- Department of Epidemiology, Ministry of Education Key Lab of Hazard Assessment and Control in Special Operational Environment, School of Public Health, Air Force Medical University, Xi’an, Shaanxi, China
Introduction: As tick-borne diseases rise to become the second most prevalent arthropod-transmitted disease globally, the increasing investigations focus on ticks correspondingly. Factors contributed to this increase include anthropogenic influences, changes in vertebrate faunal composition, social-recreational shifts, and climatic variation. Employing the 16S gene sequence method in next-generation sequencing (NGS) allows comprehensive pathogen identification in samples, facilitating the development of refined approaches to tick research omnidirectionally.
Methods: In our survey, we compared the microbial richness and biological diversity of ticks in Wuwei City, Gansu province, differentiating between questing ticks found in grass and parasitic ticks collected from sheep based on 16S NGS method.
Results: The results show Rickettsia, Coxiella, and Francisella were detected in all 50 Dermacentor nuttalli samples, suggesting that the co-infection may be linked to specific symbiotic bacteria in ticks. Our findings reveal significant differences in the composition and diversity of microorganisms, with the Friedmanniella and Bordetella genera existing more prevalent in parasitic ticks than in questing ticks (p < 0.05). Additionally, the network analysis demonstrates that the interactions among bacterial genera can be either promotive or inhibitive in ticks exhibiting different lifestyles with the correlation index |r| > 0.6. For instance, Francisella restrains the development of 10 other bacteria in parasitic ticks, whereas Phyllobacterium and Arthrobacter enhance colonization across all tick species.
Discussion: By leveraging NGS techniques, our study reveals a high degree of species and phylogenetic diversity within the tick microbiome. It further highlights the potential to investigate the interplay between bacterial genera in both parasitic and questing ticks residing in identical habitat environments.
1 Introduction
Tick-borne diseases remain a longstanding and burgeoning global health concern, with disease incidences seeing a dramatic increase since the 20th century (Rodino et al., 2020). This trend is evident worldwide and reflected in the rising number of tick-borne encephalitis and hemorrhagic fever cases reported across Europe and Asia (Rochlin and Toledo, 2020). In China, for instance, tick-borne diseases attributed to over 40 diverse pathogens have proliferated across several provinces, including Anhui, Henan, Inner Mongolia, Tibet, Zhejiang, and Liaoning, since initial reporting in 1982 with the amplification of epidemic area and infected human amount (Lan-Hua and Yi, 2019). Notwithstanding these broad findings, studies specific to Gansu Province remain scarce. However, the importance of investigating the intricate role of microbiology in environmental contexts, given ticks’ critical function as bacterial vectors, is well-documented in other regions of China.
A deeper understanding of the composition and diversity of microbiomes influencing pathogen transmission necessitates a nuanced exploration of both parasitic (feeding) ticks and questing (off-host) ticks. Differentiating these two tick lifestyles may yield crucial insights into disease manifestation in relation to varying host or off-host circumstances (Ciebiera et al., 2021), although the interplay between tick communities and the pathogens they carry remains somewhat nebulous (Kahl, 2018). Although parasitic ticks predominantly inhabit the body surfaces of hosts such as humans and domestic animals, facilitating pathogen transmission, the comparative studies of these ticks with their counterparts living in wild grass and scrub are hampered by unilateral approaches that focus narrowly on specific tick categories and pathogen transmission routes.
In the last 20 years, various techniques have been employed to elucidate the microbial composition within tick communities. The advent of next-generation sequencing (NGS) has accelerated pathogen identification, providing a rapid and efficient means to comprehensively explore pathogen community structure (Sanschagrin and Yergeau, 2014). Utilized extensively in bacterial gene detection, NGS offers high efficiency and accuracy in identifying microbes from large sample sets in a short time frame (Chaorattanakawee et al., 2022). The conservation and variability within the small subunit ribosomal RNA gene, especially the 16S rRNA gene, permit the design of unique primers for specific bacterial species, making NGS a powerful tool for disease surveillance and comprehensive microbial taxonomic identification (Rodino et al., 2021).
For this study, we selected Wuwei City in Gansu province, China, known for its rich species diversity, as our sampling site (Sun et al., 2016). Previous reports have identified new species like Ornithodoros huajianensis in the city’s Mongolian marmots (Sun et al., 2019), as well as the presence of Babesia spp. and Theileria spp. in cattle (Sun et al., 2020). Our research aims to elucidate the differences in microbiomes and tick-borne pathogens in Wuwei City, Gansu Province. A thorough investigation of both questing and parasitic ticks will be conducted, intending to bridge the gap in current studies that overlook the microbiome composition and bacterial transmission differences between these two lifestyle ticks in natural environments. Ultimately, we intend to enhance understanding of tick-borne pathogens by detailing every procedure of this study, from tick collection to NGS analysis, with a focus on characterizing the questing ticks and the feeding ticks from sheep in Wuwei City.
2 Materials and methods
2.1 Ticks collection and morphological observation
All ticks were collected from Wuwei City (102.65°E, 37.94°N), Gansu province, in May 2022. Feeding ticks were carefully extracted from sheep using tweezers while questing ticks were gathered from grassland using the Flag Cloth Law method, which involves dragging a 90 cm × 60 cm white flannelette flag across the surface. It should be noted that the sampling of both questing and feeding ticks was opportunistic and was therefore not standardized with respect to collection area or time.
Following collection, all ticks were immediately stored in tubes with perforated lids and refrigerated at 4°C to minimize tick mortality. Afterward, ticks’ surfaces were cleaned using 75% ethyl alcohol, and identification was carried out with a stereoscopic microscope (RH-2000, HIROX, Japan), based on observed structural characteristics of the ticks.
2.2 Nucleic acid extraction
Three ticks, morphologically identified as Dermacentor at the genus level, were placed in a tube and labeled according to the collecting date and lifestyles for subsequent operations. Each tube was injected with 1 mL of Stroke-Physiological Saline Solution (SPSS, 0.9% NaCl), and 3–4 0.2 mm steel balls were added before the samples were ground at a frequency of 65 Hz for 500 s. Following this, the samples were incubated in a dry water bath for 10 min at a consistent temperature of 55°C, and the supernatant fluid was carefully transferred to a new tube after a rapid centrifugation at 12,000 rpm for 1 min in a standard centrifuge. Nucleic acid extraction was then performed using the Ex-DNA/RNA nucleic acid extraction kit (TIAN LONG, China) according to the manufacturer’s instructions (Shen et al., 2020), and the extracted samples were stored at −20°C for further analysis.
2.3 PCR amplification and identification
Tick identification was achieved by amplifying DNA using PCR, specifically targeting the mitochondrial gene cytochrome c oxidase I (COI; Lv et al., 2014). The primers used for the molecular biological identification of ticks were COI (Forward primer: 5′-GGAACAATATATTTAATTTTTGG-3′, Reverse primer: 5′-ATCTATCCCTACTGTAAATATATG-3′).
The PCR reactions contained 40 ng of genomic DNA and were conducted in 50 μL reaction volumes including 25 μL 2× DreamTaq PCR Master Mix (Thermo, America), 2 μL of each primer (10 μmol/L), 2 μL of DNA (20 ng/μL), and 19 μL of ddH2O. Reactions were performed in a thermocycler under the following conditions: an initial preheating step at 95°C for 3 min, followed by 35 cycles of denaturation at 95°C for 30 s; annealing at 45°C for 30 s; extension at 72°C for 1 min; and a final extension at 72°C for 10 min.
The PCR products were subjected to 1.2% agarose gel electrophoresis (AGE) under specific conditions, including a voltage of 220 V, a current of 400 mA, and a duration of 30 min. Following this, the gel was imaged using a gel imager to visually represent the DNA fragments. For precise identification of the tick species, the nucleotide sequence of the PCR products was determined using Sanger sequencing, which was facilitated by AuGCT DNA-SYN Biotechnology Co., Ltd. located in Beijing, China.
2.4 Library preparation and sequencing
Aimed to amplify the sequences of species-specific for the 16S rRNA gene of the ribosome’s large subunit, the methods followed involved the generation of a DNA library using QIAseq FX DNA Library Kit (QIAGEN, Germany), as per the manufacturer’s instructions, before diluting the nucleic acid for subsequent sequencing, so that the concentration could be detected by the Qubit-4 (Thermo, America).
The primary protocol encompassed several steps, including library preparation via the fusion method, amplicon purification, quantification, and pooling. The final products were individually validated using AGE and any samples with concentrations falling below the standard 50 ng/μL, as defined by the Nextera XT Index Kit v2 (Illumina), were deemed unqualified and hence excluded from the pool. All pooled DNA samples were then subjected to paired-end sequencing, utilizing the MiSeq Reagent Kit V3 on the Illumina HiSeq platform (Odendaal et al., 2022). This sequencing process adhered strictly to the manufacturer’s instructions and was characterized by an insert size of 350 bp and a read length of 250 bp.
2.5 Sequence data analysis
The barcode-based data were analyzed using the Quantitative Insights Into Microbial Ecology version2 (QIIME2) software suite (Caporaso et al., 2010), which provides a comprehensive software environment, data standards, and tool wrappers. The sequence data, presented in FASTQ format, were demultiplexed and consolidated into the same directory before input as QIIME artifacts (.qza) or QIIME visualizations (.qzv). After importing the paired-end reads from the original DNA fragments into the virtual machine, we utilized the DADA2 method to denoise data by truncating both forward and reverse sequences at 230 base pairs each. Following the DADA2 protocol, the sequences were sorted and grouped into Autosave (ASV), which is replaced by Operational Taxonomic Unit (OTU) in the following. The sequences were matched against the Silva database according to the Uchime algorithm (Edgar et al., 2011). Upon creation or acquisition of the classifier artifact, the “qiime taxa barplot” command was used to perform taxonomic analysis of the OTU representative sequences. The bacterial composition of each sample was visualized in a bar figure using the R packages ggplot2 and RColorBrewer (Wickham, 2009; Neuwirth, 2022).
2.6 The alpha and beta diversity analysis
For single-sample diversity analysis or alpha diversity, we determined sufficient sequencing depth based on rarefaction curves for the observed number of OTUs across all samples shown as illustrated in the Good’s coverage. After the sequences of all samples were randomly drawn to a uniform data volume, we calculated the number of unique OTUs, the community richness as determined by the Shannon estimator, and the community evenness as indicated by the Pielou index in each sample using the R package Vegan (Dixon, 2003). The Wilcoxon rank-sum test was employed to assess whether these indices differed significantly and the analysis of similarities (ANOSIM) was used to quantify the variation in bacterial composition resulting from different tick lifestyles using package Vegan.
Following the exclusion of Rickettsiaceae, the abundance of bacteria in samples was recalculated and beta diversity analyses were performed at the family level using the R packages Vegan, phyloseq, and ggplot2 (McMurdie and Holmes, 2013). Weighted and unweighted UniFrac analyses were utilized to examine diversity and to compare different groups, which were subsequently plotted in a principal coordinate analysis (PCoA). The Welch’s t-test was applied to assess differences in bacterial composition between two tick lifestyles. Variations in bacterial composition between groups were visualized using STAMP software (Parks et al., 2014). Lastly, to identify microbial taxa with significant differences between Groups A and B from phylum to species level, we employed Linear Discriminant Analysis (LDA) Effect Size (LEfSe; Segata et al., 2011). A threshold LDA score of >2 was used within 95% confidence intervals.1
2.7 Phylogenetic analysis and network relationship
The representative COI gene sequences and pathogen reads obtained were cross-referenced with existing results in GenBank, employing the Basic Local Alignment Search Tool (BLAST) search engine provided by the National Center for Biotechnology Information (NCBI).2 Multiple sequence alignment was conducted using the ClustalW algorithm within the MEGA-11 software suite, applying default parameters. The phylogenetic tree was then constructed using the Kimura two-parameter model of Neighbor-Joining method based on MEGA-11, with bootstrap values estimated from 1,000 replicates.
The Spearman rank correlation test was conducted to present the interrelationship between two bacteria in samples using the R packages Hmisc and Igraph (Csardi and Nepusz, 2005), and the networks were constructed to visualize relationships between OTUs at the genus level. The two bacteria were linked by red or blue lines which represented a positive or negative correlativity, respectively, a process facilitated by Gephi software.3 OTUs that were either unassigned or occurred only once were disregarded at the genus level and a cut-off value was established for reads to eliminate pathogens. Microbial occurrences that represented less than 10% of the frequency distribution of reads in a microorganism were discarded, and the correlation coefficient (|r|-value) was set at >0.6 with a 95% confidence level (p < 0.05).
3 Results
3.1 Tick identification
The ticks were categorized into two groups based on their mode of capture: Group A consisted of feeding ticks, while Group B comprised questing ticks. A total of 150 adult ticks were identified as Dermacentor nuttalli (Acari: Ixodidae) according to statistics, confirmed through morphological identifications and a PCR assay of the species-specific COI region, which a high identity range of 92.66–99.75%. The phylogeny is shown in Figure 1.
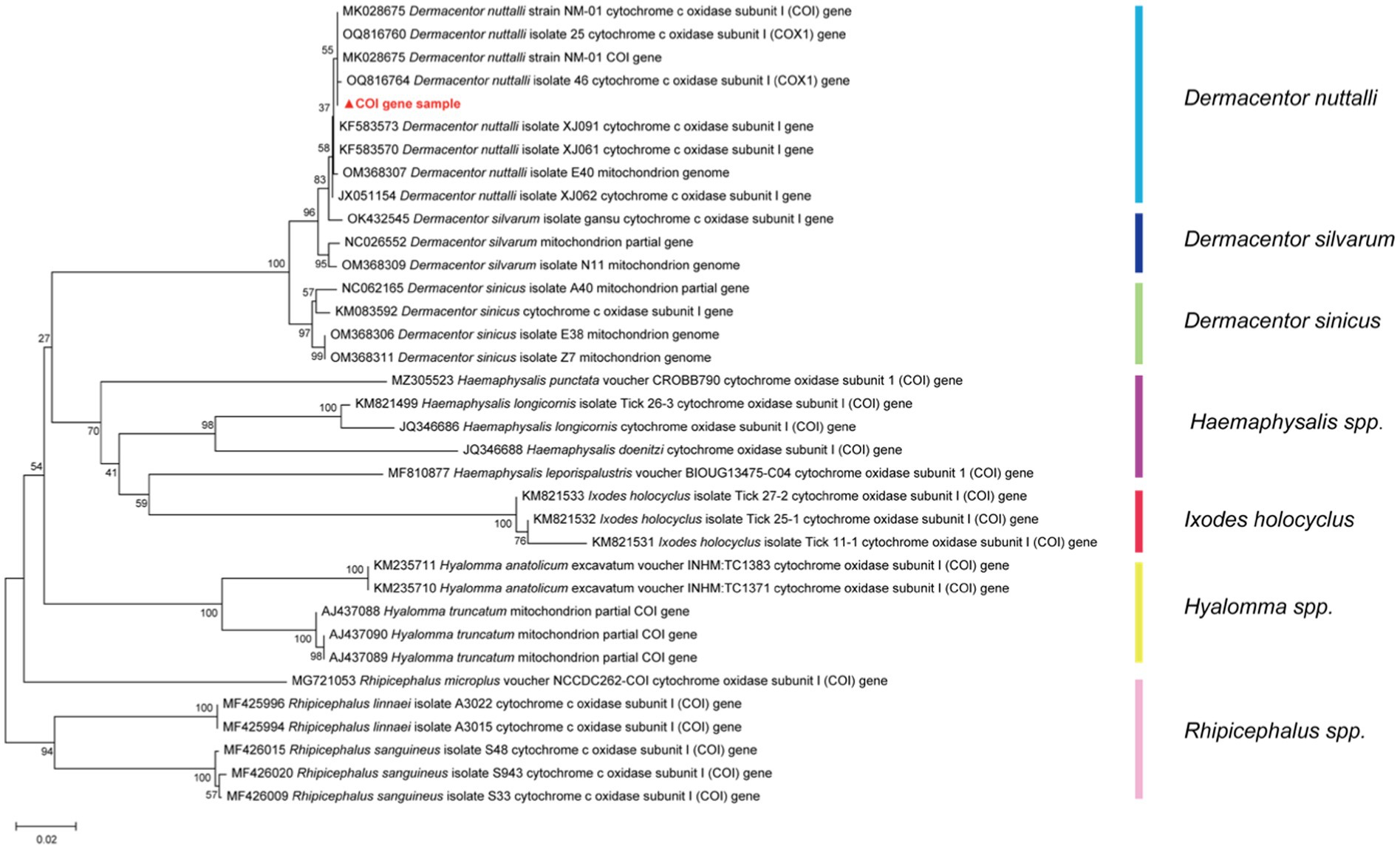
Figure 1. The phylogenetic tree of the COI gene with NCBI Blast results of tick species, was constructed based on the Kimura two-parameter model of Neighbor-Joining method using 1,000 repeated estimated bootstrap values.
3.2 Pathogen detection
Prior to the 16S metagenomics sequencing, our laboratory conducted nested PCR assays using specific primers, through which five pathogens, namely Rickettsia, Anaplasma, Borrelia burgdorferi, Babesia, and Bartonella, were detected. The primer sequences are outlined in Table 1, displayed from 5′ to 3′ for both forward and reverse sequences.
Of all the tested nucleic acid samples, the prevalence rates for Rickettsia and Anaplasma stood at 32.67 and 4.67%, respectively, surpassing those of the other three pathogens. Notably, Borrelia burgdorferi, Babesia, and Bartonella were not detected using conventional PCR assays. A subset of the samples (4.67%) demonstrated detected with Rickettsia and Anaplasma simultaneously. Results of the positive rate are presented in Supplementary Table 1.
3.3 Data general statistic
Using the Illumina HiSeq platform and after eliminating low-quality sequences, 5,480,349 reads were obtained in the data analysis. The bacterial genera were determined by comparing the taxonomic profiles at the genus level against the Silva database. Approximately 8 × 106 paired-end V3-V4 16S reads were procured from all samples, with the read count varying from 7,332 to 417,497 and Supplementary Table 2 included 16S NGS sample productions. After pre-processed (merged, quality filtered, and removal of singletons and chimeras) and post-processed, a cumulative total of 5 million sequences were obtained for the samples and their replicates. The average number of sequences was 109,607 and a range from 42,877 to 257,450 as illustrated in Figure 2A. The rarefaction curves, which level off well before 10,000 reads (Figure 2B), indicate that the number of reads is sufficient to compile a reliable list of bacterial genera. The raw data had uploaded to NCBI with the Bio-Project Accession Number PRJNA1015185.
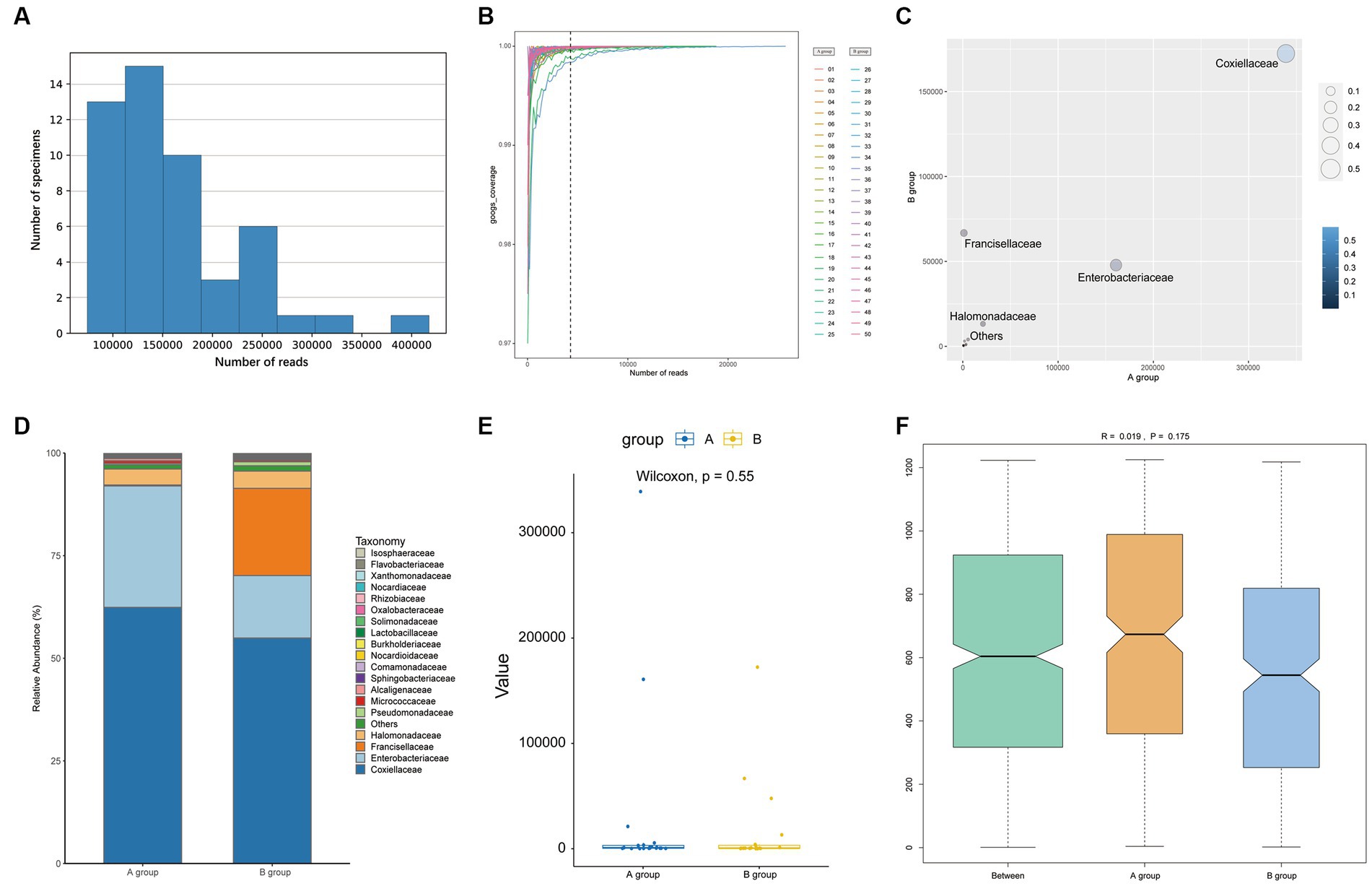
Figure 2. The plots show the information of reads (A,B) and the comparison between groups after removing Rickettsiaceae at the family level (C–F). (A) The bar shows the reads in samples. (B) Rarefaction curves estimated from reads obtained. (C) The number of reads of each family of bacteria is plotted. (D) The bar plot shows the microbial community between groups. (E) A Wilcoxon test of OTUs between two groups with a box plot. (F) The ANOSIM analysis between groups (pseudo-R = 0.019, p = 0.175).
3.4 Taxonomy and group comparison
At the family level, Rickettsiaceae, Coxiellaceae, and Enterobacteriaceae represented an average of 84.21, 9.42, and 3.84%, respectively. The top 20 taxonomics for each sample are illustrated in Figure 3A. Although Rickettsiaceae is predominant in most samples, the Coxiellaceae and the Fancisellaceae are significantly prevalent in samples number 25 (33,450 reads, 68.18%) and 50 (65,231 reads, 48.29%) respectively. As shown in Figure 3B, Rickettsiaceae comprises 2,330,700 (81.11%) and 2,241,292 (87.71%) of the reads in Groups A and B, respectively. Notably, Coxiellaceae and Enterobacteriaceae in Group A (339,077 reads, 11.80%; 160,804 reads, 5.60%) have approximately double and triple the reads in Group B (172,405 reads, 6.75%, 47,821 reads, 1.87%) respectively. In contrast, the Francisellaceae family, although less prominent, shows a slight distinction between the groups, with Group B (66,723 reads, 2.61%) having more reads and proportions than Group A (1,187 reads, 0.04%).
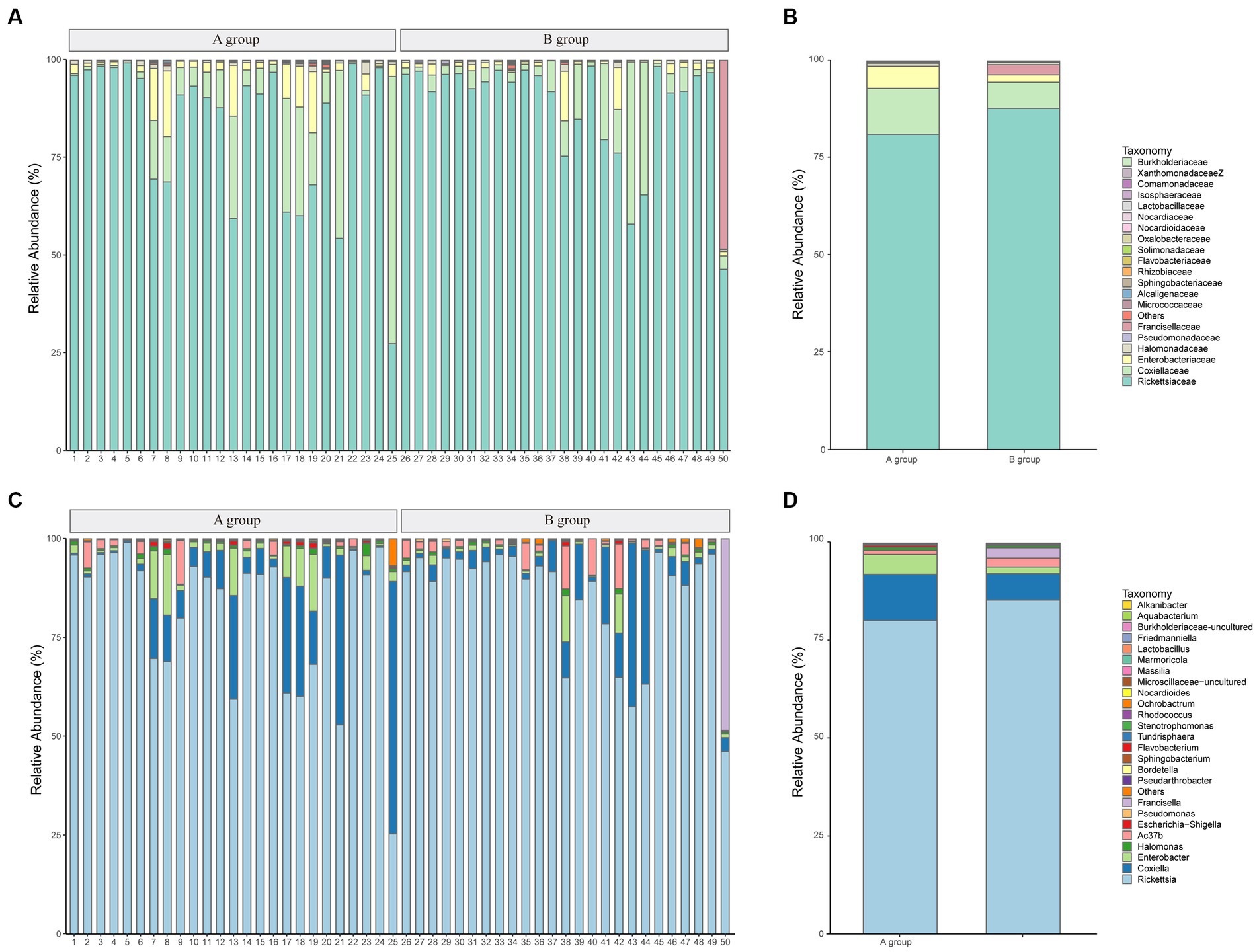
Figure 3. The microbial community bar plots of bacteria comparison. (A) The top 20 communities at the family level for samples. (B) The top 20 communities at the family level with groups. (C) The top 25 communities at the genus level for samples. (D) The top 25 communities at the genus level with groups.
And at the genus level, Rickettsia is the dominant genus across all samples, comprising between 25.36 and 98.95% of the total community (average: 82.63%), followed by Coxiella and Enterobacter, which account for averages of 9.42, and 3.49%, respectively. Figure 3C provides a visual representation of the relative abundance of the top 25 species at the genus level. The figure illustrates that the genus-level classifications of Rickettsia and Ac37b collectively constitute the family-level classification of Rickettsiaceae. The comparison of the top 25 species between groups is shown in Figure 3D.
Regarding alpha diversity, there were no significant differences between groups for either Shannon Index (W = 359, p = 0.375) or Simpson Index (W = 353, p = 0.441), despite the range of the two Index being wider for Group A compared to Group B, as presented in Supplementary Figure 1. The Wilcoxon rank test of other indexes also had no statistical differences which are shown in Supplementary Table 3. There were no significant differences in bacterial microbiome compositions between parasitic and questing ticks according to ANOSIM (pseudo-R = 0.019, p = 0.175), as illustrated previously in Figure 2F.
3.5 The diversity removed Rickettsiaceae
However, after removing Rickettsiaceae computationally, the composition unveils noteworthy variations between groups (Figure 2C). It is apparent that Figure 2D explicitly presents the dominant role of Coxiellaceae within two groups (A: 339,077 reads, 62.46%; B: 172,405 reads, 54.88%), and subsequently, a significant disparity in the presence of Enterobacteriaceae (A: 160,804 reads, 29.62%; B: 47,821 reads, 15.22%) is discernible in Figure 4. Interestingly, while the relative abundance of Francisellaceae in Group B (21.24%) surpasses that in Group A (0.22%), no statistical difference in microbiome composition at the family level is noted (p = 0.55, Wilcoxon rank-sum test) as depicted in Figure 2E. A comparative analysis of the Ixodes microbiome diversity reveals greater species evenness in Group B (0.628) relative to Group A (0.520) based on the Simpson index, while the Shannon index suggests that the species richness in Group A (1.044) is lower than in Group B (1.363) as shown in Supplementary Table 4.
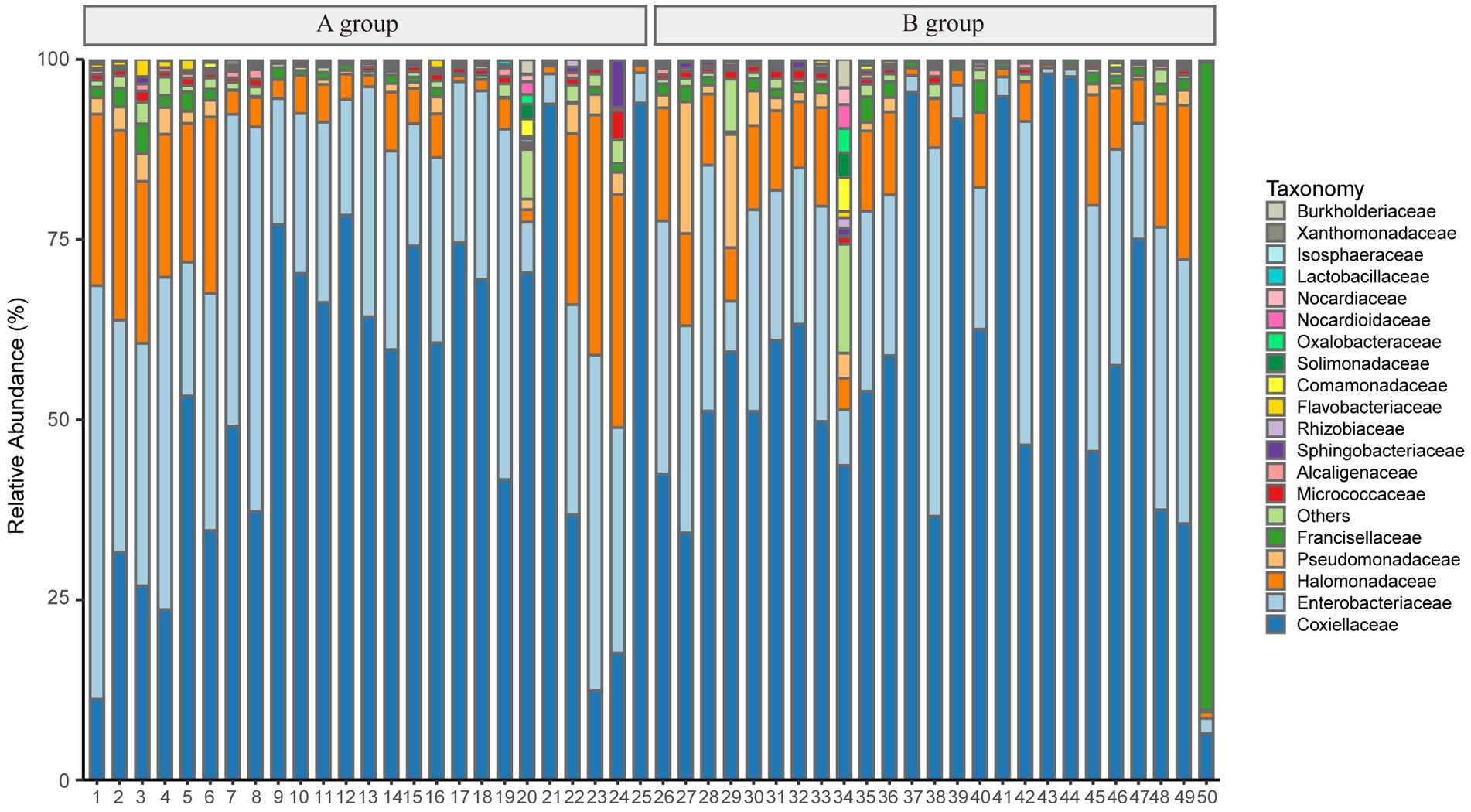
Figure 4. The plot shows the relative abundance of taxonomy after removing Rickettsiaceae at the family level.
Principal coordinate analysis identifies the species basically similar between the groups. The Unweighted UniFrac PCoA further reveals that PC1 (26.44%) surpasses PC2 (9.34%), and in the Weighted UniFrac PCoA plot, PC2 (36.13%) is inferior to PC1 (46.65%), as represented in Figures 5A,B. A comparison of the relative abundance of the top 20 bacterial genera using the Welch’s t-test (two sides), identifies no significant differences at the family level within the 95% confidence intervals, as shown in Figure 6A. While Enterobacteriaceae is marginally more prevalent in Group A than Group B, the mean proportion of Francisellaceae and Coxiellaceae is comparatively diminished. However, substantial differences become apparent at the genus level as shown in Figure 6B, with the result of the relative abundance of Friedmanniella and Bordetella being elevated in parasitic ticks as compared to questing ticks. Figure 7A demonstrates differences among specimens at p < 0.05 as determined by LEfSe analysis, and a slight discrepancy is detectable within 95% confidence intervals from phylum to species in Figure 7B, with 11 taxa in Group B and 10 taxa in Group A detected at higher relative abundance.
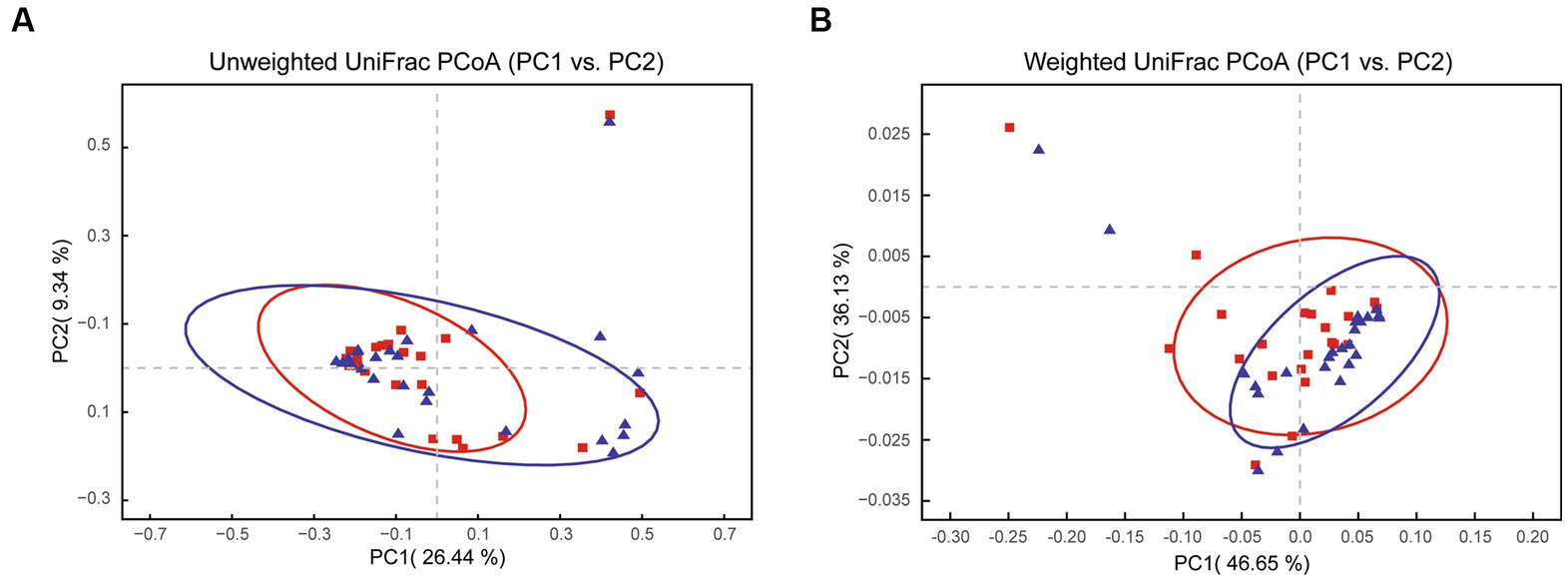
Figure 5. The plot shows PCoA results between groups after removing Rickettsiaceae. (A) The Unweighted UniFrac PCoA graph shows PC1 (26.44% variation) and PC2 (9.34% variation). (B) The Weighted UniFrac PCoA graph shows PC1 (46.65% variation) and PC2 (36.13% variation).
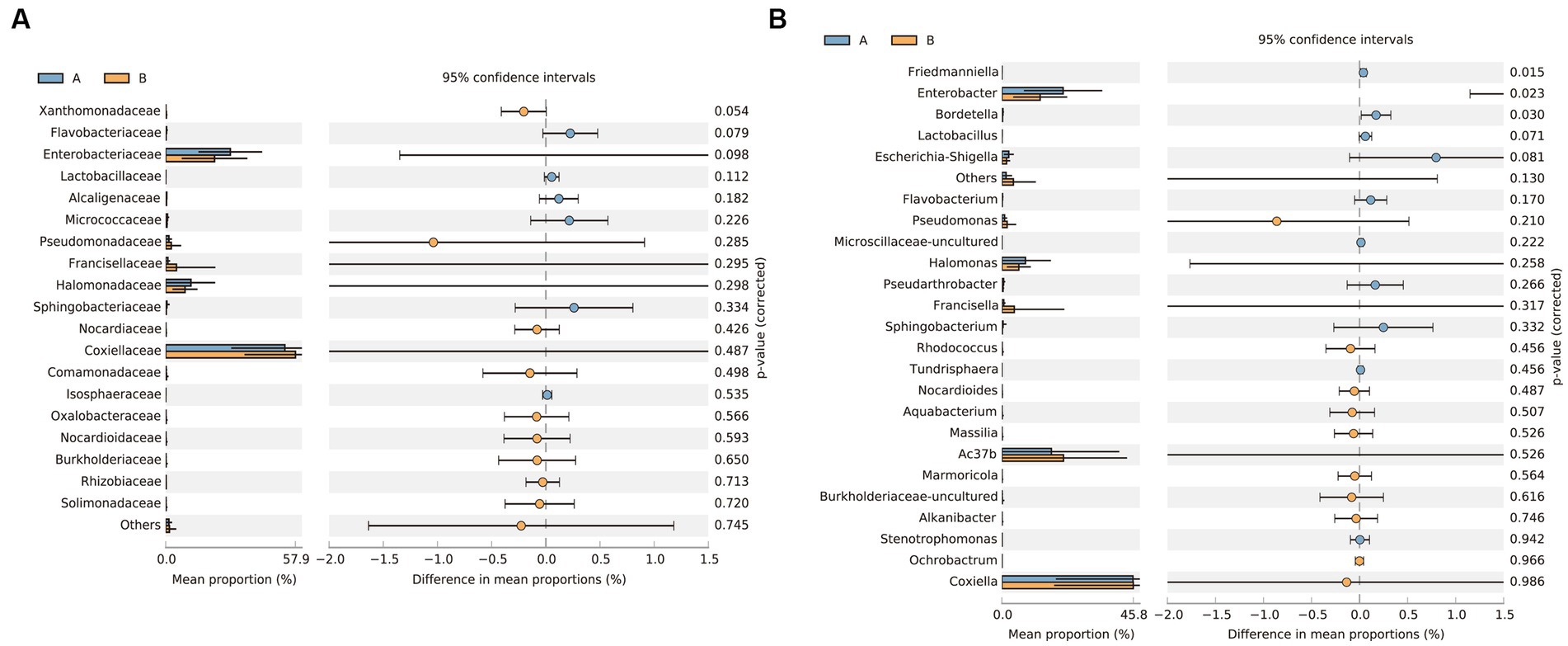
Figure 6. The plot shows the results of the Welch’s t-test between groups after removing Rickettsiaceae. (A) Differences of bacterial composition in 95% confidence intervals at the family level. (B) Differences of bacterial composition in 95% confidence intervals at the genus level.
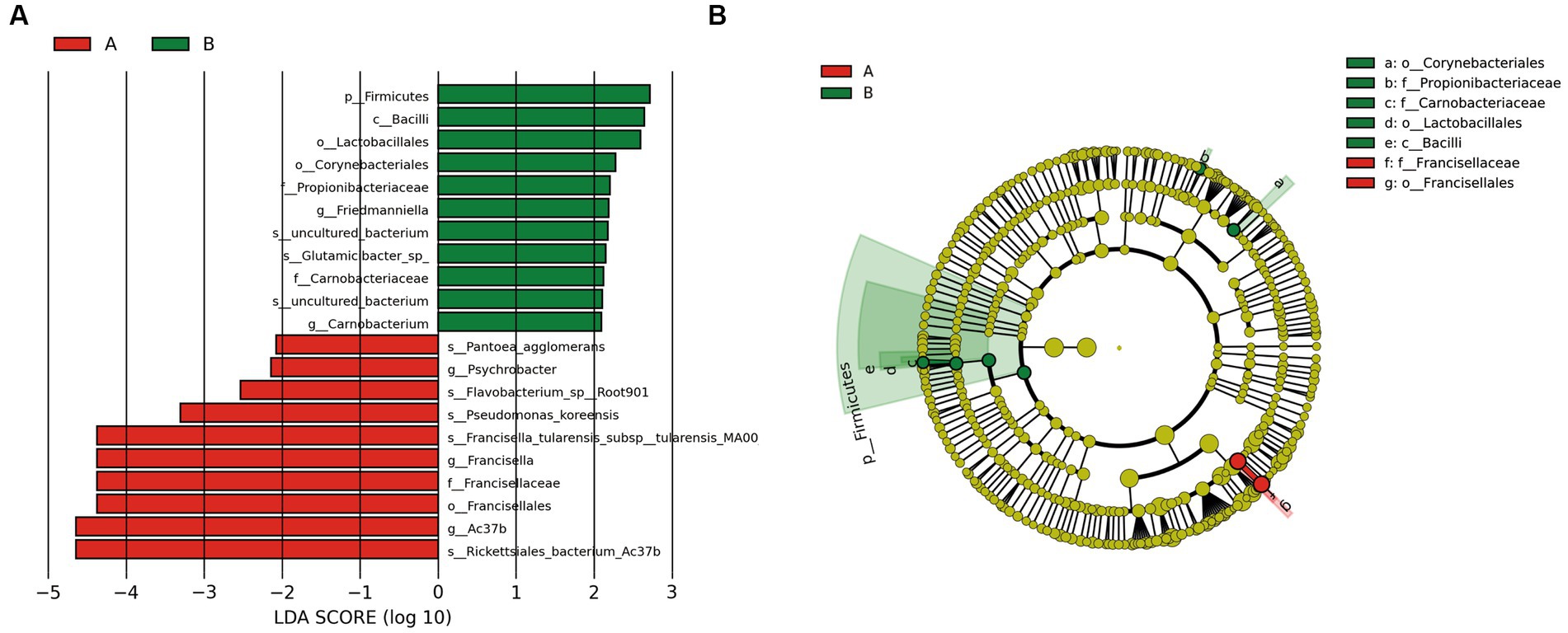
Figure 7. The plot shows LEFSe results between groups after removing Rickettsiaceae. (A) The bar plot explains the contribution degree of distinct species (LDA score > 2, p < 0.05). (B) The inner to outer circle of the cladistic map of distinct species evolution represents the classification level from phylum to family.
3.6 Phylogenetic tree and bacterial network
For the selected representative reads from Rickettsia, Anaplasma, Francisella, and Coxiella, the phylogenetic tree, as shown in Figure 8, manifests sequences alignment implemented with MEGA 11 software, utilizing the Kimura two-parameter model of Neighbor-Joining method. Table 2 presents the average intraspecies pairwise genetic distances for the four Rickettsia and 14 reference sequences retrieved from NCBI, which range from 0.045 to 0.173 and 0.000 to 0.012, respectively. The table also indicates the pairwise divergence (Divergence = 1 − percent identity) between stains, denoted above the diagonal in Table 2.
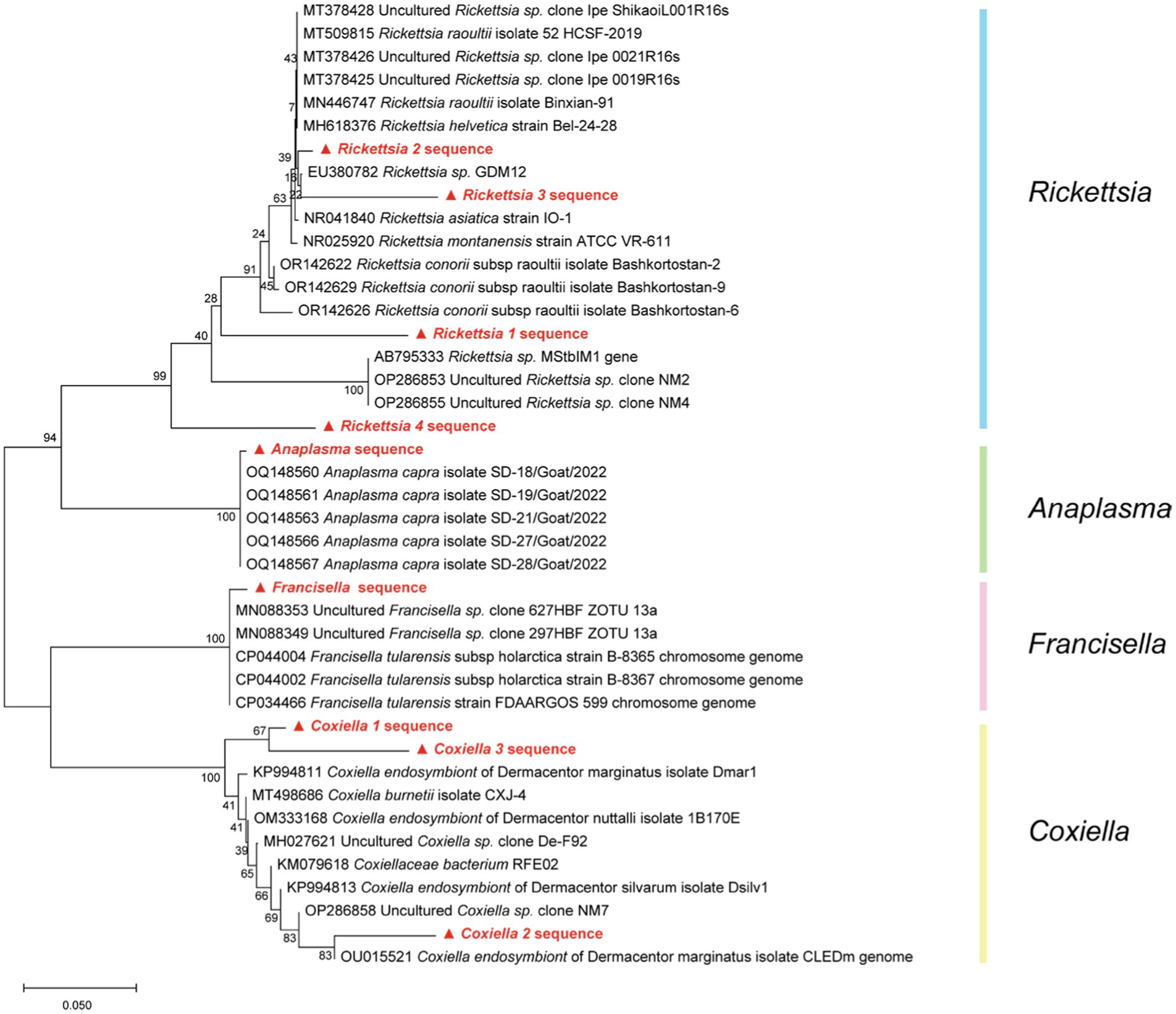
Figure 8. Based on the Kimura two-parameter model of Neighbor-Joining method, the phylogenetic tree of four pathogens (Rickettsia, Anaplasma, Francisella, and Coxiella) caused by NCBI blast result was drawn with 1,000 repeated estimated bootstrap values.
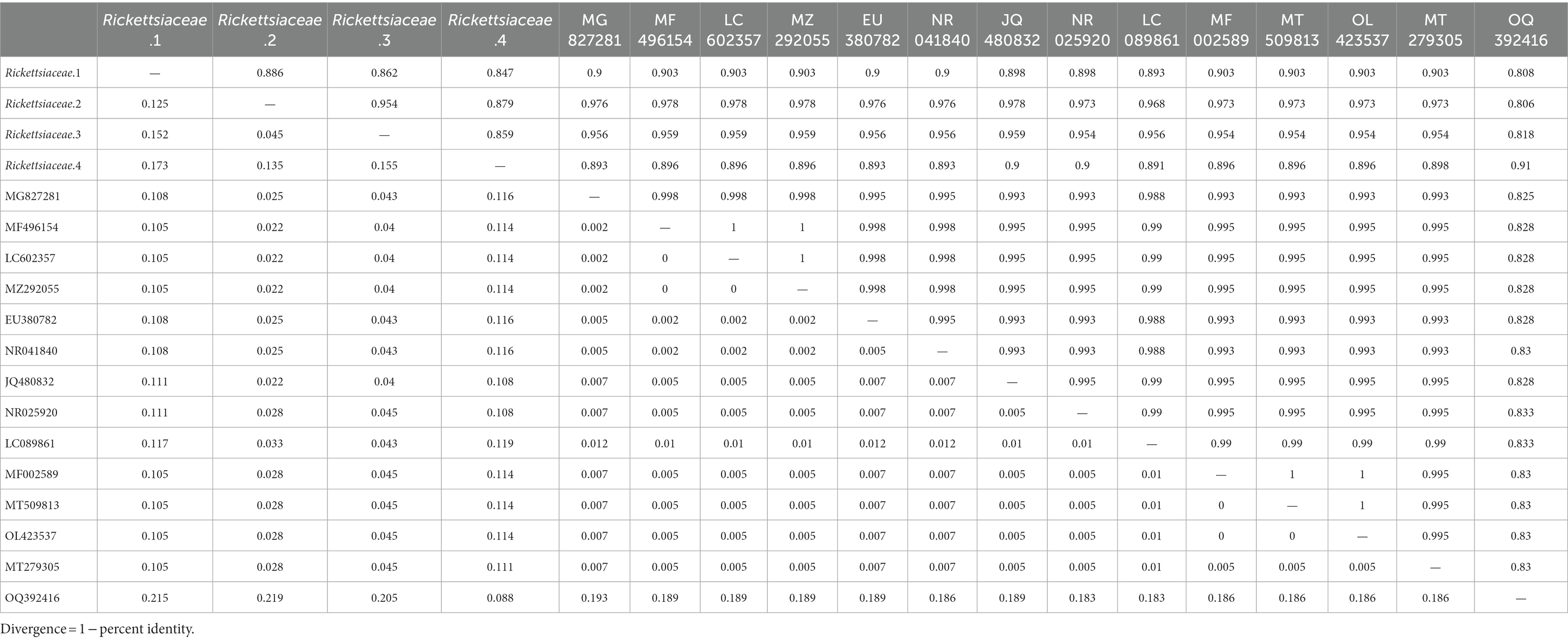
Table 2. Genetic distance (above the diagonal) and pairwise divergence (below the diagonal) in the Rickettsia sequences with NCBI reference sequence based on the phylogenetic tree.
The network, as depicted in Figure 9A, illustrates the correlational relationship among bacterial genera based on co-occurrence patterns, with varying line colors representing different relationships between two genera. The network consists of 36 nodes and 133 links, with only two instances of negative correlation noted between Francisella and Phyllobacterium (r = −0.65), and between Francisella and Arthrobacter (r = −0.63). There are 22 pairs exhibiting strong positive correlation (r > 0.8), with the top three pairs being Sphingobacteriales-uncultured and Phyllobacterium (r = 0.88), Blastocatellia-uncultured and Propionibacteriaceae-uncultured (r = 0.92), and Enterobacter and Escherichia-Shigella (r = 0.98). The detailed results for all bacteria are provided in Supplementary Table 5. In order to examine the correlation between bacterial genera across groups, we plotted network diagrams for Group A (Figure 9B) and Group B (Figure 9C). And there are 390 correlational relationships among bacteria in Group A and more than 350 in Group B, which are shown in Supplementary Tables 6, 7.
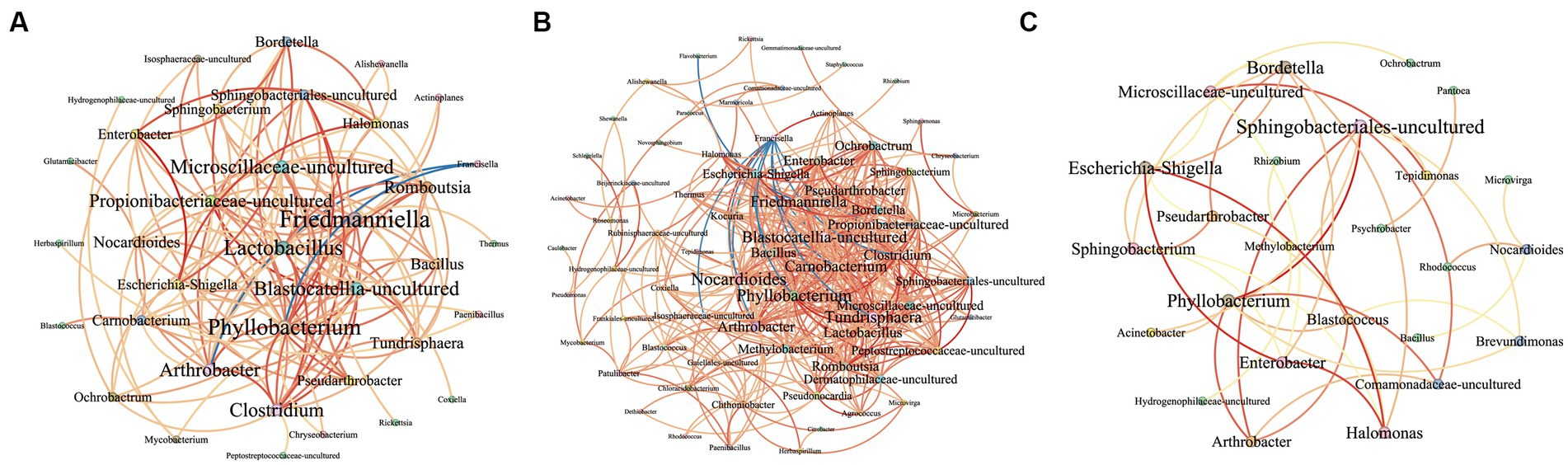
Figure 9. This image presents an interrelationship network among various bacterial genera at the genus level. Negative and positive correlations are represented by blue and red lines, respectively. The gradation from yellow to red in the lines signifies the degree of correlation, with a deeper red indicating a stronger correlation. The size of the bacterial names corresponds to the number of relationships each has within the network, with larger names indicating a greater number of connections. (A) The network shows the correlations of bacteria in all samples. (B) The network shows the correlations of bacteria in Group A. (C) The network shows the correlations of bacteria in Group B.
4 Discussion
Ticks, widely distributed blood-sucking parasitic arthropod, can be categorized into feeding ticks and questing ticks based on their living environment. While numerous studies have compared microbial compositions of various tick species morphologically (Krige et al., 2021), the presence of definitive differences in composition and diversity of abundance between feeding and questing ticks remains a debate topic. Our study, however, elucidates the presence of diverse compositions within groups, indicating the value in comparing two ticks of the same species and from the same sampling site.
Ixodidae species are widely distributed in Wuwei city, such as Hyalurus asiaticus, Haemaphysalis qinghaiensis, Dermacentor nuttalli, and Lycoris argenteus (Sun et al., 2016), and our molecular biological results for Dermacentor nuttalli align with the ecological species at the sampling site. In contrast to a study conducted in Inner Mongolia (Jiao et al., 2021), which detected the bacterial genera Rickettsia, Anaplasma, and Coxiella in D. nuttalli, our research detected the same bacteria in D. nuttalli, indicating that this species of D. nuttalli is predisposed to carry these bacteria. However, the detection rate of Anaplasma (4.67%, 7/150) in our results was lower than in the former study, where Anaplasma spp. was consistently reported and speculated to be a dominant regional species in Inner Mongolia (Jiao et al., 2021). This suggests that greater attention should be directed toward D. nuttalli in Wuwei City for comprehensive microorganism investigation, and the sample size, sampling points, and range of ticks should be expanded in further research.
In the relative abundance graph (Figure 3A), the family Rikettsiaceae constitutes the largest proportion, accounting for 81.11% in Group A and 87.71% in Group B, primarily including the genera Rickettsia and Ac37b at the genus level. Rickettsia peculiarly grows only within the cytoplasm of eucaryotic cells without producing exotoxins, and destructs host-cells from the inside, which are at high risk of infection as obligate intracellular bacteria first described by Ricketts in 1909 (Ricketts, 1909). After dengue infection, rickettsial diseases have been reported as the second most common cause of non-malarial febrile infectious illness in Southeast Asia (Acestor et al., 2012). One study reported a significantly higher prevalence of Rickettsia spp. in the genus Dermacentor than in the other tick species (Del Cerro et al., 2022), and a Malaysian study demonstrated that Rickettsia RF2125 plays a dominant role in both feeding [31.8% (7/22)] and questing [25.0% (19/76)] ticks (Kho et al., 2019), which aligns with our research that Rickettsia exhibited the highest relative abundance and implying a propensity for Dermacentor to harbor Rickettsia spp.
The blood-feeding behavior of ticks may explain the observed statistical difference between Friedmanniella and Bordetella in detected bacteria, which may be largely attributable to the host’s herbivorous traits and potential respiratory diseases. Utilizing the Welch’s t-test and LEfSe to detect possible genus-level differences, we found that Friedmanniella, a genus belonging to the Propionibacteriaceae family frequently detected in plant samples, exhibits significant differences between groups. Group A contained more reads than Group B (p = 0.015). Likewise, the Bordetella, an animal-associated genus known to cause whooping cough (Bordetella pertussis), had more reads in Group A and the difference was significant (p = 0.030). Extensive research into Bordetella has focused on the respiratory pathogens B. pertussis and B. bronchiseptica (Stevenson and Roberts, 2003), and they cause respiratory illness in children and animals including mice, dogs, pigs, and poultry, respectively (Trainor et al., 2015; Nieves and Heininger, 2016). Transmission of Bordetellae is typically attributed to respiratory droplets from coughing patients (Nieves and Heininger, 2016) and does not normally invade beyond the respiratory tract (Stevenson and Roberts, 2003). The bacteria can colonize in the respiratory tract, blood, and body fluids (such as cerebrospinal fluid; Liao et al., 2022), and B. bronchiseptica persist in a variety of environments outside the host, including water and on surfaces (Porter et al., 1991). Though no current reports manifest the presence of Bordetella in arthropods, our findings imply that Dermacentor nuttalli could be a potential vector for Bordetella, contradicting other reports that solely suggest transmission and infectious in mammals (Mattoo and Cherry, 2005). However, 75% ethyl alcohol may not be able to kill all pathogens on the surface of ticks, resulting in bacteria contaminating their surfaces, which requires further study to verify ticks as the vector of Bordetella.
Although our research detected three ticks for pathogens in one sample, it is possible that pathogens are co-infected in one tick. Tick-borne co-infections result from infection by genetically distinct pathogens (Cutler et al., 2021). In our study, the simultaneous detection rate of Coxiella, Francisella, and Rickettsia was 79.59% (39/49), as demonstrated in Figure 3C, and the prevalence indicates that these genera are remarkably commonplace in multiple tick species and possibly function as symbionts within ticks (Abreu et al., 2019). Indeed, co-infections and symbiote relationships are frequently observed among arthropods such as ticks, ranging from closely related variants within the same species to highly diverse pathogens, including parasites, bacteria, and viruses (Cutler et al., 2021). One research indicates a substantial presence of 90% prevalence rate (153/170) of R. africae in Amblyomma variegatum ticks and harbored a combination of pathogens, notably including Coxiella burnetii among others (Ehounoud et al., 2016). Furthermore, corroborative research has provided evidence of simultaneous infection with two different types of Rickettsia (namely Rickettsia spp. and Rickettsiella spp.) in cases (Raulf et al., 2018). Other bacteria of notable prevalence are Coxiella and Francisella, which have been reported to exist symbiotically in ticks such as Rickettsia-like, Coxiella-like, and Francisella-like endosymbiont (Song et al., 2022), and potentially play a crucial role in tick development by supplying B vitamins (Brenner et al., 2021).
Networks show the interrelationship between bacteria and Supplementary Tables 6, 7 present the detailed correlation results of groups. The Francisella appears to be inhibited or compete with 10 bacterial genera such as Carnobacterium (r = −0.77) and Phyllobacterium (r = −0.70), and the Flavobacterium inhibit Friedmanniella bacteria in Group A (r = −0.79). Conversely, no such competitive relations are observed in Group B. This phenomenon may be related to the competition between bacteria inherently present (such as Francisella) in questing ticks and those acquired from the host’s blood by parasitic ticks. Notably, strong positive correlation relationships (r > 0.8) are evident in both groups with the result between Phyllobacterium and Arthrobacter (Group A = 0.81, Group B = 0.86), Phyllobacterium and Sphingobacteriales-uncultured (Group A = 0.84, Group B = 0.99). And the bacteria Halomonas, Escherichia-Shigella, and Enterobacter have strong positive relationships in two groups, which three bacteria may promote growth mutually. It has been also reported that the three bacteria can be detected in animals simultaneously, such as cows, Haemonchus contortus, and Scylla paramamosain (Zhang et al., 2018; Chen et al., 2020; Mafuna et al., 2021). Interestingly, Bordetella has strong positive correlativity with the bacteria Halomonas (r = 0.81), Enterobacter (r = 0.90), and Escherichia-Shigella (r = 0.92) in Group A. Three bacteria, with more abundance in Group A, probably promoted the colonization and growth of Bordetella, which may related to the phenomenon that the Bordetella has significant differences between two groups.
Our study acknowledges that the evidence focusing on just one sampling site, one city, and the same tick species may be less sufficient. Additionally, the interactive mechanisms of symbiotic bacteria were not examined and verified. Nonetheless, our work yields significant findings. The bacterial genera Friedmanniella and Bordetella have statistical differences between parasitic and questing ticks, and the interrelationships in bacteria are different in diverse lifestyles ticks. In conclusion, our comparison of parasitic and questing ticks provides unique perspective into diversities in pathogen proportion and microbial communities, leveraging the 16S rRNA gene sequencing and analysis capabilities of NGS. In further research, the sampling range should be expanded and the mechanisms between bacterial genera warrant resolution and optimization.
5 Conclusion
The conducted experimental investigations have elucidated differences in the causative agents present in two lifestyle categories of ticks within Wuwei City. It was found that microorganisms demonstrate significant variations between parasitic and questing ticks, with the potential for bacterial genera to either inhibit or promote each other within diverse tick populations. These results underscore significance of lifestyle-based classification in researching potential pathogens.
Data availability statement
The datasets presented in this study can be found in online repositories. The names of the repository/repositories and accession number(s) can be found below: NCBI—PRJNA1015185.
Ethics statement
The manuscript presents research on animals that do not require ethical approval for their study.
Author contributions
LZ: Data curation, Formal analysis, Writing – original draft, Writing – review & editing. JH: Formal analysis, Investigation. QZ: Methodology, Supervision. ZH: Methodology, Writing – review & editing. S-WS: Methodology, Resources, Writing – review & editing. RL: Data curation, Resources, Writing – review & editing. R-SL: Investigation, Visualization, Writing – review & editing. W-KZ: Project administration, Validation, Writing – review & editing. Y-HW: Methodology, Software, Writing – review & editing. L-LX: Investigation, Supervision, Writing – review & editing. Z-HL: Investigation, Software, Writing – review & editing. Z-JS: Conceptualization, Funding acquisition, Supervision, Writing – review & editing.
Funding
The author(s) declare financial support was received for the research, authorship, and/or publication of this article. This work was supported by a grant from the National Natural Science Foundation of China (Grant No. 82273689).
Conflict of interest
The authors declare that the research was conducted in the absence of any commercial or financial relationships that could be construed as a potential conflict of interest.
Publisher’s note
All claims expressed in this article are solely those of the authors and do not necessarily represent those of their affiliated organizations, or those of the publisher, the editors and the reviewers. Any product that may be evaluated in this article, or claim that may be made by its manufacturer, is not guaranteed or endorsed by the publisher.
Supplementary material
The Supplementary material for this article can be found online at: https://www.frontiersin.org/articles/10.3389/fmicb.2023.1264939/full#supplementary-material
Footnotes
References
Abreu, D. P. B., Peixoto, M. P., Luz, H. R., Zeringóta, V., Santolin, Í., Famadas, K. M., et al. (2019). Two for the price of one: co-infection with Rickettsia bellii and spotted fever group Rickettsia in Amblyomma (Acari: Ixodidae) ticks recovered from wild birds in Brazil. Ticks Tick Borne Dis 10:101266. doi: 10.1016/j.ttbdis.2019.101266
Acestor, N., Cooksey, R., Newton, P. N., Ménard, D., Guerin, P. J., Nakagawa, J., et al. (2012). Mapping the aetiology of non-malarial febrile illness in Southeast Asia through a systematic review--terra incognita impairing treatment policies. PLoS One 7:e44269. doi: 10.1371/journal.pone.0044269
Brenner, A. E., Muñoz-Leal, S., Sachan, M., Labruna, M. B., and Raghavan, R. (2021). Coxiella burnetii and related tick endosymbionts evolved from pathogenic ancestors. Genome Biol. Evol. 13. doi: 10.1093/gbe/evab108
Caporaso, J. G., Kuczynski, J., Stombaugh, J., Bittinger, K., Bushman, F. D., Costello, E. K., et al. (2010). QIIME allows analysis of high-throughput community sequencing data. Nat. Methods 7, 335–336. doi: 10.1038/nmeth.f.303
Chaorattanakawee, S., Wofford, R. N., Takhampunya, R., Katherine Poole-Smith, B., Boldbaatar, B., Lkhagvatseren, S., et al. (2022). Tracking tick-borne diseases in Mongolian livestock using next generation sequencing (NGS). Ticks Tick Borne Dis 13:101845. doi: 10.1016/j.ttbdis.2021.101845
Chen, H., Fu, K., Pang, B., Wang, J., Li, H., Jiang, Z., et al. (2020). Determination of uterine bacterial community in postpartum dairy cows with metritis based on 16S rDNA sequencing. Vet. Anim. Sci. 10:100102. doi: 10.1016/j.vas.2020.100102
Ciebiera, O., Łopińska, A., and Gabryś, G. (2021). Ticks on game animals in the fragmented agricultural landscape of western Poland. Parasitol. Res. 120, 1781–1788. doi: 10.1007/s00436-021-07132-9
Csardi, G., and Nepusz, T. (2005). The igraph software package for complex network research. InterJ. Complex Syst. 1695, 1–9.
Cutler, S. J., Vayssier-Taussat, M., Estrada-Peña, A., Potkonjak, A., Mihalca, A. D., and Zeller, H. (2021). Tick-borne diseases and co-infection: current considerations. Ticks Tick Borne Dis 12:101607. doi: 10.1016/j.ttbdis.2020.101607
Del Cerro, A., Oleaga, A., Somoano, A., Barandika, J. F., García-Pérez, A. L., and Espí, A. (2022). Molecular identification of tick-borne pathogens (Rickettsia spp., Anaplasma phagocytophilum, Borrelia burgdorferi sensu lato, Coxiella burnetii and piroplasms) in questing and feeding hard ticks from North-Western Spain. Ticks Tick Borne Dis 13:101961. doi: 10.1016/j.ttbdis.2022.101961
Dixon, P. (2003). VEGAN, a package of R functions for community ecology. J. Veg. Sci. 14, 927–930. doi: 10.1111/j.1654-1103.2003.tb02228.x
Edgar, R. C., Haas, B. J., Clemente, J. C., Quince, C., and Knight, R. (2011). UCHIME improves sensitivity and speed of chimera detection. Bioinformatics 27, 2194–2200. doi: 10.1093/bioinformatics/btr381
Ehounoud, C. B., Yao, K. P., Dahmani, M., Achi, Y. L., Amanzougaghene, N., Kacou N'Douba, A., et al. (2016). Multiple pathogens including potential new species in tick vectors in Côte d'Ivoire. PLoS Negl. Trop. Dis. 10:e0004367. doi: 10.1371/journal.pntd.0004367
Jiao, J., Lu, Z., Yu, Y., Ou, Y., Fu, M., Zhao, Y., et al. (2021). Identification of tick-borne pathogens by metagenomic next-generation sequencing in Dermacentor nuttalli and Ixodes persulcatus in Inner Mongolia, China. Parasit. Vectors 14:287. doi: 10.1186/s13071-021-04740-3
Kahl, O. (2018). Hard ticks as vectors-some basic issues. Wien. Klin. Wochenschr. 130, 479–483. doi: 10.1007/s00508-018-1360-x
Kho, K. L., Tan, P. E., and Tay, S. T. (2019). Diversity of Rickettsiae in feeding and questing ticks collected from a Malaysian Forest reserve area. J. Med. Entomol. 56, 547–552. doi: 10.1093/jme/tjy168
Krige, A. S., Thompson, R. C. A., Seidlitz, A., Keatley, S., Wayne, J., and Clode, P. L. (2021). Molecular detection of Trypanosoma spp. in questing and feeding ticks (Ixodidae) collected from an endemic region of south-West Australia. Pathogens 10:1037. doi: 10.3390/pathogens10081037
Lan-Hua, L., and Yi, Z. (2019). Prevalence and control of tick-borne parasitic diseases in China. Chin. J. Schistosom. Control 31, 58–62. doi: 10.16250/j.32.1374.2018310
Liao, Y., Li, W. R., Zhu, Y., Luo, S. H., Liao, Q., and Wan, C. M. (2022). Invasive Bordetella pertussis infection in infants: a case report. Open Forum Infect. Dis. 9:ofac478. doi: 10.1093/ofid/ofac478
Lv, J., Wu, S., Zhang, Y., Chen, Y., Feng, C., Yuan, X., et al. (2014). Assessment of four DNA fragments (COI, 16S rDNA, ITS2, 12S rDNA) for species identification of the Ixodida (Acari: Ixodida). Parasit. Vectors 7:93. doi: 10.1186/1756-3305-7-93
Mafuna, T., Soma, P., Tsotetsi-Khambule, A. M., Hefer, C. A., Muchadeyi, F. C., Thekisoe, O. M. M., et al. (2021). Bacterial profiling of Haemonchus contortus gut microbiome infecting Dohne merino sheep in South Africa. Sci. Rep. 11:5905. doi: 10.1038/s41598-021-85282-7
Mattoo, S., and Cherry, J. D. (2005). Molecular pathogenesis, epidemiology, and clinical manifestations of respiratory infections due to Bordetella pertussis and other Bordetella subspecies. Clin. Microbiol. Rev. 18, 326–382. doi: 10.1128/CMR.18.2.326-382.2005
McMurdie, P. J., and Holmes, S. (2013). Phyloseq: an R package for reproducible interactive analysis and graphics of microbiome census data. PLoS One 8:e61217. doi: 10.1371/journal.pone.0061217
Neuwirth, E. (2022). RColorBrewer: ColorBrewer palettes. R package version 1.1-3. Available at: https://CRAN.R-project.org/package=RColorBrewer
Nieves, D. J., and Heininger, U. (2016). Bordetella pertussis. Microbiol Spectr 4:21. doi: 10.1128/microbiolspec.EI10-0008-2015
Odendaal, M. L., Groot, J. A., Hasrat, R., Chu, M., Franz, E., Bogaert, D., et al. (2022). Higher off-target amplicon detection rate in MiSeq v3 compared to v2 reagent kits in the context of 16S-rRNA-sequencing. Sci. Rep. 12:16489. doi: 10.1038/s41598-022-20573-1
Parks, D. H., Tyson, G. W., Hugenholtz, P., and Beiko, R. G. (2014). STAMP: statistical analysis of taxonomic and functional profiles. Bioinformatics 30, 3123–3124. doi: 10.1093/bioinformatics/btu494
Porter, J. F., Parton, R., and Wardlaw, A. C. (1991). Growth and survival of Bordetella bronchiseptica in natural waters and in buffered saline without added nutrients. Appl. Environ. Microbiol. 57, 1202–1206. doi: 10.1128/aem.57.4.1202-1206.1991
Raulf, M. K., Jordan, D., Fingerle, V., and Strube, C. (2018). Association of Borrelia and Rickettsia spp. and bacterial loads in Ixodes ricinus ticks. Ticks Tick Borne Dis 9, 18–24. doi: 10.1016/j.ttbdis.2017.10.014
Ricketts, H. T. (1909). A MICRO-organism which apparently has a specific relationship to ROCKY mountain spotted fever: a PRELIMINARY REPORT. J. Am. Med. Assoc. LII, 379–380. doi: 10.1001/jama.1909.25420310039002,M.D
Rochlin, I., and Toledo, A. (2020). Emerging tick-borne pathogens of public health importance: a mini-review. J. Med. Microbiol. 69, 781–791. doi: 10.1099/jmm.0.001206
Rodino, K. G., Theel, E. S., and Pritt, B. S. (2020). Tick-borne diseases in the United States. Clin. Chem. 66, 537–548. doi: 10.1093/clinchem/hvaa040
Rodino, K. G., Wolf, M. J., Sheldon, S., Kingry, L. C., Petersen, J. M., Patel, R., et al. (2021). Detection of tick-borne Bacteria from whole blood using 16S ribosomal RNA gene PCR followed by next-generation sequencing. J. Clin. Microbiol. 59. doi: 10.1128/JCM.03129-20
Sanschagrin, S., and Yergeau, E. (2014). Next-generation sequencing of 16S ribosomal RNA gene amplicons. J. Vis. Exp. 90. doi: 10.3791/51709
Segata, N., Izard, J., Waldron, L., Gevers, D., Miropolsky, L., Garrett, W. S., et al. (2011). Metagenomic biomarker discovery and explanation. Genome Biol. 12:R60. doi: 10.1186/gb-2011-12-6-r60
Shen, L., Huang, F., Chen, X., Xiong, Z., Yang, X., Li, H., et al. (2020). Diagnostic efficacy of three test kits for SARS-CoV-2 nucleic acid detection. Zhejiang Da Xue Xue Bao Yi Xue Ban 49, 185–190. doi: 10.3785/j.issn.1008-9292.2020.03.09
Song, X., Zhong, Z., Gao, L., Weiss, B. L., and Wang, J. (2022). Metabolic interactions between disease-transmitting vectors and their microbiota. Trends Parasitol. 38, 697–708. doi: 10.1016/j.pt.2022.05.002
Stevenson, A., and Roberts, M. (2003). Use of Bordetella bronchiseptica and Bordetella pertussis as live vaccines and vectors for heterologous antigens. FEMS Immunol. Med. Microbiol. 37, 121–128. doi: 10.1016/S0928-8244(03)00068-3
Sun, M., Guan, G., Liu, Z., Wang, J., Wang, D., Wang, S., et al. (2020). Molecular survey and genetic diversity of Babesia spp. and Theileria spp. in cattle in Gansu Province, China. Acta Parasitol. 65, 422–429. doi: 10.2478/s11686-020-00179-1
Sun, M., Wang, S. F., Wang, D. Q., Yang, Y. B., and Ma, C. (2016). Preliminary investigation on species and distribution of ticks and tick-borne diseases in Wuwei City, Gansu Province. Anim. Quarant. China 33, 23–25. doi: 10.3969/j.issn.1005-944X.2016.02.010
Sun, Y., Xu, R., Liu, Z., Wu, M., and Qin, T. (2019). Ornithodoros (Ornithodoros) huajianensis sp. nov. (Acari, argasidae), a new tick species from the Mongolian marmot (Marmota bobak sibirica), Gansu province in China. Int J Parasitol Parasites Wildlife 9, 209–217. doi: 10.1016/j.ijppaw.2019.05.001
Trainor, E. A., Nicholson, T. L., and Merkel, T. J. (2015). Bordetella pertussis transmission. Pathog Dis 73:ftv068. doi: 10.1093/femspd/ftv068
Keywords: parasitic tick, questing tick, symbiotic bacteria, microbiome analysis, network diagram
Citation: Zhang L, Han J, Zhou Q, He Z, Sun S-W, Li R, Li R-S, Zhang W-K, Wang Y-H, Xu L-L, Lu Z-H and Shao Z-J (2023) Differential microbial composition in parasitic vs. questing ticks based on 16S next-generation sequencing. Front. Microbiol. 14:1264939. doi: 10.3389/fmicb.2023.1264939
Edited by:
Digvijay Verma, Babasaheb Bhimrao Ambedkar University, IndiaReviewed by:
Rosa Estela Quiroz Castañeda, Instituto Nacional de Investigaciones Forestales, Agrícolas y Pecuarias (INIFAP), MexicoJianmin Zhong, Cal Poly Humboldt, United States
Copyright © 2023 Zhang, Han, Zhou, He, Sun, Li, Li, Zhang, Wang, Xu, Lu and Shao. This is an open-access article distributed under the terms of the Creative Commons Attribution License (CC BY). The use, distribution or reproduction in other forums is permitted, provided the original author(s) and the copyright owner(s) are credited and that the original publication in this journal is cited, in accordance with accepted academic practice. No use, distribution or reproduction is permitted which does not comply with these terms.
*Correspondence: Zhen-Hua Lu, bHVja3kxODcxMDM2MDMxMEAxNjMuY29t; Zhong-Jun Shao, MTM3NTk5ODE3ODNAMTYzLmNvbQ==
†Author note