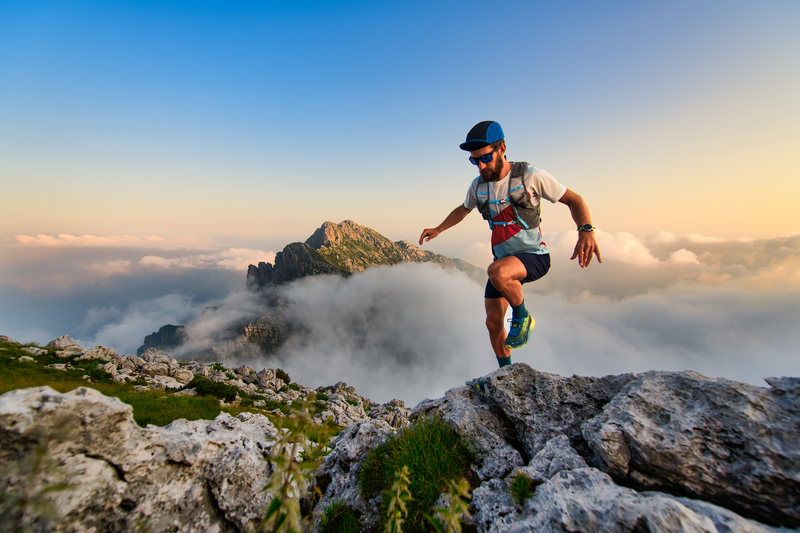
95% of researchers rate our articles as excellent or good
Learn more about the work of our research integrity team to safeguard the quality of each article we publish.
Find out more
ORIGINAL RESEARCH article
Front. Microbiol. , 03 June 2024
Sec. Microorganisms in Vertebrate Digestive Systems
Volume 15 - 2024 | https://doi.org/10.3389/fmicb.2024.1404991
Ruminal methane production is the main sink for metabolic hydrogen generated during rumen fermentation, and is a major contributor to greenhouse gas (GHG) emission. Individual ruminants exhibit varying methane production efficiency; therefore, understanding the microbial characteristics of low-methane-emitting animals could offer opportunities for mitigating enteric methane. Here, we investigated the association between rumen fermentation and rumen microbiota, focusing on methane production, and elucidated the physiological characteristics of bacteria found in low methane-producing cows. Thirteen Holstein cows in the late lactation stage were fed a corn silage-based total mixed ration (TMR), and feed digestion, milk production, rumen fermentation products, methane production, and rumen microbial composition were examined. Cows were classified into two ruminal fermentation groups using Principal component analysis: low and high methane-producing cows (36.9 vs. 43.2 L/DMI digested) with different ruminal short chain fatty acid ratio [(C2+C4)/C3] (3.54 vs. 5.03) and dry matter (DM) digestibility (67.7% vs. 65.3%). However, there were no significant differences in dry matter intake (DMI) and milk production between both groups. Additionally, there were differences in the abundance of OTUs assigned to uncultured Prevotella sp., Succinivibrio, and other 12 bacterial phylotypes between both groups. Specifically, a previously uncultured novel Prevotella sp. with lactate-producing phenotype was detected, with higher abundance in low methane-producing cows. These findings provide evidence that Prevotella may be associated with low methane and high propionate production. However, further research is required to improve the understanding of microbial relationships and metabolic processes involved in the mitigation of enteric methane.
Ruminants have a unique digestive system in their foregut that is beneficial for both the animal and rumen microbiota. Feed carbohydrates are digested by rumen microbes to yield short-chain fatty acids (SCFA) in a process known as rumen fermentation, and is associated with the generation of metabolic hydrogen (Guyader et al., 2017). Enteric methane production is the main sink for metabolic hydrogen and is recognized as a considerable contributor to greenhouse gas (GHG) emission (Janssen, 2010; Ungerfeld, 2013; Wattiaux et al., 2019). Therefore, various approaches have been proposed to mitigate methane emission, including direct inhibition of methanogens with chemical inhibitors, feed manipulation to reduce hydrogen production, and maximization of hydrogen consumption (Belanche et al., 2015; Hristov et al., 2015; Martinez-Fernandez et al., 2018; Granja-Salcedo et al., 2019). A comprehensive understanding of ruminal digestion and function will facilitate the development of a holistic and environmentally friendly approach for sustainable ruminant production (Bocquier and González-García, 2010; Wallace et al., 2019).
Rumen microbiota is considered an important factor affecting methane production and feed efficiency (Shabat et al., 2016; Auffret et al., 2018). As the rumen microbiota is different in high- and low-methane-producing cows, microbial composition analysis is necessary to understand the consistent microbial community structure. This microbial information could promote rumen manipulation for environment-friendly methane mitigation (Wallace et al., 2019).
Previous studies using chamber method have shown that the amount of digested dry matter (DM) is a key factor determining the variation in methane production in lactating Holstein cows (Brask et al., 2015). Precise datasets, including those for methane production, feed digestion, and rumen fermentation, are important for elucidating the microbial and functional characteristics of animals (Hill et al., 2016; Hristov et al., 2018). Kittelmann et al. (2014) reported that an abundance of propionate-producing Quinella and lactate-producing Sharpea in low-methane-producing sheep. Additionally, unidentified Succinivibrionaceae and/or Prevotella spp. have been detected in low methane-producing cows (Wallace et al., 2015; Danielsson et al., 2017). However, phylogenetic community analysis is sometimes insufficient to understand the functional characteristics of rumen microbiota. For instance, several operational taxonomic units (OTUs) assigned to the genera Prevotellaceae, Succinivibrionaceae, and Lachnospiraceae have been linked to low methane-emitting cows. Conversely, other OTUs assigned to the same genus were associated with high-emitting cows (Shabat et al., 2016; Danielsson et al., 2017; Tapio et al., 2017). Notably, a combination of phylogenetic and shotgun metagenomic analyses have been used to determine the functional characteristics of the rumen microbiome in low methane emitting cattle (Wallace et al., 2015; Shabat et al., 2016; Ramayo-Caldas et al., 2020). Moreover, most rumen bacteria are uncultured and physiologically unidentified (Creevey et al., 2014).
Therefore, this study aimed to investigate the association between rumen fermentation and rumen microbiota composition, focusing on methane production, and to elucidate the characteristics of bacteria found in low methane-producing cows to improve the understanding of rumen fermentation.
Thirteen lactating Holstein cows, which were maintained in the animal facility of the National Institute of Livestock and Grassland Science of NARO located in Tsukuba, in Ibaraki Prefecture, Japan, in late lactation stage (average 597 ± 56 kg) were selected for this study and fed total mixed ration (TMR) composed of corn silage and commercial concentrate pellet. The cows were housed individually in an air-conditioned animal facility (20°C, 60% humidity) for 3 weeks and fed TMR twice a day (at 09:45 and 19:00) to meet the energy requirement of the 2006 Japanese feeding standard for dairy cattle. The cows were milked twice daily before each feeding session. During the last 5 d, cows were placed in a whole-body respiration chamber to measure dry matter intake (DMI) and gaseous matter (methane, carbon dioxide, and oxygen) and to collect feces and urine for feed digestibility assay. Details of the respiration chamber system and representative sample processing procedures (feed, feces, and urine) have been previously described (Iwasaki et al., 1982; Shinkai et al., 2012). On the last day, rumen fluid was collected through the mouth before morning feeding using a rumen catheter (Sanshin Industrial, Yokohama, Japan), filtered through three layers of sterilized gauze, and pH was measured (edge, Hanna Instruments Inc., Smithfield, RI, USA). Microorganisms in the filtered rumen fluid (0.5 mL) were collected by centrifugation (14,000 × g, 5 min, 20°C) and stored at −20°C for DNA extraction. Other portions of the filtered rumen fluid were stored at −20 and −80°C for chemical analysis and bacterial isolation.
Rumen fluid stored at −20°C was centrifuged (14,000 × g, 5 min) and used for chemical analysis. SCFA concentrations were determined as described by Suzuki et al. (2021). Ammonia and lactate concentrations were determined spectrophotometrically using commercially available kits for ammonia (Wako Pure Chemical Industries, Osaka, Japan) and D- /L-lactic acid (Megazyme, Wicklow, Ireland), respectively.
Total DNA was extracted from rumen fluid (0.5 mL) using the FastPrep FP100A bead-beating system with a Fast DNA SPIN Kit and Lysing Matrix E (MP Biomedicals, Solon, OH, USA) according to previously described procedures (Mitsumori et al., 2012), and purified using the ethanol precipitation method. DNA concentration was determined using Quant-iT dsDNA HS assay kit (Invitrogen). Thereafter, DNA samples were diluted with nuclease-free water to a concentration of 5 ng and 10 μg/μL for real-time PCR and Illumina sequencing, respectively. Real-time PCR was performed for total bacteria, ciliate protozoa, and methanogen genes (mcrA), and the relative abundances of protozoa and archaea to bacteria were calculated as described previously (Takizawa et al., 2023). For Illumina sequencing, 100 ng of DNA was amplified by PCR. The V3–V4 region of the bacterial 16S rRNA gene was amplified (20 PCR cycles) using KAPA HiFi HotStart ReadyMix (Roche, Basel, Switzerland) and primers (341F, 5′-CCTACGGGNGGCWGCAG-3′; 805R, 5′-GACTACHVGGGTATCTAATCC-3′) (Herlemann et al., 2011). PCR amplicon sequencing was performed on an Illumina MiSeq (Reagent kit v3, Illumina Inc. CA, USA) platform (Bioengineering Lab. Co., Ltd., Kanagawa, Japan). Details of the PCR primers and conditions for archaea, ciliates, and anaerobic fungi have been previously described (Kittelmann et al., 2013). Briefly, the primer sets Ar915aF and Ar1386R, RP841F and Reg1302R, and MN100F and MNGM2 without an adaptor were used for PCR amplification of 16S rRNA genes of archaea, ciliate 18S rRNA genes, and the anaerobic fungal ITS1 region, respectively. Specific amplification was confirmed via electrophoresis. The PCR products for archaea, protozoa, and fungi DNA were quantified as previously described, pooled at a ratio of 1:1:0.2 (Kittelmann et al., 2013), and subjected to Illumina sequencing (MiSeq 250bp × 2; New Zealand Genomics Ltd., Otago, NZ).
Bacterial Illumina sequencing datasets (V3–V4 region of the 16S rRNA gene) were processed and analyzed using the QIIME2 version 2021.4 Quantitative Insights into Microbial Ecology software package (Bolyen et al., 2019). Briefly, primer sequences were trimmed using Cutadapt (Martin, 2011). Forward and reverse reads were merged using VSEARCH (Rognes et al., 2016), and the merged reads with a quality score < 20 were filtered. Using VSEARCH, the reads were dereplicated, clustered into OTUs with 97% similarity, and chimera-checked. The OTUs were assigned using the SILVA database version 138 99% OTUs (Quast et al., 2013), and the taxonomy of OTUs with specifically high abundance in the negative or positive PC1 score group was confirmed by searching against the NCBI rRNA/ITS databases using BLAST software. The mixture of sequence datasets for archaea, protozoa, and fungi was performed as previously described (Caporaso et al., 2010; Kittelmann et al., 2013) using QIIME2 (version 2023.9). As a significant proportion of the sequences obtained could not be merged, sequences over 200 bp in length were truncated such that the average quality score was over 20. Sequences of archaea, protozoa, and fungi were sorted using their respective forward primer sequences as barcodes and processed in the same way as those of bacteria. The databases used were for SILVA database version 138 99% OTUs for archaea and protozoa, and UNITE version 10.0 (Abarenkov et al., 2024) anaerobic fungi, respectively. Sequence data were deposited in DDBJ under accession numbers DRR360991–DRR361003 (DRA013965) for bacteria and DRR374802–DRR374814 (DRA014088) for archaea, protozoa, and fungi.
Two 16S rRNA gene clone libraries were constructed to obtain 16S full-length sequences of OTU218 using 10 ng of DNAs isolated from cows 2011G and 2013Y. 16S rRNA genes were amplified on the TaKaRa Ex Taq HS PCR amplification system using the following primers: 27F (5′- AGAGTTTGATCMTGGCTCAG-3′) and 1492R (5′-GGYTACCTTGTTACGACTT-3′). The PCR conditions were as follows: 25 cycles of denaturation at 98°C (10 s), annealing at 55°C (15 s), and extension at 72°C (30 s). The PCR products were separated electrophoretically on an agarose gel (certified low-melt agarose, Bio-Rad) and purified using a QIAquick Gel Extraction Kit (QIAGEN, Hilden, Germany). Purified PCR amplicons were ligated into the vector pCR2.1 and inserted into One Shot TOP 10 (TA Cloning Kit, Invitrogen). A total of 94 and 91 plasmids for the libraries of 2011G and 2013Y, respectively, were isolated using the QIAprep Spin Miniprep kit (QIAGEN), quantified fluorometrically (Quant-iT dsDNA HS assay kit, Invitrogen), and sequenced by Eurofins Genomics Co., Ltd. (Sanger sequencing, Tokyo, Japan). The obtained sequences were compared with that of OTU218 and the isolated strains.
Prevotella strains, detected as OTU218, were isolated from the rumen of two cows maintained at the National Institute of Livestock and Grassland Science, according to previously described procedures (Shinkai et al., 2022). Briefly, rumen fluid was collected using a rumen catheter, sieved through three layers of surgical gauge, and diluted serially (10-fold) with an anaerobic dilution solution (Bryant and Burkey, 1953). Subsequently, diluted rumen bacteria were inoculated into modified YTR agar medium (Bryant and Burkey, 1953) to form roll tubes with a gas-tight Hungate anaerobic tube. The constituents of the modified YTR agar medium (per liter) include yeast extract, 1.2 g (Oxoid); Bacto peptone, 2 g (Difco); mineral solution I, 75 mL; mineral solution II, 75 mL; clarified rumen fluid, 300 mL; glucose, 1.5 g; cellobiose, 1.5 g; resazurin (0.1%), 10 mL; hemin solution (0.5 g /L), 10 mL; NaHCO3 (8%), 50 mL; L-cysteine hydrochloride, 3 g; bacto agar, 1.2 g (Difco); distilled water, 500 mL. The hemin solution was prepared as follows: 50 mg of hemin was dissolved in 1 ml of 1 N NaOH and made up to 100 ml with distilled water. Colonies that appeared after 48–72 h of incubation at 37°C were isolated. The 16S rRNA gene sequences of the strains were amplified using Takara Ex Taq HS (Takara) with the universal primers 27f and 1492r, purified using ExoSAP-IT (Applied Biosystems), and sequenced (Eurofins Genomics Co., Ltd.). Sequences of the novel Prevotella sp. obtained from Sanger sequencing, 16S rRNA gene clone library, and isolates were aligned with representative rumen bacteria using ClustalW (1.6) and subjected to phylogenetic analysis using the neighbor-joining method with 1,000 bootstrap replications (MEGA X) (Kumar et al., 2018).
The type strains (Prevotella ruminicola B23T (JCM 8259), P. bryantii B14T (DSM 11371), and Butyrivibrio fibrisolvens D1T (JCM 6563) were obtained commercially. The type and isolated Prevotella strains were maintained in a modified YTR slant agar medium under anaerobic conditions with the Hungate tube. For growth monitoring and fermentation product analysis, the strains were grown on a glucose medium (modified PYG medium named DSMZ medium 104 by subtracting beef extract and Tween 80). Active strains were maintained by repetitive subculturing in glucose media as follows; 0.2 mL of bacterial cells at early to mid-log phase (OD660 = 0.3–0.5, OD660 = 0.1 in case of P. ruminicola) were transferred to fresh glucose media (5 mL). Bacterial growth was monitored every 10 min using an OD-Monitor C&T (TAITEC Co. Ltd.) installed in a shaking incubator (120 rpm, Bio-Shaker BR-40LF, TAITEC Co. Ltd.). The fermentation end products were determined after 44–48 h of incubation (stationary phase). The bacterial culture was repeated three times to obtain biological replicates. The organic acid profiles of the fermentation end-products were determined using a Shimadzu HPLC organic acid analysis system (Shimadzu Corp., Kyoto, Japan).
All variables, including methane production, DMI, rumen fermentation parameters, and milk production, were tested for normality and homogeneity using the Shapiro–Wilk normality test and Levene test, respectively. Normally distributed and homogeneous variables were compared using Student's t-test on R Commander (version 2.8-0). Welch's t-test (propionate % and SCFA ratio etc.) or Wilcoxon's rank sum test (ammonia concentration) were adopted based on the results of the normality and homogeneity check. Principal component analysis (PCA) was performed to elucide the interrelationships among rumen fermentation parameters using the prcomp () function in R package (version 3.6.1). The R packages ggbiplot (ver.0.55), and “vqv/ggbiplot” in “devtools” library were used for drawing correlation circle (p = 0.95), and adding normal probability ellipsoids. Rumen fermentation parameters were subjected to hierarchical cluster analysis (hclust) using the UPGMA method based on Z-score normalization, and the squared Euclidean distance was calculated to draw cluster dendrogram and heatmap using R. Spearman's rank correlation analysis was performed to determine the relationship among the data obtained in this study using R, with significance set at p < 0.05. Good's coverage index was employed to check the sequence coverage in Illumina sequences of bacteria, archaea, protozoa, and fungi. To assess the significance of community dissimilarity, non-metric multidimensional scaling (NMDS) based on Bray–Curtis dissimilarity was conducted using the R package vegan (ver. 2.6-2). Statistical significance of the clustering was analyzed using permutational multivariate analysis of variance (PERMANOVA) with the adonis2 function in the vegan package. The proportions of bacteria, archaea, protozoa, and fungi at the family and OTU levels were compared via PC1 score group and subjected to Wilcoxon's rank sum test using the coin package in R coin (version 1.4-2). OTUs that were specifically detected and undetected and that were significantly more and less abundant in the positive PC1 group were investigated. The relative composition of the fermentation products was compared among the strains. The relative proportion data of the pure culture fermentation products were subjected to normality test, Leven's test, and Welch's one-way ANOVA using R Commander, followed by Tukey's multiple comparison test for significant data. Data were considered statistically significant at p < 0.05.
The relationships among the data obtained in this study are shown in Figure 1, and all the collected datasets are listed in Supplementary Table S1. Spearman's rank correlation analysis indicated that DMI was positively correlated with daily methane and milk production, while total ruminal SCFA concentration was negatively correlated with SCFA ratio [(C2+C4)/C3]. However, DMI and methane production per unit of DMI (CH4/DMI) were poorly correlated, indicating that there were variations in both parameters among the cows.
Figure 1. Correlation of rumen fermentation parameters with milk production. The color represents the Spearman's rank correlation coefficient values from −1, indicating a negative correlation (red), to 1, indicating a positive correlation (blue). Asterisks indicate statistical significance at p < 0.05. DMI, dry matter intake; DMdigest, dry matter digestibility; DMId, digested dry matter intake; SCFA, short chain fatty acid; LA, lactic acid; mcrA, mcrA gene; C2C4.C3, (C2+C4)/C3. The dotted line represents the slash.
PCA of rumen fermentation data, including DMI, CH4/DMI, and SCFA ratio, is shown in Figure 2. Approximately 57% of the total variance was explained by the PC1 and PC2. Based on the components of the PC1 and PC2 score data (Supplementary Table S2), the PC1 score was mainly defined by the SCFA ratio and methane production per unit of DMI, while that of PC2 was defined by the input and output amounts (DMI and daily methane production). To group cows based on rumen fermentation data, the PC1 score was used to focus on CH4/DMI and related parameters. Hierarchical clustering based on squared Euclidean distance provided support for grouping the cows based on rumen fermentation parameters (positive PC1 score group: 2011G, 2013Y, 2014R, and 2012 B; negative PC1 score group: the other nine cows) (Supplementary Figure S1).
Figure 2. Principal component analysis (PCA) showing the interrelationship among rumen fermentation parameters. Normal probability ellipsoids for positive (orange) and negative (green) PC1 score groups are shown. DMI, dry matter intake; DMdigest, dry matter digestibility; DMId, digested dry matter intake; SCFA, short chain fatty acid; LA, lactic acid; C2C4.C3, (C2+C4)/C3. Dot represents slash.
Differences between the two PC1 score groups are summarized in Table 1. Specifically, CH4/DMI (25.0 vs. 28.2), CH4/digested DMI (36.9 vs. 43.2), and SCFA ratio (3.54 vs. 5.03) were lower in the positive PC1 score group than in the negative PC1 score group. Additionally, DM digestibility and propionate percentages were higher in the positive PC1 score group than in the negative PC1 score group. However, there were no significant differences in DMI, daily methane production, pH, and milk production between the two groups.
Table 1. Dry matter intake, rumen fermentation, milk production in the positive and negative PC1 score groups.
Heatmap of the rumen fermentation parameters of individual cows is shown in Figure 3. Cluster analysis based on normalized rumen fermentation datasets showed a relationship among the fermentation parameters, with a close relationship observed between DMI and daily methane production. Similarly, methane production per unit of DMI or apparently digested DMI was associated with SCFA ratio [(C2+C4)/C3].
Figure 3. Heatmap of the rumen fermentation parameters by individual cows. A cluster dendrogram was constructed via hierarchical cluster analysis (hclust) using the UPGMA method based on Z-score-normalized data.
Good's coverage index, a parameter of the sequencing depth was 98.937–99.600, 99.318–99.984, 99.588–99.985, and 99.487–99.977 for bacterial, archaeal, protozoan, and fungal sequences, respectively. Bacterial communities of the positive and negative PC1 score groups were significantly different (P = 0.03996). The differences in bacterial composition are shown in Figure 4. At the phyla level, the most abundant rumen bacterial phyla in the positive and negative PC1 score groups were Bacteroidetes (43.2% vs. 41.6%), Firmicutes (33.8% vs. 43.7%), and Proteobacteria (13.8% vs. 4.2 %). At family the level, Prevotellaceae (35.5% vs. 30.4%), Lachnospiraceae (12.2% vs. 15.8%), Succinivibrionaceae (13.4% vs. 3.7%), Ruminococcaceae (5.2% vs. 7.1%), Clostridia_UCG.014 (3.0% vs. 1.6%, P < 0.05), Rikenellaceae (2.8% vs. 4.1%), Christensenellaceae (2.7% vs. 4.7%), Oscillospiraceae (2.5% vs. 4.6%), Saccharimonadaceae (2.5% vs. 1.0%), Acidaminococcaceae (2.3% vs. 2.1%), Muribaculaceae (1.7% vs. 2.7%), family WCHB1.41 (1.5% vs. 2.4%), Spirochaetaceae (1.5% vs. 2.5%), Bacteroidales_RF16_group (1.1% vs. 2.1%), and Erysipelotrichaceae (0.003% vs. 0.015%, p < 0.05) were detected in the positive and negative PC1 score groups. Among 883 bacterial OTUs detected, 40 OTUs, including phyla Bacteroidetes (15 OTUs including Prevotella ruminicola and P. bryantii), Firmicutes (23 OTUs, including Ruminococcus flavefaciens and Ruminococcus bacterium_YRD2003), and Patescibacteria (2 OTUs) were common to both groups. The representative bacterial OTUs in the PC1 score group are shown in Table 2. Prevotella (OTU218, p < 0.1) and Succinivibrionaceae_UCG-001 (OTU851, p < 0.05) and other 12 bacterial OTUs were higher in the positive PC1 score group than in the negative PC1 score group. Specifically, OTU218 and 851 were abundantly detected in cows with positive PC1 scores (Table 2). Full-length 16S rRNA gene sequences were assigned to uncultured Prevotella sp. and uncultured Succinivibrionaceae (56 and 14 clones for OTU218 and OTU851, respectively).
No significant differences in archaeal, protozoal, and fungal communities between the positive and negative PC1 score groups were obtained with the dissimilarity analysis (P = 0.2188, 0.1948, and 0.7842, respectively). Their community compositions are shown in Supplementary Figures S2a–S2c. The most abundant archaeal groups in the positive and negative PC1 score groups were Methanobrevibacter_D_1148 (49.3% vs. 39.2%), followed by Methanobrevibacter_A (36.5% vs. 36.3%), and Unclassified Methanobacteriaceae (7.1% vs. 20.5%). Entodinium and Piromyces were the predominant protozoans and fungi, respectively, in both groups. However, there were significant differences in archaeal, protozoal, and fungal compositions between the two PC1 score groups.
Eleven strains assigned to the sequences of OTU218 and full length SSU clones were isolated from 262 colonies (Supplementary Figure S3). All 11 isolates were classified into the genus Prevotella based on the 16S rRNA gene sequences, with < 89.6% sequence similarity compared with the known Prevotella species. Succinate, lactate, acetate, formate, and malate were detected as fermentation end-products when the strains were grown on glucose (Figure 5). A comparison of the fermentation end products of the 11 isolates and P. ruminicola showed that the novel isolates produced more lactate (26.2% vs. 0%), malate (13.2% vs. 3.4%), and formate (8.2% vs. 5.0%), and less succinate (33.5% vs. 51.1%) and acetate (18.6% vs. 38.3%) than P. ruminicola.
Figure 5. The molar proportion of fermentation end products in the culture of the isolate assigned to OTU218. Succinate (orange), malate (light orange), lactate (yellow), acetate (blue), and formate (light blue) are produced from glucose. Means with different superscripts across the same fermentation products differ significantly at p < 0.05.
Ruminal methane production is a complex process affected by DMI, feed composition, rumen microbiota and their fermentation, lactation stage, host genetics, and the environment (Tapio et al., 2017; Difford et al., 2018; Lyons et al., 2018; Greening et al., 2019). Host genetics research uses spot methane measurement data to obtain animal data (Wallace et al., 2019). Although only a limited number of animals can be tested, respiration chambers can be used to collect accurate methane volumes under fixed feeding management (time and amount), sampling time, and environmental conditions (Lyons et al., 2018). Some studies have used PCA or canonical correspondence analysis and its score rank to classify ruminants according to the microbial type (Kittelmann et al., 2014; Belanche et al., 2015; Danielsson et al., 2017). In the present study, rumen fermentation efficiency and products were evaluated based on PCA of rumen fermentation parameters (Denman et al., 2015). Specifically, the relative values of the SCFA ratio [(C2 + C4)/C3] and methane production per unit of DMI and/or apparently digested DMI were defined by the PCA score (Supplementary Table S2). Hydrogen or metabolic hydrogen generated during rumen fermentation can be consumed by microbes and microbial products, including methane, propionate, and biomass (Ungerfeld, 2020). Methane and propionate syntheses can function as the main hydrogen sinks in the rumen, and a strong negative correlation can be detected between these two processes (Janssen, 2010; Brask et al., 2015; Williams et al., 2019). In the present study, the PC1 score was mainly affected by methane production per unit of apparently digested DMI and SCFA ratios, likely representing a complex hydrogen balance during ruminal fermentation. DMI and methane production contributed more to the PC2 score (Figure 2, Supplementary Table S2), and both factors were positively correlated (Figure 1) (Shibata and Terada, 2010). Additionally, the positive PC1 score group had higher DM digestibility than the negative PC1 score group (Table 1). Previous studies have indicated that feed passage rate, which affects the amount of digested DMI, is an important determinant of variation in methane production among animals (Janssen, 2010; Brask et al., 2015; Kamke et al., 2016).
Methane per unit of digested DMI and DMI were positively correlated with SCFA ratio and negatively correlated with mcrA expression per unit of bacteria (Figure 1). Methanogenic Archaea are closely associated with enteric methane production. The observed association between mcrA expression or the Archaea:Bacteria ratio and methane emission in the present study was consistent with previous findings (Roehe et al., 2016; Auffret et al., 2018). In contrast, weak or no correlation has been observed between mcrA expression or the Archaea:Bacteria ratio and methane emission (Kittelmann et al., 2014). Methanogenesis, methanogenic populations, and acetate:propionate ratios are highly correlated at low pH owing to the high sensitivity of methanogens to acidic conditions (Lana et al., 1998). Similarly, protozoan populations are sensitive to low pH. Considering the pH values and microbial abundance observed in this study, cows may maintain normal pH conditions under strict feeding management. Fauna-free sheep have been reported to produce lower amount of methane (Belanche et al., 2015). A meta-analysis revealed that 21% of experiments reported variations in methane emissions without changes in protozoa numbers, whereas methane mitigation with protozoa reduction was reported in lipid-fed animals (Guyader et al., 2014). Therefore, it could be speculated that the protozoan population may not have directly affected methane production in the present study, but indirectly regulated it by changing the SCFA ratio (Figure 1).
Additionally, differences were observed in rumen bacterial composition between the positive PC1 and negative PC1 groups (Figure 4). At the family revel, Erysipelotrichaceae had a higher abundance in the positive PC1 score group (0.003% vs. 0.015%, p < 0.05) than in the negative PC1 score group. The family Erysipelotrichaceae includes lactate producers, represented by Sharpea, which is abundant in low-methane-emitting sheep (Kamke et al., 2016). However, Erysipelotrichaceae abundance seemed to have little impact on rumen fermentation in the positive PC1 score group. OTU clustering analysis identified PC1 group-specific bacterial OTUs (Table 2), among which Prevotella OTUs were detected in both the positive and negative PC1 score groups (OTUs 469, 430, 218, 512, 227, 186, and 433), which was consistent with previous findings (Danielsson et al., 2017; Liu et al., 2017). Various OTUs of the genus Prevotella have shown negative or positive correlations with methane production, rumen fermentation, feed efficiency, milk, and milk fat yields (Tapio et al., 2017; Schären et al., 2018). Ruminal Prevotella is associate with propionate production and play an important role in ruminal metabolism by utilizing non-cellulosic plant polysaccharides and starches (Denman et al., 2015; Accetto and Avguštin, 2019; Betancur-Murillo et al., 2022). Moreover, species of the genus Prevotella (>60 species) show considerable variation in substrate preference and function (Mizrahi and Jami, 2018; Accetto and Avguštin, 2019). Although several phylogenetically different Prevotella OTUs have been detected in the rumen of cows with high and low methane emissions, it is difficult to understand the functional differences between these OTUs because most are uncultured (Danielsson et al., 2017; Liu et al., 2017; Mizrahi and Jami, 2018).
The isolates assigned to OTU218 were abundantly detected in the positive PC1 score group (0.01% vs. 15.48%, p < 0.1). Moreover, OTU218 is a phylogenetically novel species based on the 16S rRNA sequencing. Therefore, we compared OTU218 to other Prevotella species based on phylogenetic, physiological, and genomic analyses, and named it Prevotella lacticifex (Shinkai et al., 2022). The details of the genomic information of P. lacticifex can be found in GenBank/EMBL/DDBJ (accession No. LC639953 through LC639963 for the 16S rRNA gene and BioProject accession No. PRJDB12066 for the whole genome). Unlike other ruminal Prevotella species (P. ruminicola and P. bryantii), P. lacticifex produces significant amounts of lactic acid from glucose (Figure 5). The profiles of the fermentation end-products of P. ruminicola, P. bryantii, and Butyrivibrio fibrisolvens were similar to those reported in previous studies, except for one study that reported propionate production by P. ruminicola (Emerson and Weimer, 2017; Seshadri et al., 2018). Lactic acid production and utilization are characteristic traits of low-methane emitters, and two types of microbiota with low methane yields have been reported (Kittelmann et al., 2014). Kamke et al. (2016) found that L-(+)-lactate dehydrogenase genes associated with lactate formation from pyruvate were highly abundant in low-methane emitting sheep, and it was concluded that the increased lactate production and the corresponding increase in lactate conversion to butyrate resulted in a decrease in methane production. Similar microbiome differences were observed in low-methane-emitting dairy cows (Shabat et al., 2016). Propionate precursors have been evaluated as alternative electron acceptors for methanogenesis during rumen fermentation (Newbold et al., 2005). P. lacticifex isolates produce propionate precursors, including succinate, malate, and lactate. Lactate may be generated by lactate utilizers detected the in positive PC1 score group, such as the families of Selenomonadaceae (p < 0.05) and Saccharimonadaceae, a possible lactate utilizer formerly known as TM7 (Bor et al., 2019). In a previous study, it was found that Prevotella, but not P. lacticifex and Selenomonas sp. contributed to lactate conversion to succinate via the randomizing succinate pathway (Denman et al., 2015). A study on hydrogen production and consumption indicated that the consumption of hydrogen by [NiFe]-hydrogenases of methanogens and selenomonads in sheep suggests the importance of selenomonads in direct hydrogen consumption (Greening et al., 2019). Based on these findings, the abundance of the propionate precursor-producing P. lacticifex and its utilizers in the positive PC1 score group contributed to the higher propionate production and lower methane production observed in the present study.
Furthermore, a relationship between enteric methane production and uncharacterized Succcinivibrionaceae as well as Prevotella spp. has been suggested in both dairy and beef cattle (Wallace et al., 2015; Danielsson et al., 2017; Tapio et al., 2017). The family Succinivibrionaceae includes starch fermenters such as S. dextrinosolvens, Ruminobacter amylophylus, and a strain isolated from the tammar wallaby that produces succinate and acetate (Pope et al., 2011). Moreover, an uncharacterized Succinivibrionaceae, which is related to low methane production, is yet to be cultured and identified. Liu et al. (2017) reported a strong correlation between Prevotella and Methanobrevibacter in young Heifers (9–10 months), which was replaced by a correlation between Succinivibrio and Methanobrevibacter in older cows (96–120 months). A co-culture study demonstrated that the specific interaction between S. dextrinosolvens H5 and methanogens enhanced the growth of Methanomassillicoccales but inhibited Methaobrevibacter gottschalkii growth (Kamke et al., 2017). A negative correlation exists between uncharacterized Succcinivibrionaceae and methanogens (McCabe et al., 2015); therefore, further studies are necessary to elucidate these interdomain interactions to improve the understanding of enteric methane production.
This study attempted to identify novel rumen microbes associated with low methane emission in Holstein cows. Cows with low methane and high propionate production showed higher DM digestibility and total SCFA concentration without affecting DMI and milk production. Additionally, there were significant differences in the abundance of OTUs assigned to uncultured Prevotella sp., Succinivibrio, and other 12 bacterial phylotypes between low methane and high methane emitting cows. Moreover, a previously uncultured novel Prevotella sp. with a lactate-producing phenotype was detected, with a higher abundance in low methane emitting cows. Overall, this study provides evidence that Prevotella may be related to low methane and high propionate-producing cows. However, further research is required to understand the microbial relationships and metabolic processes involved in the mitigation of enteric methane.
All data generated or analyzed during this study are included in this published article and its Supplementary information files. Sequence data are deposited in DDBJ under accession numbers DRR360991 through DRR361003 (DRA013963 through DRA013975) for bacteria and DRR3748002 through DRR374814 (DRA014088) for archaea, protozoa, and fungi. The type strain of Prevotella lacticifex was obtained from JCM and DSM (JCM 34664 and DSM 112675, respectively).
The animal study was approved by the National Agricultural and Food Research Organization. The study was conducted in accordance with the local legislation and institutional requirements.
TS: Conceptualization, Data curation, Formal analysis, Funding acquisition, Investigation, Methodology, Project administration, Validation, Visualization, Writing – original draft, Writing – review & editing. ST: Data curation, Formal analysis, Investigation, Methodology, Validation, Visualization, Writing – review & editing. OE: Conceptualization, Data curation, Formal analysis, Funding acquisition, Investigation, Methodology, Project administration, Resources, Validation, Writing – review & editing. KH: Data curation, Formal analysis, Investigation, Methodology, Validation, Writing – review & editing. HO: Data curation, Formal analysis, Methodology, Resources, Validation, Writing – review & editing. MM: Conceptualization, Data curation, Funding acquisition, Investigation, Methodology, Project administration, Writing – review & editing.
The author(s) declare financial support was received for the research, authorship, and/or publication of this article. This study was supported in part by the Cabinet Office, Government of Japan, Cross-ministerial Moonshot Agriculture, Forestry and Fisheries Research and Development Program, “Technologies for Smart Bio-industry and Agriculture” (No. JPJ009237), and the Ministry of Agriculture, Forestry, and Fisheries of Japan, via the commissioned project study named “Development of the climate change mitigation technology in the livestock sector”.
This research was technically supported by AgResearch for community analysis of archaea, protozoa, and fungi. We are grateful to Drs. Christina Moon (AgResearch), Sinead Leahy (New Zealand Agricultural Greenhouse Gas Research Centre), and Dragana Gagic (Massey University) for the amplicon sequencing.
The authors declare that the research was conducted in the absence of any commercial or financial relationships that could be construed as a potential conflict of interest.
All claims expressed in this article are solely those of the authors and do not necessarily represent those of their affiliated organizations, or those of the publisher, the editors and the reviewers. Any product that may be evaluated in this article, or claim that may be made by its manufacturer, is not guaranteed or endorsed by the publisher.
The Supplementary Material for this article can be found online at: https://www.frontiersin.org/articles/10.3389/fmicb.2024.1404991/full#supplementary-material
Abarenkov, K., Zirkx, A., Piirmann, T., Pöhönen, R., Ivanov, F., Nilsson, R. H., et al. (2024). UNITE QIIME release for Fungi. Version 04.04.2024. UNITE Community.
Accetto, T., and Avguštin, G. (2019). The diverse and extensive plant polysaccharide degradative apparatuses of the rumen and hindgut Prevotella species: A factor in their ubiquity? Syst. Appl. Microbiol. 42, 107–116. doi: 10.1016/j.syapm.2018.10.001
Auffret, M. D., Stewart, R., Dewhurst, R. J., Duthie, C. A., Rooke, J. A., Wallace, R. J., et al. (2018). Identification, comparison, and validation of robust rumen microbial biomarkers for methane emissions using diverse Bos Taurus breeds and basal diets. Front. Microbiol. 8:2642. doi: 10.3389/fmicb.2017.02642
Belanche, A., de la Fuente, G., and Newbold, C. J. (2015). Effect of progressive inoculation of fauna-free sheep with holotrich protozoa and total-fauna on rumen fermentation, microbial WSSdiversity, and methane emissions. FEMS Microbiol. Ecol. 91:fiu026. doi: 10.1093/femsec/fiu026
Betancur-Murillo, C. L., Aguilar-Marín, S. B., and Jovel, J. (2022). Prevotella: a key player in ruminal metabolism. Microorganisms 11:1. doi: 10.3390/microorganisms11010001
Bocquier, F., and González-García, E. (2010). Sustainability of ruminant agriculture in the new context: feeding strategies and features of animal adaptability into the necessary holistic approach. Animal 4, 1258–1273. doi: 10.1017/S1751731110001023
Bolyen, E., Rideout, J. R., Dillon, M. R., Bokulich, N., Abnet, C. C., Al-Ghalith, G. A., et al. (2019). Reproducible, interactive, scalable and extensible microbiome data science using QIIME 2. Nat. Biotechnol. 37, 852–857. doi: 10.1038/s41587-019-0209-9
Bor, B., Bedree, J. K., Shi, W., McLean, J. S., and He, X. (2019). Saccharibacteria (TM7) in the human oral microbiome. J. Dent. Res. 98, 500–509. doi: 10.1177/0022034519831671
Brask, M., Weisbjerg, M. R., Hellwing, A. L. F., Bannink, A., and Lund, P. (2015). Methane production and diurnal variation measured in dairy cows and predicted from fermentation pattern and nutrient or carbon flow. Animal 9, 1795–1806. doi: 10.1017/S1751731115001184
Bryant, M. P., and Burkey, L. A. (1953). Cultural methods and some characteristics of some of the more numerous groups of bacteria in the bovine rumen. J. Dairy Sci. 36, 205–217. doi: 10.3168/jds.S0022-0302(53)91482-9
Caporaso, J. G., Kuczynski, J., Stombaugh, J., Bittinger, K., Bushman, F. D., et al. (2010). QIIME allows analysis of high-throughput community sequencing data. Nat. Methods 7, 335–336. doi: 10.1038/nmeth.f.303
Creevey, C. J., Kelly, W. J., Henderson, G., and Leahy, S. C. (2014). Determining the culturability of the rumen bacterial microbiome. Microb. Biotechnol. 7, 467–479. doi: 10.1111/1751-7915.12141
Danielsson, R., Dicksved, J., Sun, L., Gonda, H., Müller, B., Schnürer, A., et al. (2017). Methane production in dairy cows correlates with rumen methanogenic and bacterial community structure. Front. Microbiol. 8:226. doi: 10.3389/fmicb.2017.00226
Denman, S. E., Martinez-Fernandez, G., Shinkai, T., Mitsumori, M., and McSweeney, C. S. (2015). Metagenomic analysis of the rumen microbial community following inhibition of methane formation by a halogenated methane analog. Front. Microbiol. 6:1087. doi: 10.3389/fmicb.2015.01087
Difford, G. F., Plichta, D. R., Løvendahl, P., Lassen, J., Noel, S. J., Højberg, O., et al. (2018). Host genetics and the rumen microbiome jointly associate with methane emissions in dairy cows. PLoS Genet. 14:e1007580. doi: 10.1371/journal.pgen.1007580
Emerson, E. L., and Weimer, P. J. (2017). Fermentation of model hemicelluloses by Prevotella strains and Butyrivibrio fibrisolvens in pure culture and in ruminal enrichment cultures. Appl. Microbiol. Biotechnol. 101, 4269–4278. doi: 10.1007/s00253-017-8150-7
Granja-Salcedo, Y. T., Fernandes, R. M., de Araujo, R. C., Kishi, L. T., Berchielli, T. T., de Resende, F. D., et al. (2019). Long-term encapsulated nitrate supplementation modulates rumen microbial diversity and rumen fermentation to reduce methane emission in grazing steers. Front. Microbiol. 10:614. doi: 10.3389/fmicb.2019.00614
Greening, C., Geier, R., Wang, C., Woods, L. C., Morales, S. E., McDonald, M. J., et al. (2019). Diverse hydrogen production and consumption pathways influence methane production in ruminants. ISME J. 13, 2617–2632. doi: 10.1038/s41396-019-0464-2
Guyader, J., Eugène, M., Nozière, P., Morgavi, D. P., Doreau, M., and Martin, C. (2014). Influence of rumen protozoa on methane emission in ruminants: a meta-analysis approach. Animal 8, 1816–1825. doi: 10.1017/S1751731114001852
Guyader, J., Ungerfeld, E. M., and Beauchemin, K. A. (2017). Redirection of metabolic hydrogen by inhibiting methanogenesis in the rumen simulation technique (RUSITEC). Front. Microbiol. 8:393. doi: 10.3389/fmicb.2017.00393
Herlemann, D. P., Labrenz, M., Jürgens, K., Bertilsson, S., Waniek, J. J., and Andersson, A. F. (2011). Transitions in bacterial communities along the 2000 km salinity gradient of the Baltic Sea. ISME J. 5, 1571–1579. doi: 10.1038/ismej.2011.41
Hill, J., McSweeney, C., Wright, A. G., Bishop-Hurley, G., and Kalantar-zadeh, K. (2016). Measuring methane production from ruminants. Trends Biotecnol. 34, 26–35. doi: 10.1016/j.tibtech.2015.10.004
Hristov, A. N., Kebreab, E., Niu, M., Oh, J., Bannink, A., Bayat, A. R., et al. (2018). Uncertainties in enteric methane inventories, measurement techniques, and prediction models. J. Dairy Sci. 101, 6655–6674. doi: 10.3168/jds.2017-13536
Hristov, A. N., Oh, J., Giallongo, F., Frederick, T. W., Harper, M. T., Weeks, H. L., et al. (2015). An inhibitor persistently decreased enteric methane emission from dairy cows with no negative effect on milk production. Proc. Natl. Acad. Sci. USA. 112, 10663–10668. doi: 10.1073/pnas.1504124112
Iwasaki, K., Haryu, T., Tano, R., Terada, F., Itho, M., and Kameoka, K. (1982). New animal metabolism facility especially the description of respiration apparatus. Bull. Nat. Inst. Anim. Ind. 39, 41–78.
Janssen, P. H. (2010). Influence of hydrogen on rumen methane formation and fermentation balances through microbial growth kinetics and fermentation thermodynamics. Anim. Feed Sci. Technol. 160, 1–22. doi: 10.1016/j.anifeedsci.2010.07.002
Kamke, J., Kittelmann, S., Soni, P., Li, Y., Tavendale, M., Ganesh, S., et al. (2016). Rumen metagenome and metatranscriptome analyses of low methane yield sheep reveals a Sharpea-enriched microbiome characterised by lactic acid formation and utilization. Microbiome 4:56. doi: 10.1186/s40168-016-0201-2
Kamke, J., Soni, P., Li, Y., Ganesh, S., Kelly, W. J., Leahy, S. C., et al. (2017). Gene and transcript abundances of bacterial type III secretion systems from the rumen microbiome are correlated with methane yield in sheep. BMC Res. Notes. 10:367. doi: 10.1186/s13104-017-2671-0
Kittelmann, S., Pinares-Patiño, C. S., Seedorf, H., Kirk, M. R., Ganesh, S., McEwan, J. C., et al. (2014). Two different bacterial community types are linked with the low-methane emission trait in sheep. PLoS ONE 9:e103171. doi: 10.1371/journal.pone.0103171
Kittelmann, S., Seedorf, H., Walters, W. A., Clemente, J. C., Knight, R., Gordon, J. I., et al. (2013). Simultaneous amplicon sequencing to explore co-occurrence patterns of bacterial, archaeal and eukaryotic microorganisms in rumen microbial communities. PLoS ONE 8:e47879. doi: 10.1371/journal.pone.0047879
Kumar, S., Stecher, G., Li, M., Knyaz, C., and Tamura, K. (2018). MEGA X: Molecular evolutionary genetics analysis across computing platforms. Mol. Biol. Evol. 35, 1547–1549. doi: 10.1093/molbev/msy096
Lana, R. P., Russell, J. B., and Van Amburgh, M. E. (1998). The role of pH in regulating ruminal methane and ammonia production. J. Anim. Sci. 76, 2190–2196. doi: 10.2527/1998.7682190x
Liu, C., Meng, Q., Chen, Y., Xu, M., Shen, M., Gao, R., et al. (2017). Role of age-related shifts in rumen bacteria and methanogens in methane production in cattle. Front. Microbiol. 8:1563. doi: 10.3389/fmicb.2017.01563
Lyons, T., Bielak, A., Doyle, E., and Kuhla, B. (2018). Variations in methane yield and microbial community profiles in the rumen of dairy cows as they pass through stages of first lactation. J. Dairy Sci. 101, 5102–5114. doi: 10.3168/jds.2017-14200
Martin, M. (2011). Cutadapt removes adapter sequences from high-throughput sequencing reads. EMBnet. J. 17, 10–12. doi: 10.14806/ej.17.1.200
Martinez-Fernandez, G., Duval, S., Kindermann, M., Schirra, H. J., Denman, S. E., and McSweeney, C. S. (2018). 3-NOP vs. halogenated compound: Methane production, ruminal fermentation and microbial community response in forage fed cattle. Front. Microbiol. 9:1582. doi: 10.3389/fmicb.2018.01582
McCabe, M. S., Cormican, P., Keogh, K., O'Connor, A., O'Hara, E., Palladino, R. A., et al. (2015). Illumina MiSeq phylogenetic amplicon sequencing shows a large reduction of an uncharacterised Succinivibrionaceae and an increase of the Methanobrevibacter gottschalkii clade in feed restricted cattle. PLoS ONE 10:e0133234. doi: 10.1371/journal.pone.0133234
Mitsumori, M., Shinkai, T., Takenaka, A., Enishi, O., Higuchi, K., Kobayashi, Y., et al. (2012). Responses in digestion, rumen fermentation and microbial populations to inhibition of methane formation by a halogenated methane analogue. Br. J. Nutr. 108, 482–491. doi: 10.1017/S0007114511005794
Mizrahi, I., and Jami, E. (2018). The compositional variation of the rumen microbiome and its effect on host performance and methane emission. Animal 12, 220–232. doi: 10.1017/S1751731118001957
Newbold, C. J., López, S., Nelson, N., Ouda, J. O., Wallace, R. J., and Moss, A. R. (2005). Propionate precursors and other metabolic intermediates as possible alternative electron acceptors to methanogenesis in ruminal fermentation in vitro. Br. J. Nutr. 94, 27–35. doi: 10.1079/BJN20051445
Pope, P. B., Smith, W., Denman, S. E., Tringe, S. G., Barry, K., Hugenholtz, P., et al. (2011). Isolation of Succinivibrionaceae implicated in low methane emissions from Tammar wallabies. Science 333, 646–648. doi: 10.1126/science.1205760
Quast, C., Pruesse, E., Yilmaz, P., Gerken, J., Schweer, T., Yarza, P., et al. (2013). The SILVA ribosomal RNA gene database project: improved data processing and web-based tools. Nucleic Acids Res. 41, D590–D596. doi: 10.1093/nar/gks1219
Ramayo-Caldas, Y., Zingaretti, L., Popova, M., Estell,é, J., Bernard, A., Pons, N., et al. (2020). Identification of rumen microbial biomarkers linked to methane emission in Holstein dairy cows. J. Anim. Breed Genet. 137, 49–59. doi: 10.1111/jbg.12427
Roehe, R., Dewhurst, R. J., Duthie, C. A., Rooke, J. A., McKain, N., Ross, D. W., et al. (2016). Bovine host genetic variation influences rumen microbial methane production with best selection criterion for low methane emitting and efficiently feed converting hosts based on metagenomic gene abundance. PLoS Genet. 12:e1005846. doi: 10.1371/journal.pgen.1005846
Rognes, T., Flouri, T., Nichols, B., Quince, C., and Mahe, F. (2016). VSEARCH: a versatile open source tool for metagenomics. PeerJ. 4:e2584. doi: 10.7717/peerj.2584
Schären, M., Frahm, J., Kersten, S., Meyer, U., Hummel, J., Breves, G., et al. (2018). Interrelations between the rumen microbiota and production, behavioral, rumen fermentation, metabolic, and immunological attributes of dairy cows. J. Dairy Sci. 101, 4615–4637. doi: 10.3168/jds.2017-13736
Seshadri, R., Leahy, S. C., Attwood, G. T., Teh, K. H., Lambie, S. C., Cookson, A. L., et al. (2018). Cultivation and sequencing of rumen microbiome members from the Hungate1000 collection. Nat. Biotechnol. 36, 359–367. doi: 10.1038/nbt.4110
Shabat, S. K. B., Sasson, G., Doron-Faigenboim, A., Durman, T., Yaacoby, S., Miller, M. E. B., et al. (2016). Specific microbiome-dependent mechanisms underlie the energy harvest efficiency of ruminants. ISME J. 10, 2958–2972. doi: 10.1038/ismej.2016.62
Shibata, M., and Terada, F. (2010). Factors affecting methane production and mitigation in ruminants. Anim. Sci. J. 81, 2–10. doi: 10.1111/j.1740-0929.2009.00687.x
Shinkai, T., Enishi, O., Mitsumori, M., Higuchi, K., Kobayashi, Y., Takenaka, A., et al. (2012). Mitigation of methane production from cattle by feeding cashew nut shell liquid. J. Dairy Sci. 95, 5308–5316. doi: 10.3168/jds.2012-5554
Shinkai, T., Ikeyama, N., Kumagai, M., Ohmori, H., Sakamoto, M., Ohkuma, M., et al. (2022). Prevotella lacticifex sp. nov., isolated from the rumen of cows. Int. J. Syst. Evol. Microbiol. 72:005278. doi: 10.1099/ijsem.0.005278
Suzuki, T., Kamiya, Y., Oikawa, K., Nonaka, I., Shinkai, T., Terada, F., et al. (2021). Prediction of enteric methane emissions from lactating cows using methane to carbon dioxide ratio in the breath. Anim. Sci. J. 92:e13637. doi: 10.1111/asj.13637
Takizawa, S., Shinkai, T., Kobayashi, Y., Masuda, M., Hashiba, K., Uchisawa, K., et al. (2023). Rumen microbial composition associated with the non-glucogenic to glucogenic short-chain fatty acids ratio in Holstein cows. Anim. Sci. J. 94:e13829. doi: 10.1111/asj.13829
Tapio, I., Snelling, T. J., Strozzi, F., and Wallace, R. J. (2017). The ruminal microbiome associated with methane emissions from ruminant livestock. J. Anim. Sci. Biotechnol. 8:7. doi: 10.1186/s40104-017-0141-0
Ungerfeld, E. M. (2013). A theoretical comparison between two ruminal electron sinks. Front. Microbiol. 4:319. doi: 10.3389/fmicb.2013.00319
Ungerfeld, E. M. (2020). Metabolic hydrogen flows in rumen fermentation: principles and possibilities of interventions. Front. Microbiol. 11:589. doi: 10.3389/fmicb.2020.00589
Wallace, R. J., Rooke, J. A., McKain, N., Duthie, C. A., Hyslop, J. J., Ross, D. W., et al. (2015). The rumen microbial metagenome associated with high methane production in cattle. BMC Genom. 16:839. doi: 10.1186/s12864-015-2032-0
Wallace, R. J., Sasson, G., Garnsworthy, P. C., Tapio, I., Gregson, E., Bani, P., et al. (2019). A heritable subset of the core rumen microbiome dictates dairy cow productivity and emissions. Sci. Adv. 5:eaav8391. doi: 10.1126/sciadv.aav8391
Wattiaux, M. A., Uddin, M. E., Letelier, P., Jackson, R. D., and Larson, R. A. (2019). Emission and mitigation of greenhouse gases from dairy farms: the cow, the manure, and the field. Appl. Anim. Sci. 35, 238–254. doi: 10.15232/aas.2018-01803
Keywords: methane, propionate, dairy cow, rumen microbiota, Prevotella
Citation: Shinkai T, Takizawa S, Enishi O, Higuchi K, Ohmori H and Mitsumori M (2024) Characteristics of rumen microbiota and Prevotella isolates found in high propionate and low methane-producing dairy cows. Front. Microbiol. 15:1404991. doi: 10.3389/fmicb.2024.1404991
Received: 22 March 2024; Accepted: 21 May 2024;
Published: 03 June 2024.
Edited by:
Stefania Arioli, University of Milan, ItalyReviewed by:
Tansol Park, Chung-Ang University, Republic of KoreaCopyright © 2024 Shinkai, Takizawa, Enishi, Higuchi, Ohmori and Mitsumori. This is an open-access article distributed under the terms of the Creative Commons Attribution License (CC BY). The use, distribution or reproduction in other forums is permitted, provided the original author(s) and the copyright owner(s) are credited and that the original publication in this journal is cited, in accordance with accepted academic practice. No use, distribution or reproduction is permitted which does not comply with these terms.
*Correspondence: Takumi Shinkai, dHNoaW5rYWlAYWZmcmMuZ28uanA=
Disclaimer: All claims expressed in this article are solely those of the authors and do not necessarily represent those of their affiliated organizations, or those of the publisher, the editors and the reviewers. Any product that may be evaluated in this article or claim that may be made by its manufacturer is not guaranteed or endorsed by the publisher.
Research integrity at Frontiers
Learn more about the work of our research integrity team to safeguard the quality of each article we publish.