- 1Laboratory for Pathogenesis of Clinical Drug Resistance and Persistence, San Diego State University, San Diego, CA, United States
- 2Department of Global Public Health, Karolinska Institute, Stockholm, Sweden
- 3Division of Pulmonary, Critical Care, and Sleep Medicine, University of California, San Diego, San Diego, CA, United States
Background: Rifampicin (RIF) is a key first-line drug used to treat tuberculosis, a primarily pulmonary disease caused by Mycobacterium tuberculosis. RIF resistance is caused by mutations in rpoB, at the cost of slower growth and reduced transcription efficiency. Antibiotic resistance to RIF is prevalent despite this fitness cost. Compensatory mutations in rpoABC genes have been shown to alleviate the fitness cost of rpoB:S450L, explaining how RIF resistant strains harbor this mutation can spread so rapidly. Unfortunately, the full set of RIF compensatory mutations is still unknown, particularly those compensating for rarer RIF resistance mutations.
Objectives: We performed an association study on a globally representative set of 4,309 whole genome sequenced clinical M. tuberculosis isolates to identify novel putative compensatory mutations, determine the prevalence of known and previously reported putative compensatory mutations, and determine which RIF resistance markers associate with these compensatory mutations.
Results and conclusions: Of the 1,079 RIF resistant isolates, 638 carried previously reported putative and high-probability compensatory mutations. Our strict criteria identified 46 additional mutations in rpoABC for which no strong prior evidence of their compensatory role exists. Of these, 35 have previously been reported. As such, our independent corroboration adds to the mounting evidence that these 35 also carry a compensatory role. The remaining 11 are novel putative compensatory markers, reported here for the first time. Six of these 11 novel putative compensatory mutations had two or more mutation events. Most compensatory mutations appear to be specifically compensating for the fitness loss due to rpoB:S450L. However, an outbreak of 22 closely related isolates each carried three rpoB mutations, the rare RIFR markers D435G and L452P and the putative compensatory mutation I1106T. This suggests compensation may require specific combinations of rpoABC mutations. Here, we report only mutations that met our very strict criteria. It is highly likely that many additional rpoABC mutations compensate for rare resistance-causing mutations and therefore did not carry the statistical power to be reported here. These findings aid in the identification of RIF resistant M. tuberculosis strains with restored fitness, which pose a greater risk of causing resistant outbreaks.
Introduction
Rifampicin (RIF) is an important first-line drug used to treat tuberculosis (TB), a pulmonary disease caused by Mycobacterium tuberculosis (World Health Organization, 2022). TB is a global pandemic, with an estimated 10 million cases and causing 1.6 million deaths in 2021 (World Health Organization, 2022). Unfortunately, RIF resistance (RIFR) is prevalent. An estimated 450,000 TB cases were RIFR in 2021, including 3.6% of new TB cases and 18% of previously treated TB cases (World Health Organization, 2022). RIFR and multidrug resistant (MDR) TB require 6 to 20 months of treatment with second-line drugs (World Health Organization, 2022).
RIFR in M. tuberculosis is principally due to point mutations in the RIF resistance-determining region (RRDR) (Telenti et al., 1993; Miller et al., 1994; Ramaswamy and Musser, 1998), an 81-bp region (codons 426–452 in M. tuberculosis, codons 507–533 in E. coli) of the gene rpoB (Jin and Gross, 1988), though two rpoB mutations outside the RRDR have also been shown to cause RIF resistance (World Health Organization, 2021a). The rpoB gene encodes the β-subunit of RNA polymerase (Jin and Gross, 1988). Mutations in the RRDR typically confer resistance by a change in the three-dimensional protein structure of the β-subunit, disrupting the RIF binding site (Nusrath Unissa et al., 2016). The strong association between mutations in the RRDR and RIF resistance has led to the development of molecular tests and WHO recommended diagnostic platforms, including GeneXpert MTB/RIF, Truenat (Penn-Nicholson et al., 2021), the line probe assays GenoType MTBDRplus VER 1 and 2 (Hain Lifescience, Germany), the Genoscholar NTM + MDRTB detection kit 2 (Nipro, Japan), and others (Saiki et al., 1989; Raja et al., 2005; World Health Organization, 2021b).
However, mutations in the RRDR have a fitness cost, slowing M. tuberculosis growth in vitro (Mariam et al., 2004; Gagneux et al., 2006) and in macrophage (Mariam et al., 2004). Despite the fitness cost, RIFR strains continue to emerge, spread, and cause outbreaks (World Health Organization, 2020). This discrepancy may be due to compensatory mutations. Gagneux et al. observed that different M. tuberculosis strains with the same rpoB mutations had different fitness costs, and suggested the fitness costs were reduced in some strains by compensating mutations (Gagneux et al., 2006). Later, Gagneux and Comas et al. identified 12 high-probability compensatory mutations (HCMs) in rpoA and rpoC, which encode the and subunits of RNA polymerase (Comas et al., 2012). These 12 HCMs were carried by polyphyletic and exclusively RIFR RRDR-variant M. tuberculosis isolates, suggesting these HCMs were selected for in RIFR isolates (Comas et al., 2012). Meanwhile in Salmonella enterica, several mutations in rpoA and rpoC have also been confirmed by mutagenesis to restore wild type growth in vitro to rpoB mutants (Brandis et al., 2012).
Compensatory mutations have been shown to increase the growth rate of RIFR strains (Brandis et al., 2012; Comas et al., 2012), restore efficiency of transcription (Stefan et al., 2018), prevent reversion to wildtype(Brandis et al., 2012), and associate with increased transmission(Comas et al., 2012; de Vos et al., 2013; Li et al., 2016; Merker et al., 2018; Gygli et al., 2021). Though one contradicting study found no association between putative compensatory mutations and transmission cluster size among MDR-TB strains (Liu et al., 2018). Once the transmissibility question is fully settled, compensatory mutations might serve as markers warning which RIFR strains are more likely to cause outbreaks. However, a key obstacle to this utility is uncertainty over which rpoABC mutations compensate, and which RIFR markers they compensate for.
In this work, we sought to expand the set of putative compensatory mutations and determine which specific RIFR markers they associate with. We investigated the sequences of rpoABC genes in 4309 clinical M. tuberculosis isolates, of which 1,079 were RIFR. This work identified 18 novel putative compensatory mutations. Additionally, both novel and previously reported putative compensatory mutations were found to be associated with the specific RIFR marker rpoB:S450L, suggesting compensatory effects may be specific to particular rpoB mutations. These findings can help determine which RIFR strains are compensated, which will both aid future studies in determining the role of compensation in transmission and eventually may serve as markers warning which resistant strains are most prone to causing outbreaks.
Materials and methods
Data availability statement
All supplemental tables are available at https://doi.org/10.5281/zenodo.7324837.
Sample selection
We analyzed a total of 4,309 whole genome sequences. Of these 314 were sequenced on Pacific Bioscience’s (PacBio) Real-time Sequencer (RS) and RS II platforms (Bioproject: PRJNA353873). These isolates originated from Hinduja National Hospital (PDHNH) in Mumbai, India; the Phthisiopneumology Institute (PPI) in Chisinau, Moldova; the Tropical Disease Foundation (TDF) in Manila, Philippines; the National Health Laboratory Service of South Africa (NHLS) in Johannesburg, South Africa.
We also downloaded 3,995 whole genome sequences from NCBI’s Sequence Read Archive (SRA) database using SRA Toolkit’s fastqdump (Leinonen et al., 2011) (Bioprojects: PRJEB2221, PRJEB5162, PRJEB6276, PRJEB7281, PRJEB7727, PRJEB9680, PRJNA282721, PRJEB2138). All downloaded raw reads were sequenced on Illumina platforms. These genomes were isolated from patients originating from the UK, Sierra Leon, South Africa, Germany, Uzbekistan (Walker et al., 2015), and Russia (Casali et al., 2012, 2014; Chernyaeva et al., 2014).
Drug susceptibility testing
The RIF susceptibility testing for the PacBio and Illumina sequenced isolates were described in Casali et al. (2012, 2014), Chernyaeva et al. (2014), Rodwell et al. (2014), Garfein et al. (2015), and Walker et al. (2015), respectively. Briefly, all samples were tested on the BACTEC MGIT960 platform and a rifampicin (RIF) critical concentration of 1 μg/mL. DST on all isolates was performed prior to the 2021 change in the recommended critical concentration, and thus used the higher previous critical concentration (Köser et al., 2021).
Whole genome sequencing
The DNA sequencing protocol for the PacBio RS and RSII platforms was described previously (Torres et al., 2015). The sequencing protocol for genomes sequenced on Illumina Genome Analyzer, MiSeq, or HiSeq platforms were described previously (Casali et al., 2012, 2014; Chernyaeva et al., 2014; Walker et al., 2015).
Alignment and variant calling
For 268 isolates sequenced on the PacBio RSI platform, raw reads were aligned to M. tuberculosis H37Rv reference strain (Genbank accession number NC_000962.3) utilizing SMRT Analysis’ BLASR with default parameters (v1.3) (Chaisson and Tesler, 2012). For variant calling, a custom software, PBHoover (Ramirez-Busby et al., 2018) (manuscript submitted), corrected aligned reads and called variants based on a maximum likelihood criterion. PBHoover determines the threshold number of supporting reads for each variant based on the sequencing depth, the type of mutation, and the per base per read for the specific mutation type and sequencing platform (Ramirez-Busby et al., 2018). The software was validated by comparing PBHoover variant calls with PacBio RSI sequencing data in 348 isolates to published variant calls made from targeted Sanger sequencing of the same isolates, showing 99.95% concordance between them (801,516/801,930 calls were concordant) (Ramirez-Busby et al., 2018).
For 46 isolates sequenced on the PacBio RSII platform and passing assembly quality control, whole genomes were assembled with HGAP as described previously (Modlin et al., 2020). The assembled genomes were reference quality passing stringent quality controls, including consensus polishing and circulurization (Modlin et al., 2020). The assembled genomes were aligned to H37Rv and variant called using dnadiff (v1.3) from the mummer suite (Kurtz et al., 2004). Variants were converted to VCF format using a custom script, mummer-snps2vcf.1
All Illumina short-read data were processed as follows: trimmomatic (v0.36) (Bolger et al., 2014) trimmed adapters from raw reads; bowtie2 (v2.2.4) (Langmead and Salzberg, 2012) aligned reads to the M. tuberculosis H37Rv reference sequence (Genbank accession NC_000962.3); SAMtools (v1.3.1) (Li et al., 2009) sorted, filtered out reads with a mapping quality of less than 20, and created an mpileup file for each isolate; VarScan2 (v2.3) (Koboldt et al., 2012) called and filtered variants with a minimum quality of 20, a minimum depth of 10, and strand filter set to false.
For two Illumina sequenced isolates carrying HCMs and not carrying variant rpoB:S450L, LoFreq (Wilm et al., 2012) was used to check for potential subpopulations with that variant by calculating the fraction reads supporting rpoB:S450L as a minor variant. These two isolates were not considered to carry rpoB:S450L in subsequent analysis, though one of the two carried a potential subpopulation with that variant and was so noted.
VCF formatted files were further annotated with Variant Effect Predictor (VEP) (v87) (McLaren et al., 2016) to determine the consequence of each variant.
Lineage determination
Lineage for all isolates was determined using a SNP barcode created and implemented by Freschi et al. (2021) in the fast-lineage-caller.
Phylogeny
4,501 isolates were used to construct a phylogenetic tree, including the 4,309 isolates used in the main analysis. The tree includes the reference strain H37Rv and the outgroup Mycobacterium canetti (NC_01995.1). Variants were called and filtered using previously described methods (Modlin et al., 2021). RAxML version 8.2(Stamatakis, 2014) generated the maximum likelihood phylogenetic tree with a general time reversible model and 100 bootstrap replicates. The tree was visualized with the Interactive Tree of Life (Letunic and Bork, 2016) (iToL).
Identifying putative compensatory mutations
We identified putative compensatory mutations in rpoA, rpoB, and rpoC using the following criteria: (i) the mutation must be carried by at least one RIFR isolate lacking an HCM (Comas et al., 2012); (ii) the mutation must not be carried by any RIFS isolate; (iii) the mutation must be carried by at least two isolates. Four frameshift causing single base deletions were also excluded. To determine whether these mutations were potentially more than bystanders, we then determined whether each novel putative compensatory mutation was carried by an apparently polyphyletic group of isolates using the phylogenetic tree and ETE Toolkit v3.1.1 (Huerta-Cepas et al., 2016). To account for branch ambiguity, isolates on nearby branches were collapsed to count the number of mutation events. These mutation event counts were then used in a Fisher’s exact test of the association between each novel putative compensatory mutation and resistant isolates.
HCMs, previously reported putative compensatory, and novel compensatory mutations were mapped to the rpoA, rpoB, and rpoC genes with known domains using Lollipop (Jay and Brouwer, 2016), with manual adjustments in Inkscape to colors and the heights of mutation labels.
Results
Concordance of RIFR genetic markers with phenotypic DST
We analyzed 4,309 whole genome sequences and their phenotypic drug susceptibility testing (DST) results. In total 993 RIFR and 78 RIFS isolates (Table 1; Supplementary Table S1) harbored either a nonsynonymous mutation in the RRDR or one of the confirmed RIFR conferring mutations outside the RRDR (rpoB:V170F or rpoB:I491F) (World Health Organization, 2021a). Genotype-predicted RIF DST resulted in 92.0% sensitivity and 97.6% specificity. This was slightly lower than the sensitivity (93.8%, confidence interval 93.3–94.2%) and specificity (98.2%, confidence interval 98.0–98.3%) of prediction in a previous study of 27,063 isolates (World Health Organization, 2021a). The lower specificity was likely due to the higher critical concentration use prior to 2021 (Köser et al., 2021). The high critical concentration has been shown to inconsistently classify RIFR for isolates carrying any of six “borderline” mutations in the RRDR that confer lower level RIFR (Supplementary Table S2) (Jo et al., 2017; Köser et al., 2021; World Health Organization, 2021a).
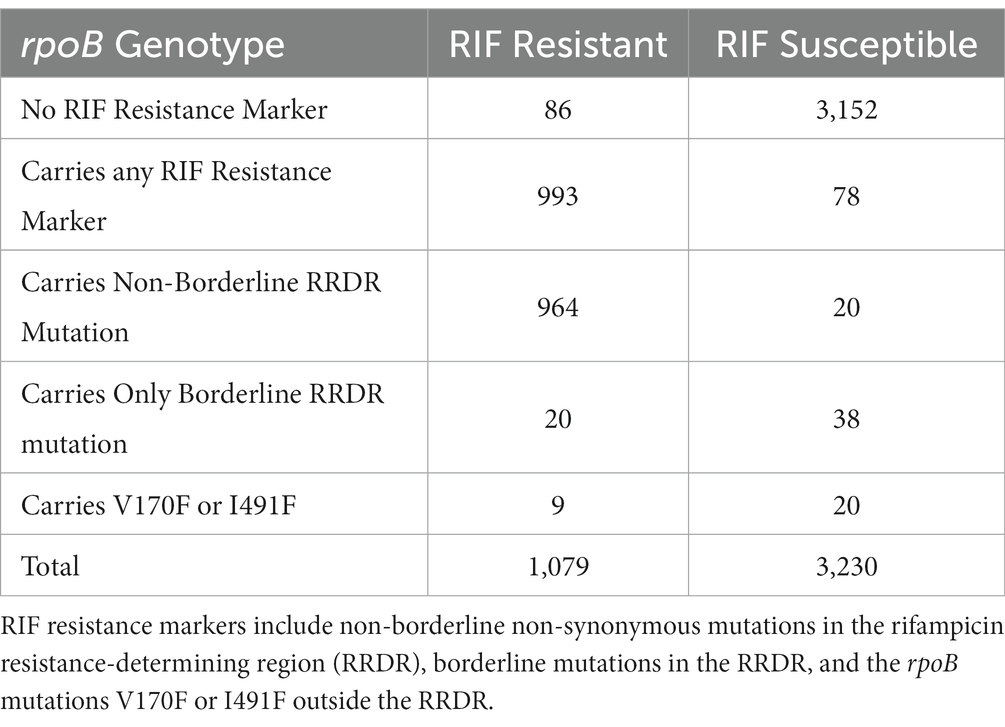
Table 1. Number of rifampicin (RIF) resistant and RIF susceptible isolates carrying or not carrying RIF resistance markers.
The lower sensitivity may be from sampling bias favoring selection of discordant isolates for whole genome sequencing. The 314 SMRT sequenced isolates had been selected to maximize phenotypic and genotypic diversity from the specimen repositories (Hillery et al., 2014). There were 86 discordant isolates (Table 1), with RIFR DST results despite lacking any non-synonymous RRDR mutation (and lacking rpoB:V170F or rpoB:I491F). These discordant isolates could be the result of DST error, a resistant subpopulation, an alternative mechanism of resistance, or a false negative genotype call due to lack of coverage. The mean read depth in rpoB was 22.1 in RSI sequenced isolates and 132.4 in Illumina sequenced isolates (Supplementary Table S3). To find potential alternative genetic mechanisms of resistance, the 86 discordant RIFR isolates were queried for variants in rpoA, rpoB, and rpoC. Among 42 of the 86 discordant RIFR isolates were 17 unique mutations, of which 7 were exclusive to RIFR isolates (Supplementary Table S4). In the remaining 44 discordant RIFR isolates there were no mutations in rpoA, rpoB, or rpoC. The alternative resistance mechanism in these isolates may through altered gene expression, rather then genotype. Multidrug resistance has previously been observed through altered expression of the ABC efflux pump (Wang et al., 2013). The regulator of this efflux pump, RaaS, may even be under epigenetic regulation, a non-genetic source of diverse gene expression (Modlin et al., 2020).
High-probability compensatory mutations associated with the common RIFR marker rpoB:S450L
Comas et al. previously identified 12 HCMs in rpoA and rpoC that likely compensated for the fitness cost of RRDR mutations in vitro (Comas et al., 2012). We searched for these HCMs in the 4,309 isolates (Table 2). In total 217 isolates carried an HCMs, including 20.0% (216/1079) of RIFR isolates. Only one isolate with an HCM was RIFS. The RIFS isolate carried the common RIFR conferring RRDR mutation rpoB:S450L and the HCM rpoC:V483G (Supplementary Table S1). The RIFS DST result in this isolate may have been due to the high pre-2021 critical concentration used, however it was more likely a laboratory mix-up, as the isolate carried known resistance markers for 5 drugs (rpoB:S450L, katG:S315T, eis:c-12 t, and gyrA:D94N) despite pan susceptible DST results.
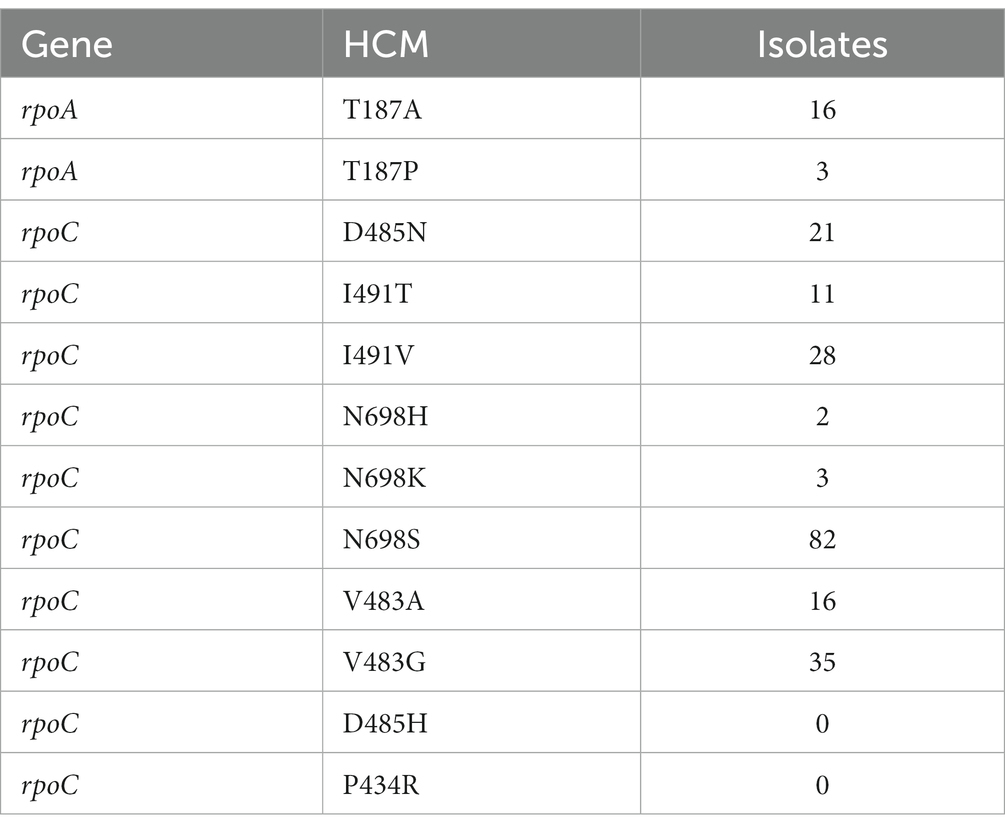
Table 2. Number of isolates carrying each of the 12 previously identified (Comas et al., 2012) high-probability compensatory mutations (HCMs) in rpoA and rpoC.
As reported previously (Song et al., 2014; Borrell and Trauner, 2017; Gygli et al., 2017; Wang et al., 2020; Ma et al., 2021), compensatory mutations were associated with the prevalent RIFR marker rpoB:S450L. Isolates carrying rpoB:S450L were 48.8 times more likely to carry HCMs than isolates carrying other RIFR markers (Table 3, odds ratio = 48.8, Fisher’s exact test p = 7.37e-28). Only two isolates carried an HCM and lacked rpoB:S450L. One such isolate carried the non-borderline RRDR mutation rpoB:Q432P and the HCM rpoC:V483G (Supplementary Table S1). The other isolate carried the RIFR marker rpoB:V170F and carried the HCM rpoA:T187P (Supplementary Table S1). This isolate also potentially included a subpopulation carrying rpoB:S450L. The rpoB:S450L variant was supported in the isolate by 7 of the 78 reads mapped to this locus. However, it is uncertain whether these sequencing reads was the result of a genuine subpopulation or sequencing error.
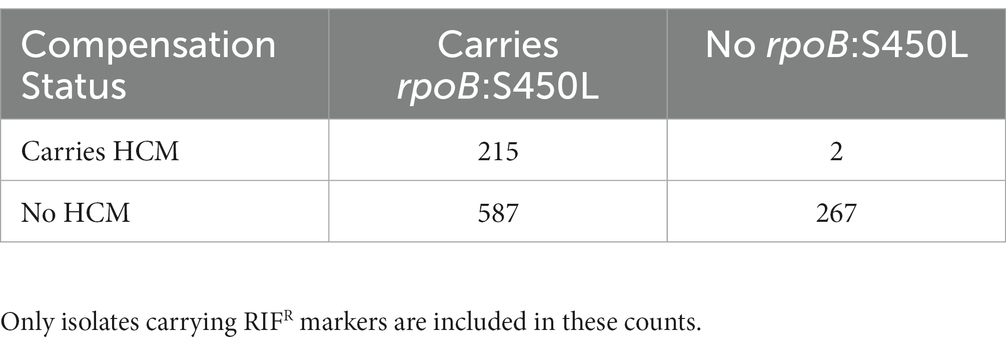
Table 3. Number of isolates carrying previously identified (Comas et al., 2012) high-probability compensatory mutations (HCMs) and the common RIFR conferring mutation rpoB:S450L.
Previously reported putative compensatory mutations
We then queried for 175 previously reported putative compensatory mutations from their study and others (Casali et al., 2012, 2014; Comas et al., 2012; de Vos et al., 2013; Song et al., 2014; Li et al., 2016; Liu et al., 2018; Ma et al., 2021). Of these 175 previously reported mutations, we observed 83 mutations across 560 of the 4,309 isolates. In total 555 RIFR and 5 RIFS isolates each carried at least one of these previously reported mutations (Supplementary Table S5). Over half the RIFR isolates (59.1%, 638/1079) carried HCMs or previously reported putative compensatory mutations.
Novel putative compensatory mutations
We then searched for novel putative compensatory mutations in rpoABC with the following criteria: (i) the mutation must be carried by at least one RIFR isolate lacking an HCM (Comas et al., 2012); (ii) the mutation must not be carried by any RIFS isolate; (iii) the mutation must be carried by at least two isolates. We then determined whether each novel putative compensatory mutation was carried by an apparent polyphyletic group of isolates, using a phylogenetic tree (Figure 1). These criteria were developed based on the initial criteria set by Comas et al. (2012) to discover candidate variants, later built upon in Liu et al. (2018), and here modified to use a phylogenetic tree to define relationships among the isolates rather than SNP distance.
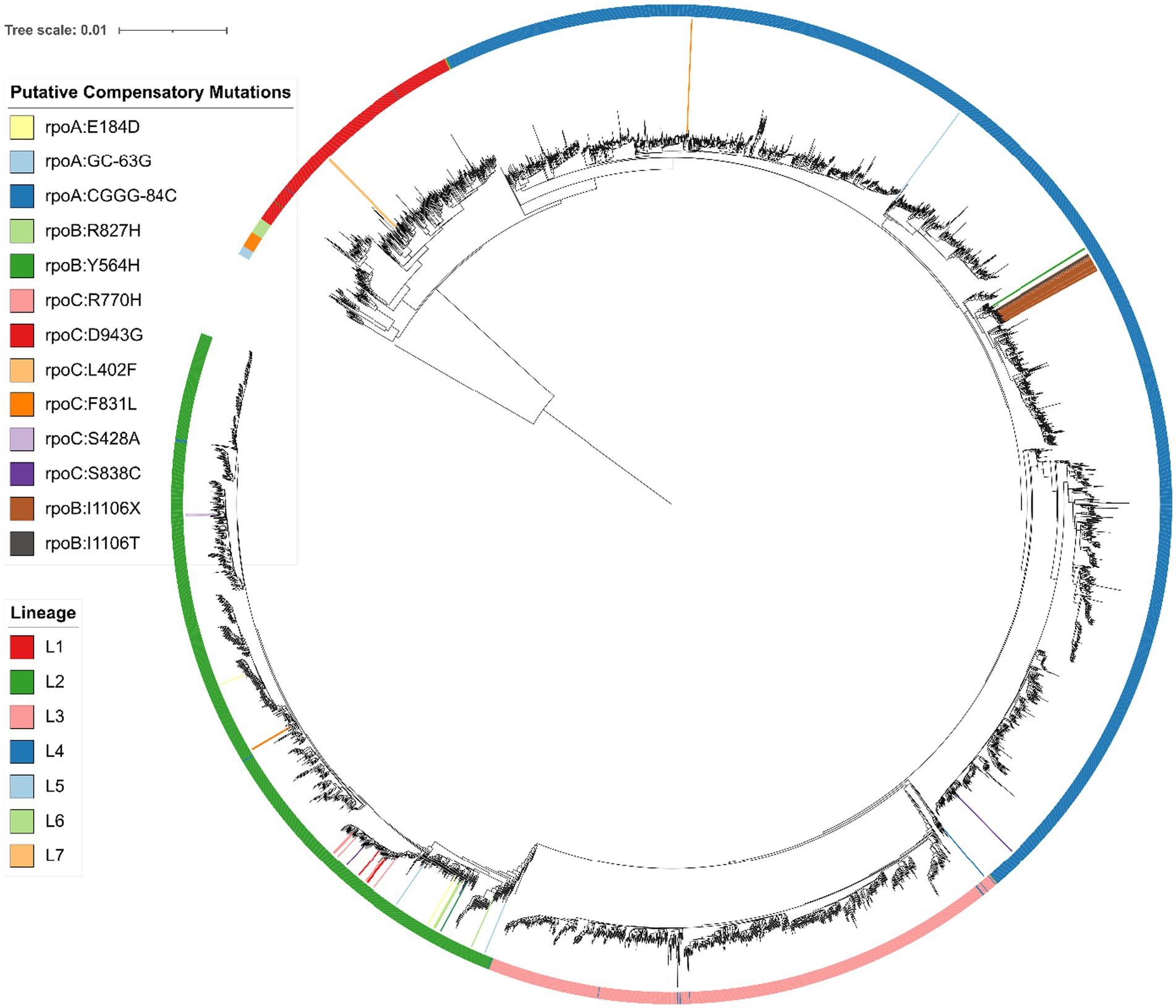
Figure 1. Phylogenetic SNP tree of 4,501 Mycobacterium tuberculosis isolates, with Mycobacterium canettii as an outgroup, created from variable regions using RAxML (Stamatakis, 2014) and visualized with iTOL (Letunic and Bork, 2016). Branches with a bootstrap support of at least 75/100 are marked with a blue circle. The colored outer ring indicates the lineage of each isolate, as determined using a SNP barcode created and implemented by Freschi et al. (2021) in the fast-lineage-caller. The inner colors indicate the presence of each novel putative compensatory mutation, or the putative compensatory mutation rpoB:I1106T (grey) or apparent frameshift rpoB:I1106X (brown). The smallest monophyletic group containing the 22 isolates with rpoB:I1106T/X includes 34 isolates and has 100/100 bootstrap support. All 34 isolates in this group were collected from the National Health Laboratory Service of South Africa in Johannesburg, South Africa. Suggesting the mutation is inherited from a single mutation event. The most frequent novel putative compensatory mutation, rpoC:R770H (pink) is also likely a single mutation event. The smallest monophyletic group that contains these 8 isolates includes 103 isolates and only has 40/100 bootstrap support, with similar ambiguity among the branches within the group. Meanwhile six of the other novel putative compensatory mutations are carried by distant isolates on the phylogenetic tree, indicating two or more mutation events.
These filters identified 11 novel putative compensatory mutations in 37 isolates, of which 10 mutations were apparently polyphyletic (Table 4). The most frequent novel putative compensatory mutation was rpoC:R770H, in eight isolates belonging to the Beijing sublineage. The eight isolates grouped closely together on the tree but did not form a monophyletic group (Figure 1), possibly because of branch ambiguity (the smallest monophyletic group containing them had 103 isolates and only 40/100 bootstrap support). These eight isolates all carried the RIFR marker rpoB:S450L. The eight isolates may represent a drug resistant outbreak enabled by compensation from rpoC:R770H, or may be a mere bystander spread by the outbreak. To account for this branch ambiguity in the tree, isolates on nearby branches were then collapsed together to count the number of mutation events for each novel putative compensatory mutation (Table 4; Figure 1). Six of the novel putative compensatory mutations each had two or more mutation events.
Together 62.1% (670/1079) of RIFR isolates carried either an HCM, a previously reported putative compensatory mutation, or a novel putative compensatory mutation. Most of the isolates with novel mutations carried rpoB:S450L (Table 5). Just as with HCMs and as reported previously (Song et al., 2014; Borrell and Trauner, 2017; Gygli et al., 2017; Wang et al., 2020; Ma et al., 2021), compensatory mutations associated rpoB:S450L. Including novel mutations, isolates carrying rpoB:S450L were 18.4 times more likely to carry an HCM or putative compensatory mutation than isolates carrying other RIFR markers (Supplementary Table S7, odds ratio = 18.4, Fisher’s exact test p = 1.18e-74).
This method also independently corroborated 35 previously reported (Casali et al., 2012, 2014; Comas et al., 2012; de Vos et al., 2013; Song et al., 2014; Li et al., 2016; Liu et al., 2018; Ma et al., 2021) putative compensatory mutations (Supplementary Table S6). One such independently identified putative compensatory mutation, rpoB:I480V, was recently confirmed by mutagenesis and competitive fitness assays to compensate in M. smegmatis in vitro (Ma et al., 2021). These independently identified putative compensatory mutations identified by this method supports the reproducibility and validity of the method.
One noteworthy independently identified putative compensatory mutation was rpoB:I1106T. This mutation was previously reported in two extremely drug resistant (XDR) isolates from South Africa from a suspected outbreak, each with the pair of RIFR markers rpoB:D435G and rpoB:L452P (Song et al., 2014). We also observed these three mutations together in two isolates from South Africa, possibly from the same outbreak as the previous study. There was also evidence of these three mutations in 20 more closely related isolates from South Africa, further corroborating that these three mutations were carried by an outbreak. These 20 closely related isolates had the variant call rpoB:I1106X (Figure 1). This suggests that rpoB:I1106X may have been a systematically miss-called variant indicating the same underlying mutation as rpoB:I1106T. At the nucleotide level, the SNP rpoB:I1106T (T3317C) extended a homopolymer (from ATCCCG to ACCCCG). The 20 isolates with rpoB:I1106X were SMRT sequenced with P4C2 chemistry and variant called with PBHoover (Ramirez-Busby et al., 2018), while the two isolates with rpoB:I1106T were SMRT sequenced with P6C4 chemistry and variant called with mummer (Kurtz et al., 2004) after de novo assembly. The PacBio RS platform with P4C2 chemistry has a significant error bias towards single base insertions and deletions, and homopolymers only exacerbate this error bias(Ross et al., 2013).
The 22 isolates with rpoB:I1106T/X were all RIFR and each carried two RRDR mutations at the codons 452 and 435 (rpoB:D435G or rpoB:D435X and rpoB:L452P or rpoB:L452X), the same RIFR markers reported in the two isolates previously reported (Song et al., 2014) with rpoB:I1106T. These rare RRDR mutations were carried in only 33 isolates. Isolates carrying rpoB:I1106T/X were at least 663 times more likely to carry either rpoB:D435G/X or rpoB:L452P/X than isolates without rpoB:I1106T/X (Supplementary Table S8, odds ratio 95% confidence interval: 663 to ∞, value of p = 1.71e-44). The rare RIFR markers rpoB:D435G and rpoB:L452P may have been able to cause the resistant outbreak despite a high fitness cost because of the previously reported (Song et al., 2014) putative compensatory mutation rpoB:I1106T.
HCMs and putative compensatory mutations in rpoA were exclusively in the RNA polymerase Rpb3/Rpb11 dimerization domain (Figure 2A). In rpoC the HCMs and putative compensatory mutations primarily clustered in Domain 2 (Figure 2C). In rpoB, there was a higher concentration of putative compensatory mutations in domain 3 (Figure 2B).
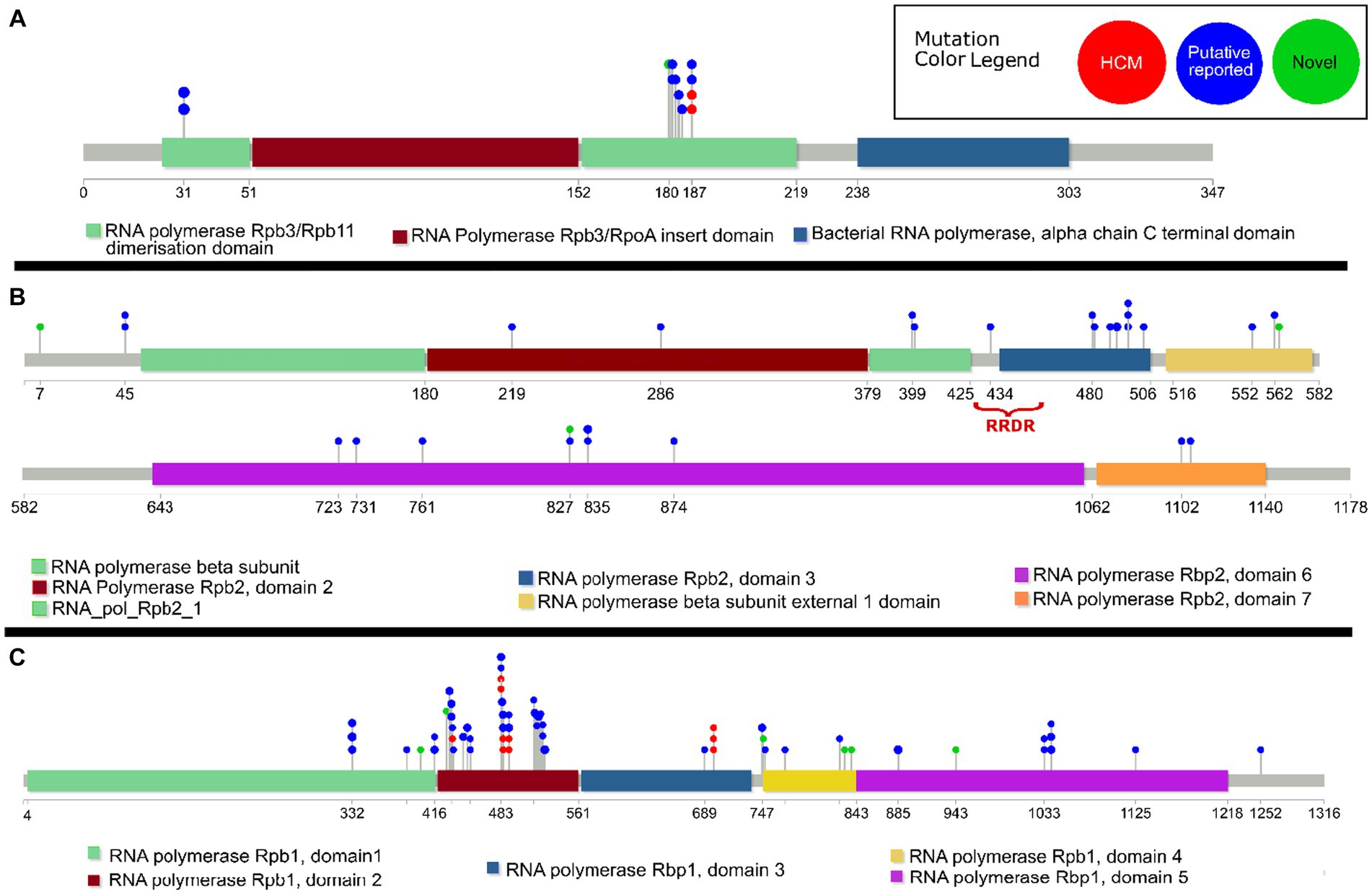
Figure 2. Lollipop diagram of previously reported14 high-probability compensatory mutations (“HCM”), previously reported (Casali et al., 2012, 2014; Comas et al., 2012; de Vos et al., 2013; Song et al., 2014; Li et al., 2016; Liu et al., 2018; Ma et al., 2021) putative compensatory mutations (“Putative reported”), and novel putative compensatory mutations (“Novel”) in the M. tuberculosis genes (A) rpoA, (B) rpoB, and (C) rpoC. The functional domains of each gene are indicated, as well as the rifampicin resistance determining region (RRDR) in rpoB. Only previously reported putative compensatory mutations carried by isolates in this dataset are included.
Discussion
Resistance to RIF is prevalent despite the fitness cost of RIFR conferring mutations. Multiple RIFR mutations in rpoB have been shown to reduce growth rate in vitro (Gagneux et al., 2006; Comas et al., 2012) and in macrophages (Mariam et al., 2004). Yet rifampicin resistant strains continue to spread, and in some countries constitute an increasing threat to an effective TB control (World Health Organization, 2020). One potential explanation for this discrepancy is compensatory mutations in rpoA, rpoB, and rpoC. Three mutations in these genes have been observed in clinical RIFR M. tuberculosis isolates with a restored wildtype growth rate in vitro (Comas et al., 2012). Two compensatory mutations have been shown to restore transcription efficiency to ribosomes with the RIFR marker rpoB:S450L (Stefan et al., 2018). Many more mutations in these genes have been associated with clinical RIFR strains (Comas et al., 2012; de Vos et al., 2013; Song et al., 2014; Li et al., 2016; Liu et al., 2018). And five mutations in rpoB have recently been confirmed by mutagenesis and competitive fitness assays to compensate for rpoB:S450L in M. smegmatis (Ma et al., 2021). RIFR strains with compensatory mutations may have higher transmission rates than RIFR strains without them (de Vos et al., 2013; Casali et al., 2014; Li et al., 2016; Merker et al., 2018; Gygli et al., 2021), though this is disputed in at least one study (Liu et al., 2018). Compensatory mutations may thus serve as markers warning which resistant strains are more likely to cause outbreaks. We expanded the set of putative compensatory mutations by examining rpoABC mutations in 4309 whole genome sequenced clinical M. tuberculosis isolates. Based on our findings we also suggest the hypothesis that each compensatory mutation may only compensate for a specific resistance-conferring mutation, rather than all compensatory mutations compensating for all resistance-conferring mutations. If confirmed, these findings will improve accuracy in determining which strains are at higher risk of causing drug resistant outbreaks.
Previously reported compensatory mutations (Casali et al., 2012, 2014; Comas et al., 2012; de Vos et al., 2013; Song et al., 2014; Li et al., 2016; Liu et al., 2018; Ma et al., 2021) were observed in over half the RIFR isolates (59.1%, 638/1079). Using strict criteria, we identified 11 novel putative compensatory mutations in 37 of the remaining isolates (Table 4). Six of these 11 novel putative compensatory mutations had two or more mutation events. Our criteria additionally independently identified 35 of the previously reported putative compensatory mutations (Supplementary Table S6). One of these independently identified mutations, rpoB:I480V, was confirmed by mutagenesis to compensate for rpoB:S450L in vitro in M. smegmatis (Ma et al., 2021). This independent corroboration of 35 putative compensatory mutations identified by our method supports the validity of the 11 novel putative compensatory mutations identified by our criteria. These 11 novel putative compensatory mutations, together with those previously reported, may serve as markers to warn clinicians which RIFR strains are more likely to cause outbreaks.
Another potential barrier to identifying compensated strains is the specificity of compensatory mutations. Only three RIFR markers were associated with compensatory mutations: rpoB:S450L, rpoB:L452P, and rpoB:D435G (Tables 3, 5; Supplementary Tables S6, S8). Most compensatory mutations were associated with the prevalent RRDR mutation rpoB:S450L, an association reported previously (Song et al., 2014; Borrell and Trauner, 2017; Gygli et al., 2017; Wang et al., 2020; Ma et al., 2021). However the previously reported (Song et al., 2014) putative compensatory mutation rpoB:I1106T was specific to isolates with a pair of rare RRDR mutations, rpoB:L452P and rpoB:D435G. These three mutations were each carried by 22 closely related isolates (Figure 1), suggesting the putative compensatory mutation overcame the fitness cost of the otherwise rare RIFR markers, enabling them to cause a resistant outbreak. We hypothesize that rpoB:L452P and rpoB:D435G are normally rare because only rpoB:I1106T can compensate for their fitness cost, while most other compensatory mutations compensate for rpoB:S450L. If each compensatory mutation only compensates for specific resistance-conferring marker, then predicting which RIFR strains have higher risk of causing outbreaks requires not only a catalogue of compensatory mutations, but knowledge of which combinations of rpoABC mutations compensate for each other.
A recent study of 27,063 isolates found rpoB:S450L in 66.2% of RIFR isolates (6,536/9869) (World Health Organization, 2021a). Our findings suggest that the high prevalence of rpoB:S450L among RIFR isolates (both in this, and previous in vitro and in vivo (Huitric et al., 2006) studies) is likely due to two factors. First, as previously reported, this variant has a lower fitness cost as compared to other resistant-causing variants (Gagneux et al., 2006). Second, as our findings indicate, mutation-specific-compensation may also be responsible for the prevalence of rpoB:S450L. In our findings most compensatory mutations, identified previously or through this study, appear to only compensate only for rpoB:S450L. Pengjiao Ma et al.’s findings support our dual reasoning hypothesis for prevalence of rpoB:S450L (Ma et al., 2021).
The clear next steps for the hypotheses generated through this study is mutagenesis and competitive fitness experiments to confirm the compensatory role. This includes the 11 novel putative compensatory mutations that this study has identified. Mutagenesis and competitive fitness is also needed to test the mutation-specific-compensation hypothesis suggested by our findings, by testing whether compensatory mutations specifically compensate for rpoB:S450L, or other RIFR mutations. Additionally, the criteria to identify these novel putative compensatory mutations was conservative and thus our expanded set of compensatory mutations is more reliable, but not comprehensive. The study also used a limited set of isolates (4309) from a limited set of world regions (9 countries), and would thus miss any region specific or rare mutations. Further study is needed to determine the full set of compensatory mutations.
This study identified 11 novel rpoABC mutations that putatively compensate for the fitness cost of RIFR mutations. This study also independently corroborated another 35 previously reported (Casali et al., 2012, 2014; Comas et al., 2012; de Vos et al., 2013; Song et al., 2014; Li et al., 2016; Liu et al., 2018; Ma et al., 2021) putative compensatory mutations. These mutations may aid future investigation of the effect of compensatory mutations on RIFR TB strain transmission, and eventually aid the detection of strains at high risk of causing RIFR outbreaks. This study additionally found that compensatory mutations were associated with specific RIFR markers, corroborating the previously reported (Song et al., 2014; Borrell and Trauner, 2017; Gygli et al., 2017; Wang et al., 2020; Ma et al., 2021) association between compensatory mutations and rpoB:S450L and greatly expanding the previously reported (Song et al., 2014) outbreak with the putative compensatory mutation rpoB:I1106T and the RIFR markers rpoB:D435G and rpoB:L452P. These associations highlight the need for future study of which combinations of RIFR markers and rpoABC mutations result in compensation.
Data availability statement
The datasets presented in this study can be found in online repositories. The names of the repository/repositories and accession number(s) can be found in the article/Supplementary material.
Author contributions
DC-G: Conceptualization, Investigation, Methodology, Validation, Writing – original draft, Writing – review & editing, Data curation, Formal analysis, Software, Visualization. SR-B: Conceptualization, Formal analysis, Investigation, Methodology, Validation, Visualization, Writing – review & editing. BG: Data curation, Formal analysis, Software, Visualization, Writing – original draft, Writing – review & editing. AE: Conceptualization, Formal analysis, Methodology, Software, Visualization, Writing – original draft, Writing – review & editing. SH: Conceptualization, Investigation, Writing – review & editing. WE: Conceptualization, Investigation, Methodology, Writing – review & editing. FV: Conceptualization, Funding acquisition, Investigation, Methodology, Project administration, Resources, Supervision, Validation, Writing – original draft, Writing – review & editing.
Funding
The author(s) declare financial support was received for the research, authorship, and/or publication of this article. This work was supported by grants from National Institute of Allergy and Infectious Diseases (NIAID R01AI105185 and R01AI163202).
Acknowledgments
We would like to acknowledge James O’Neill from the Laboratory for Pathogenesis of Clinical Drug Resistance and Persistence (LPCDRP) his role in the analysis of compensatory mutations outside of rpoC and rpoA and background research on drug susceptibility accuracy and Scott Mitchell from the LPCDRP for assistance in creating the phylogenetic tree. We also acknowledge the help of Emma Bishop in reviewing and providing critique of the manuscript.
Conflict of interest
The authors declare that the research was conducted in the absence of any commercial or financial relationships that could be construed as a potential conflict of interest.
Publisher’s note
All claims expressed in this article are solely those of the authors and do not necessarily represent those of their affiliated organizations, or those of the publisher, the editors and the reviewers. Any product that may be evaluated in this article, or claim that may be made by its manufacturer, is not guaranteed or endorsed by the publisher.
Supplementary material
The Supplementary material for this article can be found online at: https://www.frontiersin.org/articles/10.3389/fmicb.2023.1265390/full#supplementary-material
Footnotes
References
Bolger, A. M., Lohse, M., and Usadel, B. (2014). Trimmomatic: a flexible trimmer for Illumina sequence data. Bioinformatics 30, 2114–2120. doi: 10.1093/bioinformatics/btu170
Borrell, S., and Trauner, A. (2017). Strain diversity and the evolution of antibiotic resistance. Adv. Exp. Med. Biol. 1019, 263–279. doi: 10.1007/978-3-319-64371-7_14
Brandis, G., Wrande, M., Liljas, L., and Hughes, D. (2012). Fitness-compensatory mutations in rifampicin-resistant RNA polymerase. Mol. Microbiol. 85, 142–151. doi: 10.1111/j.1365-2958.2012.08099.x
Casali, N., Nikolayevskyy, V., Balabanova, Y., Harris, S. R., Ignatyeva, O., Kontsevaya, I., et al. (2014). Evolution and transmission of drug-resistant tuberculosis in a Russian population. Nat. Genet. 46, 279–286. doi: 10.1038/ng.2878
Casali, N., Nikolayevskyy, V., Balabanova, Y., Ignatyeva, O., Kontsevaya, I., Harris, S. R., et al. (2012). Microevolution of extensively drug-resistant tuberculosis in Russia. Genome Res. 22, 735–745. doi: 10.1101/gr.128678.111
Chaisson, M. J., and Tesler, G. (2012). Mapping single molecule sequencing reads using basic local alignment with successive refinement (BLASR): theory and application. BMC Bioinformatics 13:238. doi: 10.1186/1471-2105-13-238
Chernyaeva, E. N., Shulgina, M. V., Rotkevich, M. S., Dobrynin, P. V., Simonov, S. A., Shitikov, E. A., et al. (2014). Genome-wide Mycobacterium tuberculosis variation (GMTV) database: a new tool for integrating sequence variations and epidemiology. BMC Genomics 15:308. doi: 10.1186/1471-2164-15-308
Comas, I., Borrell, S., Roetzer, A., Rose, G., Malla, B., Kato-Maeda, M., et al. (2012). Whole-genome sequencing of rifampicin-resistant Mycobacterium tuberculosis strains identifies compensatory mutations in RNA polymerase genes. Nat. Genet. 44, 106–110. doi: 10.1038/ng.1038
de Vos, M., Müller, B., Borrell, S., Black, P. A., van Helden, P. D., Warren, R. M., et al. (2013). Putative compensatory mutations in the rpoC gene of rifampin-resistant Mycobacterium tuberculosis are associated with ongoing transmission. Antimicrob. Agents Chemother. 57, 827–832. doi: 10.1128/AAC.01541-12
Freschi, L., Vargas, R. Jr., Husain, A., Kamal, S. M. M., Skrahina, A., Tahseen, S., et al. (2021). Population structure, biogeography and transmissibility of Mycobacterium tuberculosis. Nat. Commun. 12:6099. doi: 10.1038/s41467-021-26248-1
Gagneux, S., Long, C. D., Small, P. M., van, T., Schoolnik, G. K., and Bohannan, B. J. M. (2006). The competitive cost of antibiotic resistance in Mycobacterium tuberculosis. Science (New York, N.Y.) 312, 1944–1946. doi: 10.1126/science.1124410
Garfein, R. S., Catanzaro, D. G., Rodwell, T. C., Avalos, E., Jackson, R. L., Kaping, J., et al. (2015). Phenotypic and genotypic diversity in a multinational sample of drug-resistant Mycobacterium tuberculosis isolates. Int. J. Tuberc. Lung Dis. 19, 420–427. doi: 10.5588/ijtld.14.0488
Gygli, S. M., Borrell, S., Trauner, A., and Gagneux, S. (2017). Antimicrobial resistance in Mycobacterium tuberculosis: mechanistic and evolutionary perspectives. FEMS Microbiol. Rev. 41, 354–373. doi: 10.1093/femsre/fux011
Gygli, S. M., Loiseau, C., Jugheli, L., Adamia, N., Trauner, A., Reinhard, M., et al. (2021). Prisons as ecological drivers of fitness-compensated multidrug-resistant Mycobacterium tuberculosis. Nat. Med. 27, 1171–1177. doi: 10.1038/s41591-021-01358-x
Hillery, N., Groessl, E. J., Trollip, A., Catanzaro, D., Jackson, L., Rodwell, T. C., et al. (2014). The global consortium for drug-resistant tuberculosis diagnostics (GCDD): design of a multi-site, head-to-head study of three rapid tests to detect extensively drug-resistant tuberculosis. Trials 15:434. doi: 10.1186/1745-6215-15-434
Huerta-Cepas, J., Serra, F., and Bork, P. (2016). ETE 3: reconstruction, analysis, and visualization of Phylogenomic data. Mol. Biol. Evol. 33, 1635–1638. doi: 10.1093/molbev/msw046
Huitric, E., Werngren, J., Juréen, P., and Hoffner, S. (2006). Resistance levels and rpoB gene mutations among in vitro-selected rifampin-resistant Mycobacterium tuberculosis mutants. Antimicrob. Agents Chemother. 50, 2860–2862. doi: 10.1128/AAC.00303-06
Jay, J. J., and Brouwer, C. (2016). Lollipops in the clinic: information dense mutation plots for precision medicine. PLoS One 11:e0160519. doi: 10.1371/journal.pone.0160519
Jin, D. J., and Gross, C. A. (1988). Mapping and sequencing of mutations in the Escherichia coli rpoB gene that lead to rifampicin resistance. J. Mol. Biol. 202, 45–58. doi: 10.1016/0022-2836(88)90517-7
Jo, K., Lee, S., Kang, M. R., Sung, H., Kim, M. N., and Shim, T. S. (2017). Frequency and type of disputed rpoB mutations in Mycobacterium tuberculosis isolates from South Korea. Tuberc. Respir. Dis. 80, 270–276. doi: 10.4046/trd.2017.80.3.270
Koboldt, D. C., Zhang, Q., Larson, D. E., Shen, D., McLellan, M. D., Lin, L., et al. (2012). VarScan 2: somatic mutation and copy number alteration discovery in cancer by exome sequencing. Genome Res. 22, 568–576. doi: 10.1101/gr.129684.111
Köser, C. U., Georghiou, S. B., Schön, T., and Salfinger, M. (2021). On the consequences of poorly defined breakpoints for Rifampin susceptibility testing of Mycobacterium tuberculosis. J. Clin. Microbiol. 59, e02328–e02320. doi: 10.1128/JCM.02328-20
Kurtz, S., Phillippy, A., Delcher, A. L., Smoot, M., Shumway, M., Antonescu, C., et al. (2004). Versatile and open software for comparing large genomes. Genome Biol. 5:R12. doi: 10.1186/gb-2004-5-2-r12
Langmead, B., and Salzberg, S. L. (2012). Fast gapped-read alignment with bowtie 2. Nat. Methods 9, 357–359. doi: 10.1038/nmeth.1923
Leinonen, R., Sugawara, H., and Shumway, M. (2011). The sequence read archive. Nucleic Acids Res. 39, D19–D21. doi: 10.1093/nar/gkq1019
Letunic, I., and Bork, P. (2016). Interactive tree of life (iTOL) v3: an online tool for the display and annotation of phylogenetic and other trees. Nucleic Acids Res. 44, W242–W245. doi: 10.1093/nar/gkw290
Li, H., Handsaker, B., Wysoker, A., Fennell, T., Ruan, J., Homer, N., et al. (2009). The sequence alignment/map format and SAMtools. Bioinformatics 25, 2078–2079. doi: 10.1093/bioinformatics/btp352
Li, Q.-J., Jiao, W.-W., Yin, Q.-Q., Xu, F., Li, J.-Q., Sun, L., et al. (2016). Compensatory mutations of rifampicin resistance are associated with transmission of multidrug resistant Mycobacterium tuberculosis Beijing genotype strains in China. Antimicrob. Agents Chemother. 60, 2807–2812. doi: 10.1128/AAC.02358-15
Liu, Q., Zuo, T., Xu, P., Jiang, Q., Wu, J., Gan, M., et al. (2018). Have compensatory mutations facilitated the current epidemic of multidrug-resistant tuberculosis? Emerg. Microbes Infect. 7:98. doi: 10.1038/s41426-018-0101-6
Ma, P., Luo, T., Ge, L., Chen, Z., Wang, X., Zhao, R., et al. (2021). Compensatory effects of M. tuberculosis rpoB mutations outside the rifampicin resistance-determining region. Emerg. Microbes Infect. 10, 743–752. doi: 10.1080/22221751.2021.1908096
Mariam, D. H., Mengistu, Y., Hoffner, S. E., and Andersson, D. I. (2004). Effect of rpoB mutations conferring rifampin resistance on fitness of Mycobacterium tuberculosis. Antimicrob. Agents Chemother. 48, 1289–1294. doi: 10.1128/AAC.48.4.1289
McLaren, W., Gil, L., Hunt, S. E., Riat, H. S., Ritchie, G. R. S., Thormann, A., et al. (2016). The Ensembl variant effect predictor. Genome Biol. 17:122. doi: 10.1186/s13059-016-0974-4
Merker, M., Barbier, M., Cox, H., Rasigade, J. P., Feuerriegel, S., Kohl, T. A., et al. (2018). Compensatory evolution drives multidrug-resistant tuberculosis in Central Asia. elife 7, 1–31. doi: 10.7554/eLife.38200
Miller, L. P., Crawford, J. T., and Shinnick, T. M. (1994). The rpoB gene of Mycobacterium tuberculosis. Antimicrob. Agents Chemother. 38, 805–811. doi: 10.1128/AAC.38.4.805.Updated
Modlin, S. J., Conkle-Gutierrez, D., Kim, C., Mitchell, S. N., Morrissey, C., Weinrick, B. C., et al. (2020). Drivers and sites of diversity in the DNA adenine methylomes of 93 Mycobacterium tuberculosis complex clinical isolates. elife 9:e58542. doi: 10.7554/eLife.58542
Modlin, S. J., Robinhold, C., Morrissey, C., Mitchell, S. N., Ramirez-Busby, S. M., Shmaya, T., et al. (2021). Exact mapping of Illumina blind spots in the Mycobacterium tuberculosis genome reveals platform-wide and workflow-specific biases. Microb. Genom. 7:mgen000465. doi: 10.1099/mgen.0.000465
Nusrath Unissa, A., Hassan, S., Indira Kumari, V., Revathy, R., and Hanna, L. E. (2016). Insights into RpoB clinical mutants in mediating rifampicin resistance in Mycobacterium tuberculosis. J. Mol. Graph. Model. 67, 20–32. doi: 10.1016/j.jmgm.2016.04.005
Penn-Nicholson, A., Gomathi, S. N., Ugarte-Gil, C., Meaza, A., Lavu, E., Patel, P., et al. (2021). A prospective multicentre diagnostic accuracy study for the Truenat tuberculosis assays. Eur. Respir. J. 58:2100526. doi: 10.1183/13993003.00526-2021
Raja, S., Ching, J., Xi, L., Hughes, S. J., Chang, R., Wong, W., et al. (2005). Technology for automated, rapid, and quantitative PCR or reverse transcription-PCR clinical testing. Clin. Chem. 51, 882–890. doi: 10.1373/clinchem.2004.046474
Ramaswamy, S., and Musser, J. M. (1998). Molecular genetic basis of antimicrobial agent resistance in Mycobacterium tuberculosis: 1998 update. Tuber. Lung Dis. 79, 3–29. doi: 10.1054/tuld.1998.0002
Ramirez-Busby, S., Elghraoui, A., Kim, Y. B., Kim, K., and Valafar, F. (2018) ‘PBHoover and CigarRoller: a method for confident haploid variant calling on Pacific biosciences data and its application to heterogeneous population analysis’, bioRxiv, [Epub ahead of preprint]. doi: 10.1101/360370
Rodwell, T. C., Valafar, F., Douglas, J., Qian, L., Garfein, R. S., Chawla, A., et al. (2014). Predicting extensively drug-resistant Mycobacterium tuberculosis phenotypes with genetic mutations. J. Clin. Microbiol. 52, 781–789. doi: 10.1128/JCM.02701-13
Ross, M. G., Russ, C., Costello, M., Hollinger, A., Lennon, N. J., Hegarty, R., et al. (2013). Characterizing and measuring bias in sequence data. Genome Biol. 14:R51. doi: 10.1186/gb-2013-14-5-r51
Saiki, R. K., Walsh, P. S., Levenson, C. H., and Erlich, H. A. (1989). Genetic analysis of amplified DNA with immobilized sequence-specific oligonucleotide probes. Proc. Natl. Acad. Sci. U. S. A. 86, 6230–6234. doi: 10.1073/pnas.86.16.6230
Song, T., Park, Y., Shamputa, I. C., Seo, S., Lee, S. Y., Jeon, H. S., et al. (2014). Fitness costs of rifampicin resistance in Mycobacterium tuberculosis are amplified under conditions of nutrient starvation and compensated by mutation in the β’ subunit of RNA polymerase. Mol. Microbiol. 91, 1106–1119. doi: 10.1111/mmi.12520
Stamatakis, A. (2014). RAxML version 8: a tool for phylogenetic analysis and post-analysis of large phylogenies. Bioinformatics 30, 1312–1313. doi: 10.1093/bioinformatics/btu033
Stefan, M. A., Ugur, F. S., and Garcia, G. A. (2018). Source of the fitness defect in Rifamycin-resistant Mycobacterium tuberculosis RNA polymerase and the mechanism of compensation by mutations in the β’ subunit. Antimicrob. Agents Chemother. 62:e00164-18. doi: 10.1128/AAC.00164-18
Telenti, A., Imboden, P., Marchesi, F., Matter, L., Schopfer, K., Bodmer, T., et al. (1993). Detection of rifampicin-resistance mutations in Mycobacterium tuberculosis. Lancet 341, 647–651. doi: 10.1016/0140-6736(93)90417-F
Torres, J. N., Paul, L. V., Rodwell, T. C., Victor, T. C., Amallraja, A. M., Elghraoui, A., et al. (2015). Novel katG mutations causing isoniazid resistance in clinical M. tuberculosis isolates. Emerg. Microbes Infect. 4:e42. doi: 10.1038/emi.2015.42
Walker, T. M., Kohl, T. A., Omar, S. V., Hedge, J., del Ojo Elias, C., Bradley, P., et al. (2015). Whole-genome sequencing for prediction of Mycobacterium tuberculosis drug susceptibility and resistance: a retrospective cohort study. Lancet Infect. Dis. 15, 1193–1202. doi: 10.1016/S1473-3099(15)00062-6
Wang, K., Pei, H., Huang, B., Zhu, X., Zhang, J., Zhou, B., et al. (2013). The expression of ABC efflux pump, Rv1217c–Rv1218c, and its association with multidrug resistance of Mycobacterium tuberculosis in China. Curr. Microbiol. 66, 222–226. doi: 10.1007/s00284-012-0215-3
Wang, S., Zhou, Y., Zhao, B., Ou, X., Xia, H., Zheng, Y., et al. (2020). Characteristics of compensatory mutations in the rpoC gene and their association with compensated transmission of Mycobacterium tuberculosis. Front. Med. 14, 51–59. doi: 10.1007/s11684-019-0720-x
Wilm, A., Aw, P. P., Bertrand, D., Yeo, G. H., Ong, S. H., Wong, C. H., et al. (2012). LoFreq: a sequence-quality aware, ultra-sensitive variant caller for uncovering cell-population heterogeneity from high-throughput sequencing datasets 40, 11189–11201. doi: 10.1093/nar/gks918
World Health Organization (2021a) WHO Catalogue of mutations in Mycobacterium tuberculosis complex and their association with drug resistance. Available at: https://www.who.int/publications/i/item/9789240028173
World Health Organization (2021b) WHO Consolidated guidelines on tuberculosis. Module 3: diagnosis -rapid diagnostics for tuberculosis detection.
World Health Organization (2022) Who Global tuberculosis report 2022. Available at: https://www.who.int/teams/global-tuberculosis-programme/tb-reports/global-tuberculosis-report-2022
Keywords: tuberculosis, Mycobacterium tuberculosis , rifampicin, antibiotic resistance, compensatory mutations, fitness
Citation: Conkle-Gutierrez D, Ramirez-Busby SM, Gorman BM, Elghraoui A, Hoffner S, Elmaraachli W and Valafar F (2024) Novel and reported compensatory mutations in rpoABC genes found in drug resistant tuberculosis outbreaks. Front. Microbiol. 14:1265390. doi: 10.3389/fmicb.2023.1265390
Edited by:
Satoshi Mitarai, Japan Anti-Tuberculosis Association, JapanReviewed by:
Prasit Palittapongarnpim, Mahidol University, ThailandKatiany Rizzieri Caleffi Ferracioli, State University of Maringá, Brazil
Copyright © 2024 Conkle-Gutierrez, Ramirez-Busby, Gorman, Elghraoui, Hoffner, Elmaraachli and Valafar. This is an open-access article distributed under the terms of the Creative Commons Attribution License (CC BY). The use, distribution or reproduction in other forums is permitted, provided the original author(s) and the copyright owner(s) are credited and that the original publication in this journal is cited, in accordance with accepted academic practice. No use, distribution or reproduction is permitted which does not comply with these terms.
*Correspondence: Faramarz Valafar, ZmFyYW1hcnpAc2RzdS5lZHU=
†These authors have contributed equally to this work