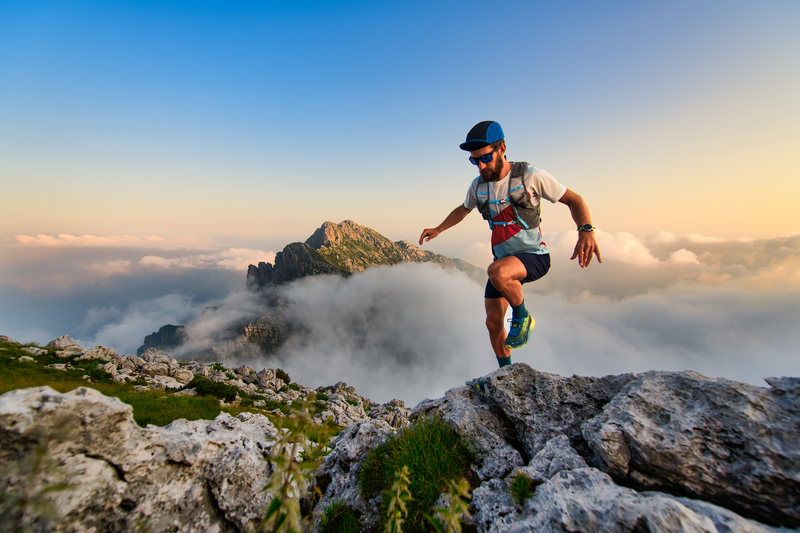
94% of researchers rate our articles as excellent or good
Learn more about the work of our research integrity team to safeguard the quality of each article we publish.
Find out more
ORIGINAL RESEARCH article
Front. Microbiol. , 07 August 2024
Sec. Systems Microbiology
Volume 15 - 2024 | https://doi.org/10.3389/fmicb.2024.1417904
Introduction: Previous research has established associations between alterations in gut microbiota composition and various gynecologic tumors. However, establishing a causal relationship between gut microbiota and these tumors remains necessary. This study employs a two-sample Mendelian randomization (MR) approach to investigate causality, aiming to identify pathogenic bacterial communities potentially involved in gynecologic tumor development.
Methods: Data from the MiBioGen consortium’s Genome-Wide Association Study (GWAS) on gut microbiota were used as the exposure variable. Four common gynecologic neoplasms, including uterine fibroids (UF), endometrial cancer (EC), ovarian cancer (OC), and cervical cancer (CC), were selected as outcome variables. Single-nucleotide polymorphisms (SNPs) significantly associated with gut microbiota were chosen as instrumental variables (IVs). The inverse variance-weighted (IVW) method was used as the primary MR analysis to assess the causal relationship. External validation An was conducted using an independent. Sensitivity analyses were performed to ensure robustness. Reverse MR analysis was also conducted to assess potential reverse causation.
Results: Combining discovery and validation cohorts, we found that higher relative abundance of Lachnospiraceae is associated with lower UF risk (OR: 0.882, 95% CI: 0.793-0.982, P = 0.022). Conversely, higher OC incidence is associated with increased relative abundance of Lachnospiraceae (OR: 1.329, 95% CI: 1.019–1.732, P = 0.036). Sensitivity analyses confirmed these findings’ reliability. Reverse MR analysis showed no evidence of reverse causation between UF, OC, and Lachnospiraceae.
Discussion: This study establishes a causal relationship between Lachnospiraceae relative abundance and both UF and OC. These findings provide new insights into the potential role of gut microbiota in mechanisms underlying gynecological tumors development.
In recent years, the incidence and mortality rates of gynecological tumors have been on the rise, posing a significant threat to women’s health. Among these tumors, uterine fibroids (UF) are the most common. Cervical cancer (CC), endometrial cancer (EC), and ovarian cancer (OC) are the three most prevalent gynecologic malignancies and rank among the top 10 in female cancer incidence (Chen et al., 2016). The occurrence of gynecological tumors is influenced by various factors, including genetics, hormonal imbalances, obesity, persistent HPV infection, and chronic conditions such as endometriosis.
The human microbiome resides in specific body areas and is essential for nutrient absorption, preserving epithelial integrity, detoxification, regulating inflammation and immunity, and defending against pathogens (Baker et al., 2017; Thursby and Juge, 2017). With advances in next-generation sequencing (NGS) technologies, we have gained a deeper understanding of the human microbiome, particularly the gut microbiome, which is dominated by Bacteroidetes and Firmicutes (Russell et al., 2013). A healthy gut microbiota produces short-chain fatty acids (SCFAs) through fermentation, maintaining gut acidity, promoting beneficial bacteria growth, inhibiting pathogen colonization, and promoting epithelial regeneration. In summary, a healthy gut microbiome significantly suppresses chronic inflammation, obesity, metabolic syndrome, and cancer-related diseases (Rinninella et al., 2019).
Microbial communities typically maintain a symbiotic balance within the host. However, several factors can impact the microbiome composition, including medication, obesity, diet, exercise, and genetics (Rinninella et al., 2019). Intestinal dysbiosis, characterized by reduced diversity and stability of gut microbiota, can lead to the overgrowth of harmful bacteria and the production of specific by-products, resulting in immunological and metabolic disturbances. It has been linked to inflammatory bowel disease, diabetes, obesity, metabolic syndrome, and cancer (Gilbert et al., 2018). Recent research has emphasized the connection between gut microbiota and tumors. Previous studies have confirmed the role of microbial dysbiosis in gastrointestinal tumors such as colorectal and liver cancer, as well as tumors outside the digestive tract, including skin, mouth, lung, and reproductive cancers (Sobhani et al., 2019). The influence of microbes on cancer mechanisms is intricate. In gynecological tumors, factors such as modulation of inflammatory responses, DNA damage, impacts on estrogen levels, and production of toxins and metabolites can disrupt the equilibrium of gut microbiota (Borella et al., 2021).
The gut microbiome influences gynecological tumor development. Comparing UF to healthy individuals, we found reduced Bifidobacteria scardovii, Ligilactobacillus saerimneri, and Lactococcus raffinolactis, and increased Pseudomonas stutzeri and Prevotella amnii (Mao et al., 2022). Sims et al. (2019)’s study revealed more Prevotella, Porphyromonas, and Dialister, and decreased Bacteroides, Alistipes, and Lachnospiracea in CC. Zhao identified gut microbiota differences in EC patients, with enriched Ruminococcus as a prognostic biomarkers (Zhao et al., 2022). Proteobacteria and Veillonella were more common in cachexia among breast and ovarian cancer patients (Ubachs et al., 2021). Chambers et al. (2022)’s study demonstrated gut microbiota dysbiosis in OC leading to tumor progression and cisplatin resistance.
However, these findings are primarily from cross-sectional studies and cannot establish causation. Determining causality is crucial for comprehending gynecological tumor development and guiding microbial interventions. We employed Mendelian randomization (MR) analysis to elucidate the causal relationship between gut microbiota and gynecological tumors, utilizing genetic variation as instrumental variables (IVs) to avoid confounding and reverse causality (Chen et al., 2020). This study aims to clarify the connection between gut microbiota and gynecological tumors, providing a theoretical foundation for potential therapeutic strategies.
In this study, we explored the causal relationship between gut microbiota and four common gynecological tumors (UF, EC, OC, and CC) using MR analysis. The gut microbiota was the exposure, SNPs were significantly associated with the exposure, and UF, EC, OC, and CC were the outcomes. We examined both the discovery and validation cohorts of gynecological tumors. The genetic IVs needed to satisfy specific criteria: (1) being significantly associated with the gut microbiome; (2) being independent of all confounding factors except the gut microbiome; and (3) influencing outcomes only through the gut microbiome. Additionally, we performed reverse MR analysis to investigate the causal relationship between these tumors and gut microbiota.
Genetic summary data for gut microbiota were obtained from MiBioGen,1 a large scale, multiethnic GWAS meta-analysis. The study included 18,340 participants from 24 cohorts, with approximately 78% from Europe. We analyzed microbial composition in three different variable regions of the 16S rRNA gene, encompassing 211 taxonomic groups, including 9 phyla, 16 classes, 20 orders, 36 families, and 131 genera. Adjustments were made for age, sex, technical covariates, and genetic principal components.
Genetic summary data for the UF, EC, OC, and CC discovery and validation cohorts were sourced from the IEU Open GWAS Project.2 The data used in this study are publicly available GWAS summary data and have received ethical approval.
To ensure the authenticity and accuracy of conclusions regarding the causal relationship between the gut microbiome and the risk of gynecological tumors, we employed quality control measures to select optimal IVs: (1) SNPs below the genome-wide statistical significance threshold (5 × 10–8) were chosen as IVs, resulting in a limited number of gut microbiota selections. To comprehensively explore the connections between gut microbiota and tumors, we applied a secondary threshold, selecting SNPs below the locus-wide significance level (1 × 10–5) to uncover additional potential causal associations (Sanna et al., 2019). (2) To mitigate bias from linkage disequilibrium (LD) among IVs, we set the LD coefficient to r2 < 0.1 and the region width to 500kb, ensuring independence between SNPs and preventing pleiotropy (Hemani et al., 2018). (3) SNPs were excluded if their P value for the outcome was less than 0.05 (Hartwig et al., 2016). (4) SNPs with inconsistent alleles between exposure and outcome samples (i.e., A/G vs. A/C) were excluded. (5) Palindromic A/T or G/C alleles were also excluded. (6) To assess the strength of the selected SNPs, we calculated the F statistics for each bacterial taxon using the formula:
where R2 is the portion of exposure variance explained by the IVs, n is the sample size, and k is the number of IVs. An F statistic ≥ 10 indicates no strong evidence of weak instrument bias. IVs with F statistics <10 were considered weak and excluded (Burgess and Thompson, 2011).
To investigate causal relationships between the gut microbiome and UF, EC, OC, and CC, we used three regression models: IVW, Weighted Median Estimation (WME), and MR-Egger regression, respectively. The IVW method was the main approach, with the other two methods serving as complementary approaches. The IVW method used the inverse variance of each IV as weights to calculate the summary causal effect estimate. The WME method applied weighted median estimation, requiring at least 50% of valid IVs and ordering SNPs based on their weights before selecting the median as the result (Bowden et al., 2016). The MR-Egger regression estimated the general linear regression model by calculating the correlation coefficients between each SNP and the outcome, as well as between the SNP and the exposure.
We validated the significant bacterial genera identified in the discovery cohort and used the same MR analysis methods in the validation cohort to ensure consistency and reliability of the findings.
We conducted sensitivity analyses to assess the robustness of our results. Firstly, we used Cochran’s Q test to assess SNP heterogeneity, considering a P value below 0.05 indicative of heterogeneity (Bowden et al., 2017). Secondly, we employed MR-Egger regression and MR-PRESSO to detect and address potential horizontal pleiotropy. MR-Egger regression assesses whether genetic instruments exhibit pleiotropic effects on the outcome, although it has lower precision and statistical power compared to MR-PRESSO. MR-PRESSO identifies and mitigates the impact of horizontal pleiotropy through outlier detection and removal. If horizontal pleiotropy was detected among selected SNPs, analyses were repeated after excluding these SNPs (Bowden et al., 2015). Finally, we conducted a leave-one-out sensitivity test to evaluate result robustness. This involved sequentially removing individual SNPs and recalculating MR scores to identify any significant deviations from the overall results, thereby ensuring the reliability of our MR analyses (Hemani et al., 2017).
We performed reverse MR analysis to explore the causal association between gynecologic tumors and significant bacterial genera. SNPs associated with these tumors served as IVs, while UFs, ECs, OCs, and CCs were considered exposures, and bacterial genera were the outcomes.
All statistical analyses were executed using R 4.0.3 software. We employed the “TwoSampleMR” package for IVW, WME, and MR-Egger regression methods, and the “MRPRESSO” package for MR-PRESSO detection.
Figure 1 shows the study flowchart, and details about the datasets in this study are presented in Table 1. After a series of quality controls steps, we identified the following association: UF was linked to 136 SNPs from 12 bacterial taxonomic groups, EC had 55 SNPs associated with 5 bacterial genera, OC exhibited an association with 65 SNPs from 6 bacterial genera, and CC had an association with 95 SNPs from 8 bacterial genera (Supplementary Table 1). The F statistics for the IVs significantly correlated with gut microbiota ranged from 11.701 to 124.169, all exceeding 10. This suggests that the estimates are unlikely to be influenced by weak instrument bias.
Figure 1. The flowchart of the study. MR, Mendelian randomization; UF, uterine fibroids; EC, endometrial cancer; OC, ovarian cancer; CC, cervical cancer.
At the genus level, the genetic prediction results indicated that the risk of UF was positively associated with an increased relative abundance of Bacteroides (OR: 1.178, 95% CI: 1.003–1.383, P = 0.046) and Turicibacter (OR: 1.129, 95% CI: 1.012–1.259, P = 0.029). In contrast, a higher genetically predicted abundance of Enterorhabdus (OR: 0.808, 95% CI: 0.688–0.948, P = 0.009), Lachnospiraceae(OR: 0.882, 95% CI: 0.793–0.982, P = 0.022), and Oscillospira (OR: 0.874, 95% CI: 0.774–0.988, P = 0.031) demonstrated a protective effect against UF. Additionally, there were suggestive causal effects of the phylum Tenericutes, class Mollicutes, order Pasteurellales, and families (Acidaminococcaceae, Bacteroidaceae, Bacteroidales S24 7group, and Pasteurellaceae) on UF.
The relative abundance of the genus Butyrivibrio (OR: 1.083, 95% CI: 1.009–1.163, P = 0.022) significantly increased and was positively associated with the risk of EC. Conversely, higher genetically predicted abundances of the genus Dorea (OR: 0.796, 95% CI: 0.657–0.964, P = 0.020), genus RuminococcaceaeUCG014 (OR: 0.820, 95% CI: 0.686–0.979, P = 0.028), and genus Turicibacter (OR: 0.843, 95% CI: 0.735–0.966, P = 0.014) were negatively correlated with EC risk. Additionally, we observed that a genetically predicted higher abundance of the family Acidaminococcaceae was causally associated with an increased risk of EC (OR: 1.228, 95% CI: 1.018–1.481, P = 0.032).
At the genus level, the genetic prediction results indicated that increased abundances of Barnesiella (OR: 1.395, 95% CI: 1.041–1.869, P = 0.026), Butyrivibrio (OR: 1.219, 95% CI: 1.048–1.418, P = 0.010), and Lachnospiraceae (OR: 1.329, 95% CI: 1.019–1.732, P = 0.036) were linked to a higher risk of OC. Moreover, higher genetically predicted levels of Coprobacter (OR: 0.773, 95% CI: 0.616–0.970, P = 0.026) and RuminococcaceaeUCG010 (OR: 0.644, 95% CI: 0.431–0.962, P = 0.032) were associated with a reduced risk of OC. Genetic prediction of a higher abundance of the phylum Cyanobacteria was significantly associated with an increased risk of ER+ BC (OR: 1.452, 95% CI: 1.132–1.864, P = 0.003).
The risk of CC was negatively correlated with the levels of genus Roseburia (OR: 0.998, 95% CI: 0.996–1.000, P = 0.038). However, two other genera, Lachnospiraceae (OR: 1.002, 95% CI: 1.000–1.004, P = 0.021) and RuminococcaceaeUCG003 (OR: 1.002, 95% CI: 1.001–1.004, P = 0.009), were positively associated with the risk of CC. Furthermore, there were also suggestive causal effects of phylum Euryarchaeota, class Methanobacteria, orders (Bacillales and Methanobacteriales) on UF (Figure 2 and Supplementary Table 2).
Figure 2. Significant MR analysis results in the discovery samples. MR, Mendelian randomization; SNP, single-nucleotide polymorphism; IVW, inverse-variance weighted; OR, odds ratio; CI, confidence interval; UF, uterine fibroids; EC, endometrial cancer; OC, ovarian cancer; CC, cervical cancer.
To further validate the gut microbiota’s association with gynecologic tumors in the discovery cohort, we conducted additional analysis. As shown in Figure 3, the causal relationship between Lachnospiraceae and both UF and OC was consistent with the findings of the discovery cohort, thereby enhancing the credibility of the true causal association (Supplementary Table 3).
Figure 3. Results of the identified bacterial taxa in the replication samples. MR, Mendelian randomization; SNP, single-nucleotide polymorphism; IVW, inverse-variance weighted; OR, odds ratio; CI, confidence interval; UF, uterine fibroids; EC, endometrial cancer; OC, ovarian cancer; CC, cervical cancer.
There is no evidence of heterogeneity among genetic SNPs in Lachnospiraceae (Supplementary Table 4). Neither the MR-Egger test nor the MR-PROSSO test provides evidence of horizontal pleiotropy among SNPs (P > 0.05, Supplementary Tables 5, 6). Furthermore, the leave-out-analysis demonstrates that the causal association between Lachnospiraceae and both UF and OC is not influenced by any single SNP (Supplementary Table 7). The reverse MR analysis shows no evidence of a causal association between UF, OC, and Lachnospiraceae (Table 2). Details of the IVs used in the reverse MR analysis are available in Supplementary Table 8.
To investigate the causal relationship between gut microbiota and four common gynecologic tumors (UF, EC, OC, and CC), we performed a two sample MR analysis. By combining the results from both the discovery and validation cohorts, we confirmed a significant association between Lachnospiraceae and UF and OC. Specifically, we observed a negative correlation between Lachnospiraceae and the risk of UF, as well as a positive correlation with the risk of OC.
The Phylum Firmicutes predominates in the gut microbiota of healthy individuals, and Lachnospiraceae belongs to this family of anaerobic bacteria. Lachnospiraceae has the potential to promote human health by converting primary bile acids into secondary bile acids and producing short-chain fatty acids (SCFAs) such as acetic acid and butyric acid (Sorbara et al., 2020). Research on melanoma patients receiving anti-PD-1 immunotherapy found that a higher abundance of Lachnospiraceae was associated with an improved systemic immune response and a positive response to anti-tumor treatment (McCulloch et al., 2022). In colorectal cancer patients, the relative abundance of Lachnospiraceae is lower compared to control groups, suggesting that Lachnospiraceae may reduce the risk of colorectal cancer and have implications for its prevention and control (Flemer et al., 2018). Studies on gynecological diseases have shown a significantly reduced relative abundance of Lachnospiraceae in patients with polycystic ovary syndrome (PCOS) (Wang et al., 2022). Siddiqui et al. (2022) found a decreased quantity of Lachnospiraceae in CC patients, while another study demonstrated a positive correlation between Lachnospiraceae and persistent HPV infection in CC and a negative correlation with HPV clearance (Ritu et al., 2019). Lachnospiraceae is also associated with the risk of BC, as BC patients have a lower relative abundance of Lachnospiraceae compared to the control group (Di Modica et al., 2021). However, there is currently limited research on the gut microbiota in relation to UF and OC, and no studies have indicated an association between Lachnospiraceae and these gynecological tumors.
Research suggests that dysbiosis of the gut microbiota may be related to an increased risk of gynecological tumors. Specifically, the presence and abundance of Lachnospiraceae are associated with estrogen and its metabolites. This bacterial group regulates β-glucuronidase, impacting endogenous estrogen metabolism, leading to the deconjugation and reabsorption of estrogen into the bloodstream, thereby affecting estrogen levels and activity. A significant positive correlation has been observed between Lachnospiraceae and circulating estrogen levels (Wu et al., 2023). Elevated estrogen levels in women have been associated with an increased risk of UF, as estrogen promotes the growth of uterine smooth muscle cells, facilitating UF proliferation. On the other hand, the gut microbiota and its metabolites may influence the host’s inflammatory status. Studies have reported an association between UF occurrence and persistent inflammation and immune response (Zannotti et al., 2021). Inflammatory mediators like interleukin (IL)-1, IL-4, and tumor necrosis factor (TNF), as well as immune cells like CD4+CD8+ T cells, regulatory T cells (Treg, CD4+), and follicular helper T cells (Tfh), are significantly elevated in UF patients (K et al., 2023). Lachnospiraceae produces SCFAs, which can exert anti-inflammatory and immune-modulatory effects by interacting with the immune system and enhancing intestinal barrier integrity (Ubachs et al., 2021). Our study results indicate that Lachnospiraceae might reduce the risk of UF, suggesting a potential protective role in UF development by influencing inflammation and immune response.
As mentioned, Lachnospiraceae can influence circulating estrogen levels. The development of OC is also correlated with abnormalities in estrogen synthesis and metabolism. A meta-analysis has shown a higher risk of OC in individuals using hormone replacement therapy (HRT) compared to those who do not use HRT, aligning with our findings, which suggest that Lachnospiraceae may increase OC risk by elevating estrogen levels. In addition, the gut-vagina microbiota axis can influence estrogen levels, potentially promoting the onset of estrogen-dependent pathologies such as UF (Takada et al., 2023). Circulating estrogens reach the cells of the vaginal epithelium, stimulating glycogen production, which lactobacilli then metabolize into lactic acid. Typically, Lactobacilli species in the cervicovaginal part of the genital tract play a protective role against OC, which contrasts with our findings. Previous literature has identified members of bacterial, viral, fungal, and parasitic families, suggesting their potential association with cancer initiation and progression (Di Tucci et al., 2023). Parasites infections, such as Trichomonas vaginalis and Schistosoma, as well as viruses like HPV, HIV, and HSV, can alter the microbiota of the female reproductive tract by influencing host immune responses and metabolism. Furthermore, serological studies have linked antibodies against C.trachomatis, such as PGp3 and CHSP60-1, with an increased risk of OC. This association may be mediated by the pathogen’s ability to promote survival in DNA-damaged host cells or facilitate the transfer of tubal- derived cells to the growth-promoting microenvironment within the ovaries (Trabert et al., 2019).
However, other studies indicate that SCFAs produced by Lachnospiraceae exert anti-tumor effects in OC progression. For instance, butyric acid can inhibit histone deacetylase (HDAC) in OC cells, leading to the transition of tumor cells from the S phase to the G0/G1 and/or G2/M phases, thereby increasing tumor cell apoptosis (Borella et al., 2021). It has been reported that the cervicovaginal microbiome is implicated in OC risk (Sheng et al., 2023). Specifically, cervicovaginal microbes containing less than 50% lactobacilli have been significantly associated with OC (Nené et al., 2019). Additionally, vaginal Lachnospiraceae, which produce SCFAs like butyrate by hydrolyzing starch and sugars, show negative correlations with OC development. Despite their potential to promote cancer progression via the induction of regulatory T cells (Tregs), the inhibitory effects of butyrate might outweigh these promotive effects, as butyrate has been shown to interfere with ovarian cancer cell growth in vitro (Chen et al., 2021).
While Lachnospiraceae may play a role in the development of UF and OC, further research is needed to validate the specific mechanisms in these two types of tumors. This will contribute to a better understanding of the underlying mechanisms in gynecological tumor development and provide more targeted strategies for the prevention, detection, and treatment of UF and OC.
This study has several advantages: Firstly, we conducted a comprehensive investigation of four common gynecological tumors. Secondly, we established causal relationships in the discovery cohort and validated them in an independent validation cohort, enhancing the credibility of the identified causal associations. Thirdly, our MR analysis has identified valuable candidate microbial taxa for subsequent functional research, contributing to the development of novel approaches targeting specific gut microbiota for the prevention and treatment of gynecological tumors.
However, our study has some limitations. Firstly, our research primarily utilized GWAS summary data from European populations, with a small portion of gut microbiota data from other ethnic groups. The variability in data sources may impact the accuracy of our results. Secondly, our bacterial classification was analyzed only at the genus level. Thirdly, the development of gynecological tumors results from multifactorial interactions, with the composition of gut microbiota being influenced by both genetic and environmental factors. Therefore, we cannot exclude the potential influence of interactions between diet and genes, or genes and the environment, on the outcomes. Finally, to further validate the functional role of the identified microbiota, we will conduct in vitro experiments to investigate the effects of manipulating the identified microbiota (especially Lachnospiraceae) on gynecological tumors such as uterine fibroids and ovarian cancer cell lines. This will include assessing changes in cell proliferation, migration, and apoptosis. Additionally, we plan to conduct in vivo studies using animal models to evaluate the therapeutic efficacy of targeting these microbiota.
Our study has established the theoretical foundation for investigating the involvement of gut microbiota in the development and treatment of gynecological tumors, particularly UF and OC. However, further research is required to achieve a deeper understanding of the connection between gut microbiota and gynecological tumors. This includes enlarging the sample size, conducting human cohort studies, and undertaking functional research to provide more precise scientific evidence for the prevention and treatment of gynecological tumors.
The original contributions presented in this study are included in this article/Supplementary material, further inquiries can be directed to the corresponding author.
Ethical approval was not required for the study involving humans in accordance with the local legislation and institutional requirements. Written informed consent to participate in this study was not required from the participants or the participants’ legal guardians/next of kin in accordance with the national legislation and the institutional requirements. Written informed consent was obtained from the individual(s) for the publication of any potentially identifiable images or data included in this article.
YX: Writing – original draft, Writing – review and editing. XZ: Conceptualization, Investigation, Writing – review and editing. XN: Software, Writing – review and editing. LZ: Data curation, Writing – review and editing. YS: Validation, Writing – review and editing. AX: Supervision, Writing – original draft.
The author(s) declare that financial support was received for the research, authorship, and/or publication of this article. This study was supported by grants from Major Medical Science and Technology Projects in Henan Province (SBGJ202001006). The funders had no role in the design and conduct of the study, collection, management, analysis, and interpretation of the data; preparation, review, or approval of the manuscript, and decision to submit the manuscript for publication.
We thank the FinnGen consortium, IEU Open GWAS Project, UK Biobank, and MiBioGen consortium for providing GWAS summary statistics data for our analysis.
The authors declare that the research was conducted in the absence of any commercial or financial relationships that could be construed as a potential conflict of interest.
All claims expressed in this article are solely those of the authors and do not necessarily represent those of their affiliated organizations, or those of the publisher, the editors and the reviewers. Any product that may be evaluated in this article, or claim that may be made by its manufacturer, is not guaranteed or endorsed by the publisher.
The Supplementary Material for this article can be found online at: https://www.frontiersin.org/articles/10.3389/fmicb.2024.1417904/full#supplementary-material
Baker, J. M., Al-Nakkash, L., and Herbst-Kralovetz, M. M. (2017). Estrogen-gut microbiome axis: Physiological and clinical implications. Maturitas 103, 45–53. doi: 10.1016/j.maturitas.2017.06.025
Borella, F., Carosso, A. R., Cosma, S., Preti, M., Collemi, G., Cassoni, P., et al. (2021). Gut microbiota and gynecological cancers: A summary of pathogenetic mechanisms and future directions. ACS Infect. Dis. 7, 987–1009. doi: 10.1021/acsinfecdis.0c00839
Bowden, J., Davey Smith, G., and Burgess, S. (2015). Mendelian randomization with invalid instruments: Effect estimation and bias detection through Egger regression. Int. J. Epidemiol. 44, 512–525. doi: 10.1093/ije/dyv080
Bowden, J., Davey Smith, G., Haycock, P. C., and Burgess, S. (2016). Consistent estimation in Mendelian randomization with some invalid instruments using a weighted median estimator. Genet. Epidemiol. 40, 304–314. doi: 10.1002/gepi.21965
Bowden, J., Del Greco, M. F., Minelli, C., Davey Smith, G., Sheehan, N., and Thompson, J. (2017). A framework for the investigation of pleiotropy in two-sample summary data Mendelian randomization. Stat. Med. 36, 1783–1802. doi: 10.1002/sim.7221
Burgess, S., and Thompson, S. G. (2011). Avoiding bias from weak instruments in Mendelian randomization studies. Int J Epidemiol. 40, 755–764. doi: 10.1093/ije/dyr036
Chambers, L. M., Esakov Rhoades, E. L., Bharti, R., Braley, C., Tewari, S., Trestan, L., et al. (2022). Disruption of the gut microbiota confers cisplatin resistance in epithelial ovarian cancer. Cancer Res. 82, 4654–4669. doi: 10.1158/0008-5472.CAN-22-0455
Chen, C., Chen, H., Zhang, Y., Thomas, H. R., Frank, M. H., He, Y., et al. (2020). TBtools: An integrative toolkit developed for interactive analyses of big biological data. Mol. Plant 13, 1194–1202. doi: 10.1016/j.molp.2020.06.009
Chen, L., Zhai, Y., Wang, Y., Fearon, E. R., Núñez, G., Inohara, N., et al. (2021). Altering the microbiome inhibits tumorigenesis in a mouse model of oviductal high-grade serous carcinoma. Cancer Res. 81, 3309–3318. doi: 10.1158/0008-5472.CAN-21-0106
Chen, W., Zheng, R., Baade, P. D., Zhang, S., Zeng, H., Bray, F., et al. (2016). Cancer statistics in China, 2015. CA Cancer J. Clin. 66, 115–132. doi: 10.3322/caac.21338
Di Modica, M., Gargari, G., Regondi, V., Bonizzi, A., Arioli, S., Belmonte, B., et al. (2021). Gut microbiota condition the therapeutic efficacy of trastuzumab in HER2-positive breast cancer. Cancer Res. 81, 2195–2206. doi: 10.1158/0008-5472.CAN-20-1659
Di Tucci, C., De Vito, I., and Muzii, L. (2023). Immune-onco-microbiome: A new revolution for gynecological cancers. Biomedicines 11:782. doi: 10.3390/biomedicines11030782
Flemer, B., Warren, R. D., Barrett, M. P., Cisek, K., Das, A., Jeffery, I. B., et al. (2018). The oral microbiota in colorectal cancer is distinctive and predictive. Gut 67, 1454–1463. doi: 10.1136/gutjnl-2017-314814
Gilbert, J. A., Blaser, M. J., Caporaso, J. G., Jansson, J. K., Lynch, S. V., and Knight, R. (2018). Current understanding of the human microbiome. Nat. Med. 24, 392–400. doi: 10.1038/nm.4517
Hartwig, F. P., Davies, N. M., Hemani, G., and Davey Smith, G. (2016). Two-sample Mendelian randomization: Avoiding the downsides of a powerful, widely applicable but potentially fallible technique. Int. J. Epidemiol. 45, 1717–1726. doi: 10.1093/ije/dyx028
Hemani, G., Tilling, K., and Davey Smith, G. (2017). Orienting the causal relationship between imprecisely measured traits using GWAS summary data. PLoS Genet. 13:e1007081. doi: 10.1371/journal.pgen.1007081
Hemani, G., Zheng, J., Elsworth, B., Wade, K. H., Haberland, V., Baird, D., et al. (2018). The MR-Base platform supports systematic causal inference across the human phenome. eLife 7:e34408. doi: 10.7554/eLife.34408
K, V. K., Bhat, R. G., Rao, B. K., and R, A. P. (2023). The gut microbiota: A novel player in the pathogenesis of uterine fibroids. Reprod. Sci. 30, 3443–3455. doi: 10.1007/s43032-023-01289-7
Mao, X., Peng, X., Pan, Q., Zhao, X., Yu, Z., and Xu, D. (2022). Uterine fibroid patients reveal alterations in the gut microbiome. Front. Cell. Infect. Microbiol. 12:863594. doi: 10.3389/fcimb.2022.863594
McCulloch, J. A., Davar, D., Rodrigues, R. R., Badger, J. H., Fang, J. R., Cole, A. M., et al. (2022). Intestinal microbiota signatures of clinical response and immune-related adverse events in melanoma patients treated with anti-PD-1. Nat. Med. 28, 545–556. doi: 10.1038/s41591-022-01698-2
Nené, N. R., Reisel, D., Leimbach, A., Franchi, D., Jones, A., Evans, I., et al. (2019). Association between the cervicovaginal microbiome, BRCA1 mutation status, and risk of ovarian cancer: A case-control study. Lancet Oncol. 20, 1171–1182. doi: 10.1016/S1470-2045(19)30340-7
Rinninella, E., Raoul, P., Cintoni, M., Franceschi, F., Miggiano, G. A. D., Gasbarrini, A., et al. (2019). What is the healthy gut microbiota composition? A changing ecosystem across age, environment, diet, and diseases. Microorganisms 7:14. doi: 10.3390/microorganisms7010014
Ritu, W., Enqi, W., Zheng, S., Wang, J., Ling, Y., and Wang, Y. (2019). Evaluation of the associations between cervical microbiota and HPV infection, clearance, and persistence in cytologically normal women. Cancer Prev. Res. 12, 43–56. doi: 10.1158/1940-6207.CAPR-18-0233
Russell, W. R., Duncan, S. H., and Flint, H. J. (2013). The gut microbial metabolome: Modulation of cancer risk in obese individuals. Proc. Nutr. Soc. 72, 178–188. doi: 10.1017/S0029665112002881
Sanna, S., van Zuydam, N. R., Mahajan, A., Kurilshikov, A., Vich Vila, A., Võsa, U., et al. (2019). Causal relationships among the gut microbiome, short-chain fatty acids and metabolic diseases. Nat. Genet. 51, 600–605. doi: 10.1038/s41588-019-0350-x
Sheng, D., Yue, K., Li, H., Zhao, L., Zhao, G., Jin, C., et al. (2023). The interaction between intratumoral microbiome and immunity is related to the prognosis of ovarian cancer. Microbiol. Spectr. 11:e0354922. doi: 10.1128/spectrum.03549-22
Siddiqui, R., Makhlouf, Z., Alharbi, A. M., Alfahemi, H., and Khan, N. A. (2022). The gut microbiome and female health. Biology (Basel). 11:1683. doi: 10.3390/biology11111683
Sims, T. T., Colbert, L. E., Zheng, J., Delgado Medrano, A. Y., Hoffman, K. L., Ramondetta, L., et al. (2019). Gut microbial diversity and genus-level differences identified in cervical cancer patients versus healthy controls. Gynecol. Oncol. 155, 237–244. doi: 10.1016/j.ygyno.2019.09.002
Sobhani, I., Bergsten, E., Couffin, S., Amiot, A., Nebbad, B., Barau, C., et al. (2019). Colorectal cancer-associated microbiota contributes to oncogenic epigenetic signatures. Proc. Natl. Acad. Sci. U.S.A. 116, 24285–24295. doi: 10.1073/pnas.1912129116
Sorbara, M. T., Littmann, E. R., Fontana, E., Moody, T. U., Kohout, C. E., Gjonbalaj, M., et al. (2020). functional and genomic variation between human-derived isolates of lachnospiraceae reveals inter- and intra-species diversity. Cell Host Microbe 28, 134–146.e4. doi: 10.1016/j.chom.2020.05.005
Takada, K., Melnikov, V. G., Kobayashi, R., Komine-Aizawa, S., Tsuji, N. M., and Hayakawa, S. (2023). Female reproductive tract-organ axes. Front. Immunol. 14:1110001. doi: 10.3389/fimmu.2023.1110001
Thursby, E., and Juge, N. (2017). Introduction to the human gut microbiota. Biochem. J. 474, 1823–1836. doi: 10.1042/BCJ20160510
Trabert, B., Waterboer, T., Idahl, A., Brenner, N., Brinton, L. A., Butt, J., et al. (2019). Antibodies against chlamydia trachomatis and ovarian cancer risk in two independent populations. J. Natl. Cancer Inst. 111, 129–136. doi: 10.1093/jnci/djy084
Ubachs, J., Ziemons, J., Soons, Z., Aarnoutse, R., van Dijk, D. P. J., Penders, J., et al. (2021). Gut microbiota and short-chain fatty acid alterations in cachectic cancer patients. J. Cachexia Sarcopenia Muscle 12, 2007–2021. doi: 10.1002/jcsm.12804
Wang, Q., Wang, Q., Zhao, L., Bin, Y., Wang, L., Wang, L., et al. (2022). Blood bacterial 16S rRNA gene alterations in women with polycystic ovary syndrome. Front. Endocrinol. 13:814520. doi: 10.3389/fendo.2022.814520
Wu, Z., Pfeiffer, R. M., Byrd, D. A., Wan, Y., Ansong, D., Clegg-Lamptey, J. N., et al. (2023). Associations of circulating estrogens and estrogen metabolites with fecal and oral microbiome in postmenopausal women in the Ghana breast health study. Microbiol. Spectr. 11:e0157223. doi: 10.1128/spectrum.01572-23
Zannotti, A., Greco, S., Pellegrino, P., Giantomassi, F., Delli Carpini, G., Goteri, G., et al. (2021). Macrophages and immune responses in uterine fibroids. Cells 10:982. doi: 10.3390/cells10050982
Keywords: Mendelian randomization, gut microbiota, gynecological tumor, uterine fibroids, ovarian cancer, Lachnospiraceae
Citation: Xiong Y, Zhang X, Niu X, Zhang L, Sheng Y and Xu A (2024) Causal relationship between gut microbiota and gynecological tumor: a two-sample Mendelian randomization study. Front. Microbiol. 15:1417904. doi: 10.3389/fmicb.2024.1417904
Received: 26 April 2024; Accepted: 22 July 2024;
Published: 07 August 2024.
Edited by:
Kaijian Hou, Shantou University, ChinaReviewed by:
George Grant, University of Aberdeen (retired) / now Independent Researcher, Aberdeen, United KingdomCopyright © 2024 Xiong, Zhang, Niu, Zhang, Sheng and Xu. This is an open-access article distributed under the terms of the Creative Commons Attribution License (CC BY). The use, distribution or reproduction in other forums is permitted, provided the original author(s) and the copyright owner(s) are credited and that the original publication in this journal is cited, in accordance with accepted academic practice. No use, distribution or reproduction is permitted which does not comply with these terms.
*Correspondence: Aiguo Xu, YWlndW94dUB6enUuZWR1LmNu
Disclaimer: All claims expressed in this article are solely those of the authors and do not necessarily represent those of their affiliated organizations, or those of the publisher, the editors and the reviewers. Any product that may be evaluated in this article or claim that may be made by its manufacturer is not guaranteed or endorsed by the publisher.
Research integrity at Frontiers
Learn more about the work of our research integrity team to safeguard the quality of each article we publish.