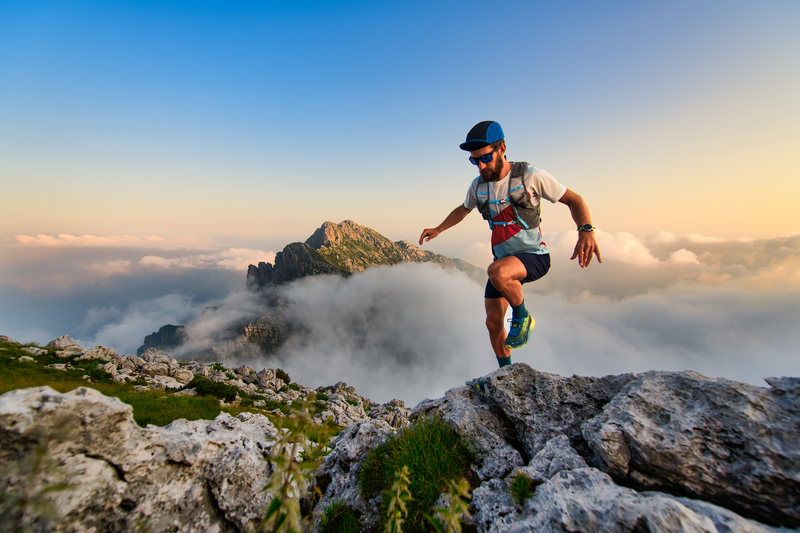
94% of researchers rate our articles as excellent or good
Learn more about the work of our research integrity team to safeguard the quality of each article we publish.
Find out more
ORIGINAL RESEARCH article
Front. Microbiol. , 23 November 2023
Sec. Infectious Agents and Disease
Volume 14 - 2023 | https://doi.org/10.3389/fmicb.2023.1276046
This article is part of the Research Topic Acute/Chronic Pathogen Infections: Roles of Resident, Probiotic, and Live Biotherapeutic Microbes in Limiting or Preventing Disease View all 26 articles
Introduction: Observational studies have reported the association between gut microbiota and the risk of lower respiratory tract infections (LRTIs). However, whether the association reflects a causal relationship remains obscure.
Methods: A bidirectional twosample Mendelian randomization (MR) analysis was conducted by assessing genome-wide association study (GWAS) summary statistics for gut microbiota taxa and five common LRTIs. MR methods including inverse-variance-weighted (IVW), MR-Egger, weighted median, simple mode, and weighted mode were used to analyze the causality. Gene pleiotropy was tested using MR-Egger regression and MR-PRESSO methods. Cochran’s Q test was used to check for heterogeneity. Leave-one-out analysis was used to assess the stability of effect sizes. Detected significant associations were validated by using an independent LRTI GWAS summary statistics dataset. An optional MR method of causal analysis using summary effect estimates (CAUSE) was further performed as a validation to avoid potential false-positive results.
Results: According to the MR-Egger estimates in forward MR analysis, a causal effect of gut Blautia on increased odds of bronchiectasis and pneumonia was suggested. MR-Egger regression pleiotropy intercept methods detected no significant horizontal pleiotropy between the instrumental variables of these associations. MR-PRESSO global test examined no potential horizontal pleiotropy. Cochran’s Q test showed that no heterogeneity biased the results. The leave-one-out sensitivity analyses suggested robust causality results. These associations with consistent effect direction were successfully replicated in IVW analysis by using the validation GWAS dataset. However, these evidence of causality did not survive after applying strict Bonferroni correction or CAUSE analysis. The reverse MR analysis failed to achieve consistent results in the effect of LRTIs on gut microbiota through comprehensive discovery and validation processes.
Discussion: This study established no strong causality between genetically predicted gut microbiome and the risk of lower respiratory tract infections. However, specific subtypes of microbial genera, such as Blautia, were identified as potential influencers and require further investigation, particularly at the species or strain levels.
Lower respiratory tract infections (LRTIs) remain a significant cause of morbidity and mortality worldwide, particularly affecting vulnerable populations such as the elderly, young children, and immunosuppressed individuals (Langelier et al., 2018). According to the Global Burden of Disease Study 2016, LRTIs were responsible for approximately 2,377,697 deaths globally in 2016, especially among people aged over 65 years (Collaborators, 2018). Despite substantial advances over the past decade, LRTIs still account for a fifth of all deaths worldwide, primarily in low- and middle-income countries. From an epidemiological point of view, definitions of LRTI mainly include bronchiectasis, pneumonia, influenza, acute bronchitis, and acute bronchiolitis (Collaborators, 2017). Treatment guidelines may vary among countries, but they generally focus on improving nutrition and hygiene, along with the distribution and usage of antimicrobial agents and vaccines (Kamata et al., 2022). Although early and appropriate antimicrobial treatment are available, many patients still succumb to LRTIs due to challenges such as drug resistance (Cazzola et al., 2017). Hence, there is a need for novel therapeutic strategies in addition to traditional antibiotics, making precision medicine for LRTIs an important approach.
In otherwise healthy individuals, the human gut is the most densely colonized organ, harboring an estimated 1014 bacteria from over 1,000 bacterial species (Bäckhed et al., 2005). Current studies have extensively explored the relationship between gastrointestinal microbiota and human diseases. Its importance in sustaining local and systemic tissue homeostasis has gained great recognition (Nenci et al., 2007; Gensollen et al., 2016). Furthermore, the metabolic by-products and ligands of gut commensal bacteria have the ability to modulate and fine-tune the development and function of the innate and adaptive immune system, which helps to protect against infections caused by diverse pathogens (Ichinohe et al., 2011; Abt et al., 2012). Studies taking advantage of germ-free mice and antibiotic-driven depletion of gut bacterial species have contributed significantly to the understanding of gut-lung axis in mediating a range of respiratory infectious diseases. For example, the disruption of gut microbiome development in infancy plays a role in increased susceptibility to pulmonary viral infections (Ichinohe et al., 2011) and the development of lung diseases including asthma and chronic obstructive pulmonary disease (Abrahamsson et al., 2014; Zeissig and Blumberg, 2014; Qu et al., 2022). Mechanically, gut microbes initiate the activation of the innate antiviral immune response via the interactions with pattern recognition receptors (ie., Toll like receptor) (Samuelson et al., 2015), and also contribute to the regulation of macrophage response and restoration of lung CD4+ and CD8+ T cells, which are crucial for improved survival in respiratory viral infections (Abt et al., 2012). Besides, imbalances in gut microbiome result in an exacerbation of cytokine-induced inflammation (Verdam et al., 2013), potentially resulting in lung morbidity. It is partially attributed to the reduced production of anti-inflammatory metabolites like short-chain fatty acids (SCFAs) (Trompette et al., 2014) and increased production of pro-inflammatory metabolites such as secondary bile acids (Duboc et al., 2013) from intestinal flora, which transported via circulation to play a role in gut-lung communication, thereby impacting respiratory health and infections. Probiotics and prebiotics have demonstrated effects in reducing the incidence of cystic fibrosis pulmonary exacerbations (Weiss et al., 2010), protecting against bacterial pneumonia (Vieira et al., 2016), and expediting recovery from respiratory viral infections (Kawahara et al., 2015; Samuelson et al., 2015). Understanding the role of gut microbiome and its influence on respiratory infections can bring further refinement to the discovery of biomarker and precision medicine for these diseases.
However, so far, it remains unclear whether there is a causal relationship between gut microbiome and LRTIs because of the confounding factors and possible reverse causation. Mendelian randomization (MR) is an emerging genetic epidemiological method that utilizes summary data from genome-wide association studies (GWASs) as instrumental variables to infer causality in exposure-outcome associations (Dan et al., 2021). It may help to identify specific causal microbe taxa to enhance the development of precision medicine in the treatment of LRTIs. Therefore, we employed a bidirectional two-sample MR analysis to investigate the causal effects of gut microbiome on the risk of five common LRTI diseases and vice versa.
The study methods were compliant with the STROBE-MR checklist (Skrivankova et al., 2021). Our analysis used publicly available GWAS summary statistics. No new data were collected, and no additional ethical approval or informed consent was required. The flowchart of the study was shown in Figure 1.
SNPs related to human 16S fecal microbiome composition were selected as instrumental variables from a large-scale multi-ethnic GWAS meta-analysis with 24 cohorts, comprising 18,340 individuals of different ethnicities and ages from the USA, Canada, Israel, South Korea, Germany, Denmark, the Netherlands, Belgium, Sweden, Finland, and the UK, most of whom had European ancestry (n = 13,266) (Kurilshikov et al., 2021). The microbial composition was profiled by targeting three distinct variable regions of the 16S rRNA gene, including V4 (10,413 samples, 13 cohorts), V3-V4 (4,211 samples, 6 cohorts), and V1-V2 (3,716 samples, 5 cohorts).
The discovery summary-level data of SNPs associated with the five common LRTI subtypes were obtained from the data of FinnGen Release 8 (released to the public on December 1, 2022),1 including bronchiectasis, acute bronchiolitis, acute bronchitis, influenza, and pneumonia. Cases were defined as participants with at least one inpatient or outpatient ICD-9/10 code as a primary diagnosis for these LRTIs. We limited LRTI data to samples of European ancestry to avoid potential bias aroused by population stratification. This GWAS consisted of 1,967 bronchiectasis cases (283,589 controls), 1,754 acute bronchiolitis cases (323,785 controls), 13,832 acute bronchitis cases (323,785 controls), 52,021 pneumonia cases (290,478 controls), and 7,580 influenza cases (286,619 controls).
The validation summary statistics of SNPs associated to the five common LRTI diseases were obtained from the GWAS reports measured in European participants from the UK Biobank by searching the GWAS catalog2 (Jiang et al., 2021), which included a total of 583 bronchiectasis cases (455,765 controls) (accession number: GCST90044075), 172 acute bronchiolitis and bronchitis cases (456,176 controls) (accession number: GCST90044069), 2,842 pneumonia cases (453,506 controls) (accession number: GCST90044067), and 248 influenza cases (456,100 controls) (accession number: GCST90044068).
We also examined the possibility of reverse causality of LRTIs on gut microbiome, using the discovery datasets of GWASs related to gut microbiome and LRTIs.
The following selection criteria were used to choose the IVs: (1) SNPs associated with each genus were collected as potential IVs using a genome-wide significance threshold of p < 1 × 10−5 for their inclusion in the current study; (2) due to the presence of strong linkage disequilibrium (LD) among selected SNPs might bias the results, SNPs were clumped within study per bacterium genus using the PLINK (version 1.961) clumping procedure to eliminate the stringent LD between included IVs (R2 < 0.01, with reference to the 1,000 Genomes Phase 3 CEU Project Panel (Abecasis et al., 2012), clumping window size = 1,000 kb) within a five megabase window; (3) to guarantee that the impact of SNPs on exposure corresponds to the same allele as the impact on the outcome, palindromic SNPs with intermediate allele frequencies were excluded; (4) when the exposure-related SNPs were not available in the outcome dataset, the proxy SNPs significantly correlated with the variants of interest were used (R2 > 0.8); (5) selected SNPs with a minor allele frequency (MAF) ≤ 0.01 were excluded.
A bidirectional two-sample MR analysis was conducted to evaluate the causal relationship between the gut microbiome and common LRTI subtypes. When only a single SNP was available to construct the IV, the ratio of coefficients method was used to obtain MR estimates with first-order weights used to generate standard errors. Where more than one SNP was available to construct the IVs for a given genus, the random effect inverse variance weighted (IVW) MR approach was used as the principal analysis to acquire an overall estimate of the causal effect. In addition, complementary methods including MR-Egger regression, weighted median, weighted model, MR-pleiotropy residual sum and outlier (MR-PRESSO), and simple model were used to examine the causal association. The MR-Egger regression is used to assess the presence of pleiotropy, which occurs when a genetic variant affects multiple traits or outcomes, violating the instrumental variable assumption of MR. It provides a robust estimate of the causal effect by accounting for pleiotropy and can detect and quantify any directional pleiotropy bias (Bowden et al., 2016b). The weighted median estimator is a robust approach to estimate the causal effect when there is heterogeneity in the causal estimates from different genetic variants. It selects the median estimate as the overall causal effect, giving more weight to instruments with smaller variances and providing valid results as long as at least 50% of the weight comes from valid instruments (Bowden et al., 2016a). Similar to the weighted median, the weighted mode estimator is also designed to handle heterogeneity. It selects the mode estimate as the causal effect, which is the most frequent estimate among valid instruments. This method is useful when there is more pronounced heterogeneity in the causal estimates (Hartwig et al., 2017). MR-PRESSO is a method used to identify and correct for outliers or genetic variants that violate the no pleiotropy assumption. It detects outliers and removes them from the analysis, allowing for a more accurate estimation of the causal effect. Additionally, MR-PRESSO provides an adjusted causal estimate after removing outliers (Verbanck et al., 2018). To account for multiple testing, a conservative Bonferroni threshold for statistical significance was set to p < 0.05 / 119 = 4 × 10−4 for the forward MR analysis and p < 0.05 / 5 = 1 × 10−2 for the reverse MR analysis.
We also employed a Bayesian posterior probabilities-based MR method namely Causal Analysis Using Summary Effect Estimates (CAUSE) (Morrison et al., 2020), as a further validation analysis for the associations replicated in both discovery dataset and validation dataset. This approach demonstrates a reduced susceptibility to false positive associations resulting from correlated and uncorrelated horizontal pleiotropy by utilizing the maximum independent SNPs to increase detection power. To include a larger number of IVs, LD pruning was performed using a threshold of r2 < 0.1 and p < 1 × 10−3 via a built-in function in the CAUSE R package, which utilized precomputed LD estimates.
To ensure the reliability of the conclusion, sensitivity analyses were performed to verify whether heterogeneity and pleiotropy within the genetic variables could bias the MR results. First, the MR-Egger regression was applied to detect and adjust for the underlying horizontal pleiotropic effects among the selected IVs through the assessment of the intercept. Second, the MR-PRESSO global test of heterogeneity was conducted to identify the underlying horizontal pleiotropy. Third, the Cochran’ IVW Q statistics were used to quantify the heterogeneity across the selected IVs. With a consideration of possible significant heterogeneity between SNPs (p < 0.05), we applied a random-effect IVW model to perform the MR analysis. Fourth, the leave-one-out sensitivity analysis was implemented by omitting each instrumental SNP in turn to identify potential heterogeneous SNPs. Fifth, considering that various confounders including smoking, pulmonary comorbidities such as chronic obstructive pulmonary disease (COPD), asthma, lung fibrosis et al. were closely associated with the incidence of LRTIs, we conservatively queried each genus-related SNP used as the instrument in the PhenoScanner V2 database to identify SNPs that were significantly associated with GWAS traits potentially confounding LRTI phenotypes or might introduce horizontal pleiotropy at risk of affecting the five LRTI subtypes independent of gut microbiome at the genome-wide significance level. Whenever the GWAS p-value of the SNP was lower than the threshold (p < 1 × 10−5), we considered it to be correlated with the confounders (Kamat et al., 2019). We assessed the effect of gut microbiome after removing those SNPs from the MR estimates to exclude potential pleiotropic effects. This MR analysis was performed based on three assumptions: (1) The IV is closely associated with the exposure. (2) The IV is not associated with any potential confounders. (3) The IV can only influence the outcome via the exposure, and not by any other ways. The CAUSE method was used to detect the false positive error due to correlated horizontal pleiotropy. The SNPs selection assumptions and MR statistical analysis workflow were shown in Figure 2.
The strength of IVs was assessed by calculating the F-statistic using the formula F = R2 × (N-1-K) / (1-R2) × K, where R2 represented the proportion of variance in the exposure explained by the genetic variants, N represented sample size, and K represented the number of instruments (Palmer et al., 2012). R2 was calculated by using the formula R2 = 2 × MAF × (1–MAF) × beta2, where beta was the effect value of the genetic variant in the exposure, and MAF was the effect allele frequency of selected SNPs (Palmer et al., 2012; Burgess et al., 2016). If the corresponding F-statistic was >10, it was considered no significant weak instrumental bias was used. The power of the MR estimates was calculated using the mRnd MR power calculator3 (Burgess et al., 2017). MR analyses were performed using R (version 4.2.3), the TwoSampleMR package 0.5.6 (Hemani et al., 2018), MR-PRESSO (version 1.0) (Verbanck et al., 2018), and CAUSE (version 1.2.0) (Morrison et al., 2020).
According to the selection criteria of IVs, a total of 1,517 SNPs associated with 119 bacterial genera were identified from the large-scale GWAS (Supplementary Table S1). After exclusion due to potential association to outcomes or outcome-related traits, unavailablility in outcome dataset (Supplementary Table S2), or palindrome, a total of 1,224 SNPs with four proxy SNPs were retained as IVs for the following forward MR analysis (Supplementary Table S3). The SNP number for bronchiectasis, acute bronchiolitis, acute bronchitis, influenza, and pneumonia in the discovery dataset was 1,220, 1,219, 1,221, 1,219, and 1,222, respectively. The main information of SNPs including effect allele, other allele, beta, SE, and p value were collected systematically for further analysis. The number of IVs associated with each bacterial genus varies from 3 to 20. The sum of F statistics for SNPs of individual bacterial genera was greater than the conventional threshold of 10, indicating that there was no significant bias from weak instrument variables (Table 1; Supplementary Table S4).
According to the five MR methods, the genetically determined gut microbiome, as a whole, had no causal relationship with LRTIs. The results of Cochran’s IVW Q test showed no significant heterogeneity of these IVs. The discovery findings were confirmed in independent validation MR analysis (Table 1). Detailed information and strengths of IVs in validation dataset were presented in Supplementary Tables S5, S6.
However, the results of MR analyses for individual gut microbial genus in discovery datasets revealed that genetically predicted relative abundance of specific genera might be causally associated with an increased or decreased risk of LRTI (Supplementary Table S7). For example, based on the IVW estimates, Bifidobacterium was negatively associated to the risk of bronchiectasis [odds ratio (OR): 0.688, 95% confidence interval (CI): 0.520–0.910, p = 0.009], but positively associated to the risk of influenza (OR: 1.225, 95% CI: 1.061–1.415, p = 0.006). While according to the MR-Egger analysis, Blautia was consistently correlated with an increased risk of bronchiectasis (OR: 2.956, 95% CI: 1.143–7.648, p = 0.049), influenza (OR: 1.856, 95% CI: 1.140–3.022, p = 0.032), and pneumonia (OR: 1.336, 95% CI: 1.036–1.724, p = 0.049). In addition, abundance of genera Erysipelatoclostridium acted as a risk factor for acute bronchiolitis (OR: 3.483, 95% CI: 1.266–9.583, p = 0.031) and influenza (OR: 1.764, 95% CI: 1.089–2.860, p = 0.038), while Oxalobacter acted as a protective factor for acute bronchiolitis (OR: 0.799, 95% CI: 0.653–0.978, p = 0.030) and bronchitis (OR: 0.913, 95% CI: 0.842–0.989, p = 0.027) (Supplementary Table S8; Supplementary Figures S1, S2).
For these identified associations, the Cochran’s Q test suggested that there was no significant heterogeneity across all these selected genetic instruments (Supplementary Table S8). The horizontal pleiotropy was evaluated by MR-Egger regression, and the results indicated that there was evidence of potential horizontal pleiotropy to skew the influence of Anaerostipes on bronchiectasis (p = 0.002), Erysipelatoclostridium on acute bronchiolitis (p = 0.030) and influenza (p = 0.041), Holdemania (p = 0.010) and Olsenellaon (p = 0.035) on acute bronchiolitis, Oscillospira on acute bronchitis (p = 0.038), and Ruminococcus_2 on pneumonia (p = 0.039) (Supplementary Table S8). The MR-Egger intercept test was sensitive to outliers and violations of INstrument Strength Independent of Direct Effect assumption, thus less efficient. Therefore, we also conducted MR-PRESSO global test, which was more robust to outliers. Nevertheless, no sign of horizontal pleiotropy was detected and no outliers were found by MR-PRESSO test (Supplementary Table S9). The leave-one-out analysis showed that no single SNP was driving these significant MR estimates, indicating that the results of the current MR analysis were robust (Supplementary Figure S3). However, after Bonferroni correction for multiple tests, none of the significant associations presented between genetically predicted gut microbiome with LRTIs.
Furthermore, to validate the causal relationship identified in the discovery sample set, summary statistics from independent LRTI GWASs were employed. The validation MR analysis solely confirmed the causal association with the same direction between genetically predicted enrichment of microbial genus Blautia with bronchiectasis (IVW, OR: 2.774, 95% CI: 1.413–5.445, p = 0.003) and pneumonia (IVW, OR: 1.386, 95% CI: 1.008–1.906, p = 0.044) (Table 2; Figure 3). Still, application of the Bonferroni correction did not yield any statistically significant differences in validation. To further confirm the validated casual links between Blautia and LRTIs, CAUSE analysis was then performed. However, it indicated that the sharing model was better than the causal model, without providing consistent significant causality (Supplementary Table S10).
Table 2. MR results of significant causal effect of gut microbiome on LRTI risk in forwad analysis (p < 0.05).
Figure 3. Validated casual links between specific gut microbial genus and LRTIs in forward MR analyses.
We further examined the causal effect of LRTI diseases on gut microbiome by reverse MR analyses. The detailed information and predictive power of selected IVs were presented in Supplementary Tables S11–S13. The conventional MR methods resulted in multiple causal relationships between LRTI and gut microbiome (Supplementary Table S14). Specifically, according to the results of bidirectional MR analyses based on the discovery dataset, we found a bidirectional causal association between Escherichia_Shigella (Forward IVW, OR: 0.851, 95% CI: 0.737–0.982, p = 0.028; Reverse IVW, OR: 0.851, 95% CI: 0.763–0.950, p = 0.004) and acute bronchitis, as well as Eubacterium_fissicatena_group (Forward IVW, OR: 0.897, 95% CI: 0.816–0.987, p = 0.025; Reverse IVW, OR: 0.793, 95% CI: 0.647–0.971, p = 0.025) and acute bronchitis (Supplementary Tables S8, S15).
The Bonferroni correction was also performed in reverse MR analysis to account for five exposures, where p < 0.01 (0.05/5) was defined as a statistically significant difference. The causal effect of Bronchiectasis on Corprobacter (Reverse MR IVW, p = 0.008) and Ruminiclostridium_6 (Reverse MR IVW, p = 0.007), acute bronchiolitis on Corprobacter (Reverse MR IVW, p = 0.006) and Eggerthella (Reverse MR IVW, p = 0.003), acute bronchitis on Escherichia_Shigella (Reverse MR IVW, p = 0.004), Oscillibacter (Reverse MR IVW, p = 0.002), and Veillonella (Reverse MR IVW, p = 0.001), as well as pneumonia on Haemophilus (Reverse MR IVW, p = 0.003) was still significant after Bonferroni correction. There was no evidence of heterogeneity between IV estimates with IVW methods from individual SNPs and no pleiotropy effect for these detected associations. No pleiotropic outliers were detected according to the MR-PRESSO tests (Supplementary Table S16). However, as there were not enough IVs used in the MR to undertake a sensitivity analysis for the causal effect of acute bronchiolitis on most microbial genera, the results should be interpreted with caution. To verify these causalities, we also conducted validation MR analysis by using independent LRTI GWAS data. Nevertheless, none of the associations were replicated in the validation dataset, indicating a poor causal effect of LRTIs on the gut microbiome.
Colonization of the intestine with commensal bacteria is known to play a major role in the maintenance of the integrity of lung tissues against foreign infections. Intestinal flora has a powerful direct and indirect regulatory effect on the human immune system by increasing the number of immune cells, producing SCFAs and immunoglobulins, enhancing oral tolerance, and controlling inflammation (Samuelson et al., 2015). Consequently, an altered gut microbiome is always associated with various ensuing diseases including respiratory diseases. In this study, using the summary statistics of gut microbiota from the largest GWAS report and the summary statistics of LRTIs from the FinnGen consortium R8 release data and UK Biobank, we performed a bidirectional two-sample MR analysis to refer the causal association between gut microbiota and five common phenotypes of LRTI, which might be helpful to shed light on the impact of gut microbiota on airway immunity and the host’s ability to defend against respiratory infections. Additionally, our findings may inspire the development of precision medicine for treating these scenarios.
Through a comprehensive discovery and validation approach, this study suggested Blautia as a potential risk factor for an increased risk of bronchiectasis and pneumonia. Despite the causality did not surpass the strict Bonferroni correction threshold and was not confirmed in CAUSE analysis, it provided suggestive evidence of a potential causal effect between genus Blautia and LRTI frequency and might stimulate further specialized studies to gain insights into its impact on respiratory health. As a dominant genus of anaerobic bacteria in the feces and intestines of mammals, the probiotic characteristics of Blautia and its protective role in various host physiological dysfunctions have been reported, such as obesity, diabetes, cancer, and inflammatory bowel diseases. Blautia contributes to maintaining environmental balance in the intestine, preventing inflammation by upregulating intestinal regulatory T cells, and producing SCFAs (Kim et al., 2014). Its ability to produce bacteriocin, one of the common secondary metabolites that possess antibacterial activity against pathogenic microorganisms such as Listeria monocytogenes, Clostridium perfringens, and Escherichia coli (Martinez et al., 2013), also gives Blautia the potential to inhibit the colonization of pathogenic bacteria in the intestine (Liu et al., 2021). Our results did not align with previous findings regarding the protective effect of Blautia in human diseases. However, higher abundance of Blautia was also reported in the fecal microbiota of patients with irritable bowel syndrome, ulcerative colitis, functional gastrointestinal symptoms (Rajilić-Stojanović et al., 2011; Nishino et al., 2018; Ohlsson, 2022), and breast cancer (Luu et al., 2017), suggested its association to the local or systemic inflammation. Indeed, Blautia, especially Blautia coccoides, activates the secretion of inflammatory cytokines such as tumor necrosis factor α (TNF-α) to an even greater extent than lipopolysaccharide (Tuovinen et al., 2013). TNF-α is a potent protective cytokine that contributing to anti-viral and anti-bacterial responses during the early phase of infection, however, excessive production causes heightened lung immunopathology and inflammation, particularly in the late phase of infection. Besides, in patients with rheumatoid arthritis, increased relative abundance of gut genus Blautia was related with lower levels of T cells, B cells, CD4+ T cells, and Tregs (Li et al., 2021). The findings suggest that Blautia species act as culprits in the pathogenesis of infectious diseases potentially due to their pro-inflammatory and anti-immune properties. Mediterranean diet (MD) is plant-based and consistently considered to be benefit on human health (Barber et al., 2023). Studies demonstrated that administration of MD reduced Blautia within gut microbiota (Merra et al., 2020; Zhu et al., 2020). Evidence for the effects of dietary fiber within MD showed a direct suppressive effect on Clostridium difficile infection. As previous study has indicated a positive association of Blautia with key inflammatory cytokines such as TNF-α, the authors hypothesized that MD improves the inflammatory milieu and infection through modulation of gut microbiota, at least in part (Merra et al., 2020; Zhu et al., 2020). The conflicting conclusions may be related to the genomic difference within the genus, as there are 12 independent Blautia species with a total of 195 genome assemblies, nevertheless, most studies focused on the genus level and did not delve into investigations at species or even strain-levels (Liu et al., 2021). Therefore, drawing general conclusions at the genus level may lead to partial understandings and misinterpretations because different species of Blautia may exert different effects on human health. For example, Blautia coccoides was reported to be positively associated to the level of cytokines including TNF-α (Tuovinen et al., 2013), while Blautia luti and B. wexlerae in the gut microbiota of obese children was negatively related to those proinflammatory cytokines and chemokines (Benítez-Páez et al., 2020).
Although not consistently validated, our results implied that specific genera of gut commensal microbiota compositions might have protective effects against respiratory infections, highlighting their potential use as medications in the context of airway infections (Wolvers et al., 2010; Ozen et al., 2015). Based on the results from the discovery dataset, an increased abundance of Oxalobacter was consistently related to a lower risk of acute bronchiolitis and bronchitis, which are primarily caused by viral infections, particularly respiratory syncytial virus (RSV) (Kinkade and Long, 2016; Joseph and Edwards, 2019). Oxalobacter is a Gram-negative bacterium that degrades oxalate in the gut to decrease urinary oxalate excretion. Its probiotics preparation has been commercially available as a biotherapeutic agent in the management of calcium oxalate renal stones (Hiremath and Viswanathan, 2022). Oxalobacter was identified to be associated with intestinal virus infection (Gozalbo-Rovira et al., 2021) and infectious urinary stone (Bruyere et al., 2008). Our analyses gave a new clue that use of Oxalobacter strains might show beneficial effects on the host immunity and/or against pathogens in respiratory system. Bifidobacterium is a genus of Gram-positive, anaerobic bacteria that are commonly found in the human gut microbiome. These bacteria are known for their immunomodulatory properties and have been shown to have a beneficial impact on human health (Hidalgo-Cantabrana et al., 2017). In our study, Bifidobacterium was suggested as a protective factor for the risk of bronchiectasis, which is associated to bacteria-related recurrent respiratory tract infections (RRTIs) (Amati et al., 2019). Studies have revealed that RRTI patients suffer from intestinal flora imbalance (Ozen et al., 2015; Li et al., 2019), manifested as a significant reduction in the number of Bifidobacteria (Peng et al., 2016; Li et al., 2019). Restoring Bifidobacteria with oral Bifidobaeterium tetravaccine tablets (Live) effectively maintained the balance of intestinal micro-ecology and reduced average annual frequency of acute respiratory tract bacterial infection and use of antibiotics (Li et al., 2019). Thus, Bifidobacterium may be viewed as potential next-generation probiotic candidates in the treatment of bacterial lung infections. Actually, studies evaluating the use of Bifidobacterium as probiotics have already demonstrated their effect in the control of respiratory viral infections, such as COVID-19 (Taufer and Rampelotto, 2023) and H7N9 (Zhang et al., 2020). The potential mechanisms of action underlying the protective effects of Bifidobacterium, drawing from current knowledge, may involve the stimulation on immune system, reduction of inflammation, competitive advantage with pathogenic microbes, and maintenance of gut barrier function (Taufer and Rampelotto, 2023). A group of SCFAs-producing bacteria including Parabacteroides (Ahmed et al., 2019), Anaerotruncus (Yan et al., 2019), and Lachnospiraceae_NC2004_group (Egerton et al., 2022) were also implied to be protective in LRTIs by our results. SCFAs, mostly acetic acid, propionic acid, and butyric acid, are metabolites produced after gut microbial fermentation of dietary fiber. Accumulated evidence supported that SCFAs not only maintain local and systemic immune homeostasis but also boost host immunity to pathogens in a range of airway inflammatory conditions (Antunes et al., 2023; Dang et al., 2023), through sophisticated modulations on the maturation, accumulation, and function of immune cells, activating the transmembrane G protein-coupled receptors, and inhibiting histone deacetylases, et al. (Tan et al., 2014).
Notably, the association between gut microbiome and LRTIs found in the observational studies may be influenced by reverse causation. To address it, we performed a reverse MR analysis to investigate the causation in the opposite direction. Although there were some initial indications of causal effects of LRTIs on the gut microbiome in the discovery sample set, no notable genetically predicted associations were observed in the validation dataset. This suggested that reverse causation is unlikely to explain the findings in forward MR analysis.
This study has several strengths. To the best of our knowledge, this is the first MR study to infer the causal relationship between the gut microbiota and LRTIs. Genetic variants used to represent the gut microbiota were sourced from the largest available GWAS analysis, ensuring the strength of instruments for MR approach. Horizontal pleiotropy was detected and excluded by using the MR-PRESSO and MR-Egger regression intercept term tests. Furthermore, we conducted a comprehensive validation process by using an independent sample data and the CAUSE method to facilitate robust causal inferences. Our study somehow deepens the understanding of the gut microbiome on human health and highlights the microbiome-related agents as potential precision therapeutics to ensure enhanced resistance toward respiratory infections.
There are several limitations that should be considered while interpreting the results of this study. First, a standard MR method assumed a linear relationship between exposure and outcome, so the non-linear association and threshold effect between gut microbiota and LRTIs could not be detected. Second, we only explored the causal links between gut microbiota and LRTIs at the taxonomic level of genus, thus their associations at the species level could not be revealed. Third, although most participants in the GWAS meta-analysis for gut microbiota data were of European descent and the outcome GWAS data were restricted only from European subjects, the interference from population stratification might still exist. Moreover, the extrapolation of the current findings to other ethnic groups was limited. Future MR studies on this topic should be considered in non-European populations to confirm the results. Fourth, although we measured LD among all selected SNPs using northern Europeans from Utah samples from the 1,000 Genomes Project, we could not exclude the possibility that our results might be affected by unmeasured confounders. Fifth, by selecting representative diseases for LRTIs, we aimed to capture a range of lower respiratory tract infections of clinical importance. The ambiguous and broad phenotype definition for LRTIs might induce the risk of non-differential mismatch between specific genera and individual diseases because high heterogeneities in LRTIs due to varied etiologies, pathogens, disease stages, clinical and radiology features, and physiology/lung functions might significantly influence the results. For example, the inclusion of influenza with a blurred boundary between upper and lower respiratory tract effects potentially introduced a potential limitation in the causal inferences drawn from our analysis. Sixth, since the data sources did not include individual level data, we failed to report the number of individuals at each stage of the study and the reasons why individuals were excluded from further study. This limitation might influence the adjustment of validity and generalisability of results by readers. Finally, the genetic instruments used in the MR analyses still might have pleiotropic effects, which could produce spurious findings. To limit this risk, we conducted multiple sensitivity analyses to provide statistical evidence of bias from pleiotropy or genetic confounding. But still, since the results failed to meet the stringent threshold in Bonferroni correction and CAUSE method, it is important to interpret our findings with caution, particularly when making specific causal inferences related to individual diseases within the LRTI group.
In summary, this study does not provide robust evidence for a causal relationship between genetically predicted gut microbiome genera and common phenotypes of lower respiratory tract infections. However, specific genera of gut commensal microbiota, such as Blautia, Oxalobacter, Bifidobacterium, and several SCFA-producing bacteria, are suggested to be potential indicators for respiratory infection susceptibility. Further specialized investigations, particularly studies focusing on the species or strain levels, are needed to gain more insights into their impact on lung health and to unlock the potential use of gut microbiota-based immune-regulatory therapies in respiratory infectious diseases.
The datasets presented in this study can be found in online repositories. The names of the repository/repositories and accession number(s) can be found in the article/supplementary material.
WL: Conceptualization, Funding acquisition, Investigation, Supervision, Writing – original draft, Writing – review & editing. XW: Formal analysis, Methodology, Writing – original draft. RF: Formal analysis, Methodology, Writing – original draft. CZ: Formal analysis, Methodology, Writing – original draft. JL: Data curation, Formal analysis, Methodology, Writing – original draft. XZ: Data curation, Formal analysis, Writing – original draft. XL: Data curation, Methodology, Software, Writing – original draft. MY: Formal analysis, Software, Writing – original draft. JM: Data curation, Methodology, Writing – original draft. BM: Formal analysis, Supervision, Writing – review & editing. HJ: Funding acquisition, Supervision, Writing – review & editing.
The author(s) declare financial support was received for the research, authorship, and/or publication of this article. The study was funded by the Science & Technology Department of Sichuan Province (grant Nos. 2021YFS0271 and 2023YFH0072). The sponsors had no role in the study design; the collection, analysis, or interpretation of the data; or the decision to submit the article for publication.
The authors express their gratitude to the participants and investigators of the FinnGen study. The authors also appreciate Kurilshikov A., Jiang L. and their colleagues for releasing the GWAS summary statistics.
The authors declare that the research was conducted in the absence of any commercial or financial relationships that could be construed as a potential conflict of interest.
All claims expressed in this article are solely those of the authors and do not necessarily represent those of their affiliated organizations, or those of the publisher, the editors and the reviewers. Any product that may be evaluated in this article, or claim that may be made by its manufacturer, is not guaranteed or endorsed by the publisher.
The Supplementary material for this article can be found online at: https://www.frontiersin.org/articles/10.3389/fmicb.2023.1276046/full#supplementary-material
Abecasis, G. R., Auton, A., Brooks, L. D., DePristo, M. A., Durbin, R. M., Handsaker, R. E., et al. (2012). An integrated map of genetic variation from 1,092 human genomes. Nature 491, 56–65. doi: 10.1038/nature11632
Abrahamsson, T. R., Jakobsson, H. E., Andersson, A. F., Björkstén, B., Engstrand, L., and Jenmalm, M. C. (2014). Low gut microbiota diversity in early infancy precedes asthma at school age. Clin. Exp. Allergy 44, 842–850. doi: 10.1111/cea.12253
Abt, M. C., Osborne, L. C., Monticelli, L. A., Doering, T. A., Alenghat, T., Sonnenberg, G. F., et al. (2012). Commensal bacteria calibrate the activation threshold of innate antiviral immunity. Immunity 37, 158–170. doi: 10.1016/j.immuni.2012.04.011
Ahmed, S., Busetti, A., Fotiadou, P., Vincy Jose, N., Reid, S., Georgieva, M., et al. (2019). In vitro characterization of gut microbiota-derived bacterial strains with neuroprotective properties. Front. Cell. Neurosci. 13:402. doi: 10.3389/fncel.2019.00402
Amati, F., Simonetta, E., Gramegna, A., Tarsia, P., Contarini, M., Blasi, F., et al. (2019). The biology of pulmonary exacerbations in bronchiectasis. Eur. Respir. Rev. 28:190055. doi: 10.1183/16000617.0055-2019
Antunes, K. H., Singanayagam, A., Williams, L., Faiez, T. S., Farias, A., Jackson, M. M., et al. (2023). Airway-delivered short-chain fatty acid acetate boosts antiviral immunity during rhinovirus infection. J. Allergy Clin. Immunol. 151, 447–457.e5. doi: 10.1016/j.jaci.2022.09.026
Bäckhed, F., Ley, R. E., Sonnenburg, J. L., Peterson, D. A., and Gordon, J. I. (2005). Host-bacterial mutualism in the human intestine. Science 307, 1915–1920. doi: 10.1126/science.1104816
Barber, T. M., Kabisch, S., Pfeiffer, A. F. H., and Weickert, M. O. (2023). The effects of the Mediterranean diet on health and gut microbiota. Nutrients 15:2150. doi: 10.3390/nu15092150
Benítez-Páez, A., Gómez Del Pugar, E. M., López-Almela, I., Moya-Pérez, Á., Codoñer-Franch, P., and Sanz, Y. (2020). Depletion of Blautia species in the microbiota of obese children relates to intestinal inflammation and metabolic phenotype worsening. mSystems 5, e00857–e00819. doi: 10.1128/mSystems.00857-19
Bowden, J., Davey Smith, G., Haycock, P. C., and Burgess, S. (2016a). Consistent estimation in Mendelian randomization with some invalid instruments using a weighted median estimator. Genet. Epidemiol. 40, 304–314. doi: 10.1002/gepi.21965
Bowden, J., Del Greco, M. F., Minelli, C., Davey Smith, G., Sheehan, N. A., and Thompson, J. R. (2016b). Assessing the suitability of summary data for two-sample Mendelian randomization analyses using MR-Egger regression: the role of the I2 statistic. Int. J. Epidemiol. 45, dyw220–dyw1974. doi: 10.1093/ije/dyw220
Bruyere, F., Traxer, O., Saussine, C., and Lechevallier, E. (2008). Infection and urinary lithiasis. Prog. Urol. 18, 1015–1020. doi: 10.1016/j.purol.2008.09.015
Burgess, S., Dudbridge, F., and Thompson, S. G. (2016). Combining information on multiple instrumental variables in Mendelian randomization: comparison of allele score and summarized data methods. Stat. Med. 35, 1880–1906. doi: 10.1002/sim.6835
Burgess, S., Small, D. S., and Thompson, S. G. (2017). A review of instrumental variable estimators for Mendelian randomization. Stat. Methods Med. Res. 26, 2333–2355. doi: 10.1177/0962280215597579
Cazzola, M., Rogliani, P., Aliberti, S., Blasi, F., and Matera, M. G. (2017). An update on the pharmacotherapeutic management of lower respiratory tract infections. Expert. Opin. Pharmacother. 18, 973–988. doi: 10.1080/14656566.2017.1328497
Collaborators, G. L. (2017). Estimates of the global, regional, and national morbidity, mortality, and aetiologies of lower respiratory tract infections in 195 countries: a systematic analysis for the global burden of disease study 2015. Lancet Infect. Dis. 17, 1133–1161. doi: 10.1016/s1473-3099(17)30396-1
Collaborators, G. L. R. I. (2018). Estimates of the global, regional, and national morbidity, mortality, and aetiologies of lower respiratory infections in 195 countries, 1990-2016: a systematic analysis for the global burden of disease study 2016. Lancet Infect. Dis. 18, 1191–1210. doi: 10.1016/s1473-3099(18)30310-4
Dan, Y. L., Wang, P., Cheng, Z., Wu, Q., Wang, X. R., Wang, D. G., et al. (2021). Circulating adiponectin levels and systemic lupus erythematosus: a two-sample Mendelian randomization study. Rheumatology (Oxford) 60, 940–946. doi: 10.1093/rheumatology/keaa506
Dang, A. T., Begka, C., Pattaroni, C., Caley, L. R., Floto, R. A., Peckham, D. G., et al. (2023). Butyrate regulates neutrophil homeostasis and impairs early antimicrobial activity in the lung. Mucosal Immunol. 16, 476–485. doi: 10.1016/j.mucimm.2023.05.005
Duboc, H., Rajca, S., Rainteau, D., Benarous, D., Maubert, M. A., Quervain, E., et al. (2013). Connecting dysbiosis, bile-acid dysmetabolism and gut inflammation in inflammatory bowel diseases. Gut 62, 531–539. doi: 10.1136/gutjnl-2012-302578
Egerton, S., Donoso, F., Fitzgerald, P., Gite, S., Fouhy, F., Whooley, J., et al. (2022). Investigating the potential of fish oil as a nutraceutical in an animal model of early life stress. Nutr. Neurosci. 25, 356–378. doi: 10.1080/1028415x.2020.1753322
Gensollen, T., Iyer, S. S., Kasper, D. L., and Blumberg, R. S. (2016). How colonization by microbiota in early life shapes the immune system. Science 352, 539–544. doi: 10.1126/science.aad9378
Gozalbo-Rovira, R., Rubio-Del-Campo, A., Santiso-Bellón, C., Vila-Vicent, S., Buesa, J., Delgado, S., et al. (2021). Interaction of intestinal bacteria with human rotavirus during infection in children. Int. J. Mol. Sci. 22:1010. doi: 10.3390/ijms22031010
Hartwig, F. P., Davey Smith, G., and Bowden, J. (2017). Robust inference in summary data Mendelian randomization via the zero modal pleiotropy assumption. Int. J. Epidemiol. 46, 1985–1998. doi: 10.1093/ije/dyx102
Hemani, G., Zheng, J., Elsworth, B., Wade, K. H., Haberland, V., Baird, D., et al. (2018). The MR-base platform supports systematic causal inference across the human phenome. elife 7:e34408. doi: 10.7554/eLife.34408
Hidalgo-Cantabrana, C., Delgado, S., Ruiz, L., Ruas-Madiedo, P., Sánchez, B., and Margolles, A. (2017). Bifidobacteria and their health-promoting effects. Microbiol Spectr 5. doi: 10.1128/microbiolspec.BAD-0010-2016
Hiremath, S., and Viswanathan, P. (2022). Oxalobacter formigenes: a new hope as a live biotherapeutic agent in the management of calcium oxalate renal stones. Anaerobe 75:102572. doi: 10.1016/j.anaerobe.2022.102572
Ichinohe, T., Pang, I. K., Kumamoto, Y., Peaper, D. R., Ho, J. H., Murray, T. S., et al. (2011). Microbiota regulates immune defense against respiratory tract influenza a virus infection. Proc. Natl. Acad. Sci. U. S. A. 108, 5354–5359. doi: 10.1073/pnas.1019378108
Jiang, L., Zheng, Z., Fang, H., and Yang, J. (2021). A generalized linear mixed model association tool for biobank-scale data. Nat. Genet. 53, 1616–1621. doi: 10.1038/s41588-021-00954-4
Joseph, M. M., and Edwards, A. (2019). Acute bronchiolitis: assessment and management in the emergency department. Pediatr. Emerg. Med. Pract. 16, 1–24.
Kamat, M. A., Blackshaw, J. A., Young, R., Surendran, P., Burgess, S., Danesh, J., et al. (2019). PhenoScanner V2: an expanded tool for searching human genotype-phenotype associations. Bioinformatics 35, 4851–4853. doi: 10.1093/bioinformatics/btz469
Kamata, K., Thein, K. N., Di Ja, L., Win, N. C., Win, S. M. K., Suzuki, Y., et al. (2022). Clinical manifestations and outcome of viral acute lower respiratory infection in hospitalised children in Myanmar. BMC Infect. Dis. 22:350. doi: 10.1186/s12879-022-07342-1
Kawahara, T., Takahashi, T., Oishi, K., Tanaka, H., Masuda, M., Takahashi, S., et al. (2015). Consecutive oral administration of Bifidobacterium longum MM-2 improves the defense system against influenza virus infection by enhancing natural killer cell activity in a murine model. Microbiol. Immunol. 59, 1–12. doi: 10.1111/1348-0421.12210
Kim, C. H., Park, J., and Kim, M. (2014). Gut microbiota-derived short-chain fatty acids, T cells, and inflammation. Immune Netw 14, 277–288. doi: 10.4110/in.2014.14.6.277
Kurilshikov, A., Medina-Gomez, C., Bacigalupe, R., Radjabzadeh, D., Wang, J., Demirkan, A., et al. (2021). Large-scale association analyses identify host factors influencing human gut microbiome composition. Nat. Genet. 53, 156–165. doi: 10.1038/s41588-020-00763-1
Langelier, C., Kalantar, K. L., Moazed, F., Wilson, M. R., Crawford, E. D., Deiss, T., et al. (2018). Integrating host response and unbiased microbe detection for lower respiratory tract infection diagnosis in critically ill adults. Proc. Natl. Acad. Sci. U. S. A. 115, E12353–e12362. doi: 10.1073/pnas.1809700115
Li, K. L., Wang, B. Z., Li, Z. P., Li, Y. L., and Liang, J. J. (2019). Alterations of intestinal flora and the effects of probiotics in children with recurrent respiratory tract infection. World J. Pediatr. 15, 255–261. doi: 10.1007/s12519-019-00248-0
Li, Y., Zhang, S. X., Yin, X. F., Zhang, M. X., Qiao, J., Xin, X. H., et al. (2021). The gut microbiota and its relevance to peripheral lymphocyte subpopulations and cytokines in patients with rheumatoid arthritis. J Immunol Res 2021:6665563. doi: 10.1155/2021/6665563
Liu, X., Mao, B., Gu, J., Wu, J., Cui, S., Wang, G., et al. (2021). Blautia-a new functional genus with potential probiotic properties? Gut Microbes 13, 1–21. doi: 10.1080/19490976.2021.1875796
Luu, T. H., Michel, C., Bard, J. M., Dravet, F., Nazih, H., and Bobin-Dubigeon, C. (2017). Intestinal proportion of Blautia sp. is associated with clinical stage and histoprognostic grade in patients with early-stage breast cancer. Nutr. Cancer 69, 267–275. doi: 10.1080/01635581.2017.1263750
Martinez, F. A., Balciunas, E. M., Converti, A., Cotter, P. D., and de Souza Oliveira, R. P. (2013). Bacteriocin production by Bifidobacterium spp. A review. Biotechnol. Adv. 31, 482–488. doi: 10.1016/j.biotechadv.2013.01.010
Merra, G., Noce, A., Marrone, G., Cintoni, M., Tarsitano, M. G., Capacci, A., et al. (2020). Influence of Mediterranean diet on human gut microbiota. Nutrients 13:7. doi: 10.3390/nu13010007
Morrison, J., Knoblauch, N., Marcus, J. H., Stephens, M., and He, X. (2020). Mendelian randomization accounting for correlated and uncorrelated pleiotropic effects using genome-wide summary statistics. Nat. Genet. 52, 740–747. doi: 10.1038/s41588-020-0631-4
Nenci, A., Becker, C., Wullaert, A., Gareus, R., van Loo, G., Danese, S., et al. (2007). Epithelial NEMO links innate immunity to chronic intestinal inflammation. Nature 446, 557–561. doi: 10.1038/nature05698
Nishino, K., Nishida, A., Inoue, R., Kawada, Y., Ohno, M., Sakai, S., et al. (2018). Analysis of endoscopic brush samples identified mucosa-associated dysbiosis in inflammatory bowel disease. J. Gastroenterol. 53, 95–106. doi: 10.1007/s00535-017-1384-4
Ohlsson, B. (2022). Functional bowel symptoms in the general population (review). Mol. Med. Rep. 26:226. doi: 10.3892/mmr.2022.12742
Ozen, M., Kocabas Sandal, G., and Dinleyici, E. C. (2015). Probiotics for the prevention of pediatric upper respiratory tract infections: a systematic review. Expert. Opin. Biol. Ther. 15, 9–20. doi: 10.1517/14712598.2015.980233
Palmer, T. M., Lawlor, D. A., Harbord, R. M., Sheehan, N. A., Tobias, J. H., Timpson, N. J., et al. (2012). Using multiple genetic variants as instrumental variables for modifiable risk factors. Stat. Methods Med. Res. 21, 223–242. doi: 10.1177/0962280210394459
Peng, S., Du, T. H., and Zhang, M. (2016). Changes in gut microbiota and serum D-lactate level and correlation analysis in children with recurrent pneumonia. Zhongguo Dang Dai Er Ke Za Zhi 18, 113–116. doi: 10.7499/j.issn.1008-8830.2016.02.004
Qu, L., Cheng, Q., Wang, Y., Mu, H., and Zhang, Y. (2022). COPD and gut-lung axis: how microbiota and host inflammasome influence COPD and related therapeutics. Front. Microbiol. 13:868086. doi: 10.3389/fmicb.2022.868086
Rajilić-Stojanović, M., Biagi, E., Heilig, H. G., Kajander, K., Kekkonen, R. A., Tims, S., et al. (2011). Global and deep molecular analysis of microbiota signatures in fecal samples from patients with irritable bowel syndrome. Gastroenterology 141, 1792–1801. doi: 10.1053/j.gastro.2011.07.043
Samuelson, D. R., Welsh, D. A., and Shellito, J. E. (2015). Regulation of lung immunity and host defense by the intestinal microbiota. Front. Microbiol. 6:1085. doi: 10.3389/fmicb.2015.01085
Skrivankova, V. W., Richmond, R. C., Woolf, B. A. R., Yarmolinsky, J., Davies, N. M., Swanson, S. A., et al. (2021). Strengthening the reporting of observational studies in epidemiology using Mendelian randomization: the STROBE-MR statement. JAMA 326, 1614–1621. doi: 10.1001/jama.2021.18236
Tan, J., McKenzie, C., Potamitis, M., Thorburn, A. N., Mackay, C. R., and Macia, L. (2014). The role of short-chain fatty acids in health and disease. Adv. Immunol. 121, 91–119. doi: 10.1016/b978-0-12-800100-4.00003-9
Taufer, C. R., and Rampelotto, P. H. (2023). The role of Bifidobacterium in COVID-19: a systematic review. Life (Basel) 13:1847. doi: 10.3390/life13091847
Trompette, A., Gollwitzer, E. S., Yadava, K., Sichelstiel, A. K., Sprenger, N., Ngom-Bru, C., et al. (2014). Gut microbiota metabolism of dietary fiber influences allergic airway disease and hematopoiesis. Nat. Med. 20, 159–166. doi: 10.1038/nm.3444
Tuovinen, E., Keto, J., Nikkilä, J., Mättö, J., and Lähteenmäki, K. (2013). Cytokine response of human mononuclear cells induced by intestinal Clostridium species. Anaerobe 19, 70–76. doi: 10.1016/j.anaerobe.2012.11.002
Verbanck, M., Chen, C. Y., Neale, B., and Do, R. (2018). Detection of widespread horizontal pleiotropy in causal relationships inferred from Mendelian randomization between complex traits and diseases. Nat. Genet. 50, 693–698. doi: 10.1038/s41588-018-0099-7
Verdam, F. J., Fuentes, S., de Jonge, C., Zoetendal, E. G., Erbil, R., Greve, J. W., et al. (2013). Human intestinal microbiota composition is associated with local and systemic inflammation in obesity. Obesity (Silver Spring) 21, E607–E615. doi: 10.1002/oby.20466
Vieira, A. T., Rocha, V. M., Tavares, L., Garcia, C. C., Teixeira, M. M., Oliveira, S. C., et al. (2016). Control of Klebsiella pneumoniae pulmonary infection and immunomodulation by oral treatment with the commensal probiotic Bifidobacterium longum 5(1A). Microbes Infect. 18, 180–189. doi: 10.1016/j.micinf.2015.10.008
Weiss, B., Bujanover, Y., Yahav, Y., Vilozni, D., Fireman, E., and Efrati, O. (2010). Probiotic supplementation affects pulmonary exacerbations in patients with cystic fibrosis: a pilot study. Pediatr. Pulmonol. 45, 536–540. doi: 10.1002/ppul.21138
Wolvers, D., Antoine, J. M., Myllyluoma, E., Schrezenmeir, J., Szajewska, H., and Rijkers, G. T. (2010). Guidance for substantiating the evidence for beneficial effects of probiotics: prevention and management of infections by probiotics. J. Nutr. 140, 698S–712S. doi: 10.3945/jn.109.113753
Yan, F., Li, N., Shi, J., Li, H., Yue, Y., Jiao, W., et al. (2019). Lactobacillus acidophilus alleviates type 2 diabetes by regulating hepatic glucose, lipid metabolism and gut microbiota in mice. Food Funct. 10, 5804–5815. doi: 10.1039/c9fo01062a
Zeissig, S., and Blumberg, R. S. (2014). Life at the beginning: perturbation of the microbiota by antibiotics in early life and its role in health and disease. Nat. Immunol. 15, 307–310. doi: 10.1038/ni.2847
Zhang, Q., Hu, J., Feng, J. W., Hu, X. T., Wang, T., Gong, W. X., et al. (2020). Influenza infection elicits an expansion of gut population of endogenous Bifidobacterium animalis which protects mice against infection. Genome Biol. 21:99. doi: 10.1186/s13059-020-02007-1
Zhu, L., Sha, L., Li, K., Wang, Z., Wang, T., Li, Y., et al. (2020). Dietary flaxseed oil rich in omega-3 suppresses severity of type 2 diabetes mellitus via anti-inflammation and modulating gut microbiota in rats. Lipids Health Dis. 19:20. doi: 10.1186/s12944-019-1167-4
Keywords: gut microbiota, lower respiratory tract infections, causal relationship, Mendelian randomization, Blautia genus
Citation: Liu W, Wang X, Feng R, Zhao C, Luo J, Zhang X, Liu X, Yang M, Min J, Mao B and Jiang H (2023) Gut microbiota and risk of lower respiratory tract infections: a bidirectional two-sample Mendelian randomization study. Front. Microbiol. 14:1276046. doi: 10.3389/fmicb.2023.1276046
Received: 11 August 2023; Accepted: 01 November 2023;
Published: 23 November 2023.
Edited by:
George Grant, University of Aberdeen, United KingdomReviewed by:
Mingxuan Cai, City University of Hong Kong, Hong Kong SAR, ChinaCopyright © 2023 Liu, Wang, Feng, Zhao, Luo, Zhang, Liu, Yang, Min, Mao and Jiang. This is an open-access article distributed under the terms of the Creative Commons Attribution License (CC BY). The use, distribution or reproduction in other forums is permitted, provided the original author(s) and the copyright owner(s) are credited and that the original publication in this journal is cited, in accordance with accepted academic practice. No use, distribution or reproduction is permitted which does not comply with these terms.
*Correspondence: Hongli Jiang, aG9uZ2xpX2ppYW5nQHNjdS5lZHUuY24=; Wei Liu, bGl1d2VpMDkyOUB3Y2hzY3UuY24=
†These authors have contributed equally to this work and share first authorship
Disclaimer: All claims expressed in this article are solely those of the authors and do not necessarily represent those of their affiliated organizations, or those of the publisher, the editors and the reviewers. Any product that may be evaluated in this article or claim that may be made by its manufacturer is not guaranteed or endorsed by the publisher.
Research integrity at Frontiers
Learn more about the work of our research integrity team to safeguard the quality of each article we publish.