- 1Xinjiang Second Medical College, Xinjiang, China
- 2Key Laboratory of Protection and Utilization of Biological Resources in Tarim Basin Co-funded by Xinjiang Production & Construction Corps and The Ministry of Science & Technology, Tarim University, Alar, China
Due to the misuse of antibiotics, there is an increasing emergence and spread of multidrug-resistant (MDR) bacteria, leading to a human health crisis. To address clinical antibiotic resistance and prevent/control pathogenic microorganisms, the development of novel antibiotics is essential. This also offers a new approach to discovering valuable actinobacterial flora capable of producing natural bioactive products. In this study, we employed bioinformatics and macro-genome sequencing to collect 15 soil samples from three different locations in the Karamay Gobi region. First, we assessed the diversity of microorganisms in soil samples from different locations, analyzing the content of bacteria, archaea, actinomycetes, and fungi. The biodiversity of soil samples from outside the Gobi was found to be higher than that of soil samples from within and in the center of the Gobi. Second, through microbial interaction network analysis, we identified actinomycetes as the dominant group in the system. We have identified the top four antibiotic genes, such as Ecol_fabG_TRC, Efac_liaR_DAP, tetA (58), and macB, by CARD. These genes are associated with peptide antibiotics, disinfecting agents and antiseptics, tetracycline antibiotics, and macrolide antibiotics. In addition, we also obtained 40 other antibiotic-related genes through CARD alignment. Through in-depth analysis of desert soil samples, we identified several unstudied microbial species belonging to different families, including Erythrobacteriaceae, Solirubrobacterales, Thermoleophilaceae, Gaiellaceae, Nocardioidaceae, Actinomycetia, Egibacteraceae, and Acidimicrobiales. These species have the capability to produce peptide antibiotics, macrolide antibiotics, and tetracycline antibiotics, as well as disinfectants and preservatives. This study provides valuable theoretical support for future in-depth research.
1 Introduction
The increase in antibiotic resistance in pathogenic bacteria poses a significant and global threat to public health. Reliable reports have shown that antimicrobial resistance (AMR) is associated with 700,000 deaths per year worldwide (Fisher et al., 2022). Antibiotics are widely used in human healthcare, veterinary medicine, and agriculture, leading to their continuous release into the environment (Su and Wen, 2022). As a result, antibiotic resistance genes (ARGs) have been introduced into various settings, including clinical facilities (hospitals and clinics), veterinary facilities, the human gastrointestinal microbiome, wastewater treatment plants, and the natural environment (soil, water, and air) (Hart et al., 2023). ARGs caused by antibiotic resistance are considered a major challenge in modern medicine, making the effective clinical treatment of bacterial infections difficult. Ongoing research by scientists aims to address this issue. In Dr. Su’s research, 36 high-risk ARGs that may threaten public health were identified, along with priority carriers of ARGs such as Escherichia coli, Enterococcus faecalis, and Pseudomonas influenzae type A. Metagenomics and bioinformatics analyses revealed 15 types of ARGs with high abundance, including 381 ARG subtypes, in a drinking water treatment plants (DWTPs) (Gu et al., 2023). Although urban sewage is effectively treated in WWTPs before discharge, combined sewer overflows (CSOs) caused by storms can directly release significant amounts of ARGs into the environment (Jang et al., 2021). The research conducted on the antibiotic resistome in the CSOs has revealed that the flow of antibiotics and host range take place at the allele level, indicating that the study of mobile genetic elements (MGEs) warrants greater attention from scientific researchers (Zhang et al., 2022).
Antibiotics are a class of chemicals that kill or inhibit bacteria. They achieve bactericidal or bacteriostatic effects by interfering with the synthesis of bacterial cell walls, changing the internal metabolism of bacteria, and interfering with the synthesis of bacterial nucleic acids, thus treating various bacterial infections (Corno et al., 2014). However, despite being one of the greatest discoveries of our time, the use of antibiotics to treat bacterial infections also presents some challenges. First, bacterial resistance is gradually increasing, rendering some antibiotics less effective or even ineffective. One possible explanation for this is that sometimes a single resistance allele mechanism can confer resistance to several different antibiotics, including antibiotics of different classes (Allen et al., 2019). Second, non-pathogenic bacteria can transform into drug-resistant strains by acquiring resistance genes. Since the introduction of penicillin antibiotics in the 1940s, antibiotic research has focused on discovering and modifying antibiotic structures, as well as utilizing enhancers and accelerators to aid in fighting bacterial infections. Bacteria produce antimicrobial substances that kill or inhibit competitors accordingly. Previous observations have indicated that natural products (NPs) serve as a significant reservoir of novel antibiotics. In a study conducted by Mr. Wang, it was demonstrated that ρ-aminobenzoic acid encoding BGCs, cloned from soil metagenomes, have played a crucial role in guiding the synthesis of albicidin and cystobactamid analogs (Wang et al., 2021). Another investigation explored the ocean as a potential source for bacterial isolation, aiming to discover new secondary metabolites (Purves et al., 2016). Metagenomics analysis of seawater samples collected from various depths in Antarctica and the Arctic reveals the presence of microorganisms capable of producing primary metabolites. These microorganisms primarily belong to Actinobacteria, Chloroflexi, Proteobacteria, Acidobacteria, and two Candidate phyla, AD3 and WPS-2 (Cao et al., 2020). The microbial bioactive metabolites produced by Actinomycetales account for 45% of the total identified compounds. Among these, the genus streptomyces alone is responsible for 75% of these metabolites (Bérdy, 2005). Consequently, numerous researchers have focused on isolating, identifying, and preserving Actinomyces strains. In our research group, we utilized Gao’s medium to isolate and identify Actinomyces, resulting in the acquisition of dozens of Streptomyces strains. However, one limitation of this method is the high proportion of strains obtained through repeated isolation. Previous studies have demonstrated that metagenomes have emerged as the most effective approach for investigating bacterial communities and their microbial bioactive metabolites. Existing research suggests that the metagenome assembly of DWSS samples can provide insights into the microbial community, antibiotic resistome, and MGEs co-existing with ARGs and ARG hosts (Ke, et al., 2023).
The Karamay Gobi region is a typical extreme environment characterized by a large temperature difference between day and night, high radiation, and low precipitation. Actinomycetes found in this environment exhibit unique characteristics compared to those found in ordinary environments. These distinct actinomycete resources have the potential to be a valuable source for the discovery of new structural antibiotics. The objective of this project is to explore the characteristics of actinomycetes in extreme environments, analyze the distribution of antibiotic genes, and provide theoretical guidance for the future isolation of actinomycetes. First, the dominant flora in the soil was analyzed using metagenome sequencing to identify the prevalent flora in this environment. Then, functional genes and species related to antibiotics were analyzed at the functional level to speculate on the potential presence of secondary actinomycetes. These metabolites serve as a foundation for designing specialized media and targeting the isolation of active substances in the future.
2 Materials and methods
2.1 Soil sample collection
The sampling sites were distributed in the Karamay Gobi region between 84°44′ and 86°1′ E longitude and 44°7′ and 46°8’ N latitude (Figure 1). The impact of the unique desert ecosystem on climate change and biodiversity in this region has always been a focal point for researchers. For sampling, we selected the outer edge, inner edge, and central part of the Karamay Gobi Region, with each sampling site separated by approximately 5 km. The labeling scheme used is as follows: the outer samples (W1–W5) correspond to the gbwb group, the inner samples (N1–N5) correspond to the gbnb group, and the central samples (Z1–Z5) correspond to the gbzx group.
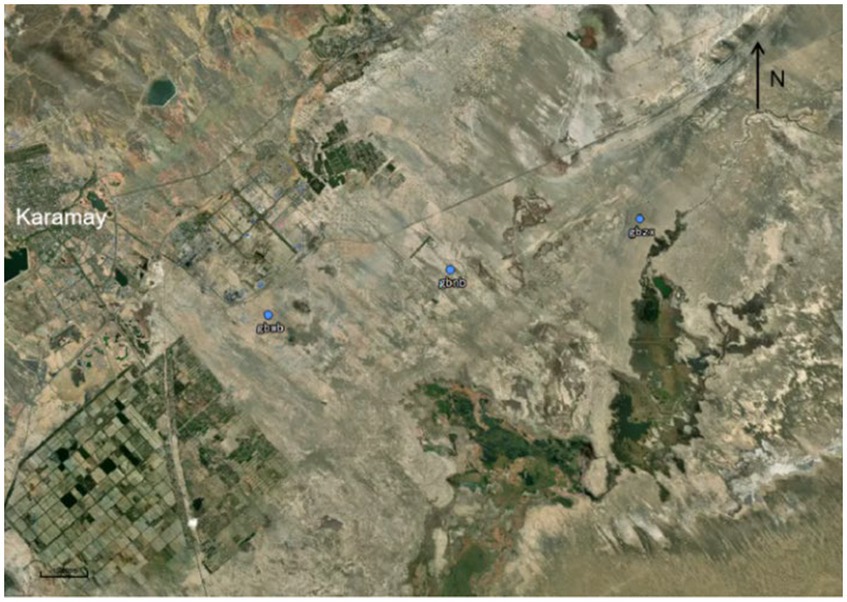
Figure 1. Sampling points were collected in the Karamay Gobi region. The locations of the samples are represented by blue dots and labels. The sampling method used was the ‘five-point sampling method’ (Wang et al., 2020). This method involved determining the central sampling point within a 1-kilometer radius as the midpoint of the diagonal line. Then, four points equidistant from the central sampling point were selected on the diagonal line as additional sampling points. A total of five samples were collected from each location, resulting in a total of 15 sample points (Xiao et al., 2022).
2.2 DNA extraction and Illumina Nova_Seq sequencing
The OMEGA Mag-Bind Soil DNA Kit (M5635-02) (Omega Bio-Tek, Norcross, GA, United States) was used to extract the total microbial genomic DNA from soil samples. A Qubit™ 4 Fluorometer (Qubit™ Assay Tubes: Q32856; Qubit™ 1X dsDNA HS Assay Kit: Q33231) (Invitrogen, United States) and agarose gel electrophoresis were used to measure the quantity and quality of extracted DNA, respectively. Then, the qualified microbial DNA was processed to construct metagenome shotgun sequencing libraries with the Illumina TruSeq Nano DNA LT Library Preparation Kit. Each eligible PE150 library was sequenced with the Illumina NovaSeq platform (Illumina, USA) at Personal Biotechnology Co., Ltd. (Shanghai, China).
2.3 Metagenome analysis
High-quality reads (clean reads) were obtained by removing sequencing adapters from sequencing reads with Cutadapt (v1.2.1) (Martin, 2011) and trimming low-quality reads with fastp (Chen et al., 2018). Then, taxonomical classifications of metagenomics sequencing reads from each sample were performed using Kaiju (1.9.0)1 (Menzel et al., 2016) against a RefSeq-derived database, which included genomes from archaea, bacteria, viruses, fungi, protozoans, metazoans, and viridiplantae. To assemble these high-quality reads into contigs, MEGAHIT (v1.1.2) (Li et al., 2015) was used with the meta-large presetted parameters. The generated contigs (longer than 300 bp) were pooled together and clustered using mmseqs2 (Steinegger and SöDing.,2017) with “easy-linclust” mode, setting the sequence identity threshold to 0.95 and covering residues of the shorter contig to 90%. Compared to diamond, mmseqs2 offers the advantage of a lower error rate (Hernández-Salmerón and Moreno-Hagelsieb, 2020). The non-redundant contigs were obtained by aligning them against the NCBI-nt database by mmseqs2 (Steinegger and SöDing, 2017) in “taxonomy” mode, and contigs assigned to Viridiplantae or metazoa were dropped in the following analysis. The prediction of the genes in the contigs was performed with MetaGeneMark (Zhu et al., 2010). The functionality of the non-redundant genes was annotated by adopting mmseqs2 (Steinegger and SöDing, 2017) with the “search” mode against the CARD (v3.2.4)2 protein databases. To assess the abundances of these genes, the high-quality reads from each sample were mapped onto the predicted gene sequences using salmon (Patro, 2015) in the quasi-mapping-based mode with “--meta --minScoreFraction = 0.55,” and the TPM (Transcripts Per Kilobase per Million mapped reads) was used to normalize abundance values in metagenomes.
3 Results
3.1 Soil characteristics analysis
The results of soil physicochemical property measurements demonstrated notable variations among sampling sites in the Gobi region for all soil physicochemical properties, except for pH. It should be noted that the variability of pH was comparatively lower, as shown in Table 1. In terms of the experimental results, the levels of organic matter, soluble salts, fast-acting potassium, and phosphate were higher in the Gobi region compared to the other two regions. We hypothesized that the soluble salts may contain growth factors that promote the growth of actinomycetes. Therefore, if we want to isolate actinomycetes in this region, it is necessary to add additional soluble salts to the basal culture medium. On the other hand, the total nitrogen and organic carbon species were more abundant in the interior of the Gobi, indicating that the soil in the central part of the Gobi is nutrient-poor.
3.2 Microbial diversity analysis
Microbial alpha diversity indices, including Chao (1984) and Shannon (1948a,b), and ACE, were used to measure species diversity complexity, richness, and sample coverage (Chao and Lee, 1992). As depicted in Figure 2A, significant differences were observed in the ACE and Chao1 indices between the gbnb group and the gbwb and gbzx groups, while a significant difference in the Shannon index was found between the gbwb and gbzx groups. Notably, the Chao1 and ACE indices of the gbzx group were the lowest, indicating a relatively lower actual species number and species richness compared to the other two groups. The gbwb group exhibited the highest Shannon value, while the gbzx group had the lowest, suggesting that the community diversity of the three groups followed the order of gbwb group, gbnb1, and gbzx from high to low. The species distribution in the gbwb group was more uniform (Supplementary Table S1). Principal coordinate analysis using the Bray–Curtis dissimilarity (Bray and Curtis, 1957) also demonstrated significant differences in the microbiota composition among the three groups (analysis of similarities; ADONIS test; R2-statistic = 0.9826, p = 0.001) (Figure 2B).
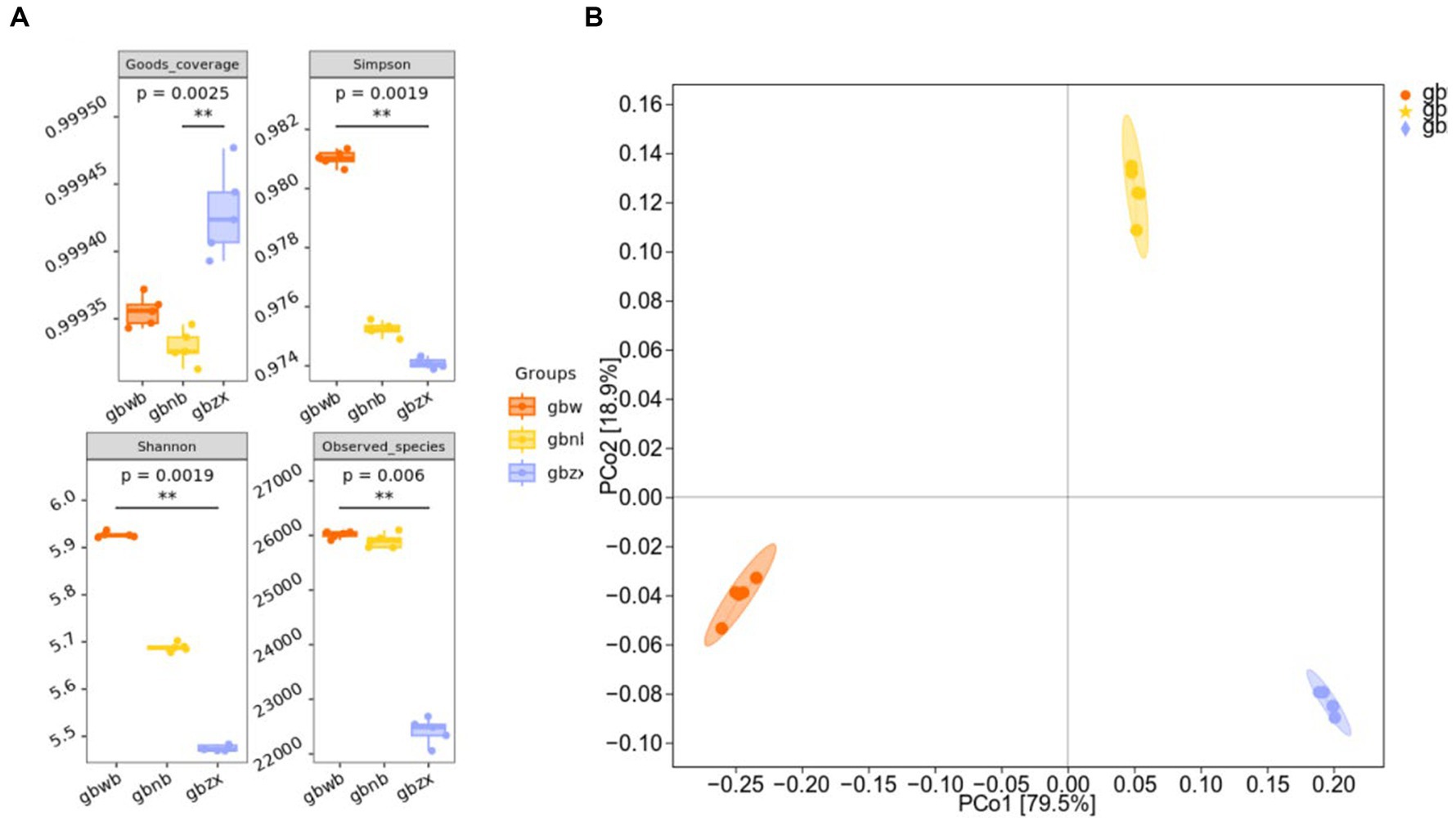
Figure 2. Microbial diversity analysis. (A) Analysis of alpha diversity box plot (Kruskal–Wallis rank-sum test and Dunn’s test were used as post-tests) among groups. (B) Analysis of beta diversity (principal coordinates analysis using the Bray–Curtis dissimilarity) among groups.
3.3 Microbial composition analysis
The dominant bacterial species in all soil samples were SCSIO-52915 sp902806075, CADDYV01 sp902810695, SCSIO-52909 sp902806015, JACDGB01 sp013812245, JACCUC01 sp013812485, SCSIO-52915 sp016781705, WHTG01 sp009377835, SCSIO-52909 sp902805975, SCSIO-52909 sp902806085, SCSIO-52915 sp016781885, WHSW01 sp009379895, SCSIO-52909 sp011492945, JACVSB01 sp014534155, JACVRW01 sp013813135, JACCYG01 sp013696595, SIRX01 sp004563715, SCSIO-52915 sp902806055, JACDBZ01 sp013820865, SCSIO-52909 sp902805985, JACDBZ01 sp013697395, JACVSB01 sp013697275, SCSIO-52915 sp902806065, SCSIO-52909 sp902806095, JACCUC01 sp902806245, JADDRA01 sp016781105, LC5-5 sp016781135, SCSIO-52909 sp902812425, CADCTB01 sp902805665, SCSIO-52909 sp016781725, and JACCUC01 sp013812065 (Figure 3A);
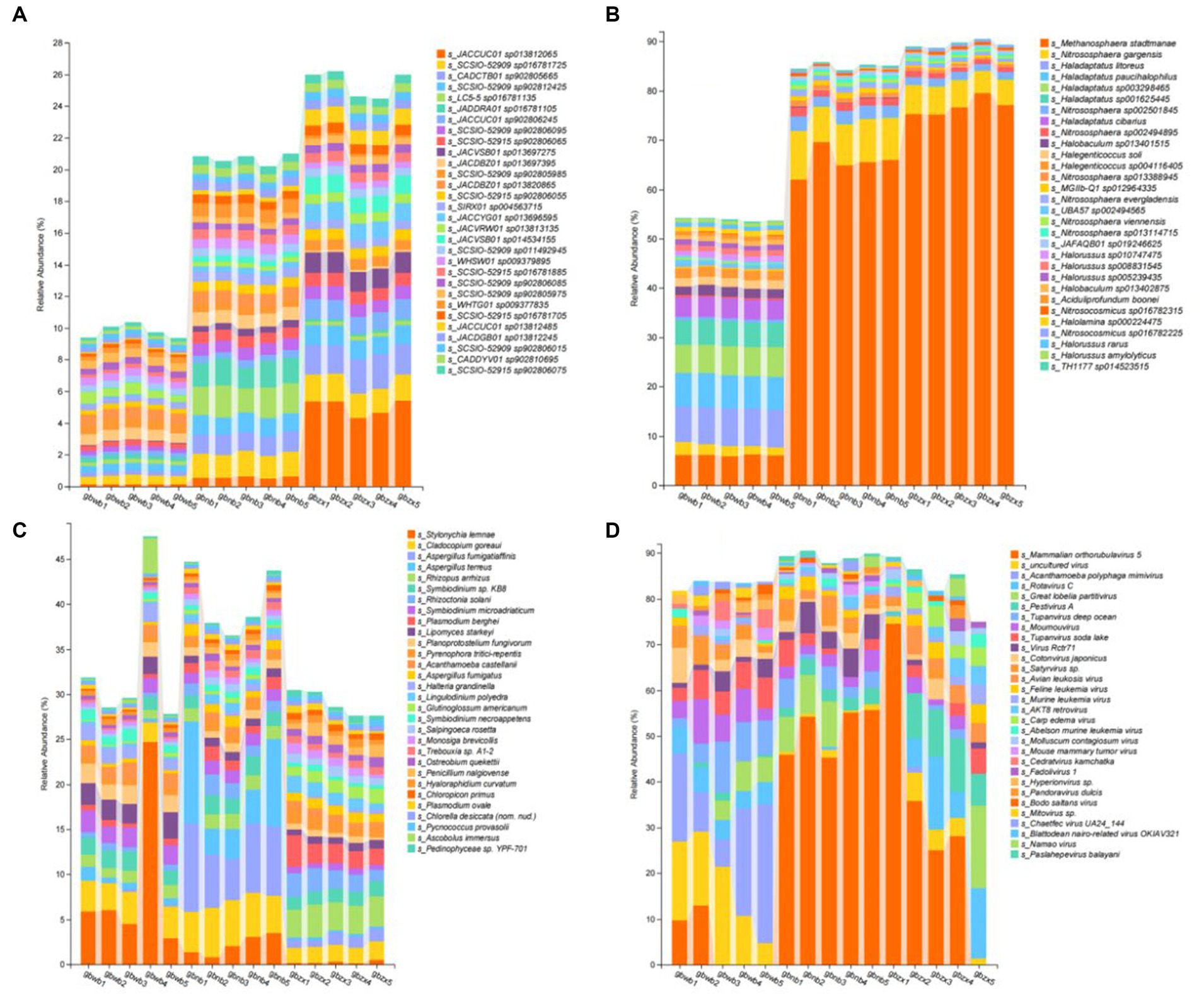
Figure 3. Bar chart reporting the composition of dominant species. (A) The top 30 species in relative abundance at the species level in bacterial communities. (B)The top 30 species in Relative abundance at the species level in archaea communities. (C) The top 30 species in relative abundance at the species level in Eukaryon communities. (D) The top 30 species in relative abundance at the species level in virus communities.
The dominant archaea species in all soil samples were TH1177 sp014523515, Halorussus amylolyticus, Halorussus rarus, Nitrosocosmicus sp016782225, Halolamina sp000224475, Nitrosocosmicus sp016782315, Aciduliprofundum boonei, Halobaculum sp013402875, Halorussus sp005239435, Halorussus sp008831545, Halorussus sp010747475, JAFAQB01 sp019246625, Nitrososphaera sp013114715, Nitrososphaera viennensis, UBA57 sp002494565, Nitrososphaera evergladensis, MGIIb-Q1 sp012964335, Nitrososphaera sp013388945, Halegenticoccus sp004116405, Halegenticoccus soli, Halobaculum sp013401515, Nitrososphaera sp002494895, Haladaptatus cibarius, Nitrososphaera sp002501845, Haladaptatus sp001625445, Haladaptatus sp003298465, Haladaptatus paucihalophilus, Haladaptatus litoreus, Nitrososphaera gargensis, and Methanosphaera stadtmanae (Figure 3B).
The dominant Eukaryon species in all soil samples were Pedinophyceae sp. YPF-701, Ascobolus immersus, Pycnococcus provasolii, Chlorella desiccata (nom. Nud.), Plasmodium ovale, Chloropicon primus, Hyaloraphidium curvatum, Penicillium nalgiovense, Ostreobium quekettii, Trebouxia sp. A1-2, Monosiga brevicollis, Salpingoeca rosetta, Symbiodinium necroappetens, Glutinoglossum americanum, Lingulodinium polyedra, Halteria grandinella, Aspergillus fumigatus, Acanthamoeba castellanii, Pyrenophora tritici-repentis, Planoprotostelium fungivorum, Lipomyces starkeyi, Plasmodium berghei, Symbiodinium microadriaticum, Rhizoctonia solani, Symbiodinium sp. KB8, Rhizopus arrhizus, Aspergillus terreus, Aspergillus fumigatiaffinis, Cladocopium goreaui, and Stylonychia lemnae (Figure 3C).
The dominant virus species in all soil samples were Paslahepevirus balayani, Namao virus, Blattodean nairo-related virus OKIAV321, Chaetfec virus UA24_144, Mitovirus sp., Bodo saltans virus, Pandoravirus dulcis, Hyperionvirus sp., Fadolivirus 1, Cedratvirus kamchatka, Mouse mammary tumor virus, Molluscum contagiosum virus, Abelson murine leukemia virus, Carp edema virus, AKT8 retrovirus, Murine leukemia virus, Feline leukemia virus, Avian leukosis virus, Satyrvirus sp., Cotonvirus japonicus, Virus Rctr71, Tupanvirus soda lake, Moumouvirus, Tupanvirus deep ocean, Pestivirus A, Great lobelia partitivirus, Rotavirus C, Acanthamoeba polyphaga mimivirus, uncultured virus, and Mammalian orthorubulavirus 5 (Figure 3D).
We analyzed the data down to the species level. In the bacterial part, it was observed that JACCUC01 sp013812065 showed significant variation in the samples. The analysis revealed that this bacterium had a high percentage in gbzx, but very little in gbwb and gbnb. However, JADDRA01 sp016781105 and LC5-5 sp016781135 exhibited a high percentage in gbnb, but very little in gbwb and gbzx. The distribution of JACCUC01 sp902806245, JACCUC01 sp902806245, JACVSB01 sp013697275, and JACCYG01 sp013696595 is characterized by gbwb being smaller than gbnb, which in turn is smaller than gbzx. In the archaeal fraction, Methanosphaera stadtmanae is the most prevalent species in the Gobi ecosystem. We observed its presence in all three samples we collected, with a particularly high representation in gbzx. On the other hand, Haladaptatus litoreus, Haladaptatus paucihalophilus, Haladaptatus sp003298465, and Haladaptatus sp001625445, which are archaea that are abundant in gbwb, show a significant decrease in abundance in both gbnb and gbzx samples. In the eukaryotic section, Stylonychia lemna is found in all parts of the Gobi region. The occurrence of gbwb and gbnb is higher than gbzx. However, it is important to note that the data from gbwb-4 samples are abnormal and should not be considered when comparing the characteristics of this species. Additionally, Aspergillus fumigatiaffinis and Aspergillus terreus are two eukaryotes that are primarily found in gbnb and occur less frequently in gbwb and gbzx. During the analysis of this module, we observed significant fluctuations in the virus section. This variability is likely attributed to the fact that viral reproduction relies on parasitizing living cells and exhibits strong host specificity. To conduct a more comprehensive study on viral diversity in the Gobi region, it might be necessary to collect additional replicates of the samples. From the data, we identified several common virus species in each region, including Mammalian orthorubulavirus 5, Pestivirus A, Tupanvirus deep ocean, and Moumouvirus.
3.4 Microbial interaction network analysis
The networking between microbes was conducted to investigate the interaction pattern of microbial communities (Faust and Raes, 2012). Spearman correlation was utilized to calculate correlation values among members of the microbial community, resulting in the creation of a correlation matrix (|R| > 0.6, p < 0.05). The dominant species seed network was then constructed by extracting the top 100 nodes with average abundance using the igraph package in R. Additionally, the generated gML file could be imported into gephi software for visual representation (Bastian et al., 2009). Two network diagrams were constructed for the top 50 actinomycetes species and the top 50 microbial species with high relative abundance (Figures 4A, C). Each of these diagrams consisted of five modules, with module 1 in Figure 4A and module 5 in Figure 4C containing similar species. The largest modules were module 2 in Figure 4A and module 3 in Figure 4C, which contained 24 and 23 nodes, respectively. However, these modules differed in terms of species composition, with 19 species being common to both and 9 species being unique. Therefore, actinomycete species account for a high proportion of the entire microbial community.
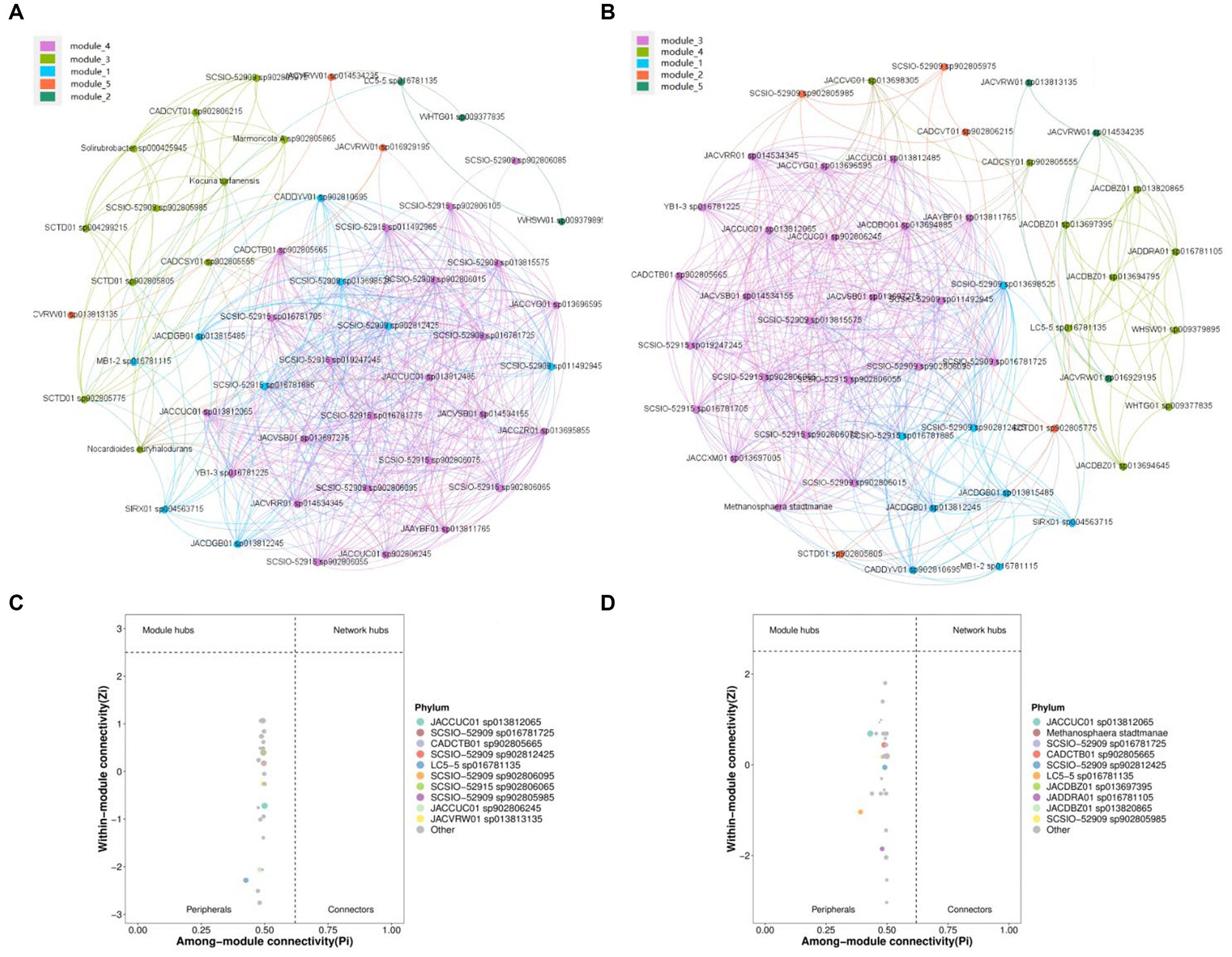
Figure 4. Visualization of the microbial interaction network. (A) Co-occurrence patterns of top 50 actinomycetes species in high relative abundance. (B) Zipi analysis based on (A) network module. (C) Co-occurrence patterns of the top 50 microbial species in high relative abundance. (D) Zipi analysis based on (c) network module.
LC5-5 sp016781135, WHSW01 sp009379895, and WHTG01 sp009377835 are the only modules contained in module 2 of Figure 4A. LC5-5 sp016781135, JADDRA01 sp016781105, JACDBZ01 sp013697395, JACDBZ01 sp013820865, WHSW01 sp009379895, WHTG01 sp009377835, JACDBZ01 sp013694795, JACDBZ01 sp013694645, JACCVC01 sp013698305, and CADCSY01 sp902805555 are the only modules contained in module 4 of Figure 4C added into the network.
Nodes in a network can be divided into four parts using Zi and Pi values, namely peripherals, connectors, module hubs, and network hubs (Karimi et al., 2020). Peripherals represent some of the specialists in the microbial network (Deng et al., 2012). Among them, five species of actinomycetes (MB1-2 sp016781115, JACVRW01 sp014534235, YB1-3 sp016781225, JACVRR01 sp014534345, and JACVRW01 sp016929195) interact with species contained in module 4. It is apparent from Figures 4C,D that all the nodes were identified as peripherals (Zi < 2.5 and Pi <0.62).
3.5 Analysis of the functional contribution of microbial species
The core of the comprehensive antibiotic resistance database (CARD) is the Antibiotic Resistance Ontology (ARO), which is used to associate antibiotic modules with their targets, resistance mechanisms, gene variants, and other information. The gene functions in all samples were annotated and analyzed in the CARD database, resulting in the functional gene abundance table. The relationship between samples and functional genes is illustrated using the Circos diagram (Figures 5A,B). In summary, we found that the functional genes were divided into a total of 44 terms based on the functional level of Drug_Class (Supplementary Table S4). The top 10 terms included peptide antibiotic, macrolide antibiotic, tetracycline antibiotic, penam, disinfecting agents and antiseptics, fluoroquinolone antibiotic, cephalosporin, aminoglycoside antibiotic, carbapenem, and cephamycin (Figure 5A). We found that Ecol_fabG_TRC, Efac_liaR_DAP, tetA (58), macB, Mtub_mshA_INH, oleC, RanA, mlaF, Efae_liaR_DAP, novA, Saur_fusA_FA, and mtrA are the top 12 terms according to the functional level of ARO_Name (Figure 5B).
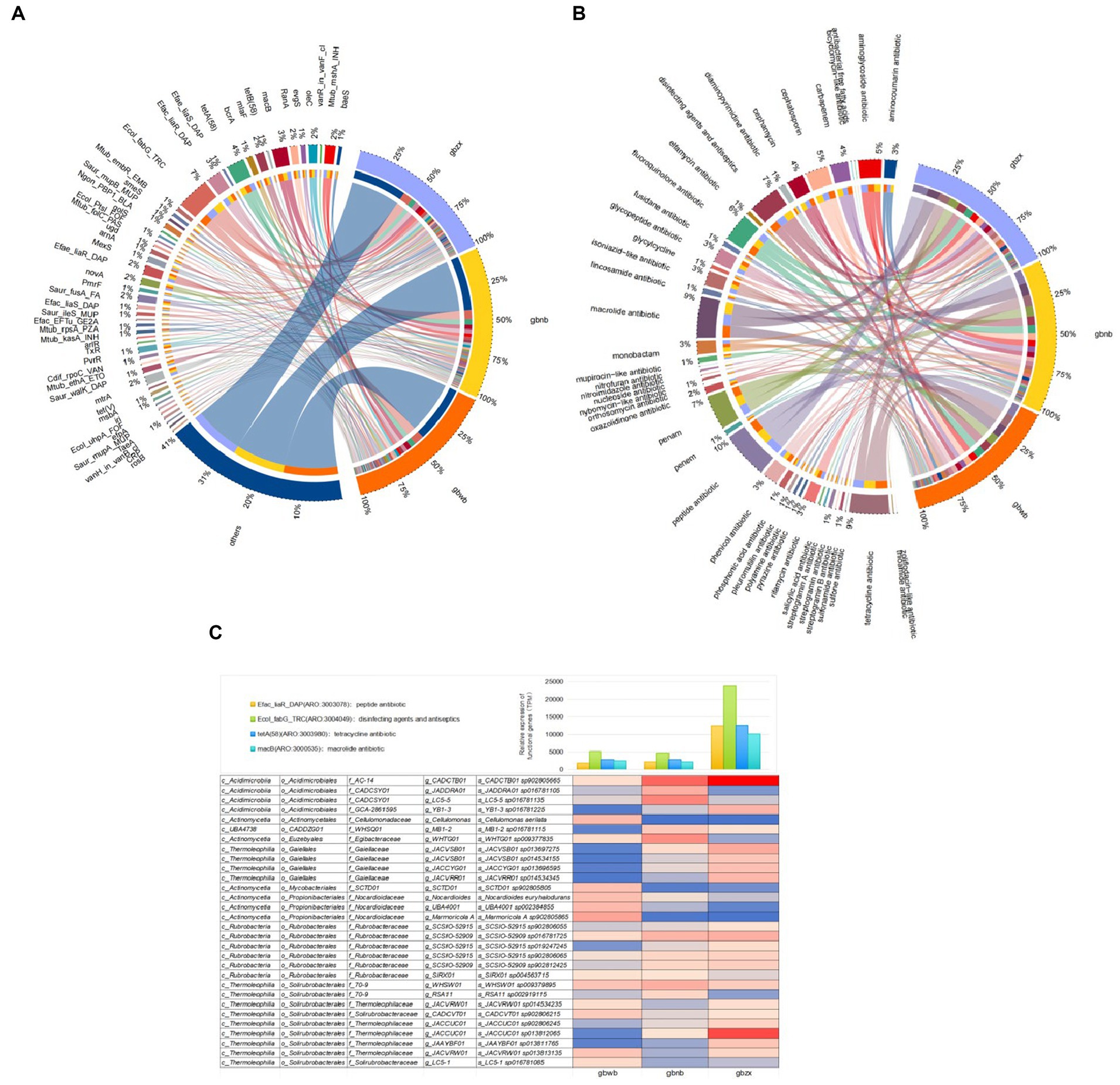
Figure 5. (A) Circos diagram of functional gene terms at the level of Drug_Class. (B) Circos diagram of functional gene terms at the level of ARO_Name. (C) Functional contribution of top 30 microbial species.
The actinomycetes species corresponding to the top four antibiotic-related genes in relative abundance are displayed in Figure 5C. It can be observed that the top 30 species in relative abundance are all associated with Ecol_fabG_TRC, Efac_liaR_DAP, tetA (58), and macB. These species belong to various taxonomic groups, including five families of the order Acidimicrobiales in the class Acidimicrobiia, one genus of the family Egibacteraceae in the order Euzebyales of the class Acidimicrobiia, three genera of the Nocardioidaceae family in the order Propionibacteriales of the class Actinomycetia, one family of the order Mycobacteriales in the class Actinomycetia, four genera of the Gaiellaceae family in the order Gaiellales of the class Thermoleophilia, five genera of the Thermoleophilaceae family in the order Solirubrobacterales of the class Thermoleophilia, two genera of the f_70–9 family in the order Solirubrobacterales of the class Thermoleophilia, and one genus of the Solirubrobacterales family in the order Solirubrobacterales of the class Thermoleophilia. Additionally, it includes six genera of the Rubrobacteraceae family in the order Rubrobacterales of the class Rubrobacteria.
4 Discussion
Alpha and beta diversity analyses were performed to evaluate the variety and abundance of actinomycetes across the three samples. The findings revealed significant variations in the number and diversity of actinomycetes. The exterior of the Gobi exhibited the highest number and diversity, followed by the interior and finally the center (Figure 2A, Supplementary Table S1). Previous literature suggests that actinomycetes primarily exist in soil as spores, requiring nutrients, trace salts, and a neutral pH environment for survival. They can be transported by rainfall, wind, sand, and arthropods. Most actinomycetes are strictly aerobic bacteria (Goodfellow and Williams, 1983). Considering the analysis of soil properties, it is evident that the external soil environment is more conducive to the growth of actinomycetes. Therefore, in studying the diversity of actinomycetes in Gobi soils, it is clear that peripheral soils are more suitable than internal and central soils. In our analysis of CARD data, we observed that the harsh environment in the center of the Gobi desert led to a greater expression of antibiotic genes compared to the periphery and interior regions of the Gobi. Therefore, for the purpose of identifying valuable compounds, we suggest conducting further research on the soil in the central area of the Gobi desert.
Metagenomic studies consistently suggest that there are still many microbial species that need to be thoroughly studied. Out of the top 30 bacteria in terms of relative abundance, 28 belong to the phylum Actinobacteriota (Supplementary Tables S2, S3). This is in good agreement with the previous studies on desert samples (Liu et al., 2018; Leung et al., 2020). Among them, 16 bacteria are from Rubrobacteraceae, Thermoleophilaceae, Gaiellaceae, Egibacteraceae, and Acidimicrobiales. Previous research revealed that members of the two orders Gaiellales and Rubrobacterales were so difficult to culture that it severely restricted their function study (Chen et al., 2018, 2021). Rubrobacter species are a potential source of bioactive compounds with applications such as radiation-resistant, desiccant-resistant, and enzymatic radical scavengers (Albuquerque et al., 2014). The Thermoleophilaceae bacteria can degrade lignin and LDACs (Levy-Booth et al., 2022). Since the first isolation of Egibaceraceae fam nov., there have been no studies describing its function, and it has been identified as a halophilic basophilic bacterium from its natural saline-alkaline habitat (Zhang et al., 2016). The causative products of I. coccineus YM16-304 can be used in pharmaceutical and chemical synthesis fields (Kim, 2019; Koopmeiners et al., 2017). Additionally, numerous functional studies have been conducted on ferrous oxidation and iron reduction (Melany et al., 2022). The high relative abundance of Halobacteriota archaea suggests their adaptability to extreme environments. Among the species with a high relative abundance of archaea, three phyla (Methanobacteriota, Thermoplasmatota, and Thermoproteota) are related to methanogenesis (Supplementary Table S2). Three types of Aspergillus with high abundance were identified in our samples (Supplementary Table S2). Among them, Aspergillus fumigatus is a significant pathogen that causes aspergillosis in humans and animals. Aspergillus terreus, on the other hand, is known for its ability to produce mevinolin and is currently being commercially produced (Alberts et al., 1980). Although Aspergillus fumigatus is closely related to Aspergillus fumigatus (Hong et al., 2005), it exhibits different susceptibility to antifungal drugs such as amphotericin B and azoles when compared to Aspergillus fumigatus (Alastruey-Izquierdo et al., 2014). Algae crust, primarily composed of desert algae, is commonly found in desert regions.
The current study identified several algae species with relatively high abundance, including Chlorella desiccata, Chloropicon primus, Cladocopium goreaui, Lingulodinium polyedra, Ostreobium quekettii, Symbiodinium necroappetens, Symbiodinium microadriaticum, Symbiodinium sp. KB8, Trebouxia sp. A1-2, and Pedinophyceae sp. YPF-701. Penicillium nalgiovense and Rhizopus arrhizus are two kinds of food fermentation fungi with relatively high abundance. The presence of plant pathogenic fungi (Pyrenophora tritici-repentis and Rhizoctonia solani) and human pathogenic protozoa (Plasmodium ovale and Acanthamoeba castellanii) is abundant. Including mimiviruses, giant viruses, marseilleviruses, pandoraviruses, pithoviruses, faustoviruses, and molliviruses have been increasingly detected in humans and amoebae. This challenges the definition and classification of viruses (Colson et al., 2017). In this study, pandoraviruses and Acanthamoeba polyphaga mimivirus were found to be among the top 30 most abundant viruses (Supplementary Table S3). Hepatitis E virus, known as Paslahepevirus balayani, is the main cause of acute viral hepatitis in humans worldwide (Caballero-Gómez et al., 2023). Mammalian orthorubulavirus 5 was detected in healthy civets (He et al., 2022). They may be potential sources of zoonotic pathogens. The results of this study revealed that a high relative abundance of Paslahepevirus balayani and Mammalian orthorubulavirus 5 had a high relative abundance (Supplementary Table S3). Molluscum contagiosum virus is an important human skin pathogen known to cause disfigurement and suffering in children (Chen et al., 2013), and Rotavirus is one of the main pathogens causing infant diarrhea (Gómez-Rial et al., 2020). Molluscum contagiosum virus and Rotavirus were both in high relative abundance in our soil samples (Supplementary Table S2). Additionally, Retroviruses offer promising prospects for gene therapy of human molecular diseases (Twardzik et al., 1982; Frederic Mushinski et al., 1983). Abelson murine leukemia virus was also observed to have a high relative abundance in our samples (Supplementary Table S3).
Our study utilized a metagenomics approach in combination with the CARD database to identify microbial species associated with antibiotics. The results revealed that the top 30 actinomycetes, in terms of relative abundance, also exhibited the highest relative abundance of antibiotic-related genes (refer to Supplementary Tables S3, S4). Peptide antibiotics are active peptides that are widely present in various organisms in nature. They possess diverse activities such as antibacterial, antifungal, antiviral, antiprotozoal, anticancer, and immunomodulatory effects. As a result, they hold potential as a new class of therapeutic drugs alongside traditional antibiotics. Several antimicrobial peptides have already entered the drug development program and have shown promising clinical efficacy with minimal side effects (Lewis, 2020; Upert et al., 2021). Researchers have employed an algorithmic strategy (Torres et al., 2022) to efficiently mine proteomic data, which has opened up new avenues for the discovery of candidate peptide antibiotics. Tetracycline antibiotics, widely used in aquaculture and agriculture, contribute to the development and spread of resistance to such antibiotics (Fang et al., 2020). By modifying the side chain of the linear fusion four-ring scaffold, the second generation of tetracycline antibiotics, represented by doxycycline and minocycline, as well as the third generation, represented by tigecycline, were designed and synthesized (Cui et al., 2022). Apart from their antibacterial effects, tetracyclines have also been explored as cancer therapeutics (Li et al., 2023). Macrolide antibiotics have demonstrated high efficacy in treating respiratory infections. However, they face the challenge of drug resistance. To overcome this, researchers have designed new antibiotics based on the structure of macrolides and their interactions with macromolecular targets (Glanzer et al., 2015; Pichkur et al., 2020). Additionally, researchers have conducted a population-based retrospective cohort study to examine the risk associated with macrolides (Trac et al., 2016). In summary, microbial NPs remain the primary sources for new antibiotic discoveries. More in-depth studies are needed to isolate and culture microbial species and identify antibiotic actives.
5 Conclusion
This study aimed to investigate the diversity of actinomycetes in extreme environments. Our findings indicate a higher prevalence of actinomycetes in the soils of the Gobi region. By analyzing the existing literature and genebanks, we made predictions about the metabolites produced by 30 highly abundant actinomycetes. However, further research is needed on those actinomycetes that are challenging to culture. Despite the difficulties, we are confident that we can identify similar species based on the physiological characteristics of known actinomycetes using high-throughput sequencing technology and bioinformatics. This study has enhanced our understanding of microbial diversity in the Gobi region and has provided new methods and theoretical support for future exploration of actinomycetes discovery and NP isolation.
Data availability statement
The datasets presented in this study can be found in online repositories. The names of the repository/repositories and accession number(s) can be found below: NCBI–SRP467880, BioProject: PRJNA1031080. The data is also available at this link: https://www.ncbi.nlm.nih.gov/sra/PRJNA1031080.
Author contributions
SY: Writing – original draft, Writing – review & editing. WZ: Writing – original draft. BY: Writing – original draft. XF: Writing – original draft. YL: Writing – original draft. XL: Writing – original draft. QL: Writing – original draft, Writing – review & editing.
Funding
The author(s) declare financial support was received for the research, authorship, and/or publication of this article. This research was funded by the Karamay Innovative Environment Construction Plan Project: Innovative Talents (Grant Nos: 2022hjcxrc0059 and 2022hjcxrc0053).
Acknowledgments
The authors are thankful for the financial support from the Karamay Science and Technology Bureau and Xinjiang Second Medical College. The authors are grateful to Chuanxing Wan and Dr.Zhanwen Liu for fruitful discussions related to the project. The authors would also like to thank the Key Laboratory of Tarim Basin Biological Resources Protection and Utilization of Xinjiang Production and Construction Corps and Personal Biotechnology Co., Ltd.
Conflict of interest
The authors declare that the research was conducted in the absence of any commercial or financial relationships that could be construed as a potential conflict of interest.
Publisher’s note
All claims expressed in this article are solely those of the authors and do not necessarily represent those of their affiliated organizations, or those of the publisher, the editors and the reviewers. Any product that may be evaluated in this article, or claim that may be made by its manufacturer, is not guaranteed or endorsed by the publisher.
Supplementary material
The Supplementary material for this article can be found online at: https://www.frontiersin.org/articles/10.3389/fmicb.2024.1330880/full#supplementary-material
Footnotes
1. ^http://kaiju.binf.ku.dk/
References
Alastruey-Izquierdo, A., Alcazar-Fuoli, L., and Cuenca-Estrella, M. (2014). Antifungal susceptibility profile of cryptic species of aspergillus. Mycopathologia 178, 427–433. doi: 10.1007/s11046-014-9775-z
Alberts, A. W., Chen, J., Kuron, G., Hunt, V., Huff, J., Hoffman, C., et al. (1980). Mevinolin: a highly potent competitive inhibitor of hydroxymethylglutaryl-coenzyme a reductase and a cholesterol-lowering agent. Proc. Natl. Acad. Sci. USA 77, 3957–3961. doi: 10.1073/pnas.77.7.3957
Albuquerque, L., Johnson, M. M., Schumann, P., Rainey, F. A., and da Costa, M. S. (2014). Description of two new thermophilic species of the genus Rubrobacter, Rubrobacter calidifluminis sp. nov. and Rubrobacter naiadicus sp. nov., and emended description of the genus Rubrobacter and the species Rubrobacter bracarensis. Syst. Appl. Microbiol. 37, 235–243. doi: 10.1016/j.syapm.2014.03.001
Allen, R. C., Angst, D. C., and Hall, A. R. (2019). Resistance gene carriage predicts growth of natural and clinical Escherichia coli isolates in the absence of antibiotics. Appl. Environ. Microbiol. 85:18. doi: 10.1128/AEM.02111-18
Bastian, M., Heymann, S., and Jacomy, M. (2009). Gephi: An open source software for exploring and manipulating networks. International AAAI Conference on Weblogs and Social Media, No. 3, pp. 361–362.
Bérdy, J. (2005). Bioactive microbial metabolites. Journal of Antibiotics, 58, 1–26. doi: 10.1038/ja.2005.1
Bray, J. R., and Curtis, J. T. (1957). An ordination of the upland forest communities of southern Wisconsin. Ecol. Monogr. 27, 325–349. doi: 10.2307/1942268
Caballero-Gómez, J., Juárez, A. R., Terriza, D. C., Fajardo, T., Buono, F., Jose-Cunilleras, E., et al. (2023). Epidemiological survey and risk factors associated with Paslahepevirus balayani in equines in Europe. One Health 17:100619. doi: 10.1016/j.onehlt.2023.100619
Cao, S., Zhang, W., Ding, W., Wang, M., Fan, S., Yang, B., et al. (2020). Structure and function of the Arctic and Antarctic marine microbiota as revealed by metagenomics. Microbiome 8:9. doi: 10.1186/s40168-020-00826-9
Chao, A. (1984). Nonparametric estimation of the number of classes in a population. Scand. J. Stat. 11, 265–270.
Chao, A., and Lee, S. M. (1992). Estimating the number of classes via sample coverage. J. Am. Stat. Assoc. 87, 210–217. doi: 10.1080/01621459.1992.10475194
Cui, C.-Y. Q. C., He, Q., Chen, C., Zhang, R.-M., Feng, Y., and Sun, J. (2022). Transferability of tigecycline resistance: characterization of the expanding Tet(X) family. WIREs Mech. Dis. 14:e1538. doi: 10.1002/wsbm.1538
Chen, X., Anstey, A. V., and Bugert, J. J. (2013). Molluscum contagiosum virus infection. Lancet Infect. Dis. 13, 877–888. doi: 10.1016/S1473-3099(13)70109-9
Chen, R. W., He, Y., Cui, L. Q., Li, C., and Tian, X. P. (2021). Diversity and distribution of uncultured and cultured Gaiellales and Rubrobacterales in South China Sea sediments. Front. Microbiol. 12:72. doi: 10.3389/fmicb.2021.657072
Chen, R., Wang, K., Wang, F., He, Y., Long, L., and Tian, X. (2018). Rubrobacter indicoceani sp. nov., a new marine actinobacterium isolated from Indian Ocean sediment. Int. J. Syst. Evol. Microbiol. 68, 3487–3493. doi: 10.1099/ijsem.0.003018
Chen, S., Zhou, Y., Chen, Y., and Gu, J. (2018). Fastp: an ultra-fast all-in-one FASTQ preprocessor. Bioinformatics 34, i884–i890. doi: 10.1093/bioinformatics/bty560
Colson, P., Scola, B. L., Levasseur, A., Caetano-Anollés, G., and Raoult, D. (2017). Mimivirus: leading the way in the discovery of giant viruses of amoebae. Nat. Rev. Microbiol. 15, 243–254. doi: 10.1038/nrmicro.2016.197
Corno, G., Coci, M., Giardina, M., Plechuk, S., Campanile, F., and Stefani, S. (2014). Antibiotics promote aggregation within aquatic bacterial communities. Front. Microbiol. 5:297. doi: 10.3389/fmicb.2014.00297
Deng, Y., Jiang, Y. H., Yang, Y., He, Z., Luo, F., and Zhou, J. (2012). Molecular ecological network analyses. BMC Bioinformatics 13:113. doi: 10.1186/1471-2105-13-113
Faust, K., and Raes, J. (2012). Microbial interactions: from networks to models. Nat. Rev. Microbiol. 10, 538–550. doi: 10.1038/nrmicro2832
Fisher, M. C., Alastruey-Izquierdo, A., Berman, J., Bicanic, T., Bignell, E. M., Bowyer, P., et al. (2022). Tackling the emerging threat of antifungal resistance to human health. Nat. Rev. Microbiol. 20, 557–571. doi: 10.1038/s41579-022-00720-1
Frederic Mushinski, J., Potter, M., Bauer, S. R., and Premkumar Reddy, E. (1983). DNA Rearrangement and Altered RNA Expression of the c-mybOncogene In Mouse Plasmacytoid Lymphosarcomas. Science, 220, 795–798. doi: 10.1126/science.6687762
Fang, L.-X., Chen, C., Cui, C.-Y., Li, X.-P., Zhang, Y., Liao, X.-P., et al. (2020). Emerging high-level Tigecycline resistance: novel tetracycline Destructases spread via the Mobile Tet(X). BioEssays 42:e2000014. doi: 10.1002/bies.202000014
Glanzer, S., Pulido, S. A., Tutz, S., Wagner, G. E., Kriechbaum, M., Gubensäk, N., et al. (2015). Structural and functional implications of the interaction between macrolide antibiotics and bile acids. Chemistry – a. Eur. J. 21, 4350–4358. doi: 10.1002/chem.201406413
Gómez-Rial, J., Rivero-Calle, I., Salas, A., and Martinón-Torres, F. (2020). Rotavirus and autoimmunity. J. Infect. 81, 183–189. doi: 10.1016/j.jinf.2020.04.041
Goodfellow, M., and Williams, S. T. (1983). Ecology of Actinomycetes. Ann. Rev. Microbiol. 37, 189–216. doi: 10.1146/annurev.mi.37.100183.001201
Gu, Q., Lin, T., Wei, X., Zhang, Y., Wu, S., Yang, X., et al. (2023). Prevalence of antimicrobial resistance in a full-scale drinking water treatment plant. J. Environ. Manag. 344:118396. doi: 10.1016/j.jenvman.2023.118396
Hart, A., Warren, J., Wilkinson, H., and Schmidt, W. (2023). Environmental surveillance of antimicrobial resistance (AMR), perspectives from a national environmental regulator in 2023. Eur. Commun. Dis. Bull. 28:2200367. doi: 10.2807/1560-7917.ES.2023.28.11.2200367
He, W.-T., Hou, X., Zhao, J., Sun, J., He, H., Si, W., et al. (2022). Virome characterization of game animals in China reveals a spectrum of emerging pathogens. Cell 185, 1117–1129. doi: 10.1016/j.cell.2022.02.014
Hernández-Salmerón, J. E., and Moreno-Hagelsieb, G. (2020). Progress in quickly finding orthologs as reciprocal best hits: comparing blast, last, diamond and MMseqs2. BMC Genomics 21:741. doi: 10.1186/s12864-020-07132-6
Hong, S.-B., Go, S.-J., Shin, H.-D., Frisvad, J. C., and Samson, R. A. (2005). Polyphasic taxonomy of aspergillus fumigatus and related species. Mycologia 97, 1316–1329. doi: 10.3852/mycologia.97.6.1316
Jang, J., Kim, M., Baek, S., Shin, J., Shin, J., Shin, S. G., et al. (2021). Hydrometeorological influence on antibiotic-resistance genes (ARGs) and bacterial Community at a Recreational Beach in Korea. J. Hazard. Mater. 403:123599. doi: 10.1016/j.jhazmat.2020.123599
Karimi, M., Hasanzadeh, A., and Shen, Y. (2020). Network-principled deep generative models for designing drug combinations as graph sets. Bioinformatics 36, i445–i454. doi: 10.1093/bioinformatics/btaa317
Ke, Y. C., Sun, W., Jing, Z., Zhu, Y., Zhao, Z., Xie, S., et al. (2023). Antibiotic resistome alteration along a full-scale drinking water supply system deciphered by metagenome assembly: regulated by seasonality, mobile gene elements and antibiotic resistant gene hosts. Sci. Total Environ. 862:160887. doi: 10.1016/j.scitotenv.2022.160887
Kim, G. H., Jeon, H., Khobragade, T. P., Patil, M. D., Sung, S., Yoon, S., et al. (2019). Enzymatic synthesis of sitagliptin intermediate using a novel ω-transaminase. Enzyme and Microbial Technology. 120:52–60. doi: 10.1016/j.enzmictec.2018.10.003
Koopmeiners, J., Diederich, C., and Jennifer, S. (2017). HheG, a Halohydrin Dehalogenase with Activity on Cyclic Epoxides. ACS Catalysis, 7, 6877–6886. doi: 10.1021/acscatal.7b01854
Leung, P. M., Bay, S. K., Meier, D. V., Chiri, E., Cowan, D. A., Gillor, O., et al. (2020). Energetic basis of microbial growth and persistence in desert ecosystems(article). mSystems 5, e00495–e00419. doi: 10.1128/mSystems.00495-19
Levy-Booth, D. J., Navas, L. E., Fetherolf, M. M., Liu, L.-Y., Dalhuisen, T., Renneckar, S., et al. (2022). Discovery of lignin-transforming bacteria and enzymes in thermophilic environments using stable isotope probing. ISME J. 16, 1944–1956. doi: 10.1038/s41396-022-01241-8
Lewis, K. (2020). The Science of Antibiotic Discovery. Cell, 181, 29–45. doi: 10.1016/j.cell.2020.02.056
Li, D., Liu, C., Luo, R., Sadakane, K., and Lam, T. (2015). MEGAHIT: an ultra-fast single-node solution for large and complex metagenomics assembly via succinct de Bruijn graph. Bioinformatics 31, 1674–1676. doi: 10.1093/bioinformatics/btv033
Li, J., Qin, Y., Zhao, C., Zhang, Z., and Zhou, Z. (2023). Tetracycline antibiotics: potential anticancer drugs. Eur. J. Pharmacol. 956:175949. doi: 10.1016/j.ejphar.2023.175949
Liu, Z., Sun, Y., Zhang, Y., Feng, W., Lai, Z., Fa, K., et al. (2018). Metagenomic and 13C tracing evidence for autotrophic atmospheric carbon absorption in a semiarid desert. Soil Biol. Biochem. 125, 156–166. doi: 10.1016/j.soilbio.2018.07.012
Melany, R-U, Shuai, Weitao, Huang, Shan, and Jaffé, Peter R. (2022). Biodegradation of PFOA in microbial electrolysis cells by Acidimicrobiaceae sp. strain A6. Chemosphere, 292, 133506–133506. doi: 10.1016/j.chemosphere.2021.133506
Martin, M. (2011). Cutadapt removes adapter sequences from high-throughput sequencing reads. EMBnet J. 17:200. doi: 10.14806/ej.17.1.200
Menzel, P., Ng, K. L., and Krogh, A. (2016). Fast and sensitive taxonomic classification for metagenomics with kaiju. Nature. Communications 7:11257. doi: 10.1038/ncomms11257
Patro, R. (2015). Salmon: Accurate, versatile and ultrafast quantification from RNA-seq data using lightweight-alignment. New York: Cold Spring Harbor Labs.
Pichkur, E. B., Paleskava, A., Tereshchenkov, A. G., Kasatsky, P., Komarova, E. S., Shiriaev, D. I., et al. (2020). Insights into the improved macrolide inhibitory activity from the high-resolution cryo-EM structure of dirithromycin bound to the E. coli 70S ribosome. E. coli 70S ribosome. RNA, 26, 715–723. doi: 10.1261/rna.073817.119
Purves, K., Macintyre, L., Brennan, D., Hreggviðsson, G., Kuttner, E., Ásgeirsdóttir, M., et al. (2016). Using molecular networking for microbial secondary metabolite bioprospecting. Meta 6:2. doi: 10.3390/metabo6010002
Shannon, C. E. (1948a). A mathematical theory of communication. Bell Syst. Tech. J. 27, 379–423. doi: 10.1002/j.1538-7305.1948.tb01338.x
Shannon, C. E. (1948b). A mathematical theory of communication. Bell Syst. Tech. J. 27, 623–656. doi: 10.1002/j.1538-7305.1948.tb00917.x
Steinegger, M., and Söding, J. (2017). MMseqs2 enables sensitive protein sequence searching for the analysis of massive data sets. Nat. Biotechnol. 35, 1026–1028. doi: 10.1038/nbt.3988
Su, Z., and Wen, D. (2022). Characterization of antibiotic resistance across Earth's microbial genomes. Sci. Total Environ. 816:151613. doi: 10.1016/j.scitotenv.2021.151613
Torres, M. D., Melo, M. C. R., Crescenzi, O., Notomista, E., and De la Fuente-Nú˜nez, C. (2022). Mining for encrypted peptide antibiotics in the human proteome. Nature. Biomed. Eng. 6, 67–75. doi: 10.1038/s41551-021-00801-1
Trac, M. H., McArthur, E., Jandoc, R., Dixon, S. N., Nash, D. M., Hackam, D. G., et al. (2016). Macrolide antibiotics and the risk of ventricular arrhythmia in older adults. Can. Med. Assoc. J. 188, E120–E129. doi: 10.1503/cmaj.150901
Twardzik, D. R., Todaro, G. J., Marquardt, H., Reynolds, F. H., and Stephenson, J. R. (1982). Transformation induced by Abelson murine leukemia virus involves production of a polypeptide growth factor. Science 216, 894–897. doi: 10.1126/science.6177040
Upert, G., Luther, A., Obrecht, D., and Ermert, P. (2021). Emerging peptide antibiotics with therapeutic potential. Med. Drug Discov. 9:100078. doi: 10.1016/j.medidd.2020.100078
Wang, Z., Kasper, A., Mehmood, R., Ternei, M., Li, S., Freundlich, J. S., et al. (2021). Metagenome-guided analogue synthesis yields improved gram-negative-active Albicidin- and Cystobactamid-type antibiotics. Angew. Chem. 60, 22172–22177. doi: 10.1002/anie.202104874
Wang, S., Zhu, G., Zhuang, L., Li, Y., Liu, L., Lavik, G., et al. (2020). Anaerobic ammonium oxidation is a major N-sink in aquifer systems around the world. ISME J. 14, 151–163. doi: 10.1038/s41396-019-0513-x
Xiao, C., Xu, C., Zhang, J., Jiang, W., Zhang, X., Yang, C., et al. (2022). Soil microbial communities affect the growth and secondary metabolite accumulation in Bletilla striata (Thunb.) Rchb. f. Front. Microbiol. 13:916418. doi: 10.3389/fmicb.2022.916418
Zhang, Y. G., Duan, Y. Q., Xiao, M., and Wen-Jun, L. (2016). Egibacter rhizosphaerae gen. Nov., sp nov., an obligately halophilic, facultatively alkaliphilic actinobacterium and proposal of Egibaceraceae fam. Nov and Egibacterales Ord. nov. Int. J. Syst. Evol. Microbiol. 66, 283–289. doi: 10.1099/ijsem.0.000713
Zhang, J., Yu, D., Dian, L., Hai, Y., Xin, Y., and Wei, Y. (2022). Metagenomics insights into the profiles of antibiotic resistome in combined sewage overflows from reads to metagenome assembly genomes. J. Hazard. Mater. 429:128277. doi: 10.1016/j.jhazmat.2022.128277
Keywords: desert soil, metagenomic sequencing, bioinformatics, actinomycetes, antibiotic
Citation: Yang S, Zhang W, Yang B, Feng X, Li Y, Li X and Liu Q (2024) Metagenomic evidence for antibiotic-associated actinomycetes in the Karamay Gobi region. Front. Microbiol. 15:1330880. doi: 10.3389/fmicb.2024.1330880
Edited by:
LinXing Chen, University of California, Berkeley, United StatesReviewed by:
Daljeet Singh Dhanjal, Lovely Professional University, IndiaInwoo Baek, Korea Atomic Energy Research Institute, Republic of Korea
Zhigang Qiu, Tianjin Institute of Environmental and Operational Medicine, China
Copyright © 2024 Yang, Zhang, Yang, Feng, Li, Li and Liu. This is an open-access article distributed under the terms of the Creative Commons Attribution License (CC BY). The use, distribution or reproduction in other forums is permitted, provided the original author(s) and the copyright owner(s) are credited and that the original publication in this journal is cited, in accordance with accepted academic practice. No use, distribution or reproduction is permitted which does not comply with these terms.
*Correspondence: Xiaolin Li, MzMyMDQ4MTY3QHFxLmNvbQ==; Qin Liu, bGl1cWluMTAyM0AxMjYuY29t
†These authors have contributed equally to this work and share first authorship