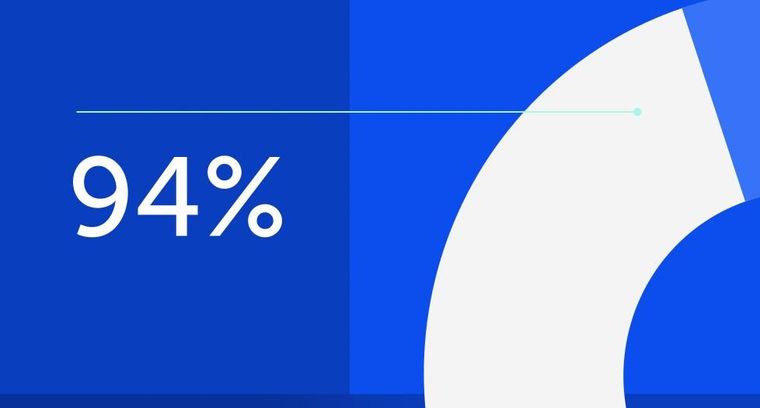
94% of researchers rate our articles as excellent or good
Learn more about the work of our research integrity team to safeguard the quality of each article we publish.
Find out more
ORIGINAL RESEARCH article
Front. Microbiol., 31 July 2024
Sec. Infectious Agents and Disease
Volume 15 - 2024 | https://doi.org/10.3389/fmicb.2024.1398886
This article is part of the Research TopicTuberculosis diagnosis, drug resistance, and drug target discoveryView all 18 articles
Background: The toxin-antitoxin (TA) system plays a vital role in the virulence and pathogenicity of Mycobacterium tuberculosis (M. tuberculosis). However, the regulatory mechanisms and the impact of gene mutations on M. tuberculosis transmission remain poorly understood.
Objective: To investigate the influence of gene mutations in the toxin-antitoxin system on M. tuberculosis transmission dynamics.
Method: We performed whole-genome sequencing on the analyzed strains of M. tuberculosis. The genes associated with the toxin-antitoxin system were obtained from the National Center for Biotechnology Information (NCBI) Gene database. Mutations correlating with enhanced transmission within the genes were identified by using random forest, gradient boosting decision tree, and generalized linear mixed models.
Results: A total of 13,518 M. tuberculosis isolates were analyzed, with 42.29% (n = 5,717) found to be part of genomic clusters. Lineage 4 accounted for the majority of isolates (n = 6488, 48%), followed by lineage 2 (n = 5133, 37.97%). 23 single nucleotide polymorphisms (SNPs) showed a positive correlation with clustering, including vapB1 G34A, vapB24 A76C, vapB2 T171C, mazF2 C85T, mazE2 G104A, vapB31 T112C, relB T226A, vapB11 C54T, mazE5 T344C, vapB14 A29G, parE1 (C103T, C88T), and parD1 C134T. Six SNPs, including vapB6 A29C, vapB31 T112C, parD1 C134T, vapB37 G205C, Rv2653c A80C, and vapB22 C167T, were associated with transmission clades across different countries. Notably, our findings highlighted the positive association of vapB6 A29C, vapB31 T112C, parD1 C134T, vapB37 G205C, vapB19 C188T, and Rv2653c A80C with transmission clades across diverse regions. Furthermore, our analysis identified 32 SNPs that exhibited significant associations with clade size.
Conclusion: Our study presents potential associations between mutations in genes related to the toxin-antitoxin system and the transmission dynamics of M. tuberculosis. However, it is important to acknowledge the presence of confounding factors and limitations in our study. Further research is required to establish causation and assess the functional significance of these mutations. These findings provide a foundation for future investigations and the formulation of strategies aimed at controlling TB transmission.
Tuberculosis (TB) is a global health threat caused by the highly successful human pathogen Mycobacterium tuberculosis (M. tuberculosis). According to a report by the World Health Organization (WHO), an estimated 10.6 million new TB cases occurred worldwide in 2022, resulting in over 1.3 million deaths (World Health Organization, 2023). Despite the substantial global burden of TB, our knowledge regarding the factors influencing its transmission remains limited. Therefore, it is imperative to delve deeper into the mechanisms underlying the spread of M. tuberculosis.
The toxin-antitoxin (TA) system plays a critical biological role in M. tuberculosis. Composed of toxins and antitoxins, this system forms a small genetic unit that is widely present in prokaryotes (Schuster and Bertram, 2013; Dai et al., 2022). TA systems have been shown to assist cells in stress adaptation, antibiotic resistance, biofilm formation, persisters, and disease development. Toxins are typically translated into proteins, while antitoxins can be either proteins or RNA (Ogura and Hiraga, 1983; Aizenman et al., 1996; Magnuson, 2007; Fineran et al., 2009; Wang and Wood, 2011; Lobato-Márquez et al., 2016). Based on the nature of antitoxins and the mechanisms which inhibit toxin activity, TA modules can be classified into six distinct types (Page and Peti, 2016). Among these types, type II TA systems are well-characterized, where antitoxins directly interact with toxins to neutralize their effects. Bioinformatics and phylogenetic analyses have revealed the presence of numerous TA systems encoded in the M. tuberculosis genome. The retention of these TA systems in members of the M. tuberculosis complex suggests their crucial role in regulating metabolic pathways essential for bacterial pathogenesis. Type II TA systems predominate in M. tuberculosis. The abundance of TA loci in the M. tuberculosis genome raises important questions about their functional diversity (Ramage et al., 2009; Tandon et al., 2019). Previous studies have extensively investigated the various functions of TA systems in M. tuberculosis and their potential impact on pathogenic mechanisms (Schippers et al., 2005; Guo et al., 2016). These systems are believed to play a key role in M. tuberculosis ‘s response to stressors such as nutrient starvation and antibiotic treatment, promoting its survival and drug resistance (Kim et al., 2018). Additionally, TA systems are associated with the formation of persistent cells, a subpopulation exhibiting drug tolerance that plays a crucial role in establishing chronic infections in M. tuberculosis (Merfa et al., 2016). While the importance of toxin-antitoxin systems in M. tuberculosis has been acknowledged, our understanding of their specific mechanisms and functions within this bacterium remains limited. Therefore, comprehensive research is required to explore the roles of TA systems and gain deeper insights into the complex biology of M. tuberculosis.
Driven by the need to better understand the mechanisms underlying M. tuberculosis transmission, we conducted an extensive study investigating the impact of mutations in TA system genes on its spread. Our research aims to elucidate how genetic variations within this system can influence M. tuberculosis strain transmission dynamics. Utilizing whole-genome sequencing (WGS), we analyzed the genetic variations present in M. tuberculosis isolates at a high-resolution level. This enabled us to identify specific mutations within the TA system genes that may be associated with M. tuberculosis transmission. Advanced statistical and bioinformatics techniques, including random forest, gradient boosting decision tree, and generalized linear mixed models, were employed for comprehensive analyses to identify key genetic variants linked to transmission dynamics. We acknowledge challenges posed by confounding factors and population dynamics in our analysis. Future research should incorporate social networks and regional interactions for a more comprehensive understanding. Limitations of our study include a focus on gene analysis, potentially overlooking other important genetic influences such as drug resistance mutations or virulence determinants. Therefore, more comprehensive studies are needed to address these limitations adequately. Our study has yielded significant results, identifying multiple single nucleotide polymorphisms (SNPs) within the toxin-antitoxin system genes that positively correlate with clustering, suggesting their potential role in M. tuberculosis transmission. Furthermore, some of these SNPs were found to be associated with transmission clades across different geographical regions, indicating their potential global impact on the spread of M. tuberculosis. These findings provide valuable insights into the transmission dynamics of this pathogen and contribute to a more thorough understanding of M. tuberculosis transmission.
We collected a total of 1,550 samples from patients with culture-positive pulmonary tuberculosis at two medical institutions in China: the Shandong Public Health Clinical Research Center (SPHCC) and Weifang Respiratory Disease Hospital (WRDH). These samples were obtained through analysis of sputum specimens. The sample collection spanned the period from 2011 to 2018. It is important to note that all samples were collected anonymously, and therefore, informed consent was not required as per the approved research protocol. Our study received ethical approval from the Ethics Committee of Shandong Provincial Hospital, which is affiliated with Shandong First Medical University (No.2017-337). This approval ensures that our research adheres to ethical guidelines and safeguards the rights and privacy of the participants involved in the study.
Genomic deoxyribonucleic acid (DNA) was successfully extracted from 1,468 of the 1,550 Shandong M. tuberculosis isolates. Gene sequencing was performed at the Beijing Genomic Institute. The genomic DNA was sequenced using an Illumina HiSeq 4,000 system. The resulting sequence data were deposited in the National Center for Biotechnology Information (NCBI) BioProject PRJNA1002108. Quality control of the sequence reads was conducted using Fast QC software, and a total of 1,447 samples passed the quality control criteria. Low-quality raw reads with a sequencing base ≤20 or sequencing fragments length ≤ 20 were excluded from the paired-end sequencing process. During the analysis, two isolates were accidentally lost, resulting in 1445 isolates being included for further analysis. The reads of these 1,445 strains, along with 12,132 M. tuberculosis isolates downloaded from previous studies and collected from 52 countries and 18 regions worldwide, were aligned to the H37Rv reference genome (NC_000962.3) using BWA-MEM (version 0.7.17-r1188) (Luo et al., 2015; Yang et al., 2017; Coll et al., 2018; Hicks et al., 2018; Koster et al., 2018; Liu et al., 2018; Chen et al., 2019; Huang et al., 2019; Jiang et al., 2020). To improve the alignment quality, clipped alignments and duplicated reads were removed using samclip (v0.4.0) and samtools markdup (v1.15), respectively. Samples with a coverage rate below 98% or a depth less than 20× were excluded from the analysis (Jajou et al., 2019; Yang et al., 2021). Additionally, 55 Mycobacterium bovis isolates, one Mycobacterium caprae isolate, and three Mycobacterium orygis isolates were also excluded. In summary, a total of 13,518 genomes were analyzed in this study. Specific sample numbers can be found in Supplementary Tables 1, 2.
After performing variant calling, we proceeded with additional filtering steps to enhance the quality of the detected variants. This involved employing Free Bayes (version 1.3.2) with an included filter parameter “FMT/GT = “1/1″ && QUAL> = 100 && FMT/DP > = 10 && (FMT/AO)/(FMT/DP) > = 0.” and Bcftools (version 1.15.1) for further refinement of the identified variants. To ensure the accuracy of our analysis, we excluded SNPs located within repetitive regions. This includes polymorphic sequences rich in GC found in PE/PPE genes, directly repeated SNPs, and repetitive bases identified using Tandem Repeat Finder (version 4.09) and RepeatMask (version 4.1.2-P1) (Li et al., 2009; Liu et al., 2019). The annotation for each candidate SNP was determined using SnpEff, version 4.11. The resulting output was obtained by utilizing the Python programming language (Cingolani et al., 2012).
Phylogenetic lineages were inferred based on specific SNPs following the methodology described by Coll et al. (2014) (Supplementary Tables 1, 2). Maximum-likelihood phylogenetic and phylogenomic analyses were conducted using IQ-TREE version 1.6.12. The phylogeny was constructed using the general time reversible (GTR) model of nucleotide substitution with the GAMMA model of rate heterogeneity, and bootstrap replicates were performed with 100 iterations. To establish the phylogenetic relationships, the genome of the Mycobacterium canettii strain CIPT 140010059 (NC_15848.1) was used as an outgroup (Nguyen et al., 2015). The resulting phylogenetic tree was visualized and annotated using the online phylogenetic tree visualization tool iTOL.1
We utilized the web-based tool TBProfiler (version 4.3.0) to analyze M. tuberculosis WGS data for drug resistance prediction (Phelan et al., 2019). Drug resistance was predicted using the curated drug-resistance Tuberculosis Database within TBProfiler. This database has undergone extensive testing on over 17,000 samples with genotypic and phenotypic data. The resistance-associated polymorphisms (SNPs and indels) identified by TBProfiler were further evaluated based on the WHO-endorsed catalog of molecular targets for M. tuberculosis complex drug-susceptibility testing and resistance interpretation (Walker et al., 2022). This additional assessment ensures reliable and accurate interpretation of drug resistance profiles. For more detailed information on the predicted drug resistance results, please refer to Supplementary Table 3.
To explore the influence of mutations in toxin-antitoxin system genes on the transmission of M. tuberculosis, we conducted analyses on transmission clusters and transmission clades (Seto et al., 2017). Building upon prior research (Walker et al., 2013), we defined genome-based transmission clusters as pairs of isolates separated by ≤12 SNPs. Genome-based transmission clades were defined as pairs of isolates separated by ≤25 SNPs. To classify the transmission clades into different categories, we adopted a classification system established by previous scholars. The transmission clades were categorized into three groups based on their size: large (above the 75th percentile), medium (between the 25th and 75th percentiles), and small (below the 25th percentile) (Chiner-Oms et al., 2019). For a comprehensive analysis of global distribution patterns and transmission dynamics among M. tuberculosis isolates, we classified them into two main groups: cross-country clades and within-country clades. Cross-country clades consisted of isolates originating from two or more different countries. Additionally, we further classified the M. tuberculosis isolates into cross-regional and within-regional clades based on their geographic location, using the United Nations standard regions (UN M.49). Cross-regional clades comprised isolates from two or more different regions.
Initially, our analysis started with the retrieval of all genes correlated with Mycobacterium tuberculosis from the NCBI database, which yielded a comprehensive set of 4,015 genes. We concentrated our study on the specific strain, Mycobacterium tuberculosis H37Rv, and meticulously filtered that list down to 4,009 genes, guided by their respective organism names. Subsequently, our attention was directed toward refining the gene selection, with a focus on identifying those associated with the toxin-antitoxin system. This involved evaluating their functional descriptions and characteristic annotations, resulting in the successful identification of 78 genes directly implicated in the toxin-antitoxin system. To further our investigation on these genes, we employed Python, a versatile programming language with robust data analysis capabilities, to identify mutations within the set of toxin-antitoxin system genes (Supplementary Table 4).
Categorical data were presented as frequencies and percentages. In order to improve statistical reliability, Mutations observed fewer than 10 times were discarded prior to continuing analysis. Statistical analyses were performed by generalized linear mixed models (GLMM) in R (version 4.2.3). In addition, Python 3.7.4 with the Scikit-learn library was used to implement random forest and gradient boosting decision tree algorithms for further data analysis. To evaluate the performance of the models, all samples were randomly divided into training and test sets at a ratio of 7:3. Various metrics such as Kappa, sensitivity, specificity, accuracy, positive predictive value (PPV), negative predictive value (NPV), positive likelihood ratio (PLR), negative likelihood ratio (NLR), and area under curve (AUC) were calculated to assess the models’ effectiveness (Luo et al., 2022). Importantly, after fitting the models, we assessed the importance of input variables on the model’s predictions. By assigning scores to each input feature, we identified the top-performing variables by taking the intersection of both conditions. This approach allowed us to identify the most influential features contributing to the precision of predicting risk factors (Bi et al., 2018; Agarwal et al., 2019). All models included lineage and geographical location as covariates to correct for potential confounding factors. All statistical tests were two-tailed, with p-values less than 0.05 considered statistically significant.
A total of 13,518 isolates were included in this study. We identified a total of 70,346 SNPs related to the toxin-antitoxin system. Out of the included strains, 6,488 (48%) belonged to lineage 4, 5,133 (37.97%) belonged to lineage 2, and only 10 strains (0.07%) belonged to lineage 6, while 29 strains (0.21%) belonged to lineage 7. By dividing the isolates into clusters based on 12 SNPs, a total of 5,717 strains clustered together, resulting in a clustering rate of 0.42. The M. tuberculosis isolates were further categorized into 1,955 clusters, with the number of isolates per cluster ranging from 2 to 146. Among the lineage 4 group, 3,245 (50.02%) isolates formed clusters, while within the lineage 2 group, 2,043 (39.80%) isolates formed clusters. Additionally, the majority of the M. tuberculosis strains analyzed in this study originated from Eastern Asia (n = 3,170, 23.45%) and Northern America (n = 1,646, 12.18%). Other regions contributing substantial sample sizes include Eastern Africa (n = 1731, 12.81%), Western Europe (n = 1,578, 11.67%), Northern Europe (n = 1,262, 9.34%), and Eastern Europe (n = 1,118, 8.27%), see Figure 1. Applying a threshold of 25 SNPs for clades, a total of 7,808 isolates claded together, resulting in a clading rate of 0.58. The M. tuberculosis isolates were further grouped into 2,218 clades, with the number of isolates per clade ranging from 2 to 192. Among these clades, there were 187 cross-country clades, consisting of 2 to 3 countries per clade, and 164 cross-regional clades, consisting of 2 to 3 regions per clade, as shown in Table 1. The phylogenetic tree of M. tuberculosis isolates was constructed as described in Figure 2.
Figure 2. A phylogenetic tree is depicted for the Mycobacterium tuberculosis isolates, with the outer circle indicating mutation sites of the toxin-antitoxin system genes. (A) Phylogenetic tree for the Mycobacterium tuberculosis isolates of lineage 2. (B) Phylogenetic tree for the Mycobacterium tuberculosis isolates of lineage 4.
We conducted a filtering process to exclude sites with less than 10 mutations, resulting in a final selection of 182 SNPs for further analysis. Our investigation aimed to explore the correlation between these 182 SNPs and clustering by comparing isolates within clusters to those outside clusters. The generalized linear mixed model (GLMM) revealed that 27 SNPs were statistically significant for clustering (p < 0.05) (Supplementary Table 5). Among these significant SNPs, there were 18 nonsynonymous SNPs, one start lost site, one stop gained site, and seven synonymous SNPs. Notably, these genetic variations showed a positive correlation with transmission clusters in M. tuberculosis isolates, see Table 2 for details. Furthermore, we employed random forest and gradient boosting decision tree models to establish prediction models (Table 3; Figure 3; Supplementary Table 14). However, the SNPs Rv0298 G213A, Rv1103c G56A, and Rv2871 G28C did not contribute significantly to the gradient boosting decision tree model. In summary, our findings suggested that the presence of Rv0064A (vapB1, G34A), Rv0239 (vapB24, A76C),Rv0300 (vapB2, T171C), Rv0659c (mazF2, C85T), Rv0660c (mazE2, G104A), Rv0748 (vapB31, T112C), Rv1247c (relB, T226A), Rv1560 (vapB11, C54T), Rv1943c (mazE5, T344C), Rv1952 (vapB14, A29G), Rv1959c (parE1, C103T, C88T), Rv1960c (parD1, C134T), Rv1991A (mazE6, G156A), Rv2009 (vapB15, T6C, G237A), Rv2142c (parE2, C48G), Rv2142A (parD2, A196G), Rv2274c (mazF8, A97G), Rv2526 (vapB17, G213C), Rv2550c (vapB20, A54C), Rv2654c T152C, Rv2862A (vapB23, T2C), and Rv3385c (vapB46, G70A) were positively associated with transmission clusters in M. tuberculosis isolates.
Table 2. Positive correlation between toxin-antitoxin system gene mutations and transmission clusters.
Table 3. The performance of various models for discriminating clustered isolates from non-clustered isolates.
Figure 3. Conduct ROC curve analysis to evaluate the performance of models for the relationship between mutations in toxin-antitoxin system genes and transmission clusters. (A) ROC analysis showing the performance of the random forest model. (B) ROC analysis showing the performance of the gradient boosting decision tree.
After excluding sites with less than 10 mutations, a total of 46 SNPs were identified and included for further analysis. Specifically focusing on clustered isolates belonging to lineage 2, we investigated the relationship between these 46 SNPs and non-clustered isolates. The GLMM analysis revealed that five SNPs showed statistical significance for clustering (p < 0.05) (Supplementary Table 6). Among these significant SNPs, there were three nonsynonymous SNPs, one start lost site, and one synonymous SNP, all of which displayed a positive correlation with clustering. Notably, these significant SNPs included Rv0239 (vapB24, A76C), Rv0659c (mazF2, C85T), Rv1959c (parE1, C25G), Rv1991A (mazE6, G156A), and Rv2862A (vapB23, T2C). Furthermore, prediction models were established using random forest and gradient boosting decision tree algorithms (Supplementary Tables 10, 15; Supplementary Figure 1). The findings demonstrated that vapB24 A76C, mazF2 C85T, parE1 C25G, mazE6 G156A, and vapB23 T2C significantly contributed to both the random forest and gradient boosting decision tree models. Overall, our results indicated a positive correlation between the SNPs vapB24 A76C, mazF2 C85T, parE1 C25G, mazE6 G156A, vapB23 T2C and transmission clusters within M. tuberculosis isolates of lineage 2.
After filtering out sites with less than 10 mutations, we selected a total of 82 SNPs for further analysis. Our focus was specifically on clustered isolates belonging to lineage 4, and we aimed to investigate the relationship between these 82 SNPs and clustered isolates. Using the GLMM analysis, we identified 17 SNPs that showed statistical significance for clustering (p < 0.05) (Supplementary Table 7). Among these significant SNPs, 11 were nonsynonymous SNPs, one was a stop gained SNP, and five were synonymous SNPs, all exhibiting a positive correlation with clustering, see Table 4 for details. Furthermore, we established prediction models using random forest and gradient boosting decision tree algorithms (Supplementary Tables 11, 16; Supplementary Figure 2). However, the SNPs Rv0064A G34A, Rv2009 T6C, Rv2104c G249T, and Rv3385c G70A did not contribute significantly to the gradient boosting decision tree model. In summary, our findings indicated a positive correlation between the SNPs Rv0300 (vapB2, T171C), Rv0660c (mazE2, G104A), Rv1560 (vapB11, C54T), Rv1943c (mazE5, T344C), Rv1952 (vapB14, A29G), Rv1959c (parE1, C88T), Rv1960c (parD1, C134T), Rv2009 (vapB15, G237A), Rv2142c (parE2, C48G), Rv2274c (mazF8, A97G), Rv2526 (vapB17, G213C), Rv2550c (vapB20, A54C), Rv2871 (vapB43, G28C), and transmission clusters within lineage 4 of M. tuberculosis isolates.
Table 4. Positive correlation between toxin-antitoxin system gene mutations and transmission clusters of lineage4.
After excluding sites with fewer than 10 mutations, a total of 128 SNPs within genes associated with the toxin-antitoxin system were identified and included for analysis. The objective was to investigate the relationship between these SNPs and cross-country transmission clades. The GLMM analysis revealed that seven nonsynonymous SNPs exhibited statistical significance for cross-country transmission clades (p < 0.05) (Supplementary Table 8). These significant SNPs included Rv0657c (vapB6, A29C), Rv0748 (vapB31, T112C), Rv1960c (parD1, C134T), Rv2104c (vapB37, G205C), Rv2547 (vapB19, C188T), Rv2653c A80C, and Rv2830c (vapB22, C167T). Additionally, random forest and gradient boosting decision tree models were employed to establish prediction models for these SNPs (Supplementary Tables 11, 17; Supplementary Figure 3). The results indicated that vapB6 A29C, vapB31 T112C, parD1 C134T, vapB37 G205C, Rv2653c A80C, and vapB22 C167T made significant contributions to both the random forest and gradient boosting decision tree models. However, the SNP vapB19 C188T did not contribute significantly to the gradient boosting decision tree model. Overall, our results showed that vapB6 A29C, vapB31 T112C, parD1 C134T, vapB37 G205C, Rv2653c A80C, and vapB22 C167T were positively correlated with transmission clades across different countries.
After excluding sites with less than 10 mutations, we identified and included a total of 128 SNPs of toxin-antitoxin system genes. The GLMM showed that seven nonsynonymous SNPs were found to be statistically significant for transmission clades of cross-country (p < 0.05) (Supplementary Table 9), including Rv0657c (vapB6, A29C), Rv0748 (vapB31, T112C), Rv1960c (parD1, C134T), Rv2104c (vapB37, G205C), Rv2547 (vapB19, C188T), Rv2653c A80C, Rv2830c (vapB22, C167T). Two prediction models were established using random forest and gradient boosting decision tree (Supplementary Tables 13, 18; Supplementary Figure 4), we found that vapB6 A29C, vapB31 T112C, parD1 C134T, vapB37 G205C, vapB19 C188T and Rv2653c A80C also contributed most to the random forest and gradient boosting decision tree. However, the SNP of vapB22 C167T did not contribute significantly to the gradient boosting decision tree model. Overall, our results showed that vapB6 A29C, vapB31 T112C, parD1 C134T, vapB37 G205C, vapB19 C188T, and Rv2653c A80C were positively correlated with transmission clades across different regions.
After excluding sites with less than 10 mutations, a total of 128 SNPs within the toxin-antitoxin system were identified and included for analysis. The results revealed that 32 SNPs were significantly associated with clade size (p < 0.05). Among these significant SNPs, there were 21 nonsynonymous SNPs, two stop gained SNPs, and nine synonymous SNPs, all of which displayed a positive correlation with clade size. Notable examples include vapB1 G34A, mazE2 G104A, vapB11 C54T, mazE5 T344C, vapB14 A29G, parE1 C88T, parD1 C134T, vapB15 T6C, parE2 C48G, mazF8 A97G, and vapB46 G70A. For more detailed information, please refer to Supplementary Figure 5.
Consistent with prior research findings, our study further emphasizes the diverse functionality of TA systems in M. tuberculosis. These redundant TA systems serve as a backup mechanism enabling cellular adaptation and survival under adverse conditions (Min et al., 2012). They play a critical role in M. tuberculosis’s stress response, including nutrient deprivation, by regulating essential cellular processes like DNA replication, protein translation, and cell division. Moreover, TA systems contribute to the formation of drug resistance and persistence in M. tuberculosis. However, it is important to acknowledge that certain studies have reported conflicting results regarding the specific contributions of TA systems to persistence formation and stress conditions (Yu et al., 2020; Sharma et al., 2021). These discrepancies may arise from variations in experimental setups or genetic differences among M. tuberculosis strains used in different investigations. Therefore, additional research is needed to precisely determine the roles of TA systems in persistence formation, stress responses, and their impact on M. tuberculosis pathogenesis. In our study, we focused on examining the relationship between gene mutations in toxin-antitoxin systems and the transmission dynamics of M. tuberculosis. The M. tuberculosis genome contains numerous toxin-antitoxin systems, including VapBC, MazEF, ParDE, and RelBE (Ramage et al., 2009; Tandon et al., 2019). To gain deeper insights into the significance of these toxin-antitoxin systems in M. tuberculosis transmission, we analyzed the prevalence and genetic variation of specific toxin-antitoxin system genes across various clusters and evolutionary branches. Our analysis detected multiple mutations in these genes, suggesting they could be involved in M. tuberculosis transmission.
In our study, we have found a strong association between SNPs in the VapB antitoxin-related genes and the transmission of M. tuberculosis. Specifically, we identified several significant SNPs that were linked to transmission, including vapB1 G34A, vapB24 A76C, vapB31 T112C, vapB14 A29G, and vapB15 (T6C, G237A). We observed that vapB24 A76C and vapB23 T2C were particularly associated with transmission, especially in lineage 2. Additionally, vapB2 T171C, vapB11 C54T, vapB14 A29G, vapB15 G237A, vapB17 G213C, and vapB20 A54C were significantly related to transmission, especially in lineage 4. Furthermore, we found that vapB43 G28C was associated with transmission in lineage 4, while vapB6 A29C, vapB31 T112C, and vapB37 G205C were correlated with cross-country and cross-regional transmission. We also found that vapB1 G34A, vapB11 C54T, vapB14 A29G, vapB15 T6C, and vapB46 G70A were related to clade size. The VapBC system is crucial for regulating the behavior and adaptation of M. tuberculosis under diverse environmental stresses. It comprises stable VapC toxins and labile VapB antitoxins, whose interplay is essential for bacterial growth, survival, and response to stress conditions (Robson et al., 2009; Winther and Gerdes, 2011). During periods of stress, antitoxin molecules are degraded, leading to the release of toxins, such as VapC, through their RNase activity (Min et al., 2012). Consequently, these toxins inhibit or slow down cellular metabolism, providing a survival advantage to the bacterium during adverse conditions. The delicate balance between VapB antitoxins and VapC toxins is crucial for maintaining bacterial homeostasis and ensuring appropriate responses to external stimuli (McKenzie et al., 2012). Overall, our study provides compelling evidence for the significant association between SNPs in VapB antitoxin-related genes and M. tuberculosis transmission. These findings shed light on the intricate role of the VapBC toxin-antitoxin system in regulating bacterial behavior and underscore the importance of genetic variations within this system in shaping transmission dynamics.
Our study has revealed the association between SNPs in other TA system genes and the transmission of M. tuberculosis. Specifically, we focused on the MazEF family, which consists of nine TA systems encoded in an operon (Ahmed et al., 2022). We found a close connection between the mazE6 G156A and mazF2 C85T gene polymorphisms and the transmission clusters, particularly within lineage 2. These variants exhibited significant correlations with the formation and expansion of transmission clusters. However, mazE6 G156A is a synonymous mutation (Arg52Arg), which does not directly alter the protein’s function but may still affect the protein through other mechanisms. For example, in certain situations, synonymous mutations can lead to changes in transcription regulatory elements, thereby influencing gene expression levels. However, further research is needed to confirm these effects. Similarly, the mazF2 C85T variant may alter the stability of the MazF and modulate the delicate balance between toxin and antitoxin interactions (Leplae et al., 2011). Furthermore, our study identified a strong correlation between the mazE2 G104A, mazE5 T344C, and mazF8 A97G gene polymorphisms and the transmission clusters, especially within lineage 4 and clade size. While it’s plausible that these genetic variations influence the MazEF system activity, stability, and domain structure, our ability to fully elucidate these mechanisms is currently limited. Therefore, it’s crucial to interpret these functional implications cautiously and consider other potential contributory factors to M. tuberculosis transmission. Furthermore, no SNPs in the MazEF system were found to be associated with cross-country and cross-regional transmission of M. tuberculosis in our study. Future investigations should aim to provide a more comprehensive understanding of these effects, confirm these hypotheses, and uncover the precise impact of these mutations on the dynamics of M. tuberculosis transmission.
The ParDE toxin-antitoxin system in M. tuberculosis plays a crucial role in bacterial transmission dynamics. Our research has identified specific genetic variations in the parE and parD genes, such as parE1 C88T, parE2 C48G, parE1 C103T, parD2 A196G, parE1 C25G, and parD1 C134T, that are closely linked to transmission clusters, particularly within lineage 4 and lineage 2. These genetic variants impact cross-country and cross-regional transmissions, highlighting the significance of the ParDE system in the spread of M. tuberculosis. Variations in the parD gene, including those involving Rv2142A (parD2) and Rv1960c (parD1), can modify the activity and regulatory mechanisms of the ParD antitoxin. Similarly, variations in the parE gene, particularly those affecting Rv1959c (parE1), influence the function and stability of the ParE toxin, thus impacting its interaction with the ParE antitoxin (Xu et al., 2018). Understanding these genetic interactions is crucial for deciphering M. tuberculosis transmission dynamics and developing targeted interventions to effectively combat tuberculosis. Additionally, our research has identified a unique SNP, T226A, in the relB gene that is associated with transmission clusters in M. tuberculosis. This genetic variation further adds to the complexity of bacterial transmission dynamics, highlighting the intricate interplay between genetic factors and the spread of M. tuberculosis.
In terms of drug development and therapeutic interventions, our research findings could potentially have significant implications. The diverse functions of TA systems suggest potential targets for novel therapeutic strategies in M. tuberculosis. Understanding the relationship between genetic variations and functional consequences within these TA systems might help us discover new methods to disrupt or modulate their activity, thereby affecting the survival and transmission dynamics of the bacterium. Firstly, interventions targeting specific SNPs in TA systems such as VapBC, MazEF, ParDE, and RelBE could possibly directly alter the stability and activity of their toxins or antitoxins, thus impacting the growth, survival, and adaptability of M. tuberculosis (Robson et al., 2009; Leplae et al., 2011; Winther and Gerdes, 2011; McKenzie et al., 2012; Ahmed et al., 2022). The SNPs we discovered, including vapB24 A76C and vapB23 T2C, have the potential to serve as genetic markers for targeted drug design, allowing for more personalized treatment approaches. Additionally, mutations like parE1 C88T, parE2 C48G, and parE1 C103T show associations with cross-national and cross-regional transmissions of M. tuberculosis, which could aid in the development of more effective treatment plans to reduce global transmission. However, it’s important to note that while these genetic insights hold potential, they still require experimental validation to confirm their clinical significance and functional implications. Each mutation may lead to different functional impacts, and there might be other complexities involved, such as drug tolerance or adaptability of the bacterium under different environmental conditions. Therefore, further research is needed to delve deeper into the functional impacts of these genetic variations and precisely determine their roles in new drug development and treatment strategies. It is crucial to validate these findings through rigorous experimental studies and clinical trials before implementing them in clinical practice. Future research should aim to elucidate the specific mechanisms underlying these genetic variations and their contributions to drug response and transmission dynamics. By gaining a better understanding of the functional implications, we can more accurately tailor treatment strategies and contribute to the development of more targeted and effective interventions.
Our findings emphasize that both synonymous and non-synonymous mutations can influence the transmission of M. tuberculosis, suggesting that synonymous mutations in TA system genes are not universally neutral, in line with prior research by Shen et al. (2022). We believe that synonymous mutations may affect mRNA stability, splicing, or secondary structure formation. Changes in these regulatory elements can influence gene expression patterns and protein folding, thereby impacting bacterial adaptability and transmission capacity. Additionally, synonymous mutations may be part of a compensatory mechanism. While synonymous mutations themselves may not directly provide selective advantages, they may be associated with compensatory changes in other regions of the genome. These compensatory mutations could restore proper interactions between proteins, maintain enzyme activity, or optimize cellular functions affected by primary mutations, ultimately enhancing transmission capacity. Although the specific mechanisms and advantages of synonymous mutations in tuberculosis transmission are not yet fully understood, we cannot overlook their potential significance. Future research should consider the functional consequences of synonymous mutations and explore their interactions with other genetic factors, including non-synonymous mutations, drug resistance mutations, or virulence determinants. In our study, we combined local and global datasets to increase sample size for robust analysis of M. tuberculosis genetic variations. This approach helped identify shared and distinct variants across regions, enhancing our understanding of global pathogen diversity. Despite potential limitations such as variability from different protocols and sequencing technologies, stringent quality control measures, including SNP filtering within repetitive regions, were applied to minimize biases. Our novel findings contribute valuable insights into global M. tuberculosis genetic characteristics, advancing knowledge on tuberculosis pathogenesis and evolution. In future research, separate and comparative analyses of local and global data can be considered to highlight region-specific variations.
In our study, we investigated the impact of mutations in TA system genes on tuberculosis transmission. However, it is crucial to acknowledge that these correlations alone do not establish a causal relationship and should be interpreted with caution. Our modeling approach has limitations, notably in addressing potential confounding factors, such as population mobility, social networks, and inter-regional interactions. These elements may influence M. tuberculosis transmission but were not fully integrated into our models. We recognize that our primary focus on mutations within TA system genes may have led us to overlook other significant genetic influences, including SNPs related to drug resistance mutations or virulence determinants. While our findings contribute to the growing body of knowledge regarding the impact of toxin-antitoxin system gene mutations on tuberculosis transmission, further research is necessary to explore these intersections and understand their functional significance in detail. Limitations also arise from the sheer number of genes and computational resources required, which restricted our ability to analyze SNPs beyond the scope of our current investigation. Moreover, we lack a clear understanding of the cross-interactions and mutual regulation among the TA systems of M. tuberculosis, adding another layer of complexity to our study. Additionally, uncertainties inherent in the phylogenetic inference method used, such as homoplasy or recombination events, can present challenges when accurately determining evolutionary relationships. Therefore, future research should consider alternative methods to validate these findings and develop a more nuanced understanding of tuberculosis transmission. Further experimental validation is necessary to confirm the specific impact of TA system gene mutations. Future investigations should focus on refining our models to account for potential biases or shortcomings, and expanding research scope to explore the functional significance of these mutations and their direct influence on tuberculosis transmission.
We also discuss the limitations of using H37Rv as a single reference genome for analyzing M. tuberculosis WGS data, particularly regarding virulence and transmission. Recent studies suggest that relying solely on H37Rv may not fully capture the virulence characteristics of M. tuberculosis. H37Rv, commonly used as a reference genome in molecular epidemiology and drug resistance studies, does not represent the genetic diversity and variations present across all M. tuberculosis strains. Polymorphic loci involving genes associated with pathogenicity and host immune response, such as phospholipase C, membrane lipoproteins, adenylate cyclase gene family members, and PE/PPE gene family members, show significant differences between H37Rv and clinical isolates. Several gene families, including PE/PPE, exhibit higher substitution frequencies compared to the entire genome. Widespread genetic variability is observed at these polymorphic loci among M. tuberculosis clinical isolates (Fleischmann et al., 2002; O’Toole and Gautam, 2017). Phylogenetic and epidemiological analyses reveal independent occurrences of these polymorphisms, suggesting selective pressures driving these changes. Future research should incorporate genome sequences of additional reference strains, especially those directly obtained from clinical isolates, to comprehensively understand factors related to M. tuberculosis virulence and enable further investigations. For drug resistance inference, our analysis primarily utilized the TBProfiler platform. While incorporating additional tools/methods such as PhyResSE or bioinformatic SNP analysis could enhance robustness, resource constraints limited their implementation in this study. Thus, our results should be interpreted within the context of utilizing TBProfiler alongside the WHO-endorsed catalog. Future studies with expanded resources could consider alternative tools/methods for validation and complementation.
The results of this study suggest that mutations in toxin-antitoxin genes may increase the risk of M. tuberculosis transmission, underscoring the significance of conducting further research to explore the impact of these mutations on M. tuberculosis control and transmission. These findings offer new insights into the development of drug treatment strategies against tuberculosis.
The whole genome sequences have been submitted to the NCBI under the accession number PRJNA1002108.
YH: Conceptualization, Formal analysis, Software, Writing – original draft, Writing – review & editing. YiL: Conceptualization, Formal analysis, Methodology, Writing – review & editing. NT: Conceptualization, Formal analysis, Investigation, Project administration, Software, Validation, Writing – review & editing. XK: Conceptualization, Data curation, Formal analysis, Investigation, Methodology, Software, Validation, Writing – original draft. YamL: Conceptualization, Data curation, Formal analysis, Investigation, Methodology, Project administration, Software, Supervision, Validation, Writing – review & editing. YaoL: Data curation, Formal analysis, Methodology, Project administration, Supervision, Validation, Writing – review & editing. HL: Conceptualization, Data curation, Funding acquisition, Investigation, Resources, Visualization, Writing – original draft, Writing – review & editing. ZW: Conceptualization, Data curation, Formal analysis, Funding acquisition, Investigation, Project administration, Resources, Software, Supervision, Visualization, Writing – original draft.
The author(s) declare that financial support was received for the research, authorship, and/or publication of this article. This study was supported by grants from the Science & Technology Fundamental Resources Investigation Program (Grant no. 2022FY102001), the Shandong Provincial Natural Science Foundation (No. ZR2020KH013; No. ZR2021MH006), the Department of Science & Technology of Shandong Province (CN) (No. 2007GG30002033; No. 2017GSF218052), and the Jinan Science and Technology Bureau (CN) (No. 201704100).It is important to note that the funding bodies provided financial support for the study but were not involved in the study design, data collection, analysis, interpretation, or manuscript writing process.
We thank Shandong Public Health Clinical Research Center and Weifang Respiratory Clinical Hospital for providing us with the clinical sample data. Additionally, we extend our thanks to all the authors who have shared their sequence datasets on NCBI.
The authors declare that the research was conducted in the absence of any commercial or financial relationships that could be construed as a potential conflict of interest.
All claims expressed in this article are solely those of the authors and do not necessarily represent those of their affiliated organizations, or those of the publisher, the editors and the reviewers. Any product that may be evaluated in this article, or claim that may be made by its manufacturer, is not guaranteed or endorsed by the publisher.
The Supplementary material for this article can be found online at:https://www.frontiersin.org/articles/10.3389/fmicb.2024.1398886/full#supplementary-material
Agarwal, G., Saade, S., Shahid, M., Tester, M., and Sun, Y. (2019). Quantile function modeling with application to salinity tolerance analysis of plant data. BMC Plant Biol. 19:526. doi: 10.1186/s12870-019-2039-9
Ahmed, S., Chattopadhyay, G., Manjunath, K., Bhasin, M., Singh, N., Rasool, M., et al. (2022). Combining cysteine scanning with chemical labeling to map protein-protein interactions and infer bound structure in an intrinsically disordered region. Front. Mol. Biosci. 9:997653. doi: 10.3389/fmolb.2022.997653
Aizenman, E., Engelberg-Kulka, H., and Glaser, G. (1996). An Escherichia coli chromosomal “addiction module” regulated by guanosine [corrected] 3′,5′-bispyrophosphate: a model for programmed bacterial cell death. Proc. Natl. Acad. Sci. USA 93, 6059–6063. doi: 10.1073/pnas.93.12.6059
Bi, X., Xu, Q., Luo, X., Sun, Q., and Wang, Z. (2018). Weighted random support vector machine clusters analysis of resting-state fMRI in mild cognitive impairment. Front. Psych. 9:340. doi: 10.3389/fpsyt.2018.00340
Chen, X., He, G., Wang, S., Lin, S., Chen, J., and Zhang, W. (2019). Evaluation of whole-genome sequence method to diagnose resistance of 13 anti-tuberculosis drugs and characterize resistance genes in clinical multi-drug resistance Mycobacterium tuberculosis isolates from China. Front. Microbiol. 10:1741. doi: 10.3389/fmicb.2019.01741
Chiner-Oms, Á., Sánchez-Busó, L., Corander, J., Gagneux, S., Harris, S. R., Young, D., et al. (2019). Genomic determinants of speciation and spread of the Mycobacterium tuberculosis complex. Sci. Adv. 5:eaaw3307. doi: 10.1126/sciadv.aaw3307
Cingolani, P., Platts, A., Wang, L. L., Coon, M., Nguyen, T., Wang, L., et al. (2012). A program for annotating and predicting the effects of single nucleotide polymorphisms, SnpEff: SNPs in the genome of Drosophila melanogaster strain w1118; iso-2; iso-3. Fly 6, 80–92. doi: 10.4161/fly.19695
Coll, F., McNerney, R., Guerra-Assunção, J. A., Glynn, J. R., Perdigão, J., Viveiros, M., et al. (2014). A robust SNP barcode for typing Mycobacterium tuberculosis complex strains. Nat. Commun. 5:4812. doi: 10.1038/ncomms5812
Coll, F., Phelan, J., Hill-Cawthorne, G. A., Nair, M. B., Mallard, K., Ali, S., et al. (2018). Genome-wide analysis of multi- and extensively drug-resistant Mycobacterium tuberculosis. Nat. Genet. 50, 307–316. doi: 10.1038/s41588-017-0029-0
Dai, Z., Wu, T., Xu, S., Zhou, L., Tang, W., Hu, E., et al. (2022). Characterization of toxin-antitoxin systems from public sequencing data: a case study in Pseudomonas aeruginosa. Front. Microbiol. 13:951774. doi: 10.3389/fmicb.2022.951774
Fineran, P. C., Blower, T. R., Foulds, I. J., Humphreys, D. P., Lilley, K. S., and Salmond, G. P. C. (2009). The phage abortive infection system, ToxIN, functions as a protein–RNA toxin–antitoxin pair. Proc. Natl. Acad. Sci. USA 106, 894–899. doi: 10.1073/pnas.0808832106
Fleischmann, R. D., Alland, D., Eisen, J. A., Carpenter, L., White, O., Peterson, J., et al. (2002). Whole-genome comparison of Mycobacterium tuberculosis clinical and laboratory strains. J. Bacteriol. 184, 5479–5490. doi: 10.1128/JB.184.19.5479-5490.2002
Guo, Y., Yao, J., Sun, C., Wen, Z., and Wang, X. (2016). Characterization of the Deep-Sea Streptomyces sp. SCSIO 02999 derived VapC/VapB toxin-antitoxin system in Escherichia coli. Toxins 8:195. doi: 10.3390/toxins8070195
Hicks, N. D., Yang, J., Zhang, X., Zhao, B., Grad, Y. H., Liu, L., et al. (2018). Clinically prevalent mutations in Mycobacterium tuberculosis alter propionate metabolism and mediate multidrug tolerance. Nat. Microbiol. 3, 1032–1042. doi: 10.1038/s41564-018-0218-3
Huang, H., Ding, N., Yang, T., Li, C., Jia, X., Wang, G., et al. (2019). Cross-sectional whole-genome sequencing and epidemiological study of multidrug-resistant Mycobacterium tuberculosis in China. Clin. Infect. Dis. 69, 405–413. doi: 10.1093/cid/ciy883
Jajou, R., Kohl, T. A., Walker, T., Norman, A., Cirillo, D. M., Tagliani, E., et al. (2019). Towards standardisation: comparison of five whole genome sequencing (WGS) analysis pipelines for detection of epidemiologically linked tuberculosis cases. Euro Surveill. 24:1900130. doi: 10.2807/1560-7917.ES.2019.24.50.1900130
Jiang, Q., Liu, Q., Ji, L., Li, J., Zeng, Y., Meng, L., et al. (2020). Citywide transmission of multidrug-resistant tuberculosis under China’s rapid urbanization: a retrospective population-based genomic spatial epidemiological study. Clin. Infect. Dis. 71, 142–151. doi: 10.1093/cid/ciz790
Kim, D.-H., Kang, S.-M., Park, S. J., Jin, C., Yoon, H.-J., and Lee, B.-J. (2018). Functional insights into the Streptococcus pneumoniae HicBA toxin–antitoxin system based on a structural study. Nucleic Acids Res. 46, 6371–6386. doi: 10.1093/nar/gky469
Koster, K. J., Largen, A., Foster, J. T., Drees, K. P., Qian, L., Desmond, E., et al. (2018). Genomic sequencing is required for identification of tuberculosis transmission in Hawaii. BMC Infect. Dis. 18:608. doi: 10.1186/s12879-018-3502-1
Leplae, R., Geeraerts, D., Hallez, R., Guglielmini, J., Drèze, P., and Van Melderen, L. (2011). Diversity of bacterial type II toxin–antitoxin systems: a comprehensive search and functional analysis of novel families. Nucleic Acids Res. 39, 5513–5525. doi: 10.1093/nar/gkr131
Li, H., Handsaker, B., Wysoker, A., Fennell, T., Ruan, J., Homer, N., et al. (2009). 1000 genome project data processing subgroup. The sequence alignment/map format and SAMtools. Bioinformatics 25, 2078–2079. doi: 10.1093/bioinformatics/btp352
Liu, F., Zhang, Y., Zhang, L., Li, Z., Fang, Q., Gao, R., et al. (2019). Systematic comparative analysis of single-nucleotide variant detection methods from single-cell RNA sequencing data. Genome Biol. 20:242. doi: 10.1186/s13059-019-1863-4
Liu, Q., Ma, A., Wei, L., Pang, Y., Wu, B., Luo, T., et al. (2018). China’s tuberculosis epidemic stems from historical expansion of four strains of Mycobacterium tuberculosis. Nat. Ecol. Evol. 2, 1982–1992. doi: 10.1038/s41559-018-0680-6
Lobato-Márquez, D., Díaz-Orejas, R., and García-Del, P. F. (2016). Toxin-antitoxins and bacterial virulence. FEMS Microbiol. Rev. 40, 592–609. doi: 10.1093/femsre/fuw022
Luo, T., Comas, I., Luo, D., Lu, B., Wu, J., Wei, L., et al. (2015). Southern east Asian origin and coexpansion of Mycobacterium tuberculosis Beijing family with Han Chinese. Proc. Natl. Acad. Sci. USA 112, 8136–8141. doi: 10.1073/pnas.1424063112
Luo, Y., Xue, Y., Song, H., Tang, G., Liu, W., Bai, H., et al. (2022). Machine learning based on routine laboratory indicators promoting the discrimination between active tuberculosis and latent tuberculosis infection. J. Infect. 84, 648–657. doi: 10.1016/j.jinf.2021.12.046
Magnuson, R. D. (2007). Hypothetical functions of toxin-antitoxin systems. J. Bacteriol. 189, 6089–6092. doi: 10.1128/JB.00958-07
McKenzie, J. L., Robson, J., Berney, M., Smith, T. C., Ruthe, A., Gardner, P. P., et al. (2012). A VapBC toxin-antitoxin module is a posttranscriptional regulator of metabolic flux in mycobacteria. J. Bacteriol. 194, 2189–2204. doi: 10.1128/JB.06790-11
Merfa, M. V., Niza, B., Takita, M. A., and De Souza, A. A. (2016). The MqsRA toxin-antitoxin system from Xylella fastidiosa plays a key role in bacterial fitness, pathogenicity, and Persister cell formation. Front. Microbiol. 7:904. doi: 10.3389/fmicb.2016.00904
Min, A. B., Miallau, L., Sawaya, M. R., Habel, J., Cascio, D., and Eisenberg, D. (2012). The crystal structure of the Rv0301-Rv0300 VapBC-3 toxin—antitoxin complex from M. tuberculosis reveals a mg 2+ ion in the active site and a putative RNA-binding site. Protein Sci. 21, 1754–1767. doi: 10.1002/pro.2161
Nguyen, L.-T., Schmidt, H. A., von Haeseler, A., and Minh, B. Q. (2015). IQ-TREE: a fast and effective stochastic algorithm for estimating maximum-likelihood phylogenies. Mol. Biol. Evol. 32, 268–274. doi: 10.1093/molbev/msu300
O’Toole, R. F., and Gautam, S. S. (2017). Limitations of the Mycobacterium tuberculosis reference genome H37Rv in the detection of virulence-related loci. Genomics 109, 471–474. doi: 10.1016/j.ygeno.2017.07.004
Ogura, T., and Hiraga, S. (1983). Mini-F plasmid genes that couple host cell division to plasmid proliferation. Proc. Natl. Acad. Sci. USA 80, 4784–4788. doi: 10.1073/pnas.80.15.4784
Page, R., and Peti, W. (2016). Toxin-antitoxin systems in bacterial growth arrest and persistence. Nat. Chem. Biol. 12, 208–214. doi: 10.1038/nchembio.2044
Phelan, J. E., O’Sullivan, D. M., Machado, D., Ramos, J., Oppong, Y. E. A., Campino, S., et al. (2019). Integrating informatics tools and portable sequencing technology for rapid detection of resistance to anti-tuberculous drugs. Genome Med. 11:41. doi: 10.1186/s13073-019-0650-x
Ramage, H. R., Connolly, L. E., and Cox, J. S. (2009). Comprehensive functional analysis of Mycobacterium tuberculosis toxin-antitoxin systems: implications for pathogenesis, stress responses, and evolution. PLoS Genet. 5:e1000767. doi: 10.1371/journal.pgen.1000767
Robson, J., McKenzie, J. L., Cursons, R., Cook, G. M., and Arcus, V. L. (2009). The vapBC operon from Mycobacterium smegmatis is an autoregulated toxin–antitoxin module that controls growth via inhibition of translation. J. Mol. Biol. 390, 353–367. doi: 10.1016/j.jmb.2009.05.006
Schippers, A., Neretin, L. N., Kallmeyer, J., Ferdelman, T. G., Cragg, B. A., Parkes, R. J., et al. (2005). Prokaryotic cells of the deep sub-seafloor biosphere identified as living bacteria. Nature 433, 861–864. doi: 10.1038/nature03302
Schuster, C. F., and Bertram, R. (2013). Toxin-antitoxin systems are ubiquitous and versatile modulators of prokaryotic cell fate. FEMS Microbiol. Lett. 340, 73–85. doi: 10.1111/1574-6968.12074
Seto, J., Wada, T., Suzuki, Y., Ikeda, T., Mizuta, K., Yamamoto, T., et al. (2017). Mycobacterium tuberculosis transmission among elderly persons, Yamagata prefecture, Japan, 2009–2015. Emerg. Infect. Dis. 23, 448–455. doi: 10.3201/eid2303.161571
Sharma, A., Sagar, K., Chauhan, N. K., Venkataraman, B., Gupta, N., Gosain, T. P., et al. (2021). HigB1 toxin in Mycobacterium tuberculosis is upregulated during stress and required to establish infection in Guinea pigs. Front. Microbiol. 12:748890. doi: 10.3389/fmicb.2021.748890
Shen, X., Song, S., Li, C., and Zhang, J. (2022). Synonymous mutations in representative yeast genes are mostly strongly non-neutral. Nature 606, 725–731. doi: 10.1038/s41586-022-04823-w
Tandon, H., Sharma, A., Wadhwa, S., Varadarajan, R., Singh, R., Srinivasan, N., et al. (2019). Bioinformatic and mutational studies of related toxin–antitoxin pairs in Mycobacterium tuberculosis predict and identify key functional residues. J. Biol. Chem. 294, 9048–9063. doi: 10.1074/jbc.RA118.006814
Walker, T. M., Ip, C. L., Harrell, R. H., Evans, J. T., Kapatai, G., Dedicoat, M. J., et al. (2013). Whole-genome sequencing to delineate Mycobacterium tuberculosis outbreaks: a retrospective observational study. Lancet Infect. Dis. 13, 137–146. doi: 10.1016/S1473-3099(12)70277-3
Walker, T. M., Miotto, P., Köser, C. U., Fowler, P. W., Knaggs, J., Iqbal, Z., et al. (2022). The 2021 WHO catalogue of Mycobacterium tuberculosis complex mutations associated with drug resistance: a genotypic analysis. Lancet Microbe 3, e265–e273. doi: 10.1016/S2666-5247(21)00301-3
Wang, X., and Wood, T. K. (2011). Toxin-antitoxin systems influence biofilm and Persister cell formation and the general stress response ▿. Appl. Environ. Microbiol. 77, 5577–5583. doi: 10.1128/AEM.05068-11
Winther, K. S., and Gerdes, K. (2011). Enteric virulence associated protein VapC inhibits translation by cleavage of initiator tRNA. Proc. Natl. Acad. Sci. USA 108, 7403–7407. doi: 10.1073/pnas.1019587108
World Health Organization (2023). Global tuberculosis report 2023. Geneva: World Health Organization.
Xu, J., Zhang, N., Cao, M., Ren, S., Zeng, T., Qin, M., et al. (2018). Identification of three type II toxin-antitoxin systems in Streptococcus suis serotype 2. Toxins 10:467. doi: 10.3390/toxins10110467
Yang, C., Luo, T., Shen, X., Wu, J., Gan, M., Xu, P., et al. (2017). Transmission of multidrug-resistant Mycobacterium tuberculosis in Shanghai, China: a retrospective observational study using whole-genome sequencing and epidemiological investigation. Lancet Infect. Dis. 17, 275–284. doi: 10.1016/S1473-3099(16)30418-2
Yang, T., Wang, Y., Liu, Q., Jiang, Q., Hong, C., Wu, L., et al. (2021). A population-based genomic epidemiological study of the source of tuberculosis infections in an emerging city: Shenzhen, China. Lancet Reg. Health West Pac. 8:100106. doi: 10.1016/j.lanwpc.2021.100106
Keywords: toxin-antitoxin system, Mycobacterium tuberculosis , transmission, whole genome sequencing, single nucleotide polymorphisms
Citation: Hou Y, Li Y, Tao N, Kong X, Li Y, Liu Y, Li H and Wang Z (2024) Toxin-antitoxin system gene mutations driving Mycobacterium tuberculosis transmission revealed by whole genome sequencing. Front. Microbiol. 15:1398886. doi: 10.3389/fmicb.2024.1398886
Received: 11 March 2024; Accepted: 22 July 2024;
Published: 31 July 2024.
Edited by:
Axel Cloeckaert, Institut National de recherche pour l’agriculture, l’alimentation et l’environnement (INRAE), FranceReviewed by:
Gopinath Chattopadhyay, University of Zurich, SwitzerlandCopyright © 2024 Hou, Li, Tao, Kong, Li, Liu, Li and Wang. This is an open-access article distributed under the terms of the Creative Commons Attribution License (CC BY). The use, distribution or reproduction in other forums is permitted, provided the original author(s) and the copyright owner(s) are credited and that the original publication in this journal is cited, in accordance with accepted academic practice. No use, distribution or reproduction is permitted which does not comply with these terms.
*Correspondence: Yao Liu, ZG9jdG9ybGl1eWFvQDEyNi5jb20=; Huaichen Li, bGlodWFpY2hlbkAxNjMuY29t; Zhenguo Wang, emhlbmd1b3dAMTI2LmNvbQ==
Disclaimer: All claims expressed in this article are solely those of the authors and do not necessarily represent those of their affiliated organizations, or those of the publisher, the editors and the reviewers. Any product that may be evaluated in this article or claim that may be made by its manufacturer is not guaranteed or endorsed by the publisher.
Research integrity at Frontiers
Learn more about the work of our research integrity team to safeguard the quality of each article we publish.