- 1Division of Systems Bioengineering, Department of Bioscience and Biotechnology, Faculty of Agriculture, Kyushu University, Fukuoka, Japan
- 2Laboratory of Physiology and Pathology of Young Animals, Institute of Veterinary Medicine, Mongolian University of Life Sciences, Ulaanbaatar, Mongolia
- 3Division of Biotechnology, School of Agro-Industry, Faculty of Agro-Industry, Chiang Mai University, Chiang Mai, Thailand
- 4Department of Endocrinology, The First Central Hospital of Mongolia, Ulaanbaatar, Mongolia
- 5Department of Endocrinology, Mongolian National University of Medical Sciences, Ulaanbaatar, Mongolia
- 6Department of Microbiology and Infection Prevention Control, Mongolian National University of Medical Sciences, Ulaanbaatar, Mongolia
- 7Department of the Gastroenterology, Mongolian National University of Medical Sciences, Ulaanbaatar, Mongolia
- 8Department of Microbiology and Immunology, National University of Singapore, Singapore, Singapore
Mongolian people possess a unique dietary habit characterized by high consumption of meat and dairy products and fewer vegetables, resulting in the highest obesity rate in East Asia. Although obesity is a known cause of type 2 diabetes (T2D), the T2D rate is moderate in this population; this is known as the “Mongolian paradox.” Since the gut microbiota plays a key role in energy and metabolic homeostasis as an interface between food and body, we investigated gut microbial factors involved in the prevention of the co-occurrence of T2D with obesity in Mongolians. We compared the gut microbiome and metabolome of Mongolian adults with obesity with T2D (DO: n = 31) or without T2D (NDO: n = 35). Dysbiotic signatures were found in the gut microbiome of the DO group; lower levels of Faecalibacterium and Anaerostipes which are known as short-chain fatty acid (SCFA) producers and higher levels of Methanobrevibacter, Desulfovibrio, and Solobacterium which are known to be associated with certain diseases. On the other hand, the NDO group exhibited a higher level of fecal SCFA concentration, particularly acetate. This is consistent with the results of the whole shotgun metagenomic analysis, which revealed a higher relative abundance of SCFA biosynthesis-related genes encoded largely by Anaerostipes hadrus in the NDO group. Multiple logistic regression analysis including host demographic parameters indicated that acetate had the highest negative contribution to the onset of T2D. These findings suggest that SCFAs produced by the gut microbial community participate in preventing the development of T2D in obesity in Mongolians.
1 Introduction
In Asia, lifestyle-related diseases are known to be associated with social problems. In particular, East Asia, where there have been rapid changes in diet owing to the introduction of an urban lifestyle, is facing an epidemic of metabolic diseases. In Asian people, however, metabolic diseases arise not only from diet but also from phenotypic and genotypic factors (Ramachandran et al., 2012; Mathis et al., 2022). Notably, East Asians tend to have higher abdominal obesity than other ethnic groups (Ramachandran et al., 2012); this is associated with their susceptibility to glucose homeostasis (Hu, 2011; Kodama et al., 2013) and lower pancreatic β-cell mass, which predisposes them to impaired insulin secretion even in relatively lean subjects. In fact, the type 2 diabetes (T2D) risk is higher in lower-body mass index (BMI) Asian populations than in lower-BMI Western populations (Chan et al., 2009).
The gut microbiota plays a key role in metabolic control and homeostasis (Pinart et al., 2022; Zhou et al., 2022). It is reported that the gut microbiome exhibits lower alpha-diversity and a different community structure in obesity than non-obesity people (Therdtatha et al., 2021; Pinart et al., 2022). Gut microbiota dysbiosis, in which reduced gut barrier function enables gut microbes and their components to enter the bloodstream, is associated with chronic diseases, including T2D (Scheithauer et al., 2020; Yang et al., 2021; Mishra et al., 2023). In contrast, microbiota-secreted short-chain fatty acids (SCFAs), specifically acetate, propionate, and butyrate, play important roles in preventing metabolic disorders by maintaining both metabolic homeostasis and the gut barrier, and via their anti-inflammatory activity (Portincasa et al., 2022). Levels of Roseburia and Faecalibacterium, SCFA-producing bacteria, are reduced in patients with T2D (Martínez-López et al., 2022).
Mongolia, a landlocked East Asian country, exhibits a unique dietary habit characterized by high consumption of animal products and low consumption of plant matter, established traditionally under a nomadic lifestyle adapted to the dry climate (Bromage et al., 2020; Delgermaa et al., 2023). This leads to insufficient dietary intake of particular components, including fiber, folate, and vitamin D, and can lead to obesity (Bromage et al., 2020). In 2016, 20.4% of the population of Mongolia suffered from obesity (BMI > 30) (NCD Risk Factor Collaboration, 2020), the highest prevalence among East Asian countries. However, the incidence of lifestyle-related diseases such as diabetes and coronary heart disease is lower than expected; this is recognized as the “Mongolian paradox” (Torii et al., 2012). This is possibly explained by their unique dietary habit; extremely high consumption of dairy products, particularly fermented animal milk with probiotic effect, and also supplementary consumption of medical plants and herbs with antioxidant effects (Torii et al., 2012).
Gut microbiota of Mongolians has attracted interest in relation to their unique dietary habit. The first study on the gut microbiota of Mongolian people (Zhang et al., 2014) examined regional and seasonal differences, finding that the core intestinal microbiota comprised Prevotella, Bacteroides, and Faecalibacterium. A comparative study with Han and European populations found that Faecalibacterium prausnitzii and Coprococcus comes are enriched in Mongolian people which may contribute to their health through the production of SCFA, notably butyrate with anti-inflammatory activity (Liu et al., 2016). Our earlier study (Shinoda et al., 2021) showed that the general Mongolian population has Prevotella-dominant microbiome in their gut and that lactic acid bacteria are enriched in Mongolian relative to other Asian populations.
The gut microbiota is now recognized as an interface between foods and host health, notably energy and metabolic homeostasis. In this context, the gut microbiome has to be investigated to address the Mongolian paradox. Therefore, we herein investigated gut microbial factors involved in the prevention of the co-occurrence of T2D with obesity in Mongolians, by comparing the gut microbiome of Mongolian individuals with obesity with and without T2D; first, the difference in the bacterial composition was investigated by the 16S rRNA amplicon sequencing, second, the difference in the metabolites was investigated by targeting short-chain fatty acids (SCFAs) and bile acids (BAs), third, the difference of their community functional potential was assessed by the shotgun metagenomics, and finally we address the alteration in the community function correlated with the development of T2D in Mongolian with obesity.
2 Materials and methods
2.1 Ethics declaration
This study was approved by the Ethics Committees of the Faculty of Agriculture in Kyushu University (Approval No. 107). Ethical clearance was obtained from the Ethics Committee of the Ministry of Health of Mongolia (Approval No. 78). All methods were performed in accordance with the relevant guidelines and regulations. Written informed consent was obtained from all participants, and the samples and data were analyzed, entered, and published anonymously.
2.2 Study design
Participants were Mongolian adults living in Ulaanbaatar (n = 39) and Bulgan (n = 27). Subjects were screened according to the inclusion and exclusion criteria for AMP phase IV study (published in the Supplementary Material of Therdtatha et al., 2021), except that some of the subjects had received antidiabetic therapy in the past 2 months. Supplementary Table S1 presents details about the subjects.
Samples were collected from the 66 participants with obesity (BMI > 30), who were classified into two groups, with T2D (DO: HbA1c ≥ 6.5) or without T2D (NDO: HbA1c < 6.5), based on the National Glycohemoglobin Standardization Program (NGSP) criteria. Table 1 summarizes the demographic and clinical characteristics of each group. There were significant differences in age, sex, and BMI between the DO and NDO groups; these were subsequently treated as non-microbial confounding factors. The NDO group was further divided into a prediabetes group (PDO, HbA1c > 5.7) and a healthy obese group (HO, HbA1c ≤ 5.7) to examine differences in microbiome community structure.
2.3 Sample collection
All subjects provided two fresh stool samples for metagenome and metabolome analyses, in separate tubes (76 × 20 mm, Sarstedt, Munich, Germany), each containing 2 mL RNAlater (Invitrogen, Thermo Fisher Scientific, Waltham, MA) or MeOH, and five YTZ 2.5 mm zirconia balls (Nikkato, Sakai, Japan). For each tube, two spoons of fecal sample were collected from different parts in the middle section of feces and immediately suspended in the solution by shaking the tubes vigorously.
The collected feces were transferred to the laboratory, vortexed to completely suspend the feces, and stored at −80°C in Mongolian University of Life Sciences. The samples were transported to Kyushu University, Japan, while kept below 4°C and were immediately stored at −80°C until DNA or metabolite extraction.
2.4 DNA extraction, 16S rRNA gene amplicon sequencing, and data processing
Total bacterial DNA was extracted from stool samples using the bead-beating method, as previously described (Matsuki et al., 2004). Briefly, DNA was extracted by first vigorously shaking the feces with the zirconium beads; the DNA was then purified by phenol–chloroform extraction followed by isopropanol precipitation (Supplementary Material of Therdtatha et al., 2021). High-throughput 16S rRNA gene sequencing was performed as previously described (Kisuse et al., 2018). Briefly, the V3 to V4 region of the bacterial 16S rRNA gene was amplified via two-step PCR, in which the bacterial universal primer set, Bakt_341F (5′-CGCTCTTCCGATCTCTGCCTACGGGNGGCWGC AG-3′) and Bakt_805R (5′-TGCTCTTCCGATCTGACGACTA CHVGGGTATCTAATCC-3′), was used in the first step; in step two, the same primers plus index-sequences were used. Amplicons with index sequences were subjected to sequencing using the MiSeq Reagent Kit v3 (MS-102-3003; Illumina, San Diego, CA, United States).
The sequence data were processed using QIIME2 pipeline 2021.2 (Bolyen et al., 2019). Briefly, after the total reads were demultiplexed using the index sequences, paired-end sequences were merged and denoised, and primer sequences were removed using DADA2 (Callahan et al., 2016). The forward and reverse primers were eliminated using the 3′ and 5′ end trim parameters “--p-trim-left-f 17” and “--p-trim-left-r 21,” respectively. The parameters “p-trunc-len-f 290” and “p-trunc-len-r 210” were used to trim the forward and reverse reads to lengths of 290 and 210 bases, respectively. The sequence of each amplicon sequence variant (ASV) with 97% sequence identity was taxonomically classified via the QIIME “classify-sklearn” feature using the SILVA 138 database.
2.5 Beta-diversity analysis
Beta diversity was assessed using Bray Curtis, Jaccard, weighted UniFrac, and unweighted UniFrac distances, and permutational multivariate analysis of variance (PERMANOVA) was used to investigate statistical differences between the PDO and HO groups and between the DO and NDO groups.
2.6 Whole shotgun metagenomics
For whole shotgun metagenomic analysis, nine samples were selected from both the DO and NDO groups to be evenly distributed in the weighted and unweighted UniFrac-PCoA ordinations of both groups (Supplementary Figure S1). The DNA extract prepared for the 16S rRNA amplicon sequencing was treated using RNase (NIPPON GENE Co., Ltd., Tokyo, Japan) and was purified using a Gel/PCR extraction kit (NIPPON GENE Co., Ltd., Tokyo, Japan) to remove RNA. DNA concentration was quantified using the Quant-iT PicoGreen dsDNA Assay Kits with a dsDNA standard (Invitrogen, Thermo Fisher Scientific, Waltham, MA). More than 1 μg of DNA in TE buffer (>12.5 ng/μL) was subjected to 150 bp paired-end sequencing on a DNBSEQ system (BGI, Shenzhen, China).
The obtained raw sequences were filtered using the SOAPnuke tool (Chen et al., 2018) to remove (i) reads matching ≥25.0% of the adapter sequences (up to three base mismatches allowed); (ii) short reads (<150 bp); (iii) reads in which undetermined nucleotides account for ≥0.1% of the entire read; and (iv) low-quality reads in which bases with quality <20 accounts for ≥40% of the entire read. Then, the cleaned pair-ended reads were merged by using BBMerge (Bushnell et al., 2017). Human DNA sequences were then removed using the KneadData tool and the database Homo_sapiens_hg37_and_human_contamination_Bowtie2_v0.1. The sequences were further processed using the KneadData tool (version 0.12.0) with the default settings and the human DNA sequences were removed by Bowtie2 (version 2.4.2) on very-sensitive-local mode, phred33 parameter, and the database Homo_sapiens_hg37_and_human_contamination_Bowtie2_v0.1.
These quality-filtered sequences were functionally profiled using the HUMAnN pipeline (v. 3.6.1) using MetaPhlAn 4.0; the mpa_vJan21_CHOCOPhlAnSGB_202103 nucleotide database was used as an intermediate step in the taxonomic classification of each DNA fragment. Each DNA fragment was functionally annotated to UniRef90 gene families, and then categorized into Kyoto Encyclopedia of Genes and Genomes (KEGG) categories at the enzyme, orthology, and pathway levels. Gene abundance in each sample (and similarly in each category) was calculated as reads per kilobase (RPK) and was normalized to obtain the count per million (CPM).
2.7 Quantification of SCFAs and bile acids
SCFAs and BAs in the feces were quantified as previously described (Tanaka et al., 2020; Therdtatha et al., 2021). Briefly, feces collected in MeOH were placed in a SpeedVac concentrator (Thermo Fisher Scientific, Waltham, MA) to evaporate the methanol, and were resuspended in PBS in deuterated water (100 mM, pH 7.4) containing 3-(trimethylsilyl) propionate-2.2.3.3-d4 as an internal standard. The supernatant was used for quantitative 1-H nuclear magnetic resonance (NMR) spectroscopy to quantify acetate, propionate, and butyrate, using a 400 MHz spectrometer (JNM-ECZ400, JEOL, Tokyo, Japan). The SCFA data of each sample was deposited in Supplementary Table S3.
BAs were extracted from the fecal pellet by hot ethanol with an internal standard of nor-deoxycholic acid at 60°C for 30 min and subsequently at 100°C for 3 min. After cleaning using an Oasis HLB cartridge column (WAT094225, Waters, Milford, MA), the extract was subjected to liquid chromatography with a triple quadrupole mass spectrometry system, in which 15 major human fecal BAs were quantified (LCMS-8050, Shimadzu, Japan). These procedures are described in detail by Tanaka et al. (2020). The BA data of each sample was deposited in Supplementary Table S4.
2.8 Statistical analysis
Statistical analyses and data visualization were performed using RStudio 2022.07.2, R 4.2.1 (R Core Team, 2023), Stata/SE 12.0 (Stata Technical Support, 2023), and EZR 4.0.3 (Kanda, 2013).
To evaluate statistical differences between two groups, the Wilcoxon rank-sum test was applied using “wilcox.test” in R. To evaluate statistical differences in qualitative variables between groups, Fisher’s exact test was used in EZR. Spearman’s correlation analysis was performed to calculate correlations between indices, using Stata/SE12. Multiple logistic regression analysis was performed using EZR to assess the risk of each factor to the occurrence of T2D. Linear discriminant analysis of effect size (LEfSe) was performed using the online Galaxy platform (Segata et al., 2011).
2.9 Accessions of sequence data
The raw sequence data obtained was deposited in the DNA Data Bank of Japan (DDBJ) (Fukuda et al., 2021) under BioProject no. PRJDB5860. The Sequence read archives of 16S rRNA gene amplicon sequences (DRA017379) and whole shotgun metagenomic sequence data (DRA017451) were deposited with fecal sampling data in the BioSample database (BioSample, 2023) (accessions SAMD00656652-717 and SAMD00657900-17, respectively).
3 Results
3.1 Differences in the microbial community composition between the DO and NDO groups
The gut microbial community composition was profiled by barcode sequencing of 16S rRNA V3-V4 region. Eventually, 812,093 high quality sequences corresponding to 12,304 ± 2,774 reads (minimum 6,231) per sample were obtained from 17,273 ± 3,757 raw reads per sample and were clustered into 1,722 ASVs; these were assigned to 2 domains, 13 phyla, 19 classes, 40 orders, 72 families, 218 genera, and 502 species. The counts of each ASV for each sample were tabulated in Supplementary Table S2 with taxonomic information for each ASV.
Differences in gut microbial composition were calculated using four distance matrices, and discreteness between the different diabetic status groups was examined using permutation analysis (Table 2). The DO and NDO groups differed significantly, based on the Jaccard, Bray-Curtis, and unweighted UniFrac distances (Supplementary Figure S1 for the ordination plot). When the NDO group was further divided into PDO and HO groups only the Jaccard distance showed a significant difference between DO and PDO, while there were no significant differences between the PDO and HO groups. This indicates that the DO group harbored a significantly different gut microbial community from the NDO group, whereas the community did not differ significantly between the PDO and HO groups. Therefore, the grouping of DO and NDO including PDO and HO subjects was henceforth used in this study.
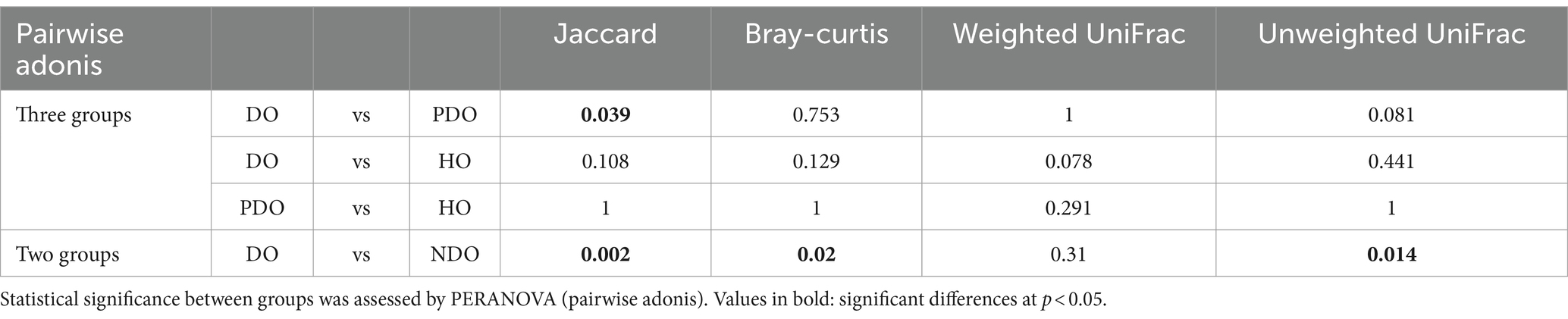
Table 2. Pairwise differences in microbial community composition, based on Jaccard, Bray-Curtis, weighted UniFrac, and unweighted UniFrac distances.
The relative abundances of the 20 most abundant genera are illustrated separately for the DO and NDO groups (Figure 1A). In both groups, the most abundant genus was Prevotella_9, followed by Faecalibacterium and Blautia. Although there was no significant difference in the abundances of the top 20 genera between the DO and NDO groups, some trends were observed, including elevated Faecalibacterium (p = 0.066) levels and lower Holdemanella (p = 0.089) levels in the NDO group. Correlations between the abundance of the most or second most dominant genera (Prevotella_9 and Faecalibacterium, respectively) and BMI or HbA1c were calculated using the Spearman’s rank sum test (Figure 1B); Prevotella_9 was significantly positively correlated with BMI.
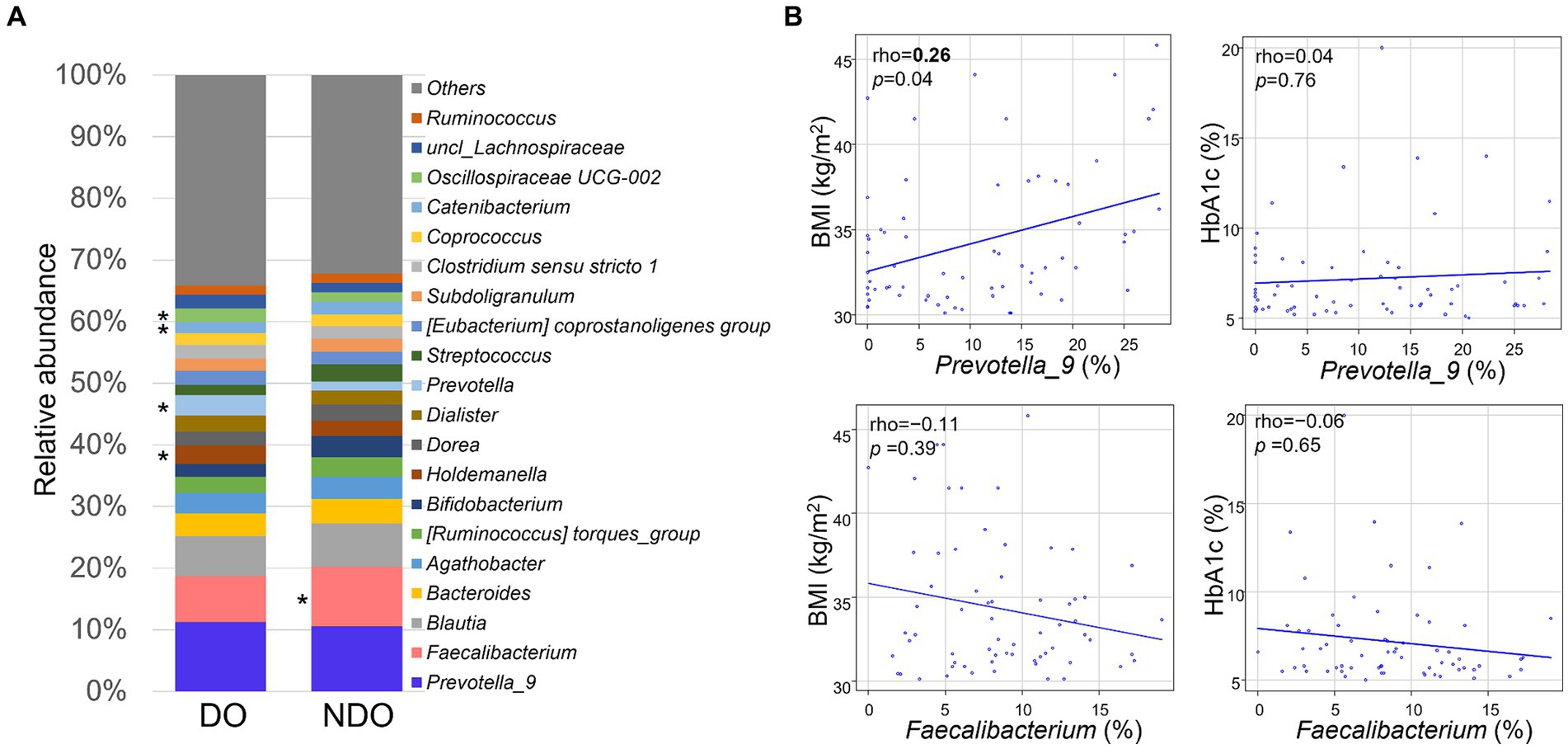
Figure 1. Composition and abundance of the dominant fecal bacterial genera in fecal samples from Mongolian subjects with obesity. (A) Comparison of the top 20 genera in the diabetic obese (DO) and nondiabetic obese (NDO) groups. The abundance of each genus is shown in the bar graph. *, higher values in the relevant group, approaching significance (p < 0.1). (B) Correlations between the abundance of Prevotella_9 or Faecalibacterium and BMI or HbA1c levels. Correlations were analyzed using Spearman’s rank sum test.
LEfSe analysis was performed to examine differences in gut microbiota composition at different taxonomic levels between the DO and NDO groups (Figure 2A): the genera Anaerostipes, Limosilactobacillus and Parasutterella were significantly abundant in the NDO group, whereas in the DO group, a wide variety of taxonomic groups belonging to domain Archaea, six bacterial families, and nine bacterial genera, including Methanobrevibacter, Desulfovibrio, and Solobacterium, were significantly abundant (Figure 2B). Further, correlation analysis (Supplementary Table S5) indicated that Methanobrevibacter and Solobacterium positively correlated with HbA1c.
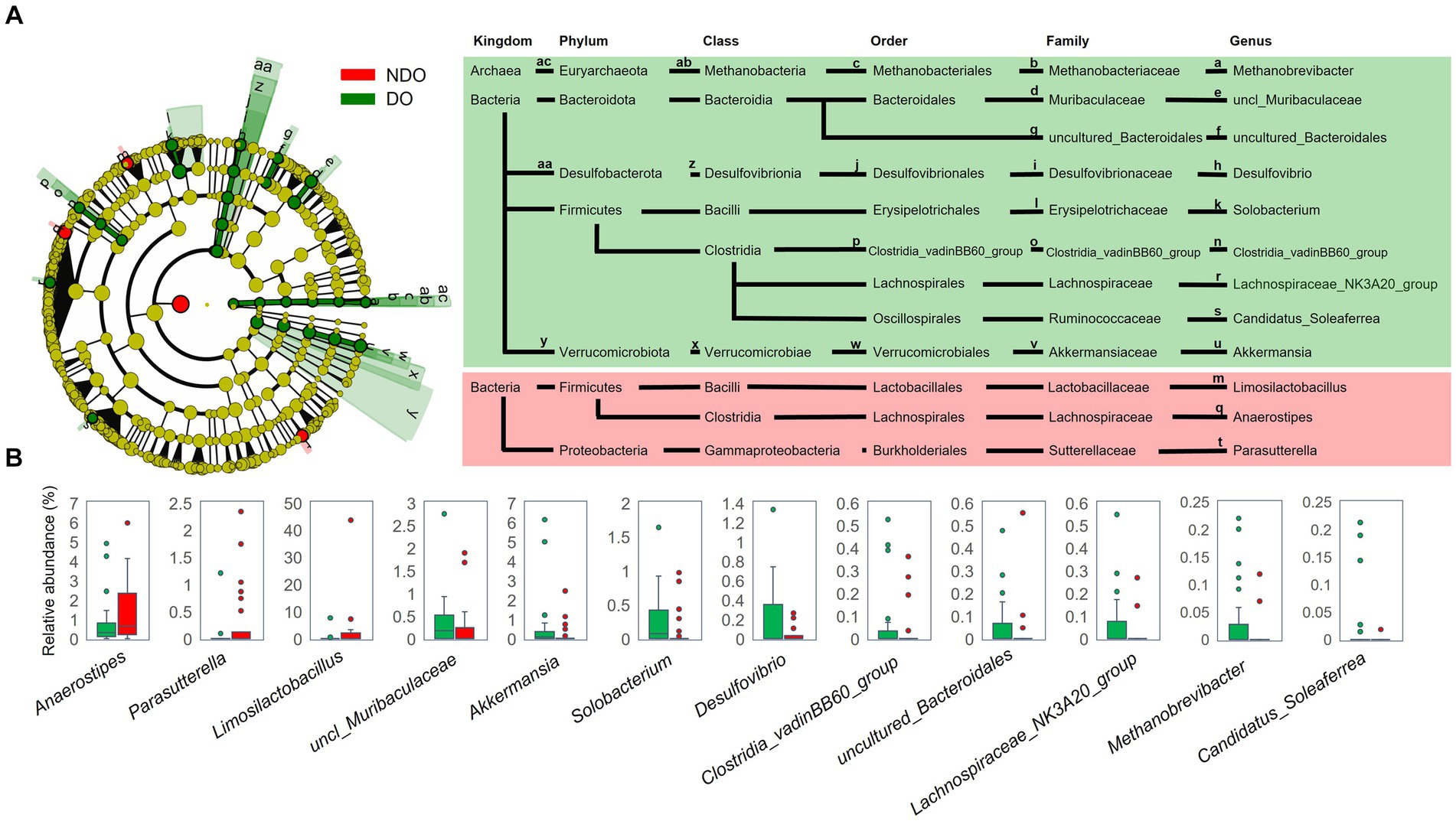
Figure 2. Comparison of fecal bacterial composition between the diabetic obese (DO; green) and nondiabetic obese (NDO; red) groups. (A) Linear discriminant analysis of effect size (LEfSe) analysis to compare the fecal bacterial composition at each taxonomic rank between the DO and NDO groups. The Wilcoxon rank-sum test was used to calculate the linear discriminant analysis (LDA) scores. Taxonomic groups with LDA > 2.0 and p < 0.05 are highlighted by the indicated color on the cladogram. (B) Box plots of the abundance of genera that differed significantly between the DO and NDO groups in the LEfSe analysis.
3.2 Comparison of fecal SCFA levels between the DO and NDO groups
We compared fecal SCFA concentrations between the DO and NDO groups (Figure 3A). The levels of the three major SCFAs–acetate, propionate, and butyrate–tended to be higher in the NDO group, and acetate levels were significantly higher in the NDO group. Figure 3B illustrates the abundance of each SCFA stacked in the bar graph and ordered according to total SCFA concentration, together with a heat map of HbA1c levels (Figure 3B). In most samples, the ratio of acetate, propionate, and butyrate was approximately 6:3:2. The Spearman’s rank sum test revealed an inverse correlation between total SCFA concentration and HbA1c with approached significance (r = −0.23, p = 0.06).
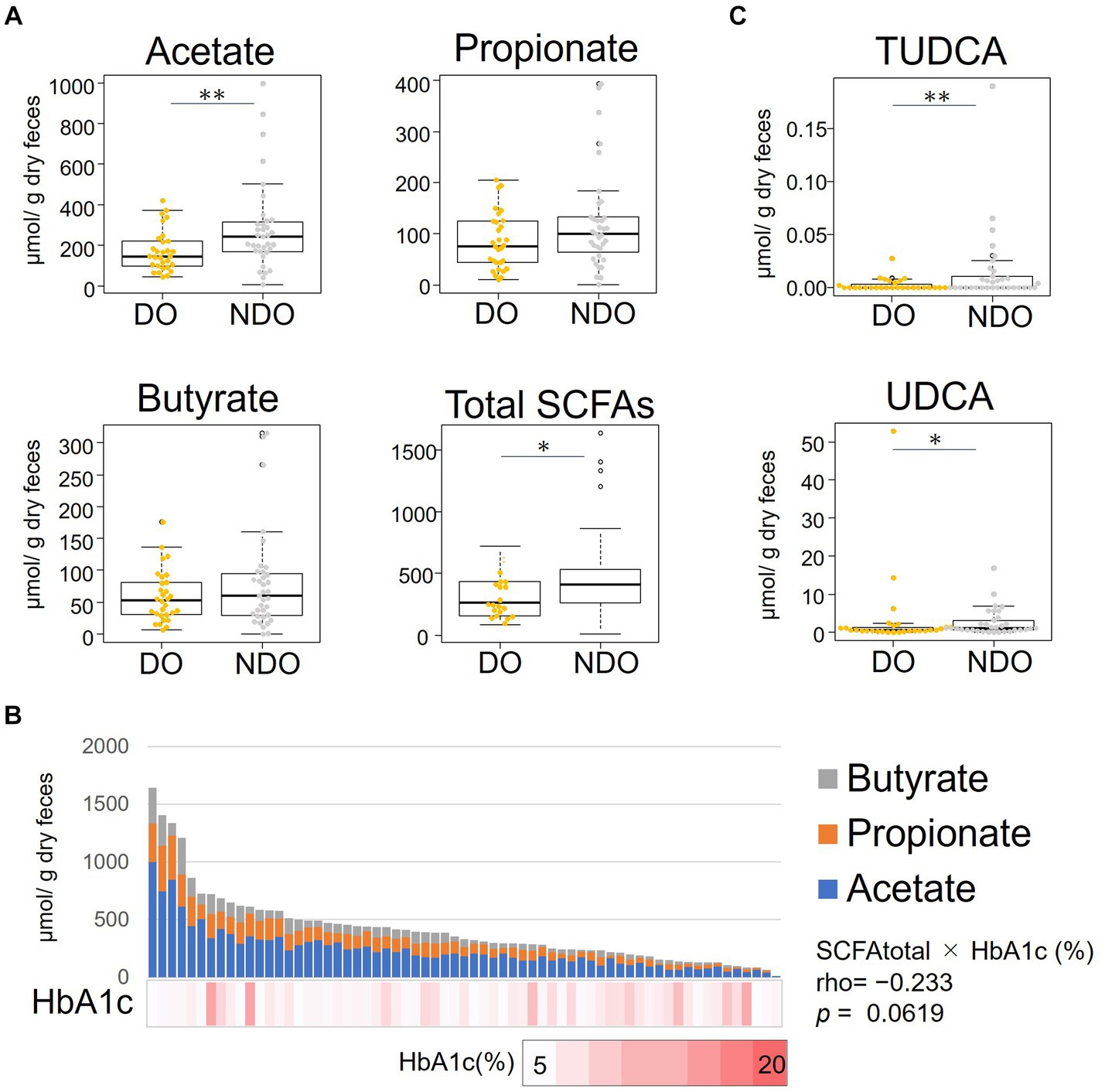
Figure 3. Distribution of the abundances of short-chain fatty acids (SCFAs) and bile acids (BA) in the feces of the diabetic obese (DO) and nondiabetic obese (NDO) groups. (A) Comparison of fecal concentrations of acetate, propionate, butyrate and of total SCFA (sum of acetate, propionate, and butyrate). The box plot illustrates these levels in the DO and NDO groups; statistical significance was evaluated using the Wilcoxon rank-sum test. *, 0.05 < p < 0.1, **, p ≤ 0.05. (B) Stacked bar plot showing the levels of the three SCFAs, order by total abundance, in each of the 66 Mongolian subjects. The HbA1c level of each subject is shown in the heatmap below the bar graph. Correlations between the levels of total SCFA and HbA1c were analyzed using Spearman’s rank sum test. (C) Comparison of fecal concentrations of tauroursodeoxycholic acid and ursodeoxycholic acid. Statistical differences between groups were calculated as in (A). *, 0.05 < p < 0.1, **, p ≤ 0.05.
3.3 Comparison of fecal BA levels between the DO and NDO groups
We measured the concentrations of 15 major BAs in fecal samples (Figure 3C). Ursodeoxycholic acid and its taurine conjugated forms, tauroursodeoxycholic acid (TUDCA), tended to be more abundant in the NDO group. In particular, levels of TUDCA were significantly higher in the NDO group than in the DO group (p = 0.049).
3.4 Comparison of metagenomic profile between the DO and NDO groups
To address differences in the functional potential of the microbial communities in the DO and NDO groups, we performed a whole shotgun metagenomic analysis by using 9 DO and 9 NDO samples. Ultimately, per sample, 24.01 ± 2.48 million high-quality non-human reads, corresponding to 5.60 ± 0.48 Gb sequences, were obtained from 24.30 ± 2.50 million raw reads corresponding to 5.64 ± 0.48 Gb sequences. In the KEGG pathway categories, only the phosphotransferase system (PTS) exhibited significantly differential enrichment (Figures 4A,B). The enrichment of PTS genes in the NDO group can be attributed to the marked enrichment of Anaerostipes hadrus (Figure 4C). Further, genes involved in SCFA biosynthesis tended to be more abundant (although not significantly) in the NDO group, with A. hadrus contributing to this enrichment (Figure 5).
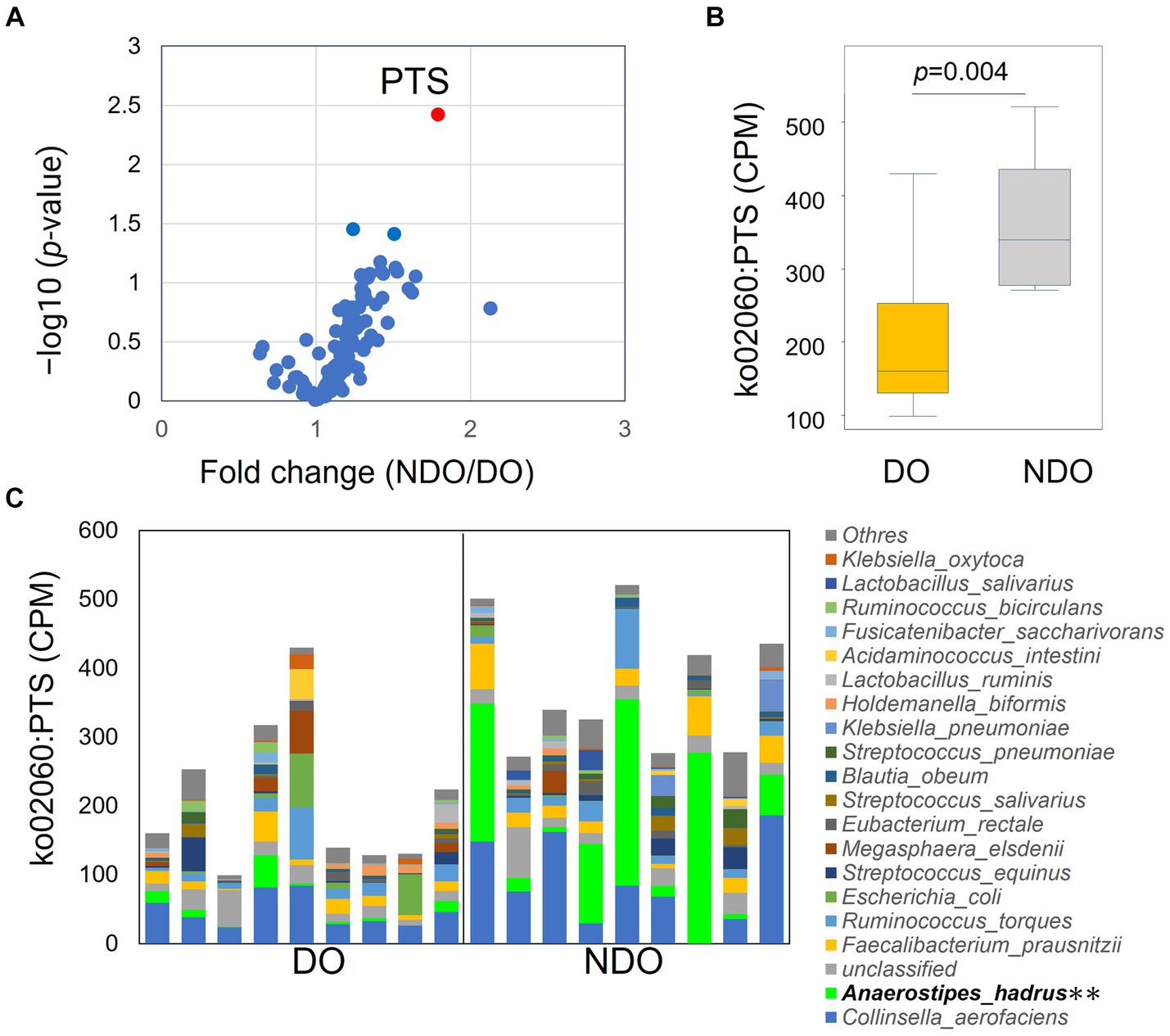
Figure 4. KEGG pathway differential enrichment in the diabetic obese (DO) and nondiabetic obese (NDO) groups. (A) Volcano plot illustrating differences in the abundance of each KEGG pathway between the DO and NDO groups. The vertical axis displays –log10p and the horizontal axis displays the linear fold change (NDO/DO). (B) Box plot of ko02060 (PTS) expression (in count per million, CPM) in the DO and NDO groups. (C) Stacked bar plot of ko02060 (PTS) expression (in count per million, CPM) in each species. **p < 0.05.
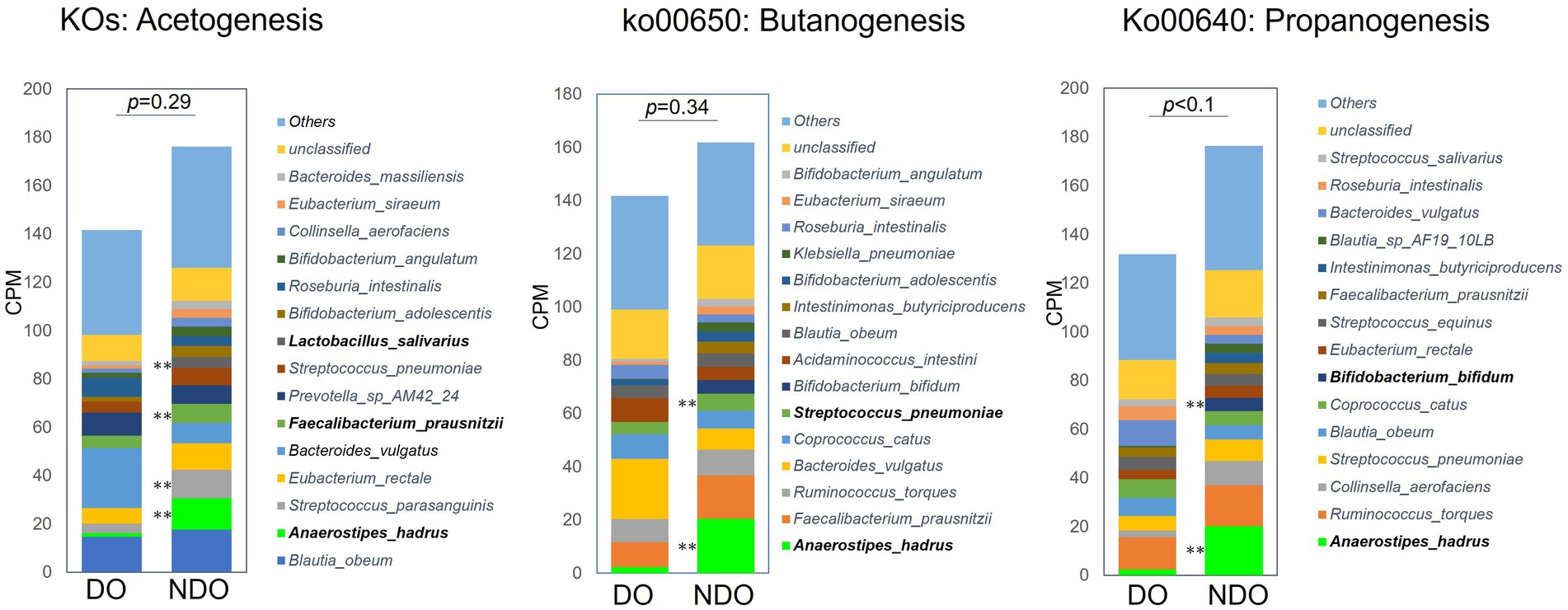
Figure 5. Abundances of KEGG pathway/orthology involved in short-chain fatty acid (SCFA) biosynthesis in the diabetic obese (DO) and nondiabetic obese (NDO) groups. The three stacked bar plots refer, respectively, to the KEGG pathways/orthologies involved in acetogenesis, propanogenesis (ko00640), and butanogenesis (ko00650), and reflect count per million (CPM). For acetogenesis, the counts of KEGG orthologies involved in the Wood–Ljungdahl pathway (17 KOs) and of the K00158 (pyruvate oxidase) and K01512 (acyl phosphatase) KOs, were summed. **p < 0.05 comparing the DO and NDO groups.
A series of genes encoding enzymes involved in acetogenesis, pyruvate oxidase (EC 1.2.3.3), phosphate acetyltransferase (EC 2.3.1.8), and acylphosphatase (EC 3.6.1.7), were higher in the NDO group than in the DO group (Figures 6A,B), although nonsignificantly. Figure 6B illustrates the composition of the bacteria encoding the enzymes (based on KEGG database) in each group. The enrichment of pyruvate oxidase (EC 1.2.3.3), which metabolizes acetyl phosphate from pyruvate, was attributed to the higher abundance of oral lactic acid bacteria (Lactobacillus and Streptococcus species) in the NDO group. The higher abundance of A. hadrus was responsible for the enrichment in the NDO group of phosphate acetyltransferase (EC 2.3.1.8) and acylphosphatase (EC 3.6.1.7) which consecutively metabolize acetyl-CoA to acetate.
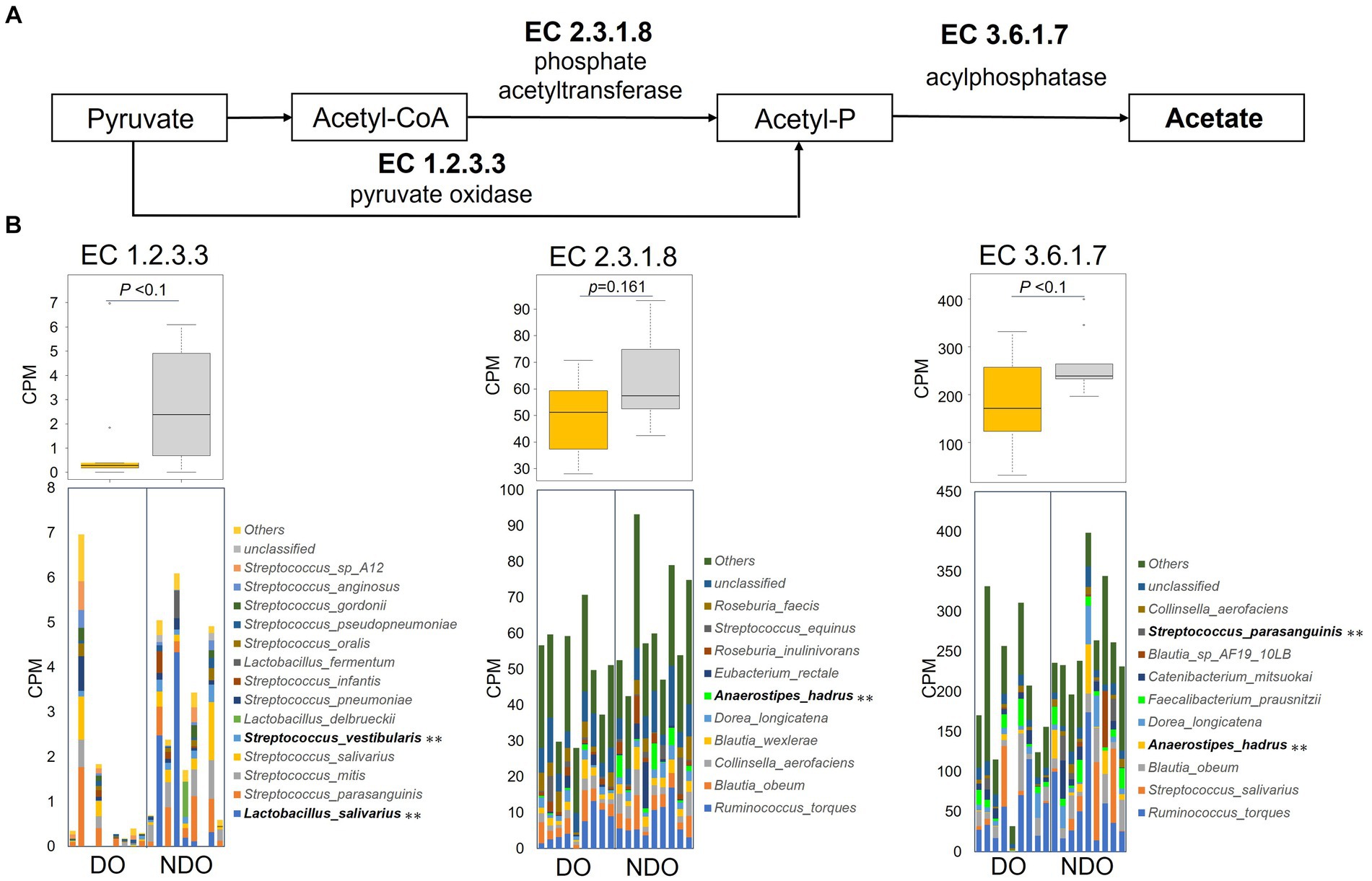
Figure 6. Abundances of KEGG enzymes involved in acetogenesis in the diabetic obese (DO) and nondiabetic obese (NDO) groups. (A) KEGG enzyme map for the acetogenesis pathway from pyruvate to acetate. (B) Box plot showing the counts of each acetogenesis KEGG enzyme (EC1.2.3.3, pyruvate oxidase; EC2.3.1.8, phosphate acetyltransferase; and EC3.6.1.7, acylphosphatase), and stacked bar plots showing the counts of KEGG enzymes per species per subject. **p < 0.05 comparing the DO and NDO groups.
3.5 Multiple logistic regression of factors associated with the occurrence of T2D in obesity
To examine the effect of fecal SCFA levels and host demographic factors on the occurrence of T2D in obesity in this population, we performed multiple logistic regression analyses. Model 1A and Model 1B were obtained with all sets of possible host demographic confounding factors and the fecal concentration of either acetate or total SCFA (acetate + propionate + butyrate), respectively, as microbial factors (Table 3). This model predicted the occurrence of T2D with statistical significance; acetate and total SCFA contributed the most respectively, and age and BMI exhibited significant correlations, whereas sampling region, sex, and metformin intake did not. Regarding sampling region, we further investigated the differences in the levels of acetate and total SCFA in Ulaanbaatar and Bulgan, separately, and confirmed their higher levels in both regions, although not statistically significant (Supplementary Figure S2). Regarding metformin, it is known that metformin intake increases the level of SCFA (Mueller et al., 2021). However, we observed an opposite trend that the acetate and total SCFA levels decreased in the metformin-prescribed subjects in the DO group (DO-Met (+)) (Supplementary Figure S3). The DO-Met (+) group showed significantly higher BMI, HbA1c and fasting blood glucose levels than the non-metformin-prescribed subjects in the DO group (DO-Met (−)). The fact that the DO-Met (+) group had significantly more sever diabetic symptom appears to cause the decrease of the SCFA level rather than the direct effect by metformin.
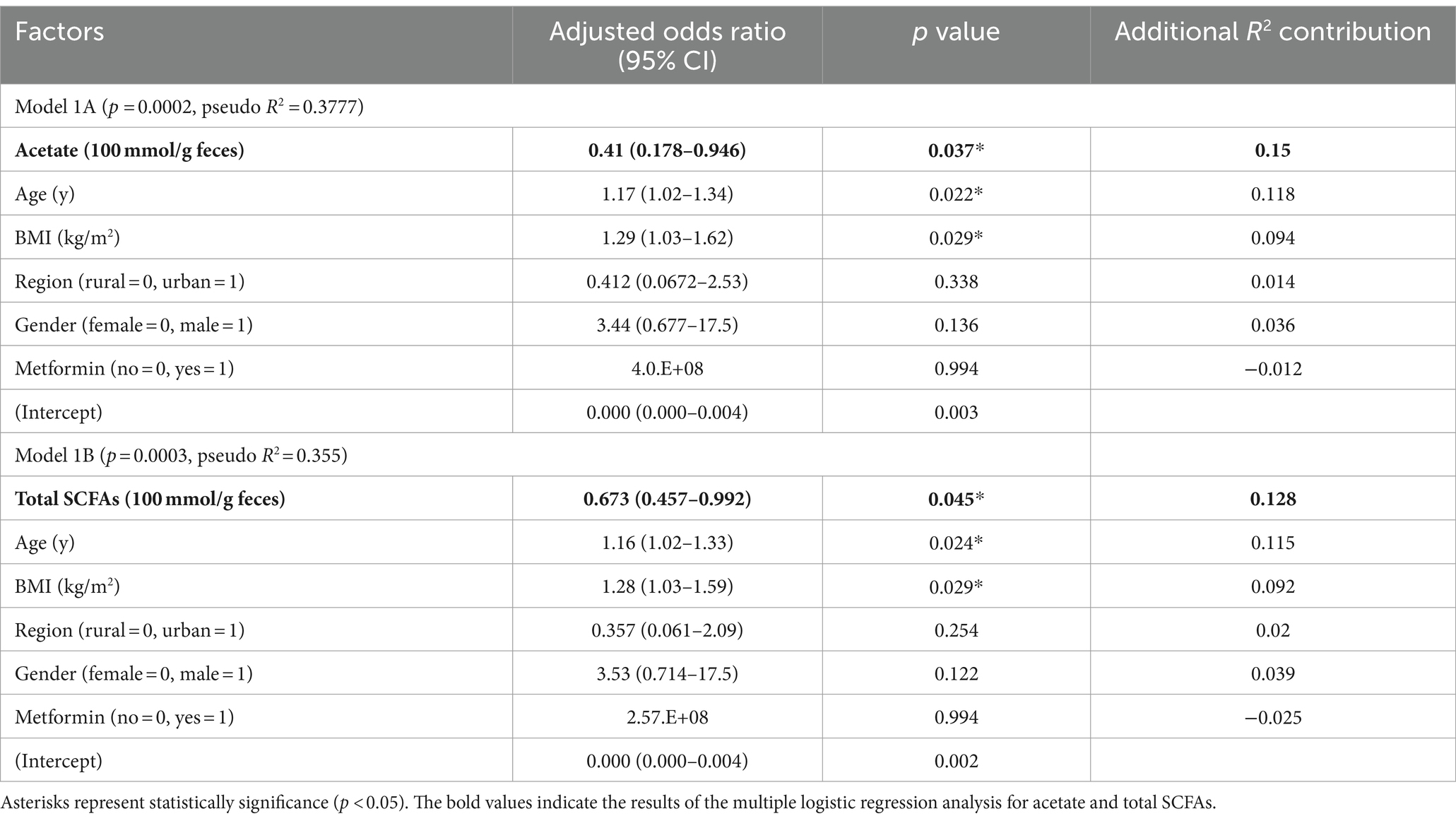
Table 3. Model 1 of multiple logistic regression analysis of factors potentially associated with the occurrence of T2D in obesity in the Mongolian subjects.
Following Model 1, we did the multiple logistic regression analysis excluding these insignificant valuables, resulting in Model 2 with enhanced significance and reasonably high prediction strength (p < 0.0001 and pseudo R2 = 0.3285 for the model including acetate and p < 0.0001 and pseudo R2 = 0.3091 for the model including total SCFA). As shown in Figure 7, the odds ratios of acetate and total SCFA were −0.42 and −0.65 per 100 μmol/g feces, respectively. By using this model, we further examined the odds ratios of the abundances of the certain bacteria genera and TUDCA. Prevotella_9, Faecalibacterium, Anaerostipes, and TUDCA showed the tendency of the negative risk associations for the occurrence of T2D, although nonsignificantly.
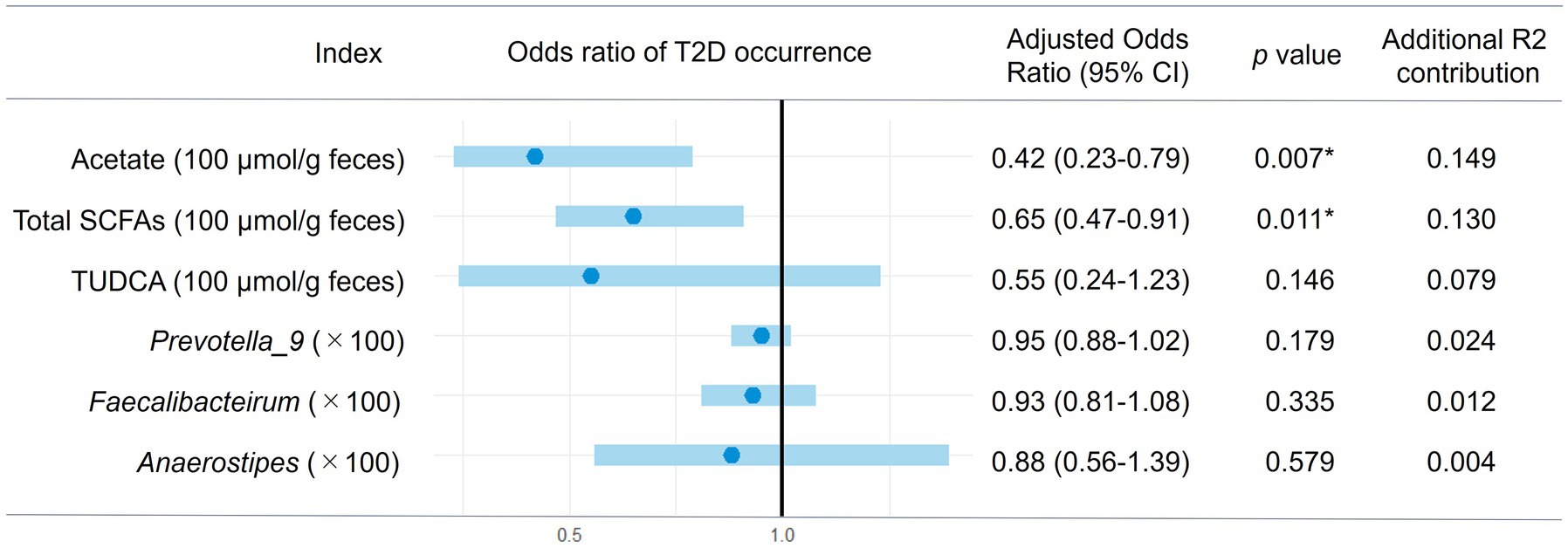
Figure 7. Multiple logistic regression of gut microbial factors for the occurrence of T2D in obesity. The multiple logistic regression analysis of each gut microbial factor was performed with age and BMI (Model 2) and the estimated 95% confidence interval for odds ratio of each factor are shown in the forest plot. Asterisks represent statistically significance (p < 0.05).
4 Discussion
The main aim of this study was to address the gap of the lower-than-expected frequency of diabetes in obesity in the Mongolian population in terms of intestinal microbiology. First of all, we overviewed the difference in the fecal microbiome structure between the DO and NDO subjects and also between PDO and HO subjects to clarify how the intestinal microbial community differ by the level of diabetes. Community structure differed between the DO and NDO groups, but not between the PDO and HO groups. Various studies have reported significant alterations in the gut microbiota of patients with prediabetes (Letchumanan et al., 2022; Rathi et al., 2023). Our current analysis may have missed small-scale alterations unique to the PDO group, owing to the small sample size. However, the community composition of the NDO group including PDO subjects was clearly different from that of the DO group (Table 2). These differences might be involved in the prevention of T2D development in obesity in Mongolia and were further analyzed.
Prevotella_9 was the most dominant genus in both DO and NDO groups. Although Prevotella_9 did not differ in abundance between the DO and NDO groups, it showed positive correlation with BMI (Figure 1B) and also showed marginally lower risk associations with the occurrence of T2D in the multiple logistic regression analysis (Figure 7). This is consistent with prior findings showing a link between obesity and the abundance of Prevotella, which exhibits substantial potential to produce energy via high levels of sugar fermentation activity (Precup and Vodnar, 2019). Prevotella is also known to exert an antidiabetic function (Kovatcheva-Datchary et al., 2015). Therefore, high levels of Prevotella may be involved in the low frequency of T2D in obesity in the Mongolian population.
The second dominant genus, Faecalibacterium, was less abundant in the DO group with approached significance (Figure 1A). Lower Faecalibacterium abundance is commonly observed in individuals with diabetes (Gurung et al., 2020). A reduction in Faecalibacterium prausnitzii abundance was observed even at the prediabetic stage (Wu et al., 2020). Since Faecalibacterium prausnitzii is recognized as beneficial, notably as a butyrate producer, its reduction represents dysbiosis and this might be the case in the DO group.
Dysbiotic trend in the DO subjects was also observed in the increase of subdominant genera such as Methanobrevibacter, Desulfovibrio, and Solobacterium (Figure 2). Methanobrevibacter is known to induce inflammation by activating dendritic cells to produce inflammatory cytokines (Bang et al., 2014) and its increase has been observed in patients with inflammatory bowel disease (Ghoshal et al., 2016; Ghavami et al., 2018). Desulfovibrio, a sulfate-reducing bacterium enriched in T2D subjects (Wang et al., 2012) and in women with gestational diabetes mellitus (Crusell et al., 2018), is generally associated with poor host health (Goldstein et al., 2003). T2D patients who consume higher amounts of dietary fiber exhibited reduced levels of Desulfovibrio with reduction of HbA1c (Fu et al., 2022). Solobacteirum known as a septicemic infectional bacterium (Detry et al., 2006) is reported to associate with colon cancer (Avuthu and Guda, 2022). These dysbiotic features observed in the DO subjects appear to link with the occurrence of T2D with obesity in Mongolians.
On the other hand, in NDO fecal samples, anti-inflammatory and antidiabetic metabolites, namely TUDCA and SCFAs were found to be elevated (Figure 3). TUDCA acts as an agonist of Takeda G-protein-coupled receptor 5 (TGR5) (Song et al., 2022) and an antagonist of farnesoid X receptor (FXR) (Sun et al., 2018) and thereby shows an anti-inflammatory effect and promotes insulin sensitivity, respectively. The acetate and total SCFA fulfilled statistical significance, even after adjusting for potential confounding variables via multiple logistic regression analysis. The estimated odds ratios of acetate (−0.42 per 100 μmol/g feces) and total SCFA (−0.65 per 100 μmol/g feces) (Figure 7) suggest that increasing their gut levels within the physiological range could have a substantial preventative impact on the development of T2D. The antidiabetic efficacy of SCFAs has been demonstrated in other cohort studies (Zhao et al., 2019, 2020) and in animal model research (Huang et al., 2023). Obesity induces insulin resistance by causing the secretion of proinflammatory cytokines from adipose tissue and via oxidative-stress-induced β-cell dysfunction, resulting in the development of diabetes (Al-Goblan et al., 2014). SCFAs promote the release of glucagon-like peptide 1 (GLP-1) from L cells by activating the epithelial cell receptors FFAR2 (GPR43) and FFAR3 (GPR41), thereby improving insulin sensitivity and secretion (Brown et al., 2003; Kimura et al., 2013; Kaku, 2020). On the other hand, there is an animal study showing that acetate induces metabolic syndrome with hyperphagia through the activation of parasympathetic nervous system (Perry et al., 2016). In our Mongolian subjects, acetate may have these two aspects which promote obesity but protect β-cell dysfunction. Acetate can also be a precursor of acetyl-CoA and subsequently involved in the TCA cycle contribute to glucose synthesis. This kind of gluconeogenesis occurs during fasting conditions and plays a role in thermogenesis, especially under the low-glucose or ketogenic conditions, and is crucially required for avoid of life-threatening hypoglycemia and survival (Gray et al., 2007; Sakakibara et al., 2009). This might be an option equipped in Mongolian who have survived under the nomadic life with severe winter during their long history.
The elevated production of SCFA in the DO group was further demonstrated using whole shotgun metagenomic analysis. Genes for enzymes involved in the SCFA biosynthesis were enriched in the NDO samples, along with the enrichment of PTS genes which participate in carbohydrate uptake (Figure 4) (Kuang et al., 2017; Takeuchi et al., 2023), suggesting higher catabolic potential of gut microbial community in NDO subjects. The metagenomic results identified A. hadrus as the primary source of these SCFA-synthesizing enzymes (Figure 5). Indeed, A. hadrus is known as acetate, propionate, and butyrate producer equipped with high potential for sugar catabolism with high content of genes encoding phosphotransferase system (Low et al., 2023). A lower abundance of Anaerostipes in individuals with diabetes was observed both in the Netherlands (Balvers et al., 2021) and Nigeria (Doumatey et al., 2020). Anaerostipes is associated with improved β-cell function and insulin efficiency (Diener et al., 2021). Taken together, A. hadrus may play a pivotal role in SCFA catabolism in the NDO microbiome community, reducing the risk of T2D in obesity in Mongolians.
In conclusion, this study provided the insight into the role of the gut microbiome in the prevention of T2D associated with obesity in Mongolians. Different microbial community was observed in obese subjects in Mongolia depending on the presence or absence of diabetes. Gut microbial bacteria such as Prevotella, Faecalibacterium, and Anaerostipes, as well as metabolites such as TUDCA, acetate, and total SCFA, were considered to play a foundational role in preventing the onset of diabetes, while certain disease-related bacteria potentially contribute to the onset of diabetes. However, this study has some limitations. As the number of subjects and sampling regions were limited, the generalization of the results of this study is restricted. It should be also noted that there are a number of hidden factors associated with the development of T2D other than the microbiome factors. On the other hand, even after adjusting for potential confounding variables, the elevated levels of acetate and total SCFA could have a substantial preventative impact on the development of T2D; this might be achieved by re-establishing the gut microbiome, with Anaerostipes hadrus as a keystone species.
Data availability statement
The datasets presented in this study can be found in online repositories. The names of the repository/repositories and accession number(s) can be found in the article/Supplementary material.
Ethics statement
The studies involving humans were approved by Ethics Committees of the Faculty of Agriculture in Kyushu University (Approval No. 107) and Committee of the Ministry of Health of Mongolia (Approval No. 78). The studies were conducted in accordance with the local legislation and institutional requirements. The participants provided their written informed consent to participate in this study.
Author contributions
AS: Writing – original draft, Methodology, Investigation, Funding acquisition, Formal analysis. TL: Writing – original draft, Investigation. RM: Writing – original draft, Supervision, Investigation. PT: Supervision, Writing – review & editing. DJ: Writing – original draft, Investigation. CP: Writing – original draft, Investigation. SS: Writing – original draft, Investigation. BC: Writing – original draft, Investigation. BN: Writing – original draft, Investigation. YL: Writing – review & editing, Supervision. SD: Writing – review & editing, Supervision. JN: Writing – review & editing, Supervision, Project administration, Methodology, Funding acquisition, Formal analysis, Conceptualization.
Funding
The author(s) declare that financial support was received for the research, authorship, and/or publication of this article. This work was supported by Grants-in-Aids for Scientific Research from the Japan Society for the Promotion of Science (JSPS KAKENHI grant numbers 22KJ2447 and 20KK0130), the JSPS Core-to-Core program on “Establishment of Gut Microbiome Research Core linking Asian Foods and Health,” and an ANRI fellowship.
Acknowledgments
We thank the members of the Asian Microbiome Project as well as Yuki Morinaga and Masato Shinoda, for their helpful discussions. We would like to express our gratitude for the technical support provided by the Center for Advanced Instrumental and Educational Supports, Faculty of Agriculture, Kyushu University, in operating the MiSeq and LCMS-8050 instruments. We appreciate the technical assistance for the operation of NMR from the Center of Advanced Instrumental Analysis, Kyushu University.
Conflict of interest
The authors declare that the research was conducted in the absence of any commercial or financial relationships that could be construed as a potential conflict of interest.
The author(s) declared that they were an editorial board member of Frontiers, at the time of submission. This had no impact on the peer review process and the final decision.
Publisher’s note
All claims expressed in this article are solely those of the authors and do not necessarily represent those of their affiliated organizations, or those of the publisher, the editors and the reviewers. Any product that may be evaluated in this article, or claim that may be made by its manufacturer, is not guaranteed or endorsed by the publisher.
Supplementary material
The Supplementary material for this article can be found online at: https://www.frontiersin.org/articles/10.3389/fmicb.2024.1355396/full#supplementary-material
Abbreviations
DO, Diabetic obese; HO, Healthy obese; NDO, Nondiabetic obese; PDO, Prediabetic obese.
References
Al-Goblan, A. S., Al-Alfi, M. A., and Khan, M. Z. (2014). Mechanism linking diabetes mellitus and obesity. Diabetes Metab. Syndr. Obes. 7, 587–591. doi: 10.2147/DMSO.S67400
Avuthu, N., and Guda, C. (2022). Meta-analysis of altered gut microbiota reveals microbial and metabolic biomarkers for colorectal Cancer. Microbiol. Spectr. 10:e0001322. doi: 10.1128/spectrum.00013-22
Balvers, M., Deschasaux, M., van den Born, B. J., Zwinderman, K., Nieuwdorp, M., and Levin, E. (2021). Analyzing type 2 diabetes associations with the gut microbiome in individuals from two ethnic backgrounds living in the same geographic area. Nutrients 13:3289. doi: 10.3390/nu13093289
Bang, C., Weidenbach, K., Gutsmann, T., Heine, H., and Schmitz, R. A. (2014). The intestinal archaea Methanosphaera stadtmanae and Methanobrevibacter smithii activate human dendritic cells. PLoS One 9:e99411. doi: 10.1371/journal.pone.0099411
BioSample (2023). Available at: https://www.ddbj.nig.ac.jp/biosample/index.html (Accessed December 9, 2023).
Bolyen, E., Rideout, J. R., Dillon, M. R., Bokulich, N. A., Abnet, C. C., Al-Ghalith, G. A., et al. (2019). Reproducible, interactive, scalable and extensible microbiome data science using QIIME 2. Nat. Biotechnol. 37, 852–857. doi: 10.1038/s41587-019-0209-9
Bromage, S., Daria, T., Lander, R. L., Tsolmon, S., Houghton, L. A., Tserennadmid, E., et al. (2020). Diet and nutrition status of Mongolian adults. Nutrients 12:1514. doi: 10.3390/nu12051514
Brown, A. J., Goldsworthy, S. M., Barnes, A. A., Eilert, M. M., Tcheang, L., Daniels, D., et al. (2003). The orphan G protein-coupled receptors GPR41 and GPR43 are activated by propionate and other short chain carboxylic acids. J. Biol. Chem. 278, 11312–11319. doi: 10.1074/jbc.M211609200
Bushnell, B., Rood, J., and Singer, E. (2017). BBMerge–accurate paired shotgun read merging via overlap. PLoS One 12:e0185056. doi: 10.1371/journal.pone.0185056
Callahan, B. J., McMurdie, P. J., Rosen, M. J., Han, A. W., Johnson, A. J. A., and Holmes, S. P. (2016). DADA2: high-resolution sample inference from Illumina amplicon data. Nat. Methods 13, 581–583. doi: 10.1038/nmeth.3869
Chan, J. C. N., Malik, V., Jia, W., Kadowaki, T., Yajnik, C. S., Yoon, K.-H., et al. (2009). Diabetes in Asia epidemiology, risk factors, and pathophysiology. Available at: https://jamanetwork.com/.
Chen, Y., Chen, Y., Shi, C., Huang, Z., Zhang, Y., Li, S., et al. (2018). SOAPnuke: a MapReduce acceleration-supported software for integrated quality control and preprocessing of high-throughput sequencing data. Gigascience 7, 1–6. doi: 10.1093/gigascience/gix120
Crusell, M. K. W., Hansen, T. H., Nielsen, T., Allin, K. H., Rühlemann, M. C., Damm, P., et al. (2018). Gestational diabetes is associated with change in the gut microbiota composition in third trimester of pregnancy and postpartum. Microbiome 6:89. doi: 10.1186/s40168-018-0472-x
Delgermaa, D., Yamaguchi, M., Nomura, M., and Nishi, N. (2023). Assessment of Mongolian dietary intake for planetary and human health. PLOS Glob. Public Health 3:e0001229. doi: 10.1371/journal.pgph.0001229
Detry, G., Pierard, D., Vandoorslaer, K., Wauters, G., Avesani, V., and Glupczynski, Y. (2006). Septicemia due to Solobacterium moorei in a patient with multiple myeloma. Anaerobe 12, 160–162. doi: 10.1016/j.anaerobe.2006.04.002
Diener, C., de Lourdes Reyes-Escogido, M., Jimenez-Ceja, L. M., Matus, M., Gomez-Navarro, C. M., Chu, N. D., et al. (2021). Progressive shifts in the gut microbiome reflect prediabetes and diabetes development in a treatment-naive Mexican cohort. Front. Endocrinol. (Lausanne) 11:602326. doi: 10.3389/fendo.2020.602326
Doumatey, A. P., Adeyemo, A., Zhou, J., Lei, L., Adebamowo, S. N., Adebamowo, C., et al. (2020). Gut microbiome profiles are associated with type 2 diabetes in urban Africans. Front. Cell. Infect. Microbiol. 10:63. doi: 10.3389/fcimb.2020.00063
Fu, J., Xu, K., Ni, X., Li, X., Zhu, X., and Xu, W. (2022). Habitual dietary Fiber intake, fecal microbiota, and hemoglobin A1c level in Chinese patients with type 2 diabetes. Nutrients 14:1003. doi: 10.3390/nu14051003
Fukuda, A., Kodama, Y., Mashima, J., Fujisawa, T., and Ogasawara, O. (2021). DDBJ update: streamlining submission and access of human data. Nucleic Acids Res. 49, D71–D75. doi: 10.1093/nar/gkaa982
Ghavami, S. B., Rostami, E., Sephay, A. A., Shahrokh, S., Balaii, H., Aghdaei, H. A., et al. (2018). Alterations of the human gut Methanobrevibacter smithii as a biomarker for inflammatory bowel diseases. Microb. Pathog. 117, 285–289. doi: 10.1016/j.micpath.2018.01.029
Ghoshal, U., Shukla, R., Srivastava, D., and Ghoshal, U. C. (2016). Irritable bowel syndrome, particularly the constipation-predominant form, involves an increase in Methanobrevibacter smithii, which is associated with higher methane production. Gut Liver 10, 932–938. doi: 10.5009/gnl15588
Goldstein, E. J. C., Citron, D. M., Peraino, V. A., and Cross, S. A. (2003). Desulfovibrio desulfuricans bacteremia and review of human Desulfovibrio infections. J. Clin. Microbiol. 41, 2752–2754. doi: 10.1128/JCM.41.6.2752-2754.2003
Gray, S., Wang, B., Orihuela, Y., Hong, E. G., Fisch, S., Haldar, S., et al. (2007). Regulation of gluconeogenesis by Krüppel-like factor 15. Cell Metab. 5, 305–312. doi: 10.1016/j.cmet.2007.03.002
Gurung, M., Li, Z., You, H., Rodrigues, R., Jump, D. B., Morgun, A., et al. (2020). Role of gut microbiota in type 2 diabetes pathophysiology. EBioMedicine 51:102590. doi: 10.1016/j.ebiom.2019.11.051
Hu, F. B. (2011). Globalization of diabetes: the role of diet, lifestyle, and genes. Diabetes Care 34, 1249–1257. doi: 10.2337/dc11-0442
Huang, Y., Wang, Z., Ye, B., Ma, J. H., Ji, S., Sheng, W., et al. (2023). Sodium butyrate ameliorates diabetic retinopathy in mice via the regulation of gut microbiota and related short-chain fatty acids. J. Transl. Med. 21:451. doi: 10.1186/s12967-023-04259-4
Kaku, K. (2020). New concept of the glucagon-like peptide-1 signaling pathway on pancreatic insulin secretion. J. Diab. Invest. 11, 265–267. doi: 10.1111/jdi.13136
Kanda, Y. (2013). Investigation of the freely available easy-to-use software “EZR” for medical statistics. Bone Marrow Transplant. 48, 452–458. doi: 10.1038/bmt.2012.244
Kimura, I., Ozawa, K., Inoue, D., Imamura, T., Kimura, K., Maeda, T., et al. (2013). The gut microbiota suppresses insulin-mediated fat accumulation via the short-chain fatty acid receptor GPR43. Nat. Commun. 4:1829. doi: 10.1038/ncomms2852
Kisuse, J., La-ongkham, O., Nakphaichit, M., Therdtatha, P., Momoda, R., Tanaka, M., et al. (2018). Urban diets linked to gut microbiome and metabolome alterations in children: A comparative cross-sectional study in Thailand. Front Microbiol. 22:1345. doi: 10.3389/fmicb.2018.01345
Kodama, K., Tojjar, D., Yamada, S., Toda, K., Patel, C. J., and Butte, A. J. (2013). Ethnic differences in the relationship between insulin sensitivity and insulin response: a systematic review and meta-analysis. Diabetes Care 36, 1789–1796. doi: 10.2337/dc12-1235
Kovatcheva-Datchary, P., Nilsson, A., Akrami, R., Lee, Y. S., De Vadder, F., Arora, T., et al. (2015). Dietary Fiber-induced improvement in glucose metabolism is associated with increased abundance of Prevotella. Cell Metab. 22, 971–982. doi: 10.1016/j.cmet.2015.10.001
Kuang, Y. S., Lu, J. H., Li, S. H., Li, J. H., Yuan, M. Y., He, J. R., et al. (2017). Connections between the human gut microbiome and gestational diabetes mellitus. Gigascience 6, 1–12. doi: 10.1093/gigascience/gix058
Letchumanan, G., Abdullah, N., Marlini, M., Baharom, N., Lawley, B., Omar, M. R., et al. (2022). Gut microbiota composition in prediabetes and newly diagnosed type 2 diabetes: a systematic review of observational studies. Front. Cell. Infect. Microbiol. 12:943427. doi: 10.3389/fcimb.2022.943427
Liu, W., Zhang, J., Wu, C., Cai, S., Huang, W., Chen, J., et al. (2016). Unique features of ethnic Mongolian gut microbiome revealed by metagenomic analysis. Sci. Rep. 6:34826. doi: 10.1038/srep34826
Low, A., Sheludchenko, M., Cheng, H. E., Koh, X. Q., and Lee, J. W. J. (2023). Complete genome sequences of butyrate producing Anaerostipes hadrus strains BA1 and GIF7 isolated from the terminal ileum of a healthy lean male. Microbiol. Resour. Announc. 12:e0070123. doi: 10.1128/MRA.00701-23
Martínez-López, Y. E., Esquivel-Hernández, D. A., Sánchez-Castañeda, J. P., Neri-Rosario, D., Guardado-Mendoza, R., and Resendis-Antonio, O. (2022). Type 2 diabetes, gut microbiome, and systems biology: a novel perspective for a new era. Gut Microbes 14:2111952. doi: 10.1080/19490976.2022.2111952
Mathis, B. J., Tanaka, K., and Hiramatsu, Y. (2022). Factors of obesity and metabolically healthy obesity in Asia. Medicina (Lithuania) 58:1271. doi: 10.3390/medicina58091271
Matsuki, T., Watanabe, K., Fujimoto, J., Takada, T., and Tanaka, R. (2004). Use of 16S rRNA gene-targeted group-specific primers for real-time PCR analysis of predominant bacteria in human feces. Appl. Environ. Microbiol. 70, 7220–7228. doi: 10.1128/AEM.70.12.7220-7228.2004
Mishra, S. P., Wang, B., Jain, S., Ding, J., Rejeski, J., Furdui, C. M., et al. (2023). A mechanism by which gut microbiota elevates permeability and inflammation in obese/diabetic mice and human gut. Gut 72, 1848–1865. doi: 10.1136/gutjnl-2022-327365
Mueller, N. T., Differding, M. K., Zhang, M., Maruthur, N. M., Juraschek, S. P., Miller, E. R., et al. (2021). Metformin affects gut microbiome composition and function and circulating short-chain fatty acids: a randomized trial. Diabetes Care 44, 1462–1471. doi: 10.2337/dc20-2257
NCD Risk Factor Collaboration (2020). NCD risk factor collaboration. Available at: www.ncdrisc.org (Accessed November 17, 2023).
Perry, R. J., Peng, L., Barry, N. A., Cline, G. W., Zhang, D., Cardone, R. L., et al. (2016). Acetate mediates a microbiome-brain-β-cell axis to promote metabolic syndrome. Nature 534, 213–217. doi: 10.1038/nature18309
Pinart, M., Dötsch, A., Schlicht, K., Laudes, M., Bouwman, J., Forslund, S. K., et al. (2022). Gut microbiome composition in obese and non-obese persons: a systematic review and meta-analysis. Nutrients 14:12. doi: 10.3390/nu14010012
Portincasa, P., Bonfrate, L., Vacca, M., De Angelis, M., Farella, I., Lanza, E., et al. (2022). Gut microbiota and short chain fatty acids: implications in glucose homeostasis. Int. J. Mol. Sci. 23:1105. doi: 10.3390/ijms23031105
Precup, G., and Vodnar, D. C. (2019). Gut Prevotella as a possible biomarker of diet and its eubiotic versus dysbiotic roles: a comprehensive literature review. Br. J. Nutr. 122, 131–140. doi: 10.1017/S0007114519000680
R Core Team (2023). The R project for statistical computing. Available at: https://www.r-project.org/ (Accessed November 17, 2023).
Ramachandran, A., Chamukuttan, S., Shetty, S. A., Arun, N., and Susairaj, P. (2012). Obesity in Asia - is it different from rest of the world. Diabetes Metab. Res. Rev. 28, 47–51. doi: 10.1002/dmrr.2353
Rathi, K. K., Kumari, N., Javaid, M. D., Saleem, U., Mortensen, E., Zhou, Y., et al. (2023). Gut microbiome and prediabetes - a review. Front. Bacteriol. 2:1242297. doi: 10.3389/fbrio.2023.1242297
Sakakibara, I., Fujino, T., Ishii, M., Tanaka, T., Shimosawa, T., Miura, S., et al. (2009). Fasting-induced hypothermia and reduced energy production in mice lacking acetyl-CoA Synthetase 2. Cell Metab. 9, 191–202. doi: 10.1016/j.cmet.2008.12.008
Scheithauer, T. P. M., Rampanelli, E., Nieuwdorp, M., Vallance, B. A., Verchere, C. B., van Raalte, D. H., et al. (2020). Gut microbiota as a trigger for metabolic inflammation in obesity and type 2 diabetes. Front. Immunol. 11:571731. doi: 10.3389/fimmu.2020.571731
Segata, N., Izard, J., Waldron, L., Gevers, D., Miropolsky, L., Garrett, W. S., et al. (2011). Metagenomic biomarker discovery and explanation. Genome Biol. 12:R60. doi: 10.1186/gb-2011-12-6-r60
Shinoda, A., Shirchin, D., Jamiyan, D., Lkhagvajav, T., Purevdorj, C., Sonomtseren, S., et al. (2021). Comparative study of gut microbiota Mongolian and Asian people. Mongolian J. Agricult. Sci. 33, 1–7. doi: 10.5564/mjas.v33i2.1744
Song, M., Zhang, F., Fu, Y., Yi, X., Feng, S., Liu, Z., et al. (2022). Tauroursodeoxycholic acid (TUDCA) improves intestinal barrier function associated with TGR5-MLCK pathway and the alteration of serum metabolites and gut bacteria in weaned piglets. J. Anim. Sci. Biotechnol. 13:73. doi: 10.1186/s40104-022-00713-3
Stata Technical Support (2023). Citing Stata software, documentation, and FAQs. Available at: https://www.stata.com/support/faqs/resources/citing-software-documentation-faqs/ (Accessed November 17, 2023).
Sun, L., Xie, C., Wang, G., Wu, Y., Wu, Q., Wang, X., et al. (2018). Gut microbiota and intestinal FXR mediate the clinical benefits of metformin. Nat. Med. 24, 1919–1929. doi: 10.1038/s41591-018-0222-4
Takeuchi, T., Kubota, T., Nakanishi, Y., Tsugawa, H., Suda, W., Kwon, A. T. J., et al. (2023). Gut microbial carbohydrate metabolism contributes to insulin resistance. Nature 621, 389–395. doi: 10.1038/s41586-023-06466-x
Tanaka, M., Sanefuji, M., Morokuma, S., Yoden, M., Momoda, R., Sonomoto, K., et al. (2020). The association between gut microbiota development and maturation of intestinal bile acid metabolism in the first 3 y of healthy Japanese infants. Gut Microbes 11, 205–216. doi: 10.1080/19490976.2019.1650997
Therdtatha, P., Song, Y., Tanaka, M., Mariyatun, M., Almunifah, M., Manurung, N. E. P., et al. (2021). Gut microbiome of indonesian adults associated with obesity and type 2 diabetes: a cross-sectional study in an asian city, Yogyakarta. Microorganisms 9:897. doi: 10.3390/microorganisms9050897
Torii, Y., Shimizu, K., and Takenaga, F. (2012). Antioxidant activities of Mongolian medical plants: Pentaphylloides fruticosa as a traditional source of natural antioxidants. Food Preservat. Sci. 38, 25–30. doi: 10.5891/jafps.38.25
Wang, J., Qin, J., Li, Y., Cai, Z., Li, S., Zhu, J., et al. (2012). A metagenome-wide association study of gut microbiota in type 2 diabetes. Nature 490, 55–60. doi: 10.1038/nature11450
Wu, H., Tremaroli, V., Schmidt, C., Lundqvist, A., Olsson, L. M., Krämer, M., et al. (2020). The gut microbiota in prediabetes and diabetes: a population-based Cross-sectional study. Cell Metab. 32, 379–390.e3. doi: 10.1016/j.cmet.2020.06.011
Yang, G., Wei, J., Liu, P., Zhang, Q., Tian, Y., Hou, G., et al. (2021). Role of the gut microbiota in type 2 diabetes and related diseases. Metabolism 117:154712. doi: 10.1016/j.metabol.2021.154712
Zhang, J., Guo, Z., Lim, A. A. Q., Zheng, Y., Koh, E. Y., Ho, D., et al. (2014). Mongolians core gut microbiota and its correlation with seasonal dietary changes. Sci. Rep. 4:5001. doi: 10.1038/srep05001
Zhao, L., Lou, H., Peng, Y., Chen, S., Fan, L., and Li, X. (2020). Elevated levels of circulating short-chain fatty acids and bile acids in type 2 diabetes are linked to gut barrier disruption and disordered gut microbiota. Diabetes Res. Clin. Pract. 169:108418. doi: 10.1016/j.diabres.2020.108418
Zhao, L., Lou, H., Peng, Y., Chen, S., Zhang, Y., and Li, X. (2019). Comprehensive relationships between gut microbiome and faecal metabolome in individuals with type 2 diabetes and its complications. Endocrine 66, 526–537. doi: 10.1007/s12020-019-02103-8
Keywords: gut microbiome, Mongolian, obesity, type 2 diabetes, Anaerostipes hadrus, short-chain fatty acid, whole shotgun metagenomics, tauroursodeoxycholic acid
Citation: Shinoda A, Lkhagvajav T, Mishima R, Therdtatha P, Jamiyan D, Purevdorj C, Sonomtseren S, Chimeddorj B, Namdag B, Lee YK, Demberel S and Nakayama J (2024) Gut microbiome signatures associated with type 2 diabetes in obesity in Mongolia. Front. Microbiol. 15:1355396. doi: 10.3389/fmicb.2024.1355396
Edited by:
Velmurugan Ganesan, KMCH Research Foundation, IndiaReviewed by:
Medha Priyadarshini, University of Illinois Chicago, United StatesGowdham Manivel, Kovai Medical Center and Hospitals (KMCH), India
Copyright © 2024 Shinoda, Lkhagvajav, Mishima, Therdtatha, Jamiyan, Purevdorj, Sonomtseren, Chimeddorj, Namdag, Lee, Demberel and Nakayama. This is an open-access article distributed under the terms of the Creative Commons Attribution License (CC BY). The use, distribution or reproduction in other forums is permitted, provided the original author(s) and the copyright owner(s) are credited and that the original publication in this journal is cited, in accordance with accepted academic practice. No use, distribution or reproduction is permitted which does not comply with these terms.
*Correspondence: Jiro Nakayama, bmFrYXlhbWFAYWdyLmt5dXNodS11LmFjLmpw