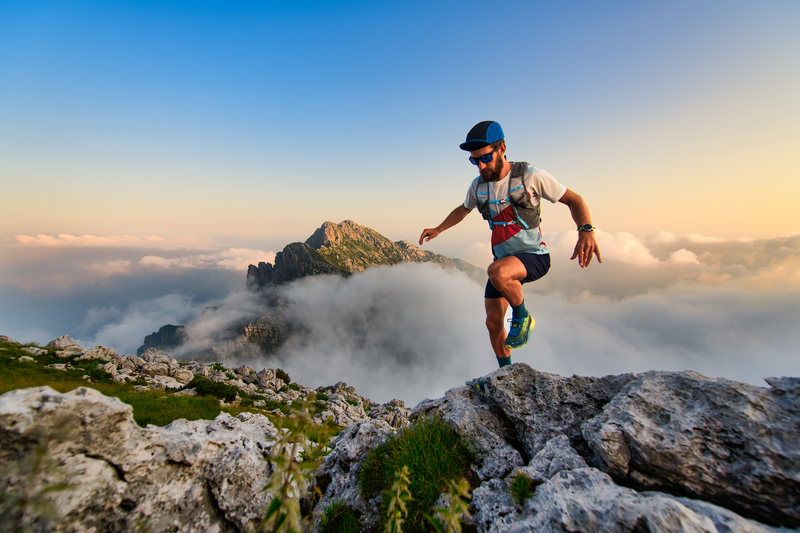
94% of researchers rate our articles as excellent or good
Learn more about the work of our research integrity team to safeguard the quality of each article we publish.
Find out more
ORIGINAL RESEARCH article
Front. Microbiol. , 19 October 2023
Sec. Microbe and Virus Interactions with Plants
Volume 14 - 2023 | https://doi.org/10.3389/fmicb.2023.1241076
This article is part of the Research Topic Studying Plant Virus-Host Interactions: Elucidating the Natural Resistance Mechanism View all 6 articles
As a high-value oilseed crop, soybean [Glycine max (L.) Merr.] is limited by various biotic stresses during its growth and development. Soybean mosaic virus (SMV) is a devastating viral infection of soybean that primarily affects young leaves and causes significant production and economic losses; however, the synergistic molecular mechanisms underlying the soybean response to SMV are largely unknown. Therefore, we performed RNA sequencing on SMV-infected resistant and susceptible soybean lines to determine the molecular mechanism of resistance to SMV. When the clean reads were aligned to the G. max reference genome, a total of 36,260 genes were identified as expressed genes and used for further research. Most of the differentially expressed genes (DEGs) associated with resistance were found to be enriched in plant hormone signal transduction and circadian rhythm according to Kyoto Encyclopedia of Genes and Genomes analysis. In addition to salicylic acid and jasmonic acid, which are well known in plant disease resistance, abscisic acid, indole-3-acetic acid, and cytokinin are also involved in the immune response to SMV in soybean. Most of the Ca2+ signaling related DEGs enriched in plant-pathogen interaction negatively influence SMV resistance. Furthermore, the MAPK cascade was involved in either resistant or susceptible responses to SMV, depending on different downstream proteins. The phytochrome interacting factor-cryptochrome-R protein module and the MEKK3/MKK9/MPK7-WRKY33-CML/CDPK module were found to play essential roles in soybean response to SMV based on protein-protein interaction prediction. Our findings provide general insights into the molecular regulatory networks associated with soybean response to SMV and have the potential to improve legume resistance to viral infection.
Glycine max is a high-quality source of vegetable protein and oil, as well as a major economic crop worldwide (Liu et al., 2012). However, various pathogens and insects have a significant impact on the productivity and quality of soybean. Among the diseases, SMV (genus Potyvirus, family Potyviridae), is a widespread virus that causes leaf discoloration, curling, and seed mottling (Yang et al., 2014). SMV can spread from the initial site of infection to nearby cells and is transmitted by numerous aphid vectors (Irwin and Goodman, 1981), symptoms caused by some SMV strains can also be transmitted by seed (Hobbs et al., 2003). Diseases caused by SMV are widespread in major soybean production areas around the world, causing production losses of up to 10–35% each year under field conditions (Ross, 1983; Yang et al., 2013), and are the most important disease affecting global soybean production.
Similar to other plant viruses, SMV infection in field-grown soybeans has been difficult to eradicate with chemical agents (Nakano et al., 1987; Pedersen et al., 2007), and the most effective strategy for controlling this disease is the use of resistant cultivars, which is both economical and environmentally friendly. Screening and discovery of resistance genes not only provides the basis for breeding resistant germplasms, but also provides insight into the detailed mechanisms of soybean-SMV interaction. Previous studies have focused on mapping major SMV resistance QTLs (quantitative trait loci), and a number of genetic loci have been identified (Yang and Gai, 2011; Karthikeyan et al., 2018; Yuan Y. et al., 2020). Rsv (resistance to SMV) loci, which comprise dominant resistance (R) genes (Hayes et al., 2000; Gunduz et al., 2002; Liao et al., 2002; Zheng et al., 2005; Cervantes-Martinez et al., 2015), with alleles Rsv1, Rsv3 and Rsv4 have been reported to be effective against several North American SMV strains (Wen et al., 2013). Resistance to the strains from China is derived from Rsc loci, mapped to chromosomes 2, 13, 14, and 6 in various resistant cultivars (Ma et al., 2011; Wang D. G. et al., 2011; Zheng et al., 2014; Rui et al., 2017). Another mechanism of plant resistance to viruses is referred to as recessive resistance, which is also exploited in crops (Truniger and Aranda, 2009; Wang and Krishnaswamy, 2012). Recessive resistance traits can be introduced into crop species by crossing, or by random mutagenesis and selection (Piron et al., 2010). Most recessive virus resistance genes isolated to date are the eukaryotic translation initiation factors 4E and 4G, and their isoforms (Hashimoto et al., 2016). Although there has been some progress in the study of soybean resistance to SMV, specific resistance genes and molecular mechanisms of the soybean defense signaling remain largely unknown.
To survive and prevent the invasion and proliferation of pathogens (nematodes, fungi, bacteria, and viruses), plants have developed a complex defense strategy that includes structural (such as cuticular wax, and xylogen deposition on the cell walls) (Kaur et al., 2022) and chemical barriers [such as phenols, saponins, GSLs (glucosinolates), and phytoalexins, etc.] (Zaynab et al., 2018). Once these defenses are breached, host plants immediately activate the PAMPs (pathogen-associated molecular patterns)-triggered immunity (PTI) system. PRRs (pattern recognition receptors) produced by plants can recognize and bind to conserved sequences in microbes, and inhibit pathogen growth (Jones and Dangl, 2006). In addition, effectors secreted by pathogens can activate ETI (effector-triggered immunity) (Howden and Huitema, 2012), resulting in HR (hypersensitive response) and expression of defense-related genes (Win et al., 2012). The transcriptional dynamics of R genes and hormone signaling induced by PTI and ETI are crucial for the defense response of plants against the pathogens (Moore et al., 2011).
In recent years, the development of high-throughput sequencing, and other “-omics” studies are providing new insights into the molecular mechanisms of plant defense against viruses and other pathogens (Chen et al., 2017; Awika et al., 2019; Liu et al., 2022). In soybean, the genes involved in SA (salicylic acid) signaling and members of the NBS-LRR (nucleotide binding site leucine-rich repeat) family were involved in SMV pathogenicity (Zhao et al., 2018). Comparative transcriptome analysis of soybean in response to two SMV strains (avirulent strain G5H and virulent strain G7H) revealed that the JA (jasmonic acid) pathway and WRKY transcription factors (TFs) need to be added after these two words. were associated with SMV infection (Alazem et al., 2018). RNA-seq analysis was also used to assess DEGs in soybean under normal and shaded light conditions to investigate the light-regulated response to SMV infection (Zhang L. et al., 2019). Taken together, these studies discussed the effects of hormones, viral strains, and environmental factors (light intensity and quality) on soybean response to SMV, respectively.
The response mechanism of plants to biotic stresses is highly complicated and requires the activation of several combined pathways. Previous studies indicated that the genes associated with cell wall modification, chitinase synthesis, Ca2+ signaling, and reactive oxygen gene activation were all significantly up-regulated in pathogen-resistant plants (Zou et al., 2021; Duan et al., 2022; Yang et al., 2022; Zhou et al., 2023). In soybean, the transcriptomic changes during SMV infection are not fully understood. Here, we used two soybean cultivars with different responses to SMV to investigate the physiological and transcriptional changes following SMV infection. Compared to previous studies, we aim to provide more comprehensive information for elucidating the complex regulatory networks associated with the soybean response to SMV. Meanwhile, it is of great theoretical and practical importance to improve disease resistance to SMV through molecular breeding or genetic engineering.
Two breeding lines of G. max, ‘Kefeng 1’ (resistant, R-line) and ‘NN1138-2’ (susceptible, S-line) were selected and grown in a greenhouse at 23–28°C with a 14-h light/10-h dark photoperiod. The SMV isolate 6067–1 (strain SC15, GenBank accession: JF833015.1) used in this study was previously collected and sequenced. Leaves of three-leaf stage seedlings were brushed with SMV buffer [prepared by grinding frozen SMV-infected soybean leaves with 600 mesh silicon carbide and 10 mM phosphate buffer (a mixture of NaH2PO4 and K2HPO4, pH = 7.4)]. An identical buffer without SMV inoculum was used concurrently for mock treatments. SMV-infected or mock-treated soybean leaves at 1 day post inoculation (dpi) were used as controls for the corresponding materials obtained at subsequent sampling points. Leaf samples taken at 1, 3, 5, 7, 9, and 11 dpi were used to determine SMV accumulation between the two soybean cultivars (three biological replicates per group). All samples were collected, immediately frozen in liquid nitrogen, and stored at −80°C prior to RNA extraction and further analysis.
Total RNA was extracted using RNAprep Pure Plant Kit (Tiangen, Beijing, China) following the manufacturer’s instructions. The extracted RNA was assessed by 1% agarose gel electrophoresis for degradation, K5800 micro-spectrophotometer (Kaiao, Beijing, China) for concentration and purity, and Bioanalyzer 2100 system (Agilent Technologies, Santa Clara, CA, United States) for integrity. A total of 2 μg RNA per sample was used for cDNA library construction. Sequencing libraries were generated using NEBNext® Ultra™ RNA Library Prep Kit for Illumina® (NEB, Ipswich, MA, United States) following the manufacturer’s instructions. The 48 libraries (RNA samples at 1, 3, 5, and 7 dpi in two cultivars, mock inoculation and virus inoculation, and three biological replicates per group) were sequenced on the Illumina Genome Analyzer IIx at Novogene Bioinformatics Technology Co., Ltd. (Beijing, China).
The raw data were filtered by removing the adaptor sequences, N-sequences, and low-quality reads with a quality score Q less than 20. The remaining reads were termed clean reads, and were assembled de novo using Trinity software (v2.0.6) with the k-mer size parameter set to 25 by default (Grabherr et al., 2011) to construct full-length transcripts. The longest transcript of each cluster was referred to as a unigene (Wang et al., 2013). The clean reads from each sample were aligned to the G. max reference genome1 (Schmutz et al., 2010). All unigenes were annotated by NR (NCBI non-redundant protein), Pfam (Protein family), GO (Gene Ontology), KEGG (Kyoto Encyclopedia of Genes and Genomes), and Swiss-Prot protein databases with e-value ≤10−5.
Gene expression levels were calculated by the FPKM (fragments per kilobase of transcript per million reads) method (Trapnell et al., 2010). The DEGs were analyzed using the DESeq2 R package (v1.18.0) (Love et al., 2014) and defined as unigenes with |log2 FC (fold changes)| ≥1, and adjusted p-values (padj) < 0.05. GO and KEGG enrichment analyzes were conducted to identify the biological functions of the DEGs using the GOseq R software package (Young et al., 2010) and DAVID Bioinformatics Resources2 (Sherman et al., 2022), respectively. Plant Transcription Factor Database3 (Jin et al., 2017) was used to identify TFs in all gene sequences. R genes were identified using all transcriptome data via the HMM search module in TBtools software (v1.33206.0.0) (Chen et al., 2020). To establish a protein interaction network, candidate proteins in G. max were used and analyzed by employing the online STRING database4 (Szklarczyk et al., 2021). The interaction network was visualized using Cytoscape 3.7.1 (Shannon et al., 2003), and the betweenness centrality (BC) value of each node was calculated using the CytoNCA plug-in (Tang et al., 2015) in Cytoscape, and was used to show the size of nodes, thus indicating the strength of interactions.
SMV-infected leaf samples were collected using the same method as described for RNA-seq analysis. In addition, samples were collected from non-inoculated and mock-inoculated plants. The extractions were performed according to the protocol (Pan et al., 2010) with minor modifications. The concentrations of endogenous ABA (abscisic acid), CTK (cytokinin, represented by zeatin in this study), IAA (indole-3-acetic acid, a member of the auxins), and SA were quantified using a G6420A HPLC-MS (high-performance liquid chromatography-mass spectrometry) system (Agilent Technologies). The phytohormone standards were purchased from Yuanye (Shanghai, China), and other reagents were purchased from CSP Co., Ltd. (Changshu, China). Each hormone was analyzed in three biological replicates.
qRT-PCR (quantitative reverse transcription PCR) assays were performed to confirm the expression of SMV resistance-related genes and to test for the presence or absence of SMV for each soybean line. Reverse transcription was performed with HiScript III 1st Strand cDNA Synthesis Kit (Vazyme, Nanjing, China) following the manufacturer’s instructions. qRT-PCR was performed with ChamQ SYBR qPCR Master Mix (Vazyme) on a LightCycler® 480 II system (Roche, Basel, Switzerland). The expression of each gene was calculated after being normalized to the soybean GmElF1β (elongation factor 1β) gene (Chen et al., 2015). The relative gene expression level was calculated using the 2-ΔΔCt method. The parameters of a thermal cycle were 95°C for 30 s, followed by 40 cycles of 95°C for 10 s, 60°C for 30 s at a volume of 20 μL, and melting curve analysis. All qPCR experiments were performed in triplicate and primers for qPCR were designed on the Primer-BLAST tool5 (Ye et al., 2012) and listed in Supplementary Table S1.
After SMV inoculation, no obvious symptoms were observed in either R-line ‘Kefeng 1’ or S-line ‘NN1138-2’ until 5 dpi. S-line shoots showed clear symptoms: wrinkling on infected trifoliate leaves at 7 dpi, chlorosis at 9 dpi, and leaf curling at 11 dpi, whereas all R-line shoots were asymptomatic until 9 dpi and some of them had small yellow spots on leaves at 11 dpi (Figure 1A).
Figure 1. Symptom development and SMV detection in two soybean cultivars. (A) Evaluation of the response of ‘NN1138-2’ and ‘Kefeng 1’ to SMV infection in the fully expanded leaf at the top of each plant. Lesions on inoculated leaves from 1 dpi to 5 dpi are caused by brush friction. (B) Relative accumulation of SMV RNA (detected by qRT-PCR results of CP gene) in inoculated leaves (from 1 dpi to 5 dpi) or systemically infected leaves (from 7 dpi to 11 dpi) of ‘NN1138-2’ and ‘Kefeng 1’ plants, respectively.
To quantify the relative SMV accumulation in both ‘Kefeng 1’ and ‘NN1138-2’ seedlings, a qRT-PCR assay was performed, using mock-infected seedlings as controls. Virus accumulation was low in both lines from 1 to 5 dpi (compared with 1 dpi, the fold changes of CP gene were less than 0.5 in ‘NN1138-2’ and less than 0.1 in ‘Kefeng 1’ from 3 to 5 dpi). Significant differences were detected since 7 dpi, the accumulation of SMV genomic RNA in ‘Kefeng 1’ remained low until the end of the assay (less than 1-fold), while it continued to increase from 9 dpi (more than 157-fold), and reached maximum level at 11 dpi (more than 293-fold) in ‘NN1138-2’ (Figure 1B).
After observing the phenotype of soybean inoculated with SMV and calculating the relative expression levels of the CP gene (which encodes the viral coat protein), we consider 7 dpi as the onset of the difference in response to SMV between the two soybean cultivars. Therefore, samples at 1, 3, 5, and 7 dpi were selected for transcriptome sequencing. 54,536 genes were obtained after de novo assembly (Supplementary Table S2). After filtering and trimming, a total of 2,259,866,196 clean reads were generated from 48 libraries by 150-bp paired-end RNA sequencing (Supplementary Table S3). The quality of the clean data was evaluated by FastQC (Brown et al., 2017). On average, 95.75% of clean reads were mapped to the soybean reference genome [Williams 82 Assembly 4 Annotation 1 (Wm82.a4.v1)] using HISAT2 (Kim et al., 2015), indicating good RNA-seq quality in the present study. According to the total mapped reads, 36,260 genes with an average FPKM of >1 in at least one treatment were considered as expressed genes and further analyzed (Supplementary Figure S1A). Unigenes annotated in NR, Pfam, GO, KO (KEGG ortholog), and Swiss-Prot were used to draw a Venn diagram (Supplementary Figure S1B; Supplementary Table S4), and 7,818 (21.56%) of the unigenes were annotated in these five databases.
To evaluate the alterations in unigene expression of SMV-infected soybean, 16,433 DEGs were determined compared with 1 dpi in total, of which 6,893, 7,537, and 7,446 DEGs were identified at 3, 5, and 7 dpi in ‘Kefeng 1’, respectively; and 1,653, 393, and 7,331 DEGs were identified at 3, 5, and 7 dpi in ‘NN1138-2’, respectively (Figure 2A). Furthermore, 5,733 genes were up-regulated at least once, 6,742 genes were down-regulated at least once in three sampling points in ‘Kefeng 1’, and 3,073 DEGs showed a differential expression at all three sampling points, of which 1,313 were upregulated and 1,680 were downregulated (Figures 2B–D). The number of corresponding DEGs in ‘NN1138-2’ at all three sampling points was smaller than that in ‘Kefeng 1’ (Figures 2E–G), and the number of DEGs at 7 dpi of ‘NN1138-2’ is greatly large compared to other time points, indicating that 7 dpi is the initial point for ‘NN1138-2’ to activate the defense response to SMV, which is slower than that of ‘Kefeng 1’.
Figure 2. Number of DEGs in SMV-infected soybean seedlings at 3, 5, and 7 dpi compared to 1 dpi. (A) Number of up-regulated and down-regulated DEGs at 3, 5, and 7 dpi in ‘Kefeng 1’ (KF1) and ‘NN1138-2’ (NN38). (B–D) Venn diagram of total, up-regulated and down-regulated DEGs in ‘Kefeng 1’, respectively. (E–G) Venn diagram of total, up-regulated and down-regulated DEGs in ‘NN1138-2’, respectively. DEGs, differentially expressed genes (cutoff ratio of >2, p-value <0.05, and q-value <0.05). U, up-regulated DEGs; D, down-regulated DEGs.
To investigate the key determinant genes of soybean associated with resistant or susceptible response to SMV, DEGs with opposite expression patterns were analyzed in ‘Kefeng 1’ (R-line) and ‘NN1138-2’ (S-line), respectively. The up-regulated genes in ‘Kefeng 1’ and the down-regulated genes in ‘NN1138-2’ were taken as resistance-response DEGs, while the down-regulated genes in ‘Kefeng 1’ and the up-regulated genes in ‘NN1138-2’ were considered as susceptibility-response DEGs. Similar classification methods were also applied to study the transcriptome dynamics in Brassica rapa clubroot caused by Plasmodiophora brassicae (Wei et al., 2021) and peanut wilt infected by Ralstonia solanacearum (Yang et al., 2022).
At 3 dpi, 53 genes were significantly up-regulated in R-line and down-regulated in S-line. On the contrary, 144 genes were down-regulated in R-line and up-regulated in S-line (Supplementary Figure S2A). At 5 dpi, 5 genes were up-regulated in R-line and down-regulated in S-line, 6 genes were down-regulated in R-line and up-regulated in S-line (Supplementary Figure S2B). At 7 dpi, 47 genes were up-regulated in R-line and down-regulated in S-line, 62 genes were down-regulated in R-line and up-regulated in S-line (Supplementary Figure S2C). These genes are good candidates for functional studies or host breeding for virus resistance, and their possible functions are listed in Supplementary Table S5.
Since TFs and R genes are important players in plant defense against pathogen infection, we focused on these two types of genes in the study. 3,771 TF genes generated from the Plant Transcription Factor Database and 729 R genes predicted by HMMER were annotated (Supplementary Table S6, Sheet 1 and Sheet 2). A total of 1,363 genes encoding TFs and 293 putative R genes were differentially expressed once at least at three groups (3 dpi vs. 1 dpi, 5 dpi vs. 1 dpi, and 7 dpi vs. 1 dpi) of both cultivars inoculated with SMV (Supplementary Table S6, Sheet 3 and Sheet 4).
The number of differentially expressed TF genes in each group was listed in Table 1. TF families with larger number of DEGs were shown in Figure 3A. In ‘Kefeng 1’, the majority of differentially expressed TFs belong to the ERF family, followed by MYB, bHLH, and WRKY families. In addition, the bHLH family has the most DEGs in ‘NN1138-2’, followed by ERF, WRKY, and MYB families. Since a large number of TF genes were differentially expressed in soybean after SMV infection, we subsequently focused on those genes enriched in plant hormone signal transduction, plant-pathogen interaction, and MAPK (mitogen-activated protein kinase) signaling pathways, because these three metabolic pathways have been reported to play important roles in the process of pathogen infection in host plants. There were 51 TF genes enriched in the above three KEGG pathways in ‘Kefeng 1’, and the heatmap showed that 2/3 of them were up-regulated after SMV inoculation (Figure 3B). In ‘NN1138-2’, none of the TFs were enriched in MAPK signaling pathway and the DEGs at 5 dpi were not enriched in any of the pathways. A total of 32 TFs were enriched in plant hormone signal transduction and plant-pathogen interaction pathways, and 26 TFs showed upregulated expression (Figure 3C).
Figure 3. Differentially expressed transcription factors (TFs) in two soybean cultivars. (A) The percentage of TFs among all DEGs in SMV-infected soybean seedlings at 3, 5, and 7 dpi coupled with 1 dpi. (B) Heatmap of differentially expressed TFs enriched in three KEGG pathways in ‘Kefeng 1’. (C) Heatmap of differentially expressed TFs enriched in three KEGG pathways in ‘NN1138-2’. The expression level was calculated using log2(FC). FC, fold change.
Two hundred thirty-four putative R genes were differentially expressed in ‘Kefeng 1’, and 106 of them were down-regulated in at least one group (Figure 4A; Supplementary Table S6, Sheet 4), 66 genes were down-regulated in the 3 dpi vs. 1 dpi group, which was the highest number and percentage among the three groups (Figure 4B). Moreover, 133 putative R genes were differentially expressed in ‘NN1138-2’, which was significantly lower than the number in ‘Kefeng 1’, and 56 of them were down-regulated in at least one group, which was slightly lower than the proportion of corresponding genes in ‘Kefeng 1’ (Figure 4A; Supplementary Table S6, Sheet 4). Interestingly, when the down-regulated R genes in three groups of NN118-2 were compared, among which 37 genes were found in the 7 dpi vs. 1 dpi group, which was the largest number but the smallest proportion among the three groups (Figure 4B). The Venn diagram also showed that 74 common R genes were differentially expressed in both cultivars (Figure 4C).
Figure 4. Differential expression of putative R genes in two soybean cultivars. (A) Heatmap showing the expression profile of putative R genes upon SMV infection. (B) Number and percentage of up- and down-regulated R genes in each group. (C) Venn diagram showing individual and common differentially expressed R genes. KF1: ‘Kefeng 1’ (resistant line); NN38: ‘NN1138-2’ (susceptible line).
To investigate the key biological progresses and pathways involved in soybean defense against SMV, all the up-regulated and down-regulated DEGs were annotated in GO database, separately. For example, up-regulated DEGs in ‘Kefeng 1’ can be enriched in plant-type secondary cell wall biogenesis (GO:0009834), and down-regulated DEGs in both cultivars can be enriched in cell wall (GO:0005618), cell wall biogenesis (GO:0042546) and cell wall organization (GO:0071555; Supplementary Figure S3). Detailed information on these three groups of DEGs is provided in Supplementary Table S7.
These up-regulated and down-regulated DEGs in both cultivars were also used for KEGG enrichment analysis, respectively. Resistance-response DEGs and susceptibility-response DEGs were performed to investigate the biological mechanisms of interaction between soybean and SMV. The significantly enriched KEGG pathways are summarized in Figure 5, and the genes under each pathway are listed in Supplementary Table S8.
Figure 5. KEGG enrichment analysis of common, up-regulated, and down-regulated DEGs in each treatment comparison in ‘Kefeng 1’ (KF1) and ‘NN1138-2’ (NN38) seedlings, respectively. (A) Common_1 indicates the common up-regulated DEGs in KF1_3 dpi vs. 1 dpi, KF1_5 dpi vs. 1 dpi, and KF1_7 dpi vs. 1 dpi. Common_2 indicates the common down-regulated DEGs in NN38_3 dpi vs. 1 dpi, NN38_5 dpi vs. 1 dpi, and NN38_7 dpi vs. 1 dpi. (B) Common_1 indicates the common down-regulated DEGs in KF1_3 dpi vs. 1 dpi, KF1_5 dpi vs. 1 dpi, and KF1_7 dpi vs. 1 dpi. No common_2 in the x-axis because the common up-regulated DEGs in NN38_3 dpi vs. 1 dpi, NN38_5 dpi vs. 1 dpi, and NN38_7 dpi vs. 1 dpi cannot be enriched in any KEGG pathway with padj <0.05.
When the leaves of ‘Kefeng 1’ seedlings were infected with SMV, a number of genes were induced. It is widely believed that these genes are involved in the activation of immune responses. There are 3,019, 3,182, and 3,911 up-regulated DEGs in KF1_3 dpi vs. KF1_1 dpi, KF1_5 dpi vs. KF1_1 dpi, and KF1_7 dpi vs. KF1_1 dpi, respectively (Figure 2A). KEGG enrichment analysis showed that the number of DEGs enriched in plant hormone signal transduction (gmx04075), photosynthesis-antenna proteins (gmx000196), circadian rhythm-plant (gmx04712) and MAPK signaling pathway-plant (gmx04016) ranked the top four among the up-regulated DEGs of ‘Kefeng 1’ (Figure 5A; Supplementary Table S8, Sheet 1).
Similarly, the expression of many genes was reduced in the leaves of susceptible soybean ‘NN1138-2’ when infected with SMV. These genes were also resistance response genes because they negatively regulated susceptibility. There are 838, 256, and 3,114 down-regulated DEGs in NN38_3 dpi vs. NN38_1 dpi, NN38_5 dpi vs. NN38_1 dpi, and NN38_7 dpi vs. NN38_1 dpi, respectively (Figure 2A). The number of significantly enriched KEGG pathways involved in the down-regulated DEGs of ‘NN1138-2’ is much smaller than those involved in the up-regulated DEGs of ‘Kefeng 1’ (Figure 5A).
To further narrow down the scope of vital candidate resistance-response genes, three groups of DEGs in ‘Kefeng 1’ and ‘NN1138-2’ were subjected to Venn analysis to find the common DEGs, and 1,313 DEGs in ‘Kefeng 1’ and 97 DEGs in ‘NN1138-2’ were obtained, respectively (Figures 2C,G). KEGG enrichment analysis suggested that the common DEGs in ‘Kefeng 1’ were enriched in photosynthesis-antenna proteins, circadian rhythm-plant, plant hormone signal transduction, and β-Alanine metabolism (gmx000410), and the common DEGs in ‘NN1138-2’ were enriched only in phenylpropanoid biosynthesis (Figure 5A).
As SMV enters into host cells, the virulence factors will attack the plant’s immune system. The expression of several genes will be increased in susceptible genotypes. To investigate these susceptibility-response DEGs, the transcriptome dynamics of SMV-infected ‘NN1138-2’ leaves were examined. There are 815, 137, and 4,217 up-regulated DEGs in NN38_3 dpi vs. NN38_1 dpi, NN38_5 dpi vs. NN38_1 dpi, and NN38_7 dpi vs. NN38_1 dpi, respectively (Figure 2A). The number of up-regulated DEGs in NN38_7 dpi vs. NN38_1 dpi was significantly higher than those in the other two comparison groups, and DEGs in this group were involved in starch and sucrose metabolism (gmx000500), circadian rhythm-plant, photosynthesis-antenna proteins, and ribosome biogenesis in eukaryotes (gmx03008). Several upregulated DEGs in NN38_3 dpi vs. NN38_1 dpi were enriched in MAPK signaling pathway-plant and plant-pathogen interaction (gmx04626). Ribosome biogenesis in eukaryotes was the significant enrichment KEGG pathway associated with up-regulated DEGs of NN38_5 dpi vs. NN38_1 dpi (Figure 5B). Venn analysis revealed that 19 DEGs were common in these comparison groups (Figure 2F), but these DEGs cannot be enriched in any of the KEGG pathways due to their small account.
Furthermore, when the ‘Kefeng 1’ plants were infected with SMV, the expression of some genes was decreased. These genes were also considered as susceptibility-response genes. There were 3,874, 4,355, and 3,535 down-regulated DEGs in KF1_3 dpi vs. KF1_1 dpi, KF1_5 dpi vs. KF1_1 dpi, and KF1_7 dpi vs. KF1_1 dpi, respectively (Figure 2A). Biosynthesis of amino acids (gmx01230), protein processing in endoplasmic reticulum (gmx04141), phenylpropanoid biosynthesis, and cysteine and methionine metabolism (gmx000270) were the top 4 significantly enriched KEGG pathways among these down-regulated DEGs. 1,680 common DEGs were identified by Venn analysis (Figure 2D), and these genes were significantly enriched in DNA replication and glutathione metabolism pathways (Figure 5B; Supplementary Table S8, Sheet 2).
KEGG enrichment analysis showed that the number of DEGs enriched in the “plant hormone signal transduction” pathway was the highest among all immune response-related pathways, and this pathway was also the unique that could enrich all three groups of DEGs in both cultivars (Figure 5A; Supplementary Table S8, Sheet 1). SMV infection positively or negatively regulated the expression of genes associated with hormone [IAA, ET (ethylene), SA, CTK, ABA, GA (gibberellic acid), JA, and BR (brassinosteroid)] signaling but with quantitative differences in two cultivars (Figure 6A; Supplementary Table S9).
Figure 6. Expression analysis of phytohormone-related DEGs and quantitative analysis of four representative hormones in soybean leaves. (A) Heatmap analysis of phytohormone-related DEGs. (B) Number of resistance-response DEGs associated with each hormone. ABA (C,D), IAA (E,F), SA (G,H), and CTK (zeatin) (I,J) contents in uninfected (mock-inoculated) and infected (SMV-inoculated) leaves of Kefeng 1 (KF1) and NN1138-2 (NN38) plants. Values are the mean ± SE (standard error) of three biological replicates per treatment. Different letters above each column indicate a significant difference (upper and lower case letters suggest that the two sets of data were significantly analyzed independently; p < 0.05; n = 3). FW indicates fresh weight of soybean leaves and N.D. represents that zeatin content was not detectable in this sample.
In this study, 58 DEGs were associated with IAA signaling, which was the highest among all hormones. The transcriptome sequencing data clearly showed that 5 dpi was the most important time point for IAA signaling compared to other inoculation time points, as the majority of up-regulated IAA-related genes were in the 5 dpi vs. 1 dpi group (23 genes, e.g., IAA4-like, GH3.6, SAUR32, and LAX12), and most of the down-regulated IAA-related genes were also in this group (8 genes, e.g., GH3.2, SAUR71, and IAA13-like). Six DEGs encoding PP2C (protein phosphatase 2C) in the ABA signaling pathway were differentially expressed, only one gene (Glyma.09G066500) was up-regulated, while the rest of the genes were down-regulated. Cytokinins have been shown to regulate the expression of defense genes and the activation of immune responses (Choi et al., 2010). Most of the genes related to CTK signaling (13/18) were upregulated in ‘Kefeng 1’, including four ARR genes (homologs of Arabidopsis response regulator).
After SMV infection, only the DEGs enriched in SA signaling were all up-regulated in both cultivars (Figure 6A; Supplementary Table S9), these DEGs can be divided into three categories: bZIP (basic leucine zipper) transcription factors, PR1 (pathogenesis-related protein 1), and NPR1 (non-expressor of PR1). The family of TFs containing a bZIP domain is one of the largest families of TFs in plants that can regulate genes in response to seed maturation, flower development, abiotic stress, and pathogen defense (Jakoby et al., 2002). 7 members of the bZIP TFs contain the so-called TGA (TGACG cis-DNA binding) motif (they are considered members of the TGA subfamily, see Supplementary Table S9), their homologs in A. thaliana act as regulators in SA signaling and are linked to biotic stress responses (Singh et al., 2002; Dröge-Laser et al., 2018). NPR1 acts as a key regulator of SA-mediated resistance in A. thaliana, its two homologs in soybean (Glyma.14G031300 and Glyma.02G283300) were up-regulated in ‘Kefeng 1’, but did not show differential expression in ‘NN1138-2’ compared to the control.
To further explore the relationship between hormone signaling and soybean resistance to SMV, a bar graph of the number of resistance-response DEGs associated with eight hormones was plotted (Figure 6B). The number of DEGs involved in IAA, ET, SA, CTK, and ABA signaling ranked among the top 5 of all hormones. Since ethylene is gaseous under natural conditions, the levels of the remaining four intracellular hormones were measured. The ABA content in SMV-inoculated ‘NN1138-2’ seedlings at 7 dpi was significantly higher than that of the other four sampling points, while there were no significant differences among the five sampling points in ‘Kefeng’ (Figures 6C,D). Compared with non-inoculated leaves, IAA content in inoculated leaves was significantly reduced (p < 0.05) during both mock inoculation and SMV infection in two cultivars (Figures 6E,F). In contrast, the SA content of both cultivars was significantly higher in at least one sampling point (mock inoculation or SMV inoculation) than in the uninoculated state (Figures 6G,H). An interesting finding was that the level of zeatin in ‘Kefeng 1’ after SMV infection was higher than that in the mock inoculation group, while the level of this hormone in ‘NN1138-2’ showed the opposite trend (Figures 6I,J).
Only susceptibility-response DEGs in ‘NN1138-2’ can be enriched in starch and sucrose metabolism (Figure 5B). There are 45 DEGs enriched in this pathway, including nine genes encoding glucose-1-phosphate adenylyltransferase large (or small) subunits (AGP, ENZYME entry: EC 2.7.7.27), six genes encoding β-glucosidase (BGLU, EC 3.2.1.21), five genes encoding starch synthase (SS, EC 2.4.1.242), four genes encoding endoglucanase (EG, EC 3.2.1.4), three genes encoding glucose-6-phosphate isomerase (G6P, EC 5.3.1.9), three genes encoding sucrose synthase (SUS, EC 2.4.1.13), three genes encoding trehalose-6-phosphate phosphatase (TPP, EC 3.1.3.12), two genes encoding hexokinase-1 (HXK1, EC 2.7.1.1), and other genes that modify the structure of starch or its intermediates (Bolouri Moghaddam and Van den Ende, 2012; Jeandet et al., 2022). However, the majority of these members (25/45) were not differentially expressed in ‘Kefeng 1’ after SMV inoculation (Supplementary Table S10, Sheet 1). The results suggest that genes related to sugar metabolism play an important role in the interaction between SMV and ‘NN1138-2’ rather than ‘Kefeng 1’, and 7 dpi is the starting point of this immune response.
Calcium ion (Ca2+) is a universal secondary messenger involved in all aspects of life, including growth regulation, development, reproduction, abiotic stresses, and other environmental stimuli (Kudla et al., 2018). In this study, 53 Ca2+ signaling-related DEGs were enriched in plant-pathogen interactions (Figure 7; Supplementary Table S10, Sheet 2). These genes were grouped into four subfamilies: CaM (calmodulin) and CMLs (CaM-like proteins), CDPKs (calcium-dependent protein kinases), CNGCs (cyclic nucleotide-gated ion channels), and Rboh (respiratory burst oxidase homolog protein). Notably, when pathogens invade plant cells, cellular responses during both PTI and ETI involve dynamic changes in cytosolic Ca2+ concentrations (Zhivotovsky and Orrenius, 2011; Yuan P. et al., 2020; Yuan et al., 2021). Therefore, the differential expression of Ca2+ signaling-related genes regulates dynamic changes in cytosolic Ca2+ concentrations, which is an early event during immune responses. Compared with other groups, the 5 dpi vs. 1 dpi group in ‘Kefeng 1’ had the largest number of DEGs (50), and 35 Ca2+ signaling-related DEGs were susceptibility-response genes as they were downregulated in ‘Kefeng 1’ and not differentially expressed in ‘NN1138-2’.
Figure 7. Expression profiles of key DEGs involved in the Ca2+ signaling pathway in two soybean cultivars after SMV inoculation. The gene IDs highlighted in yellowgreen indicate the susceptibility-response DEGs. KF1: ‘Kefeng 1’; NN38: ‘NN1138-2’.
Forty DEGs in ‘Kefeng 1’ were enriched in MAPK signaling pathway, and half of them were also enriched in plant hormone signal transduction. Among the 20 DEGs exclusively enriched in MAPK signaling pathway (Supplementary Table S11, Sheet 1), CHIA1 (chitinase class I, Glyma.02G042500), MAPKK9 (mitogen-activated protein kinase kinase 9, Glyma.07G105700) and a member of MKS1 (MKS1-1, a MAP kinase substrate: Glyma.04G239400) were up-regulated in at least two sampling points in ‘Kefeng 1’, but they were not differentially expressed in ‘NN1138-2’. The expression patterns of the above three genes in soybean suggest that they are involved in the biosynthesis of defense-related secondary metabolites and play an important role in ‘Kefeng 1’ resistance to SMV. Furthermore, the homolog of RbohA (respiratory burst oxidase homolog protein A, Glyma.01G222700) in rice (Oryza sativa) triggers the pathogen-induced ROS (reactive oxygen species) burst (Nagano et al., 2016). CML has been linked to cell signaling and a variety of biotic and abiotic stimuli (Zhang et al., 2014; Yuan et al., 2017), and EPF (epidermal patterning factor) has been correlated to regulate many aspects of plant growth and development (Lu et al., 2019). Several homologous genes of these proteins were up-regulated in both ‘Kefeng 1’ and ‘NN1138-2’ but with variant expression levels. These results suggest that partial members of RbohAs, CMLs and EPFs are involved in the regulation of soybean resistance to SMV.
Some candidate susceptibility-related DEGs in both cultivars: down-regulated DEGs in ‘Kefeng 1’ (5 dpi vs. 1 dpi) and up-regulated DEGs in ‘NN1138-2’ (3 dpi vs. 1 dpi) were also enriched in MAPK signaling pathway (Figure 5B). Ten of them were simultaneously enriched in plant hormone signal transduction, were excluded, and the expression profiles of the remaining 50 genes are listed in Supplementary Table S11, Sheet 2. Among them, 46 genes were differentially expressed in ‘‘‘Kefeng 1’ vs. CK” (meaning one of the three groups in ‘Kefeng 1’), of which six were up-regulated, 38 were down-regulated, and two were up- or down-regulated. It is noteworthy that three genes encoding PP2C proteins (Glyma.01G225100, Glyma.11G222600, and Glyma.11G018000) were significantly down-regulated in ‘Kefeng 1’ after SMV infection, while they were not differentially expressed in ‘NN1138-2’, suggesting that they may be involved in the negative regulation of SMV resistance in soybean. A similar report in A. thaliana showed that PP2C38 acts as a negative regulator of BIK1 (brassinosteroid-insensitive 1-associated receptor kinase 1) activity and BIK1-mediated immunity (Couto et al., 2016).
Combining the expression patterns of DEGs enriched in the MAPK signaling pathway in two cultivars, 39 genes were susceptibility-response DEGs, of which 35 genes were downregulated in ‘Kefeng 1’ and not differentially expressed in ‘NN1138-2’, and the remaining four genes (another gene encoding MKS1, MKS1-2: Glyma.05G190000, WRKY5: Glyma.01G128100, MAPKK2: Glyma.15G172600, and MYC1: Glyma.09G204500) were not differentially expressed in ‘Kefeng 1’ but upregulated in ‘NN1138-2’. An interesting finding was that most of the WRKY transcription factors enriched in the MAPK signaling pathway (7/11) were involved in the susceptibility response to SMV, suggesting that these WRKY proteins negatively regulate soybean defense against SMV. Previous studies indicated that WRKYs regulate transcription, signaling, plant defense, and other physiological processes by interacting with a variety of plant proteins (Chi et al., 2013; Wani et al., 2021). However, little is known about the transcriptional regulation of WRKY transcription factors during plant virus infection. Therefore, their regulatory mechanisms in soybean-SMV interaction will be of great significance in the future.
Plant immunity is regulated by a complex network of proteins. Previous studies have confirmed the roles of multiple MAPKs, R proteins, TFs and hormone-related proteins in resistance to plant pathogens (Robert-Seilaniantz et al., 2011; Spoel and Dong, 2012; Xu et al., 2018; Tellez et al., 2020; Wani et al., 2021). However, their synergistic regulation of plant responses to viruses has not been reported. In this study, besides plant hormone signal transduction, photosynthesis-antenna proteins and circadian rhythm-plant are two other KEGG pathways that can enrich all the three groups of up-regulated DEGs in ‘Kefeng 1’ (Figure 5A). The DEGs enriched in photosynthesis-antenna proteins or circadian rhythm-plant pathways were submitted to STRING database with the above four types of proteins to construct a protein–protein interaction (PPI) network. The majority of photosynthesis-antenna proteins do not interact with these proteins (data not shown), while the interaction network generated from six circadian rhythm-related proteins and these resistance-response proteins was predicted from 39 node proteins with the enrichment p value <1.0 × 10−16 at the medium confidence parameter level (Figure 8A; Supplementary Table S12, Sheet 1).
Figure 8. Protein-protein interaction (PPI) analysis of resistance-response DEGs and simple schematic diagram of soybean response to SMV in our study. (A) PPI network generated from 39 resistance-response DEGs. Circles represent the nodes of proteins, and the sizes of the circles represent the intensity of protein interactions as inferred by the value of the betweenness parameter. Lines between two nodes indicate that two proteins may interact. Cyan circles represent proteins encoded by DEGs, red triangles represent transcription factors (TFs), and pink circles represent proteins encoded by candidate hub genes. (B) Modulation of plant immune responses by MAPK cascades, clock proteins, TFs, and Ca2+ signaling. Note that not all components of abiotic and immune responses are depicted and interactions between defense molecules (such as between SA, JA, and ET) or abiotic factors (such as between light and clock) are not illustrated.
There were 77 combinations (also known as edges) in the PPI network, of which 10 interactions occurred between the clock proteins (proteins enriched in the circadian rhythm-plant pathway; Supplementary Table S12, Sheet 2). A member of CCAs (circadian clock-associated proteins): FKF1 (flavin-binding, kelch repeat, f-box 1: Glyma.05G239400) had 12 potential interacting partners, and four members of them [EIN3 (ETHYLENE INSENSITIVE 3)-like 1 (EIL1): Glyma.13G076800 and three EBF1 (EIN3-binding F-box protein 1) genes: Glyma.13G166200, Glyma.04G066900 and Glyma.14G116800] are involved in the ethylene signaling pathway. Another clock protein: ADO3 (adagio protein 3, Glyma.08G046500) may also interact with EIL1 and EBF1. A previous study indicated that the A. thaliana ADO3 homolog is a component of an E3 ubiquitin ligase complex that plays a central role in blue light-dependent circadian cycles (Jarillo et al., 2001). Since most of the ethylene signaling-related DEGs (16/21) are resistance-response genes (Supplementary Table S9), we suppose that endogenous ethylene plays an important role in the immune response of soybean to SMV and this process is regulated by FKF1 protein and blue light.
Phytochrome-Interacting Factors (PIFs) are members of the basic helix–loop–helix (bHLH) domain-containing transcription factor superfamily, and were originally recognized for their role in promoting plant growth (Li et al., 2012; Park et al., 2012). Recent studies have demonstrated novel functions of PIFs in regulating multiple signaling pathways: endogenous (e.g., hormonal) as well as abiotic (light, circadian, and elevated temperature) and biotic (defense responses) pathways (Sun et al., 2012; Xu et al., 2015; De Wit et al., 2016; Soy et al., 2016; Zust and Agrawal, 2017; Zhao et al., 2021). Phytochromes and cryptochromes (CRYs) have been reported as photoreceptors responsible for the light entrainment of the circadian clock in A. thaliana (Somers et al., 1998). Our PPI prediction suggested that two blue light photoreceptors: CRY1 (Glyma.06G103200) and CRY2 (Glyma.10G180600) may interact with four PIFs (PIF3, PIF4, PIF4A, and PIF4B) (Figure 8A; Supplementary Table S12, Sheet 2). Studies on the Arabidopsis-turnip crinkle virus (TCV) pathosystem revealed that CRY1 and CRY2, together with PHOT1 (phototropin 1, another blue light receptor) and PHOT2, but not phytochromes, are required for resistance to TCV (Chandra-Shekara et al., 2006; Jeong et al., 2010a,b). Prediction also suggests that CRY1 may interact with a candidate R protein: F-box/LRR-repeat protein 3 (FBXL3, Glyma.14G200300). All these results demonstrated that the PIF-CRY-R protein module might mediate soybean resistance to SMV.
In addition to EIL1 and PIF transcription factors, two genes (Glyma.09G280200 and Glyma.18G208800) encoding putative GmWRKY33 in the plant-pathogen interaction pathway, could interact with MKS1 (Glyma.04G239400; Figure 8A). These two genes were identified as significant DEGs with a 1.2- to 2.2-fold increase in ‘Kefeng 1’, but they were not differentially expressed in ‘NN1138-2’ (Supplementary Table S12, Sheet 1). AtWRKY33 can also interact with an MKS1 protein [AtVQ21, a calcium-binding protein carrying a conserved VQ (Valine-Glutamine) motif], and induce the expression of PAD3 (phytoalexin deficient 3) to enhance the defense response to P. syringae pv. tomato (Pst.) DC3000 (Andreasson et al., 2005; Cheng et al., 2012). The interaction between GmWRKY33 and another MKS1 (GmVQ24, Glyma.06G124400) was predicted to be involved in soybean cyst nematode resistance (Huang et al., 2022). Our prediction suggests that the interaction between WRKY33 and MKS1 may play a role in the broad-spectrum resistance of soybean to multiple pathogens.
Pathogen infection causes a series of early signaling events in plants, such as ROS production, activation of MAPKs, induction of plant hormone biosynthesis, and calcium flux (Yang et al., 2022). An interesting finding was that two members of putative WRKY33 (Glyma.18G208800 and Glyma.09G280200) can interact with CML36, and CDPK SK5-like can interact with both CML36 and MAPK7-like. DEGs encoding these five proteins were all up-regulated in ‘Kefeng 1’ but show no differential expression in ‘NN1138-2’. Two other MAPKs (MAPKK9 and MAPKKK3) encoded by Glyma.07G105700 and Glyma.04G213000, which could bind to MAPK7-like, were found with a 1.6-fold and 1.0-fold increased expression levels in ‘Kefeng 1’ (Supplementary Table S12, Sheet 1). All these results demonstrated that MAPKKK/MAPKK/MAPK-WRKY-CML/CDPK might indicate a new complex network in soybean for defense against SMV infection. By summarizing the PPI analysis in this study and combining it with previous reviews on the role of circadian rhythm in plant immunity (Hua, 2013; Wang et al., 2021), we drew a model diagram of soybean resistance response to SMV (Figure 8B).
The accuracy of our transcriptome data was validated by qRT-PCR. Eight DEGs closely associated with resistance to SMV infection were selected as targets based on high FPKM and fold change. The results showed that the expression patterns of DEGs in ‘Kefeng 1’ at 1, 3, 5, and 7 dpi after SMV inoculation were consistent with those calculated by RNA-Seq analysis (Figure 9). These findings support the high reproducibility between replicates of the transcriptome analysis.
Figure 9. Relative expression levels of eight candidate genes at different time points (1, 3, 5, and 7 dpi) of SMV inoculation in ‘Kefeng 1’ (KF1) and ‘NN1138-2’ (NN38) by qRT-PCR. Left y-axis (in cyan) represents the relative expression level of qRT-PCR. Right y-axis (in orange) represents the FPKM value of RNA-Seq. N.A. represents the qRT-PCR data that were not available. Error bars represent the standard deviation of the mean relative expression value. Significance between groups was determined by Duncan’s multiple range test (p < 0.05).
Soybean can be infected by more than 20 genera of viruses either naturally or by laboratory inoculation (Loebenstein and Thottappilly, 2003; Hill and Whitham, 2014). Among these viruses, SMV is generally considered to be the most economically important and widespread. In this study, two soybean cultivars with opposite resistance traits and the SMV 6067–1 isolate were used to perform transcriptome sequencing. The results of GO enrichment analysis showed that genes associated with cell wall biogenesis and organization were differentially expressed in both cultivars after SMV inoculation. Among them, 45 members showed up-regulated expression in at least one group of ‘Kefeng 1’ (Supplementary Table S7, Sheet 1), DEGs encoding fasciclin 1 (FAS1) domain-containing proteins (27/45) were the most abundant in this category, and fasciclin-like arabinogalactan proteins (FLAs) are reported to be involved in cell wall biosynthesis and responses to biotic and abiotic stresses (MacMillan et al., 2010, 2015; Zang et al., 2015). Homologs of this class of genes in ‘Kefeng 1’ showed up-regulated expression after SMV infection, but the specific mechanism of resistance and the influence on cell wall metabolism in the process are still unclear. The plant cell wall consists of several enzymes capable of modifying polysaccharides, of which xyloglucan endotransglucosylase/hydrolase (Xet, XTH) is important, as it is essential for wall architecture and elongation (Fry, 1995). XTH/Xet has also been implicated in cell wall loosening and expansion during pathogen infection (Rose et al., 2002). SMV inoculation caused downregulation of the expression of several XTHs in both soybean cultivars (Supplementary Table S7, Sheet 2 and Sheet 3), which may be related to inhibition of viral penetration of the cell wall barrier. Similarly, multiple genes encoding pectate lyase (PL) and pectinesterase (PE) were down-regulated after SMV infection in Kefeng 1 and NN1138-2. It is suggested that the synthesis and degree of modification of pectins in the cell wall also affect soybean resistance to SMV.
The KEGG enrichment results highlighted massive genes and multiple metabolic pathways relevant to resistance to SMV. Our study suggests that various proteins involved in the MAPK cascade, hormone signaling, Ca2+ signaling, starch and sucrose metabolism, and circadian rhythm, together with transcription factors and R proteins, may form a complex network to regulate the immune response to SMV in soybean, which will be discussed in detail later.
TFs play a key role in regulating plant responses to biotic stresses and in regulating transcriptional reprogramming associated with stress response (Buscaill and Rivas, 2014; Amorim et al., 2017; Wei and Chen, 2018), which is crucial to elucidate the mechanism in soybean resistance to SMV. Here, we found that four major TF families (bHLH, ERF, MYB, and WRKY) possessed a higher number of DEGs in both cultivars (Figure 3A). Previous studies have shown that members of the WRKY family are particularly important in plant cells, as they are extensively involved in the response to diverse biotic/abiotic stresses and in physiological/developmental processes (Jiang et al., 2015; Sun S. et al., 2023). In this study, ERF and bHLH families have the largest number of differentially expressed TF genes in SMV-inoculated ‘Kefeng 1’ and ‘NN1138-2’, respectively. An interesting finding is that none of the members in MYB family, which plays a broad-spectrum role in plant immune and defense responses (Yu et al., 2023), is enriched in MAPK signaling pathway, plant hormone signal transduction, or plant-pathogen interaction pathway in this study. The possible reason is that this phenomenon depends on the type of plant or virus or the sampling time, i.e., it is not universal. Moreover, the number of differentially expressed bZIP genes enriched in these three pathways was the highest (Figures 3B,C). Since this family of TFs is an important component in SA signaling, we believe that SA plays a critical role in soybean defense against SMV.
Plant hormones are very important signaling molecules associated with the regulation of host-virus interactions (Alazem and Lin, 2015), and previous studies indicated that pathogen invasion will cause changes in endogenous hormone levels in plants (Adie et al., 2007; Robert-Seilaniantz et al., 2007). Until recently, most studies on the role of hormones in plant-pathogen interactions have focused on SA, JA, and ET, which have been recognized as primary defense hormones. However, this study shows that the majority of defense response-related DEGs are associated with IAA signaling (Figures 6A,B).
Previous studies have shown that different DNA-binding AUXIN RESPONSIVE FACTORS (ARFs, also known as auxin-responsive proteins) positively or negatively regulate resistance to rice dwarf virus (RDV) infection in Oryza sativa (Qin et al., 2020). In two other studies of viral infection in rice, the SP8 protein of Southern rice black-streaked dwarf virus (SRBSDV) can interact with OsARF17 and inhibit its ability to bind DNA (Zhang H. et al., 2019). Similarly, the M protein of rice strip mosaic virus (RSMV) and the SP2 protein of rice strip virus (RSV) also interact with and inhibit OsARF17 (Zhang et al., 2020). Viruses can also manipulate specific ARFs in dicotyledonous plants to influence symptom development. The tobacco mosaic virus (TMV) replicase interacts directly with the Arabidopsis PAP1 (PHYTOCHROME-ASSOCIATED PROTEIN 1)/IAA26 (INDOLE-3-ACETIC ACID INDUCIBLE 26), IAA18 and IAA27 proteins through the helicase domain, and enhances TMV pathogenicity (Padmanabhan et al., 2005, 2008). These results suggest that five different viruses have evolved strategies to promote virulence by interacting with specific host proteins and interfering with their different functions in auxin signaling. In the present study, the number of DEGs associated with auxin signaling was the highest among all eight hormones. Among the auxin signaling-related DEGs, those encoding ARFs were the most abundant (37/58; Supplementary Table S9), implying that they play an important role in soybean defense against SMV. In ‘NN1138-2’ seedlings inoculated with SMV, the number of down-regulated ARF genes was higher than that in ‘Kefeng 1’. Mock inoculation and SMV infection could decrease the IAA content in the leaves of both soybean cultivars (Figures 6E,F), indicating that mechanical injury and virus infection could interfere with IAA biosynthesis and thus reduce the cell growth rate. How auxin modulates soybean response to SMV requires further experiments to verify. A possible idea in the future is to use the appropriate ARF obtained from RNA-seq as a target protein, screen the SMV protein that can directly interact with it, and further investigate whether the viral protein can interfere with the activity of GmARF, thereby exploring the function of GmARF in viral infection.
Considering only the SMV inoculation condition, the endogenous SA content was consistently increased in both soybean cultivars (Figures 6G,H). The results indicated that SA plays an important role and is a fundamental resistance-related hormone in soybean. We speculate that several bZIP transcription factors connected with SA signaling in Supplementary Table S9 may be involved in the regulation of isochorismate synthase (ICS) activity, thereby promoting SA biosynthesis. The zeatin content of ‘Kefeng 1’ and ‘NN1138-2’ cultivars showed an opposite trend under mock and SMV inoculation conditions (Figures 6I,J), suggesting that it may be involved in the process of soybean resistance and susceptibility to SMV, respectively. Finally, in SMV-infected seedlings, the levels of ABA (Figures 6C,D) and SA peaked at 5 dpi or 7 dpi, indicating that their involvement in the regulation of disease resistance signal transduction takes some time.
Carbohydrates such as glucose, fructose, sucrose and starch are recognized as sources of carbon and energy (Koch, 2004). They can also interact with other signaling molecules, including hormones, to control plant growth and development (Rolland et al., 2006; Smeekens et al., 2010). Among the DEGs enriched in starch and sucrose metabolism, BGLU genes encoding β-glucosidase are involved in several physiological processes as follows: cell wall catabolism (Patro et al., 2014), cell wall lignification (Dos Santos et al., 2019), defense compound activation (Lacchini et al., 2023), plant hormone activation (Han et al., 2020), and release of aromatic volatiles (Sun Y. et al., 2023). As a PR protein belonging to the PR-2 family, one member of GmBGLU (Glyma.15G142400) interacts with a Phakopsora pachyrhizi effector, suppressing PTI and promoting virulence (Bueno et al., 2022). In our previous study, the P3 movement protein in SMV was demonstrated to interact with an endo-1,3-β-glucanase to promote viral pathogenicity (Shi et al., 2020). We hypothesize that screening for effector proteins in various pathogens that can interact with BGLU and exploring their functions in the pathogen life cycle will help elucidate the mechanism of pathogenicity induced by BGLU.
In addition, the remaining sugar signaling molecules such as HXK1 may also potentially regulate plant defense. The HXKs are the best studied sugar sensors and have been implicated in the glucose-mediated repression of photosynthetic genes (chlorophyll a/b binding protein and plastocyanin) (Moore et al., 2003; Cho et al., 2006). Furthermore, HSKs potentially promote the degradation of EIN3, a key transcriptional regulator in ethylene signaling and plant defense (Karve et al., 2012). In this study, two HXK1 genes (Glyma.05G226600 and Glyma.08G033300) were not differentially expressed in ‘Kefeng 1’ at all sampling points, but were up-regulated at 7 dpi vs. 1 dpi in ‘NN1138-2’ (Supplementary Table S10, Sheet 1). Therefore, how HXK-dependent or HXK-independent metabolic pathways activated by sugar signaling can regulate transcription, translation, and enzyme activity, and then enhance the pathogenicity of SMV in soybean, is an interesting direction of research.
Plants deploy cell surface and intercellular receptors to sense pathogen infection and initiate immune signaling (Zhou and Zhang, 2020), which are referred to as pattern recognized receptors (PRRs). The MAPK cascade is one of the early signaling events in PTI and ETI (Meng and Zhang, 2013), that can transfer extracellular signals to the intracellular environment, which is also an important regulator of hormonal responses as well as innate immunity (Bi and Zhou, 2017; Thulasi Devendrakumar et al., 2018). This cascade system consists of MAPKKK (MEKK)-MAPKK (MKK)-MAPK (MPK) modules that link upstream receptors and downstream target sensors to form a complete signaling complex capable of recognizing invading pathogens and activating specific defense responses (Zhang and Zhang, 2022). The results of this study indicated that some resistance-response DEGs and susceptibility-response DEGs can be enriched in the MAPK signal pathway simultaneously (Figures 5A,B). Similar report was found in A. thaliana, AtMPK4 regulated plant immunity both positively and negatively. The possible reason is that AtMPK4 has three distinct substrates: MKS1, calmodulin-binding receptor-like cytoplasmic kinase 3 (CRCK3) and ARABIDOPSIS SH4-RELATED 3 (ASR3), each of which can interact with different downstream target proteins, to positively or negatively regulate PTI responses (Andreasson et al., 2005; Li et al., 2015; Thulasi Devendrakumar et al., 2018). Therefore, screening the substrates of MAPK in soybean for functional validation will facilitate the investigation of the MAPK signaling cascade in the defense response.
DEGs related to Ca2+ signals constitute the largest group in the plant-pathogen interaction pathway (Supplementary Table S10, Sheet 2). CaM and CML proteins are primary Ca2+ sensors that control various cellular functions by regulating the activity of different target proteins (Cheval et al., 2013). The effects of various CaMs/CMLs were quite different in pathogen-infected plants. For instance, silencing the expression of NtCaM13 in tobacco increased susceptibility to viral, bacterial and fungal pathogens, whereas knockdown of NtCaM1 did not (Takabatake et al., 2007). Another report in Arabidopsis suggests that CML37 acts as a positive and CML42 as a negative regulator in defense responses after inoculated with a fungus: Alternaria brassicicola (Heyer et al., 2022). In this study, most of the CaM/CML genes enriched in plant-pathogen interaction showed down-regulated expression, while two genes (Glyma.18G260700 and Glyma.09G236800) were up-regulated after viral infection (Figure 7), suggesting that CMLs in soybean act antagonistically in the regulation of induced defense responses. In plants, CaM can bind to some pathogen-induced TFs and induce plant immunity, some of these TFs can link Ca2+ signaling and SA, and activate both PTI and ETI (Bari and Jones, 2009). Numerous studies have also suggested that Ca2+ is involved in auxin signaling or responses [reviewed in (Vanneste and Friml, 2013)]. It is hypothesized that SAUR (SMALL AUXIN UP RNA) genes might play a role in linking Ca2+ to auxin signaling (Ren and Gray, 2015). Multiple CaMs/CMLs in this study showed opposite expression patterns, implying that they may bind to specific TFs, thereby activating different phytohormone signaling pathways and thus positively or negatively regulating the SMV infestation process. Interestingly, WRKY33 showed different expression patterns between the two soybean cultivars (Supplementary Table S12, Sheet 1). Calcium sensors might promote the binding of WRKY33 to the resistance- or susceptibility-related genes to regulate their transcriptional reprogramming together with the soybean response to SMV. The mechanism of the MEKK3/MKK9/MPK7-WRKY33-CML/CDPK module-mediated response to SMV needs more detailed experimental data to be fully understood.
Many living organisms on Earth, such as bacteria, cyanobacteria, fungi, animals, and plants, have evolved the ability to measure time, using the endogenous oscillator known as the circadian clock, which is critical for the physiological, developmental, and biochemical processes in multiple organisms (Greenham and McClung, 2015; Inoue et al., 2018). It has been reported that the plant’s defensive response to pathogens and pests is also regulated by the circadian clock (Lu et al., 2017). CCA1 (circadian clock associated 1) is a central circadian regulator, CCA1-RNAi transgenic A. thaliana compromised resistance to the downy mildew while CCA1 overexpression lines enhanced resistance to this pathogen (Wang W. et al., 2011). One of the CCA1 genes, namely FKF1, was increased by more than 2-fold in both cultivars after SMV infection (Supplementary Table S12, Sheet 1), suggesting its involvement in the immune response to SMV. Our prediction also indicates that soybean FKF1 has 12 target protein partners for PPI, including CRY1, CRY2, and three PIF transcription factors (PIF4, PIF4A, and PIF4B) (Supplementary Table S12, Sheet 2), suggesting that they may contribute to soybean resistance by forming a modular regulatory mechanism.
Circadian rhythm processes in plants are accompanied by changes in light intensity and quality. The light environment has a major influence on the photosynthesis of plants and their response to pathogens or insect herbivores. Plants have evolved multiple photoreceptor systems, including red (R)/far-red (FR) light-absorbing phytochromes (phyA-phyE in A. thaliana), blue/UV-A light-absorbing cryptochromes (CRYs), phototropins (PHOTs), and UV-B-absorbing UVR8 (Ni et al., 1998; Martinez et al., 2018), which regulate various photoreactions through their interactions with downstream target proteins, of which PIF3 is the best characterized (Ni et al., 1998). As one of the pivotal transcription factors involved in photoreceptor-mediated light response, PIFs play important roles in plant defense against necrotrophic pathogens. It has been proposed that PIFs play a role downstream of Phytochrome B (phyB) and participate in a variety of physiological processes, including seed germination, flowering, senescence, and shade avoidance (Kumar et al., 2012; Xie et al., 2017; Zhang R. et al., 2019; Qi et al., 2020). Recently, some results have reported that PIFs redundantly control Arabidopsis defense against Botrytis cinerea by modulating the expression of a number of defense-related genes, some of which are involved in ET signaling (Xiang et al., 2020). In the present study, PIF3 was up-regulated only in ‘Kefeng 1’, can interact with CRY1 and CRY2, and CRY1 can also interact with a candidate R protein (FBXL3), suggesting that PIF3 is involved in soybean resistance to SMV. How PIFs modulate the response of soybean to SMV requires further experiments, and in particular, their downstream target proteins need to be identified.
Breeding resistant germplasms is the most effective strategy for controlling viral diseases in soybean industry. KEGG enrichment together with PPI analysis revealed that most of the DEGs enriched in plant hormone signal transduction and circadian rhythm pathways, together with transcription factors of ERF, WRKY, and PIF families were the prominent components for resistance responses to SMV. MAPK cascades and Ca2+ signaling can positively or negatively regulate soybean resistance to SMV. Through the transcriptome profiling, we demonstrated that the MEKK3/MKK9/MPK7-WRKY33-CML/CDPK module and the PIF-CRY-R protein module can regulate the expression of resistance-related genes. These results will help us understand the regulation of resistance and susceptibility patterns between soybean and SMV, and further functional studies of candidate genes will aid to uncover new control strategies against SMV.
The raw datasets presented in this study have been deposited in the NCBI SRA (Sequence Read Archive) database under accession No. PRJNA1024691.
XYC conceived the project. XYC and XC supervised the work. HL performed most of the experiments with assistance from JL, XY, XC, and XYC. HL, XC, and XYC wrote the manuscript with contributions from all the authors. All authors contributed to the article and approved the submitted version.
This research was funded by the National Natural Science Foundation of China (31101411) and the open competition project of seed industry revitalization of Jiangsu Province (JBGS[2021]057).
We thank Stephen J Wylie (Murdoch University, Australia) for critical reading and helpful suggestions. We thank Wang Ning for helping in liquid chromatography–tandem mass spectrometry and Wang Xiaojing for help with pretreatment of plant samples.
The authors declare that the research was conducted in the absence of any commercial or financial relationships that could be construed as a potential conflict of interest.
All claims expressed in this article are solely those of the authors and do not necessarily represent those of their affiliated organizations, or those of the publisher, the editors and the reviewers. Any product that may be evaluated in this article, or claim that may be made by its manufacturer, is not guaranteed or endorsed by the publisher.
The Supplementary material for this article can be found online at: https://www.frontiersin.org/articles/10.3389/fmicb.2023.1241076/full#supplementary-material
1. ^https://www.soybase.org/GlycineBlastPages/
2. ^https://david.ncifcrf.gov/home.jsp
Adie, B. A. T., PéRez-PéRez, J. N., PéRez-PéRez, M. M., Godoy, M., SáNchez-Serrano, J.-J., Schmelz, E. A., et al. (2007). ABA is an essential signal for plant resistance to pathogens affecting JA biosynthesis and the activation of defenses in Arabidopsis. Plant Cell 19, 1665–1681. doi: 10.1105/tpc.106.048041
Alazem, M., and Lin, N. S. (2015). Roles of plant hormones in the regulation of host-virus interactions. Mol. Plant Pathol. 16, 529–540. doi: 10.1111/mpp.12204
Alazem, M., Tseng, K. C., Chang, W. C., Seo, J. K., and Kim, K. H. (2018). Elements involved in the Rsv3-mediated extreme resistance against an Avirulent strain of soybean mosaic virus. Viruses 10:581. doi: 10.3390/v10110581
Amorim, L. L. B., Santos, R., Neto, J. P. B., Guida-Santos, M., Crovella, S., and Benko-Iseppon, A. M. (2017). Transcription factors involved in plant resistance to pathogens. Curr. Protein Pept. Sci. 18, 335–351. doi: 10.2174/1389203717666160619185308
Andreasson, E., Jenkins, T., Brodersen, P., Thorgrimsen, S., Petersen, N. H., Zhu, S., et al. (2005). The MAP kinase substrate MKS1 is a regulator of plant defense responses. EMBO J. 24, 2579–2589. doi: 10.1038/sj.emboj.7600737
Awika, H. O., Bedre, R., Yeom, J., Marconi, T. G., Enciso, J., Mandadi, K. K., et al. (2019). Developing growth-associated molecular markers via high-throughput phenotyping in spinach. Plant. Genome 12, 1–19. doi: 10.3835/plantgenome2019.03.0027
Bari, R., and Jones, J. D. (2009). Role of plant hormones in plant defence responses. Plant Mol. Biol. 69, 473–488. doi: 10.1007/s11103-008-9435-0
Bi, G., and Zhou, J. M. (2017). MAP kinase signaling pathways: a hub of plant-microbe interactions. Cell Host Microbe 21, 270–273. doi: 10.1016/j.chom.2017.02.004
Bolouri Moghaddam, M. R., and Van Den Ende, W. (2012). Sugars and plant innate immunity. J. Exp. Bot. 63, 3989–3998. doi: 10.1093/jxb/ers129
Brown, J., Pirrung, M., and Mccue, L. A. (2017). FQC dashboard: integrates FastQC results into a web-based, interactive, and extensible FASTQ quality control tool. Bioinformatics 33, 3137–3139. doi: 10.1093/bioinformatics/btx373
Bueno, T. V., Fontes, P. P., Abe, V. Y., Utiyama, A. S., Senra, R. L., Oliveira, L. S., et al. (2022). A Phakopsora pachyrhizi effector suppresses PAMP-triggered immunity and interacts with a soybean glucan Endo-1,3-β-glucosidase to promote virulence. Mol. Plant-Microbe Interact. 35, 779–790. doi: 10.1094/MPMI-12-21-0301-R
Buscaill, P., and Rivas, S. (2014). Transcriptional control of plant defence responses. Curr. Opin. Plant Biol. 20, 35–46. doi: 10.1016/j.pbi.2014.04.004
Cervantes-Martinez, I., Chen, P. Y., Orazaly, M., and Klepadlo, M. (2015). Identification of a new allele at the Rsv3 locus for resistance to soybean mosaic virus in PI 61944 soybean accession. Crop Sci. 55, 999–1005. doi: 10.2135/cropsci2014.08.0569
Chandra-Shekara, A. C., Gupte, M., Navarre, D., Raina, S., Raina, R., Klessig, D., et al. (2006). Light-dependent hypersensitive response and resistance signaling against turnip crinkle virus in Arabidopsis. Plant J. 45, 320–334. doi: 10.1111/j.1365-313X.2005.02618.x
Chen, H., Adam Arsovski, A., Yu, K. F., and Wang, A. M. (2017). Deep sequencing leads to the identification of eukaryotic translation initiation factor 5A as a key element in Rsv1-mediated lethal systemic hypersensitive response to soybean mosaic virus infection in soybean. Mol. Plant Pathol. 18, 391–404. doi: 10.1111/mpp.12407
Chen, C. J., Chen, H., Zhang, Y., Thomas, H. R., Frank, M. H., He, Y. H., et al. (2020). TBtools: an integrative toolkit developed for interactive analyses of big biological data. Mol. Plant 13, 1194–1202. doi: 10.1016/j.molp.2020.06.009
Chen, H., Zhang, L. R., Yu, K. F., and Wang, A. M. (2015). Pathogenesis of soybean mosaic virus in soybean carrying Rsv1 gene is associated with miRNA and siRNA pathways, and breakdown of AGO1 homeostasis. Virology 476, 395–404. doi: 10.1016/j.virol.2014.12.034
Cheng, Y., Zhou, Y., Yang, Y., Chi, Y. J., Zhou, J., Chen, J. Y., et al. (2012). Structural and functional analysis of VQ motif-containing proteins in Arabidopsis as interacting proteins of WRKY transcription factors. Plant Physiol. 159, 810–825. doi: 10.1104/pp.112.196816
Cheval, C., Aldon, D., Galaud, J. P., and Ranty, B. (2013). Calcium/calmodulin-mediated regulation of plant immunity. Biochim. Biophys. Acta 1833, 1766–1771. doi: 10.1016/j.bbamcr.2013.01.031
Chi, Y., Yang, Y., Zhou, Y., Zhou, J., Fan, B., Yu, J. Q., et al. (2013). Protein-protein interactions in the regulation of WRKY transcription factors. Mol. Plant 6, 287–300. doi: 10.1093/mp/sst026
Cho, Y. H., Yoo, S. D., and Sheen, J. (2006). Regulatory functions of nuclear hexokinase1 complex in glucose signaling. Cells 127, 579–589. doi: 10.1016/j.cell.2006.09.028
Choi, J., Huh, S. U., Kojima, M., Sakakibara, H., Paek, K. H., and Hwang, I. (2010). The cytokinin-activated transcription factor ARR2 promotes plant immunity via TGA3/NPR1-dependent salicylic acid signaling in Arabidopsis. Dev. Cell 19, 284–295. doi: 10.1016/j.devcel.2010.07.011
Couto, D., Niebergall, R., Liang, X., Bucherl, C. A., Sklenar, J., Macho, A. P., et al. (2016). The Arabidopsis protein phosphatase PP2C38 negatively regulates the central immune kinase BIK1. PLoS Pathog. 12:e1005811. doi: 10.1371/journal.ppat.1005811
De Wit, M., Galvao, V. C., and Fankhauser, C. (2016). Light-mediated hormonal regulation of plant growth and development. Annu. Rev. Plant Biol. 67, 513–537. doi: 10.1146/annurev-arplant-043015-112252
Dos Santos, A. C., Ximenes, E., Kim, Y., and Ladisch, M. R. (2019). Lignin-enzyme interactions in the hydrolysis of lignocellulosic biomass. Trends Biotechnol. 37, 518–531. doi: 10.1016/j.tibtech.2018.10.010
Dröge-Laser, W., Snoek, B. L., Snel, B., and Weiste, C. (2018). The Arabidopsis bZIP transcription factor family—an update. Curr. Opin. Plant Biol. 45, 36–49. doi: 10.1016/j.pbi.2018.05.001
Duan, Y., Ma, S., Chen, X., Shen, X., Yin, C., and Mao, Z. (2022). Transcriptome changes associated with apple (Malus domestica) root defense response after Fusarium proliferatum f. sp. Malus domestica infection. BMC Genomics 23:484. doi: 10.1186/s12864-022-08721-3
Fry, S. C. (1995). Polysaccharide-modifying enzymes in the plant Cell Wall. Annu. Rev. Plant Physiol. Plant Mol. Biol. 46, 497–520. doi: 10.1146/annurev.pp.46.060195.002433
Grabherr, M. G., Haas, B. J., Yassour, M., Levin, J. Z., Thompson, D. A., Amit, I., et al. (2011). Full-length transcriptome assembly from RNA-Seq data without a reference genome. Nat. Biotechnol. 29, 644–652. doi: 10.1038/nbt.1883
Greenham, K., and Mcclung, C. R. (2015). Integrating circadian dynamics with physiological processes in plants. Nat. Rev. Genet. 16, 598–610. doi: 10.1038/nrg3976
Gunduz, I., Buss, G. R., Chen, P., and Tolin, S. A. (2002). Characterization of SMV resistance genes in Tousan 140 and Hourei soybean. Crop Sci. 42, 90–95. doi: 10.2135/cropsci2002.9000
Han, Y., Watanabe, S., Shimada, H., and Sakamoto, A. (2020). Dynamics of the leaf endoplasmic reticulum modulate beta-glucosidase-mediated stress-activated ABA production from its glucosyl ester. J. Exp. Bot. 71, 2058–2071. doi: 10.1093/jxb/erz528
Hashimoto, M., Neriya, Y., Yamaji, Y., and Namba, S. (2016). Recessive resistance to plant viruses: potential resistance genes beyond translation initiation factors. Front. Microbiol. 7:1695. doi: 10.3389/fmicb.2016.01695
Hayes, A. J., Ma, G. R., Buss, G. R., and Maroof, M. A. S. (2000). Molecular Marker Mapping ofRSV4, a Gene Conferring Resistance to all Known Strains of Soybean Mosaic Virus. Crop Sci. 40, 1434–1437. doi: 10.2135/cropsci2000.4051434x
Heyer, M., Scholz, S. S., Reichelt, M., Kunert, G., Oelmuller, R., and Mithofer, A. (2022). The ca(2+) sensor proteins CML37 and CML42 antagonistically regulate plant stress responses by altering phytohormone signals. Plant Mol. Biol. 109, 611–625. doi: 10.1007/s11103-021-01184-2
Hill, J. H., and Whitham, S. A. (2014). Control of virus diseases in soybeans. Adv. Virus Res. 90, 355–390. doi: 10.1016/B978-0-12-801246-8.00007-X
Hobbs, H. A., Hartman, G. L., Wang, Y., Hill, C. B., Bernard, R. L., Pedersen, W. L., et al. (2003). Occurrence of seed coat mottling in soybean plants inoculated with bean pod mottle virus and soybean mosaic virus. Plant Dis. 87, 1333–1336. doi: 10.1094/PDIS.2003.87.11.1333
Howden, A. J., and Huitema, E. (2012). Effector-triggered post-translational modifications and their role in suppression of plant immunity. Front. Plant Sci. 3:160. doi: 10.3389/fpls.2012.00160
Hua, J. (2013). Modulation of plant immunity by light, circadian rhythm, and temperature. Curr. Opin. Plant Biol. 16, 406–413. doi: 10.1016/j.pbi.2013.06.017
Huang, M., Jiang, Y., Qin, R., Jiang, D., Chang, D., Tian, Z., et al. (2022). Full-length transcriptional analysis of the same soybean genotype with compatible and incompatible reactions to Heterodera glycines reveals nematode infection activating plant defense response. Front. Plant Sci. 13:866322. doi: 10.3389/fpls.2022.866322
Inoue, K., Araki, T., and Endo, M. (2018). Circadian clock during plant development. J. Plant Res. 131, 59–66. doi: 10.1007/s10265-017-0991-8
Irwin, M. E., and Goodman, R. M. (1981). “Ecology and control of soybean mosaic virus” in Plant diseases and vectors: Ecology and epidemiology. eds. K. Maramorosch and K. F. Harris (Cambridge, MA: Academic Press), 181–220.
Jakoby, M., Weisshaar, B., Dröge-Laser, W., Vicente-Carbajosa, J., Tiedemann, J., Kroj, T., et al. (2002). bZIP transcription factors in Arabidopsis. Trends Plant Sci. 7, 106–111. doi: 10.1016/S1360-1385(01)02223-3
Jarillo, J. A., Capel, J., Tang, R. H., Yang, H. Q., Alonso, J. M., Ecker, J. R., et al. (2001). An Arabidopsis circadian clock component interacts with both CRY1 and phyB. Nature 410, 487–490. doi: 10.1038/35068589
Jeandet, P., Formela-Luboinska, M., Labudda, M., and Morkunas, I. (2022). The role of sugars in plant responses to stress and their regulatory function during development. Int. J. Mol. Sci. 23:5161. doi: 10.3390/ijms23095161
Jeong, R. D., Chandra-Shekara, A. C., Barman, S. R., Navarre, D., Klessig, D. F., Kachroo, A., et al. (2010a). Cryptochrome 2 and phototropin 2 regulate resistance protein-mediated viral defense by negatively regulating an E3 ubiquitin ligase. Proc. Natl. Acad. Sci. U. S. A. 107, 13538–13543. doi: 10.1073/pnas.1004529107
Jeong, R. D., Kachroo, A., and Kachroo, P. (2010b). Blue light photoreceptors are required for the stability and function of a resistance protein mediating viral defense in Arabidopsis. Plant Signal. Behav. 5, 1504–1509. doi: 10.4161/psb.5.11.13705
Jiang, W., Wu, J., Zhang, Y., Yin, L., and Lu, J. (2015). Isolation of a WRKY30 gene from Muscadinia rotundifolia (Michx) and validation of its function under biotic and abiotic stresses. Protoplasma 252, 1361–1374. doi: 10.1007/s00709-015-0769-6
Jin, J. P., Tian, F., Yang, D. C., Meng, Y. Q., Kong, L., Luo, J. C., et al. (2017). PlantTFDB 4.0: toward a central hub for transcription factors and regulatory interactions in plants. Nucleic Acids Res. 45, D1040–D1045. doi: 10.1093/nar/gkw982
Jones, J. D., and Dangl, J. L. (2006). The plant immune system. Nature 444, 323–329. doi: 10.1038/nature05286
Karthikeyan, A., Li, K., Li, C., Yin, J. L., Li, N., Yang, Y. H., et al. (2018). Fine-mapping and identifying candidate genes conferring resistance to soybean mosaic virus strain SC20 in soybean. Theor. Appl. Genet. 131, 461–476. doi: 10.1007/s00122-017-3014-1
Karve, A., Xia, X., and Moore, B. (2012). Arabidopsis hexokinase-Like1 and Hexokinase1 form a critical node in mediating plant glucose and ethylene responses. Plant Physiol. 158, 1965–1975. doi: 10.1104/pp.112.195636
Kaur, S., Samota, M. K., Choudhary, M., Choudhary, M., Pandey, A. K., Sharma, A., et al. (2022). How do plants defend themselves against pathogens-biochemical mechanisms and genetic interventions. Physiol. Mol. Biol. Plants 28, 485–504. doi: 10.1007/s12298-022-01146-y
Kim, D., Langmead, B., and Salzberg, S. L. (2015). HISAT: a fast spliced aligner with low memory requirements. Nat. Methods 12, 357–360. doi: 10.1038/nmeth.3317
Koch, K. (2004). Sucrose metabolism: regulatory mechanisms and pivotal roles in sugar sensing and plant development. Curr. Opin. Plant Biol. 7, 235–246. doi: 10.1016/j.pbi.2004.03.014
Kudla, J., Becker, D., Grill, E., Hedrich, R., Hippler, M., Kummer, U., et al. (2018). Advances and current challenges in calcium signaling. New Phytol. 218, 414–431. doi: 10.1111/nph.14966
Kumar, S. V., Lucyshyn, D., Jaeger, K. E., Alos, E., Alvey, E., Harberd, N. P., et al. (2012). Transcription factor PIF4 controls the thermosensory activation of flowering. Nature 484, 242–245. doi: 10.1038/nature10928
Lacchini, E., Erffelinck, M. L., Mertens, J., Marcou, S., Molina-Hidalgo, F. J., Tzfadia, O., et al. (2023). The saponin bomb: a nucleolar-localized beta-glucosidase hydrolyzes triterpene saponins in Medicago truncatula. New Phytol. 239, 705–719. doi: 10.1111/nph.18763
Li, B., Jiang, S., Yu, X., Cheng, C., Chen, S., Cheng, Y., et al. (2015). Phosphorylation of trihelix transcriptional repressor ASR3 by MAP KINASE4 negatively regulates Arabidopsis immunity. Plant Cell 27, 839–856. doi: 10.1105/tpc.114.134809
Li, L., Ljung, K., Breton, G., Schmitz, R. J., Pruneda-Paz, J., Cowing-Zitron, C., et al. (2012). Linking photoreceptor excitation to changes in plant architecture. Genes Dev. 26, 785–790. doi: 10.1101/gad.187849.112
Liao, L., Chen, P., Buss, G. R., Yang, Q., and Tolin, S. A. (2002). Inheritance and allelism of resistance to soybean mosaic virus in Zao18 soybean from China. J. Hered. 93, 447–452. doi: 10.1093/jhered/93.6.447
Liu, X., Fang, P., Wang, Z., Cao, X., Yu, Z., Chen, X., et al. (2022). Comparative RNA-seq analysis reveals a critical role for ethylene in rose (Rosa hybrida) susceptible response to Podosphera pannosa. Front. Plant Sci. 13:1018427. doi: 10.3389/fpls.2022.1018427
Liu, S. M., Kandoth, P. K., Warren, S. D., Yeckel, G., Heinz, R., Alden, J., et al. (2012). A soybean cyst nematode resistance gene points to a new mechanism of plant resistance to pathogens. Nature 492:256. doi: 10.1038/nature11651
Loebenstein, G., and Thottappilly, G. (2003). Virus and virus-like diseases of major crops in developing countries. Netherlands: Springer Netherlands.
Love, M. I., Huber, W., and Anders, S. (2014). Moderated estimation of fold change and dispersion for RNA-seq data with DESeq2. Genome Biol. 15:550. doi: 10.1186/s13059-014-0550-8
Lu, J., He, J., Zhou, X., Zhong, J., Li, J., and Liang, Y. K. (2019). Homologous genes of epidermal patterning factor regulate stomatal development in rice. J. Plant Physiol. 234-235, 18–27. doi: 10.1016/j.jplph.2019.01.010
Lu, H., Mcclung, C. R., and Zhang, C. (2017). Tick tock: circadian regulation of plant innate immunity. Annu. Rev. Phytopathol. 55, 287–311. doi: 10.1146/annurev-phyto-080516-035451
Ma, Y., Wang, D. G., Li, H. C., Zheng, G. J., Yang, Y. Q., Li, H. W., et al. (2011). Fine mapping of the R-SC14Q locus for resistance to soybean mosaic virus in soybean. Euphytica 181, 127–135. doi: 10.1007/s10681-011-0457-3
Macmillan, C. P., Mansfield, S. D., Stachurski, Z. H., Evans, R., and Southerton, S. G. (2010). Fasciclin-like arabinogalactan proteins: specialization for stem biomechanics and cell wall architecture in Arabidopsis and Eucalyptus. Plant J. 62, 689–703. doi: 10.1111/j.1365-313X.2010.04181.x
Macmillan, C. P., Taylor, L., Bi, Y., Southerton, S. G., Evans, R., and Spokevicius, A. (2015). The fasciclin-like arabinogalactan protein family of Eucalyptus grandis contains members that impact wood biology and biomechanics. New Phytol. 206, 1314–1327. doi: 10.1111/nph.13320
Martinez, C., Nieto, C., and Prat, S. (2018). Convergent regulation of PIFs and the E3 ligase COP1/SPA1 mediates thermosensory hypocotyl elongation by plant phytochromes. Curr. Opin. Plant Biol. 45, 188–203. doi: 10.1016/j.pbi.2018.09.006
Meng, X. Z., and Zhang, S. Q. (2013). MAPK cascades in plant disease resistance signaling. Annu. Rev. Phytopathol. 51, 245–266. doi: 10.1146/annurev-phyto-082712-102314
Moore, J. W., Loake, G. J., and Spoel, S. H. (2011). Transcription dynamics in plant immunity. Plant Cell 23, 2809–2820. doi: 10.1105/tpc.111.087346
Moore, B., Zhou, L., Rolland, F., Hall, Q., Cheng, W. H., Liu, Y. X., et al. (2003). Role of the Arabidopsis glucose sensor HXK1 in nutrient, light, and hormonal signaling. Science 300, 332–336. doi: 10.1126/science.1080585
Nagano, M., Ishikawa, T., Fujiwara, M., Fukao, Y., Kawano, Y., Kawai-Yamada, M., et al. (2016). Plasma membrane microdomains are essential for Rac1-RbohB/H-mediated immunity in Rice. Plant Cell 28, 1966–1983. doi: 10.1105/tpc.16.00201
Nakano, M., Usugi, T., and Shinkai, A. (1987). Effects of the application of chemicals on the spread of soybean mosaic virus. Kyushu Plant Prot. Res. 33, 33–35. doi: 10.4241/kyubyochu.33.33
Ni, M., Tepperman, J. M., and Quail, P. H. (1998). PIF3, a phytochrome-interacting factor necessary for normal photoinduced signal transduction, is a novel basic helix-loop-helix protein. Cell(Cambridge,Mass) 95, 657–667. doi: 10.1016/S0092-8674(00)81636-0
Padmanabhan, M. S., Goregaoker, S. P., Golem, S., Shiferaw, H., and Culver, J. N. (2005). Interaction of the tobacco mosaic virus replicase protein with the aux/IAA protein PAP1/IAA26 is associated with disease development. J. Virol. 79, 2549–2558. doi: 10.1128/JVI.79.4.2549-2558.2005
Padmanabhan, M. S., Kramer, S. R., Wang, X., and Culver, J. N. (2008). Tobacco mosaic virus replicase-auxin/indole acetic acid protein interactions: reprogramming the auxin response pathway to enhance virus infection. J. Virol. 82, 2477–2485. doi: 10.1128/JVI.01865-07
Pan, X., Welti, R., and Wang, X. (2010). Quantitative analysis of major plant hormones in crude plant extracts by high-performance liquid chromatography–mass spectrometry. Nat. Protoc. 5, 986–992. doi: 10.1038/nprot.2010.37
Park, E., Park, J., Kim, J., Nagatani, A., Lagarias, J. C., and Choi, G. (2012). Phytochrome B inhibits binding of phytochrome-interacting factors to their target promoters. Plant J. 72, 537–546. doi: 10.1111/j.1365-313X.2012.05114.x
Patro, L., Mohapatra, P. K., Biswal, U. C., and Biswal, B. (2014). Dehydration induced loss of photosynthesis in Arabidopsis leaves during senescence is accompanied by the reversible enhancement in the activity of cell wall β-glucosidase. J. Photochem. Photobiol. B 137, 49–54. doi: 10.1016/j.jphotobiol.2014.03.018
Pedersen, P., Grau, C., Cullen, E., Koval, N., and Hill, J. H. (2007). Potential for integrated Management of Soybean Virus Disease. Plant Dis. 91, 1255–1259. doi: 10.1094/PDIS-91-10-1255
Piron, F., Nicolai, M., Minoia, S., Piednoir, E., Moretti, A., Salgues, A., et al. (2010). An induced mutation in tomato eIF4E leads to immunity to two potyviruses. PLoS One 5:e11313. doi: 10.1371/journal.pone.0011313
Qi, L., Liu, S., Li, C., Fu, J., Jing, Y., Cheng, J., et al. (2020). Phytochrome-interacting factors interact with the ABA receptors PYL8 and PYL9 to orchestrate ABA signaling in darkness. Mol. Plant 13, 414–430. doi: 10.1016/j.molp.2020.02.001
Qin, Q., Li, G., Jin, L., Huang, Y., Wang, Y., Wei, C., et al. (2020). Auxin response factors (ARFs) differentially regulate rice antiviral immune response against rice dwarf virus. PLoS Pathog. 16:e1009118. doi: 10.1371/journal.ppat.1009118
Ren, H., and Gray, W. M. (2015). SAUR proteins as effectors of hormonal and environmental signals in plant growth. Mol. Plant 8, 1153–1164. doi: 10.1016/j.molp.2015.05.003
Robert-Seilaniantz, A., Grant, M., and Jones, J. D. (2011). Hormone crosstalk in plant disease and defense: more than just jasmonate-salicylate antagonism. Annu. Rev. Phytopathol. 49, 317–343. doi: 10.1146/annurev-phyto-073009-114447
Robert-Seilaniantz, A., Navarro, L., Bari, R., and Jones, J. D. (2007). Pathological hormone imbalances. Curr. Opin. Plant Biol. 10, 372–379. doi: 10.1016/j.pbi.2007.06.003
Rolland, F., Baena-Gonzalez, E., and Sheen, J. (2006). Sugar sensing and signaling in plants: conserved and novel mechanisms. Annu. Rev. Plant Biol. 57, 675–709. doi: 10.1146/annurev.arplant.57.032905.105441
Rose, J. K., Braam, J., Fry, S. C., and Nishitani, K. (2002). The XTH family of enzymes involved in xyloglucan endotransglucosylation and endohydrolysis: current perspectives and a new unifying nomenclature. Plant Cell Physiol. 43, 1421–1435. doi: 10.1093/pcp/pcf171
Ross, J. P. (1983). Effect of soybean Mosiac on component yields from blends of Mosiac resistant and susceptible Soybeans1. Crop Sci. 23, 343–346. doi: 10.2135/cropsci1983.0011183X002300020038x
Rui, R., Liu, S. C., Karthikeyan, A., Wang, T., Niu, H. P., Yin, J. L., et al. (2017). Fine-mapping and identification of a novel locus Rsc15 underlying soybean resistance to soybean mosaic virus. Theor. Appl. Genet. 130, 2395–2410. doi: 10.1007/s00122-017-2966-5
Schmutz, J., Cannon, S. B., Schlueter, J., Ma, J., Mitros, T., Nelson, W., et al. (2010). Genome sequence of the palaeopolyploid soybean. Nature 463, 178–183. doi: 10.1038/nature08670
Shannon, P., Markiel, A., Ozier, O., Baliga, N. S., Wang, J. T., Ramage, D., et al. (2003). Cytoscape: a software environment for integrated models of biomolecular interaction networks. Genome Res. 13, 2498–2504. doi: 10.1101/gr.1239303
Sherman, B. T., Hao, M., Qiu, J., Jiao, X. L., Baseler, M. W., Lane, H. C., et al. (2022). DAVID: a web server for functional enrichment analysis and functional annotation of gene lists (2021 update). Nucleic Acids Res. 50, W216–W221. doi: 10.1093/nar/gkac194
Shi, F., Wang, Y., Zhang, F., Yuan, X., Chen, H., Chen, X., et al. (2020). Soybean Endo-1,3-Beta-Glucanase (GmGLU) interaction with soybean mosaic virus-encoded P3 protein May contribute to the Intercelluar movement. Front. Genet. 11:536771. doi: 10.3389/fgene.2020.536771
Singh, K., Foley, R. C., and Onate-Sanchez, L. (2002). Transcription factors in plant defense and stress responses. Curr. Opin. Plant Biol. 5, 430–436. doi: 10.1016/S1369-5266(02)00289-3
Smeekens, S., Ma, J., Hanson, J., and Rolland, F. (2010). Sugar signals and molecular networks controlling plant growth. Curr. Opin. Plant Biol. 13, 274–279. doi: 10.1016/j.pbi.2009.12.002
Somers, D. E., Devlin, P. F., and Kay, S. A. (1998). Phytochromes and cryptochromes in the entrainment of the Arabidopsis circadian clock. Science 282, 1488–1490. doi: 10.1126/science.282.5393.1488
Soy, J., Leivar, P., Gonzalez-Schain, N., Martin, G., Diaz, C., Sentandreu, M., et al. (2016). Molecular convergence of clock and photosensory pathways through PIF3-TOC1 interaction and co-occupancy of target promoters. Proc. Natl. Acad. Sci. U. S. A. 113, 4870–4875. doi: 10.1073/pnas.1603745113
Spoel, S. H., and Dong, X. (2012). How do plants achieve immunity? Defence without specialized immune cells. Nat. Rev. Immunol. 12, 89–100. doi: 10.1038/nri3141
Sun, S., Li, S., Zhou, X., and Yang, X. (2023). WRKY1 represses the WHIRLY1 transcription factor to positively regulate plant defense against geminivirus infection. PLoS Pathog. 19:e1011319. doi: 10.1371/journal.ppat.1011319
Sun, J., Qi, L., Li, Y., Chu, J., and Li, C. (2012). PIF4-mediated activation of YUCCA8 expression integrates temperature into the auxin pathway in regulating arabidopsis hypocotyl growth. PLoS Genet. 8:e1002594. doi: 10.1371/journal.pgen.1002594
Sun, Y., Zhang, H., Peng, W., Sun, P., and Ye, X. (2023). Release of glycosidically-bound volatiles in orange juice under natural conditions. Food Chem. 429:136827. doi: 10.1016/j.foodchem.2023.136827
Szklarczyk, D., Gable, A. L., Nastou, K. C., Lyon, D., Kirsch, R., Pyysalo, S., et al. (2021). The STRING database in 2021: customizable protein-protein networks, and functional characterization of user-uploaded gene/measurement sets. Nucleic Acids Res. 49, D605–D612. doi: 10.1093/nar/gkaa1074
Takabatake, R., Karita, E., Seo, S., Mitsuhara, I., Kuchitsu, K., and Ohashi, Y. (2007). Pathogen-induced calmodulin isoforms in basal resistance against bacterial and fungal pathogens in tobacco. Plant Cell Physiol. 48, 414–423. doi: 10.1093/pcp/pcm011
Tang, Y., Li, M., Wang, J. X., Pan, Y., and Wu, F. X. (2015). CytoNCA: a cytoscape plugin for centrality analysis and evaluation of protein interaction networks. Biosystems 127, 67–72. doi: 10.1016/j.biosystems.2014.11.005
Tellez, J., Munoz-Barrios, A., Sopena-Torres, S., Martin-Forero, A. F., Ortega, A., Perez, R., et al. (2020). YODA kinase controls a novel immune pathway of tomato conferring enhanced disease resistance to the bacterium Pseudomonas syringae. Front. Plant Sci. 11:584471. doi: 10.3389/fpls.2020.584471
Thulasi Devendrakumar, K., Li, X., and Zhang, Y. (2018). MAP kinase signalling: interplays between plant PAMP- and effector-triggered immunity. Cell. Mol. Life Sci. 75, 2981–2989. doi: 10.1007/s00018-018-2839-3
Trapnell, C., Williams, B. A., Pertea, G., Mortazavi, A., Kwan, G., Van Baren, M. J., et al. (2010). Transcript assembly and quantification by RNA-Seq reveals unannotated transcripts and isoform switching during cell differentiation. Nat. Biotechnol. 28, 511–515. doi: 10.1038/nbt.1621
Truniger, V., and Aranda, M. A. (2009). Recessive resistance to plant viruses. Adv. Virus Res. 75, 119–159. doi: 10.1016/S0065-3527(09)07504-6
Vanneste, S., and Friml, J. (2013). Calcium: the missing link in auxin action. Plants (Basel) 2, 650–675. doi: 10.3390/plants2040650
Wang, W., Barnaby, J. Y., Tada, Y., Li, H., Tor, M., Caldelari, D., et al. (2011). Timing of plant immune responses by a central circadian regulator. Nature 470, 110–114. doi: 10.1038/nature09766
Wang, D., Dawadi, B., Qu, J., and Ye, J. (2021). Light-engineering Technology for Enhancing Plant Disease Resistance. Front. Plant Sci. 12:805614. doi: 10.3389/fpls.2021.805614
Wang, A., and Krishnaswamy, S. (2012). Eukaryotic translation initiation factor 4E-mediated recessive resistance to plant viruses and its utility in crop improvement. Mol. Plant Pathol. 13, 795–803. doi: 10.1111/j.1364-3703.2012.00791.x
Wang, D. G., Ma, Y., Yang, Y. Q., Liu, N., Li, C. Y., Song, Y. P., et al. (2011). Fine mapping and analyses of R-SC8 resistance candidate genes to soybean mosaic virus in soybean. Theor. Appl. Genet. 122, 555–565. doi: 10.1007/s00122-010-1469-4
Wang, Y., Pan, Y., Liu, Z., Zhu, X. W., Zhai, L. L., Xu, L., et al. (2013). De novo transcriptome sequencing of radish (Raphanus sativus L.) and analysis of major genes involved in glucosinolate metabolism. BMC Genomics 14:836. doi: 10.1186/1471-2164-14-836
Wani, S. H., Anand, S., Singh, B., Bohra, A., and Joshi, R. (2021). WRKY transcription factors and plant defense responses: latest discoveries and future prospects. Plant Cell Rep. 40, 1071–1085. doi: 10.1007/s00299-021-02691-8
Wei, K., and Chen, H. (2018). Comparative functional genomics analysis of bHLH gene family in rice, maize and wheat. BMC Plant Biol. 18:309. doi: 10.1186/s12870-018-1529-5
Wei, X., Zhang, Y., Zhao, Y., Xie, Z., Hossain, M. R., Yang, S., et al. (2021). Root transcriptome and metabolome profiling reveal key Phytohormone-related genes and pathways involved Clubroot resistance in Brassica rapa L. Front. Plant Sci. 12:759623. doi: 10.3389/fpls.2021.759623
Wen, R. H., Khatabi, B., Ashfield, T., Maroof, M. A. S., and Hajimorad, M. R. (2013). The HC-pro and P3 Cistrons of an Avirulent soybean mosaic virus are recognized by different resistance genes at the complex Rsv1 locus. Mol. Plant-Microbe Interact. 26, 203–215. doi: 10.1094/MPMI-06-12-0156-R
Win, J., Chaparro-Garcia, A., Belhaj, K., Saunders, D. G., Yoshida, K., Dong, S., et al. (2012). Effector biology of plant-associated organisms: concepts and perspectives. Cold Spring Harb. Symp. Quant. Biol. 77, 235–247. doi: 10.1101/sqb.2012.77.015933
Xiang, S., Wu, S., Zhang, H., Mou, M., Chen, Y., Li, D., et al. (2020). The PIFs redundantly control plant defense response against Botrytis cinerea in Arabidopsis. Plants (Basel) 9:1246. doi: 10.3390/plants9091246
Xie, Y., Liu, Y., Wang, H., Ma, X., Wang, B., Wu, G., et al. (2017). Phytochrome-interacting factors directly suppress MIR156 expression to enhance shade-avoidance syndrome in Arabidopsis. Nat. Commun. 8:348. doi: 10.1038/s41467-017-00404-y
Xu, X., Paik, I., Zhu, L., and Huq, E. (2015). Illuminating Progress in Phytochrome-mediated light signaling pathways. Trends Plant Sci. 20, 641–650. doi: 10.1016/j.tplants.2015.06.010
Xu, H. Y., Zhang, C., Li, Z. C., Wang, Z. R., Jiang, X. X., Shi, Y. F., et al. (2018). The MAPK kinase kinase GmMEKK1 regulates cell death and defense responses. Plant Physiol. 178, 907–922. doi: 10.1104/pp.18.00903
Yang, Y., Chen, T., Dai, X., Yang, D., Wu, Y., Chen, H., et al. (2022). Comparative transcriptome analysis revealed molecular mechanisms of peanut leaves responding to Ralstonia solanacearum and its type III secretion system mutant. Front. Microbiol. 13:998817. doi: 10.3389/fmicb.2022.998817
Yang, Q. H., and Gai, J. Y. (2011). Identification, inheritance and gene mapping of resistance to a virulent soybean mosaic virus strain SC15 in soybean. Plant Breed. 130, 128–132. doi: 10.1111/j.1439-0523.2010.01797.x
Yang, Y. Q., Lin, J., Zheng, G. J., Zhang, M. C., and Zhi, H. J. (2014). Recombinant soybean mosaic virus is prevalent in Chinese soybean fields. Arch. Virol. 159, 1793–1796. doi: 10.1007/s00705-014-1980-z
Yang, Y. Q., Zheng, G. J., Han, L., Wang, D. G., Yang, X. F., Yuan, Y., et al. (2013). Genetic analysis and mapping of genes for resistance to multiple strains of soybean mosaic virus in a single resistant soybean accession PI 96983. Theor. Appl. Genet. 126, 1783–1791. doi: 10.1007/s00122-013-2092-y
Ye, J., Coulouris, G., Zaretskaya, I., Cutcutache, I., Rozen, S., and Madden, T. L. (2012). Primer-BLAST: a tool to design target-specific primers for polymerase chain reaction. BMC Bioinformatics 13:134. doi: 10.1186/1471-2105-13-134
Young, M. D., Wakefield, M. J., Smyth, G. K., and Oshlack, A. (2010). Gene ontology analysis for RNA-seq: accounting for selection bias. Genome Biol. 11:R14. doi: 10.1186/gb-2010-11-2-r14
Yu, Y., Zhang, S., Yu, Y., Cui, N., Yu, G., Zhao, H., et al. (2023). The pivotal role of MYB transcription factors in plant disease resistance. Planta 258:16. doi: 10.1007/s00425-023-04180-6
Yuan, P., Jauregui, E., Du, L., Tanaka, K., and Poovaiah, B. W. (2017). Calcium signatures and signaling events orchestrate plant-microbe interactions. Curr. Opin. Plant Biol. 38, 173–183. doi: 10.1016/j.pbi.2017.06.003
Yuan, P., Jewell, J. B., Behera, S., Tanaka, K., and Poovaiah, B. W. (2020). Distinct molecular pattern-induced calcium signatures Lead to different downstream transcriptional regulations via AtSR1/CAMTA3. Int. J. Mol. Sci. 21:8163. doi: 10.3390/ijms21218163
Yuan, P., Tanaka, K., and Poovaiah, B. W. (2021). Calmodulin-binding transcription activator AtSR1/CAMTA3 fine-tunes plant immune response by transcriptional regulation of the salicylate receptor NPR1. Plant Cell Environ. 44, 3140–3154. doi: 10.1111/pce.14123
Yuan, Y., Yang, Y. Q., Shen, Y. C., Yu, K. S., Wang, L. Q., Ren, R., et al. (2020). Mapping and functional analysis of candidate genes involved in resistance to soybean (Glycine max) mosaic virus strain SC3. Plant Breed. 139, 618–625. doi: 10.1111/pbr.12799
Zang, L., Zheng, T., Chu, Y., Ding, C., Zhang, W., Huang, Q., et al. (2015). Genome-wide analysis of the Fasciclin-like arabinogalactan protein gene family reveals differential expression patterns, localization, and salt stress response in Populus. Frontiers in Plant Sci. 6:1140. doi: 10.3389/fpls.2015.01140
Zaynab, M., Fatima, M., Abbas, S., Sharif, Y., Umair, M., Zafar, M. H., et al. (2018). Role of secondary metabolites in plant defense against pathogens. Microb. Pathog. 124, 198–202. doi: 10.1016/j.micpath.2018.08.034
Zhang, L., Du, L., and Poovaiah, B. W. (2014). Calcium signaling and biotic defense responses in plants. Plant Signal. Behav. 9:e973818. doi: 10.4161/15592324.2014.973818
Zhang, H., Li, L., He, Y., Qin, Q., Chen, C., Wei, Z., et al. (2020). Distinct modes of manipulation of rice auxin response factor OsARF17 by different plant RNA viruses for infection. Proc. Natl. Acad. Sci. U. S. A. 117, 9112–9121. doi: 10.1073/pnas.1918254117
Zhang, L., Shang, J., Wang, W., Du, J., Li, K., Wu, X., et al. (2019). Comparison of transcriptome differences in soybean response to soybean mosaic virus under Normal light and in the shade. Viruses 11:793. doi: 10.3390/v11090793
Zhang, H., Tan, X., Li, L., He, Y., Hong, G., Li, J., et al. (2019). Suppression of auxin signalling promotes rice susceptibility to Rice black streaked dwarf virus infection. Mol. Plant Pathol. 20, 1093–1104. doi: 10.1111/mpp.12814
Zhang, R., Yang, C., Jiang, Y., and Li, L. (2019). A PIF7-CONSTANS-centered molecular regulatory network underlying shade-accelerated flowering. Mol. Plant 12, 1587–1597. doi: 10.1016/j.molp.2019.09.007
Zhang, M., and Zhang, S. (2022). Mitogen-activated protein kinase cascades in plant signaling. J. Integr. Plant Biol. 64, 301–341. doi: 10.1111/jipb.13215
Zhao, Q., Li, H., Sun, H., Li, A., Liu, S., Yu, R., et al. (2018). Salicylic acid and broad spectrum of NBS-LRR family genes are involved in SMV-soybean interactions. Plant Physiol. Biochem. 123, 132–140. doi: 10.1016/j.plaphy.2017.12.011
Zhao, P., Zhang, X., Gong, Y., Wang, D., Xu, D., Wang, N., et al. (2021). Red-light is an environmental effector for mutualism between begomovirus and its vector whitefly. PLoS Pathog. 17:e1008770. doi: 10.1371/journal.ppat.1008770
Zheng, C., Chen, P., and Gergerich, R. (2005). Characterization of resistance to soybean mosaic virus in diverse soybean germplasm. Crop Sci. 45, 2503–2509. doi: 10.2135/cropsci2005.0114
Zheng, G. J., Yang, Y. Q., Ma, Y., Yang, X. F., Chen, S. Y., Ren, R., et al. (2014). Fine mapping and candidate gene analysis of resistance gene R-SC3Q to soybean mosaic virus in Qihuang 1. J. Integr. Agric. 13, 2608–2615. doi: 10.1016/S2095-3119(13)60738-8
Zhivotovsky, B., and Orrenius, S. (2011). Calcium and cell death mechanisms: a perspective from the cell death community. Cell Calcium 50, 211–221. doi: 10.1016/j.ceca.2011.03.003
Zhou, X., Gao, H., Zhang, X., Khashi, U. R. M., Mazzoleni, S., Du, M., et al. (2023). Plant extracellular self-DNA inhibits growth and induces immunity via the jasmonate signaling pathway. Plant Physiol. 192, 2475–2491. doi: 10.1093/plphys/kiad195
Zhou, J. M., and Zhang, Y. (2020). Plant immunity: danger perception and signaling. Cells 181, 978–989. doi: 10.1016/j.cell.2020.04.028
Zou, X., Du, M., Liu, Y., Wu, L., Xu, L., Long, Q., et al. (2021). CsLOB1 regulates susceptibility to citrus canker through promoting cell proliferation in citrus. Plant J. 106, 1039–1057. doi: 10.1111/tpj.15217
Keywords: soybean, soybean mosaic virus, RNA sequencing, DEGs, PPI network
Citation: Li H, Liu J, Yuan X, Chen X and Cui X (2023) Comparative transcriptome analysis reveals key pathways and regulatory networks in early resistance of Glycine max to soybean mosaic virus. Front. Microbiol. 14:1241076. doi: 10.3389/fmicb.2023.1241076
Received: 16 June 2023; Accepted: 22 September 2023;
Published: 19 October 2023.
Edited by:
Prabu Gnanasekaran, Washington State University, United StatesReviewed by:
Ved Prakash, Kansas State University, United StatesCopyright © 2023 Li, Liu, Yuan, Chen and Cui. This is an open-access article distributed under the terms of the Creative Commons Attribution License (CC BY). The use, distribution or reproduction in other forums is permitted, provided the original author(s) and the copyright owner(s) are credited and that the original publication in this journal is cited, in accordance with accepted academic practice. No use, distribution or reproduction is permitted which does not comply with these terms.
*Correspondence: Xin Chen, Y3hAamFhcy5hYy5jbg==; Xiaoyan Cui, Y3h5QGphYXMuYWMuY24=
Disclaimer: All claims expressed in this article are solely those of the authors and do not necessarily represent those of their affiliated organizations, or those of the publisher, the editors and the reviewers. Any product that may be evaluated in this article or claim that may be made by its manufacturer is not guaranteed or endorsed by the publisher.
Research integrity at Frontiers
Learn more about the work of our research integrity team to safeguard the quality of each article we publish.