- 1College of Food Science and Technology, Southwest Minzu University, Chengdu, China
- 2College of Animal and Veterinary Sciences, Southwest Minzu University, Chengdu, China
- 3Department of Agricultural and Food Sciences, University of Bologna, Cesena, Italy
Mastitis is commonly recognized as a localized inflammatory udder disease induced by the infiltration of exogenous pathogens. In the present study, our objective was to discern fecal and milk variations in both microbiota composition and metabolite profiles among three distinct groups of cows: healthy cows, cows with subclinical mastitis and cows with clinical mastitis. The fecal microbial community of cows with clinical mastitis was significantly less rich and diverse than the one harbored by healthy cows. In parallel, mastitis caused a strong disturbance in milk microbiota. Metabolomic profiles showed that eleven and twenty-eight molecules exhibited significant differences among the three groups in feces and milk, respectively. Similarly, to microbiota profile, milk metabolome was affected by mastitis more extensively than fecal metabolome, with particular reference to amino acids and sugars. Pathway analysis revealed that amino acids metabolism and energy metabolism could be considered as the main pathways altered by mastitis. These findings underscore the notable distinctions of fecal and milk samples among groups, from microbiome and metabolomic points of view. This observation stands to enhance our comprehension of mastitis in dairy cows.
1 Introduction
Mastitis is the disease that most often causes inflammation of cows’ mammary glands, due to the infection by various pathogenic bacteria, which leads serious problems to dairy cows’ health and, in turn, profitability of dairy farms (Ezzat Alnakip et al., 2014). Moreover, the excessive utilization of veterinary drugs for mastitis treatment could potentially engender a looming concern for human health, given the potential connection to antibiotic residues. An exceptionally critical facet in the battle against mastitis lies in the potential to achieve prompt, dependable, and precise disease diagnosis. In fact, mastitis, when subclinical, could spread unnoticed within a herd, resulting in more losses than clinical mastitis (Kaczorowski et al., 2022). Till now, the California mastitis test (CMT) and somatic cell count (SCC) are widely accepted as convenient and rapid methods to diagnose subclinical mastitis. However, several noninfectious factors can affect SCC, in turn leading to falsely positive results (Sargeant et al., 2001). Hence, the desirability of incorporating supplementary diagnostic methods emerges to augment the precision of cow mastitis detection. Metabolomics perfectly fulfill the above requirements, which can provide a platform for biomarker discovery and pathway identification of diseases. In our recent research endeavors focused on investigating the impact of clinical mastitis on cow biofluids, we have effectively employed 1H-NMR-based metabolomics to comprehensively analyze the metabolic distinctions between milk samples obtained from healthy cows and those afflicted with clinical mastitis (Zhu et al., 2021). Through the integration of findings from fecal, serum, and urinary analyses, it becomes evident that clinical mastitis exerts an influence on various pathways associated with energy metabolism and amino acid metabolism (Zhu et al., 2023).
Although previous studies by analysis of metabolic fingerprints have already rendered promising results, in terms of biomarkers identification and pathways elucidation, there is a growing recognition that the gut microbiota could potentially assume a pivotal role in upholding the physiological homeostasis of the host (Ma et al., 2018). Specifically, the entero-mammary pathway hypothesis has delineated a potential correlation between certain indigenous gut bacteria and the onset and progression of mastitis (Hu et al., 2020). In this context, Zhao et al. demonstrated that gut dysbiosis contributes to the pathogenesis of mastitis through the augmentation of bacterial translocation and systemic inflammation. In addition, administration of commensal Roseburia to mice was found to alleviate gut-dysbiosis-induced mastitis, by means of butyrate production, associated to barrier repair and reduced inflammation (Zhao et al., 2022).
To the best of our knowledge, the parallel analysis of metabolome and microbiome of feces and milk has only been conducted in a few studies (Wang et al., 2020; Hu et al., 2021a). Furthermore, the specific mechanism of mastitis remains not entirely clear. To examine the gut inner environment (microbiota and metabolites) and inflammatory reaction during mastitis, we collected feces and milk samples from healthy, subclinically mastitic and clinically mastitic cows. Then, we used 16S rRNA gene sequencing and untargeted metabolomics to analyze the profile of microbiota and metabolites in both feces and milk. These data provide further understanding of the correlations between gut and milk microbial community and metabolite profiles of dairy cows with differing udder health status, as well as a new perspective for the early diagnosis of dairy cows with subclinical mastitis.
2 Materials and methods
2.1 Animals and samples
All experimental designs and protocols were approved by the Animal Ethics Committee of Southwest Minzu University (Chengdu, China; approval number: SWUN-A-0050) and were in accordance with the recommendations of the academy’s guidelines for animal research.
The study was conducted during the period from July to August 2022. Chinese Holstein cows (parity: 2–4, days in milk: 150–195 days) were carefully chosen from a meticulously maintained large-scale dairy farm situated in the outskirts of Jiangsu, China. These cows were accommodated within free housing systems and were provided with total mixed rations (TMR) in adherence to established norms during their indoor tenure.
In the present study, the udder status of cows was comprehensively judged according to the degree of inflammation in each quarter, based on the clinical manifestations and on the results of the CMT and SCC in milk. Following the suggestions of Wang et al. (2022b) and according to the records, 30 Chinese Holstein cows were selected and divided into 3 groups: the H group was constituted by ten cows with healthy udder (SCC <100,000 cells/mL; no clinical symptoms of inflammation in udders; negative CMT results); the S group comprised ten cows with subclinical mastitis (500,000 < SCC < 800,000 cells/mL, no obvious clinical symptoms of inflammation in the udders, weakly positive CMT results); the C group was made by ten cows with clinical mastitis (SCC > 1,000,000 cells/mL; obvious signs of inflammation in the udders, including udder swelling, redness and milk clots, etc.; positive or strongly positive CMT results). Along the present work, the fecal samples from the three groups will be identified with FH, FS, and FC, while the corresponding milk samples will be identified by MH, MS, and MC. Milking procedures were conducted thrice daily through the utilization of an automated milking system (Ruishengyuan Machinery Assembly Co., Ltd., Hengshui, Hebei, China). To prevent the spread of inflammation through the entire dairy herd, cows designated as H, SM, and CM were housed in 3 separate cowsheds. None of the cows received treatments with antibiotics or other drugs. A total of 15 mL of composite milk was extracted in a sterile centrifuge tube from each animal, with roughly equivalent volumes obtained from each lactating udder quarter. For fecal sampling, sterile gloves were employed to collect rectal samples from each cow, with the initial few fecal streams being discarded prior to actual sample collection. Sampled feces were collected into sterile airtight bags, which were placed in a foam box with ice packs. Following the collection of fecal and milk samples, all specimens were promptly placed under refrigeration with ice to facilitate their transportation to the laboratory within a 2 h timeframe.
2.2 Metabolome analysis
The preparation of fecal and milk samples for 1H-NMR analysis followed the methods outlined by Zhu et al. (2021) and Zhang et al. (2024). Briefly, fecal samples were processed for 1H-NMR analysis by vortex mixing 80 mg of stool with 1 mL of deionized water for 5 min. The resulting mixtures were subsequently centrifuged at 18,630 g and 4°C for 15 min, and 0.7 mL of the supernatant was combined with 0.2 mL of NMR analysis solution. This solution consisted of D2O containing 10 mmol/L of 3-(trimethylsilyl)-propionic-2,2,3,3-d4 acid sodium salt (TSP) as the NMR chemical-shift reference, and 2 mmol/L of NaN3 to inhibit microbial growth. The pH of the solution was buffered at 7.00 ± 0.02 using 1 mol/L phosphate buffer.
For milk samples, 0.7 mL was mixed with 0.8 mL of CHCl3, then vortexed for 3 min and centrifuged at 18,630 g and 4°C for 15 min to remove fat. Afterward, 0.5 mL of the supernatant was combined with 0.2 mL of the NMR analysis solution. Each sample underwent another round of centrifugation under the aforementioned conditions.
The 1H-NMR spectra were acquired at 298 K using an AVANCE III spectrometer (Bruker, Wuhan, China) operating at a frequency of 600.13 MHz. Following the approach by Zhu et al. (2020), the suppression of signals from broad resonances originating from large molecules was accomplished using a CPMG-filter consisting of 400 echoes with a τ of 400 μs and a 180° pulse of 24 μs, resulting in a total filter duration of 330 ms. The residual signal from HOD was suppressed through presaturation using the cpmgpr1d sequence from the standard pulse sequence library.
Each spectrum was acquired by summing 256 transients with 32 K data points over a 7,184 Hz spectral window, employing an acquisition time of 2.28 s and a recycle delay of 5 s. Baseline adjustment of the 1H-NMR spectra was conducted using peak detection following the “rolling ball” principle (Kneen and Annegarn, 1996) and implemented using the baseline R package (Liland et al., 2010). To account for differences in water and fiber content among samples, probabilistic quotient normalization was applied to the entire spectrum array according to Dieterle et al. (2006). Signal assignments were made by comparing their chemical shift and multiplicity with the Chenomx software library (Chenomx Inc., Canada, ver 8.4), following the methodology of Zhu et al. (2020).
2.3 Microbiota analysis
Total microbial genomic DNA was extracted from fecal and milk samples using the FastDNA® Spin Kit for Soil (MP Biomedicals, United States) according to manufacturer’s instructions. The quality and concentration of DNA were determined by 1.0% agarose gel electrophoresis and a NanoDrop2000 spectrophotometer (Thermo Scientific, United States) and kept at −80°C prior to further use. This DNA served as the template for PCR amplification of bacterial 16S rRNA genes utilizing barcoded primers and Takara Ex Taq (Takara, China). The hypervariable region V3–V4 of the bacterial 16S rRNA gene were amplified with primer pairs 343F (5’-TACGGRAGGCAGCAG-3′) and 798R(5’-AGGGTATCTAATCCT-3′) by T100 Thermal Cycler PCR thermocycler (BIO-RAD, United States).
The PCR product was extracted from 2% agarose gel and purified using the PCR Clean-Up Kit according to manufacturer’s instructions and quantified using Qubit 4.0 (Thermo Fisher Scientific, United States). Purified amplicons were pooled in equimolar amounts and paired-end sequenced on an Illumina PE300 platform (Illumina, United States) according to the standard protocols.
Raw sequencing data was obtained in FASTQ format. Paired-end reads underwent initial preprocessing using the cutadapt software to identify and remove adapter sequences. Following adapter trimming, paired-end reads underwent further processing to eliminate low-quality sequences, denoising, merging, and detection of chimera reads using DADA2 within the QIIME2 (2020.11) framework (Callahan et al., 2016). This culminated in the acquisition of representative reads and an abundance table for amplicon sequence variants (ASVs). The selection of a representative read for each ASV was accomplished using the QIIME2 package (Bolyen et al., 2019). Subsequently, all representative reads underwent annotation and BLAST-based comparisons against the Silva database, Version 138 (16S rDNA) using the q2-feature-classifier with default parameters. The raw sequencing reads were deposited into the NCBI Sequence Read Archive (SRA) database (Accession Number: PRJNA1095683).
2.4 Statistical analysis
Statistical analysis was executed using the R computational language. ANOVA followed by the Tukey HSD test was employed to ascertain statistically significant variations in microbiota and molecules across the three groups. A significance threshold of p < 0.05 was adopted for this purpose. In instances where variables exhibited non-normal distribution, transformations were applied according to the methodology outlined by Box and Cox (1964).
To detect differences in microbial abundance among distinct groups and discern the contribution of the microbiota profile to these differences, Linear Discriminant Analysis Effect Size (LEfSe) and Linear Discriminant Analysis (LDA) were performed. These analyses were carried out using the Oebiotech Cloud Platform.1
To elucidate the underlying trends within the metabolome of the samples, a robust Principal Component Analysis (rPCA) model was constructed (Hubert et al., 2005), focusing on the molecules identified through the aforementioned univariate analysis. The model encompassed a score plot, visualizing sample projection within the PC space, designed to emphasize the inherent data structure. Additionally, a Pearson correlation plot was generated, correlating the concentration of each molecule with the model’s components.
Pathway enrichment analysis was conducted using MetaboAnalyst 5.0 (Chong et al., 2018) to identify pertinent pathways by amalgamating the results obtained from the analysis. This approach concentrated on molecules that demonstrated significant concentration variations as per the univariate analysis.
To investigate correlations between fecal microbiota and metabolites in both feces and milk, Spearman correlation analysis was performed using the aforementioned online tool.
3 Results
3.1 Fecal and milk microbiota among cows in different groups
A total of 15,860 ASV were obtained from the fecal and milk samples analyzed. The α-diversity of fecal and milk microbiota among the three groups showed significant differences, as shown in Tables 1, 2, respectively.
Referring to fecal samples, as for community richness, compared with the FH group, FS and FC groups showed lower ACE and observed species indexes. As for community diversity, Shannon indexes were significantly lower in the FC group compared with FH and FS groups. According to the number of ASV sampled, rarefaction curves were obtained, which leveled off as the number of tags increased, indicating sufficient sequencing depth (Supplementary Figure S1). Similar to fecal samples, as for community richness, MC samples showed lower values of ACE and observed species compared to MH and MS samples. As for community diversity, Simpson and Shannon indexes were significantly lower in the MS and MC samples, compared to MH. According to the number of ASV sampled, rarefaction curves were obtained, which leveled off as the number of tags increased, indicating sufficient sequencing depth (Supplementary Figure S2).
The difference of fecal and milk microbial profiles among groups were initially distinguished using β-diversity analysis, as shown in Figures 1A,B.
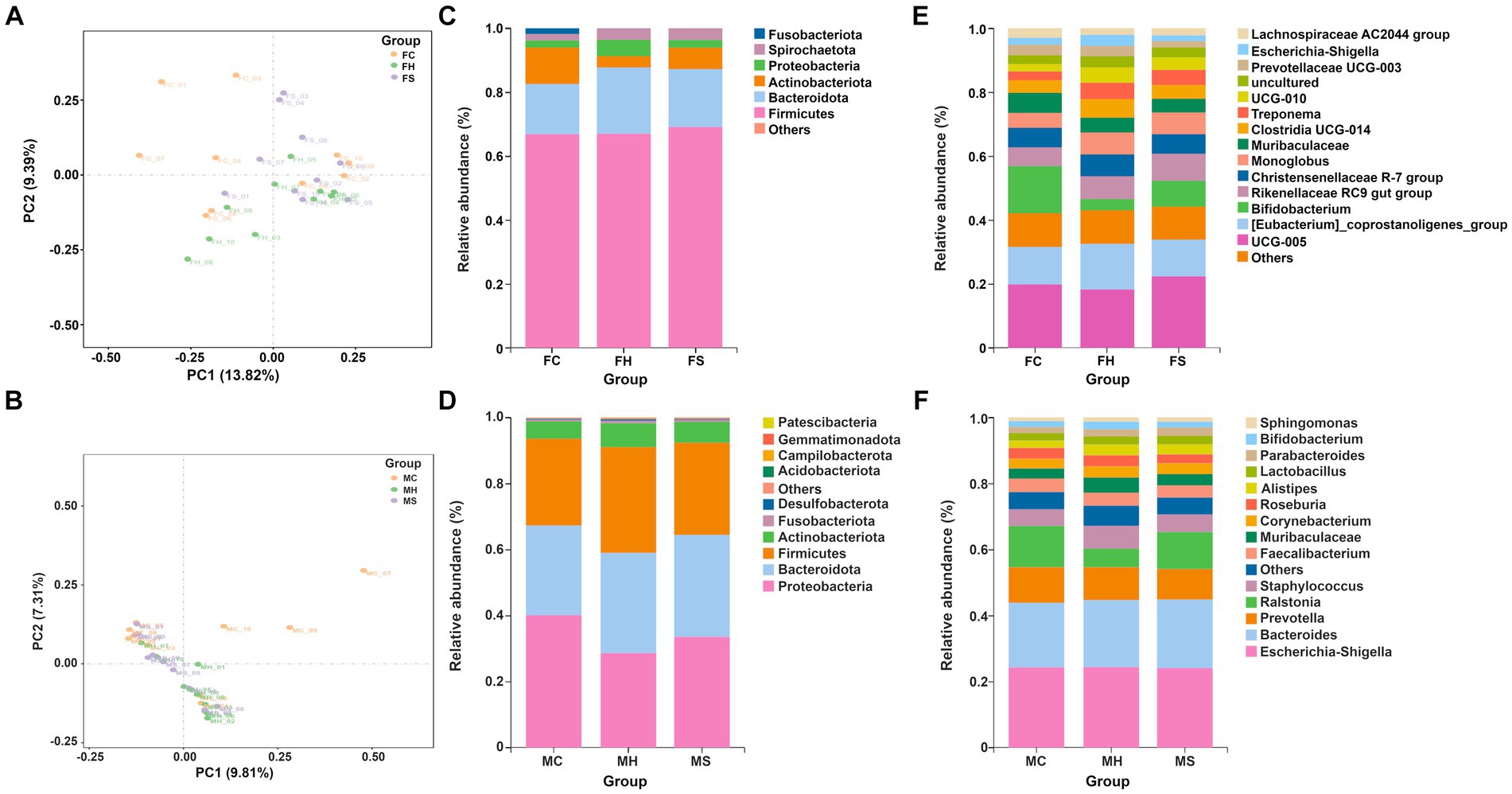
Figure 1. The β-diversity and microbiota compositions of fecal and milk among groups separately (n = 10). (A) PCA analysis for feces; (B) PCA analysis for milk; (C) Microbiota composition of feces at phyla level; (D) Microbiota composition of milk at phyla level; (E) Microbiota composition of feces at genus level; (F) Microbiota composition of feces at genus level.
As shown in Figure 1A, PCA analysis showed spatial separation in fecal microbiota among FH, FS, and FC groups. The distances between the FC and FH samples were greater than those between the FS and FH samples, indicating greater differences in fecal microorganism profiles between cows healthy and with clinical mastitis. In terms of milk samples, as depicted in Figure 1B, PCA analysis showed spatial separation in milk microbiota among MH, MS, and MC groups. The distances between the MC and MH group samples were greater than those between the MS and MH groups, indicating greater differences in milk microorganism profiles between cows with clinical mastitis and healthy.
Taxonomic annotation on ASV was conducted to identify the composition and relative abundance of fecal and milk microbiota. As shown in Figures 1C,D, Firmicutes, Bacteroidota, Actinobacteriota and Proteobacteria had the highest abundance in both fecal and milk samples. At the genus level, 136 genera were detected in all fecal microbiota, in which UCG-005, Bifidobacterium, Eubacterium_coprostanoligenes_group, and Rikenellaceae_RC9_gut_group predominated in fecal samples, as shown in Figure 1E. Moreover, among the detected genera, Escherichia-Shigella, Bacteroides, Prevotella and Ralstonia predominated in milk samples, as shown in Figure 1F.
Referring to fecal samples, as shown in Figures 2A,B, none of the bacterial phyla showed significant differences among the three groups. Among the detected genera, 14 (average relative abundance >0.10%) were significantly different among three groups. In detail, UCG-010, bacteroides, prevotella, ruminococcus, ralstonia, lachnospiraceae_NK4A136_group, clostridia vadinBB60_group, faecalibacterium, prevotellaceae UCG-004, parabacteroides, ruminiclostridium and sediminibacterium were more abundant in the FC group, whereas dgA-11 gut group tended to be less abundant in the FC group compared to the other groups.
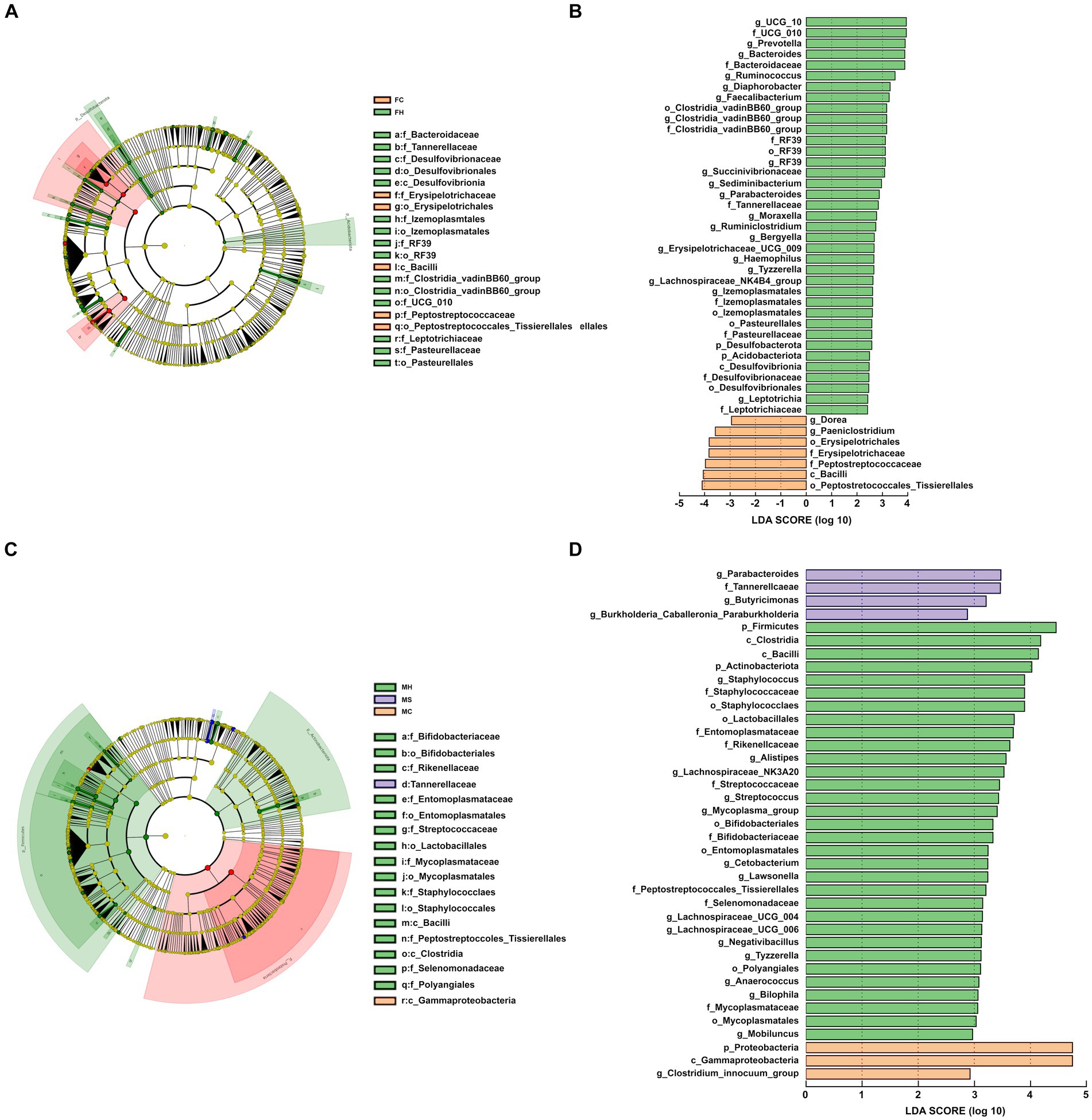
Figure 2. Cladogram shows linear discriminant analysis effect size (LEfSe) analysis of different microbiota among the three groups from phylum to genus level; linear discriminant analysis (LDA) score plot indicating the effects of different microbiota on difference among the three groups. (A,B) for feces; (C,D) for milk.
In terms of milk samples, as shown in Figures 2C,D, three of the bacterial phyla showed significant differences among the three groups (p < 0.05). Higher levels of Firmicutes and Actinobacteriota were registered in MH samples, while higher amounts of Proteobacteria were found in MC samples compared to the other two groups of samples. Among the detected genera, 11 (average relative abundance >0.10%) were significantly different among three groups. In detail, Alistipes, Bifidobacterium, Streptococcus, Lawsonella, Lacnnospiraceae UCG-004, Fusicatenibacter, Bilophila and Anaerococcus were more abundances in the MH group, whereas Bilophila and [clostridium] Innocuum group tended to be less abundant in the MH group compared with both other groups.
3.2 Fecal and milk metabolomic profiles among cows in different groups
Sixty-six and forty-six molecules were characterized by means of 1H-NMR in feces and milk samples, respectively. Their concentrations were provided in supporting materials. The characterized molecules could be sorted in six categories, namely carbohydrates and derivatives, organic acids and derivatives, amino acids, peptides, and derivatives, nucleosides, nucleotides, and analogues, alcohols, and miscellaneous Their relative concentrations were shown in Figures 3A,B. In fecal samples, eleven of the quantified molecules were significantly different among the three groups, namely glycine, O-acetylcholine, benzoate, glutamine, O-phosphocholine, tyrosine, creatine, methanol, valerate, acetoacetate, 1,3-dihydroxyacetone, pyruvate, propionate, acetate, 2,3-butanediol and ethanol. To gain a comprehensive insight into the trends exhibited by the identified molecules, their concentrations were utilized to construct a rPCA model, as depicted in Figure 2C. The first principal component (PC1) of its score plot, which encapsulates a substantial 60.3% of the total variability among the samples represented by the model, effectively captures the distinctions among samples from the three groups. Specifically, fecal samples obtained from cows experiencing clinical mastitis exhibited prominent features, characterized by elevated concentrations of propionate, acetate, pyruvate, acetoacetate, 2,3-butanediol, and ethanol. Conversely, lower concentrations were noted for glycine, O-acetylcholine, glutamine, O-phosphocholine, benzoate, tyrosine, and creatine.
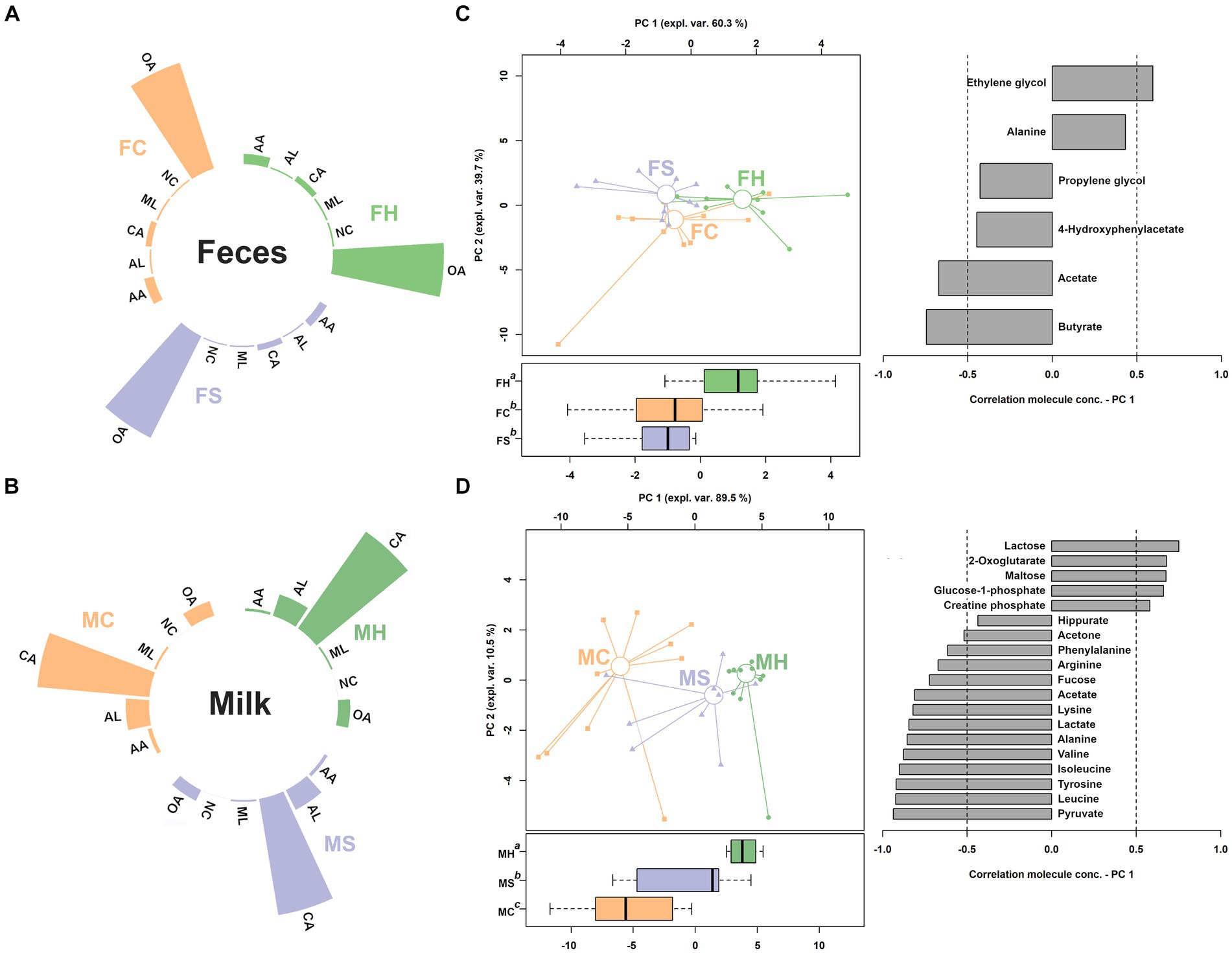
Figure 3. Relative abundance of the classes of molecules assigned in feces and milk metabolome (A,B). CA, carbohydrates and derivatives; OA, organic acids and derivatives; AA, amino acids, peptides, and derivatives; NC, nucleosides, nucleotides, and analogues; AL, alcohols; ML, miscellaneous. (C,D) rPCA models for feces and milk, respectively.
Among the quantified molecules in milk samples, a total of twenty-eight exhibited significant variations across the three groups. Similar to feces samples, their concentrations were employed as a basis for an rPCA model, as shown in Figure 3D. PC1 of its score plot, which captures a substantial 89.5% of the overall sample variability as depicted by the model, effectively encapsulates the distinctions among samples from the three groups. In detail, milk from clinical mastitis cows was found to be mainly characterized by higher concentrations of lactose, 2-oxoglutarate, maltose, glucose—phosphate and creatine phosphate, and lower levels of hippurate, acetone, phenylalanine, arginine, fucose, acetate, lysine, lactate, alanine, valine, isoleucine, tyrosine, leucine and pyruvate.
Molecules that exhibited significant concentration changes among the three groups were selected as the foundation for a pathway enrichment analysis aimed at identifying the most pertinent pathways distinguishing these groups. This analysis revealed nine highlighted pathways: pyruvate metabolism, alanine, aspartate, and glutamate metabolism, phenylalanine metabolism, phenylalanine, tyrosine, and tryptophan biosynthesis, D-glutamine and D-glutamate metabolism, glyoxylate and dicarboxylate metabolism, glycolysis/gluconeogenesis, glycine, serine, and threonine metabolism, and the citrate cycle (depicted in Figure 4).
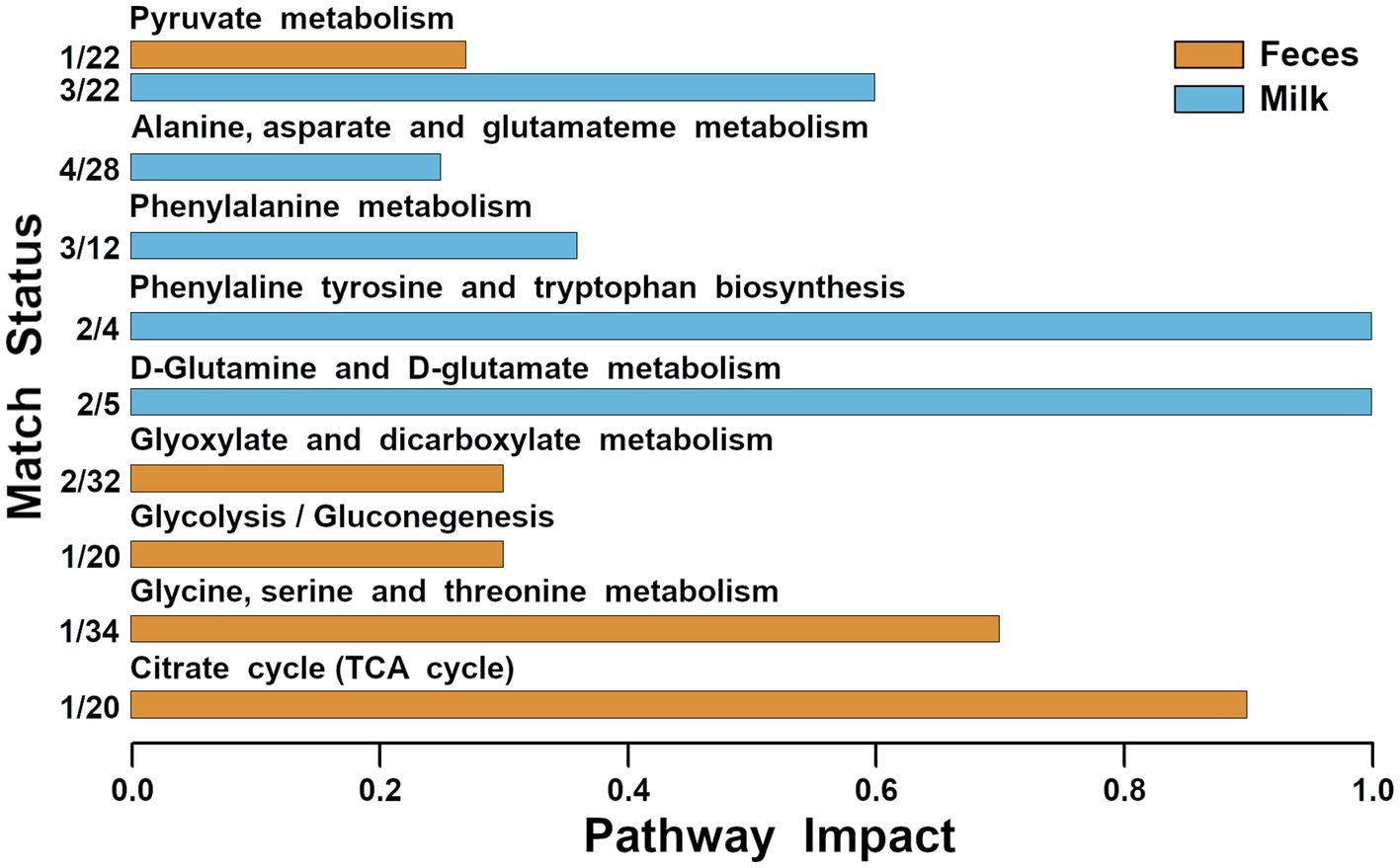
Figure 4. Metabolic pathways evidenced by enrichment analysis performed on the metabolites of feces and milk significantly different among the three groups (impact value >0.2).
3.3 Network analysis between microbiome and metabolome
Network analysis between microbiota and metabolites was performed, as shown in Figure 5. Metabolites play a central role in the network and there were correlations among their concentrations, such as glutamate, galactose, lactate and 3-hydroxybutyrate in milk. For microbiota, primarily the relative abundance of microbiota was correlated with each other. In milk, the relative abundance of Staphylococcus was correlated with that of Clostridium_innocuum_group. In feces, there was a correlation between the relative abundance of Ruminococcus and Dorea. Moreover, some correlations were also observed between the relative abundance of microbiota and the metabolite concentrations.
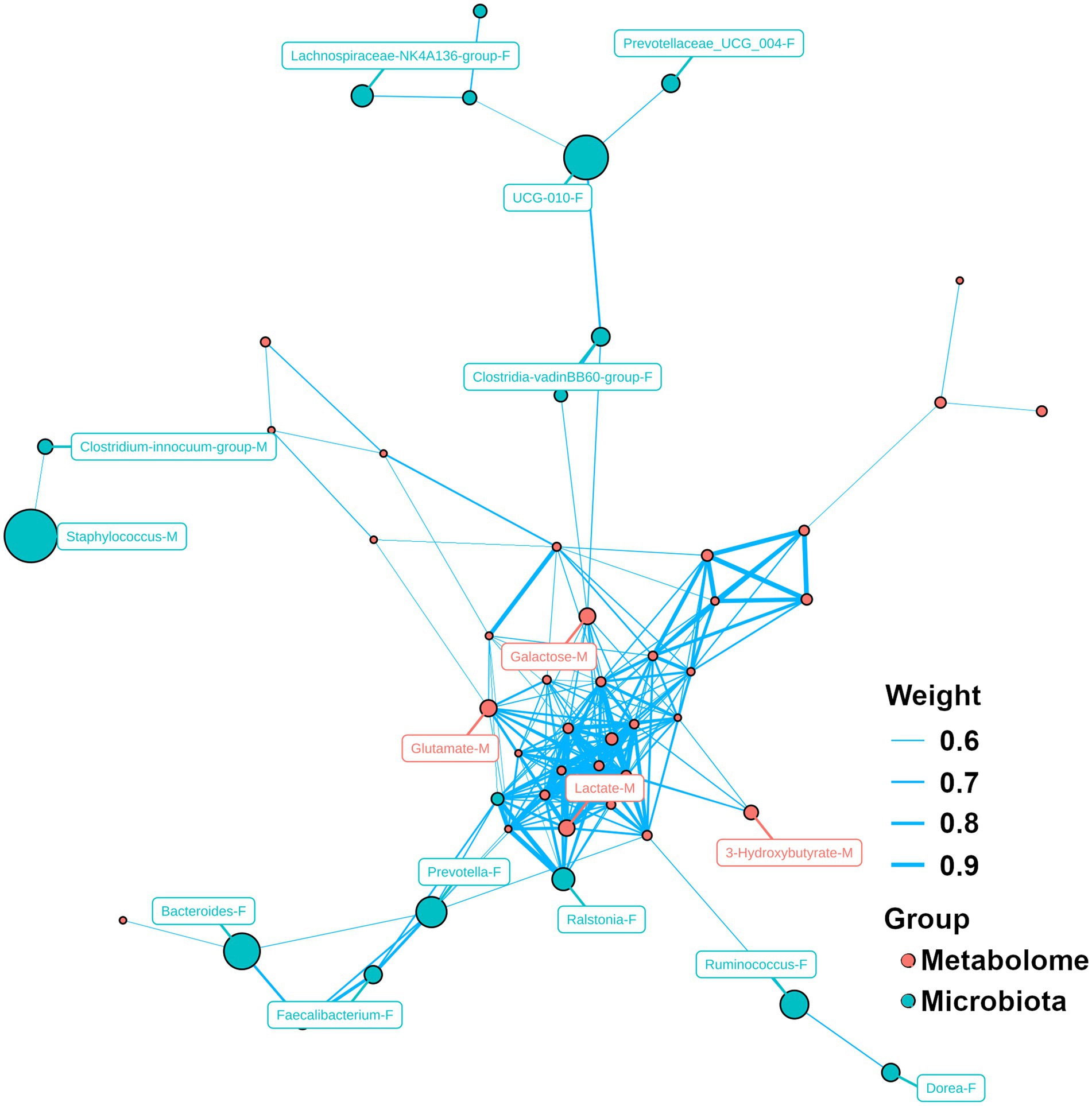
Figure 5. Network analysis for microbiome and metabolome. Capital letter (F and M) following by the name of bacteria/metabolite indicates the bacteria/molecule obtained from feces and milk, respectively.
4 Discussion
In recent decades, the timely diagnosis and treatment of dairy cow mastitis have emerged as pivotal aspects within the dairy industry. Addressing this matter holds significant importance not only for farmers and processing industries but also for consumers. Such efforts contribute to heightened sensitivity and awareness concerning animal health and welfare across the entire dairy chain, in particular for public health threats posed by zoonotic bacteria (Halasa et al., 2007; Wang et al., 2023; Teng et al., 2024). Furthermore, the entero-mammary pathway theory proposes a potential connection between certain indigenous gut bacteria and the onset and progression of mastitis (Hu et al., 2020). Till now, the combination of microbiota and metabolomic analyses has exhibited the potential ability to further investigate the mechanism of mastitis. However, researchers have paid sufficient attention to the changes of milk metabolome and microbiota affected by mastitis (Luangwilai et al., 2021; Wang et al., 2022b), while only a few papers devoted their attention to the other biofluids, such as feces (Wang et al., 2022a), serum (Dervishi et al., 2018; Zhang et al., 2022), and urine (Zwierzchowski et al., 2020). Moreover, limited data exist concerning the assessment of correlations between the microbiota and metabolome of feces and milk influenced by mastitis, despite its proven potential to offer a comprehensive understanding of an animal’s response to both internal and external stimuli (Zhu et al., 2019; Eom et al., 2020; Kim et al., 2021). Addressing these gaps, this study represents the initial endeavor to concurrently profile the microbiota and metabolites present in both feces and milk obtained from the same dairy cows, with or without mastitis, through the application of both 16S rRNA and 1H-NMR techniques.
In our study, the community richness and diversity were significantly decreased in feces of cows with clinical mastitis compared to healthy and subclinically mstitic individuals (10 individuals/group), in agreement with Wang et al. (2022a). Moreover, the predominant bacteria were similar to previous studies (Bronzo et al., 2020), both in at the levels of phyla and genus. Within the dominant population of beneficial bacteria, a range of Bacteroides species can be identified. These species play a crucial role in the breakdown of polysaccharides and oligosaccharides, thereby furnishing essential nutrients and vitamins to the host and the wider community of intestinal microorganisms (Zafar and Saier, 2021).
In feces samples, the concentrations of eleven molecules showed significant differences among clinically mastitic, subclinically mastitic and healthy individuals. Among them, six molecules were highlighted by rPCA model, because their concentrations were significantly correlated with their importance in determining PC 1 orientation. They revealed higher levels of ethylene glycol and alanine and lower levels of propylene glycol, 4-hydroxyphenylacetate, acetate and butyrate in the feces of healthy dairy cows compared to the individuals with mastitis. Such results were in line with the findings of our previous study that mastitis could promote energy metabolism, while amino acids metabolism could be suppressed (Zhu et al., 2023). Propylene glycol undergoes conversion to propionate within the rumen, and subsequent absorption leads to its transformation into glucose within the liver. Recent investigations have reported that administering propylene glycol treatment to cows with subclinical ketosis yielded positive outcomes. These cows displayed an increased likelihood of recovery, reduced susceptibility to clinical ketosis and displaced abomasum, elevated milk yield during the initial lactation month, enhanced conception rates in their first service, and diminished risk of culling (Jenkins et al., 2015). Hoedemaker et al. found that peripartal propylene glycol supplementation could have positive effects on the metabolism of cows in a short-term period, referring to significantly decrease the concentrations of no esterified fatty acids and β-hydroxybutyrate, while concentrations of insulin-like growth factor (IGF)-I were significantly higher (Hoedemaker et al., 2004). This phenomenon has been corroborated by Hubner et al., who found that propylene glycol supplementation had improvements in health and milk production of cows with hypoglycemic (Hubner et al., 2022). Moreover, propylene glycol serves as a vital source for gluconeogenesis in ruminants and effectively curtails ketone formation. Administering propylene glycol to perinatal dairy cows represents an effective strategy to mitigate negative energy imbalances (Zhang et al., 2020). Furthermore, Lomander et al. revealed that early lactation-stage supplementation with propylene glycol led to heightened milk yield without subsequent metabolic compromise (Lomander et al., 2012). Hu et al. documented that cold exposure impacted the excretion levels of 4-hydroxyphenylacetate, a compound implicated in gut microbiome metabolism (Hu et al., 2021b). Notably, an elevated concentration of 4-hydroxyphenylacetate could potentially serve as a biomarker for predicting metritis risk in dairy cows (Dervishi et al., 2018). Intriguingly, all molecules displaying elevated levels in mastitic dairy cows are linked to energy metabolism. Nearly all dietary carbohydrates undergo fermentation to volatile fatty acids (acetate, propionate, and butyrate) within the rumen of dairy cows, with propionate being the predominant substrate for gluconeogenesis (Zhang et al., 2015). Additionally, evidence supports the migration of short-chain fatty acids (SCFAs) from the rumen to the mammary gland through an endogenous route (Hu et al., 2023). Pyruvate, a pivotal component in gluconeogenesis and the end product of glycolysis (Denton and Halestrap, 1979), holds significance as an intermediate metabolite for generating propionate from the succinic pathway or the lactate pathway (Jeyanathan et al., 2014). Conversion via the pyruvate dehydrogenase complex yields acetyl-CoA, which subsequently enters the TCA cycle. The TCA cycle plays a central role in cellular respiration and energy supply to living cells (Grassian et al., 2014), critically influencing cellular metabolic efficiency, cow metabolism, and production (Antunes-Fernandes et al., 2016). Within this context, our study suggests that mastitis-induced alterations in energy-related metabolic pathways contribute to adverse effects on milk production (Bravo and Wall, 2016). Furthermore, SCFAs possess the ability to activate aryl hydrocarbon receptor expression in intestinal epithelial cells, subsequently inducing IL-22 production and participating in the preservation of gut barrier integrity (Mudaliar et al., 2016; Li et al., 2022). Gut commensal microbes’ production of SCFAs has been demonstrated to enhance barrier integrity. Butyrate, by inhibiting HDAC and regulating the actin-associated protein synaptopodin, influences epithelial homeostasis (Wang et al., 2022a). Activation of PPARc by microbiota-derived butyrate also contributes to sustaining barrier integrity (Byndloss et al., 2017).
Compared to healthy animals, concentrations of nine molecules exhibited significant elevation in the milk of cows with both subclinical and clinical mastitis. In addition, the levels of another five molecules significantly increased only in the milk of cows with clinical mastitis, compared to that of healthy ones. These above molecules include hippurate, acetone, phenylalanine, arginine, fucose, acetate, lysine, lactate, alanine, valine, isoleucine, tyrosine, leucine, and pyruvate. Notably, eight out of the fourteen molecules belong to the chemical group of amino acids. This observation aligns with prior studies that have reported heightened concentrations of free amino acids, such as arginine, valine, and isoleucine, in milk samples with clinical mastitis (Mansor, 2012; Sundekilde et al., 2013). Statistically significant increases in milk amino acids could be attributed to augmented pathogen-specific fermentative processes and protein degradation activities (Ritter and Hanni, 1960). Pathway analysis results for milk corroborate this phenomenon. Among the various pathways involved, the phenylalanine, tyrosine, and tryptophan biosynthesis pathway emerges as particularly noteworthy. Phenylalanine, an indispensable amino acid, can be enzymatically converted into tyrosine with the assistance of phenylalanine hydroxylase and a biopterin cofactor (Brown, 1992). This molecule also serves as a precursor to catecholamines, neurotransmitters, and adrenaline-like substances (Waisbren et al., 2007). Tyrosine, an essential amino acid, is a fundamental constituent of numerous proteins, peptides, and enkephalins. It also functions as the precursor for hormones like catecholoestrogens and thyroxin (Lemmon and Schlessinger, 2010). Lactate, a significant end product of carbohydrate metabolism, can be generated by milk microorganisms or through anaerobic epithelial respiration in oxygen-deprived conditions following mastitis (Kneen and Annegarn, 1996). The presence of bacteria in milk has been associated with a distinctive metabolic fingerprint characterized by elevated lactate levels (Davis et al., 2004). In line with previous reports, the approximately 30-fold change in lactate further supports this finding (Davis et al., 2004).
Several molecules exhibited a declining trend from healthy to mastitis-affected cows. These molecules primarily provided insights into energy generation (lactose, maltose, 2-oxoglutarate, and glucose-1-phosphate) or protein digestion (creatine phosphate). These findings are in consonance with previous research, which documented lower concentrations of carbohydrates and energy-related metabolites, such as 2-oxoglutarate, in the milk of cows with clinical mastitis (Clara and Dvm, 2015). Pathway analyses undertaken in this study also substantiate this observation, with pyruvate metabolism and TCA cycle pathways highlighted. Collectively, our findings imply that clinical mastitis perturbs the metabolite equilibrium in cow milk by disrupting the TCA cycle within the mammary gland. N-acetylglucosamine, an amidic derivative of glucose and a secondary amide linking glucosamine and acetic acid, stems from amino sugar metabolism (Boudonck et al., 2009). Lactose is exocytosed by mammary epithelial cells and might also play a role in milk coagulation, thereby influencing milk quality, yield, and composition (Sundekilde et al., 2011). Reduced lactose content is observed in the milk of ruminants with mammary gland inflammation. The integrity of mammary cell membranes is compromised during clinical mastitis, allowing blood constituents to enter the milk (Bannerman, 2009). To maintain consistent osmotic pressure, lactose levels decrease accordingly.
5 Conclusion
In the current study, for the first time, we attempted to investigate differences in the microbiota profile and metabolite composition in feces and milk from healthy cows and from those with subclinical and clinical mastitis. Compared with those of healthy cows, the microbial community richness and diversity in the feces and milk of cows with clinical mastitis were significantly lower. The differences of feces microbiota were mainly evident at the level of genera. Mastitis caused also a strong disturbance in milk microbiota. Similarly, to microbiota profile, milk metabolome was affected by mastitis more deeply than fecal metabolome. Pathway analysis showed that amino acids metabolism and energy metabolism could be considered as the main pathways altered by mastitis. Further study would be benefited from enlarged sample size and the influence of animal host genetics would be considered due to their variated mastitis susceptibility, and microbial and metabolomic responses. Anyway, these findings would enrich the knowledge about the profiles of feces and milk microbiota and metabolites in cows with different udder health status, which might suggest new ways to prevent and treat mastitis, less reliant on drugs with potentially huge long-term side effects.
Data availability statement
The original contributions presented in the study are publicly available. This data can be found in the NCBI Sequence Read Archive (SRA) database [Accession Number: PRJNA1095683].
Ethics statement
The animal study was approved by the Animal Ethics Committee of Southwest Minzu University. The study was conducted in accordance with the local legislation and institutional requirements.
Author contributions
CZ: Conceptualization, Data curation, Formal analysis, Investigation, Methodology, Writing – review & editing, Writing – original draft. YZ: Writing – original draft, Writing – review & editing. FY: Writing – review & editing, Writing – original draft, Supervision, Project administration, Methodology, Funding acquisition, Conceptualization. QZ: Writing – review & editing, Writing – original draft, Investigation, Formal analysis. XZ: Writing – review & editing, Writing – original draft. ZY: Writing – review & editing, Writing – original draft, Formal analysis. XD: Writing – review & editing, Writing – original draft. LL: Writing – review & editing, Writing – original draft, Methodology.
Funding
The author(s) declare that financial support was received for the research, authorship, and/or publication of this article. This research was funded by Southwest Minzu University Double World-Class Project (XM2023012) and Sichuan provincial Key R&D projects (2021YFN0008).
Conflict of interest
The authors declare that the research was conducted in the absence of any commercial or financial relationships that could be construed as a potential conflict of interest.
The author(s) declared that they were an editorial board member of Frontiers, at the time of submission. This had no impact on the peer review process and the final decision.
Publisher’s note
All claims expressed in this article are solely those of the authors and do not necessarily represent those of their affiliated organizations, or those of the publisher, the editors and the reviewers. Any product that may be evaluated in this article, or claim that may be made by its manufacturer, is not guaranteed or endorsed by the publisher.
Supplementary material
The Supplementary material for this article can be found online at: https://www.frontiersin.org/articles/10.3389/fmicb.2024.1374911/full#supplementary-material
Footnotes
References
Antunes-Fernandes, E. C., van Gastelen, S., Dijkstra, J., Hettinga, K. A., and Vervoort, J. (2016). Milk metabolome relates enteric methane emission to milk synthesis and energy metabolism pathways. J. Dairy Sci. 99, 6251–6262. doi: 10.3168/jds.2015-10248
Bannerman, D. D. (2009). Pathogen-dependent induction of cytokines and other soluble inflammatory mediators during intramammary infection of dairy cows1. J. Anim. Sci. 87, 10–25. doi: 10.2527/jas.2008-1187
Bolyen, E., Rideout, J. R., Dillon, M. R., Bokulich, N. A., Abnet, C. C., Al-Ghalith, G. A., et al. (2019). Reproducible, interactive, scalable and extensible microbiome data science using QIIME 2. Nat. Biotechnol. 37, 852–857. doi: 10.1038/s41587-019-0209-9
Boudonck, K. J., Mitchell, M. W., Wulff, J., and Ryals, J. A. (2009). Characterization of the biochemical variability of bovine milk using metabolomics. Metabolomics 5, 375–386. doi: 10.1007/s11306-009-0160-8
Box, G. E. P., and Cox, D. R. (1964). An analysis of transformations. J. R. Stat. Soc. 26, 211–243. doi: 10.1111/j.2517-6161.1964.tb00553.x
Bravo, D. M., and Wall, E. H. (2016). The rumen and beyond: nutritional physiology of the modern dairy cow. J. Dairy Sci. 99, 4939–4940. doi: 10.3168/jds.2015-10191
Bronzo, V., Lopreiato, V., Riva, F., Amadori, M., Curone, G., Addis, M. F., et al. (2020). The role of innate immune response and microbiome in resilience of dairy cattle to disease: the mastitis model. Animals 10:81397. doi: 10.3390/ani10081397
Brown, B. (1992). Basic concepts in biochemistry: a Student’s survival guide. Biochem. Educ. 20:186. doi: 10.1016/0307-4412(92)90079-2
Byndloss, M. X., Olsan, E. E., Rivera-Chávez, F., Tiffany, C. R., Cevallos, S. A., Lokken, K. L., et al. (2017). Microbiota-activated PPAR-γ signaling inhibits dysbiotic Enterobacteriaceae expansion. Science 357, 570–575. doi: 10.1126/science.aam9949
Callahan, B. J., McMurdie, P. J., Rosen, M. J., Han, A. W., Johnson, A. J. A., and Holmes, S. P. (2016). DADA2: high-resolution sample inference from Illumina amplicon data. Nat. Methods 13, 581–583. doi: 10.1038/nmeth.3869
Chong, J., Soufan, O., Li, C., Caraus, I., Li, S., Bourque, G., et al. (2018). MetaboAnalyst 4.0: towards more transparent and integrative metabolomics analysis. Nucleic Acids Res. 46, W486–W494. doi: 10.1093/nar/gky310
Clara, F., and Dvm, T. (2015). Acute phase proteins, proteomics and metabolomics in the diagnosis of bovine mastitis
Davis, S. R., Farr, V. C., Prosser, C. G., Nicholas, G. D., Turner, S.-A., Lee, J., et al. (2004). Milk L-lactate concentration is increased during mastitis. J. Dairy Res. 71, 175–181. doi: 10.1017/S002202990400007X
Denton, R. M., and Halestrap, A. P. (1979). Regulation of pyruvate metabolism in mammalian tissues. Essays Biochem. 15, 37–77
Dervishi, E., Zhang, G., Hailemariam, D., Mandal, R., Wishart, D. S., and Ametaj, B. N. (2018). Urine metabolic fingerprinting can be used to predict the risk of metritis and highlight the pathobiology of the disease in dairy cows. Metabolomics 14:83. doi: 10.1007/s11306-018-1379-z
Dieterle, F., Ross, A., Schlotterbeck, G., and Senn, H. (2006). Probabilistic Quotient Normalization as Robust Method to Account for Dilution of Complex Biological Mixtures. Application in 1H NMR Metabonomics. Anal. Chem. 78, 4281–4290. doi: 10.1021/ac051632c
Eom, J. S., Lee, S. J., Kim, H. S., Choi, Y. Y., Kim, S. H., Lee, Y. G., et al. (2020). Metabolomics comparison of Hanwoo (Bos taurus coreanae) biofluids using proton nuclear magnetic resonance spectroscopy. Meta 10:333. doi: 10.3390/metabo10080333
Ezzat Alnakip, M., Quintela-Baluja, M., Böhme, K., Fernández-No, I., Caamaño-Antelo, S., Calo-Mata, P., et al. (2014). The immunology of mammary gland of dairy ruminants between healthy and inflammatory conditions. J. Vet. Med. 2014, 1–31. doi: 10.1155/2014/659801
Grassian, A. R., Parker, S. J., Davidson, S. M., Divakaruni, A. S., Green, C. R., Zhang, X., et al. (2014). IDH1 mutations alter citric acid cycle metabolism and increase dependence on oxidative mitochondrial metabolism. Cancer Res. 74, 3317–3331. doi: 10.1158/0008-5472.CAN-14-0772-T
Halasa, T., Huijps, K., Østerås, O., and Hogeveen, H. (2007). Economic effects of bovine mastitis and mastitis management: a review. Vet. Q. 29, 18–31. doi: 10.1080/01652176.2007.9695224
Hoedemaker, M., Prange, D., Zerbe, H., Frank, J., Daxenberger, A., and Meyer, H. H. D. (2004). Peripartal propylene glycol supplementation and metabolism, animal health, fertility, and production in dairy cows. J. Dairy Sci. 87, 2136–2145. doi: 10.3168/jds.S0022-0302(04)70033-8
Hu, L., Brito, L. F., Abbas, Z., Sammad, A., Kang, L., Wang, D., et al. (2021b). Investigating the short-term effects of cold stress on metabolite responses and metabolic pathways in inner-Mongolia Sanhe cattle. Animals 11:2493. doi: 10.3390/ani11092493
Hu, H., Fang, Z., Mu, T., Wang, Z., Ma, Y., and Ma, Y. (2021a). Application of metabolomics in diagnosis of cow mastitis: a review. Front Vet Sci 8:747519. doi: 10.3389/fvets.2021.747519
Hu, X., Guo, J., Zhao, C., Jiang, P., Maimai, T., Yanyi, L., et al. (2020). The gut microbiota contributes to the development of Staphylococcus aureus-induced mastitis in mice. ISME J. 14, 1897–1910. doi: 10.1038/s41396-020-0651-1
Hu, X., He, Z., Zhao, C., He, Y., Qiu, M., Xiang, K., et al. (2023). Gut/rumen-mammary gland axis in mastitis: gut/rumen microbiota-mediated gastroenterogenic mastitis. J. Adv. Res. 55, 159–171. doi: 10.1016/j.jare.2023.02.009
Hubert, M., Rousseeuw, P. J., and Vanden Branden, K. (2005). ROBPCA: a new approach to robust principal component analysis. Technometrics 47, 64–79. doi: 10.1198/004017004000000563
Hubner, A. M., Canisso, I. F., Coelho, W. M., Ribeiro, L., Aldridge, B. M., and Lima, F. S. (2022). A randomized controlled trial examining the effects of treatment with propylene glycol and injectable cyanocobalamin on naturally occurring disease, milk production, and reproductive outcomes of dairy cows diagnosed with concurrent hyperketonemia and hypoglycemia. J. Dairy Sci. 105, 9070–9083. doi: 10.3168/jds.2021-21328
Jenkins, N. T., Peña, G., Risco, C., Barbosa, C. C., Vieira-Neto, A., and Galvão, K. N. (2015). Utility of inline milk fat and protein ratio to diagnose subclinical ketosis and to assign propylene glycol treatment in lactating dairy cows. Can. Vet. J. 56, 850–854
Jeyanathan, J., Martin, C., and Morgavi, D. P. (2014). The use of direct-fed microbials for mitigation of ruminant methane emissions: a review. Animal 8, 250–261. doi: 10.1017/S1751731113002085
Kaczorowski, Ł., Powierska-Czarny, J., Wolko, Ł., Piotrowska-Cyplik, A., Cyplik, P., and Czarny, J. (2022). The influence of bacteria causing subclinical mastitis on the structure of the cow’s milk microbiome. Molecules 27:1829. doi: 10.3390/molecules27061829
Kim, H. S., Kim, E. T., Eom, J. S., Choi, Y. Y., Lee, S. J., Lee, S. S., et al. (2021). Exploration of metabolite profiles in the biofluids of dairy cows by proton nuclear magnetic resonance analysis. PLoS One 16:e0246290. doi: 10.1371/journal.pone.0246290
Kneen, M. A., and Annegarn, H. J. (1996). Algorithm for fitting XRF, SEM and PIXE X-ray spectra backgrounds. Nucl Instrum Methods Phys Res B 109–110, 209–213. doi: 10.1016/0168-583X(95)00908-6
Lemmon, M. A., and Schlessinger, J. (2010). Cell signaling by receptor tyrosine kinases. Cell 141, 1117–1134. doi: 10.1016/j.cell.2010.06.011
Li, Y., Wang, X., Su, Y., Wang, Q., Huang, S., Pan, Z., et al. (2022). Baicalein ameliorates ulcerative colitis by improving intestinal epithelial barrier via AhR/IL-22 pathway in ILC3s. Acta Pharmacol. Sin. 43, 1495–1507. doi: 10.1038/s41401-021-00781-7
Liland, K. H., Almøy, T., and Mevik, B.-H. (2010). Optimal choice of baseline correction for multivariate calibration of spectra. Appl. Spectrosc. 64, 1007–1016. doi: 10.1366/000370210792434350
Lomander, H., Frössling, J., Ingvartsen, K. L., Gustafsson, H., and Svensson, C. (2012). Supplemental feeding with glycerol or propylene glycol of dairy cows in early lactation—effects on metabolic status, body condition, and milk yield. J. Dairy Sci. 95, 2397–2408. doi: 10.3168/jds.2011-4535
Luangwilai, M., Duangmal, K., Chantaprasarn, N., and Settachaimongkon, S. (2021). Comparative metabolite profiling of raw milk from subclinical and clinical mastitis cows using 1 H-NMR combined with chemometric analysis. Int. J. Food Sci. Technol. 56, 493–503. doi: 10.1111/ijfs.14665
Ma, C., Sun, Z., Zeng, B., Huang, S., Zhao, J., Zhang, Y., et al. (2018). Cow-to-mouse fecal transplantations suggest intestinal microbiome as one cause of mastitis. Microbiome 6:200. doi: 10.1186/s40168-018-0578-1
Mudaliar, M., Tassi, R., Thomas, F. C., McNeilly, T. N., Weidt, S. K., McLaughlin, M., et al. (2016). Mastitomics, the integrated omics of bovine milk in an experimental model of: Streptococcus uberis mastitis: 2 label free-relative quantitative proteomics. Mol. Biosyst. 12, 2748–2761. doi: 10.1039/c6mb00290k
Ritter, W, and Hanni, H (1960). The application of gas chromatography in dairying: II. Detection and determination of volatile fatty acids in dairy products and cultures
Sargeant, J. M., Leslie, K. E., Shirley, J. E., Pulkrabek, B. J., and Lim, G. H. (2001). Sensitivity and specificity of somatic cell count and California mastitis test for identifying intramammary infection in early lactation. J. Dairy Sci. 84, 2018–2024. doi: 10.3168/jds.S0022-0302(01)74645-0
Sundekilde, U. K., Frederiksen, P. D., Clausen, M. R., Larsen, L. B., and Bertram, H. C. (2011). Relationship between the metabolite profile and technological properties of bovine milk from two dairy breeds elucidated by NMR-based metabolomics. J. Agric. Food Chem. 59, 7360–7367. doi: 10.1021/jf202057x
Sundekilde, U. K., Poulsen, N. A., Larsen, L. B., and Bertram, H. C. (2013). Nuclear magnetic resonance metabonomics reveals strong association between milk metabolites and somatic cell count in bovine milk. J. Dairy Sci. 96, 290–299. doi: 10.3168/jds.2012-5819
Teng, L., Huang, L., Zhou, H., Wang, B., Yue, M., and Li, Y. (2024). Microbiological hazards in infant and toddler food in China: a comprehensive study between 2004 and 2022. Food Res. Int. 180:114100. doi: 10.1016/j.foodres.2024.114100
Waisbren, S. E., Noel, K., Fahrbach, K., Cella, C., Frame, D., Dorenbaum, A., et al. (2007). Phenylalanine blood levels and clinical outcomes in phenylketonuria: a systematic literature review and meta-analysis. Mol. Genet. Metab. 92, 63–70. doi: 10.1016/j.ymgme.2007.05.006
Wang, Z., Liao, S., Huang, G., Feng, M., Yin, R., Teng, L., et al. (2023). Infant food production environments: a potential reservoir for vancomycin-resistant enterococci non-nosocomial infections. Int. J. Food Microbiol. 389:110105. doi: 10.1016/j.ijfoodmicro.2023.110105
Wang, Y., Nan, X., Zhao, Y., Jiang, L., Wang, H., Zhang, F., et al. (2022a). Consumption of supplementary inulin modulates milk microbiota and metabolites in dairy cows with subclinical mastitis. Appl. Environ. Microbiol. 88:e0205921. doi: 10.1128/aem.02059-21
Wang, Y., Nan, X., Zhao, Y., Jiang, L., Wang, H., Zhang, F., et al. (2022b). Discrepancies among healthy, subclinical mastitic, and clinical mastitic cows in fecal microbiome and metabolome and serum metabolome. J. Dairy Sci. 105, 7668–7688. doi: 10.3168/jds.2021-21654
Wang, Y., Nan, X., Zhao, Y., Wang, H., Wang, M., Jiang, L., et al. (2020). Coupling 16S rDNA sequencing and untargeted mass spectrometry for milk microbial composition and metabolites from dairy cows with clinical and subclinical mastitis. J. Agric. Food Chem. 68, 8496–8508. doi: 10.1021/acs.jafc.0c03738
Zafar, H., and Saier, M. H. (2021). Gut bacteroides species in health and disease. Gut Microbes 13, 1–20. doi: 10.1080/19490976.2020.1848158
Zhang, Q., Koser, S. L., Bequette, B. J., and Donkin, S. S. (2015). Effect of propionate on mRNA expression of key genes for gluconeogenesis in liver of dairy cattle. J. Dairy Sci. 98, 8698–8709. doi: 10.3168/jds.2015-9590
Zhang, F., Nan, X., Wang, H., Zhao, Y., Guo, Y., and Xiong, B. (2020). Effects of propylene glycol on negative energy balance of postpartum dairy cows. Animals 10:1526. doi: 10.3390/ani10091526
Zhang, G., Tobolski, D., Zwierzchowski, G., Mandal, R., Wishart, D. S., and Ametaj, B. N. (2022). Identification of serum-predictive biomarkers for subclinical mastitis in dairy cows and new insights into the pathobiology of the disease. J. Agric. Food Chem. 70, 1724–1746. doi: 10.1021/acs.jafc.1c07281
Zhang, Q., Zeng, R., Tang, J., Jiang, X., and Zhu, C. (2024). The “crosstalk” between microbiota and metabolomic profile in high-fat-diet-induced obese mice supplemented with Bletilla striata polysaccharides and composite polysaccharides. Int. J. Biol. Macromol. 262:130018. doi: 10.1016/j.ijbiomac.2024.130018
Zhao, C., Bao, L., Qiu, M., Wu, K., Zhao, Y., Feng, L., et al. (2022). Commensal cow Roseburia reduces gut-dysbiosis-induced mastitis through inhibiting bacterial translocation by producing butyrate in mice. Cell Rep. 41:111681. doi: 10.1016/j.celrep.2022.111681
Zhu, C., Li, C., Wang, Y., and Laghi, L. (2019). Characterization of yak common biofluids metabolome by means of proton nuclear magnetic resonance spectroscopy. Meta 9:e41. doi: 10.3390/metabo9030041
Zhu, C., Petracci, M., Li, C., Fiore, E., and Laghi, L. (2020). An untargeted metabolomics investigation of Jiulong yak (Bos grunniens) meat by 1H-NMR. Food Secur. 9:481. doi: 10.3390/foods9040481
Zhu, C., Tang, K., Lu, X., Tang, J., and Laghi, L. (2021). An untargeted metabolomics investigation of milk from dairy cows with clinical mastitis by 1H-NMR. Food Secur. 10:1707. doi: 10.3390/foods10081707
Zhu, C., Zhang, Q., Zhao, X., Yang, Z., Yang, F., Yang, Y., et al. (2023). Metabolomic analysis of multiple biological specimens (feces, serum, and urine) by 1H-NMR spectroscopy from dairy cows with clinical mastitis. Animals 13:741. doi: 10.3390/ani13040741
Keywords: dairy cows, mastitis, milk, feces, microbiome, metabolome
Citation: Zhu C, Zhao Y, Yang F, Zhang Q, Zhao X, Yang Z, Dao X and Laghi L (2024) Microbiome and metabolome analyses of milk and feces from dairy cows with healthy, subclinical, and clinical mastitis. Front. Microbiol. 15:1374911. doi: 10.3389/fmicb.2024.1374911
Edited by:
Min Yue, University of Chinese Academy of Science, ChinaReviewed by:
Joao Carlos Gomes-Neto, The Ohio State University, United StatesPaola Cremonesi, National Research Council (CNR), Italy
Copyright © 2024 Zhu, Zhao, Yang, Zhang, Zhao, Yang, Dao and Laghi. This is an open-access article distributed under the terms of the Creative Commons Attribution License (CC BY). The use, distribution or reproduction in other forums is permitted, provided the original author(s) and the copyright owner(s) are credited and that the original publication in this journal is cited, in accordance with accepted academic practice. No use, distribution or reproduction is permitted which does not comply with these terms.
*Correspondence: Falong Yang, falong.yang@swun.edu.cn