- 1College of Food Science and Biology, Hebei University of Science and Technology, Shijiazhuang, Hebei, China
- 2School of Health Science and Engineering, University of Shanghai for Science and Technology (USST), Shanghai, China
Objective: Lactate dehydrogenase (ldh) in lactic acid bacteria is an important enzyme that is involved in the process of milk fermentation. This study aimed to explore the changes and effects of fermented milk metabolites in mutant strains after knocking out the ldh gene of Lacticaseibacillus paracasei.
Methods: The ldh mutant ΔAF91_07315 was obtained from L. paracasei using clustered regularly interspaced short palindromic repeats technology, and we determined fermented milk pH, titratable acidity, viable count, and differential metabolites in the different stages of milk fermentation that were identified using metabolomic analysis.
Results: The results showed that the growth rate and acidification ability of the mutant strain were lower than those of the wild-type strain before the end of fermentation, and analysis of the differential metabolites showed that lactate, L-cysteine, proline, and intermediate metabolites of phenylalanine, tryptophan, and methionine were downregulated (P < 0.05), which affected the growth initiation rate and acidification ability of the strain. At the end of fermentation (pH 4.5), the fermentation time of the mutant strain was prolonged and all differential metabolites were upregulated (P < 0.05), including amino acids and precursors, acetyl coenzyme A, and other metabolites involved in amino acid and fatty acid synthesis, which are associated with the regulation of fermented milk flavors. In addition, riboflavin was upregulated to promote the growth of the strain and compensate for the growth defects caused by the mutation.
Conclusion: Our data established a link between the AF91_07315 gene and strain growth and metabolism and provided a target for the regulation of fermented milk flavor substances.
1. Introduction
Fermented milk is a nutrient product made from milk co-cultured with a starter culture and is widely accepted for its unique taste and flavor (Wang et al., 2021c). Lactic acid bacteria (LAB) are a traditional starter culture that uses milk as a means to produce nutrients and flavored substances. These substances include organic acid, peptides, amino acids, and short-chain fatty acids, which improve the digestion and bioavailability of milk components, inhibit harmful bacteria in the gastrointestinal tract, prevent cancer, reduce lactose intolerance, and lower cholesterol levels (Shiby and Mishra, 2013). Organic acid is the main component of fermented milk, and lactic acid enhances the flavor of fermented milk and maintains probiotics in the human gastrointestinal gut, which is beneficial against septic and bacterial infections, stimulates intestinal motility, and enhances the immune response to antigenic invasion (Shukla et al., 2008; Vieco-Saiz et al., 2019; Mathur et al., 2020). However, the mechanism of metabolic regulation by LAB in fermented milk is unclear, and understanding LAB metabolic pathways can help regulate the production of flavored substances in fermented milk.
Lactate dehydrogenase, a key enzyme in the glycolytic pathway for lactate production, uses pyruvate as an electron acceptor, accompanied by the oxidation of nicotinamide adenine dinucleotide, to catalyze the pyruvate reaction to produce lactate (Kandler, 1983). To date, genetic engineering has been applied to knockout and overexpress ldh to change the energy flow and achieve high industrial lactate production (Singhvi et al., 2018; Bleckwedel et al., 2020). Furthermore, changes in the flavor of fermented milk caused by ldh gene deletion have been investigated. This study showed that lactic acid production was eliminated by knocking out the ldh gene in Thermoanaerobacterium saccharolyticum JW/SL-YS485, thereby increasing acetic acid and ethanol production (Desai et al., 2004). In addition, the elimination of ldhD using the double cross strategy increased the production of acetaldehyde, diacetyl, and acetoin in Lactobacillus johnsonii (Lapierre et al., 1999). These flavored substances have only been characterized in the medium; however, few studies have investigated the role of lactate dehydrogenase in fermented milk, and effective characterization of its metabolic components during fermentation is important for improving its flavor.
Mass spectrometry-based untargeted metabolomics is a highly specific and sensitive technology for monitoring small molecular substrates produced by microorganisms, and it screens samples for biomarkers or differential substances and helps identify differential metabolites that modulate phenotypes (Johnson et al., 2016; Guijas et al., 2018). Research on milk metabolomics has mainly focused on the effect of Streptococcus thermophilus (S. thermophilus), co-cultured with lactobacilli on the fermentation process and quality (Tomassini et al., 2019; Li et al., 2021), and there are few metabolic studies on single-strain fermented milk. We used L. paracasei CGMCC4691 as a test bacterium owing to its beneficial characteristics. As a co-fermenter of fermented milk, it can enhance immunity, improve intestinal metabolites, and regulate intestinal flora. In addition, the inactivated strain can improve the brain–intestinal axis and reduce continuous damage to brain nerves (Miao et al., 2022; Zhang et al., 2022). In this study, the mutant strain ΔAF91_07315 was generated from L. paracasei using the clustered regularly interspaced short palindromic repeats (CRISPR) technology, and pH, titratable acidity (TA), and bacterial counts were compared between the wild-type (WT) and ΔAF91_07315 strains. In addition, differential metabolites were identified using non-targeted metabolomics to assess the metabolic role of ΔAF91_07315 and provide targets for the modulation of flavored substances.
2. Materials and methods
2.1. Strains, culture media, and growth conditions
L. paracasei CGMCC4691 was obtained from our laboratory. The pLL plasmid was provided by the University of Shanghai for Science and Technology. E. coli Top10 competent cell was purchased from TianGen Biotech. L. paracasei CGMCC4691 and ΔAF91_07315 mutants were cultured in De Man Rogosa Sharpe (MRS) medium at 37 °C for 14–16 h for skim milk fermentation. E. coli Top10 was used for cloning and was grown on Luria Bertani medium at 30 °C for 12–14 h. When needed, a suitable antibiotic was supplemented at 10 μg/ml for ΔAF91_07315 and 50 μg/ml for E. coli Top10.
2.2. Construction of L. paracasei CGMCC4691 ΔAF91_07315
2.2.1. Construction of knockout plasmids
The knockout plasmid was constructed using polymerase chain reaction (PCR) and seamless cloning technology. The primers used in this study are presented in Table 1. First, the vector skeleton was generated using an ApaI-XbaI (Vazyme Biotech Co., Ltd.)-digested pLL plasmid. Three fragments, 1,300 bp homologous arms upstream and downstream (obtained by PCR using primers up/down-F/R with L. paracasei CGMCC4691 genome as a template), and sgRNA (obtained by PCR using primers sgRNA-F/R with pLL as a template) were generated and purified using a gel purification kit (Axygen, SV, USA), all of which were connected to generate up–down-sgRNA fragments. The resulting fragment was ligated into the vector skeleton to produce pLL-07315 using a seamless cloning kit (Vazyme Biotech Co., Ltd.) and verified using ldhYZ-F/R following transformation into E. coli Top10 competent cells.
2.2.2. Deletion of the AF91_07315 gene
A competent cell of L. paracasei CGMCC4691 was prepared as described by Song et al. (2017). The constructed plasmid was electroporated into cells using a GenePulser Xcell (Bio-Rad) and a 2 mm cuvette (BTX) at 2.0 KV, 200Ω, and 25 μF. After recovery for 3 h in the MRS medium, the cells were separated on agar plates and grown for 72 h. A single strain was selected and verified by PCR and named pLL: 07315. Precise sequencing was also performed using BGI.
2.2.3. Fermented milk preparation
Skim milk (10% w/v) was prepared from skim milk powder (Shijiazhuang Junlebao Dairy Co., Ltd.). Skim milk powder was dissolved in 50°C sterile water and autoclaved at 115 °C for 15 min. After cooling to 37 °C, 400 ml of milk was transferred into six different sterile flasks. Pre-cultured L. paracasei CGMCC4691 and its mutants were inoculated in triplicate at a 5% inoculum size (2×107 CFU). The mixed milk was fermented until the pH reached an end-point of 4.5. Subsequently, a sample from each flask was collected before (4 h) and after (8 h) the fermentation periods and end-points. Before analysis, these samples were stored at −80 °C.
2.3. TA and bacterial count determination
2.3.1. Determination of pH and TA
pH was measured using a pH meter (METTLER TOLEDO, China). TA was determined using 0.1 mol/L NaOH with phenolphthalein as a color indicator, and the result was expressed as °T.
2.3.2. Determination of viable count
The viable counts of different periods during milk fermentation were determined as follows: 1 ml of the sample was diluted in 9 ml of normal saline (0.85%) by vortex oscillation, and the dilution was performed continuously. The diluted liquid (100 μl) was cultured using the MRS agar plate pouring method. The viable counts were calculated after incubation at 37 °C for 48 h and expressed as CFU/ml.
2.4. UPLC-Q-TOF MSE analysis
2.4.1. Sample pretreatment
The fermented milk samples stored at −80 °C were thawed. We transferred 50 mg of each sample into a fresh centrifuge tube, and 400 μl methanol solution (containing 5 μg/ml L-2-chloro-phenylalanine as the internal standard) was added and vortexed for 1 min. The sample was then mixed two times at 60 Hz for 3 min and centrifuged at 13,000 rpm at 4°C for 10 min using a cryogenic centrifuge (Thermofisher FRESCO21, USA). Supernatants were collected for analysis. Equal volumes of all samples were mixed as quality control samples. All reagents were analytically pure and purchased from Shanghai.
2.4.2. Ultra performance liquid chromatography analysis
The samples were analyzed on an Agilent 1290 infinity (USA), incorporating a HSS T3 chromatographic column (water, 2.5 μm, 100*2.1 mm). The mobile phases A and B consisted of water and acetonitrile (mixed with 0.1% formic acid). The flow rate of the mobile phase was 0.4 ml/min at a column temperature of 40°C. The injection volume was 4 μl. The optimized gradient elution was 0–3 min, 20% B; 3–9 min, 20–95% B; 9–13 min, 95% B; 13–13.1 min, 95–5% B; and 13.1–16 min, 5% B.
2.4.3. Mass spectrometry (MS) analysis
Mass spectrometry (MS) analysis was performed using a Q-TOF-MSE (Agilent 6545 UHD, USA), coupled with positive (ESI+) and negative (ESI–) modes. The capillary voltage was 4.5 kV and 3.5 kV in ESI+ and ESI–, respectively, and the drying gas flow was 8 L/min and 10 L/min at a temperature of 325°C, respectively. The nebulizer voltage was set at 20 psi. The fragmentation voltage was set to 120 V. The MS scans were performed over an entire information range of 50–1,500 m/z.
2.5. Statistical analysis
Fermentation was performed in triplicate for the starter culture. Metabolic data were collected using Agilent MassHunter Qualitative Analysis B.0 8.0 software. A series of procedures were performed using the R software XCMS package, including peak identification, retention time correction, and automatic integration. The chromatographic peak values were normalized by dividing the original peak area by the internal standard peak. Differences in metabolic profiles and visual differences were achieved using R-script, such as principal component analysis and orthogonal projections to latent structures discriminant analysis (OPLS-DA).
Differential metabolites were identified using the OPLS-DA model, variable importance in the projection (VIP ≥1), independent sample t-test (P < 0.05), and fold change (FC ≥1.1 or ≤0.9). The differential metabolites of interest were identified by matching accurate molecular weights against the Kyoto Encyclopedia of Genes and Genomes (KEGG; https://www.kegg.jp) online database using the following qualitative methods: the adduct ions in ESI+ mode included [M+H]+ and [M+Na+]+ and those in ESI– mode included [M–H] –; the quality error value was selected as 30 ppm. Thereafter, the metabolic pathway was matched against KEGG using MetaboAnalyst software, and the metabolic effects were assessed by metabolic enrichment analysis.
3. Results
3.1. Construction of L. paracasei CGMCC4691ΔAF91_07315
To produce mutant strains, cells were transformed with pLL-07315. After obtaining a single-clone strain, specific primers flanking the homologous arms were designed for verification using PCR (Figure 1A). The results are shown in Figure 1. The sequencing results further confirmed the gene deletion (Figure 1B).
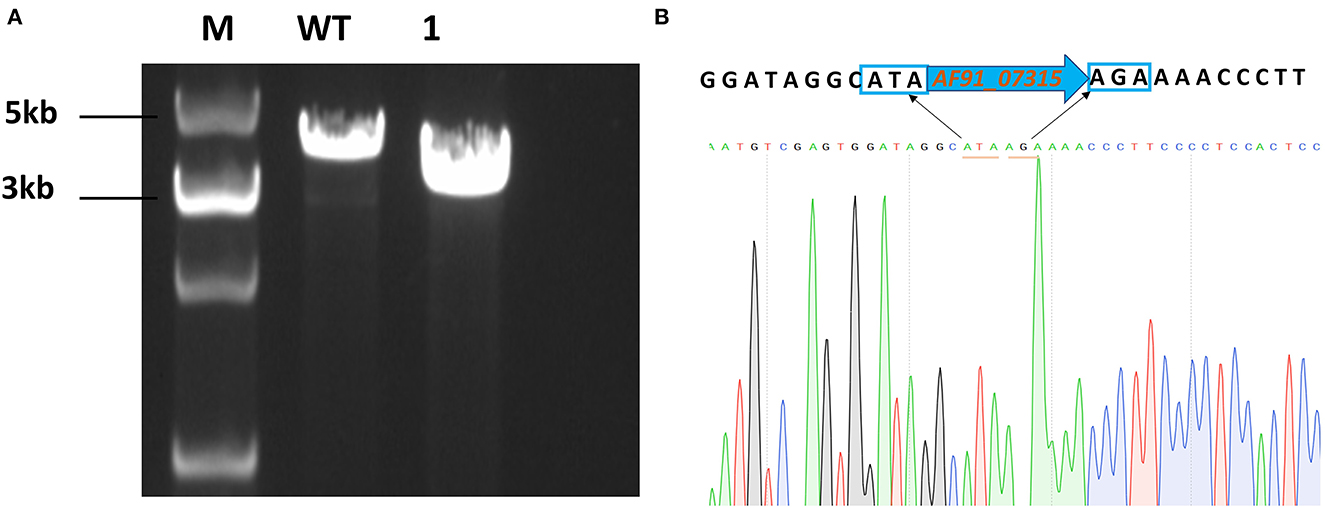
Figure 1. Chromosomal deletion of ldh (AF91_07315). (A) PCR identification of ΔAF91_07315 mutants. M:5000 bp DNA marker; WT: wild-type strain was used as control (3735bp); lane 1: a mutant strain of AF91_07315 (2844bp). (B) Sequencing of ΔAF91_07315 mutant.
3.2. Fermented milk characteristics of L. paracasei CGMCC4691 and mutants
The pH, TA, and viable counts in the fermented milk were measured every 2 h until the pH reached 4.5. The results are shown in Figure 2. The pH and TA showed opposite trends, and differences were observed as pH decreased (Figure 2A). At 8 h of fermentation, the pH of the mutant strain was 0.4 points higher than the WT strain. Simultaneously, the TA of the mutant strain increased to 33 °T, which was 12 °T higher than that of the WT strain. The fermentation times of the WT and mutant strains were 10 h and 12 h, respectively, at the fermentation end-point. The viable counts consistently decreased during fermentation, and the growth of the WT and mutant strains reached the exponential phase at 4 h (6.4 × 107 CFU/ml) and 6 h (7.5 × 107 CFU/ml), respectively. Next, samples were collected for metabolomic analysis.
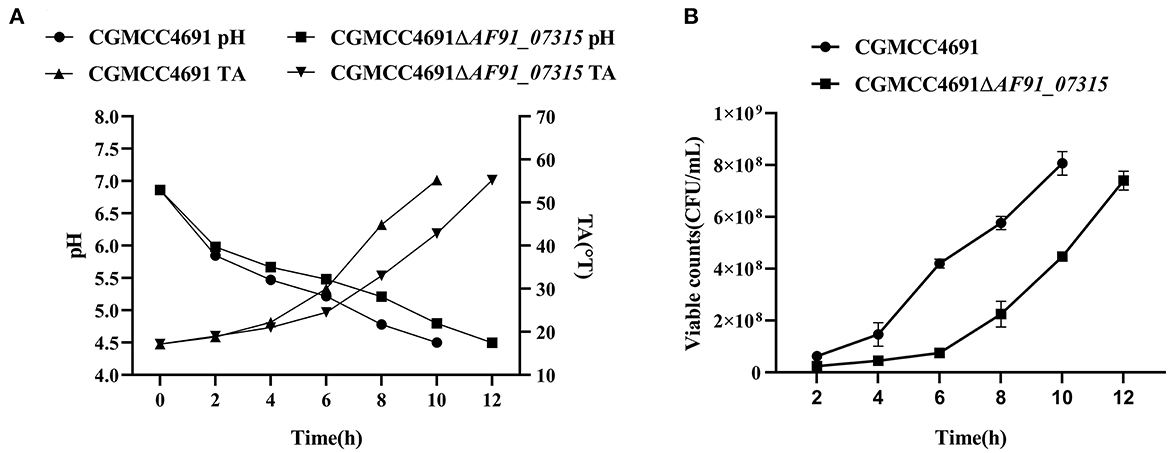
Figure 2. Summary of fermentation characteristics; pH, titration acidity (A), and viable counts (B) of L. paracasei CGMCC4691 and L. paracasei CGMCC4691 ΔAF91_07315. The average was calculated from three independent biological replication. The error bars represent the standard deviation.
3.3. Untargeted metabolic analysis of L. paracasei CGMCC4691 and L. paracasei CGMCC4691ΔAF91_07315
3.3.1. Differential metabolic analysis of fermented milk at pre-fermentation
OPLS-DA, a common classification method, was performed to identify the differences between the two groups, which exploits dimension reduction by combining regression models; the regression results were analyzed using a discriminant threshold. A total of 1,096 and 927 substances were generated from the fermented milk in the ESI+ and ESI– modes, respectively. The OPLS-DA of fermented milk is shown in Figure 3. The abscissa represents the principal component score during the quadrature signal correlation; the farther the location of the two milk samples, the greater the difference between the two groups. The ordinate represents the quadrature component score value; the farther the parallel samples are, the greater the difference within the group. The milk fermented by the WT strain at 4 h (W1) was located away from the milk fermented by the mutant strain (M1), indicating that the two groups had significant differences, and a total of 52.4% was explained by the dataset. The critical parameters used to evaluate the OPLS-DA model are presented in Table 2. In the ESI+ model, the R2X, R2Y, and Q2 values were 0.524, 1, and 0.654, respectively. In the ESI– model, the R2X, R2Y, and Q2 values were 0.632, 0.999, and 0.762, respectively, where R2 and Q2 represent the explanatory ratio and predictability, respectively. The closer the value is to 1, the higher the model accuracy. Generally, when R2 and Q2 >0.5, the model fits well.
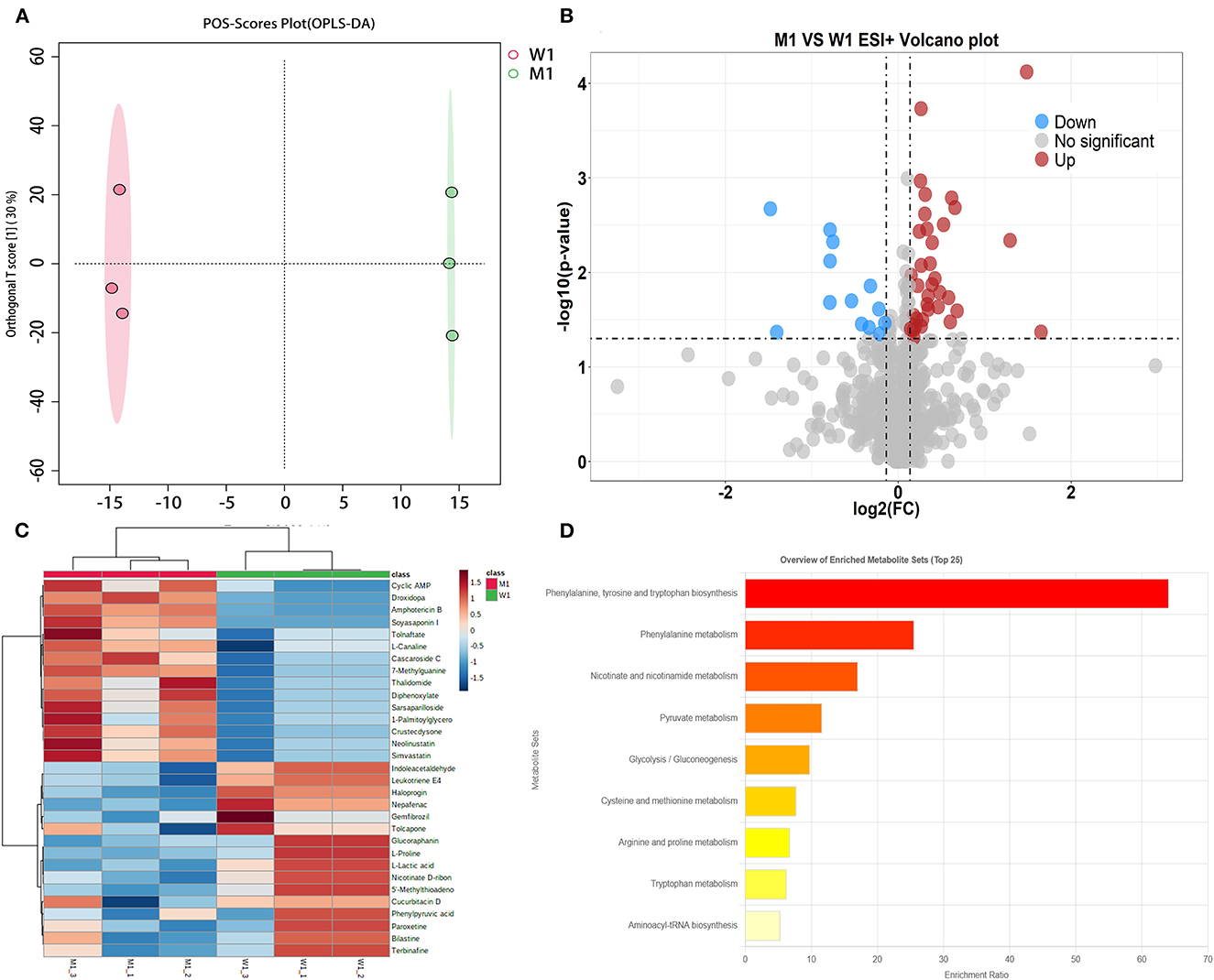
Figure 3. Fermented milk metabolomic analysis of wild-type strain CGMCC4691 (W1) and its mutant strain ΔAF91_07315 (M1) at 4 h. (A) Score plot of orthogonal partial least squares discriminant analysis (OPLS-DA). (B) Volcano plot showing differential metabolites in comparison with W1 in the positive ion (ESI+) model, where each dot represents a substance. The abscissa and ordinate indicate fold change (FC) and p-value, respectively, after data processing. (C) Heatmap indicates the relative abundance of differential metabolites between the two sample groups. (D) Pathway enrichment analysis of differential metabolites. The x-axis indicates metabolic pathway impact values obtained from topological analysis and the color scale represents the contribution of metabolites in the pathway. Red indicates the highest and yellow indicates the lowest.
To select the differential metabolites, a volcano plot was generated from the OPLA-DA filtering results (Figure 3B). In ESI+, the red dots indicate that the metabolites were upregulated, with FC >1.1 and P ≤ 0.05, and the blue dots indicate that the metabolites were downregulated, with FC <0.9 and P ≤ 0.05. The farther the dot is from the origin, the greater the difference between the two groups. A total of 51 metabolites were identified. Next, these potentially differential compounds were further used for enrichment analysis by (1) KEGG ID conversion using the MBRole online database and (2) importing the ID into the 4,691 KEGG metabolic pathways for pathway enrichment analysis. This resulted in 31 compounds, and a heatmap was generated to show their relative abundances (Figure 3C). Enrichment analysis of the two groups was performed using L. paracasei CGMCC4691 (lpq), and nine differential metabolites were enriched in the pathways. The results are shown in Figure 3D, and the metabolites are presented in Table 3. The key pathways include phenylalanine, tyrosine, and tryptophan biosynthesis, as well as phenylalanine metabolism, pyruvate metabolism, and nicotinate and nicotinamide metabolism.
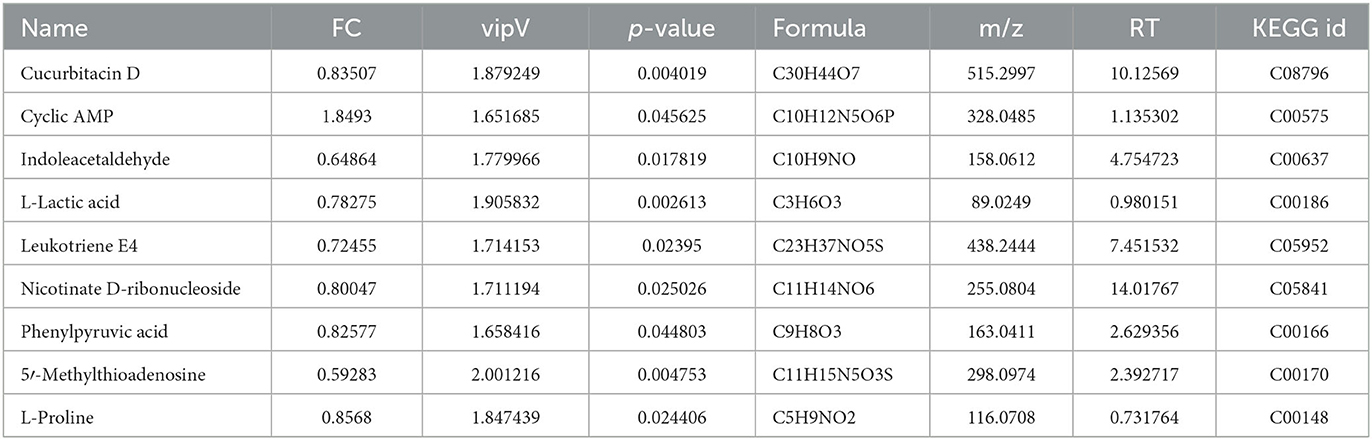
Table 3. Differential metabolites identified at the initial stage of fermentation compared with the wild-type strain.
3.3.2. Differential metabolic analysis of fermented milk at mid-fermentation
A method similar to that described above was used to identify differential metabolites between the two sample groups (M2 and W2) at 8 h. In total, 1,117 differential metabolites were identified as potential candidates (VIP >1) in the OPLS-DA plot, and significant separation was observed in the positive mode (Figure 4A). The model evaluation parameters (R2X, R2Y, and Q2 were 0.806, 1, and 0.896 for ESI+; and 0.541, 0.994, and 0.837 for ESI–) indicated that the model was both reliable and valid. In ESI+, the volcano plot showing the FC of these candidates is presented in Figure 4B, and the criteria were FC >1.1 or ≤0.9 and P ≤ 0.05. We obtained 207 differential substances from the positive model. The dot represents a variable, and the farther the variable is from the origin, the greater the contribution to the difference between the two groups. Subsequently, the KEGG ID of 80 variables was obtained from the MBRole database, and a heatmap was generated to visualize these substances (Figure 4C). Pathway enrichment analysis was performed using these metabolites (Table 4). A total of 18 signaling pathways are shown in Figure 4D, and the top signaling pathways were phenylalanine metabolism, cysteine and methionine metabolism, biotin metabolism, aminoacyl-tRNA biosynthesis, and pyruvate metabolism.
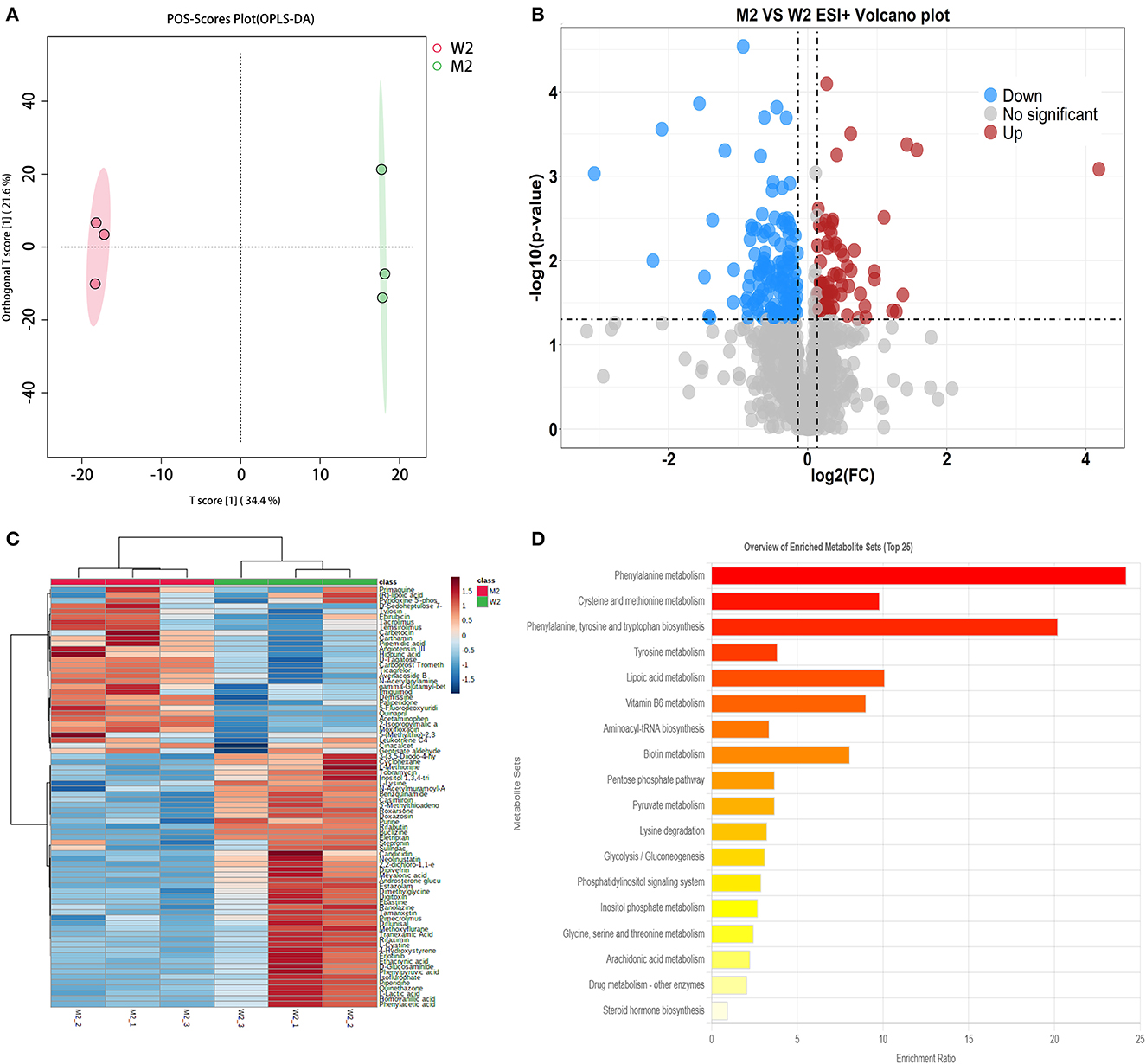
Figure 4. Fermented milk metabolomic analysis of wild-type strain CGMCC4691 (W2) and its mutant strain Δldh (M2) at 8 h. (A) Score plot of orthogonal partial least squares discriminant analysis (OPLS-DA). (B) Volcano plot showing differential metabolites in comparison with W1 in the positive ion (ESI+) model, where each dot represents a substance. The abscissa and ordinate indicate fold change (FC) and p-value, respectively, after data processing. (C) Heatmap represents the relative abundance of differential metabolites between the two sample groups. (D) Pathway enrichment analysis of differential metabolites. The x-axis represents the metabolic pathway impact values obtained from topological analysis, and the color scale represents the contribution of metabolites in the pathway. Red indicates the highest and yellow indicates the lowest.
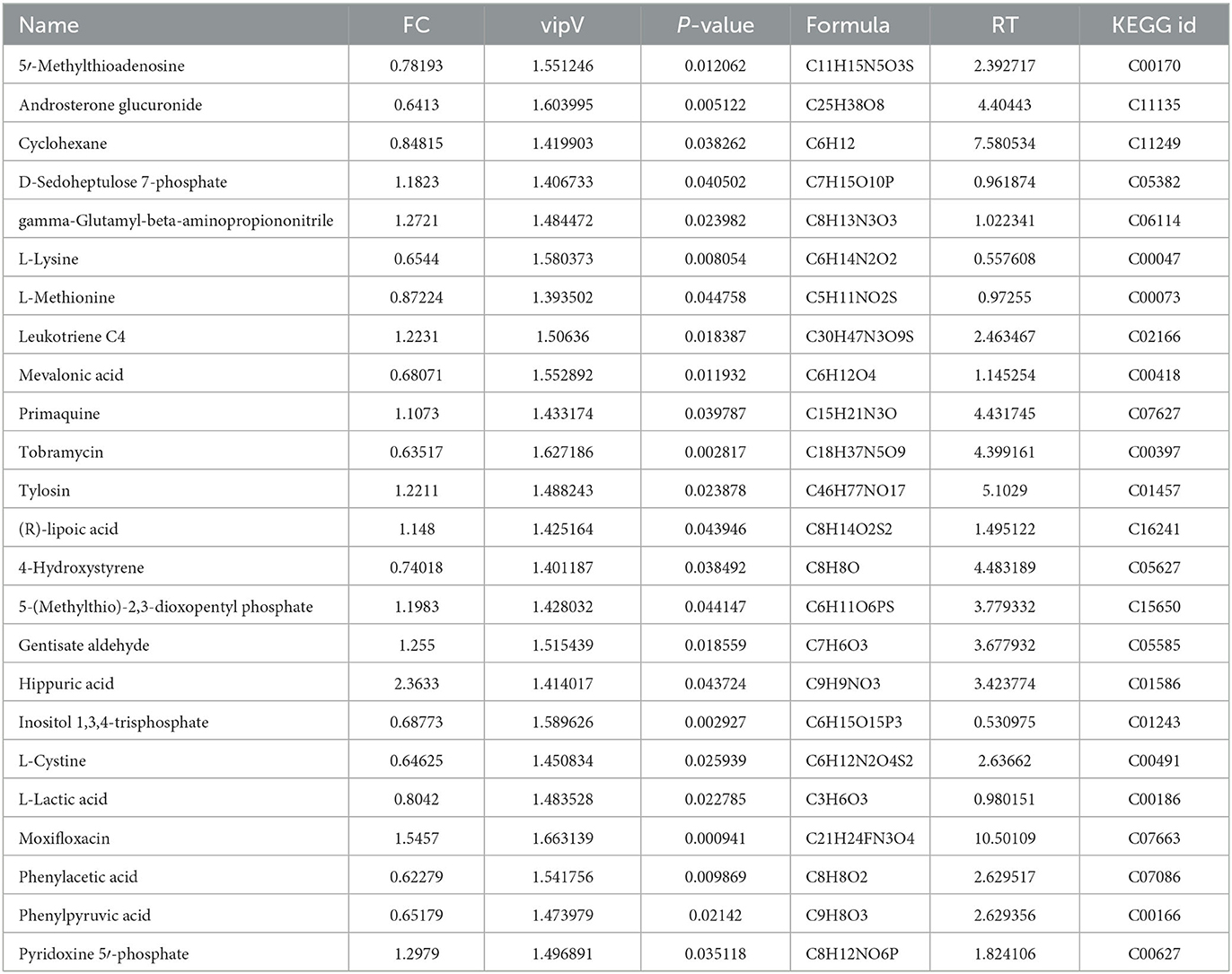
Table 4. Differential metabolites identified at the middle stage of fermentation compared with the wild-type strain.
3.3.3. Differential metabolic analysis of fermented milk at the end of fermentation
The differential metabolites in fermented milk at pH 4.5 were compared using the multiple analyses described above (Figure 5). A total of 1,132 were identified, and OPLS-DA showed significant separation in the positive mode (Figure 5A). The model evaluation parameters (R2X, R2Y, and Q2 = 0.592, 0.997, and 0.759, respectively, in ESI+; and 0.584, 0.996, and 0.73, respectively, in ESI–) are presented in Table 2, which indicates that the model has good stability and predictability. In ESI+, the volcano plot showed 123 differential metabolites according to the criteria mentioned above, where the red and blue dots represent significantly differential metabolites (Figure 5B). Next, two statistical analysis methods were performed to further select featured substances: 49 KEGG IDs were obtained from the MBRole database, and a heatmap was used to visualize their relative abundance (Figure 5C). Pathway enrichment analysis was performed using these metabolites, and the details are presented in Table 5. A total of 20 signaling pathways are presented in Figure 5D, and phenylalanine, tyrosine, and tryptophan biosynthesis, as well as biotin metabolism and pyruvate metabolism were involved in key metabolic pathways.
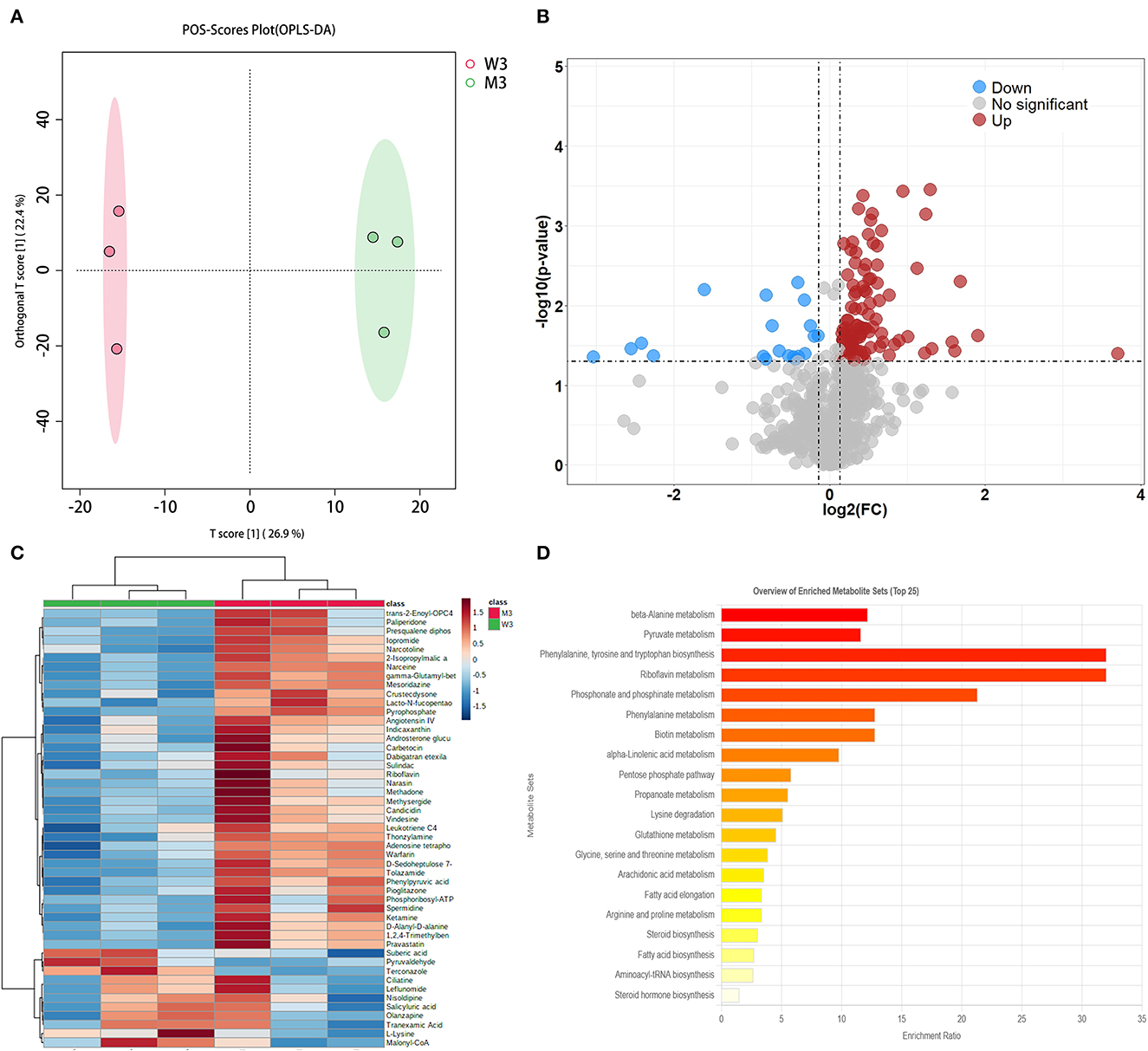
Figure 5. Fermented milk metabolomic analysis of wild-type strain CGMCC4691 (W3) and its mutant strain Δldh (M3) at the end of the fermentation. (A) Score plot of orthogonal partial least squares discriminant analysis (OPLS-DA). (B) Volcano plot showing differential metabolites in comparison with W1 in the positive ion (ESI+) model, where each dot represents a substance. The abscissa and ordinate indicate fold change (FC) and p-value, respectively, after data processing. (C) Heatmap represents the relative abundance of differential metabolites between the two sample groups. (D) Pathway enrichment analysis of differential metabolites. The x-axis indicates the metabolic pathway impact values obtained from topological analysis, and the color scale represents the contribution of metabolites in the pathway. Red indicates the highest and yellow indicates the lowest.
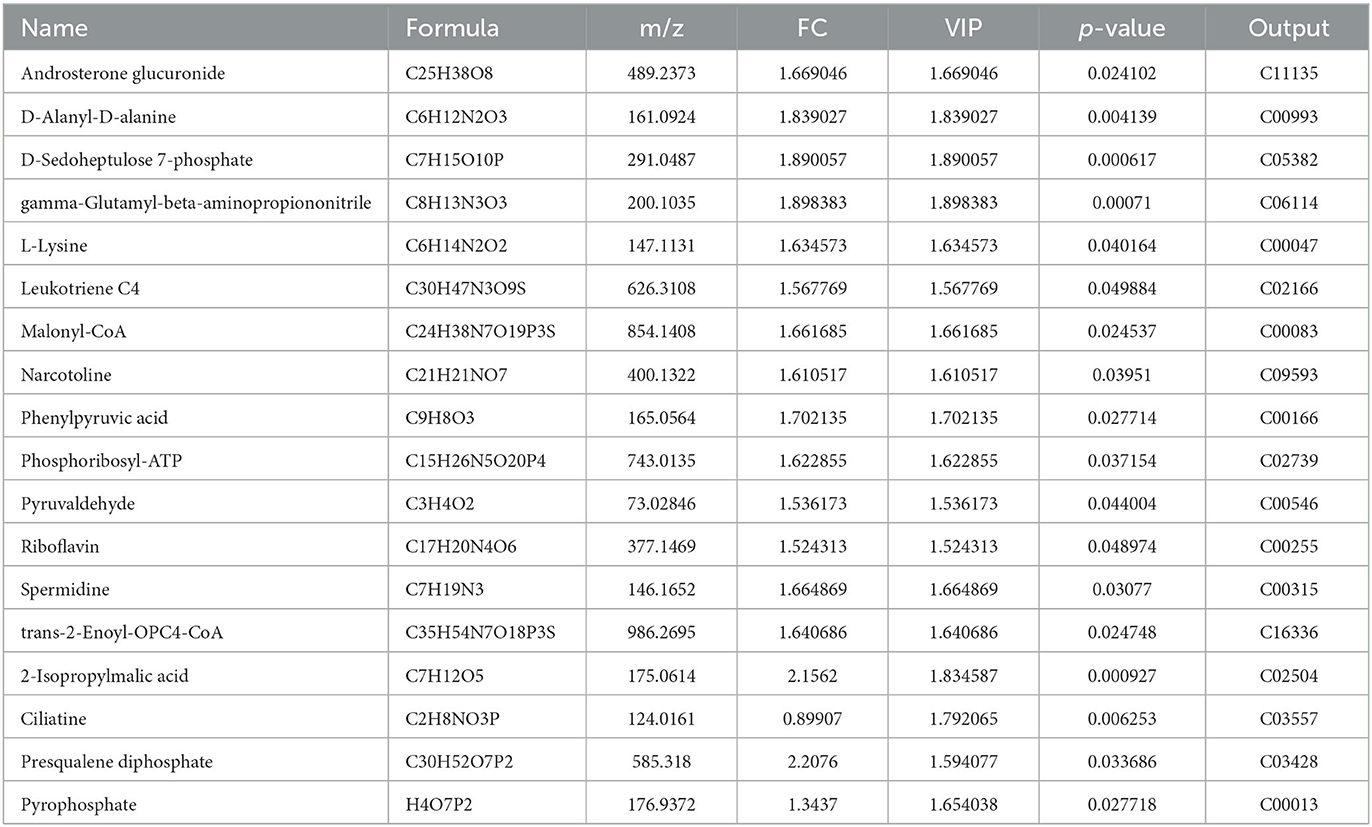
Table 5. Differential metabolites identified at the end of fermentation compared with the wild-type strain.
4. Discussion
4.1. Fermented milk serves as a vector for studying genetic functions
Lactic acid bacteria (LAB) mutants can change the flavor and texture of fermented milk, and the potential functions of these genes can be inferred from changes in the properties of fermented milk (Sorensen et al., 2016). Courtin et al. (2002) developed prtS and prtB mutants of S. thermophilus and L. bulgaricus and confirmed the importance of both genes for strain growth and acidification. Yamauchi et al. (2019) developed a urease-deficient mutant ΔureC of S. thermophilus and confirmed that urease is the essential factor for yogurt acidification. In addition, Xu et al. (2021) inactivated the glutathione transport protein (Ptrp) gene and measured the glutathione content on the cell surface to determine the transport function of Ptrp-2 and Ptrp-4. Therefore, it is important to study the fermentation characteristics of ΔAF91_07315 strains using milk as a carrier. Furthermore, mutant strains affected the production of fermented milk metabolites. Although the AF91_07315 gene mutants obtained using CRISPR technology cannot be applied to food production, the mutant strains in this study can enable us to understand the key metabolic pathways during dairy product development and provide targets for flavor substance interventions.
4.2. Advantages of CRISPR technology
The methods used to generate gene mutants of LAB are classified into natural mutagenesis and genetic engineering. Natural mutagenesis has been applied to L. bulgaricus and L. helveticus to improve the quality and taste of production (Moller et al., 2007; Guan et al., 2021), which mainly depends on random mutagenesis and large-scale labor-intensive screening. Although the double-crossover homologous recombination strategy compensates for this deficiency and has been used to produce highly purified lactate by cloning the ldh gene (Jin et al., 2016; Okano et al., 2018), this method requires the screening of markers. Furthermore, CRISPR gene editing technology has the advantages of high specificity and ease of operation. Tian et al. (2021) used the CRISPR system to knockout and overexpress the ldh gene in L. paracasei NCBIO01-M2 and screened L-lactate strains with a yield of 221 g/L at 45°C and 99% optical and chemical purity. Our previous study optimized the CRISPR system for L. paracasei CGMCC4691; thus, this is the optimal choice to knockout ldh using this technique.
4.3. Viable count differences between the ΔAF91_07315 and WT strains
Yogurt is considered beneficial when the bacterial counts reach 107 CFU/ml (Corcoran et al., 2006). TA and pH directly affect the taste. Therefore, fermentation was completed at a pH of 4.5 to ensure that the fermentation process met commercial criteria. The result showed that the ΔAF91_07315 strain has a lag in both acidity and viability, which is in agreement with Bleckwedel et al., where the ldh1 gene (mainly responsible for lactate production) of Fructobacillus tropaeoli CRL 2034 was inactivated, and cell counts and acidity were lagging (Bleckwedel et al., 2020). Viana et al. (2005) reported that the knockout of ldh affected the growth rate of mutants in different sugars, showing a higher final pH (5.2) than the WT strain (4.5). Even though lag growth was observed, the viable counts at the end of fermentation were consistent with the WT strain; hence, it had no effect on the fundamental metabolism throughout life.
4.4. Differential metabolite screening of fermented milk at different time periods
Non-volatile metabolite profiles were analyzed using liquid chromatography-MS metabolomics during the fermentation process of L. paracasei CGMCC4691, and most compounds were upregulated or downregulated compared to those in the WT strain. Specifically, 31, 80, and 49 differential metabolites were identified in the pre, mid, and end stages of fermentation, respectively. The heatmap shows the relative abundance of differential metabolites, where the mid-term metabolites were higher than the pre-term and end stages of fermentation, suggesting the main role of lactate dehydrogenase in mid-fermentation. After enrichment analysis of the metabolic pathway, 9, 24, and 18 substances were identified; however, other substances were not identified, which could be explained by the selection of a model strain for ID conversion. We used L. paracasei ATCC334 as a model strain for metabolic pathway analysis, and some metabolites were not involved in metabolic pathway conversion as separate substances in L. paracasei CGMCC4691.
4.4.1. Differential metabolites in pre-fermentation
In total, nine differential metabolites were detected in the mutant strains during pre-fermentation when compared to the WT strain. Figure 3D shows the results of the enrichment analysis. The metabolites involved in pyruvate, amino acid, and pyrimidine metabolism included (S)-lactate, phenylpyruvate, L-proline, 5′-methylthioadenosine, and indole-3-acetaldehyde, all of which were downregulated. (S)-lactate, which is catalyzed by lactate dehydrogenase in the last step of glycolysis, provides energy to cells and is the main substance that adds flavor to fermented milk. The ΔAF91_07315 strain failed to reach the exponential growth phase during pre-fermentation, which may be related to reduced lactate metabolism, insufficient energy supply, and delayed growth of the strain (Rico et al., 2008; Jingjing et al., 2021). In addition, proline, phenylpyruvate, and indole-3-acetaldehyde were downregulated, and the growth-promoting effect of proline on Lactobacillus has been demonstrated (Wang et al., 2021a). Sun et al. (2019) added amino acids, uracil, and other substances during the fermentation of L. rhamnosus, which improved the start-up speed. In this study, phenylpyruvic acid, indole-3-acetaldehyde, and 5′-methylthioadenosine as the precursors of phenylalanine, tryptophan, and methionine were less synthesized, which affected the growth of the strain (Shapiro, 1962; Yonezawa et al., 2010; Meng et al., 2021).
4.4.2. Differential metabolites in mid-fermentation
During mid-fermentation, the largest gap in pH and viability was observed when compared to the WT strain, and the metabolites detected at this time were mainly L-methionine, 5′-methylthioadenosine, L-cysteine, phenylpyruvate, phenylacetic acid, L-lysine, and L-lactic acid. In particular, the gap in lactic acid content increased compared to the previous stage, which indicates the major role of lactic acid production in regulating the pH of fermented milk (Morelli et al., 1986; Gaspar et al., 2013), and the knockout of ldh had a significant effect on lactic acid production. Nevertheless, lactic acid was still detected throughout the fermentation phase in the mutant strain, probably owing to the synergistic effects of other genes encoding acid-producing enzymes (Viana et al., 2005). The synthesis of nutrients, such as amino acids, in LAB is limited to fermented milk; therefore, exogenous nitrogen sources are used to promote their growth, such as the breakdown of amino acids and peptides. In this study, the L-cysteine content was reduced in the ΔAF91_07315 strain except for methionine, phenylalanine, and its precursors. Wang et al. (2021b) reported different pathways of amino acid biosynthesis at different stages of fermentation, and the synthesis of L-cysteine may be due to different protein hydrolase activities.
4.4.3. Differential metabolites at the end of fermentation
Fermentation was stopped at pH 4.5 to identify the various differential metabolite strains and the differential metabolites detected were all upregulated in the ΔAF91_07315 strain, including malonyl-CoA, L-lysine, phenylpyruvate, riboflavin, alpha-isopropylmalate, and spermidine, which may be due to the prolonged fermentation time of the mutant strain. In addition, alpha-isopropylmalate and spermidine were involved in pyruvate and arginine metabolism, respectively, providing energy and improving the nutritional quality of fermented milk. Malonyl-CoA is involved in fatty acid synthesis and carbon metabolism, which is related to yogurt flavor (Foster, 2012). Riboflavin is a trace organic substance required for growth and metabolism that stimulates the growth of LAB (Sun et al., 2022). The fermented milk of the ΔAF91_07315 strain did not complete fermentation when the WT strain was fermented at a pH of 4.5, after which the viable counts of the ΔAF91_07315 strain continued fermentation and reached the same pH as the WT strain, probably because riboflavin, L-lysine, alpha-isopropylmalate, and spermidine compensated for the growth defect in the ΔAF91_07315 strain. Further investigation is required because the metabolites of the mutants were upregulated at the end of fermentation, some of which may be toxic, and it is unclear whether there are biosafety issues with fermented milk.
In this study, the ldh gene of L. paracasei CGMCC4691 was knocked out using CRISPR gene editing technology. The fermented milk results showed that the ΔAF91_07315 strain had slower growth and acidification capacities than the WT strain. At the initial stage of fermentation, the WT strain entered the exponential growth phase, and metabolites, including (S)-lactate, phenylpyruvate, L-proline, and indole-3-acetaldehyde, were downregulated compared to the WT strain, which affected the start-up speed of the ΔAF91_07315 strain. At the mid-stage of fermentation, pH and viable counts were the most disparate, with (S)-lactate being the main cause of the differences in pH. In addition, L-cysteine and precursor metabolites involved in amino acid metabolism were reduced because of the influence of protease activity. Although the fermentation time of the mutant strain was prolonged at the end of fermentation, malonyl-CoA, L-lysine, the amino acid intermediate metabolites, phenylpyruvate, and spermidine were upregulated, contributing to the formation of flavored substances. Upregulation of metabolites, such as riboflavin, compensated for the growth defects of the mutant strain. In conclusion, lactate dehydrogenase plays an important role in the growth and acidification of L. paracasei and affects the production of flavored milk substances.
Data availability statement
The original contributions presented in the study are included in the article/supplementary material, further inquiries can be directed to the corresponding author/s.
Author contributions
XS and SW designed this project and revised the article. SF and LC processed the samples and performed the experiments. SF wrote the article. SF and JB collected the data. HL analyzed the data. SW guided the experiment and provided funding for the research. All the authors read and approved the final submission.
Acknowledgments
We would like to acknowledge the financial support from the National Natural Science Foundation of China [grant number 32272361] and 2022-Research and Development-14.
Conflict of interest
The authors declare that the research was conducted in the absence of any commercial or financial relationships that could be construed as a potential conflict of interest.
Publisher's note
All claims expressed in this article are solely those of the authors and do not necessarily represent those of their affiliated organizations, or those of the publisher, the editors and the reviewers. Any product that may be evaluated in this article, or claim that may be made by its manufacturer, is not guaranteed or endorsed by the publisher.
References
Bleckwedel, J., Mohamed, F., Mozzi, F., and Raya, R. R. (2020). Major role of lactate dehydrogenase D-LDH1 for the synthesis of lactic acid in Fructobacillus tropaeoli CRL 2034. Appl. Microbiol. Biotechnol. 104, 7409–7426. doi: 10.1007/s00253-020-10776-9
Corcoran, B. M., Ross, R. P., Fitzgerald, G. F., Dockery, P., and Stanton, C. (2006). Enhanced survival of GroESL-overproducing Lactobacillus paracasei NFBC 338 under stressful conditions induced by drying. Appl. Environ. Microbiol. 72, 5104–5107. doi: 10.1128/AEM.02626-05
Courtin, P., Monnet, V., and Rul, F. (2002). Cell-wall proteinases PrtS and PrtB have a different role in Streptococcus thermophilus/Lactobacillus bulgaricus mixed cultures in milk. Microbiology (Reading). 148, 3413–3421. doi: 10.1099/00221287-148-11-3413
Desai, S. G., Guerinot, M. L., and Lynd, L. R. (2004). Cloning of L-lactate dehydrogenase and elimination of lactic acid production via gene knockout in Thermoanaerobacterium saccharolyticum JW/SL-YS485. Appl. Microbiol. Biotechnol. 65, 600–605. doi: 10.1007/s00253-004-1575-9
Foster, D. W. (2012). Malonyl-CoA: the regulator of fatty acid synthesis and oxidation. J. Clin. Invest. 122, 1958–1959. doi: 10.1172/JCI63967
Gaspar, P., Carvalho, A. L., Vinga, S., Santos, H., and Neves, A. R. (2013). From physiology to systems metabolic engineering for the production of biochemicals by lactic acid bacteria. Biotechnol. Adv. 31, 764–788. doi: 10.1016/j.biotechadv.2013.03.011
Guan, C., Chen, X., Zhao, R., Yuan, Y., Huang, X., Su, J., et al. (2021). A weak post-acidification Lactobacillus helveticus UV mutant with improved textural properties. Food Sci Nutr. 9, 469–479. doi: 10.1002/fsn3.2016
Guijas, C., Montenegro-Burke, J. R., Warth, B., Spilker, M. E., and Siuzdak, G. (2018). Metabolomics activity screening for identifying metabolites that modulate phenotype. Nat. Biotechnol. 36, 316–320. doi: 10.1038/nbt.4101
Jin, Q., Li, L., Moon, J. S., Cho, S. K., Kim, Y. J., Lee, S. J., et al. (2016). Reduction of D-lactate content in sauerkraut using starter cultures of recombinant Leuconostoc mesenteroides expressing the ldhL gene. J. Biosci. Bioeng. 121, 479–483. doi: 10.1016/j.jbiosc.2015.09.007
Jingjing, E., Chen, J., Chen, Z., Ma, R., Zhang, J., Yao, C., et al. (2021). Effects of different initial pH values on freeze-drying resistance of Lactiplantibacillus plantarum LIP-1 based on transcriptomics and proteomics. Food Res. Int. 149, 110694. doi: 10.1016/j.foodres.2021.110694
Johnson, C. H., Ivanisevic, J., and Siuzdak, G. (2016). Metabolomics: beyond biomarkers and towards mechanisms. Nat. Rev. Mol. Cell Biol. 17, 451–459. doi: 10.1038/nrm.2016.25
Kandler, O. (1983). Carbohydrate metabolism in lactic acid bacteria. Antonie Van Leeuwenhoek. 49, 209–224. doi: 10.1007/BF00399499
Lapierre, L., Germond, J. E., Ott, A., Delley, M., and Mollet, B. (1999). D-Lactate dehydrogenase gene (ldhD) inactivation and resulting metabolic effects in the Lactobacillus johnsonii strains La1 and N312. Appl. Environ. Microbiol. 65, 4002–4007. doi: 10.1128/AEM.65.9.4002-4007.1999
Li, S. N., Tang, S. H., Ren, R., Gong, J. X., and Chen, Y. M. (2021). Metabolomic profile of milk fermented with Streptococcus thermophilus cocultured with Bifidobacterium animalis ssp. lactis, Lactiplantibacillus plantarum, or both during storage. J. Dairy Sci. 104, 8493–8505. doi: 10.3168/jds.2021-20270
Mathur, H., Beresford, T. P., and Cotter, P. D. (2020). Health benefits of cactic acid bacteria (LAB) fermentates. Nutrients. 12, 1679. doi: 10.3390/nu12061679
Meng, L., Li, S., Liu, G., Fan, X., Qiao, Y., Zhang, A., et al. (2021). The nutrient requirements of Lactobacillus acidophilus LA-5 and their application to fermented milk. J. Dairy Sci. 104, 138–150. doi: 10.3168/jds.2020-18953
Miao, Z. H., Wang, J. N., Shen, X., Zhou, Q. Q., Luo, Y. T., Liang, H. J., et al. (2022). Long-term use of Lacticaseibacillus paracasei N1115 from early life alleviates high-fat-diet-induced obesity and dysmetabolism in mice. Benef. Microbes. 13, 407–416. doi: 10.3920/BM2021.0171
Moller, C., Bockelmann, W., Ammann, A., and Heller, K. J. (2007). Production of yoghurt with mild taste by a Lactobacillus delbrueckii subsp. bulgaricus mutant with altered proteolytic properties. Biotechnol. J. 2, 469–479. doi: 10.1002/biot.200600225
Morelli, L., Vescovo, M., Cocconcelli, P. S., and Bottazzi, V. (1986). Fast and slow milk-coagulating variants of Lactobacillus helveticus HLM 1. Can. J. Microbiol. 32, 758–760. doi: 10.1139/m86-138
Okano, K., Uematsu, G., Hama, S., Tanaka, T., Noda, H., Kondo, A., et al. (2018). Metabolic engineering of Lactobacillus plantarum for direct l-lactic acid production from raw corn starch. Biotechnol. J. 13:e1700517. doi: 10.1002/biot.201700517
Rico, J., Yebra, M. J., Pérez-Martínez, G., Deutscher, J., and Monedero, V. (2008). Analysis of ldh genes in Lactobacillus casei BL23: role on lactic acid production. J. Ind. Microbiol. Biotechnol. 35, 579–586. doi: 10.1007/s10295-008-0319-8
Shapiro, S. K. (1962). Utilization of S-adenosylmethionine by micro-organisms. J. Bacteriol. 83, 169–174. doi: 10.1128/jb.83.1.169-174.1962
Shiby, V. K., and Mishra, H. N. (2013). Fermented milks and milk products as functional foods–a review. Crit. Rev. Food Sci. Nutr. 53, 482–496. doi: 10.1080/10408398.2010.547398
Shukla, R., Kumar, A., Prasad, C. S., Srivastava, B., and Dubey, N. K. (2008). Antimycotic and antiaflatoxigenic potency of Adenocalymma alliaceum Miers. on fungi causing biodeterioration of food commodities and raw herbal drugs. Int. Biodeterior. Biodegradation 62, 348–351. doi: 10.1016/j.ibiod.2007.11.006
Singhvi, M., Zendo, T., and Sonomoto, K. (2018). Free lactic acid production under acidic conditions by lactic acid bacteria strains: challenges and future prospects. Appl. Microbiol. Biotechnol. 102, 5911–5924. doi: 10.1007/s00253-018-9092-4
Song, X., Huang, H., Xiong, Z., Ai, L., and Yang, S. (2017). CRISPR-Cas9D10A nickase-assisted genome editing in Lactobacillus casei. Appl. Environ. Microbiol. 83, e01259–e01217. doi: 10.1128/AEM.01259-17
Sorensen, K. I., Curic-Bawden,N, M., Junge, M. P., Janzen ohansen, T., and J0, E. (2016). Enhancing the sweetness of yoghurt through metabolic remodeling of carbohydrate metabolism in Streptococcus thermophilus and Lactobacillus delbrueckii subsp. bulgaricus. Appl. Environ. Microbiol. 82, 3683–3692. doi: 10.1128/AEM.00462-16
Sun, J., Chen, H., Qiao, Y., Liu, G., Leng, C., Zhang, Y., et al. (2019). The nutrient requirements of Lactobacillus rhamnosus GG and their application to fermented milk. J. Dairy Sci. 102, 5971–5978. doi: 10.3168/jds.2018-15834
Sun, Y., Peng, C., Wang, J., Guo, S., Sun, Z., and Zhang, H. (2022). Mesopic fermentation contributes more to the formation of important flavor compounds and increased growth of Lactobacillus casei Zhang than does high temperature during milk fermentation and storage. J. Dairy Sci. 105, 4857–4867. doi: 10.3168/jds.2021-20949
Tian, X., Liu, X., Zhang, Y., Chen, Y., Hang, H., Chu, J., et al. (2021). Metabolic engineering coupled with adaptive evolution strategies for the efficient production of high-quality L-lactic acid by Lactobacillus paracasei. Bioresour. Technol. 323, 124549. doi: 10.1016/j.biortech.2020.124549
Tomassini, A., Curone, G., Solè, M., Capuani, G., Sciubba, F., Conta, G., et al. (2019). NMR-based metabolomics to evaluate the milk composition from Friesian and autochthonous cows of Northern Italy at different lactation times. Nat. Prod. Res. 33, 1085–1091. doi: 10.1080/14786419.2018.1462183
Viana, R., Yebra, M. J., Galán, J. L., Monedero, V., and Pérez-Martínez, G. (2005). Pleiotropic effects of lactate dehydrogenase inactivation in Lactobacillus casei. Res. Microbiol. 156, 641–649. doi: 10.1016/j.resmic.2005.02.011
Vieco-Saiz, N., Beleguesmia, Y., Raspoet, R., Auclair, E., Gancel, F., Kempf, I., et al. (2019). Benefits and inputs from lactic acid bacteria and their bacteriocins as alternatives to antibiotic growth promoters during food-animal production. Front. Microbiol. 10, 57. doi: 10.3389/fmicb.2019.00057
Wang, J., Sun, H., Guo, S., Sun, Y., Kwok, L. Y., Zhang, H., et al. (2021a). Comparison of the effects of single probiotic strains Lactobacillus casei Zhang and Bifidobacterium animalis ssp. lactis Probio-M8 and their combination on volatile and nonvolatile metabolomic profiles of yogurt. J. Dairy Sci. 104, 7509–7521. doi: 10.3168/jds.2020-20099
Wang, J., Zhao, W., Guo, S., Sun, Y., and Yao, K., Liu, Z., et al. (2021b). Different growth behaviors and metabolomic profiles in yogurts induced by multistrain probiotics of Lactobacillus casei Zhang and Bifidobacterium lactis V9 under different fermentation temperatures. J. Dairy Sci. 104, 10528–10539. doi: 10.3168/jds.2021-20352
Wang, Y., Wu, J., Lv, M., Shao, Z., Hungwe, M., Wang, J., et al. (2021c). Metabolism characteristics of lactic acid bacteria and the expanding applications in food industry. Front Bioeng Biotechnol. 9, 612285. doi: 10.3389/fbioe.2021.612285
Xu, Z. S., Wang, Z., Cui, X., Liang, Y., Wang, T., and Kong, J. (2021). Peptide transporter-related protein 2 plays an important role in glutathione transport of Streptococcus thermophilus. J. Dairy Sci. 104, 3990–4001. doi: 10.3168/jds.2020-19234
Yamauchi, R., Maguin, E., Horiuchi, H., Hosokawa, M., and Sasaki, Y. (2019). The critical role of urease in yogurt fermentation with various combinations of Streptococcus thermophilus and Lactobacillus delbrueckii ssp. bulgaricus. J. Dairy Sci. 102, 1033–1043. doi: 10.3168/jds.2018-15192
Yonezawa, S., Xiao, J. Z., Odamaki, T., Ishida, T., Miyaji, K., Yamada, A., et al. (2010). Improved growth of bifidobacteria by cocultivation with Lactococcus lactis subspecies lactis. J. Dairy Sci. 93, 1815–1823. doi: 10.3168/jds.2009-2708
Keywords: Lacticaseibacillus paracasei, lactate dehydrogenase, CRISPR, fermented milk, metabolomics
Citation: Fang S, Song X, Cui L, Bai J, Lu H and Wang S (2023) The lactate dehydrogenase gene is involved in the growth and metabolism of Lacticaseibacillus paracasei and the production of fermented milk flavor substances. Front. Microbiol. 14:1195360. doi: 10.3389/fmicb.2023.1195360
Received: 28 March 2023; Accepted: 15 May 2023;
Published: 09 June 2023.
Edited by:
Zhaojie Li, Qingdao Agricultural University, ChinaReviewed by:
Huaxi Yi, Ocean University of China, ChinaWei Zhao, Jiangnan University, China
Fang He, Sichuan University, China
Copyright © 2023 Fang, Song, Cui, Bai, Lu and Wang. This is an open-access article distributed under the terms of the Creative Commons Attribution License (CC BY). The use, distribution or reproduction in other forums is permitted, provided the original author(s) and the copyright owner(s) are credited and that the original publication in this journal is cited, in accordance with accepted academic practice. No use, distribution or reproduction is permitted which does not comply with these terms.
*Correspondence: Shijie Wang, wangshijie@hebust.edu.cn