Erratum: Causal relationship between gut microbiota and immune thrombocytopenia: a Mendelian randomization study of two samples
- 1Department of Hematology, Qilu Hospital (Qingdao), Cheeloo College of Medicine, Shandong University, Shandong, China
- 2The Affiliated Taian City Central Hospital of Qingdao University, Taian, China
- 3Centre of Neuro-Encephalology, Taian City Central Hospital, Qingdao University, Shandong, China
- 4Department of Orthopedics, Qilu Hospital (Qingdao), Cheeloo College of Medicine, Shandong University, Shandong, China
- 5Department of Orthopedics, The Affiliated Taian City Central Hospital of Qingdao University, Taian, China
Background: Some observational studies have shown that immune thrombocytopenia (ITP) is highly associated with the alteration-composition of gut microbiota. However, the causality of gut microbiota on ITP has not yet been determined.
Methods: Based on accessible summary statistics of the genome-wide union, the latent connection between ITP and gut microbiota was estimated using bi-directional Mendelian randomization (MR) and multivariable MR (MVMR) analyses. Inverse variance weighted (IVW), weighted median analyses, and MR-Egger regression methods were performed to examine the causal correlation between ITP and the gut microbiota. Several sensitivity analyses verified the MR results. The strength of causal relationships was evaluated using the MR-Steiger test. MVMR analysis was undertaken to test the independent causal effect. MR analyses of reverse direction were made to exclude the potential of reverse correlations. Finally, GO enrichment analyses were carried out to explore the biological functions.
Results: After FDR adjustment, two microbial taxa were identified to be causally associated with ITP (PFDR < 0.10), namely Alcaligenaceae (PFDR = 7.31 × 10–2) and Methanobacteriaceae (PFDR = 7.31 × 10–2). In addition, eight microbial taxa were considered as potentially causal features under the nominal significance (P < 0.05): Actinobacteria, Lachnospiraceae, Methanobacteria, Bacillales, Methanobacteriales, Coprococcus2, Gordonibacter, and Veillonella. According to the reverse-direction MR study findings, the gut microbiota was not significantly affected by ITP. There was no discernible horizontal pleiotropy or instrument heterogeneity. Finally, GO enrichment analyses showed how the identified microbial taxa participate in ITP through their underlying biological mechanisms.
Conclusion: Several microbial taxa were discovered to be causally linked to ITP in this MR investigation. The findings improve our understanding of the gut microbiome in the risk of ITP.
Background
Immune thrombocytopenia (ITP) is defined as an acquired autoimmune disorder resulting in bleeding symptoms caused by the destruction of megakaryocytes and the decrease of peripheral blood platelets (counts of platelet < 100 × 109/L) (Anat, 2023; Liu et al., 2023). The annual incidence of ITP was about 2–4/100,000 adults (Lambert and Gernsheimer, 2017). Disregarding common hemorrhage events, patients with ITP often feel fatigued in their daily activities and anxious about the burden of monitoring or treatment (Efficace et al., 2016). The pathogenesis of ITP is intricate. A variety of triggering mechanisms have been identified, such as predisposing factors (Audia et al., 2017), viral infection factors (Provan and Semple, 2022), drug-induced factors (Marini et al., 2022), and vaccine-induced factors (Arepally and Ortel, 2021), as well as those without a clear underlying cause (Swinkels et al., 2018).
The human gut microbiome is considered the biggest and most complicated symbiotic ecosystem, with the host playing a pivotal role in maintaining gut homeostasis (Shi et al., 2017). An imbalanced composition in the gut microbiome has been shown to play a role in autoimmune diseases (Clemente et al., 2018; Beam et al., 2021; Tong et al., 2021; Pianko and Golob, 2022). Several studies have found a connection between gut microbiota and ITP. Borody et al. (2011) reported that the first immune-mediated ITP was successfully reversed using fecal microbiota transplantation (FMT) in 2011. Further studies on probiotics may promote the prevention and treatment of ITP. However, results from previously published studies have been inconsistent. For example, Liu C. et al. (2020) discovered that the compositional change of intestinal microbiota occurred in ITP, with a more significant percentage of Proteobacteria and Bacteroidetes and a lower ratio of Firmicutes/Bacteroidetes compared with healthy controls. Yu et al. (2022) obtained results showing that Actinobacteria and the Firmicutes/Bacteroidetes ratio decreased, while Zhang et al. (2020) reported the opposite results about the Firmicutes/Bacteroidetes ratio. Most of the previous studies about ITP were conducted as case-control studies, in which it was difficult to confirm the causal correlation between the exposure and outcome. Moreover, for observational studies, the relationship between ITP and gut microbiome is vulnerable to confounders such as environment, age, sex, dietary habits, and lifestyle (Wang et al., 2021). Furthermore, it is not easy to prevent these confounding factors from affecting the results of observational studies. The above circumstances restrict us from investigating the causality between ITP and the gut microbiome.
Studies using Mendelian randomization (MR) have widely examined the causal relationship between the gut microbiome and disorders such as autoimmune disorders (Xiang et al., 2021; Xu et al., 2022), metabolic disorders (Sanna et al., 2019; Xu et al., 2021), and psychiatric disorders (Ni et al., 2022). Using MR in studies takes advantage of genetic variants serving as the instrumental variable (IV) to determine the assumption that exposure causally affects the outcome. Confounders cannot affect the link between outcome and genetic variants because genotype variation is randomized among children by their parents. Therefore, we can get a reasonable causal inference from studies using MR (Birney, 2022). This study uses the summary statistics from the genome-wide association study (GWAS) and then assesses the causal relationship between ITP and gut microbiota via a two-sample MR design.
Materials and method
Study design
As shown in Figure 1, this study aims to reveal the causal effect of gut microbiota and ITP based on two-sample MR approach (Bowden and Holmes, 2019).
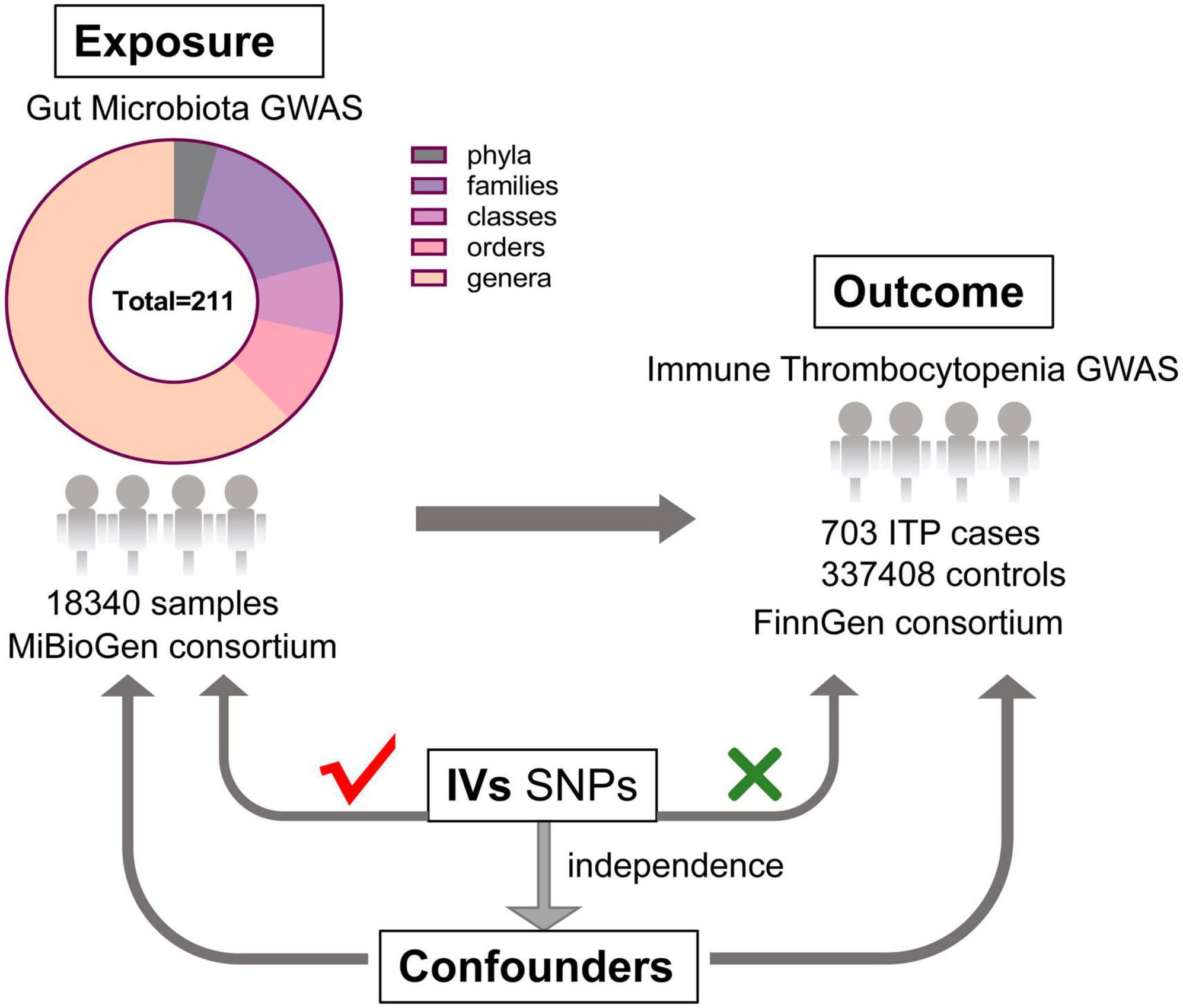
Figure 1. The design of this MR study of the association between gut microbiota and immune thrombocytopenia disease (GWAS, genome-wide association study; IVs, instrumental variables; SNPs, single nucleotide polymorphisms).
Data sources
GWAS comprises 18,340 persons from 24 cohorts, of various ethnicities, a large amount of whom are of European descent, which produced the most extensively published GWAS summary data for gut microbiota (Davey Smith and Hemani, 2014; Kurilshikov et al., 2021). As Kurilshikov et al. (2021) described, after post-sequencing quality control, standardized 16S rRNA processing pipelines were implemented for all participating cohorts, and the taxonomic resolution was truncated to the genus level. In quantitative microbiome signature loci (mbQTL) mapping, adequate sample sizes of at least 3,000 samples and the presence in no less than three studies were used as study-wide cutoffs, while taxa with less than 10% of representatives present in each participating cohort were also discarded.
To ensure no overlap between the sample and the exposure, we sourced the ITP-GWAS summary statistics from the FinnGen consortium’s R8 release (Hartwig et al., 2017). The FinnGen project aims to generate genomic data linked to the national health registry data of 500,000 Finnish individuals enriched for disease endpoints. Demographic information, such as age and gender, of participants in the FinnGen study was provided. We set the first 10 main components during analysis: gender, age, and genotyping batch. For our analysis, ITP-GWAS included 703 ITP cases and 337,408 controls. Table 1 shows the ITP and GWAS summary of ITP and GWAS data.
Instrument selection
Our criteria for selecting each feature’s IVs is as follows: (1) candidate single nucleotide polymorphisms (SNPs) with P < 1.0 × 10–5 statistical significance were selected as being connected with each feature (Hartwig et al., 2017); (2) the linkage disequilibrium (LD) threshold between the SNPs was fixed as r2 < 0.001 (clumping window size = 10,000 kb) based on the reference panel data from 1,000 Genomes Project European samples (phase 3), to retain the independent SNPs with the lowest P-values (Bowden et al., 2015); (3) SNPs with ambiguous alleles (for example, A/C vs. A/G) between the exposure and outcome GWAS were excluded; (4) a sensitivity analysis was conducted to prevent distortion from allele coding or strand orientation, where palindromic SNPs (for example., with G/C or A/T alleles) were taken forward to ruled out.
Weak instrument bias could lead to misleading estimates of causal effects. Thus, the F-statistic was obtained to evaluate the intensity of IVs via the formula , where the ratio of variance in the phenotype shown by the number of instruments (K), sample size (N), and genetic variants (R2) was needed (Bowden et al., 2016). If the corresponding F-statistic was larger than 10, this indicated sufficient strength to ensure the validity of IVs. R2 was worked out via the formula “R2 = 2 × MAF × (1–MAF) × beta2,” where “beta” denotes the genetically estimated impact on the exposure, and means denotes the frequency of the minor allele (Verbanck et al., 2018).
Statistical analysis
We conducted several analyses based on the two-sample MR framework to investigate the potential causal correlation between ITP and gut microbiome, including inverse variance weighted (IVW), weighted median analysis, and MR-Egger regression method. The IVW approach was used as the primary analysis, assuming that all SNPs were valid but vulnerable to horizontal pleiotropy (Abecasis et al., 2010). A weighted median analysis can allow consistent evaluations if up to 50% of the instrumental variables are invalid (Lin et al., 2022). The MR-Egger regression model is similar to the IVW model, except for its additional intercept term for estimating the presence of pleiotropy (Burgess et al., 2013). Additionally, Mendelian Randomization Pleiotropy Residual Sum and Outlier (MR-PRESSO) were taken to identify and adjust for the influence of outliers in the data (Bowden et al., 2015). We also performed a leave-one-out analysis, where each SNP was taken off one at a time while calculating Cochran’s Q-statistic to determine the heterogeneity of IVs to confirm all results were not skewed by a single SNP (Bowden et al., 2016).
If the results of all MR analyses were significant at a statistics level (P < 0.05), we speculated that the gut microbiome may be linked to the risk of ITP. The directional causality of the gut microbiome on ITP was evaluated using the MR-Steiger test (Hemani et al., 2017), and MR analysis in the reverse direction was performed. The procedures and settings used were in accordance with those of the forward MR study. If the subsequent four conditions were satisfied, we held the belief that a substantial causal link between gut microbiome and ITP risk existed: (1) a substantial disparity was evident using the IVW method (P < 0.05); (2) the outcome estimations of the IVW, weighted median, and MR-Egger methodologies demonstrated congruence; (3) both the MR-Egger intercept test and the MR-PRESSO global test yielded non-significant results (P > 0.05); and (4) the MR-Steiger directionality tests indicated TRUE (P < 0.05). Multivariable MR (MVMR) analysis was performed to estimate the independent causal relationship between gut microbiota and ITP conditioning on the effects of other exposures. IVW was also the primary analysis method. Moreover, we conducted GO enrichment analysis based on lead SNPs for all identified gut microbial taxa to further study the biological function of gut microbial taxa on the risk of ITP.
Two Sample MR software (version 0.5.6) was used for all analyses. MR-PRESSO (version 1.0) R package was done by the R version 4.2.1 software package. GO enrichment analysis was carried out by the “FUMA” website tool (Watanabe et al., 2017). In order to exclude any potential false positive signal, the false discovery rate (FDR) method was used to adjust for the number of exposures tested under each attribute. PFDR < 0.1 was set as the significant threshold. Microbial taxa and ITP were considered to have a potential association when P < 0.05 but PFDR ≥ 0.1.
Results
MR results
A total of 2,248 SNPs were employed as IVs for 211 bacterial taxa in accordance with the selection criteria for IVs. The bias of weak IVs was eliminated by the F-statistics of the IVs, which ranged from 12.91 to 187.70. Supplementary Table 1 provides additional information regarding the chosen instrumental factors.
Based on IVW methods, a total of 10 microbial taxa were identified to be associated with ITP, where one belongs to phyla, three belong to families, one belongs to classes, two belong to orders, and three belong to genera (Figures 2, 3 and Supplementary Table 2). After FDR correction (Supplementary Table 13), two significant taxa were identified. Alcaligenaceae (OR = 2.40; 95% CI, 1.28–4.50; P = 6.56 × 10–3, PFDR = 7.31 × 10–2) had a risk effect on ITP, and Methanobacteriaceae (OR = 0.63; 95% CI, 0.45–0.88; P = 6.85 × 10–3, PFDR = 7.31 × 10–2) is negatively associated with ITP risk. In addition, eight microbial taxa were considered as potentially causal features under the nominal significance (P < 0.05). Specifically, Gordonibacter (OR = 1.35; 95% CI, 1.01–1.81; P = 4.38 × 10–2, PFDR = 9.07 × 10–1) and Veillonella (OR = 2.04; 95% CI, 1.03–4.05; P = 4.04 × 10–2, PFDR = 9.07 × 10–1) had a risk effect on ITP, and Methanobacteria (OR = 0.63; 95% CI, 0.45–0.88; P = 6.85 × 10–3, PFDR = 1.10 × 10–1), Methanobacteriales (OR = 0.63; 95% CI, 0.45–088; P = 6.85 × 10–3, PFDR = 1.02 × 10–1), Lachnospiraceae (OR = 0.54; 95% CI, 0.31–0.97; P = 3.80 × 10–2, PFDR = 3.40 × 10–1), Coprococcus2 (OR = 0.52; 95% CI, 0.28–0.96; P = 3.74 × 10–2, PFDR = 9.07 × 10–1), Bacillales (OR = 0.66; 95% CI, 0.48–0.91; P = 1.01 × 10–2, PFDR = 1.02 × 10–1), and Actinobacteria (OR = 0.52; 95% CI, 0.28–0.97; P = 3.89 × 10–2, PFDR = 3.50 × 10–1) had a protective effect on ITP.
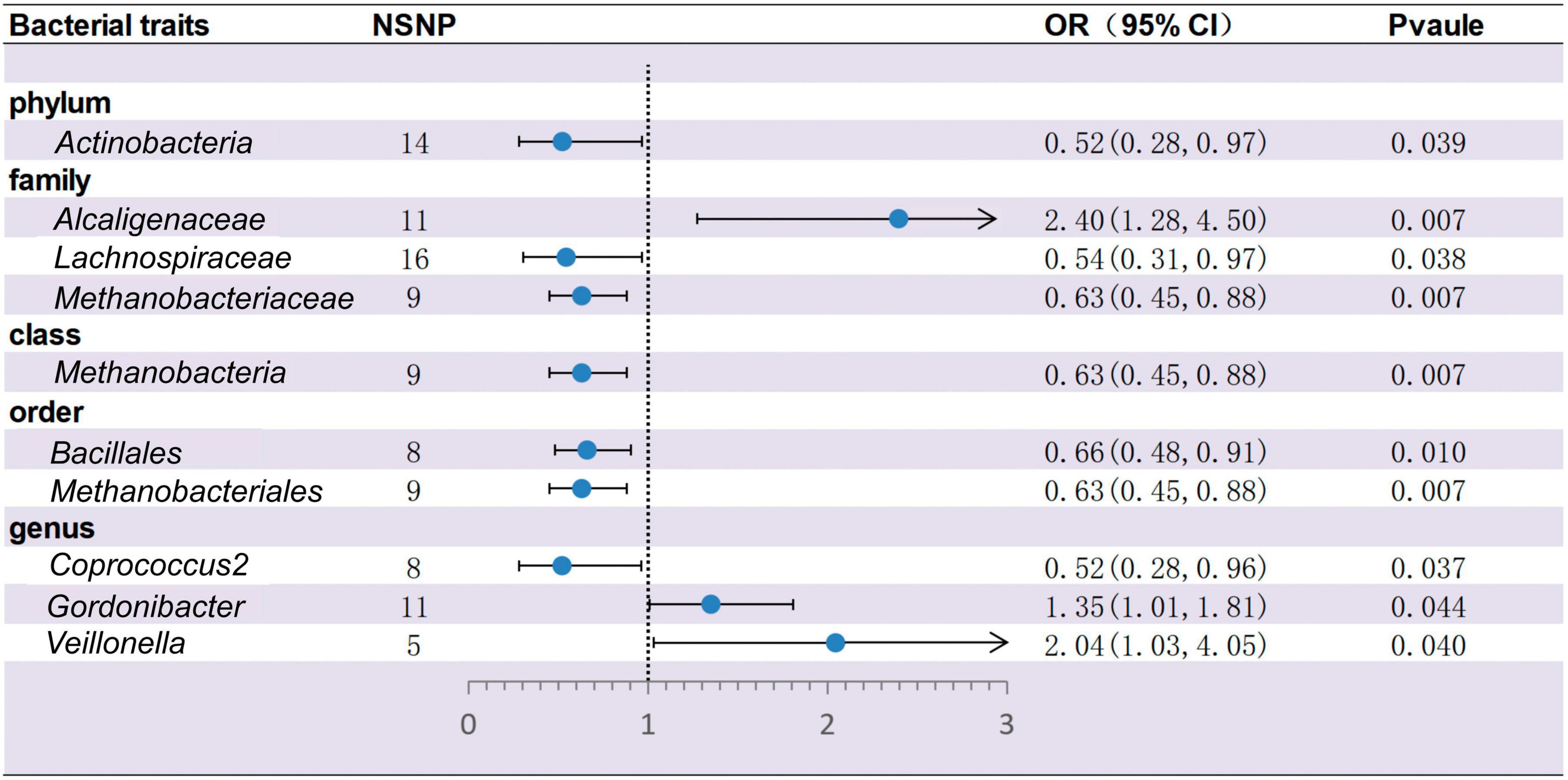
Figure 2. Forest plots for the causal association between gut microbiota and ITP on the IVW method (IVW, inverse variance-weighted; NSNP, numbers of single nucleotide polymorphism; OR, odds ratio; 95% CI, 95% confidence interval).
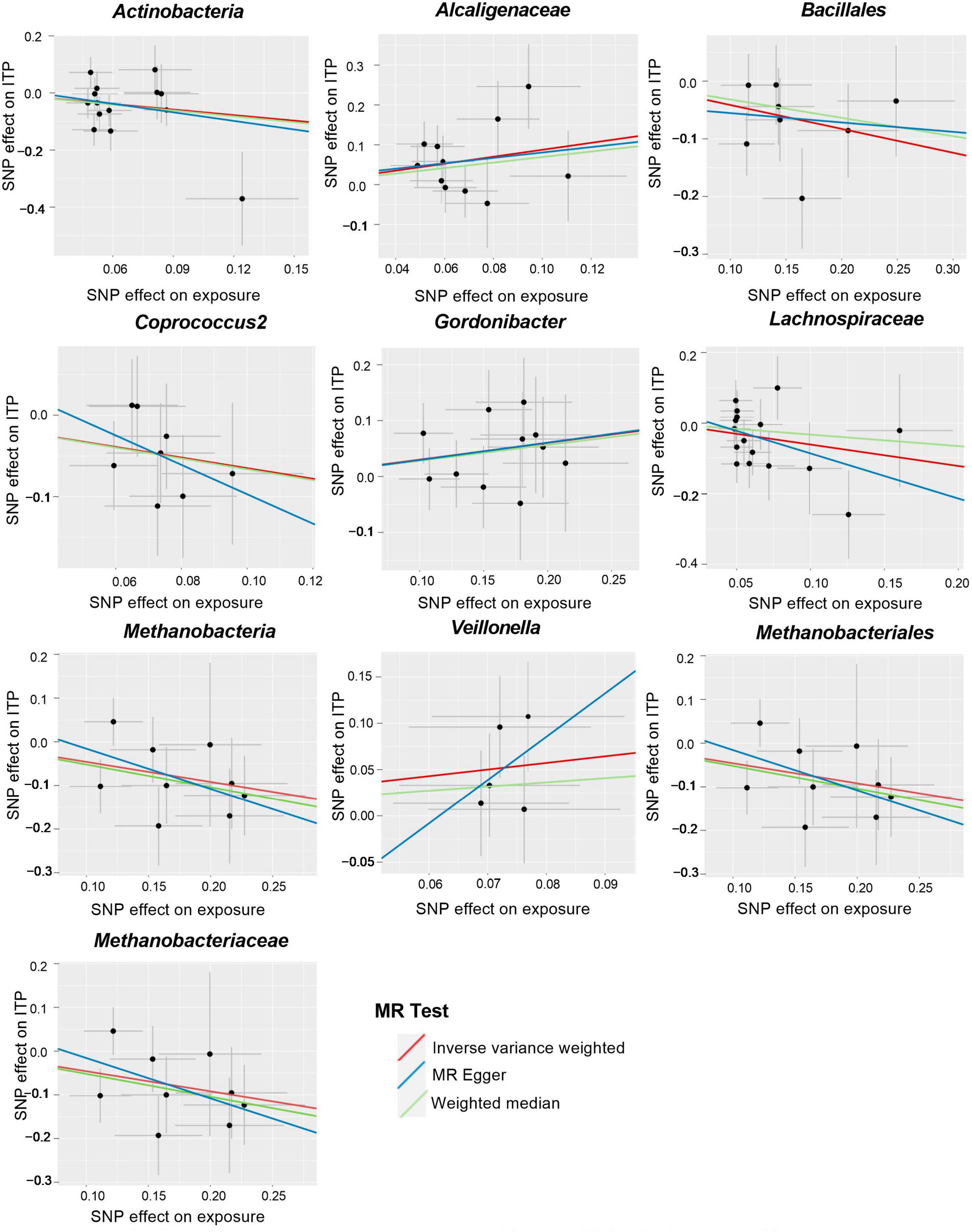
Figure 3. Scatter plots for the causal association between gut microbiota and ITP (SNP, single nucleotide polymorphism; ITP, immune thrombocytopenia).
Cochran’s IVW Q test results showed no evidence of significant heterogeneity in these IVs (Supplementary Table 3). The results of the intercept analysis of the MR-Egger regression likewise suggested that there was no substantial horizontal pleiotropy in either direction (Supplementary Table 4). Further testing of the MR-Egger regression’s accuracy using MR-PRESSO revealed no horizontal pleiotropy (Supplementary Table 5). Also, the leave-one-out results offered additional proof of the data’s reliability (Figure 4). The IVW results were accurate when pleiotropy and heterogeneity were absent. The results of the MR-Steiger directionality tests were TRUE (Supplementary Table 11). Thereby, these bacteria were causally related to ITP.
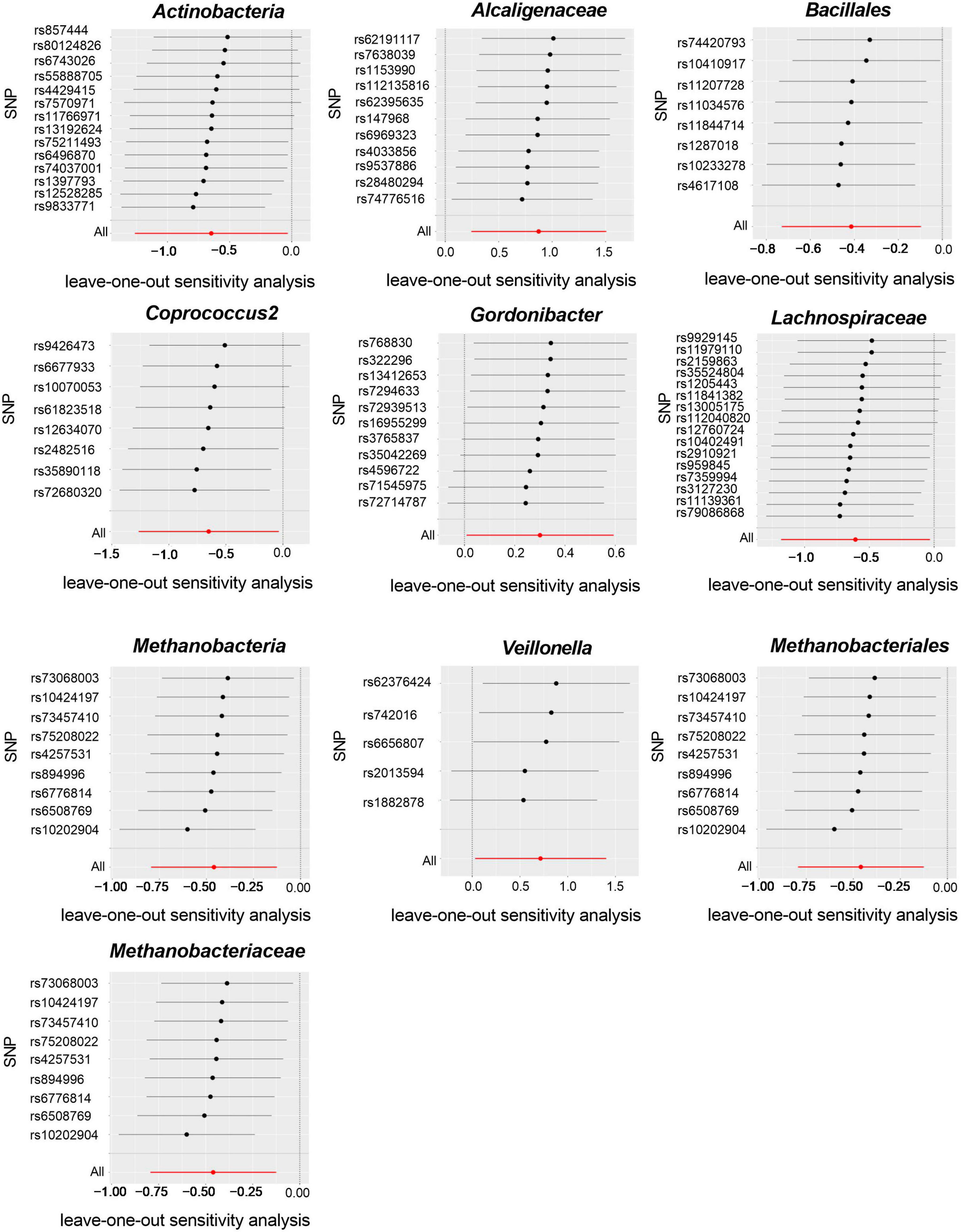
Figure 4. Leave-one-out plots for the causal association between gut microbiota and ITP (SNP, single nucleotide polymorphism).
To eliminate the error in estimating causal effects caused by the potential correlations between exposures, MVMR was performed based on the findings from the univariate analysis at genus, family, and order levels. At the genus level, three independent causal associations were found, including family Coprococcus2 (OR = 0.55; 95% CI, 0.34–0.89; PIVW = 1.40 × 10–2), Gordonibacter (OR = 1.36; 95% CI, 1.10–1.67; PIVW = 4.0 × 10–3), and Veillonella (OR = 1.71; 95% CI, 1.05–2.78; PIVW = 3.0 × 10–2). At the family level, we found the independent causal effect in Alcaligenaceae (OR = 2.37; 95% CI, 1.20–4.67; PIVW = 1.3 × 10–2). However, the results for suggestive microbial taxa turned out to be insignificant after adjustment, including Lachnospiraceae (OR = 0.59; 95% CI, 0.32–1.12; PIVW = 1.06 × 10–1) and Methanobacteriaceae (OR = 0.73; 95% CI, 0.53–1.02; PIVW = 6.7 × 10–2). At the order level, the MVMR confirmed the results for Bacillales (OR = 0.65; 95% CI, 0.48–0.89; PIVW = 8.0 × 10–3), while the results for Methanobacteriales (OR = 0.69; 95% CI, 0.48–1.00; PIVW = 5.1 × 10–2) were not significant. All the results above indicated a potential pleiotropy for gut microbial taxa with ITP at the family and order levels, respectively (Supplementary Table 14).
Reverse-direction MR analyses
ITP had no significant causal relationship with the other gut microbes (Supplementary Tables 6, 7). Cochran’s IVW Q test revealed no substantial heterogeneity in ITP IVs (Supplementary Table 8). There was no discernible horizontal pleiotropy in the MR-Egger regression intercepted item analysis (Supplementary Table 9) or the MR-PRESSO analysis (Supplementary Table 10).
FUMA analysis and GO enrichment analysis
We conducted a functional mapping and annotation (FUMA) analysis to functionally map and annotate the genetic associations to understand better the underlying molecular mechanism between the 10 bacterial genera and ITP risk (Lozupone et al., 2012). Lead SNPs for which P < 5 × 10–6 and r2 < 0.1 were identified from the 10 GWAS results (Supplementary Table 12). After SNP-to-genes mapping and annotating the mapped genes in biological contexts, three GO biological processes (“sodium-independent organic anion transport,” “defense response to bacteria,” and “bile acid and bile salt transport”) were observed that could affect ITP (Figure 5).
Discussion
To the best of our knowledge, this is the first investigation of the causal link between gut microbiome and ITP using publicly accessible genetic datasets. In our study, we utilized the summary statistics of the gut microbiome from the MiBioGen consortium GWAS meta-analysis and the summary statistics of ITP from the FinnGen consortium (R8 released data) to conduct a two-sample MR analysis to find the possible impact of gut microbiota on the risk of ITP. Our MR analysis identified 10 gut microbial taxa as having potential effects on the risk of ITP. The family Alcaligenaceae, genus Gordonibacter, and genus Veillonella could lead to a higher risk of ITP. In contrast, the phylum Actinobacteria, order Bacillales, genus Coprococcus2, family Methanobacteriaceae, class Methanobacteria, family Lachnospiraceae, and order Methanobacteriales were linked to a decrease in ITP risk. MVMR indicated a potential pleiotropy at the family and order levels, respectively.
The human intestinal mucosa houses a diverse microbiota population, including over 1,000 species that serve vital roles in maintaining good health (Tremaroli and Bäckhed, 2012; Yu et al., 2021). The gut microbiota primarily comprises Actinobacteria, Bacteroidetes, Cyanobacteria, Proteobacteria, Firmicutes, Fusobacteria, and Verrucomicrobia (Lozupone et al., 2012; Adak and Khan, 2019). It is worth noting that an imbalance in gut microbiota can lead to various diseases related to immunology, psychology, and metabolism (Wang and Zhao, 2018; Góralczyk-Bińkowska et al., 2022; Miyauchi et al., 2023). Dysbiosis of the gut microbiome can significantly affect the development of autoimmune conditions such as type 1 diabetes (T1DM) (Murri et al., 2013), Crohn’s disease (CD) (Caparrós et al., 2021), multiple sclerosis (MS) (Pröbstel et al., 2020), inflammatory bowel diseases (IBD) (Parada Venegas et al., 2019), autoimmune hepatitis disease (AHD) (Cheng et al., 2022), asthma (Arrieta et al., 2015), allergies (Liu X. et al., 2020), and psoriasis (Tan et al., 2018). Recent studies have suggested that Veillonella and Gordonibacter may promote inflammation, while Lachnospiraceae, Actinobacteria, and Methanobrevibacter may have protective effects against autoimmune conditions. The abundance of Veillonella increased in AHD (Cheng et al., 2022) and CD patients (Caparrós et al., 2021) compared to healthy controls. Meanwhile, Gordonibacter was found to stimulate the release of pro-inflammatory cytokines and damage the epithelial barrier in intestinal epithelium-specific Fut2 deficiency mice (Tang et al., 2021). Conversely, Lachnospiraceae was noted to exert anti-inflammatory effects in intestinal epithelial cells and immune cells by producing short-chain fatty acids (SCFAs) (Parada Venegas et al., 2019). Actinobacteria, specifically Bifidobacteria species, can regulate the immune system’s inflammatory and autoimmune responses by activating regulatory Treg cells (Binda et al., 2018). Methanobrevibacter, the predominant anaerobic archaeon enriched among MS gut microbiota, was found to participate in the immunomodulatory process and recruit inflammatory cells (Bang et al., 2014; Zoledziewska, 2019). These studies corroborate the findings of our MR study. However, further research is necessary to understand the specific mechanisms of gut microbiota in autoimmune disease development.
In recent years, advancements in macrogenomics and sequencing technologies have enabled researchers to explore variations in the gut microbiota between ITP patients and healthy individuals (Liu C. et al., 2020; Zhang et al., 2020; Yu et al., 2022). Through our MR study, we found three gut microbiota taxa, Bacillales, Coprococcus2, and Lachnospiraceae, belonging to the phylum Firmicutes, consistent with other studies’ findings (Liu C. et al., 2020; Yu et al., 2022). However, at the phylum level, we did not find any causal connections between Firmicutes and ITP risk. Another study explored the differences in gut microbiota between children with ITP and healthy individuals, and found a decrease in the proportion of Actinobacteria in the phylum level, which aligns with our findings (Li et al., 2023). There are several reasons to explain the different results, including the vulnerability of gut microbiota composition to age, dietary habits, geographical environment, and other factors (Milani et al., 2017). Moreover, the microbiome contains a large variety of taxa, and multiple taxa interacting with each other may ultimately override the role of the phylum. Additionally, although compositional variations of the gut microbiome in ITP patients were observed in previous studies, they could not ensure a causal connection between ITP and gut microbiota. In contrast, our two-sample MR study eliminated the reverse causality for the ITP effects on gut microbiota, providing a more robust understanding of the relationship between the ITP and gut microbiota.
GO enrichment analysis highlighted three biological processes that may be linked to the relationship between the gut microbiota and ITP (“defense response to bacteria,” “bile acid and bile salt transport,” and “sodium-independent organic anion transport.”). Gut microbiome dysbiosis has been linked to various immune-related diseases, but its role in initiating ITP is not yet known. When an imbalance of intestinal flora occurs due to invasive infections, it may activate T cells and B cells to secrete multiple inflammatory factors, which could have a protective or harmful effect on the human body (Lozupone et al., 2012). For instance, chronic exposure to certain infections such as Helicobacter pylori, VZV (Varicella-Zoster virus), CMV (cytomegalovirus), and hepatitis C increases the risk of secondary ITP (LeVine and Brooks, 2019). Studies have shown that treating H. pylori infection effectively increases platelet counts in ITP patients, which supports the “defense response to bacteria” finding in our GO enrichment study (Vishnu et al., 2021; Dogan et al., 2022; Takeuchi and Okamoto, 2022). Moreover, the target taxa identified in this study, such as Actinobacteria, Methanobrevibacter, and Lachnospiraceae, activate T cells to promote inflammatory responses, further confirming the “defense response” of gut microbiota in ITP risk (Arrieta et al., 2015; Binda et al., 2018; Tan et al., 2018; Parada Venegas et al., 2019; Zoledziewska, 2019; Liu X. et al., 2020; Tang et al., 2021). Bile acids (BA) produced in the liver and transformed in the intestine are closely associated with the gut microbiota’s bioconversion (Thomas et al., 2008; Malhotra et al., 2019; Vishnu et al., 2021; Dogan et al., 2022). Several studies have shown that BA signaling through farnesoid X receptor (FXR) and TGR5 binding can attenuate pro-inflammatory innate immune responses such as MS (Martín et al., 2010). Additionally, BA-dependent FXR can induce the transcription of various genes involved in intestinal mucosal defense against microbes to control bacterial overgrowth and maintain mucosal integrity in the intestine under physiological conditions (Rizzetto et al., 2018). These findings suggest that ITP may involve “bile acid and bile salt transport” of the gut microbiota. However, the influence of “sodium-independent organic anion transport” in immune-related disorders, especially ITP, has been overlooked by researchers.
There are some advantages to this study. The MR design was used to speculate the causal correlation between ITP and gut microbiota, excluding the deviation of confounders and the inference of reverse causality. With the MiBioGen consortium, the most significant GWAS meta-analysis was applied to gather genetic variants related to the gut microbiota, assuring the correctness of the tools. Horizontal pleiotropy was discovered and eliminated using MR-Egger regression and MR-PRESSO. A two-sample MR study can effectively avoid bias in comparison to observational studies.
Despite its benefits, the MR analysis conducted has a few limitations. Firstly, the analysis only explored the bacterial taxa at the genus level, preventing us from establishing a causal correlation between ITP and gut microbiota at the species level. Secondly, the MR analysis did not allow for subgroup analyses, such as distinguishing between primary (isolate) ITP and secondary ITP, as only summary data was used instead of raw data. Lastly, since the GWAS meta-analysis of gut microbiome data only included individuals of European origin, our MR investigation was unable to assess the non-European population.
Conclusion
In brief, this two-sample MR study established a causal link between gut microbiota components and ITP. Future clinical and animal studies research is required to fully understand the potential mechanism between gut microbiota and ITP.
Data availability statement
The datasets presented in this study can be found in online repositories. The names of the repository/repositories and accession number(s) can be found in this article/Supplementary material.
Author contributions
DG and GW: study design. CL and DG: data collection and data analysis. CL and QC: data interpretation and drafting the manuscript. All authors participated in and define the final version of this manuscript, responsible for the integrity of data analysis, and approved the submitted version.
Acknowledgments
We would like to thank all investigators and participants in this present study. We appreciate the general summary data provided by the MiBioGen consortium and the FinnGen consortium.
Conflict of interest
The authors declare that the research was conducted in the absence of any commercial or financial relationships that could be construed as a potential conflict of interest.
Publisher’s note
All claims expressed in this article are solely those of the authors and do not necessarily represent those of their affiliated organizations, or those of the publisher, the editors and the reviewers. Any product that may be evaluated in this article, or claim that may be made by its manufacturer, is not guaranteed or endorsed by the publisher.
Supplementary material
The Supplementary Material for this article can be found online at: https://www.frontiersin.org/articles/10.3389/fmicb.2023.1190866/full#supplementary-material
References
Abecasis, G. R., Altshuler, D., Auton, A., Brooks, L. D., Durbin, R. M., Gibbs, R. A., et al. (2010). A map of human genome variation from population-scale sequencing. Nature 467, 1061–1073. doi: 10.1038/nature09534
Adak, A., and Khan, M. R. (2019). An insight into gut microbiota and its functionalities. Cell Mol. Life Sci. 76, 473–493. doi: 10.1007/s00018-018-2943-4
Anat, G. G. (2023). Current approaches for the diagnosis and management of immune thrombocytopenia. Eur. J. Intern. Med. 108, 18–24. doi: 10.1016/j.ejim.2022.11.022
Arepally, G., and Ortel, T. (2021). Vaccine-induced immune thrombotic thrombocytopenia: what we know and do not know. Blood 138, 293–298. doi: 10.1182/blood.2021012152
Arrieta, M. C., Stiemsma, L. T., Dimitriu, P. A., Thorson, L., Russell, S., Yurist-Doutsch, S., et al. (2015). CHILD Study Investigators: Early infancy microbial and metabolic alterations affect risk of childhood asthma. Sci. Transl. Med. 7:307ra152. doi: 10.1126/scitranslmed.aab2271
Audia, S., Mahévas, M., Samson, M., Godeau, B., and Bonnotte, B. (2017). Pathogenesis of immune thrombocytopenia. Autoimmun. Rev. 16, 620–632. doi: 10.1016/j.autrev.2017.04.012
Bang, C., Weidenbach, K., Gutsmann, T., Heine, H., and Schmitz, R. (2014). The intestinal archaea Methanosphaera stadtmanae and Methanobrevibacter smithii activate human dendritic cells. PLoS One 9:e99411. doi: 10.1371/journal.pone.0099411
Beam, A., Clinger, E., and Hao, L. (2021). Effect of diet and dietary components on the composition of the gut microbiota. Nutrients 13:2795. doi: 10.3390/nu13082795
Binda, C., Lopetuso, L., Rizzatti, G., Gibiino, G., Cennamo, V., and Gasbarrini, A. (2018). Actinobacteria: A relevant minority for the maintenance of gut homeostasis. Dig. Liver Dis. 50, 421–428. doi: 10.1016/j.dld.2018.02.012
Birney, E. (2022). Mendelian Randomization. Cold Spring Harb. Perspect. Med. 12:a041302. doi: 10.1101/cshperspect.a041302
Borody, T., Campbell, J., and Torers, M. (2011). Reversal of idiopathic thrombocytopenic 7 purpura with fecal microbiota transplantatino (FMT). Am. J. Gastroenterol. 106:941.
Bowden, J., Davey Smith, G., and Burgess, S. (2015). Mendelian randomization with invalid instruments: effect estimation and bias detection through Egger regression. Int. J. Epidemiol. 44, 512–525. doi: 10.1093/ije/dyv080
Bowden, J., Davey Smith, G., Haycock, P. C., and Burgess, S. (2016). Consistent Estimation in Mendelian Randomization with Some Invalid Instruments Using a Weighted Median Estimator. Genet. Epidemiol. 40, 304–314. doi: 10.1002/gepi.21965
Bowden, J., and Holmes, M. V. (2019). Meta-analysis and Mendelian randomization: A review. Res. Synth Methods. 10, 486–496. doi: 10.1002/jrsm.1346
Burgess, S., Butterworth, A., and Thompson, S. G. (2013). Mendelian randomization analysis with multiple genetic variants using summarized data. Genet. Epidemiol. 37, 658–665. doi: 10.1002/gepi.21758
Caparrós, E., Wiest, R., Scharl, M., Rogler, G., Gutiérrez Casbas, A., Yilmaz, B., et al. (2021). Dysbiotic microbiota interactions in Crohn’s disease. Gut Microbes 13:1949096. doi: 10.1080/19490976.2021.1949096
Cheng, Z., Yang, L., and Chu, H. (2022). The Gut Microbiota: A Novel Player in Autoimmune Hepatitis. Front. Cell Infect. Microbiol. 12:947382. doi: 10.3389/fcimb.2022.947382
Clemente, J. C., Manasson, J., and Scher, J. U. (2018). The role of the gut microbiome in systemic inflammatory disease. BMJ 360:j5145. doi: 10.1136/bmj.j5145
Davey Smith, G., and Hemani, G. (2014). Mendelian randomization: genetic anchors for causal inference in epidemiological studies. Hum. Mol. Genet. 23, R89–R98. doi: 10.1093/hmg/ddu328
Dogan, A., Ekinci, O., and Ebinc, S. (2022). Effect of Helicobacter pylori infection on the first-line treatment outcomes in patients with immune thrombocytopenic purpura. Eur. Rev. Med. Pharmacol. Sci. 26, 3995–4000. doi: 10.26355/eurrev_202206_28970
Efficace, F., Mandelli, F., Fazi, P., Santoro, C., Gaidano, G., Cottone, F., et al. (2016). Health-related quality of life and burden of fatigue in patients with primary immune thrombocytopenia by phase of disease. Am. J. Hematol. 91, 995–1001. doi: 10.1002/ajh.24463
Góralczyk-Bińkowska, A., Szmajda-Krygier, D., and Kozłowska, E. (2022). The Microbiota-Gut-Brain Axis in Psychiatric Disorders. Int. J. Mol. Sci. 23, 11245. doi: 10.3390/ijms231911245
Hartwig, F. P., Davey Smith, G., and Bowden, J. (2017). Robust inference in summary data Mendelian randomization via the zero modal pleiotropy assumption. Int. J. Epidemiol. 46, 1985–1998. doi: 10.1093/ije/dyx102
Hemani, G., Tilling, K., and Davey, S. G. (2017). Orienting the causal relationship between imprecisely measured traits using GWAS summary data. PLoS Genet. 13:e1007081. doi: 10.1371/journal.pgen.1007081
Kurilshikov, A., Medina-Gomez, C., Bacigalupe, R., Radjabzadeh, D., Wang, J., Demirkan, A., et al. (2021). Large-scale association analyses identify host factors influencing human gut microbiome composition. Nat. Genet. 53, 156–165. doi: 10.1038/s41588-020-00763-1
Lambert, M. P., and Gernsheimer, T. B. (2017). Clinical updates in adult immune thrombocytopenia. Blood 129, 2829–2835. doi: 10.1182/blood-2017-03-754119
LeVine, D. N., and Brooks, M. B. (2019). Immune thrombocytopenia (ITP): Pathophysiology update and diagnostic dilemmas. Vet. Clin. Pathol. 48, 17–28. doi: 10.1111/vcp.12774
Li, X., Zhang, M., He, L., Zhou, J., Shen, P., Dai, W., et al. (2023). Gut microbiota alterations in children and their relationship with primary immune thrombocytopenia. Front. Pediatr. 11:1213607. doi: 10.3389/fped.2023.1213607
Lin, L., Lin, J., Qiu, J., Wei, F., Bai, X., Ma, W., et al. (2022). Gut microbiota alterations may increase the risk of prescription opioid use, but not vice versa: A two-sample bi-directional Mendelian randomization study. Front. Microbiol. 13:994170. doi: 10.3389/fmicb.2022.994170
Liu, C., Cheng, L., Ji, L., Li, F., Zhan, Y., Wu, B., et al. (2020). Intestinal microbiota dysbiosis play a role in pathogenesis of patients with primary immune thrombocytopenia. Thromb. Res. 190, 11–19. doi: 10.1016/j.thromres.2020.03.012
Liu, X., Tao, J., Li, J., Cao, X., Li, Y., Gao, X., et al. (2020). Dysbiosis of fecal microbiota in allergic rhinitis patients. Am. J. Rhinol. Allergy 34, 650–660. doi: 10.1177/1945892420920477
Liu, X. G., Hou, Y., and Hou, M. (2023). How we treat primary immune thrombocytopenia in adults. J. Hematol. Oncol. 16, 4. doi: 10.1186/s13045-023-01401-z
Lozupone, C. A., Stombaugh, J. I., Gordon, J. I., Jansson, J. K., and Knight, R. (2012). Diversity, stability and resilience of the human gut microbiota. Nature 489, 220–230. doi: 10.1038/nature11550
Malhotra, A. P., Dudeja, P. K., Gill, R. K., and Alrefai, W. A. (2019). Intestinal absorption of bile acids in health and disease. Compr. Physiol. 10, 21–56. doi: 10.1002/cphy.c190007
Marini, I., Uzun, G., Jamal, K., and Bakchoul, T. (2022). Treatment of drug-induced immune thrombocytopenias. Haematologica 107, 1264–1277. doi: 10.3324/haematol.2021.279484
Martín, R., Carvalho-Tavares, J., Hernández, M., Arnés, M., Ruiz-Gutiérrez, V., and Nieto, M. (2010). Beneficial actions of oleanolic acid in an experimental model of multiple sclerosis: a potential therapeutic role. Biochem. Pharmacol. 79, 198–208. doi: 10.1016/j.bcp.2009.08.002
Milani, C., Duranti, S., Bottacini, F., Casey, E., Turroni, F., Mahony, J., et al. (2017). The first microbial colonizers of the human gut: composition, activities, and health implications of the infant gut microbiota. Microbiol. Mol. Biol. Rev. 81, e36–e17. doi: 10.1128/MMBR.00036-17
Miyauchi, E., Shimokawa, C., Steimle, A., Desai, M. S., and Ohno, H. (2023). The impact of the gut microbiome on extra-intestinal autoimmune diseases. Nat. Rev. Immunol. 23, 9–23. doi: 10.1038/s41577-022-00727-y
Murri, M., Leiva, I., Gomez-Zumaquero, J., Tinahones, F., Cardona, F., Soriguer, F., et al. (2013). Gut microbiota in children with type 1 diabetes differs from that in healthy children: a case-control study. BMC Med. 11:46. doi: 10.1186/1741-7015-11-46
Ni, J. J., Xu, Q., Yan, S. S., Han, B. X., Zhang, H., Wei, X. T., et al. (2022). Gut microbiota and psychiatric disorders: a two-sample Mendelian randomization study. Front. Microbiol. 12:737197. doi: 10.3389/fmicb.2021.737197
Parada Venegas, D., De la Fuente, M. K., Landskron, G., González, M. J., Quera, R., and Dijkstra, G. (2019). Short chain fatty acids (SCFAs)-mediated gut epithelial and immune regulation and its relevance for inflammatory bowel diseases. Front. Immunol. 10:277. doi: 10.3389/fimmu.2019.00277
Pianko, M. J., and Golob, J. L. (2022). Host-microbe interactions and outcomes in multiple myeloma and hematopoietic stem cell transplantation. Cancer Metastasis Rev. 41, 367–382. doi: 10.1007/s10555-022-10033-7
Pröbstel, A. K., Zhou, X., Baumann, R., Wischnewski, S., Kutza, M., Rojas, O., et al. (2020). Gut microbiota-specific IgA+ B cells traffic to the CNS in active multiple sclerosis. Sci. Immunol. 5:eabc7191. doi: 10.1126/sciimmunol.abc7191
Provan, D., and Semple, J. W. (2022). Recent advances in the mechanisms and treatment of immune thrombocytopenia. EBioMedicine 76:103820. doi: 10.1016/j.ebiom.2022.103820
Rizzetto, L., Fava, F., Tuohy, K. M., and Selmi, C. (2018). Connecting the immune system, systemic chronic inflammation and the gut microbiome: The role of sex. J. Autoimmun. 92, 12–34. doi: 10.1016/j.jaut.2018.05.008
Sanna, S., van Zuydam, N. R., Mahajan, A., Kurilshikov, A., Vich Vila, A., Võsa, U., et al. (2019). Causal relationships among the gut microbiome, short-chain fatty acids and metabolic diseases. Nat. Genet. 51, 600–605. doi: 10.1038/s41588-019-0350-x
Shi, N., Li, N., Duan, X., and Niu, H. (2017). Interaction between the gut microbiome and mucosal immune system. Mil. Med. Res. 4:14. doi: 10.1186/s40779-017-0122-9
Swinkels, M., Rijkers, M., Voorberg, J., Vidarsson, G., Leebeek, F. W., and Jansen, A. J. (2018). Emerging concepts in immune thrombocytopenia. Front. Immunol. 9:880. doi: 10.3389/fimmu.2018.00880
Takeuchi, H., and Okamoto, A. (2022). Helicobacter pylori Infection and Chronic Immune Thrombocytopenia. J. Clin. Med. 11:4822. doi: 10.3390/jcm11164822
Tan, L., Zhao, S., Zhu, W., Wu, L., Li, J., Shen, M., et al. (2018). The Akkermansia muciniphila is a gut microbiota signature in psoriasis. Exp. Dermatol. 27, 144–149. doi: 10.1111/exd.13463
Tang, X., Wang, W., Hong, G., Duan, C., Zhu, S., Tian, Y., et al. (2021). Gut microbiota-mediated lysophosphatidylcholine generation promotes colitis in intestinal epithelium-specific Fut2 deficiency. J. Biomed. Sci. 28:20. doi: 10.1186/s12929-021-00711-z
Thomas, C., Pellicciari, R., Pruzanski, M., Auwerx, J., and Schoonjans, K. (2008). Targeting bile-acid signalling for metabolic diseases. Nat. Rev. Drug Discov. 7, 678–693. doi: 10.1038/nrd2619
Tong, Y., Gao, H., Qi, Q., Liu, X., Li, J., Gao, J., et al. (2021). High fat diet, gut microbiome and gastrointestinal cancer. Theranostics 11, 5889–5910. doi: 10.7150/thno.56157
Tremaroli, V., and Bäckhed, F. (2012). Functional interactions between the gut microbiota and host metabolism. Nature 489, 242–249. doi: 10.1038/nature11552
Verbanck, M., Chen, C. Y., Neale, B., and Do, R. (2018). Detection of widespread horizontal pleiotropy in causal relationships inferred from Mendelian randomization between complex traits and diseases. Nat. Genet. 50, 693–698. doi: 10.1038/s41588-018-0099-7
Vishnu, P., Duncan, J., Connell, N., Cooper, N., Lim, W., Rodeghiero, F., et al. (2021). Platelet Immunology Scientific. Standardization Committee. International survey on Helicobacter pylori testing in patients with immune thrombocytopenia: Communication of the platelet immunology scientific and standardization committee. J. Thromb. Haemost. 19, 287–296. doi: 10.1111/jth.15136
Wang, Y., Liu, F., Zhang, G., Su, Y., Sun, X., Chen, Q., et al. (2021). Gut microbiome alterations and its link to corticosteroid resistance in immune thrombocytopenia. Sci. China Life Sci. 64, 766–783. doi: 10.1007/s11427-020-1788-2
Wang, Z., and Zhao, Y. (2018). Gut microbiota derived metabolites in cardiovascular health and disease. Protein Cell 9, 416–431. doi: 10.1007/s13238-018-0549-0
Watanabe, K., Taskesen, E., van Bochoven, A., and Posthuma, D. (2017). Functional mapping and annotation of genetic associations with FUMA. Nat. Commun. 8:1826. doi: 10.1038/s41467-017-01261-5
Xiang, K., Wang, P., Xu, Z., Hu, Y. Q., He, Y. S., Chen, Y., et al. (2021). Causal effects of gut microbiome on systemic lupus erythematosus: A two-sample Mendelian randomization study. Front. Immunol. 12:667097. doi: 10.3389/fimmu.2021.667097
Xu, Q., Ni, J. J., Han, B. X., Yan, S. S., Wei, X. T., Feng, G. J., et al. (2022). Causal relationship between gut microbiota and autoimmune diseases: a two-sample Mendelian randomization study. Front. Immunol. 12:746998. doi: 10.3389/fimmu.2021.746998
Xu, Q., Zhang, S. S., Wang, R. R., Weng, Y. J., Cui, X., Wei, X. T., et al. (2021). Mendelian randomization analysis reveals causal effects of the human gut microbiota on abdominal obesity. J. Nutr. 151, 1401–1406. doi: 10.1093/jn/nxab025
Yu, X., Zheng, Q., He, Y., Yu, D., Chang, G., Chen, C., et al. (2022). Associations of gut microbiota and fatty metabolism with immune thrombocytopenia. Front. Med. 9:810612. doi: 10.3389/fmed.2022.810612
Yu, X. H., Yang, Y. Q., Cao, R. R., Bo, L., and Lei, S. F. (2021). The causal role of gut microbiota in development of osteoarthritis. Osteoarthr. Cartil. 29, 1741–1750. doi: 10.1016/j.joca.2021.08.003
Zhang, X., Gu, S., You, L., Xu, Y., Zhou, D., Chen, Y., et al. (2020). Gut microbiome and metabolome were altered and strongly associated with platelet count in adult patients with primary immune thrombocytopenia. Front. Microbiol. 11:1550. doi: 10.3389/fmicb.2020.01550
Keywords: immune thrombocytopenia, ITP, gut microbiota, Mendelian randomization study, the causal relationship
Citation: Guo D, Chen Q, Wang G, Li C and FinnGen consortium (2023) Causal relationship between gut microbiota and immune thrombocytopenia: a Mendelian randomization study of two samples. Front. Microbiol. 14:1190866. doi: 10.3389/fmicb.2023.1190866
Received: 04 May 2023; Accepted: 03 October 2023;
Published: 23 November 2023.
Edited by:
Qi Zhao, University of Science and Technology Liaoning, ChinaReviewed by:
Natalia Zeber-Lubecka, Medical Centre for Postgraduate Education, PolandShitao Rao, Fujian Medical University, China
Copyright © 2023 Guo, Chen, Wang, Li and FinnGen consortium. This is an open-access article distributed under the terms of the Creative Commons Attribution License (CC BY). The use, distribution or reproduction in other forums is permitted, provided the original author(s) and the copyright owner(s) are credited and that the original publication in this journal is cited, in accordance with accepted academic practice. No use, distribution or reproduction is permitted which does not comply with these terms.
*Correspondence: ChunPu Li, bGk0NWxpQDEyNi5jb20=; Guojun Wang, d2d1b2p1bjIwMjBAMTYzLmNvbQ==
†These authors share first authorship