHFE varied across different sites, with the highest prevalence in Bat cave (100.00%), and the lowest prevalence in Temple cave1 (80.00%).
Taxonomic composition of skin fungal communities in bat species
The skin fungal communities of five bat species were mainly composed of four phyla (Supplementary Figure S1C), of which Ascomycota was the most common. Mortierellomycota and Basidiomycota formed the largest taxonomic phylum in RHFE, while Mucoromycota was the largest in MYRI. At the genus level, there were differences in the relative abundance of the five bat skin fungal communities (Figure 1A). The most common genus in MULE was Debaryomyces, while the dominant genus in RHFE was Mortierella. The dominant genus in MYRI was Debaryomyces, while in RHPU it was Alternaria, and in RHMA it was Mortierella. Meanwhile, Venn diagram and UpSet analyses revealed that 104 ASVs (5.65%) were shared among bat species (Figure 1B). RHFE possessed the most unique ASVs (2709), followed by MULE (1110), while RHMA had the lowest (100).
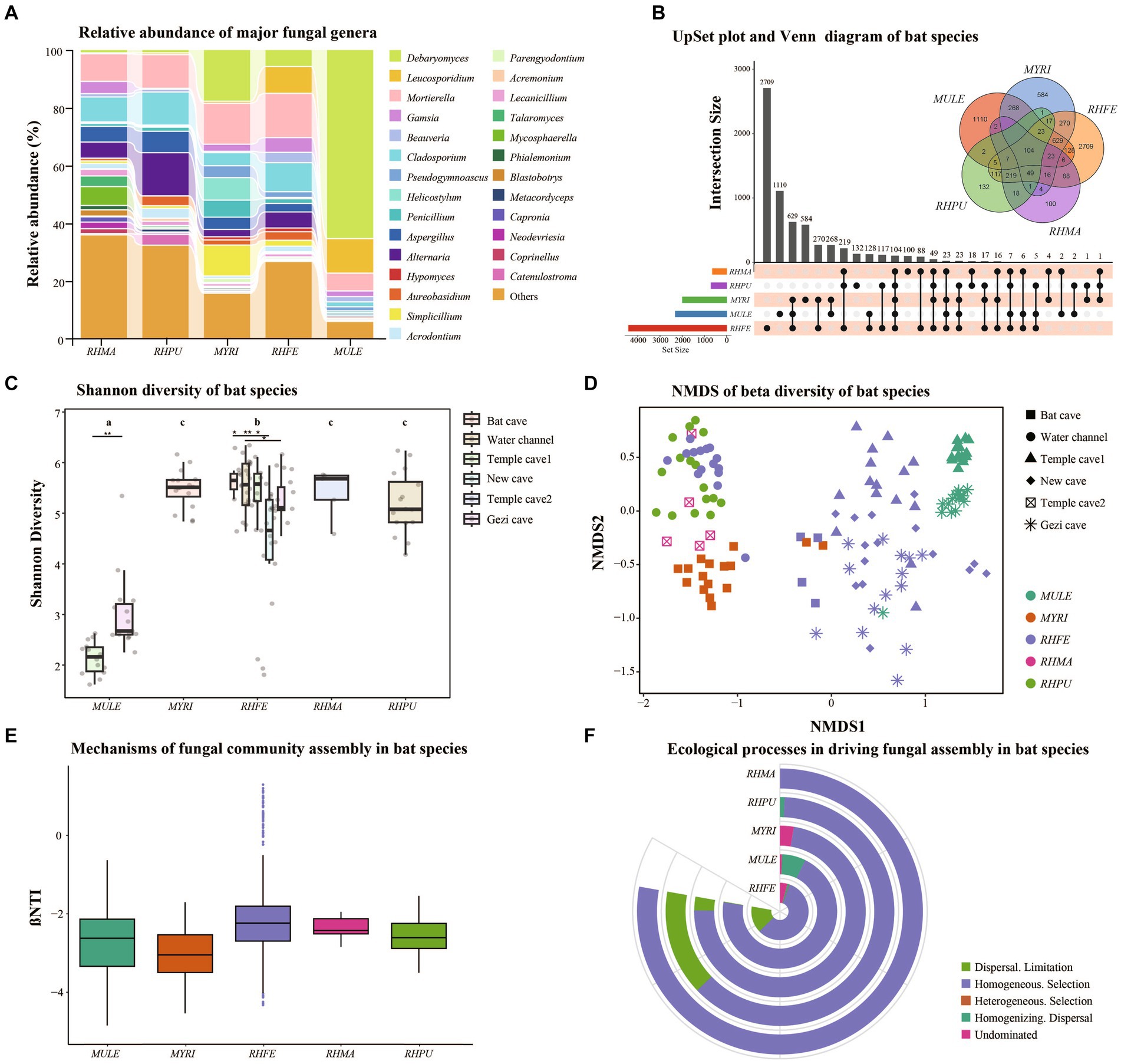
Figure 1. Skin fungal community structure among bat species. (A) Alluvial diagram of major skin fungal taxa with relative abundance greater than 0.01 at the genus level. (B) UpSet plot and Venn diagram of amplicon sequence variants (ASVs) among bat species. (C) Shannon diversity of bat species, with letters and * represent significant differences among groups, *p < 0.05, **p < 0.01. (D) Beta diversity of bat species, based on NMDS plot using Bray–Curtis dissimilarity matrices. (E) Box plot showing the relative contributions of deterministic processes (|βNTI| ≥ 2) and stochastic processes (|βNTI| < 2) in the assembly of bat species. (F) Relative contributions of different ecological processes driving the assembly of bat species.
Structure of skin fungal communities across bat species
Shannon diversity (Figure 1C) and Observation richness (Supplementary Figure S1D) were significantly different between bat species (Shannon diversity: Chi-squared = 57.71, p < 0.001; Observation richness: Chi-squared = 68.11, p < 0.001). Further research clarified significant differences in the alpha diversity of skin fungal communities in RHFE across sites (Shannon diversity: Chi-squared = 65.63, p < 0.001; Observation richness: Chi-squared = 73.76, p < 0.001). In the GLM analysis, it was found that sites (Shannon diversity: Chi-squared = 413.27, p < 0.001; Observation richness: Chi-squared = 83.41, p < 0.001) and temperature (Shannon diversity: Chi-squared = 12.24, p < 0.001; Observation richness: Chi-squared = 39.20, p < 0.001) were the main factors influencing the alpha diversity of skin fungal communities. However, Sex (Shannon diversity: p = 0.067; Observation richness: p = 0.056), BMI (Shannon diversity: p = 0.055; Observation richness: p = 0.051), Pd load (Shannon diversity: p = 0.071; Observation richness: p = 0.079) and infection status (Shannon diversity: p = 0.086; Observation richness: p = 0.071) did not significantly affect alpha diversity.
The NMDS and clustering analyses based on Bray–Curtis dissimilarity (Figure 1D) revealed significant differences in fungal community structure among bat species (NMDS with stress = 0.13; PERMANOVA, Pseudo-F4, 125 = 16.53, p = 0.001, R2 = 0.35). Similarly, significant differences were observed in beta diversity among sites (NMDS with stress = 0.14; PERMANOVA, Pseudo-F5, 124 = 15.91, p = 0.001, R2 = 0.39). Further investigation showed significant differences in beta diversity of RHFE skin mycobiomes across sites (NMDS with stress = 0.14; PERMANOVA, Pseudo-F4, 58 = 8.62, p = 0.001, R2 = 0.37). According to the partial db-RDA analysis, temperature and BMI significantly affected the skin fungal communities (Temperature: Bray–Curtis, PERMANOVA, Pseudo-F1, 128 = 17.25, p = 0.0001, R2 = 0.12. BMI: Bray–Curtis, PERMANOVA, Pseudo-F1, 128 = 3.18, p = 0.0001, R2 = 0.02), when controlling for the effects of sites and bat species. Sex (p = 0.089), Pd load (p = 0.071), and infection status (p = 0.058) did not significantly change the beta diversity of skin fungal communities.
Deterministic vs. stochastic processes in the assembly of skin fungal communities of bat species
Null model analysis indicated that skin fungal community assembly was primarily driven by deterministic processes (|βNTI| > 2), accounting for 87.1% of the ecological processes (Figure 1E). The mechanisms of skin fungal community assembly in bat species were analyzed separately (Figure 1F), and homogeneous selection was the main factor driving community assembly, followed by dispersal limitation and homogenizing dispersal. FUNGuild analysis confirmed the presence of multiple fungal trophic modes in bat skin mycobiomes (Supplementary Figure S1E), which significantly differed among bat species (Figure 2A). Of these, Saprotroph accounted for the largest proportion (16.52%) and was the most abundant in MULE (Kruskal–Wallis: Chi-squared = 87.87, p < 0.001); Symbiotroph accounted for the least amount (2.39%) and was highest in RHPU (Chi-squared = 48.82, p < 0.001). Concurrently, the trophic modes of the skin fungal community in RHFE across sites were also significantly different, with Pathotroph-Symbiotroph being the most marked (Chi-squared = 36.33, p < 0.001).
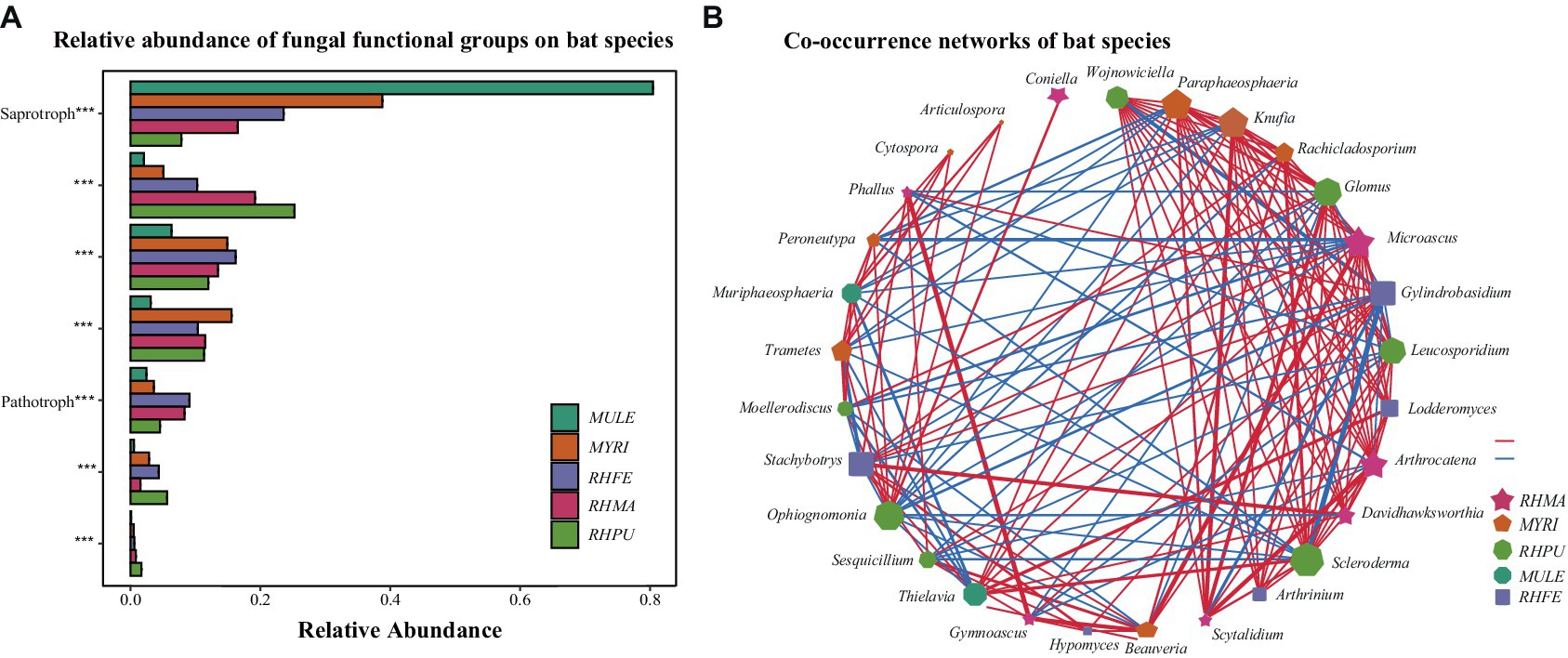
Figure 2. Predicted functional profiles and correlation network of skin fungal communities among bat species. (A) Functional classifications of bat species. * indicates significant differences among groups, ***p < 0.001. (B) Correlation network of five bat species, lines represent Spearman correlations (p < 0.05), with red and blue lines indicating significant negative and positive correlations, respectively, and thicker lines indicating stronger correlations.
LEfSe analysis of skin fungal communities well explained differences between species (LDA > 3.5, p < 0.001) (Supplementary Figure S2). After MENA analysis, it was found that the skin fungal community structure of RHMA was the most complicated (Total nodes = 210, Total links = 767), followed by MYRI (Total nodes = 162, Total links = 415), and the simplest was RHPU (Total nodes = 28, Total links = 27). Correlation networks showed that some taxa were strongly correlated among bat species (Figure 2B), but their functions were also different (Supplementary Table S1).
Relationship between skin fungal community structure and environmental fungal reservoir
Shannon diversity and Observation richness of the skin mycobiome in RHFE was higher than in environmental samples (Shannon diversity: t = 3.83, p = 0.003; Observation richness: t = 4.15, p = 0.001), while the Shannon diversity and Observation richness of skin mycobiomes in MULE were lower than in environmental samples (Shannon diversity: W = 4, p = 0.027; Observation richness: W = 0, p = 0.009) (Figure 3A and Supplementary Figure S1F). Beta diversity indicated that the community structure varied between the environmental fungal reservoir and bat skin mycobiomes (NMDS with stress = 0.10; PERMANOVA, Pseudo-F1, 117 = 16.86, p = 0.001, R2 = 0.05; RHFE: PERMANOVA, Pseudo-F1, 84 = 7.62, p = 0.001, R2 = 0.05; MULE: PERMANOVA, Pseudo-F1, 32 = 15.44, p = 0.003, R2 = 0.34, Figure 3B). Procrustes analysis exhibited good consistency between bat skin mycobiomes and environmental samples (Figure 3C), thus suggesting that the environmental fungal reservoir markedly affected the skin fungal community structure (PROTSET, M2 = 0.5822, p < 0.001).
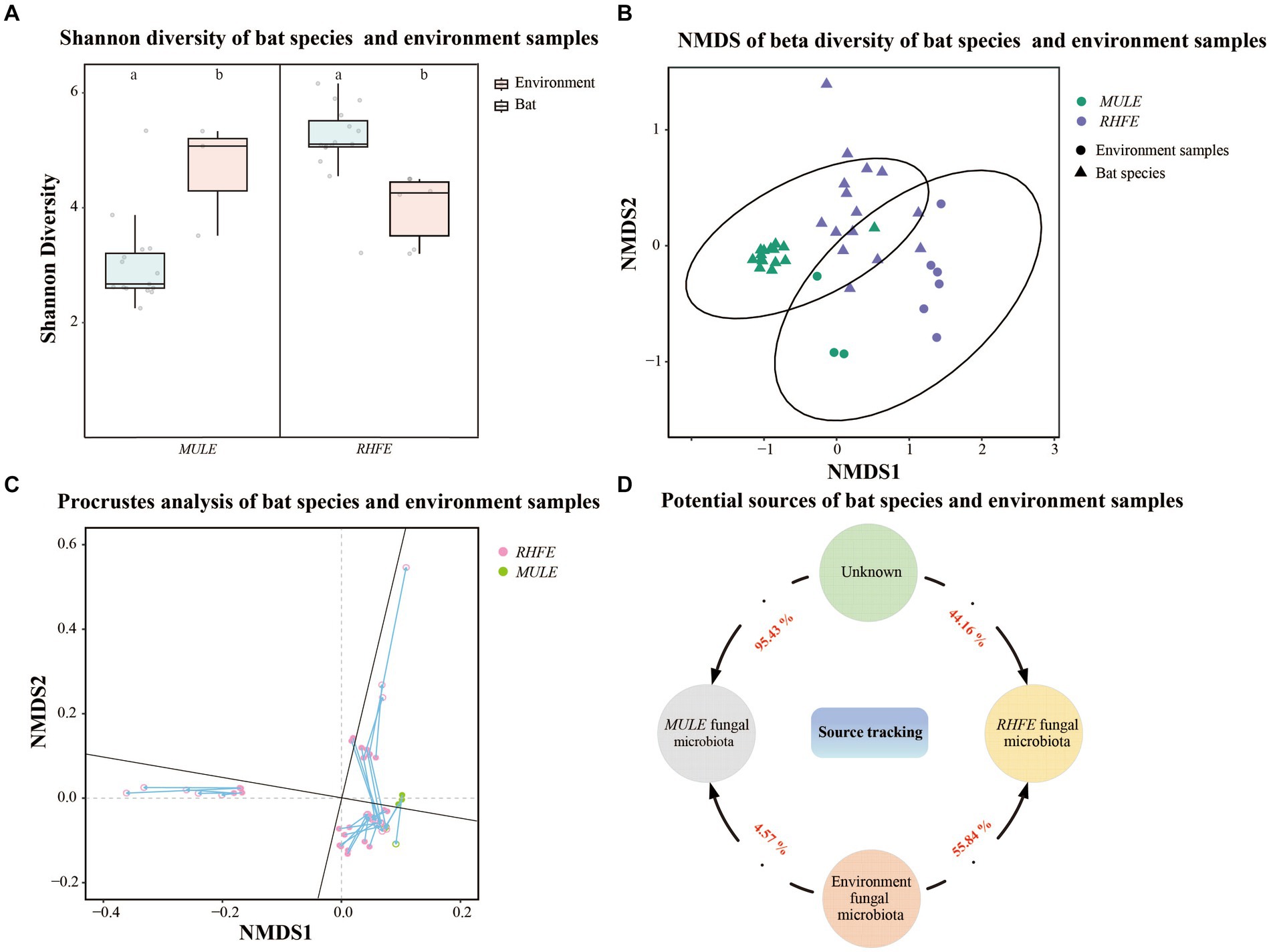
Figure 3. Relationship between bat skin fungal communities and environmental fungal reservoir. (A) Shannon diversity of bat species and environment samples. Letters represent significant differences among groups. (B) Beta diversity of bat species and environment samples. NMDS plot based on Bray-Curtis distance, with ellipses representing 95% confidence intervals (CI). (C) Procrustes analysis of bat species and environment samples. Environmental sample points (solid), skin sample points (hollow). (D) Potential sources of bat species. Numbers next the arrows indicate the proportion of RHFE and MULE potentially originating from the environmental fungal reservoir and unknown sources.
Source tracking analysis proved that RHFE and MULE acquired 55.54 and 4.57% of available environmental fungi, respectively (Figure 3D). Neutral models explored the shared ASVs between bat skin mycobiomes and environmental samples, which 56.32% (green points) and 9.94% of ASVs in RHFE deviated from neutral predictions (red points in Figure 4A), while 42.86% (green points) and 22.03% (red points in Figure 4B) of ASVs in MULE were subjected to positive and negative selection. Of course, neutral selection also accounted for a certain proportion. In RHFE, there were 905 ASVs that fell into the over-represented groups and 142 ASVs into the under-represented categories. For MULE skin mycobiomes, 177 ASVs were divided into the over-represented groups, and 91 ASVs into the under-represented categories (Table 2).
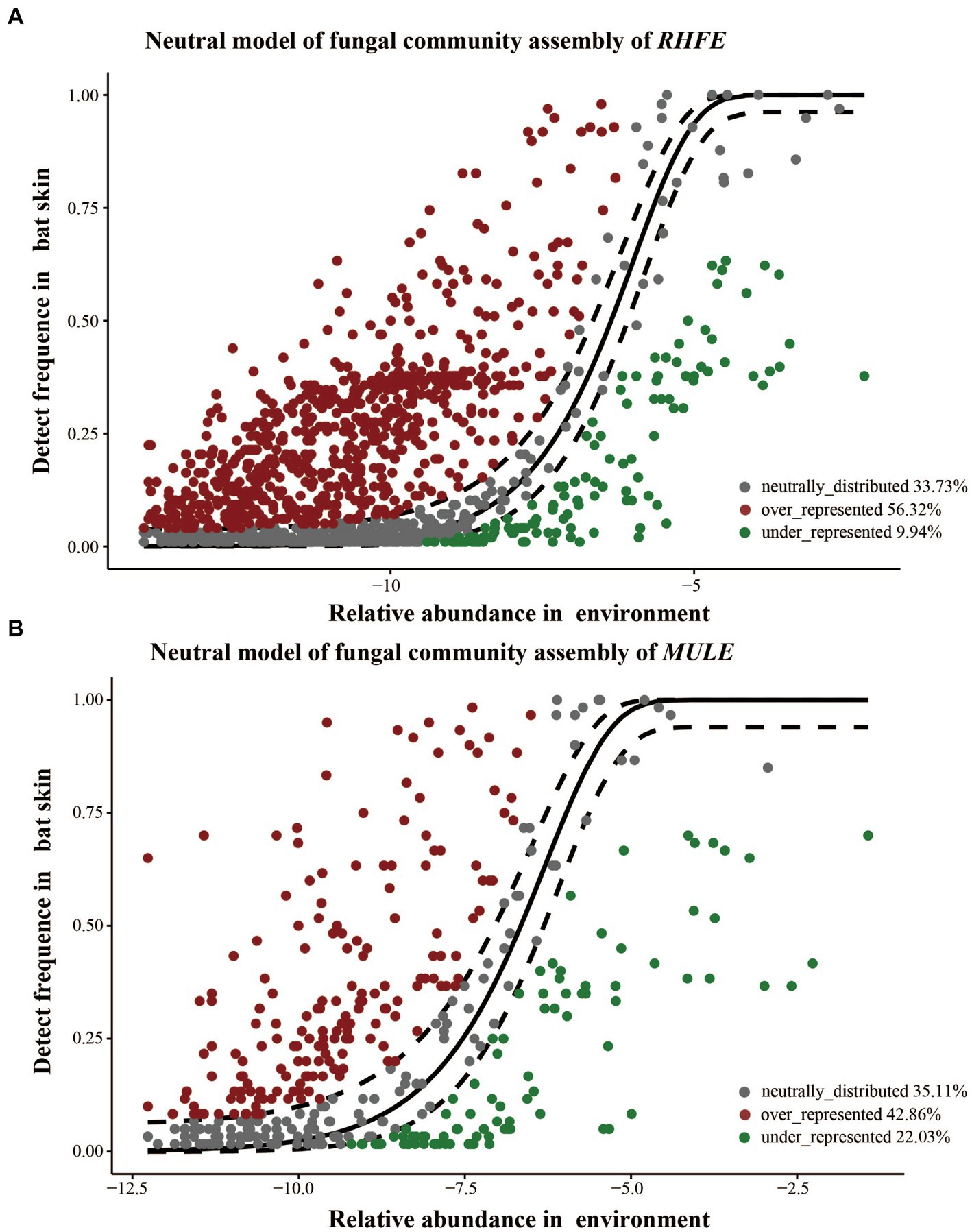
Figure 4. Construction of a neutral model for skin mycobiomes of RHFE (A) and MULE (B) using the environmental fungal reservoir as the source. ASVs that positively deviating from the model expectations are shown as red dots, those negatively deviated from model expectations are represented as green dots, and ASVs with neutral distribution are shown as gray dots. The solid black line represents the 95% confidence interval around the model prediction.
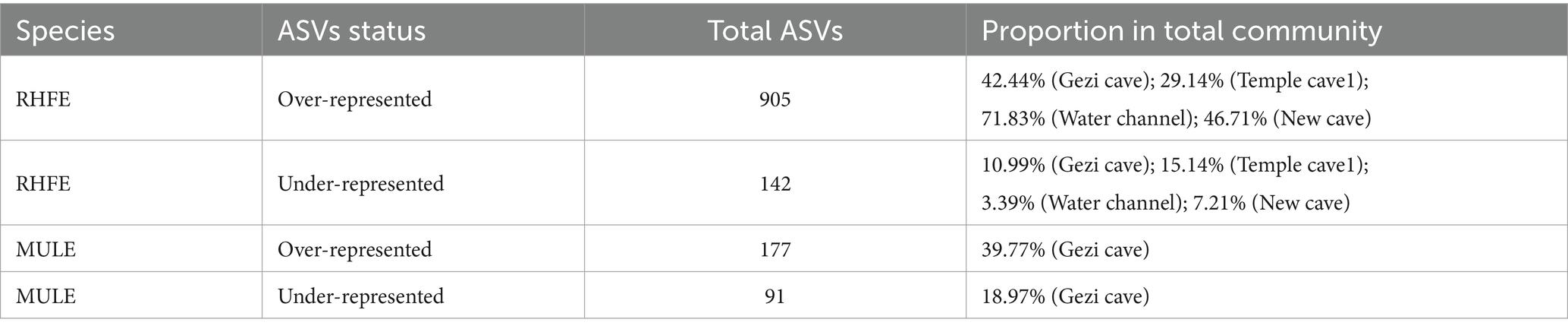
Table 2. Number of over-represented or under-represented ASVs in bat skin fungal communities and the corresponding proportions in the total community.
Discussion
Fungal loads are considered one of the indicators for assessing the transmission risk of WNS, as bats with higher fungal loads exhibit more severe disease symptoms and spread risks (Langwig et al., 2015). This study found no significant difference in Pd loads among five bat species in northern China (Supplementary Figure S1A), which was inconsistent with research results from bat species in North America (Frick et al., 2017). In RHFE, there were significant differences in Pd loads at different locations (Supplementary Figure S1A), perhaps due to the effect of environmental conditions, seasonal variation, and specific behaviors of bat populations on Pd growth (Langwig et al., 2017).
The complexity of fungal community composition can hinder research on microbe-related diseases (Nguyen and Kalan, 2022). These bat skin fungal mycobiomes were mainly composed of five phyla (Supplementary Figure S1B), which are also common in soils worldwide (Schadt and Rosling, 2015), with Ascomycota and Basidiomycota containing mycorrhizal fungi (Peay et al., 2016), and others occupying important ecological niches in animals, including among human, skin fungal communities (Huffnagle and Noverr, 2013). At the genus level, different skin fungal compositions were observed among bat species (Figure 1A), and these Debaryomyces, Leucosporidium, and Penicillium were isolated from the surface of bat skin, with different lifestyles on bat skin. Debaryomyces and Leucosporidium are symbiotic genera of bat skin, which have the ability to resist pathogen invasion (Vanderwolf et al., 2021a), while Penicillium considerably inhibited the growth of Pd (Wilson et al., 2017). Only 5.65% of the skin fungal community was shared among the five bat species (Figure 1B), indicating that species-specific fungal communities dominated. However, RHFE had the highest number of both shared and unique ASVs in the skin fungal community composition among bat species, implying that skin fungal diversity was not the sole pathway regulating disease resistance (Mallon et al., 2015), and thus might be associated with key adaptive cohabiting fungal communities (Siomko et al., 2023).
The MENA analysis identified discrepancies in the complexity of skin fungal communities among bat species (Figure 2B). Skin fungal diversity, richness, and interactions affected the number of network nodes and connections, and we found variation in the composition and trophic modes of skin fungal communities among bat species (Supplementary Figure S2 and Figure 2A). Because fungi with different trophic modes exhibit different functions (SupplementaryFigure S1E) (Qin and Han, 2021), their relative composition may be impacted by various factors such as the ecological niche, environmental conditions, and fungal interactions (Vanderwolf et al., 2013). Subsequently, our analysis disclosed that the interaction patterns of skin fungal communities among bat species were distinct (Figure 2B), possibly representing differences in functional niches (Xun et al., 2019). For example, Arthrinium and Stachybotrys in the skin fungal group of RHFE belong to the Ascomycota and exhibit Pathotroph-Saprotroph, while Phaeosphaeriaceae and Hypomyces belong to the Ascomycota and exhibit Saprotroph (Cannon and Kirk, 2007) (Supplementary Table S1). These fungi were involved in organic matter decomposition and nutrient release, especially Phaeosphaeriaceae, which is extremely important to maintaining soil ecosystem function (Wanasinghe and Maharachchikumbura, 2023). Meanwhile, most Ascomycota were saprophytic fungi, with the optimal pH for growth being 7–8 (Yamanaka, 2003). The alkaline pH of skin enhances the virulence of some fungal pathogens (Vylkova, 2017), as demonstrated in Batrachochytrium dendrobatidis (Kearns et al., 2017).
Studies of the skin fungal diversity of bat are beneficial for biodiversity conservation and the prevention of pathogen transmission (Langwig et al., 2015). We demonstrated huge differences in both alpha diversity (Figure 1C and Supplementary Figure S1D) and beta diversity (Figure 1D) of skin fungal communities, which may be attributed to habitat conditions and ecological characteristics (Vanderwolf et al., 2015). The simultaneous discovery revealed that both site and temperature drove variation in the diversity and structure of skin fungal communities is similar to findings from a study of bat skin bacterial communities (Grisnik et al., 2020). The invasion of fungal pathogens can lead to a decrease in the richness and diversity of host skin microbiota (Allender et al., 2018). However, in our study the Pd load and infection status had no significant effect on the skin fungal diversity of bat species, consistent with other previous research (Vanderwolf et al., 2016). The impact of Pd on the skin microbiota of bat species varied. For example, the presence of Pd did not affect the richness or evenness of fungal taxonomic units in Eptesicus fuscus or M. lucifugus, but affected the evenness in P. subflavus, with Pd-positive bats having a dramatically higher evenness (Ange-Stark et al., 2023). Once again, species and environment were important factors shaping the skin fungal community.
The assembly processes of skin fungal communities are crucial for understanding the adaptability of skin microbiota to environmental changes in ecosystems (Zhou and Ning, 2017). We found that deterministic processes, particularly homogeneous selection, were the main mechanisms regulating the assembly of bat skin mycobiomes (Figure 1E), similar to the assembly processes of microbial communities in agricultural soils and termite mounds in eastern China (Jiao et al., 2020). High levels of homogeneous selection may be influenced by ecological habits, interspecies interactions, and environmental factors, leading to convergence in the composition of skin fungal communities (Fodelianakis et al., 2022). The assembly mechanisms of skin fungal communities also varied among bat species (Figure 1F). For example, in the assembly of skin fungal communities in RHFE, five distinct driving processes were identified, with four showing stochasticity, accounting for 49.55%. This suggests that the assembly mechanisms of skin fungal communities are strongly effected by host selective pressures (Adair and Douglas, 2017), such as, pathogen attack (Holz et al., 2018) and skin physiological characteristics (including pH, temperature, and humidity) (Chen et al., 2018). Additionally, this study found that stochastic processes (homogenizing dispersal, undominated processes, and dispersal limitation) explain a small proportion of skin fungal community diversity across bat species (Figure 1F), highlighting the synergistic effect of multiple assembly process (Gao et al., 2020). Although stochastic processes were essential in the assembly of bat skin fungal communities, deterministic processes remained the primary driving force in assembly.
Environmental factors also influenced skin microbial community structure (Skowron et al., 2021). Procrustes analysis identified a significant correlation between bat skin mycobiomes and adjacent environmental samples (Figure 3C). However, the dramatic differences in the diversity and structure of skin fungal communities among bat species compared to the environmental fungal reservoir (Figures 3A,B), illustrates how the assembly of skin fungal communities could not be entirely mapped onto environmental fungal communities but were filtered by abiotic conditions, species, and diseases (Ange-Stark et al., 2023). Source tracking analysis confirmed that the proportion of skin fungi originating from the environmental fungal reservoir is not high (Figure 3D). Likewise, for many environmental fungal taxa, these characteristics make them more suitable for free-living in environmental media, resulting in low levels of dispersal and selection on host skin (Kearns et al., 2017).
Ecological processes also played a vital role in driving the assembly of skin fungal communities (Li J. et al., 2023). Neutral models illustrated the differences in ecological processes driving the assembly of bat skin mycobiomes (Figure 4) compared to skin bacterial communities (Li A. Q. et al., 2023). Deviations from neutral processes drove the composition of skin fungal communities on bat species, with similar results found in the assembly of microbial communities in diseased lungs (Venkataraman et al., 2015), possibly on account of differences in dormancy strategies between bacteria and fungi, thereby affecting the breadth and composition of community ecology (Chen et al., 2021). ASVs deviating from the neutral model distribution may be well-adapted to hosts or selected by hosts and other environmental pressures (Tong et al., 2019), with the moisture and nutrients of the skin surface potentially favoring the growth of certain microorganisms (Grice and Segre, 2011). Simultaneously, a higher proportion of over-represented fungal taxa (Table 2) suggest that the host could autonomously select beneficial fungi to attach to the skin surface, thereby resisting the adverse effects of the external environment (Chen et al., 2018). For example, haze exposure disturbs the skin fungal community, which promotes the growth of Talaromyces and deviates from neutral assembly process (Yan et al., 2023). Certainly, there were some potential limitations to consider in the interpretation of our study results. For instance, the fit of this neutral model was constrained by assumptions about ecological characteristics, as well as temporal and spatial scales (Morris et al., 2013). Moreover, there remained a considerable amount of unexplained variation in the residual partition, which could attribute to undetected environmental variables and species assemblages. However, the results of both the null and neutral models supported the robustness of our conclusions.
Conclusion
Our research findings showed significant differences in the structure, composition, and trophic modes of skin fungal communities among bat species. Habitat and temperature in particular were significant drivers of changes in the skin fungal community. Using null model analysis to examine the deterministic processes driving the assembly of skin fungal communities, we found evidence for significant differences in the assembly mechanisms among bat species. The diversity and structure of bat skin fungal communities was remarkably different from the environmental fungal reservoir, with the neutral model implying that deviation from neutral processes was the primary driver for the structure of skin fungal communities in RHFE and MULE. These results led to a deeper understanding of structure and quantify the relative importance of assembly processes of skin fungal communities among bat species, enrich the study of skin mycobiomes, understand the interrelationships between host-environment-pathogen-fungal community dynamics, and provide a scientific basis for protecting the health of bat populations.
Data availability statement
All raw sequence data are deposited in the NCBI sequence read archive with accession no. PRJNA1086123.
Ethics statement
The manuscript presents research on animals that do not require ethical approval for their study.
Author contributions
DW: Conceptualization, Data curation, Formal analysis, Investigation, Methodology, Project administration, Resources, Software, Supervision, Validation, Visualization, Writing – original draft, Writing – review & editing. FW: Investigation, Writing – review & editing. ZH: Investigation, Writing – review & editing. AL: Investigation, Writing – review & editing. WD: Investigation, Writing – review & editing. HL: Investigation, Writing – review & editing. LJ: Investigation, Writing – review & editing. ZL: Data curation, Investigation, Writing – review & editing, Conceptualization, Project administration, Resources, Writing – original draft. KS: Investigation, Writing – review & editing, Data curation, Funding acquisition. JF: Investigation, Writing – review & editing.
Funding
The author(s) declare that financial support was received for the research, authorship, and/or publication of this article. This work was supported by the National Natural Science Foundation of China (Grant nos. 32300425, 32171525, 31961123001, and 32171481) and Jilin Provincial Natural Science Foundation (grant numbers YDZJ202401501ZYTS and 20220101291JC).
Conflict of interest
The authors declare that the research was conducted in the absence of any commercial or financial relationships that could be construed as a potential conflict of interest.
Publisher’s note
All claims expressed in this article are solely those of the authors and do not necessarily represent those of their affiliated organizations, or those of the publisher, the editors and the reviewers. Any product that may be evaluated in this article, or claim that may be made by its manufacturer, is not guaranteed or endorsed by the publisher.
Supplementary material
The Supplementary material for this article can be found online at: https://www.frontiersin.org/articles/10.3389/fmicb.2024.1458258/full#supplementary-material
Footnotes
References
Adair, K. L., and Douglas, A. E. (2017). Making a microbiome: the many determinants of host-associated microbial community composition. Curr. Opin. Microbiol. 35, 23–29. doi: 10.1016/j.mib.2016.11.002
Allender, M. C., Baker, S., Britton, M., and Kent, A. D. (2018). Snake fungal disease alters skin bacterial and fungal diversity in an endangered rattlesnake. Sci. Rep. 8:12147. doi: 10.1038/s41598018-30709-x
Ange-Stark, M., Parise, K. L., Cheng, T. L., Hoyt, J. R., Langwig, K. E., Frick, W. F., et al. (2023). White-nose syndrome restructures bat skin microbiomes. Microbiol. Spectr. 11:e0271523. doi: 10.1128/spectrum.02715-23
Callahan, B. J., McMurdie, P. J., Rosen, M. J., Han, A. W., Johnson, A. J. A., and Holmes, S. P. (2016). DADA2: high-resolution sample inference from Illumina amplicon data. Nat. Methods 13, 581–583. doi: 10.1038/nmeth.3869
Chen, Y. E., Fischbach, M. A., and Belkaid, Y. (2018). Skin microbiota-host interactions. Nature 555:543. doi: 10.1038/nature25994
Chen, Q. L., Hu, H. W., Yan, Z. Z., Li, C. Y., Nguyen, B. A. T., Sun, A. Q., et al. (2021). Deterministic selection dominates microbial community assembly in termite mounds. Soil Biol. Biochem. 152:108073. doi: 10.1016/j.soilbio.2020.108073
Coyte, K. Z., Rao, C., Rakoff-Nahoum, S., and Foster, K. R. (2021). Ecological rules for the assembly of microbiome communities. PLoS Biol. 19:e3001116. doi: 10.1371/journal.pbio.3001116
Estaki, M., Jiang, L., Bokulich, N. A., McDonald, D., Gonzalez, A., Kosciolek, T., et al. (2020). QIIME 2 enables comprehensive end-to-end analysis of diverse microbiome data and comparative studies with publicly available data. Curr. Protoc. Bioinformatics 70:e100. doi: 10.1002/cpbi.100
Fang, W. K., Fan, T. Y., Wang, S., Yu, X. K., Lu, A. K., Wang, X. M., et al. (2023). Seasonal changes driving shifts in microbial community assembly and species coexistence in an urban river. Sci. Total Environ. 905:167027. doi: 10.1016/j.scitotenv.2023.167027
Fodelianakis, S., Washburne, A. D., Bourquin, M., Pramateftaki, P., Kohler, T. J., Styllas, M., et al. (2022). Microdiversity characterizes prevalent phylogenetic clades in the glacier-fed stream microbiome. ISME J. 16, 666–675. doi: 10.1038/s41396-021-01106-6
Fouquier, J., Rideout, J. R., Bolyen, E., Chase, J., Shiffer, A., McDonald, D., et al. (2016). Ghost-tree: creating hybrid-gene phylogenetic trees for diversity analyses. Microbiome. 4:11. doi: 10.1186/s40168-016-0153-6
Frick, W. F., Cheng, T. L., Langwig, K. E., Hoyt, J. R., Janicki, A. F., Parise, K. L., et al. (2017). Pathogen dynamics during invasion and establishment of white-nose syndrome explain mechanisms of host persistence. Ecology 98, 624–631. doi: 10.1002/ecy.1706
Gao, C., Montoya, L., Xu, L., Madera, M., Hollingsworth, J., Purdom, E., et al. (2020). Fungal community assembly in drought-stressed sorghum shows stochasticity, selection, and universal ecological dynamics. Nat. Commun. 11:34. doi: 10.1038/s41467-019-13913-9
Gao, Y., Zhang, G., Jiang, S., and Liu, Y. X. (2024). Wekemo Bioincloud: a user-friendly platform for meta-omics data analyses. iMeta 3:e175. doi: 10.1002/imt2.175
Grice, E. A., and Segre, J. A. (2011). The skin microbiome. Nat. Rev. Microbiol. 9, 244–253. doi: 10.1038/nrmicro2537
Grimaudo, A. T., Hoyt, J. R., Yamada, S. A., Herzog, C. J., Bennett, A. B., and Langwig, K. E. (2022). Host traits and environment interact to determine persistence of bat populations impacted by white-nose syndrome. Ecol. Lett. 25, 483–497. doi: 10.1111/ele.13942
Grisnik, M., Bowers, O., Moore, A. J., Jones, B. F., Campbell, J. R., and Walker, D. M. (2020). The skin microbiota of bats has in vitro antifungal activity against this white nose pathogens. FEMS Microbiol. Ecol. 96:fiz193. doi: 10.1093/femsec/fiz193
Harris-Tryon, T. A., and Grice, E. A. (2022). Microbiota and maintenance of skin barrier function. Science 376, 940–945. doi: 10.1126/science.abo0693
Holz, P. H., Lumsden, L. F., Marenda, M. S., Browning, G. F., and Hufschmid, J. (2018). Two subspecies of bent-winged bats (Miniopterus orianae bassanii and oceanensis) in southern Australia have diverse fungal skin flora but not Pseudogymnoascus destructans. PLoS One 13:e0204282. doi: 10.1371/journal.pone.0204282
Hoyt, J. R., Kilpatrick, A. M., and Langwig, K. E. (2021). Ecology and impacts of white-nose syndrome on bats. Nat. Rev. Microbiol. 19, 196–210. doi: 10.1038/s41579-020-00493-5
Hoyt, J. R., Langwig, K. E., Sun, K., Parise, K. L., Li, A., Wang, Y., et al. (2020). Environmental reservoir dynamics predict global infection patterns and population impacts for the fungal disease white-nose syndrome. Proc. Natl. Acad. Sci. USA 117, 7255–7262. doi: 10.1073/pnas.1914794117
Hoyt, J. R., Langwig, K. E., Sung, K. P., Lu, G. J., Parise, K. L., Jiang, T. L., et al. (2016). Host persistence or extinction from emerging infectious disease: insights from white-nose syndrome in endemic and invading regions. Proc. Biol. Sci. 283:20152861. doi: 10.1098/rspb.2015.2861
Huffnagle, G. B., and Noverr, M. C. (2013). The emerging world of the fungal microbiome. Trends Microbiol. 21, 334–341. doi: 10.1016/j.tim.2013.04.002
Ingala, M. R., Ravenelle, R. E., Monro, J. J., and Frank, C. L. (2017). The effects of epidermal fatty acid profiles, 1-oleoglycerol, and triacylglycerols on the susceptibility of hibernating bats to Pseudogymnoascus destructans. PLoS One 12:e0187195. doi: 10.1371/journal.pone.0187195
Jiao, S., Yang, Y. F., Xu, Y. Q., Zhang, J., and Lu, Y. H. (2020). Balance between community assembly processes mediates species coexistence in agricultural soil microbiomes across eastern China. ISME J. 14, 202–216. doi: 10.1038/s41396-019-0522-9
Kearns, P. J., Fischer, S., Fernandez-Beaskoetxea, S., Gabor, C. R., Bosch, J., Bowen, J. L., et al. (2017). Fight fungi with fungi: antifungal properties of the amphibian mycobiome. Front. Microbiol. 8:2494. doi: 10.3389/fmicb.2017.02494
Kearns, P. J., Winter, A. S., Woodhams, D. C., and Northup, D. E. (2023). The mycobiome of bats in the American southwest is structured by geography, bat species, and behavior. Microb. Ecol. 86, 1565–1574. doi: 10.1007/s00248-023-02230-w
Knights, D., Kuczynski, J., Charlson, E. S., Zaneveld, J., Mozer, M. C., Collman, R. G., et al. (2011). Bayesian community-wide culture-independent microbial source tracking. Nat. Methods 8, 761–763. doi: 10.1038/nmeth.1650
Langwig, K. E., Frick, W. F., Reynolds, R., Parise, K. L., Drees, K. P., Hoyt, J. R., et al. (2015). Host and pathogen ecology drive the seasonal dynamics of a fungal disease, white-nose syndrome. Proc. Biol. Sci. 282:20142335. doi: 10.1098/rspb.2014.2335
Langwig, K. E., Hoyt, J. R., Parise, K. L., Frick, W. F., Foster, J. T., and Kilpatrick, A. M. (2017). Resistance in persisting bat populations after white-nose syndrome invasion. Philos. Trans. R. Soc. Lond. Ser. B Biol. Sci. 372:20160044. doi: 10.1098/rstb.2016.0044
Li, Z. L., Li, A. Q., Dai, W. T., Leng, H. X., Liu, S., Jin, L. R., et al. (2022a). Skin microbiota variation among bat species in China and their potential defense against pathogens. Front. Microbiol. 13:808788. doi: 10.3389/fmicb.2022.808788
Li, J., Li, X., and Guo, L. (2023). Research progress on community assembly mechanisms of fungi. Mycosystema 42, 13–25. doi: 10.13346/j.mycosystema.220434
Li, Z. L., Li, A. Q., Hoyt, J. R., Dai, W. T., Leng, H. X., Li, Y. F., et al. (2022b). Activity of bacteria isolated from bats against Pseudogymnoascus destructans in China. Microb. Biotechnol. 15, 469–481. doi: 10.1111/1751-7915.13765
Li, A. Q., Li, Z. L., Leng, H. X., Jin, L. R., Xiao, Y. H., Sun, K. P., et al. (2023). Seasonal assembly of skin microbiota driven by neutral and selective processes in the greater horseshoe bat. Mol. Ecol. 32, 4695–4707. doi: 10.1111/mec.17051
Mallon, C. A., van Elsas, J. D., and Salles, J. F. (2015). Microbial invasions: the process, patterns, and mechanisms. Trends Microbiol. 23, 719–729. doi: 10.1016/j.tim.2015.07.013
Martinson, V. G., Douglas, A. E., and Jaenike, J. (2017). Community structure of the gut microbiota in sympatric species of wild Drosophila. Ecol. Lett. 20, 629–639. doi: 10.1111/ele.12761
McMurdie, P. J., and Holmes, S. (2013). Phyloseq: an R package for reproducible interactive analysis and graphics of microbiome census data. PLoS One 8:e61217. doi: 10.1371/journal.pone.0061217
Morris, A., Beck, J. M., Schloss, P. D., Campbell, T. B., Crothers, K., Curtis, J. L., et al. (2013). Lung HIV microbiome project. Comparison of the respiratory microbiome in healthy nonsmokers and smokers. Am. J. Respir. Crit. Care Med. 187, 1067–1075. doi: 10.1164/rccm.201210-1913OC
Nguyen, U. T., and Kalan, L. R. (2022). Forgotten fungi: the importance of the skin mycobiome. Curr. Opin. Microbiol. 70:102235. doi: 10.1016/j.mib.2022.102235
Nguyen, N. H., Song, Z. W., Bates, S. T., Branco, S., Tedersoo, L., Menke, J., et al. (2016). FUNGuild: an open annotation tool for parsing fungal community datasets by ecological guild. Fungal Ecol. 20, 241–248. doi: 10.1016/j.funeco.2015.06.006
Oksanen, J., Kindt, R., Legendre, P., O'Hara, B., Stevens, M. H. H., Oksanen, M. J., et al. (2015). Vegan community ecology package: ordination methods, diversity analysis and other functions for community and vegetation ecologists.Available at: https://CRAN.R-priject.org/package=vegan
Peay, K. G., Kennedy, P. G., and Talbot, J. M. (2016). Dimensions of biodiversity in the earth mycobiome. Nat. Rev. Microbiol. 14, 434–447. doi: 10.1038/nrmicro.2016.59
Qin, Y., and Han, C. (2021). Prediction and characteristic analysis of secreted protein in different fungi. Acta Microbiol Sin. 61, 4106–4117. doi: 10.13343/j.cnki.wsxb.20210199
Rusman, Y., Wilson, M. B., Williams, J. M., Held, B. W., Blanchette, R. A., Anderson, B. N., et al. (2020). Antifungal Norditerpene Oidiolactones from the fungus Oidiodendron truncatum, a potential biocontrol agent for white-nose syndrome in bats. J. Nat. Prod. 83, 344–353. doi: 10.1021/acs.jnatprod.9b00789
Schadt, C. W., and Rosling, A. (2015). Comment on “global diversity and geography of soil fungi”. Science 348:1438. doi: 10.1126/science.aaa4269
Shenhav, L., Thompson, M., Joseph, T. A., Briscoe, L., Furman, O., Bogumil, D., et al. (2019). FEAST: fast expectation-maximization for microbial source tracking. Nat. Methods 16, 627–632. doi: 10.1038/s41592-019-0431-x
Siomko, S. A., Greenspan, S. E., Barnett, K. M., Neely, W. J., Chtarbanova, S., Woodhams, D. C., et al. (2023). Selection of an anti-pathogen skin microbiome following prophylaxis treatment in an amphibian model system. Philos. Trans. R. Soc. Lond. Ser. B Biol. Sci. 378:20220126. doi: 10.1098/rstb.2022.0126
Skowron, K., Bauza-Kaszewska, J., Kraszewska, Z., Wiktorczyk-Kapischke, N., Grudlewska-Buda, K., Kwiecinska-Piróg, J., et al. (2021). Human skin microbiome: impact of intrinsic and extrinsic factors on skin microbiota. Microorganisms 9:543. doi: 10.3390/microorganisms9030543
Sun, M. L., Shi, C. H., Huang, Y., Wang, H. C., Li, J. J., Cai, L. T., et al. (2023). Effect of disease severity on the structure and diversity of the phyllosphere microbial community in tobacco. Front. Microbiol. 13:1081576. doi: 10.3389/fmicb.2022.1081576
Tong, X. Z., Leung, M. H. Y., Wilkins, D., Cheung, H. H. L., and Lee, P. K. H. (2019). Neutral processes drive seasonal assembly of the skin mycobiome. mSystems 4, e00004–e00019. doi: 10.1128/mSystems.00004-19
Vanderwolf, K. J., Campbell, L. J., Goldberg, T. L., Blehert, D. S., and Lorch, J. M. (2021a). Skin fungal assemblages of bats vary based on susceptibility to white-nose syndrome. ISME J. 15, 909–920. doi: 10.1038/s41396-020-00821-w
Vanderwolf, K. J., Campbell, L. J., Taylor, D. R., Goldberg, T. L., Blehert, D. S., and Lorch, J. M. (2021b). Mycobiome traits associated with disease tolerance predict many western north American bat species will be susceptible to white-nose syndrome. Microbiol. Spectr. 9:e0025421. doi: 10.1128/Spectrum.00254-21
Vanderwolf, K. J., Malloch, D., and McAlpine, D. F. (2015). Fungi associated with over-wintering tricolored bats, Perimyotis subflavus in a White-nose syndrome region of eastern Canada. J. Cave. Karst. Stud. 77, 145–151. doi: 10.4311/2015MB0122
Vanderwolf, K. J., Malloch, D., and McAlpine, D. F. (2016). Fungi on white-nose infected bats (Myotis spp.) in eastern Canada show no decline in diversity associated with Pseudogymnoascus destructans (Ascomycota: Pseudeurotiaceae). Int. J. Speleol. 45, 43–50. doi: 10.5038/1827806X.45.1.1946
Vanderwolf, K. J., McAlpine, D. F., Malloch, D., and Forbes, G. J. (2013). Ectomycota associated with hibernating bats in eastern Canadian caves prior to the emergence of white-nose syndrome. Northeast. Nat. 20, 115–130. doi: 10.1656/045.020.0109
Venkataraman, A., Bassis, C. M., Beck, J. M., Young, V. B., Curtis, J. L., Huffnagle, G. B., et al. (2015). Application of a neutral community model to assess structuring of the human lung microbiome. MBio 6, E02284–E02214. doi: 10.1128/mBio.02284-14
Vylkova, S. (2017). Environmental pH modulation by pathogenic fungi as a strategy to conquer the host. PLoS Pathog. 13:e1006149. doi: 10.1371/journal.ppat.1006149
Wanasinghe, D. N., and Maharachchikumbura, S. S. N. (2023). Exploring the diversity and systematics of Phaeosphaeriaceae: taxonomic novelties from ecologically diverse habitats and their phylogenetic resolution. J. Fungi 9:853. doi: 10.3390/jof9080853
Whiting-Fawcett, F., Blomberg, A. S., Troitsky, T., Meierhofer, M. B., Field, K. A., Puechmaille, S. J., et al. (2024). A Palearctic view of a bat fungal disease. Conserv. Biol. c14265:e14265. doi: 10.1111/cobi.14265
Wilber, M. Q., Jani, A. J., Mihaljevic, J. R., and Briggs, C. J. (2020). Fungal infection alters the selection, dispersal and drift processes structuring the amphibian skin microbiome. Ecol. Lett. 23, 88–98. doi: 10.1111/ele.13414
Wilson, M. B., Held, B. W., Freiborg, A. H., Blanchette, R. A., and Salomon, C. E. (2017). Resource capture and competitive ability of non-pathogenic Pseudogymnoascus spp. and P. Destructans, the cause of white-nose syndrome in bats. PLoS One 12:e0178968. doi: 10.1371/journal.pone.0178968
Xun, W. B., Li, W., Xiong, W., Ren, Y., Liu, Y. P., Miao, Y. Z., et al. (2019). Diversity-triggered deterministic bacterial assembly constrains community functions. Nat. Commun. 10:3833. doi: 10.1038/s41467-019-11787-5
Yamanaka, T. (2003). The effect of pH on the growth of saprotrophic and ectomycorrhizal ammonia fungi in vitro. Mycologia 95, 584–589. doi: 10.1080/15572536.2004.11833062
Yan, D., Li, M., Si, W. H., Ni, S. J., Liu, X., Chang, Y. H., et al. (2023). Haze exposure changes the skin fungal community and promotes the growth of Talaromyces strains. Microbiol. Spectr. 11:e0118822. doi: 10.1128/spectrum.01188-22
Zhang, Y., Shen, F. X., Yang, Y., Niu, M. T., Chen, D., Chen, L. F., et al. (2022). Insights into the profile of the human expiratory microbiota and its associations with indoor microbiotas. Environ. Sci. Technol. 56, 6282–6293. doi: 10.1021/acs.est.2c00688
Zhou, J. Z., and Ning, D. L. (2017). Stochastic community assembly: does it matter in microbial ecology? Microbiol. Mol. Biol. Rev. 81, e00002–e00017. doi: 10.1128/MMBR.00002-17
Zhu, Y. X., Huo, Q. B., Wen, T., Wang, X. Y., Zhao, M. Y., and Du, Y. Z. (2022a). Mechanisms of fungal community assembly in wild stoneflies moderated by host characteristics and local environment. Npj. Biofilms Microbiomes 8:31. doi: 10.1038/s41522-022-00298-9
Zhu, Y., Ma, R. F., Hu, L., Yang, H. Q., Gong, H. Z., and He, K. (2024). Structure variation and assembly of body-wide microbiomes in endangered crested ibis Nipponia nippon. Mol. Ecol. 33:e17238. doi: 10.1111/mec.17238
Zhu, Y. X., Yang, R., Wang, X. Y., Wen, T., Gong, M. H., Shen, Y., et al. (2022b). Gut microbiota composition in the sympatric and diet-sharing Drosophila simulans and Dicranocephalus wallichii bowringi shaped largely by community assembly processes rather than regional species pool. Imeta 1:e57. doi: 10.1002/imt2.57
Keywords: bats, skin fungal community structure, community assembly, driving processes, environmental fungal reservoir
Citation: Wang D, Wang F, Huang Z, Li A, Dai W, Leng H, Jin L, Li Z, Sun K and Feng J (2024) Structure and assembly process of skin fungal communities among bat species in northern China. Front. Microbiol. 15:1458258. doi: 10.3389/fmicb.2024.1458258
Edited by:
Aoyun Li, Huazhong Agricultural University, ChinaReviewed by:
Laura Tipton, James Madison University, United StatesQinlong Dai, Liziping National Nature Reserve, China
Copyright © 2024 Wang, Wang, Huang, Li, Dai, Leng, Jin, Li, Sun and Feng. This is an open-access article distributed under the terms of the Creative Commons Attribution License (CC BY). The use, distribution or reproduction in other forums is permitted, provided the original author(s) and the copyright owner(s) are credited and that the original publication in this journal is cited, in accordance with accepted academic practice. No use, distribution or reproduction is permitted which does not comply with these terms.
*Correspondence: Zhongle Li, bHp5MTUxNDMxNkAxMjYuY29t; Keping Sun, c3Vua3AxMjlAbmVudS5lZHUuY24=