- 1Centro de Biotecnologia FEMSA, Escuela de Ingeniería y Ciencias, Tecnológico de Monterrey, Monterrey, Mexico
- 2Centro de Biotecnología Daniel Alkalay, Universidad Técnica Federico Santa María, Valparaíso, Chile
- 3The Novo Nordisk Foundation Center for Biosustainability, Technical University of Denmark, Lyngby, Denmark
- 4Centro de Ciencias Matemáticas, UNAM, Morelia, Mexico
- 5Integrative Biology Unit, The Institute for Obesity Research, Tecnológico de Monterrey, Monterrey, Mexico
The natural products (NPs) biosynthetic gene clusters (BGCs) represent the adapting biochemical toolkit for microorganisms to thrive different microenvironments. Despite their high diversity, particularly at the genomic level, detecting them in a shake-flask is challenging and remains the primary obstacle limiting our access to valuable chemicals. Studying the molecular mechanisms that regulate BGC expression is crucial to design of artificial conditions that derive on their expression. Here, we propose a phylogenetic analysis of regulatory elements linked to biosynthesis gene clusters, to classify BGCs to regulatory mechanisms based on protein domain information. We utilized Hidden Markov Models from the Pfam database to retrieve regulatory elements, such as histidine kinases and transcription factors, from BGCs in the MIBiG database, focusing on actinobacterial strains from three distinct environments: oligotrophic basins, rainforests, and marine environments. Despite the environmental variations, our isolated microorganisms share similar regulatory mechanisms, suggesting the potential to activate new BGCs using activators known to affect previously characterized BGCs.
Key message
Our study represents the first approach that classifies biosynthetic gene clusters according to a regulatory structure. Through the study of known activators of well-characterized BGCs, we have identified common patterns in regulatory mechanisms, offering potential activators for previously unexplored BGCs. This research contributes to NP discovery by presenting a framework for identifying potential activators for novel BGCs, opening the door to the discovery of new Natural Products.
1. Introduction
The exploration of natural products (NPs) from microbial sources has played a key role in the search of pharmaceuticals, insecticides, and herbicides (Katz and Baltz, 2016). However, discovery of novel chemical classes of antimicrobials has dramatically decreased since the discovery of lincosamides in 1963 (González-Zorn and Escudero, 2012; Aminov, 2017). To address this challenge, extensive sequencing efforts have been dedicated to expanding the genomic space of biosynthetic gene clusters (BGCs) within microbial genomes. Through bioinformatics and extensive curation, different databases have rapidly emerged categorizing NPs according to chemical structures, functions and other features (Hammami et al., 2010; Diminic et al., 2013; Ichikawa et al., 2013; Tremblay et al., 2016; Palaniappan et al., 2020; Blin et al., 2021). The presence of BGCs in bacteria has a taxonomic correlation across strains of the same species (Adamek et al., 2018). However, horizontal gene transference also plays an important role in BGC acquisition by evolutionary pressure (Osbourn, 2010).
The goal of harnessing the full potential of NPs often centers around understanding the molecular mechanisms governing their biosynthesis. Notably, NP production is subject to regulation influenced by several factors, including pleiotropic and pathway-specific factors. This regulation often involves a complex interplay of regulatory genes (transcription factors and sigma factors), within the BGCs (Ortet et al., 2012; Otani et al., 2022). While biosynthetic gene-centered databases like antiSMASH (Blin et al., 2019), MIBiG (Kautsar et al., 2020) or Bactibase (Hammami et al., 2010) have been crucial for studying the genetic diversity, databases focusing on accessory genes (e.g., regulatory genes) are less utilized. Furthermore, despite the success of accessory genes-centered databases in studying regulation [such as ARTS (Mungan et al., 2020) and P2TF (Ortet et al., 2012; Otani et al., 2022)], limited information is available regarding regulatory genes across the diversity of BGCs.
Regulatory mechanisms are crucial for controlling the transient expression of NPs in nature and artificial cultures (Barka et al., 2016). Genes on bacteria are regulated by a series of sensing-effector mechanisms, the sensing mechanism consists of a sensor domain (input domain) responsible for detecting a specific stimulus, and the effector mechanism consists of a domain responsible for making the corresponding action in response to the stimuli (output domain). The regulatory mechanism in bacteria is directed by Transcription Factors (TFs), which regulate genes by activating their output domain in response to a stimulus. One-component systems (OCS), the main regulatory mechanism found in microbial BGCs, are TF with both sensing and effector domains (Ulrich et al., 2005). On the other hand, external stimuli are sensed by Two-component systems (TCSs), which consist of an autophosphorable histidine kinase (HK) and a Response Regulator (RR) (McLean et al., 2019). Typical HKs have an extracellular sensor domain, a conserved histidine residue domain, and a catalytic domain. RRs have a phosphoacceptor region with a conserved aspartate residue and an effector domain (DNA interaction, proteins interaction, or enzymatic region) (Groisman, 2016; McLean et al., 2019). There have been some analyses of regulatory genes of BGC in Streptomyces and other relevant NP producers. For example, McLean et al. (2019) described a survey of potential TCS on S. venezuelae and some of them show a regulatory function on antibiotics. Also, a pangenome analysis recently reported that Streptomyces Antibiotic Regulatory Proteins (SARPs) TFs are less conserved on Streptomyces species (strain-specific) and control the BGCs in which they are encoded (Otani et al., 2022).
Accessing microbial NPs from environmental strains remains challenging because replicating natural conditions under artificial settings is practically impossible. Given the conservation of BGCs among taxonomic-related strains, known regulatory mechanisms can be transposed to environmental strains to activate un-expressed (silent) BGCs (Martínez-Burgo et al., 2019; Krause et al., 2020). While, several studies now incorporate ecological insights into the design of drug discovery pipelines (Som et al., 2017), novel approaches are required to understand potential BGC activation mechanisms and facilitate NP discovery from environmental strains.
In this study, we propose a framework to identify closely related regulatory elements from environmental strains from the Cuatro Cienegas Basin (CCB), the Calakmul rainforest, in Mexico; and the Comau fjord, Penas Gulf, and Valparaíso beach in Chile. By utilizing Hidden Markov Models from the Pfam database to detect key protein domains and a well curated BGC database (MIBiG), we generated a database of 2,694 BGCs including 784 BGCs from our strain collection. We conducted phylogenetic analyses of regulatory mechanisms (TFs and TCS) on both known and predicted BGCs to study activation mechanisms. This research contributes to our understanding of the diversity of regulatory mechanisms within BGCs and enhances our ability to predict activators in environmental isolates, thereby advancing the field of NP discovery.
2. Materials and methods
2.1. Genome sequences
High quality genomic information from a total of 40 environmental strains are included in this study (Supplementary material). 23 Environmental strains were isolated from Cuatro Cienegas Basin, and eight strains from Calakmul in Mexico. Genome sequences were obtained using methods previously reported (Gallegos-Lopez et al., 2020). On the other hand, nine genomes of strains isolated from various locations in Chile (4 from Valparaiso, 4 from Comau Fjord, Huinay and 1 from Penas Gulf) were also included. All sequences are available on GenBank database, and raw data were deposited to a BioProject with accession numbers provided in Supplementary material. All genomes were assembled using PATRIC (Davis et al., 2020) and annotated using Rapid Annotation of microbial genomes using Subsystems Technology (RAST) (Overbeek et al., 2014). Preliminary taxonomic classification was determined based on average amino acid identity (AAI) using The Microbial Genomes Atlas (MiGA) (Rodriguez-R et al., 2018).
2.2. BGC detection
Biosynthetic gene cluster was predicted in all genomes using antiSMASH version 6.0 (Blin et al., 2021). BGC completeness was calculated using the BiG-FAM database (Kautsar et al., 2021). Only regulatory proteins of complete BGCs were used in the analysis. Sequences of BGC reference were downloaded from Minimum Information about a biosynthetic gene cluster (MIBiG) (15).
2.3. Histidine kinase detection
Histidine kinase class I and II have a different structure, class I has a dimerization and histidine phosphoacceptor (DHp) domain and class II has a histidine-containing phosphotransfer (HPt) domain, but both share a similar CAtalytic domain (CA domain). To detect all HK first all CA-containing were recovered and classified as class I (DHp-containing) and class II (HPt-containing). Proteins with Histidine Kinase CA domain, a domain present in HK class I and II, were used to recover all potential HK in MIBiG and genome sequences. Protein sequences with CA domain were detected with HMMER version 3.3.2 (Nov 2020)1 using Hidden Markov models (HMM) of CA domains (Table 1) from Pfam-A database at default values (per-sequence E-value of 0.01 or less and a per-domain conditional E-value of 0.01 or less).
Histidine phospho-acceptor domain (DHp) was detected in CA domain proteins. DHp was detected with HMMER using HMM from the Pfam database (Table 2) at default values. Class II HK were protein sequences with a CA domain and Histidin phosphotransfer domain (Hpt), and Class I HK were protein sequences with a CA domain and DHp. HKs from TCS were considered kinases with a Response Regulator next to in the same direction frame.
2.4. Transcription factor and response regulation detection
TFs from BGC were detected using selected TF domains using HMM from the Pfam database (Table 3). TF domains were detected using HMMER with default parameters. RRs were detected from previously detected TF. Response regulator receiver domain (PF0072) with the phosphorable aspartate residue. Were detected and filtered, after that, a TF was classified as Response Regulator if its gen is next to a previously detected HK in the same strand, the rest TF were discarded as RR. All TF with both, the OmpR domain (PF00486) and Bacterial Transcriptional Activator Domain (BTAD) (PF03704) were classified SARP.
2.5. Phylogenetic analysis
Protein sequences with the same phosphoacceptor domain or transcription factor domain were used to calculate the phylogenetic tree. All sequences were aligned using MUSCLE (Edgar, 2004) using default parameters. Phylogenetic trees were calculated using FastTree (Price et al., 2010) at default parameters (Jukes-Cantor + CAT model). Microreact (Argimón et al., 2016) web was used for tree visualization. All the phylogenetic trees obtained in this study are available as Supplementary material.
3. Results
Our database comprises a total of 2,694 complete BGCs, with 1,910 originating from MIBiG and 784 newly reported from environmental samples in our strain collection. Of these BGCs, 204 contain at least one histidine kinase, while 1,291 encode at least one transcription factor. Notably, 2,213 BGCs do not contain either an HK or TF. We identified a total of 225 HKs, with 137 previously identified in MIBiG BGCs and 88 from environmental samples. Additionally, we identified a diverse array of 2,777 distinct TFs, encompassing 1,952 from MIBiG BGCs and 825 from environmental samples. The remaining 2,213 BGCs do not encode any HKs or TFs within the cluster.
To analyze the preference for NP classes among actinobacterial strains from different environments, we examined the distribution of BGC classes. We calculated the percentage of BGC classes that contain at least one HK, as shown in Figure 1A. For BGCs belonging to strains from the Cuatro Cienegas Basin that contain an HK, we observed the following distribution: Polyketide (25.6%), non-ribosomal peptide (NRP, 43.9%), ribosomally synthesized and post-translationally modified peptide (RiPP, 13.4%), terpene (6.1%) and other (11%). For rainforest samples, the distribution was as follows: polyketide (28.6%), NRP (28.6%), RiPP (23.8%), terpene (9.5%), saccharides (4.8%), alkaloids (0%), and other (4.8%). In contrast, the BGCs from marine strains exhibited the following distribution: polyketide (16.7%), NRP (41.7%), RiPP (25%), terpene (8.3%) and other (8.3%). Finally, the distribution of BGCs from the MIBiG dataset was as follows: polyketide (38.5%), NRP (27.6%), RiPP (17.9%), terpene (1.9%), saccharides (7.7%), alkaloid (1.9%), and other (4.5%).
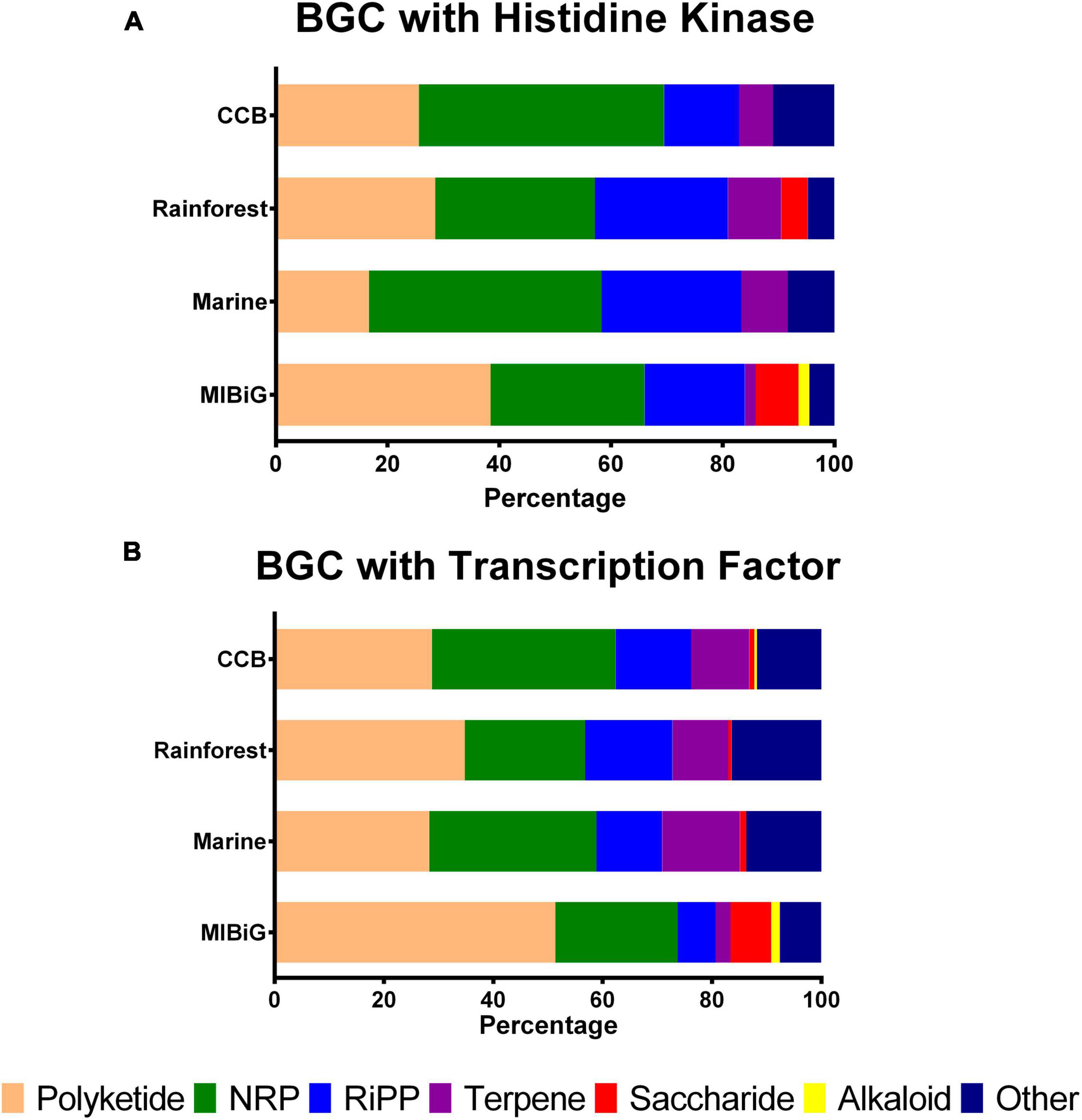
Figure 1. Biosynthetic gene clusters classes distribution with at least one regulatory element by environment. (A) BGCs with a histidine kinase by product class (B) BGCs with transcription factors by product class. NRPs, non-ribosomal peptide, RiPP, ribosomally synthesized and post-transcriptionally modified peptides.
Histidine kinases were predominantly associated with BGCs from Polyketides in the MIBiG database and rainforest samples, while BGCs from NRP were primarily linked to the Cuatro Cienegas Basin and marine strains. Alkaloid-containing BGCs were exclusively found in the MIBiG dataset, and saccharide-containing BGCs were identified in both Cuatro Cienegas Basin and MIBiG samples.
On the other hand, Figure 1B illustrates the percentage of BGC classes containing TFs. In BGCs from the Cuatro Cienegas Basin with TFs, we observed the following distribution: polyketide, 28.8%; NRP, 33.6%; RiPP, 13.8%; terpene, 10.6%; saccharide, 0.9%; alkaloid, 0.5% and other, 11.8%. For rainforest BGCs with TFs, the distribution was as follows: polyketide, 34.8%; NRP, 22%; RiPP, 16%; terpene, 10.2%; saccharide, 0.6%; and other, 16.4%. Conversely, BGCs from marine environments with TFs exhibited the following distribution: polyketide, 28.3%; NRP, 30.6%; RiPP, 12%; terpene, 14.3%; saccharide, 1.1% and other, 13.7%. Lastly, BGCs from the MIBiG dataset with TFs were distributed as follows: polyketide, 51.4%; NRP, 22.4%; RiPP, 6.9%; terpene, 2.8%; saccharide, 7.5%; alkaloid, 1.5% and other, 7.6%.
Similar to the distribution of HKs, BGCs from the polyketide class were more prevalent in strains from the rainforest and the MIBiG dataset, with polyketides accounting for over half of the BGCs containing TFs in the MIBiG dataset. Similarly, NRPs with TFs were more common in both the Cuatro Cienegas Basin and marine strains. Notably, BGCs containing TFs from the alkaloid class were absent in rainforest and marine strains.
We identified the presence of Two-Component system regulatory mechanisms when both a HK and a TF were encoded in tandem, transcribed within the same strand, and the TF encoded a response regulator protein domain (PF00072). Additionally, we classified HKs without a corresponding TF as “orphan HKs,” and TFs without an associated HK as “orphan TFs.” All regulatory proteins, including HKs and TFs, were categorized based on their regulatory mechanisms into TCS, orphan HKs, or orphan TFs, as depicted in Figure 2A. Our analysis revealed that 66% of the HKs are part of a TCS, with a relative abundance of 69% in the MIBiG dataset, 58% in BGCs from strains in the Cuatro Cienegas Basin, 66% in BGCs from the rainforest, and 80% in BGCs from strains originating from marine environments.
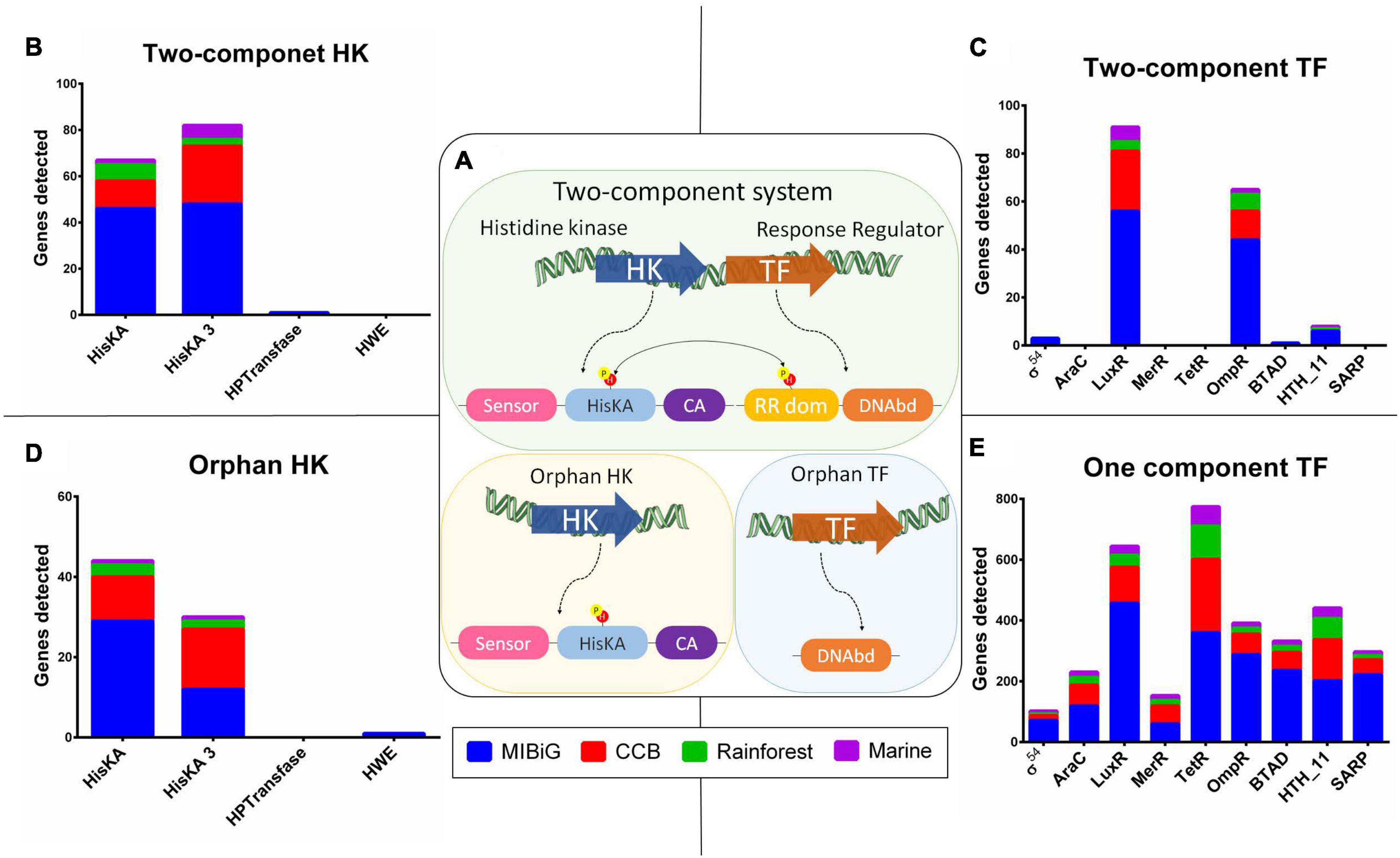
Figure 2. Histidine kinase and transcription factor Pfam domain distribution. (A) Schematic representation two-component system (TCS), structure of HK without a conjugated response regulator (orphan HK), and one-component system TF (OCS TF). (B) HK with a response regulator from a TCS. (C) TF with DNAbd, and response regulator domain (RR dom) from a TCS. (D) HK without response regulator (orphan HK). (E) TF with a DNA binding domain (DNAbd), from OCS.
Within the TCS, HisKA and HisKA3 were the predominant contributors among HKs, accounting for 49.3 and 49.7%, respectively (Figure 2B). In contrast, HPTransfase and HWE made minimal contributions, comprising only 1 and 0%, respectively. Among RR, LuxR and OmpR protein family domains were the major contributors, representing 54 and 38%, respectively. BTAD and Helix-turn-helix (HTH_11) protein families contributed to RRs with 0.6 and 4.7%, respectively. Sigma-54 domain was present in 2% of all RRs, while the remaining domains were not associated with a TCS (Figure 2C). As expected, the MIBiG database significantly contributed to the presence of TCS due to its higher number of BGCs compared to environmental samples. The LuxR domain was predominant in RRs across most cases, with occurrences in 50.9% of MIBiG RRs, 67.6% of CCB RRs, and 66.7% of marine RRs. The exception was in rainforest RRs, where the LuxR domain was found in 58.3% of cases.
In a similar pattern to HKs within the TCS, we observed that HisKA and HisKA3 domains were present in almost all orphan HKs, accounting for 58.7 and 40%, respectively, while HWE contributed 1.3% (Figure 2D). Among the TFs associated with the orphan control system (OCS) mechanism, the TerR family dominated with a representation of 23%. The LuxR domain constituted the second most prevalent family at 19.1% within OCS mechanisms, followed by the OmpR family at 11.6%. Notably, TetR was more prevalent in OCS from the Cuatro Cienegas Basin, rainforest, and marine environments, accounting for 29.7, 34, and 29.6%, respectively (Figure 2E). In contrast, within the MIBiG dataset, 22.7% of OCS-associated BGCs contained a LuxR domain.
To examine the impact of environmental factors on BGC diversity across different genera, we analyzed the diversity of BGC classes and compared them to the isolation environments of each genus. Our analysis revealed a clear relationship between the occurrence and diversity of BGCs and the variety of environments where microorganisms were isolated. Figure 3 illustrates the diversity of BGC classes containing a HK from a TCS across orders and phyla. Host-associated environments encompass both pathogenic and symbiotic cases. Some phyla exhibit limited diversity; for instance, the Firmicutes phylum contains only NRP, RiPP, and other compound classes. Proteobacteria exhibit NRP, Polyketides, and Saccharides. In contrast, the Actinobacteria phylum boasts the highest number of members, covering all BGC classes. Within this phylum, the Micromonospora and Streptomyces genera are found in all environments, while most orders in the database were isolated from only one or two environments, with Bacillus being an exception, isolated from three different environments.
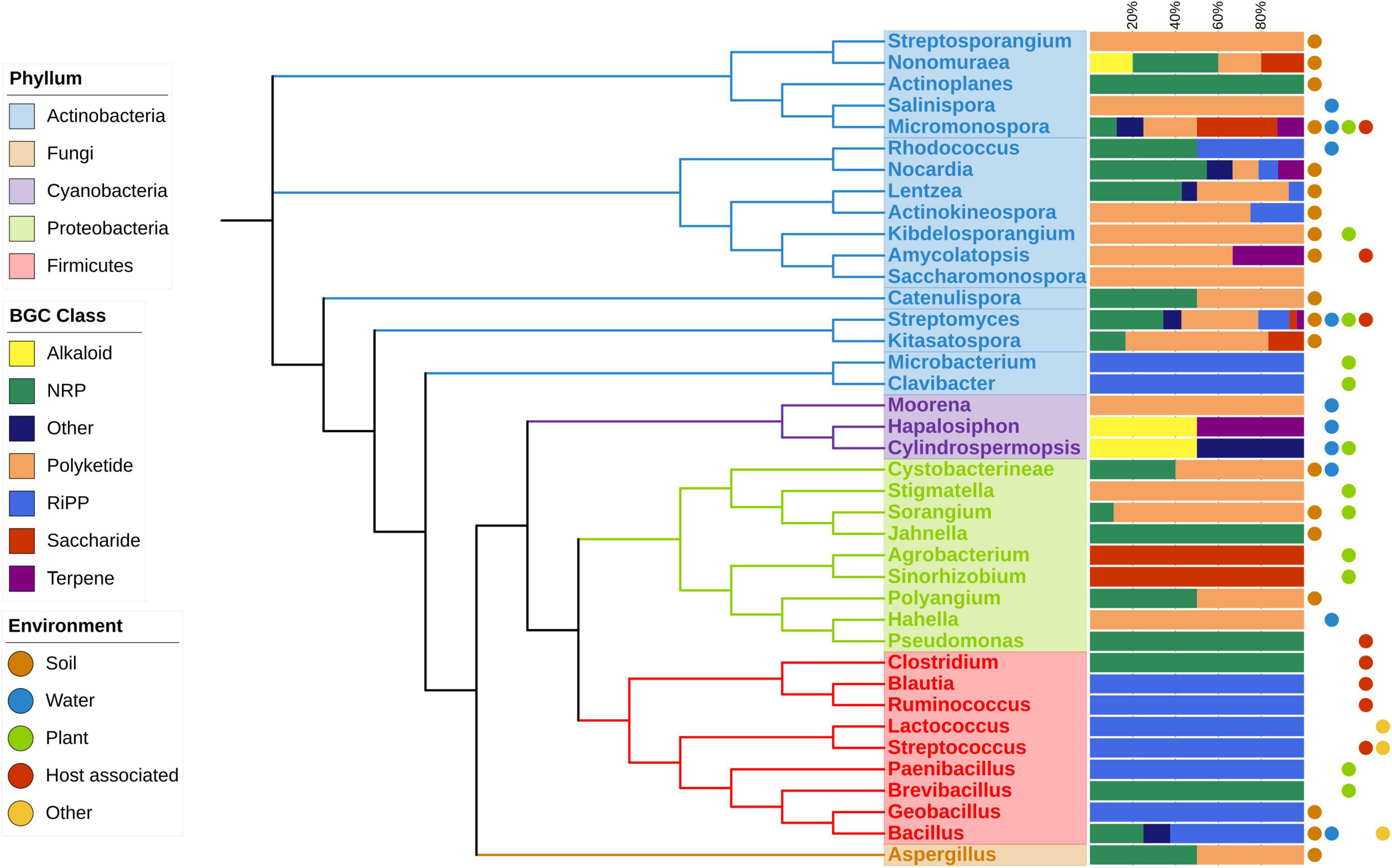
Figure 3. Phylogenetic distribution of strains with BGCs with a HK TCS, BGC class and isolation environment are depicted. The phylogenetic tree was inferred using rRNA of the most representative species on each genus. Dots at the right of the figure show the type of environments where at least one strain was isolated.
3.1. Distribution of His kinase A domain
Histidine kinase A (PF00512) and the catalytic ATP-binding domain (PF02518) were identified in a total of 111 protein sequences, with 75 originating from the MIBiG database and 36 from environmental samples. Specifically, HKs associated with lanthipeptide-producing BGCs formed a distinct clade within the phylogenetic tree (Figure 4, blue region), all originating from Gram-positive bacteria. In contrast, glycopeptide-producing BGCs form a clade (Figure 4, orange region) and were primarily composed of actinobacterial strains, including two environmental strains: one from Lentzea sp. in the Cuatro Cienegas Basin and another from Streptomyces sp. in the rainforest.
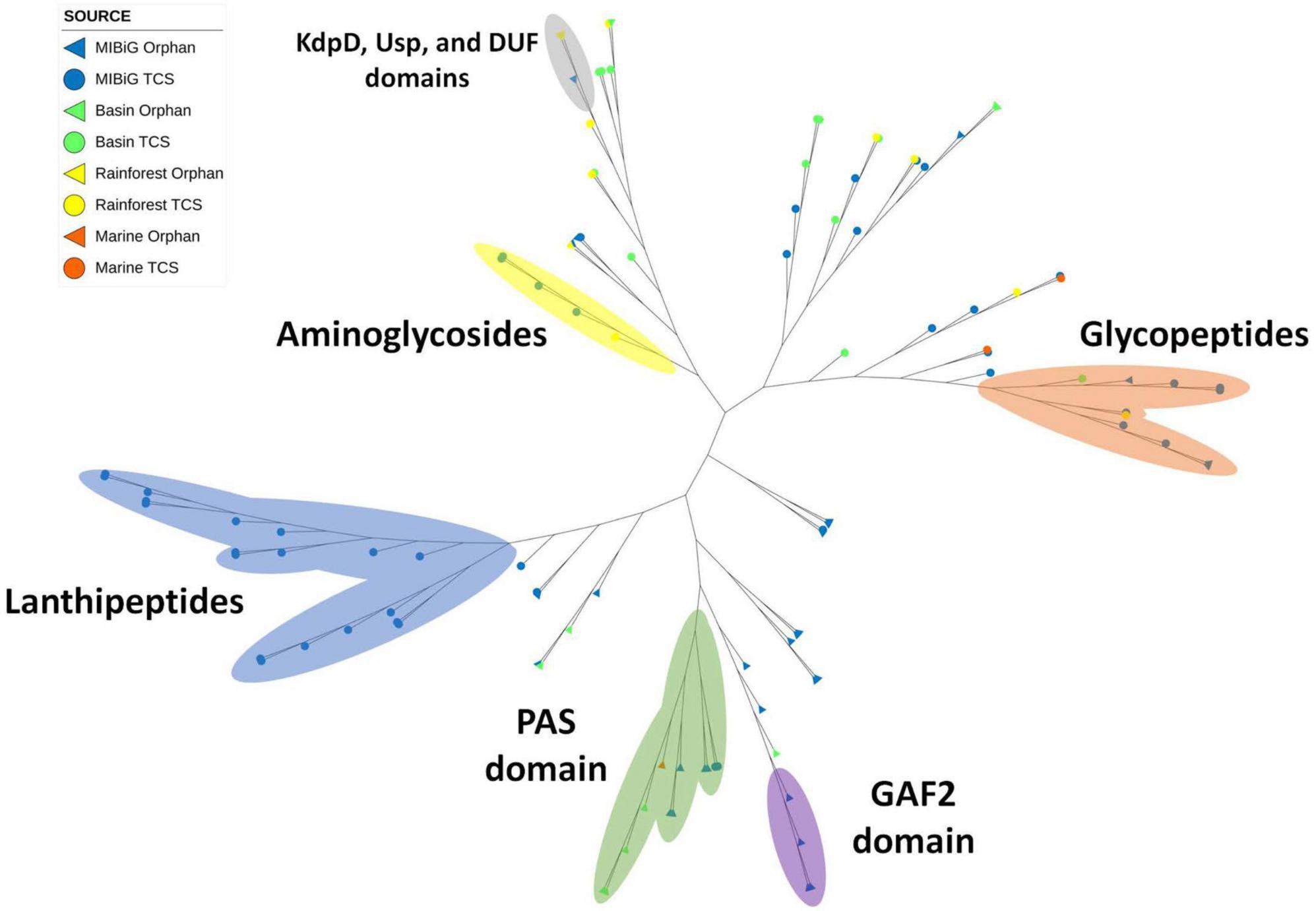
Figure 4. Phylogenetic tree of histidine kinase with HisKA domain (PF00512). Regions depicted in blue, orange, and yellow correspond to clades with HK from lanthipeptides, glycopeptides, and aminoglycosides producing BGCs, respectively. Regions in green, purple, and gray correspond to proteins with PAS, GAF2, and KdpD-Usp-DUF domains, respectively.
A smaller clade comprised of aminoglycoside-producing bacteria was also identified, with one Streptomyces sp. strain from a rainforest sample clustering within it (Figure 4, yellow region). Diverse protein domains were detected within the potential sensor region. Notably, an atypical structure containing an osmosensitive K + channel His kinase sensor domain: Kdp (PF02702), universal stress protein family (PF00582), and domain of unknown function DUF4118 (PF13493) was identified within a Streptomyces-exclusive clade. This clade included three Streptomyces spp. from rainforest sequences and one laidlomycin-producing BGC from Streptomyces sp. CS684 in the MIBiG database (Figure 4, gray region).
Additionally, the PAS domain was detected in 20 sequences, with 12 of them forming a distinct clade consisting of diverse bacterial genera associated with toxin and stress-response BGCs (Figure 4, green region). This clade encompassed a wide range of microorganism genera, including Pseudomonas, Agrobacterium, Cylindrospermopsis polyangium, and Jahnella. Furthermore, four environmental samples from Nocardia sp. and one from Streptomyces sp. were found within this clade, originating from the Cuatro Cienegas Basin and marine environments, respectively. Finally, GAF2 domains were identified in four sequences from soil bacteria, including two from Sorangium cellulosum, one from Streptomyces sp. CNT302, and one from Amycolatopsis tolypomycina (Figure 4, purple region).
3.2. Distribution of histidine kinase 3
Histidine Kinase 3 (PF07730) and the catalytic ATP-binding domain (PF02518) were identified in a total of 112 sequences, including 60 from MIBiG, 40 from the Cuatro Cienegas Basin, 7 from marine environments, and 5 from the rainforest. A distinct clade associated with calcium-dependent antibiotics BGCs was identified (Figure 5, orange region). Within this clade, two environmental samples were clustered, one originating from Streptomyces sp. SS52 and the other from Lentzea sp. CC55. Notably, this clade predominantly comprised soil actinobacteria or samples obtained from soil environments.
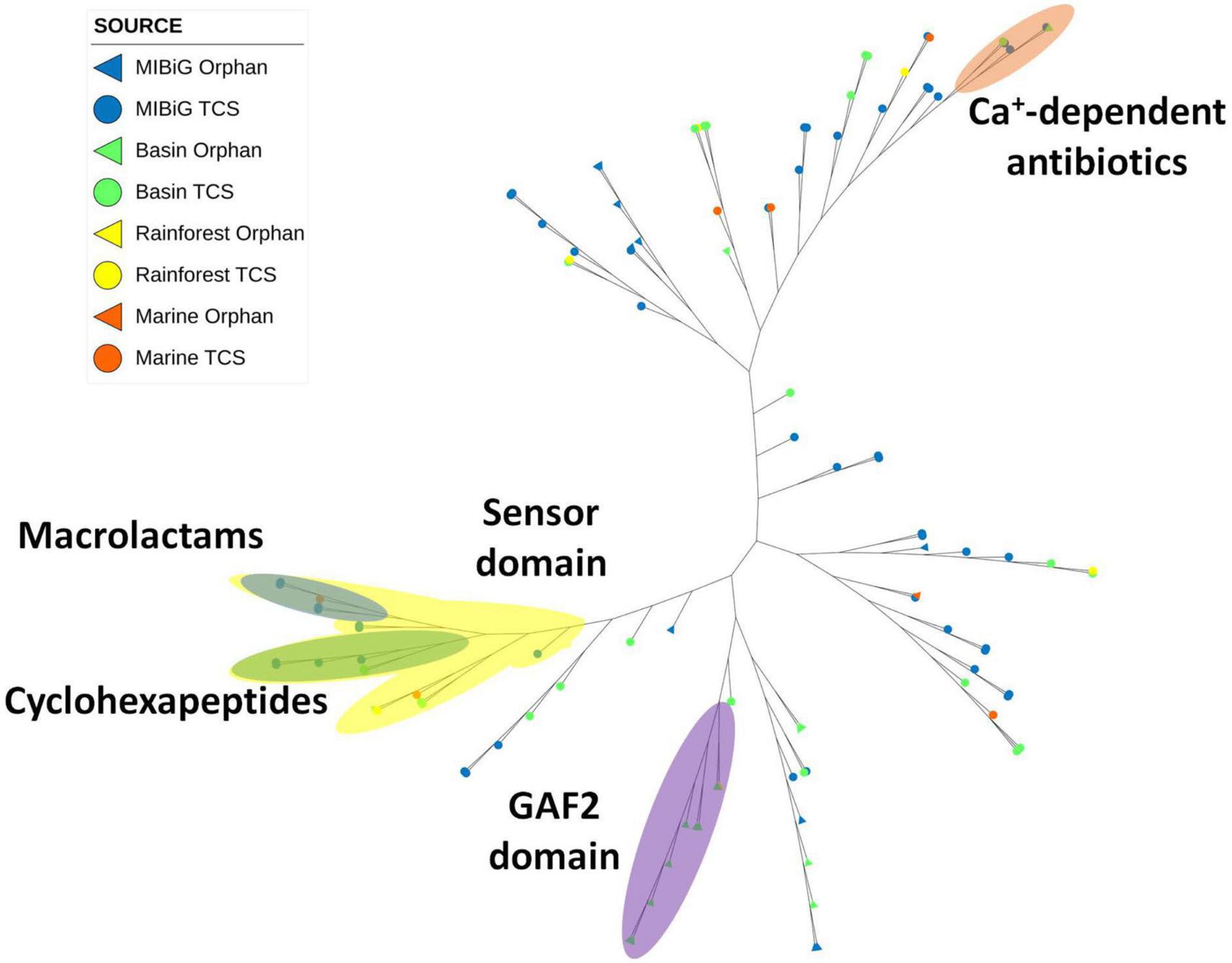
Figure 5. Phylogenetic tree of histidine kinase HisKA_3 domain (PF07730). The orange region corresponds to HK from calcium-dependent antibiotics BGC. The yellow region corresponds to sequences with the sensor domain (PF13796). The blue region corresponds to sequences from macrolactams BGC. The green region corresponds to cyclohexapeptides BGC. The purple region corresponds to HK with the GAF2 domain (PF13185).
Another clade with BGCs responsible for producing macrolactams was identified (Figure 5, blue region). This clade primarily consisted of actinobacterial strains isolated from aquatic environments, with one sample originating from the marine environment. Another clade associated with the production of cyclohexapeptides was also observed. This clade comprised Streptomyces sp. strains from MIBiG and was a subclade of a larger group containing the sensor domain (PF13796) in the sensor region. This sensor domain is associated with proteins containing histidine kinase 3 and the catalytic ATP-binding domain. Notably, the majority of these histidine kinases were associated with BGCs responsible for producing NRPs products.
Additionally, a clade containing environmental samples characterized by the presence of the GAF2 domain in the N-terminal region was identified (Figure 5, purple region). All members within this clade were isolated from soil environments, with only one from the rainforest, while the rest were from the Cuatro Cienegas Basin. Most of the BGCs within this clade produced products classified as polyketides.
3.3. Distribution of LuxR-family domains
A total of 735 transcription factors carrying the LuxR domain were identified across all databases, with 513 originating from MIBiG, 143 from the Cuatro Ciénegas Basin, 44 from the rainforest, and 35 from marine environments. Notably, polyketide and NRPs classes were the most abundant, constituting 35.8 and 36.2% of the total, respectively. Furthermore, the AAA ATPase domain (PF13191) was detected in 191 sequences featuring a LuxR domain. Most of these sequences clustered within a distinct clade (Figure 6, yellow region). This domain is involved in various cellular functions, all of which require energy derived from ATP. These functions encompass roles such as transcriptional activation, and 36 sequences within this clade are derived from environmental samples.
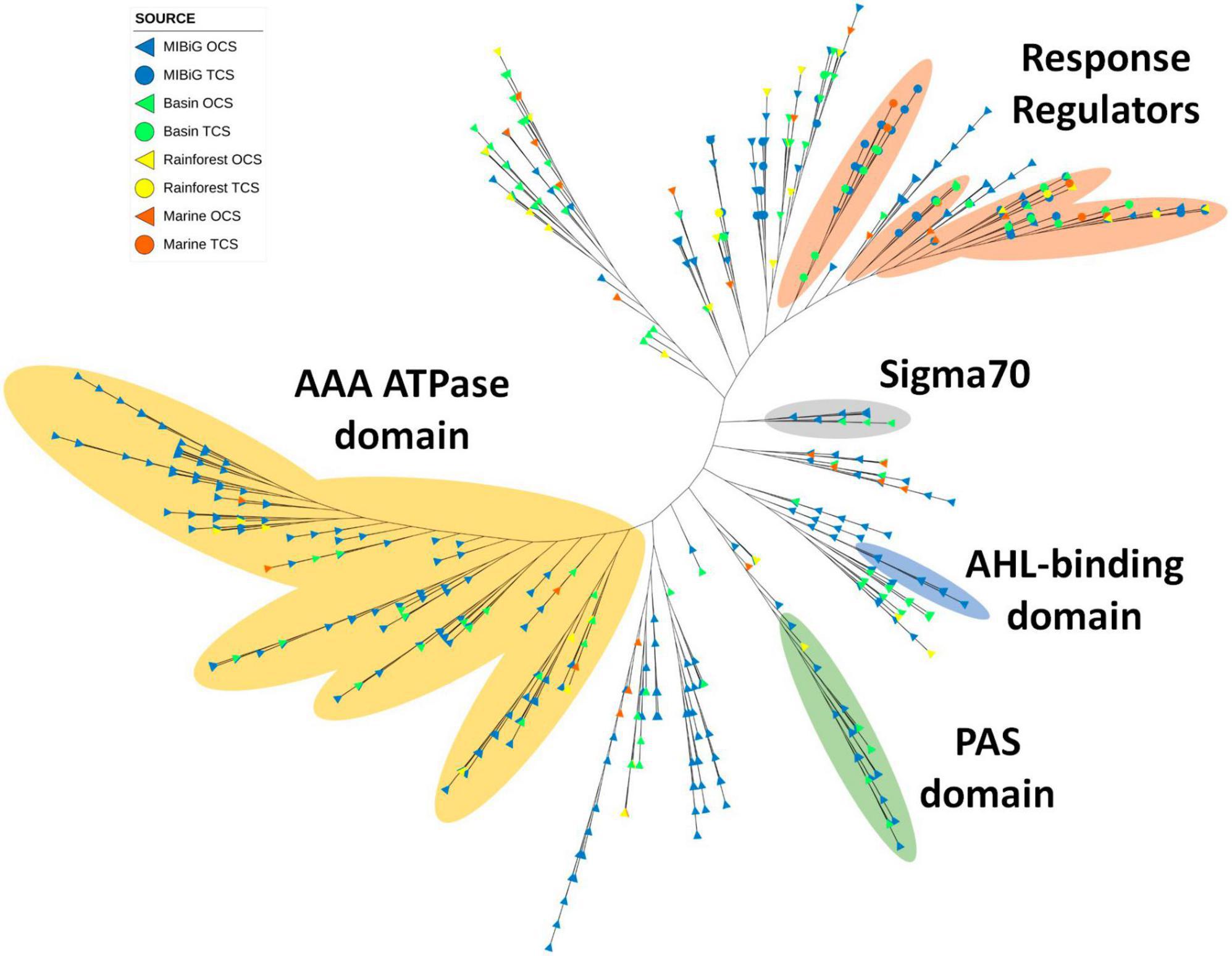
Figure 6. Phylogenetic tree of TF with LuxR domain family (PF00196). Yellow region corresponds to TF clade with AAA ATPase domain. Regions depicted in orange, gray, blue, and green correspond to TFs with response regulator protein domain (PF00072), sigma70, N-acyl homoserine lactone (AHL) binding domain and PAS domain, respectively.
Four closely situated clades primarily corresponded to LuxR proteins with response regulator activity. Approximately half of the response regulators were associated with NRPs metabolites, followed by polyketides (32.5%). Interestingly, response regulators were evenly distributed across these clades. Another small clade featuring the sigma 70 region was detected, with members predominantly originating from the Cuatro Ciénegas Basin and all corresponding to Streptomyces strains. The transcription factors associated with BGCs in this clade were responsible for the production of BGCs and polyketides, except for those linked to saccharide (kanamycin) and terpene (tiancilactone) products.
Additionally, a clade contained LuxR proteins with the autoinducer binding domain, with all sequences originating from the MIBiG database and associated with polyketide and NR-like BGCs. Among these BGCs, four belonged to Pseudomonas, four to Burkholderia, and one to Methylobacter. Finally, the PAS domain was identified in 27 sequences clustered within a single clade, with six originating from environmental samples: five from the Cuatro Ciénegas Basin and one from the marine environment. Most of the transcription factors within this clade were associated with polyketide BGCs.
3.4. TetR family diversity
The TetR domain emerged as the most prevalent domain among transcription factor proteins associated with BGCs, with 22% of all transcription factors featuring this domain. Following closely was the LuxR domain, found in 21% of all transcription factors. Notably, 40% of these transcription factors were linked to polyketide BGCs, while 33% were associated with NRPs. Furthermore, 46% of all transcription factors were sourced from the MIBiG database.
One clade comprising 42 proteins featured the TetR C-terminal 14 (MftR C-terminal domain) (Figure 7). The majority of these transcription factors were associated with polyketides, with 14 originating from environmental samples. Among the environmental samples, rainforest sequences were more prevalent, accounting for six transcription factors. Another 37 transcription factors were identified with the TetR C-terminal 11 domain, with 12 of these sequences originating from environmental samples. Within the MIBiG database, polyketides were more common, while NRPs were more prevalent among environmental samples.
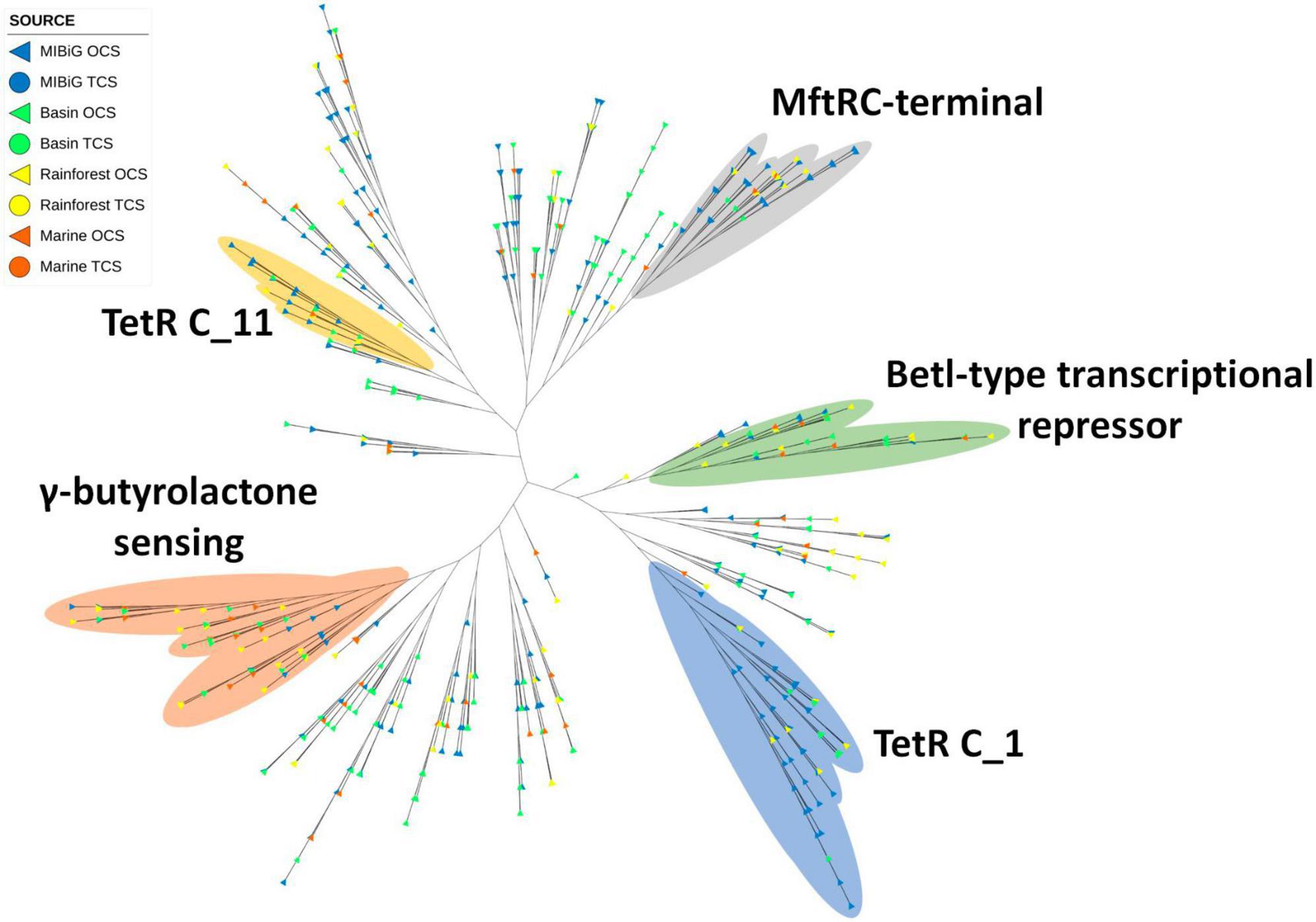
Figure 7. Phylogenetic tree of TF with a TetR family domain (PF00440). Yellow and blue regions correspond to TF clades with a TetR C_1 and TetRC_11 domain (PF02909 and PF16859) respectively. Regions depicted in gray, green, and orange correspond to TF with MftRC-terminal, Betl-type transcriptional repressor domain, and γ-butyrolactone sensing domain, respectively.
A separate clade containing 40 transcription factors featured the TetR C-terminal 6 domain. This clade included a higher proportion of environmental samples (23 proteins). Similar to the TetR C-terminal 11 clade, sequences from the MIBiG database were predominantly associated with polyketides, while environmental samples showed a preference for NRPs. Additionally, a clade encompassing 66 proteins contained the TetR C-terminal 1 domain. Among all TetR C-terminal proteins, this domain was the most prevalent. Within this clade, polyketide and NRP BGCs were more common, with 22 and 20 BGCs, respectively. Lastly, from a review of the literature, a clade of transcription factors activated by γ-butyrolactone was identified. This clade comprised five proteins (ScbR, ScbR2, SabR, CprA, and CprB) clustered together.
3.5. OmpR family
A total of 456 proteins containing the OmpR family domain. Among them, 50.9% are found in polyketie BGCs, and 25.0% are located in NRP-producing BGCs. Phylogenetic trees reveal a clade of proteins with a bacterial transcriptional activator domain. The presence of these two domains is characteristic of SARP. The majority of sequences in this clade originate from Streptomyces, accounting for 76% in our database (72% overall). All product classes are evenly distributed along the tree (see Figure 8).
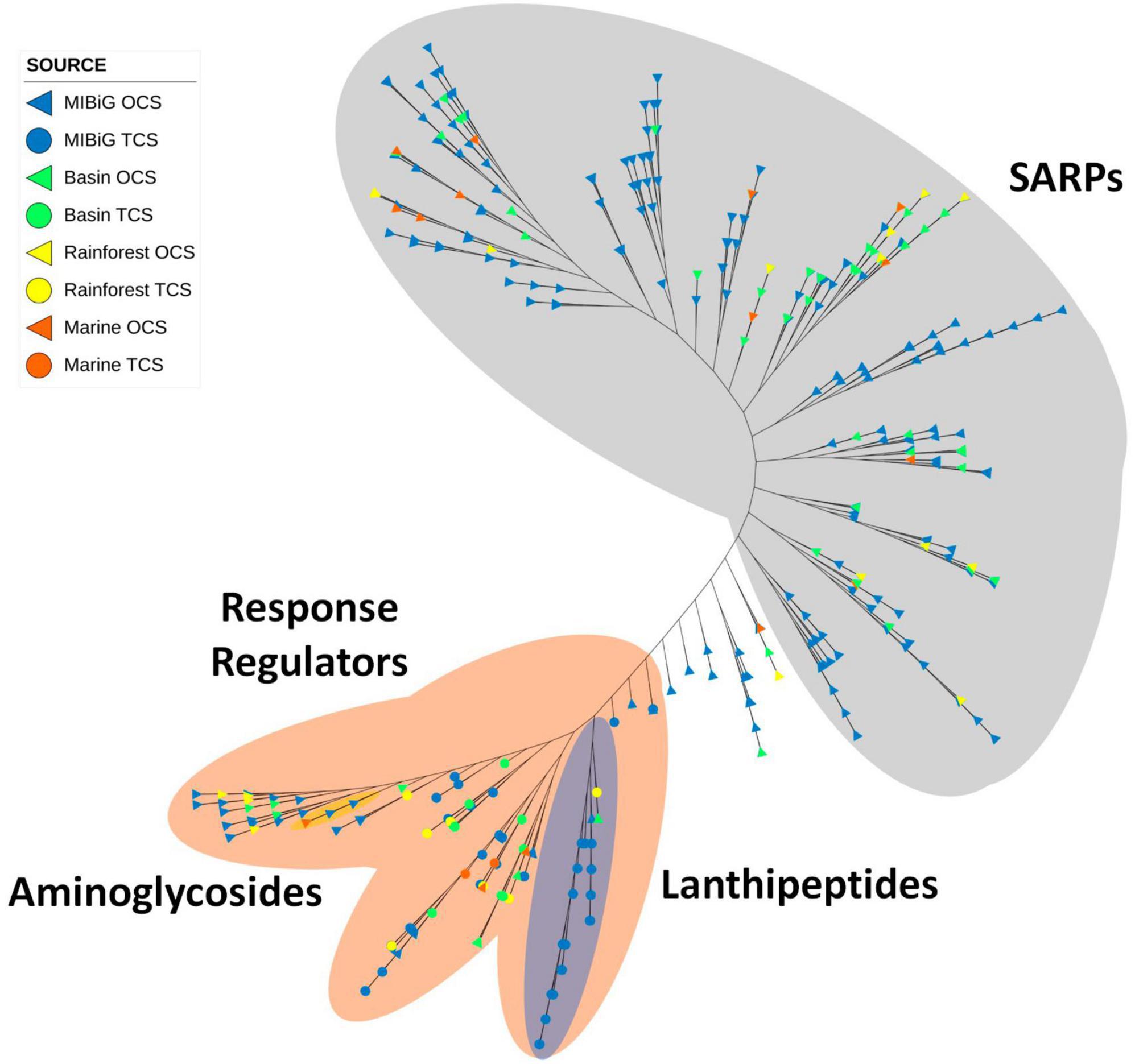
Figure 8. Phylogenetic tree of TF with OmpR family domain. Gray area corresponds to proteins with SARP structure. The orange area corresponds to proteins with RR structure. The blue area indicates a clade with lanthipeptide-produced BGC. The yellow area corresponds to aminoglycoside-produced BGC.
In Figure 8, all 66 RR proteins from TCS were grouped within the orange area. Protein sequences from lanthipeptide-producer BGCs were clustered in the blue area of Figure 8. Within the SARP clade, most sequences from environmental samples are grouped into conserved clades. Specifically, the majority of the 11 samples sequences within SARP were detected in polyketide-producing BGCs, whereas MIBiG sequences in the same clade are also from polyketide-producing BGCs.
Most proteins with a RR-structure belong to a TCS and correspond to NRP-producing BGCs. Within these clades, one subclade with an RR structure does not have a histidine kinase associated with it; most of these are part of polyketide-producing BGCs. Environmental samples are represented in slightly higher proportion among RRs from TCS (33.3%) compared to SARP (25.3%).
3.6. Helix-turn-helix family
A total of 450 TFs encode an HTH domain (PF08279), with the majority originating from NRPs and polyketide-producing BGCs (41.6 and 33.3%, respectively). The distribution by isolation site is similar to that of TetR, where 46% of the proteins come from MIBiG database, 30% from the Cuatro Cienegas Basin, 16% from rainforests, and 8% from marine environments. A significant portion of these proteins also contains a LuxR domain, as shown in the green area in Figure 9. In this clade, NRPs and polyketide BGCs are common, RiPPs at 28, 29, and 24%, respectively. More than half of these proteins originate from the MIBiG database, accounting for 57%.
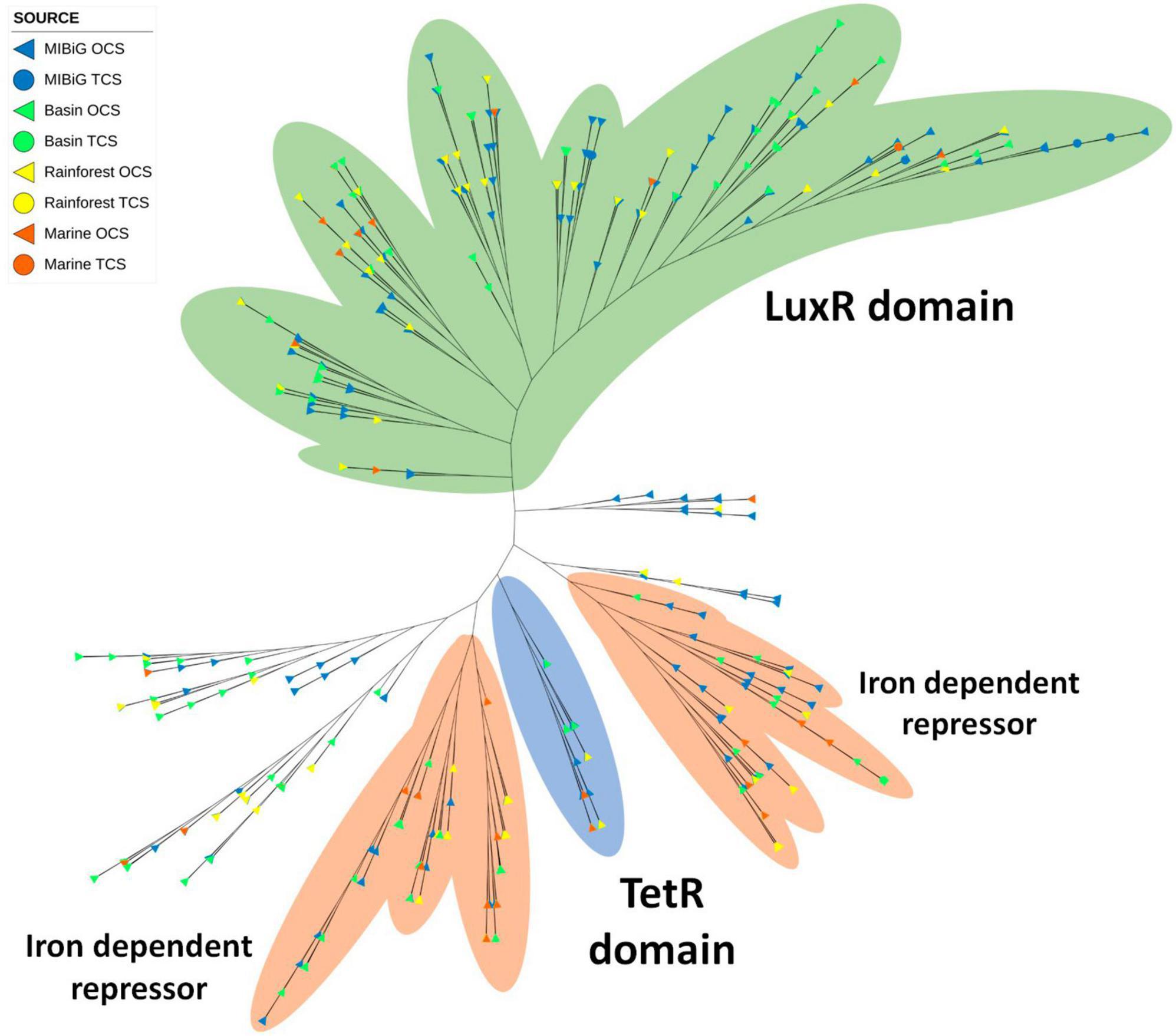
Figure 9. Phylogenetic tree of transcription factor of Helix-turn-helix family (PF08279). The green area corresponds to sequences with an LuxR domain. The orange area corresponds to sequences with Iron dependent repressor domain (PF01325). Blue area contains the sequences with the TetR domain.
TetR domains were identified in 40 TFs, and many of them are scattered throughout the phylogenetic tree. Among these 40 sequences, 7 form a distinct clade of TFs with the TetR domain. Most of them are associated with polyketide BGCs, followed by NRPs BGCs. The Iron repressor domain (PF01325) was detected in 125 TFs, forming two closely related clades. These two clades are separated by the TetR clade. Within all TFs containing an Iron repressor domain, 42% are associated with polyketide BGCs, and 38% are linked to NRPs BGCs. Additionally, potential siderophore-producing BGCs from environmental samples were identified, including heterobactin and coelichelin.
4. Discussion
The relationships between organisms and their environments can influence genetic variation among species, and this variation is correlated to the environment independently of physical locations (Wang and Bradburd, 2014). To investigate diversity and the correlation between regulatory mechanism variations and the regulation of BGCs in different environments, we conducted a bioinformatic analysis of regulatory elements in 42 strains from three distinct environments: 24 strains from a low-phosphate cambric-like environment (the Cuatro Cienegas Basin), 9 strains from the Calakmul rainforest in Mexico; and 9 strains from various marine environments in Chile. We also included well curated BGCs from MIBiG database. Our analysis primarily focused on the phylogenetic relationships among 2,777 selected transcription factors, which are the primary known mechanisms of gene regulation, 225 histidine kinases (HKs), the primary known mechanism for environmental sensing, and the union of both, which encompassed 168 two-component systems (TCSs). TFs and HK isolated from ES do not show a significant difference compared to the MIBiG database, this can be explained by the size of the sample used, with few organisms from each environment, Tidjani et al. (2019) found that microorganisms isolated from the same environment, same genus, even same sample can have different genes, at a scale of mm3, especially in BGC regions. To find a difference in the environment-exclusive regulation mechanism a big sample should be used.
Our analysis revealed that one-component systems are the primary regulatory system for BGCs. However, a substantial number of BGCs lack a regulatory mechanism within the cluster, suggesting external regulatory mechanisms. Regulatory genes located outside the BGCs, with multiple regulation targets or pleiotropic effects, control many secondary metabolites or morphological development (Lu et al., 2017). Most BGCs with a TF or an HK corresponded to the polyketides or NRPs products. This might be attributed to a bias in natural product research, resulting in an overrepresentation of these two molecular families in databases. In contrast, among other BGC classes, only five saccharide BGCs (three from CCB, one from the rainforest, and one from marine environment) were detected as complete, but only two from CCB had a TF or an HK. Additionally, only one alkaloid BGC from the environmental samples was detected, but it did not have a TF or an HK.
In the HisKA phylogenetic analysis, a clade of lanthipeptide BGCs was observed, with all HKs within this clade being part of a two-component system. For instance, nisin and Bovicin HJ50, two lanthipeptides, are well-known natural products with an autoinducible mechanism (Ni et al., 2011; Özel et al., 2018). Some defined clades containing natural products from similar chemical families were also identified, such as glycopeptides and aminoglycosides. In the glycopeptide clade, various glycopeptides were grouped together, including A40926, A-47934, teicoplanin, UK-68,597, feglymycin, auroramycin, and kistamicin A. Interestingly, three natural products in this clade, namely rapamycin, feglymycin, and auroramycin, are also part of this clade. Two environmental samples producing NRPs (Lentzea sp. CC55 from CCB and Streptomyces sp. KL110B from the rainforest), were also grouped in this clade. The aminoglycoside clade included gentamicin, sisomicin, and catenulisporolides (a glycosylated macrolide). Additionally, this clade included an environmental sample, Streptomyces sp. KL110B from the rainforest, which produces a polyketide.
The HisK 3 domain phylogenetic tree has a marked clade with BGCs for calcium-dependent antibiotics from the NRPs class, which included two of our environmental samples, Lentzea sp. CC55 and Streptomyces sp. SS52, both from CCB, with one of them having the potential to produce CDA1. Another different clade within the same analysis contained proteins with the putative sensor domain (PF13796), which was identified in proteins with the HisK 3 domain. This clade encompassed two subclades of cyclic proteins: macrolactams and cyclohexapeptides. The macrolactams subclade included BGCs from salinilactam, lobosamide, and sceliphrolactam, and one of the nodes corresponded to an environmental sample, Streptomyces sp. Vc67-4 from the marine environment, which has the potential to produce sceliphrolatam. The cyclohexapeptides subclade included BGCs similar to desotamide, curacomycin, dechlorocuracomycin, and ulleungmycin, along with two environmental samples from CCB, Lentzea sp. CC55, and Streptomyces sp. CC208A. Most of the members of the sensor clade produced cyclic NRPs.
The LuxR domain was the predominant contributor to the response regulator. The LuxR family is associated with quorum sensing and molecular sensing (Santos et al., 2012). Within the LuxR phylogenetic analysis, two major branches were identified. One of these branches contained the AAA ATPase domain, also known as the AAA + superfamily, which is involved in regulatory functions (Snider et al., 2008), including σ54-related transcription factors (Khan et al., 2022). In this branch, none of the TFs were part of a TCS, and it included environmental samples from all environments. Santos et al. (2012) reported an analysis of LuxR proteins in Actinobacteria genomes, revealing that only 0.81% of proteins with a LuxR domain also had an AAA ATPase domain. For the same correlation we found a total of 31.7% proteins. In three different clades on the phylogenetic analysis, we observed that most of the RR from TCS were associated with environmental samples from all environments sampled. Out of the 637 TFs belonging to the actinomyceted, only 13% (83 TFs) were RR, which differed from the 53% previously observed (Santos et al., 2012). In one of the clades, we identified proteins with a sigma70 domain, and four environmental samples, all from different Nocardia species from CCB, were included, and they were predicted to produce NRPs.
In addition, a clade containing N-Acyl homoserine lactone (AHL) binding domains was identified from previously characterized Gram negative bacteria. AHL is a known molecule involved in quorum sensing in Gram negative bacteria while GBL molecules are used for Gram positives microorganisms (Santos et al., 2012). We also observed a clade with PAS domains [PAS (PF00989), PAS_4 (PF08448), and PAS_9 (PF13426)]. Interestingly, 3.9% of Actinobacteria TFs were found to encode PAS domains, compared to the 0.10% reported previously (Santos et al., 2012). PAS domains are associated with sensing factors like oxygen, light, and redox states and are widely distributed across living organisms. In our analysis, we detected five Streptomyces strains within the PAS clade, four of which were isolated from CCB (with the potential to produce 2-methylisoborneol and candicidin) and one from rainforest (with a 2-methylisoborneol like BGC).
The TetR domain emerged as the most prevalent protein family among TF BGCs, accounting for 21.9% of the cases. The TetR family typically acts as a repressor in most instances (Ramos et al., 2005). Several proteins from our database, including ChlF1, ScbR, ScbR2, SabR, CprA, and CprB, had previously been reported as transcriptional repressors (Li et al., 2016). Some of these proteins are regulated by the presence of γ-butyrolactone, a molecule utilized as a hormone-like signal in quorum sensing, morphological differentiation, and secondary metabolism (Romero-Rodríguez et al., 2015). TetR is generally present in BGCs to repress the production of NPs until specific conditions release the signal. GBL can activate BGCs containing a TetR domain, particularly within the GBL sensing clade observed in the TetR phylogenetic tree.
The TetR phylogenetic tree revealed four marked clades. One of these branches includes the MftR C-terminal domain (PF17754) from environmental samples (rainforest, marine, and CCB). Another branch included TFs with the TetR C-terminal domain 1 (PF02909) and BetI-type transcriptional repressor, C-terminal (PF13977), both of which are associated with antibiotic expression and osmoregulation via pumps (Ramos et al., 2005). The TetR C-terminal 1 clade includes environmental samples from the rainforest and CCB, while the BetI-type clade includes samples from the three environments tested. Another branch featured TFs with the TetR C-terminal domain 11 (PF16859), which is linked to antibiotic expression, multidrug pumps, and toxin response (Ramos et al., 2005). Additionally, one of the branches contained a clade with GBL sensing TFs, which included samples from all environments and featured a predicted BGC for GBL in Streptomyces sp. CC219B from CCB.
OmpR domain proteins are typically part of SARP or TCS. Nearly all reported genes containing an OmpR domain positively regulate their BGCs (Guder et al., 2002; Ni et al., 2011; Li et al., 2020), and many of them are essential to produce their respective BGC. A significant portion of reported OmpR-containing proteins with activation mechanisms belongs to SARPs associated with antibiotic-producing BGCs. OmpR is predominantly utilized as part of a SARP or TCS, serving as an activator, which makes them potential tools for enhancing NP production through genetic manipulation (Krause et al., 2020). The OmpR phylogenetic tree featured two main clades, one consisting almost exclusively of SARPs, and the other branch including RRs and OCS. Within this branch, a clade of lanthipeptides was identified, comprising RR from the HisKA HK on the lanthipeptide clade. A small clade containing aminoglycosides was also detected, with one marine isolate.
Most DNA-binding proteins possess a helix-turn-helix motif, which is responsible for DNA binding in almost 95% of transcription factors (Ramos et al., 2005). In our phylogenetic analysis, we identified three major branches. One of these branches comprised TFs with the LuxR domain, previously detected in samples from all environments tested. Within this branch, only seven RRs were present, including two in isolates from the rainforest (with the potential to produce sarpeptin) and the marine environment. Additionally, this branch contained a clade with the Iron-dependent repressor, N-terminal DNA binding domain (PF01325). Another clade consisted of TFs with the previously identified TetR domain, although none of them possessed the Iron-dependent repressor. Isolates from all environments were found within this branch, including Streptomyces strains potentially producing venemycin, chloramphenicol, and 7-prenylisatin from CCB and the rainforest. The Iron-dependent repressor was commonly observed in multiple clades of the HTH phylogenetic tree, with four such clades and 27.8% of TFs featuring this domain. When considering a possible relationship between BGCs producing siderophores and the presence of the Iron-dependent repressor, we detected three siderophore BGCs (two for pyoverdine and one for yersiniabactin) from MIBiG, as well as one previously reported potential siderophore BGC (heterobactin) Rhodococcus sp. H-CA8f. However, none of these BGCs contained the Iron-dependent repressor. Some Nocardia strains from CCB possessed the Iron-dependent repressor and had the potential to produce a siderophore, although with similarities of 72% and 63% for heterobactin, and 27% for coelibactin.
5. Conclusion
In this study, we propose a phylogenetic analysis of regulatory elements linked to biosynthesis gene clusters. Our analysis allowed us to explore the diversity (based on protein domain information) of regulatory mechanisms present in curated BGCs from database. More importantly, our analysis permits to cross-correlate regulatory mechanisms of newly sequenced environmental samples in order to formulate informed hypothesis on activation of BGCs. As a proof of principle, we conducted a comprehensive genome analysis of Actinobacteria isolated from three distinct environments: the low-nutrient Cuatro Ciénegas Basin, the Calakmul Rainforest in Mexico, and the marine environments of Chile. This approach provided valuable insights into the regulatory mechanisms governing biosynthetic gene clusters (BGCs) across diverse ecological niches. Despite variations in isolation sites, our findings revealed striking similarities in the regulatory elements of similar natural products (NPs) compared to those previously documented in the MIBiG database. This innovative approach holds promise for selectively identifying and activating known NPs with analogous activity or structure, albeit with unique characteristics in environmental strains. Furthermore, it offers the potential to uncover potential elicitors for BGCs in strains suitable for NP discovery. However, it’s important to note that this method is most effective for BGCs with internal regulatory elements and may be less efficient for those relying on external activation mechanisms. This research significantly contributes to our understanding of BGC regulatory mechanisms by exploring the roles of transcription factors (TFs) and histidine kinases (HKs) in relation to NP structures and environmental contexts, offering valuable insights for NP mining endeavors.
Data availability statement
The datasets presented in this study can be found in online repositories. The names of the repository/repositories and accession number(s) can be found in the article/Supplementary material.
Author contributions
AR-S: Data curation, Formal analysis, Methodology, Writing – original draft, Writing – review and editing. LG-S: Data curation, Formal analysis, Writing – review and editing. LR-O: Data curation, Formal analysis, Writing – review and editing. ÁC: Formal analysis, Writing – review and editing. AU: Data curation, Writing – review and editing. BC: Data curation, Writing – review and editing. NS-M: Conceptualization, Data curation, Formal analysis, Investigation, Methodology, Supervision, Writing – review and editing. CL-C: Conceptualization, Data curation, Formal analysis, Funding acquisition, Investigation, Methodology, Project administration, Resources, Supervision, Validation, Visualization, Writing – original draft, Writing – review and editing.
Funding
The author(s) declare financial support was received for the research, authorship, and/or publication of this article. This work was done with the financial support of the Centro de Biotecnologia FEMSA from Tecnologico de Monterrey (grant no. 20240I-14) and UCMEXUS (no. CN-18-10).
Acknowledgments
We appreciate the support of the Centro de Biotecnologia FEMSA from Tecnologico de Monterrey Authors also thank StrainBiotech for the financial support to perform genome sequencing and genome minging. We would like to thank Dra. Susana De la Torre-Zavala from the Autonomous University of Nuevo Leon (UANL) for providing access to the Cuatro Cienegas Basin. We would also thank the Consejo Nacional de Ciencia y Tecnologia (CONACyT) for providing economic support to AR-S, LG-S, and LR-O.
Conflict of interest
The authors declare that the research was conducted in the absence of any commercial or financial relationships that could be construed as a potential conflict of interest.
Publisher’s note
All claims expressed in this article are solely those of the authors and do not necessarily represent those of their affiliated organizations, or those of the publisher, the editors and the reviewers. Any product that may be evaluated in this article, or claim that may be made by its manufacturer, is not guaranteed or endorsed by the publisher.
Supplementary material
The Supplementary Material for this article can be found online at: https://www.frontiersin.org/articles/10.3389/fmicb.2023.1290473/full#supplementary-material
Footnotes
References
Adamek, M., Alanjary, M., Sales-Ortells, H., Goodfellow, M., Bull, A., Winkler, A., et al. (2018). Comparative genomics reveals phylogenetic distribution patterns of secondary metabolites in Amycolatopsis species. BMC Genomics 19:426. doi: 10.1186/s12864-018-4809-4
Aminov, R. (2017). History of antimicrobial drug discovery: Major classes and health impact. Biochem. Pharmacol. 133, 4–19. doi: 10.1016/j.bcp.2016.10.001
Argimón, S., Abudahab, K., Goater, R., Fedosejev, A., Bhai, J., Glasner, C., et al. (2016). Microreact: visualizing and sharing data for genomic epidemiology and phylogeography. Microb. Genom. 2:e000093. doi: 10.1099/mgen.0.000093
Barka, E., Vatsa, P., Sanchez, L., Gaveau-Vaillant, N., Jacquard, C., Meier-Kolthoff, J., et al. (2016). Taxonomy, Physiology, and Natural Products of Actinobacteria. Microbiol. Mol. Biol. Rev. 80, 1–43. doi: 10.1128/MMBR.00019-15
Blin, K., Pascal Andreu, V., de Los Santos, E., Del Carratore, F., Lee, S., Medema, M., et al. (2019). The antiSMASH database version 2: a comprehensive resource on secondary metabolite biosynthetic gene clusters. Nucleic Acids Res. 47, D625–D630. doi: 10.1093/nar/gky1060
Blin, K., Shaw, S., Kloosterman, A., Charlop-Powers, Z., van Wezel, G., Medema, M., et al. (2021). antiSMASH 6.0: improving cluster detection and comparison capabilities. Nucleic Acids Res. 49, W29–W35. doi: 10.1093/nar/gkab335
Davis, J., Wattam, A., Aziz, R., Brettin, T., Butler, R., Butler, R., et al. (2020). The PATRIC Bioinformatics Resource Center: expanding data and analysis capabilities. Nucleic Acids Res. 48, D606–D612. doi: 10.1093/nar/gkz943
Diminic, J., Zucko, J., Ruzic, I., Gacesa, R., Hranueli, D., Long, P., et al. (2013). Databases of the thiotemplate modular systems (CSDB) and their in silico recombinants (r-CSDB). J. Ind. Microbiol. Biotechnol. 40, 653–659. doi: 10.1007/s10295-013-1252-z
Edgar, R. (2004). MUSCLE: multiple sequence alignment with high accuracy and high throughput. Nucleic Acids Res. 32, 1792–1797. doi: 10.1093/nar/gkh340
Gallegos-Lopez, S., Mejia-Ponce, P., Gonzalez-Salazar, L., Rodriguez-Orduña, L., Souza-Saldivar, V., and Licona-Cassani, C. (2020). Draft genome sequence of Streptomyces sp. Strain C8S0, Isolated from a highly oligotrophic sediment. Microbiol. Resour. Announc. 9, e1441–e1419. doi: 10.1128/MRA.01441-19
González-Zorn, B., and Escudero, J. (2012). Ecology of antimicrobial resistance: humans, animals, food and environment. Int. Microbiol. 15, 101–109. doi: 10.2436/20.1501.01.163
Groisman, E. (2016). Feedback Control of Two-Component Regulatory Systems. Annu. Rev. Microbiol. 70, 103–124. doi: 10.1146/annurev-micro-102215-095331
Guder, A., Schmitter, T., Wiedemann, I., Sahl, H., and Bierbaum, G. (2002). Role of the single regulator MrsR1 and the two-component system MrsR2/K2 in the regulation of mersacidin production and immunity. Appl. Environ. Microbiol. 68, 106–113. doi: 10.1128/AEM.68.1.106-113.2002
Hammami, R., Zouhir, A., Le Lay, C., Ben Hamida, J., and Fliss, I. (2010). BACTIBASE second release: a database and tool platform for bacteriocin characterization. BMC Microbiol. 10:22. doi: 10.1186/1471-2180-10-22
Ichikawa, N., Sasagawa, M., Yamamoto, M., Komaki, H., Yoshida, Y., Yamazaki, S., et al. (2013). DoBISCUIT: a database of secondary metabolite biosynthetic gene clusters. Nucleic Acids Res. 41, D408–D414. doi: 10.1093/nar/gks1177
Katz, L., and Baltz, R. (2016). Natural product discovery: past, present, and future. J. Ind. Microbiol. Biotechnol. 43, 155–176. doi: 10.1007/s10295-015-1723-5
Kautsar, S., Blin, K., Shaw, S., Navarro-Muñoz, J., Terlouw, B., van der Hooft, J., et al. (2020). MIBiG 2.0: a repository for biosynthetic gene clusters of known function. Nucleic Acids Res. 48, D454–D458. doi: 10.1093/nar/gkz882
Kautsar, S., Blin, K., Shaw, S., Weber, T., and Medema, M. (2021). BiG-FAM: the biosynthetic gene cluster families database. Nucleic Acids Res. 49, D490–D497. doi: 10.1093/nar/gkaa812
Khan, Y., White, K., and Brunger, A. (2022). The AAA+ superfamily: a review of the structural and mechanistic principles of these molecular machines. Crit. Rev. Biochem. Mol. Biol. 57, 156–187. doi: 10.1080/10409238.2021.1979460
Krause, J., Handayani, I., Blin, K., Kulik, A., and Mast, Y. (2020). Disclosing the Potential of the SARP-Type Regulator PapR2 for the Activation of Antibiotic Gene Clusters in Streptomycetes. Front. Microbiol. 11:225. doi: 10.3389/fmicb.2020.00225
Li, Y., Li, J., Tian, Z., Xu, Y., Zhang, J., Liu, W., et al. (2016). Coordinative Modulation of Chlorothricin Biosynthesis by Binding of the Glycosylated Intermediates and End Product to a Responsive Regulator ChlF1. J. Biol. Chem. 291, 5406–5417. doi: 10.1074/jbc.M115.695874
Li, Y., Zhang, J., Zheng, J., Guan, H., Liu, W., and Tan, H. (2020). Co-expression of a SARP Family Activator ChlF2 and a Type II Thioesterase ChlK Led to High Production of Chlorothricin in Streptomyces antibioticus DSM 40725. Front. Bioeng. Biotechnol. 8:1013. doi: 10.3389/fbioe.2020.01013
Lu, F., Hou, Y., Zhang, H., Chu, Y., Xia, H., and Tian, Y. (2017). Regulatory genes and their roles for improvement of antibiotic biosynthesis in Streptomyces. 3 Biotech 7:250. doi: 10.1007/s13205-017-0875-6
Martínez-Burgo, Y., Santos-Aberturas, J., Rodríguez-García, A., Barreales, E., Tormo, J., Truman, A., et al. (2019). Activation of secondary metabolite gene clusters in streptomyces clavuligerus by the pimm regulator of Streptomyces natalensis. Front. Microbiol. 10:580. doi: 10.3389/fmicb.2019.00580
McLean, T., Lo, R., Tschowri, N., Hoskisson, P., Al Bassam, M., Hutchings, M., et al. (2019). Sensing and responding to diverse extracellular signals: an updated analysis of the sensor kinases and response regulators of Streptomyces species. Microbiology 165, 929–952. doi: 10.1099/mic.0.000817
Mungan, M., Alanjary, M., Blin, K., Weber, T., Medema, M., and Ziemert, N. (2020). ARTS 2.0: feature updates and expansion of the antibiotic resistant target seeker for comparative genome mining. Nucleic Acids Res. 48, W546–W552. doi: 10.1093/nar/gkaa374
Ni, J., Teng, K., Liu, G., Qiao, C., Huan, L., and Zhong, J. (2011). Autoregulation of lantibiotic bovicin HJ50 biosynthesis by the BovK-BovR two-component signal transduction system in Streptococcus bovis HJ50. Appl. Environ. Microbiol. 77, 407–415. doi: 10.1128/AEM.01278-10
Ortet, P., De Luca, G., Whitworth, D., and Barakat, M. (2012). P2TF: a comprehensive resource for analysis of prokaryotic transcription factors. BMC Genomics 13:628. doi: 10.1186/1471-2164-13-628
Osbourn, A. (2010). Secondary metabolic gene clusters: evolutionary toolkits for chemical innovation. Trends Genet. 26, 449–457. doi: 10.1016/j.tig.2010.07.001
Otani, H., Udwary, D., and Mouncey, N. (2022). Comparative and pangenomic analysis of the genus Streptomyces. Sci. Rep. 12, 18909. doi: 10.1038/s41598-022-21731-1
Overbeek, R., Olson, R., Pusch, G., Olsen, G., Davis, J., Disz, T., et al. (2014). The SEED and the Rapid Annotation of microbial genomes using Subsystems Technology (RAST). Nucleic Acids Res. 42, D206–D214. doi: 10.1093/nar/gkt1226
Özel, B., Şimşek, Ö, Akçelik, M., and Saris, P. (2018). Innovative approaches to nisin production. Appl. Microbiol. Biotechnol. 102, 6299–6307. doi: 10.1007/s00253-018-9098-y
Palaniappan, K., Chen, I., Chu, K., Ratner, A., Seshadri, R., Kyrpides, N., et al. (2020). IMG-ABC v.5.0: an update to the IMG/Atlas of biosynthetic gene clusters knowledgebase. Nucleic Acids Res. 48, D422–D430. doi: 10.1093/nar/gkz932
Price, M., Dehal, P., and Arkin, A. (2010). FastTree 2–approximately maximum-likelihood trees for large alignments. PLoS One 5:e9490. doi: 10.1371/journal.pone.0009490
Ramos, J., Martínez-Bueno, M., Molina-Henares, A., Terán, W., Watanabe, K., Zhang, X., et al. (2005). The TetR family of transcriptional repressors. Microbiol. Mol. Biol. Rev. 69, 326–356. doi: 10.1128/MMBR.69.2.326-356.2005
Rodriguez-R, L., Gunturu, S., Harvey, W., Rosselló-Mora, R., Tiedje, J., Cole, J., et al. (2018). The Microbial Genomes Atlas (MiGA) webserver: taxonomic and gene diversity analysis of Archaea and Bacteria at the whole genome level. Nucleic Acids Res. 46, W282–W288. doi: 10.1093/nar/gky467
Romero-Rodríguez, A., Robledo-Casados, I., and Sánchez, S. (2015). An overview on transcriptional regulators in Streptomyces. Biochim. Biophys. Acta 1849, 1017–1039. doi: 10.1016/j.bbagrm.2015.06.007
Santos, C., Correia-Neves, M., Moradas-Ferreira, P., and Mendes, M. V. (2012). A walk into the LuxR regulators of Actinobacteria: phylogenomic distribution and functional diversity. PLoS One 7:e46758. doi: 10.1371/journal.pone.0046758
Snider, J., Thibault, G., and Houry, W. (2008). The AAA+ superfamily of functionally diverse proteins. Genome Biol. 9:216. doi: 10.1186/gb-2008-9-4-216
Som, N., Heine, D., Holmes, N., Knowles, F., Chandra, G., Seipke, R., et al. (2017). The MtrAB two-component system controls antibiotic production in Streptomyces coelicolor A3(2). Microbiology 163, 1415–1419. doi: 10.1099/mic.0.000524
Tidjani, A., Lorenzi, J., Toussaint, M., van Dijk, E., Naquin, D., Lespinet, O., et al. (2019). Massive gene flux drives genome diversity between sympatric streptomyces conspecifics. mBio 10, e1533–e1519. doi: 10.1128/mBio.01533-19
Tremblay, N., Hill, P., Conway, K., and Boddy, C. (2016). The use of clustermine360 for the analysis of polyketide and nonribosomal peptide biosynthetic pathways. Methods Mol. Biol. 1401, 233–252. doi: 10.1007/978-1-4939-3375-4_15
Ulrich, L., Koonin, E., and Zhulin, I. (2005). One-component systems dominate signal transduction in prokaryotes. Trends Microbiol. 13, 52–56. doi: 10.1016/j.tim.2004.12.006
Keywords: gene regulation, biosynthetic gene cluster, natural product, transcription factor, histidine kinase, two component system
Citation: Rodriguez-Sanchez AC, Gónzalez-Salazar LA, Rodriguez-Orduña L, Cumsille A, Undabarrena A, Camara B, Sélem-Mojica N and Licona-Cassani C (2023) Phylogenetic classification of natural product biosynthetic gene clusters based on regulatory mechanisms. Front. Microbiol. 14:1290473. doi: 10.3389/fmicb.2023.1290473
Received: 07 September 2023; Accepted: 23 October 2023;
Published: 08 November 2023.
Edited by:
Sylvie Lautru, Centre National de la Recherche Scientifique (CNRS), FranceReviewed by:
Margarita Díaz, University of Salamanca, SpainSébastien Rigali, University of Liège, Belgium
Copyright © 2023 Rodriguez-Sanchez, Gónzalez-Salazar, Rodriguez-Orduña, Cumsille, Undabarrena, Camara, Sélem-Mojica and Licona-Cassani. This is an open-access article distributed under the terms of the Creative Commons Attribution License (CC BY). The use, distribution or reproduction in other forums is permitted, provided the original author(s) and the copyright owner(s) are credited and that the original publication in this journal is cited, in accordance with accepted academic practice. No use, distribution or reproduction is permitted which does not comply with these terms.
*Correspondence: Cuauhtemoc Licona-Cassani, Y2xpY29uYUB0ZWMubXg=