- 1Department of Food Science and Technology, National University of Singapore, Singapore, Singapore
- 2National Engineering Research Center of Cereal Fermentation and Food Biomanufacturing, School of Food Science and Technology, Jiangnan University, Wuxi, Jiangsu, China
- 3Shaoxing Key Laboratory of Traditional Fermentation Food and Human Health, Jiangnan University (Shaoxing) Industrial Technology Research Institute, Shaoxing, Zhejiang, China
Sufu, a traditional Chinese fermented food, is famous for its unique flavor, especially umami. However, the formation mechanism of its umami peptides is still unclear. Here, we investigated the dynamic change of both umami peptides and microbial communities during sufu production. Based on peptidomic analysis, 9081 key differential peptides were identified, which mainly involved in amino acid transport and metabolism, peptidase activity and hydrolase activity. Twenty-six high-quality umami peptides with ascending trend were recognized by machine learning methods and Fuzzy c-means clustering. Then, through correlation analysis, five bacterial species (Enterococcus italicus, Leuconostoc citreum, L. mesenteroides, L. pseudomesenteroides, Tetragenococcus halophilus) and two fungi species (Cladosporium colombiae, Hannaella oryzae) were identified to be the core functional microorganisms for umami peptides formation. Functional annotation of five lactic acid bacteria indicated their important functions to be carbohydrate metabolism, amino acid metabolism and nucleotide metabolism, which proved their umami peptides production ability. Overall, our results enhanced the understanding of microbial communities and the formation mechanism of umami peptides in sufu, providing novel insights for quality control and flavor improvement of tofu products.
1. Introduction
Food-derived peptides exhibit various functions, such as antihypertension, antimicrobial, and antioxidant, and may also contribute to the taste of food (Raikos and Dassios, 2014; Kamran and Reddy, 2018). Umami, which is described as a pleasant savory taste, is the fifth basic taste aside from sweet, sour, salty, and bitter (Yamaguchi and Ninomiya, 1998; Lindemann et al., 2002). Umami plays an important role in food flavor, and many umami ingredients such as monosodium glutamate (MSG), 5′-inosine monophosphate (IMP), and 5′-guanosine monophosphate (GMP) (Yamaguchi, 1967; Ault, 2004; Zhang et al., 2013) are widely used in food seasoning. After the first discovery of umami peptides from soybean protein hydrolyzates (Arai et al., 1972), umami peptides have attracted extensive attention from scientists (Zhang et al., 2017, 2019). Besides adding umami to the food, umami peptides can also modulate the overall flavor through a synergistic effect via taste receptors (Shim et al., 2015; Dang et al., 2019; Wang et al., 2020; Xie et al., 2023). Umami peptides generally have a molecular weight of less than 3000 Da (Yu et al., 2017; Zhang et al., 2019; Liu et al., 2020), and those with a molecular weight between 500 and 1000 Da are assumed to have the strongest umami taste (Rhyu and Kim, 2011). Fermentation is an effective way to create umami peptides (Zhao et al., 2016, 2019). During fermentation, protein and large peptides are hydrolyzed by proteases through microbial metabolism to form abundant small peptides. Umami peptides have been identified from many traditional fermented foods, including soy sauce (Lioe et al., 2006; Zhuang et al., 2016), fish sauce (Bu et al., 2021; Zhu et al., 2021), and ham (Dang et al., 2015; Xie et al., 2023). Recently, researchers also discovered umami peptides in sufu, and their salt-enhancing effect had been proven (Xie et al., 2018a; Chen et al., 2021).
Sufu, also called fermented soybean curd or furu, is a traditional Chinese dish with a long history of over a thousand years (Han et al., 2001b). Sufu is widely consumed as a condiment due to its mellow texture, special fragrance, and umami flavor (Han et al., 2004a; Cai et al., 2021; Liu et al., 2022). Made by fermentation of tofu, sufu also has very rich nutritional value, especially high protein content of about 40% (dry matter basis) (Han et al., 2004b). Microorganisms is believed to be of vital importance to the quality of sufu. During fermentation, various kinds of enzymes produced by microbial communities hydrolyze carbohydrate, lipid and especially proteins in soybeans into small molecules, which are more volatile, bioactive and easy to digest (Xu et al., 2016; Cai et al., 2021; Li et al., 2022). Although most sufu products are fermented with a pure culture starter, bacterial and fungal communities from the environment also play a non-negligible role in sufu fermentation due to the open production environment (Han et al., 2001a, 2004a; Cai et al., 2016). Studies have been conducted on the diversity of environmental microorganisms based on geography, production methods, and fermentation stages (Han et al., 2004a; Cai et al., 2021). And the correlation between microorganisms and volatile flavor components in sufu is also well studied (Xie et al., 2018b; He and Chung, 2020; Li et al., 2022). However, there is still little knowledge of how microorganisms contribute to the formation of umami peptides during fermentation.
Until now, about 208 umami peptides have been reported. While most research on umami peptides focused on isolation and identification, their formation mechanism is still unclear. With the development of high-throughput sequencing technology, using peptidomics and microbiomics to discover new umami peptides and to study the mechanism of microorganisms and umami peptides are of great value for subsequent industrial applications. The study on peptidomics throughout sufu fermentation is relatively lacking. And the research on the changes of endogenous polypeptides as well as the function of precursor proteins during the whole fermentation process is still blank. Also, studies on fermenting microorganisms in sufu are mainly at the genus level rather than species level. Based on these facts, 24 sufu samples at different fermentation stages were collected in this study. First, the endogenous peptides during the whole fermentation process were identified. The key functional peptides were filtered, and the function of their precursor proteins was analyzed. Then, high-quality potential umami peptides were identified based on multiple machine-learning methods. The structural characteristics and diversity of the microbial community during sufu fermentation were determined by full-length amplicon sequencing. Finally, the microbial strains significantly correlated with umami peptides were identified. Overall, this study provides a theoretical reference for mining key microorganisms that determine or regulate the entire fermentation process of sufu, and provides guidance for the development and industrial application of umami peptides.
2. Materials and methods
2.1. Sampling and sample treatment
Fresh white sufu samples at different fermentation stages were harvested at a representative sufu factory located at Shaoxing, Zhejiang Province, China (120°32′E, 31°51′N). The manufacturing process of white sufu is shown in Figure 1. Soybeans were first washed and soaked in water, then grinded to obtain soymilk. Then, the filtered soymilk was boiled and coagulated by adding gesso, and pressed to make tofu. After cutting into small cubes, the diced tofu, also called white pehtze, was inoculated with Mucor spp. and fermented at 25–30°C for about 25 h to allow full growth of hypha. Subsequently, the molded pehtze was marinated by salt for about 2 days. Finally, the salted pehtze was post-aged in closed bottles after adding a dressing mixture containing edible alcohol until ripening. The samples of white pehtze (WP), molded pehtze after inoculating for 12 and 25 h (P12, P25), salted pehtze (SP), posted-fermented sufu at 7, 30, 60, 90 days (F7, F30, F60, F90) were collected for analysis. Nine bottles of samples were randomly collected at different stages as described above, and samples from each three bottles were mixed into one sample. All samples were stored at −80°C until further analysis.
2.2. Free peptide determination by peptidomics analysis
2.2.1. Peptide extraction
Sufu samples were ground in liquid nitrogen, and then mixed with methanol, chloroform and water at a ratio of 3:1:4 (V: V). After a brief vortex, the mixture was centrifuged at 4700 rpm for 10 min at 4°C. The upper aqueous supernatant was collected. Then, the collected supernatant was desalted using Waters Oasis MCX desalination column (Waters, Milford, MA, USA). The flow-through was collected and lyophilized.
2.2.2. Identification by LC-MS/MS
The lyophilized sample was redissolved in distilled water with 0.1% formic acid for 10 μg/μL. The sample was analyzed using Nanoflow LC-MS/MS on a quadrupole Orbitrap mass spectrometer (Q Exactive HF-X, Thermo Fisher Scientific, Bremen, Germany) coupled to an EASY nLC 1200 ultra-high-pressure system (Thermo Fisher Scientific) via a nano-electrospray ion source. Peptides were separated using a gradient from 8 to 12% B in 5 min, then 12–30% B in 33 min and stepped up to 40% in 7 min followed by a 15 min wash at 95% B with a flow rate of 0.60 μL/min onto the Thermo C-18 column (150 μm × 250 mm, Thermo, Waltham, MA, USA), where solvent A was 0.1% formic acid in water and solvent B was 80% ACN and 0.1% formic acid in water. The total duration of the run was 60 min, and the column temperature was kept at 60°C. Briefly, the mass spectrometer was operated in “top-40” data-dependent mode, collecting MS spectra in the Orbitrap mass analyzer (120,000 resolution, 100–1500 m/z range) with an automatic gain control (AGC) target of 3E6 and a maximum ion injection time of 80 ms. The most intense ions from the full scan were isolated with an isolation width of 1.6 m/z. Following higher-energy collisional dissociation (HCD) with a normalized collision energy (NCE) of 27, MS/MS spectra were collected in the Orbitrap (15,000 resolution) with an AGC target of 5E4 and a maximum ion injection time of 45 ms. Precursor dynamic exclusion was enabled with a duration of 16 s. All RAW files were analyzed using PEAKS (version 8, Bioinformatics Solutions Inc.) (Ma et al., 2003). MS2 spectra collected in the Orbitrap was searched against the Glycine max (protein number: 74,863, database: uniport, download time: 2022.09.15) with parameters as following: precursor mass tolerance of 15 ppm and a fragment mass tolerance of 0.02 Da; ALC (%) setting greater than 50%; false discovery rate (FDR) of less than 1% (Tran et al., 2019).
2.2.3. Identification of differential peptides and function analysis of precursor protein
Based on the quantitative results, T-test was used for differential analysis between each group and the unfermented group WP, respectively. Differential peptides were screened based on the standard | log2(FoldChange)| > 1 and false discovery rate (FDR) < 0.05. The function of precursor protein was annotated using COG (Eukaryotic Orthologous Groups) protein orthologous cluster database,1 SwissPro protein sequence database,2 KEGG (Kyoto Encyclopedia of Genes and Genomes) Kyoto Gene Encyclopedia Database,3 GO (Gene Ontology) Gene Ontology Database.4 KEGG and GO functional enrichment analysis were performed using R studio.
2.2.4. Screening of umami peptides
In order to improve the efficiency, three machine learning methods iUmami-SCM,5 UMPred-FRL6, and Umami_YYDS7 were used for screening of umami peptides. All the three databases predicted umami peptides mainly based on their primary sequence information. However, iUmami-SCM database used a scoring card method (SCM) (Charoenkwan et al., 2020), while UMPred-FRL used a feature representation learning method (FRL) (Charoenkwan et al., 2021). Umami_YYDS built a larger database by collecting reported umami peptide information to improve accuracy (Cui et al., 2023). Umami peptides were screened based on three criteria: (a) Peptide length less than 10. (b) Predicted as umami by all three methods. (c) The probability rate should be over 80%.
2.3. Amplicon sequencing
2.3.1. Genomic DNA extraction, PCR amplification and sequencing
The metagenomic DNA of samples was extracted using CTAB-based method (Allen et al., 2006). The quality of each extracted DNA was determined by a NanoDrop 2000c Spectrophotometers (Thermo Fisher Scientific, Wilmington, MA, USA) and 1% agarose gel electrophoresis. For bacterial, the full-length 16S rRNA V1–V9 region was amplified by PCR with universal primers 27F (5′-AGGRGTTTGATYNTGGCTCAG-3′) and 1492R (5′-TASGGHTACCTTGTTASGACTT-3′) (Lane, 1991). For fungus, the fungal internal transcribed spacer (ITS) region was amplified with primer sets of ITS1f (5′-CTTGGTTCATTTAGAGGAAGTAA-3′) (Gardes and Bruns, 1993) and ITS4 (5′-TCCTCCGCTTATTGATATGC-3′) (White et al., 1990). Sequencing of both libraries was performed in the Circular Consensus Sequencing (CCS) mode on the PacBio RS II platform (Pacific Biosciences, Menlo Park, CA, USA).
2.3.2. Sequence analysis
Lima (v2.7.1)8 was used to identify CCS through barcode, and get raw sequence data. Raw sequence data was corrected and filtered with 99% accuracy and desired sequence length to remove adapter pollution and low-quality data by cutadapt (v1.9.1) (Martin, 2011). UCHIME (v4.2) software was used to identify and remove chimeric sequences to obtain Effective sequences (Edgar, 2016). Based on 97% similarity, UCLUST was used to cluster high-quality sequences into different Operational Taxonomic Units (OTUs) (Edgar, 2010). A single representative sequence from each clustered OTU was used for annotation with the Silva database (v13.2) and the UNITE fungal database (v12.11) to obtain taxonomic information (Quast et al., 2012; Nilsson et al., 2019). Singleton OTUs was discarded before further analysis to eliminate errors. Chao1 richness index and Shannon diversity index were calculated using QIIME2 (v2022.11) (Dunn, 1973; Bolyen et al., 2019). Visualization of microbial community structure composition was provided by Principal Co-ordinates Analysis (PCoA).
3. Results and discussion
3.1. Peptidomics and umami peptides during sufu production
3.1.1. Peptide identification based on de novo sequencing
As a branch of proteomics, peptidomics focuses specifically on endogenous peptides present in the sample, and can reflect the process of proteolytic cleavages (Schrader et al., 2014; Dallas et al., 2015). Peptidomics is usually composed of peptide identification and quantitation, function prediction, and high-throughput analysis. Mass spectrometry (MS) is a powerful tool for peptide identification and quantification. In this study, peptidomics composed of MS/MS data analyzed by PEAKS software through de novo sequencing and Glycine max database matching was used to quickly identify and characterize peptides from different fermentation stages of sufu. A total of 40,479 peptides were identified in this study at different stages of fermentation. As shown in Figure 2A, principal component analysis (PCA) indicated good parallelism for three biological replicates in each group. The peptides in WP exhibited more difference than other groups, while no significant difference was shown among SP, F7, and F30. The distribution of peptide molecular weight was analyzed in Figure 2B. Peptides with molecular weight less than 1000 Da were dominant during sufu production, and were over 33.3% in all samples. The percentage of peptides less than 1500 Da generally increased through both early and post stage of fermentation. In contrast, peptides with molecular weight greater than 2000 Da displayed a descending tendency in abundance. This phenomenon supported the idea that peptides were hydrolyzed into smaller molecules during sufu fermentation (Xu et al., 2016; Cai et al., 2021; Li et al., 2022).
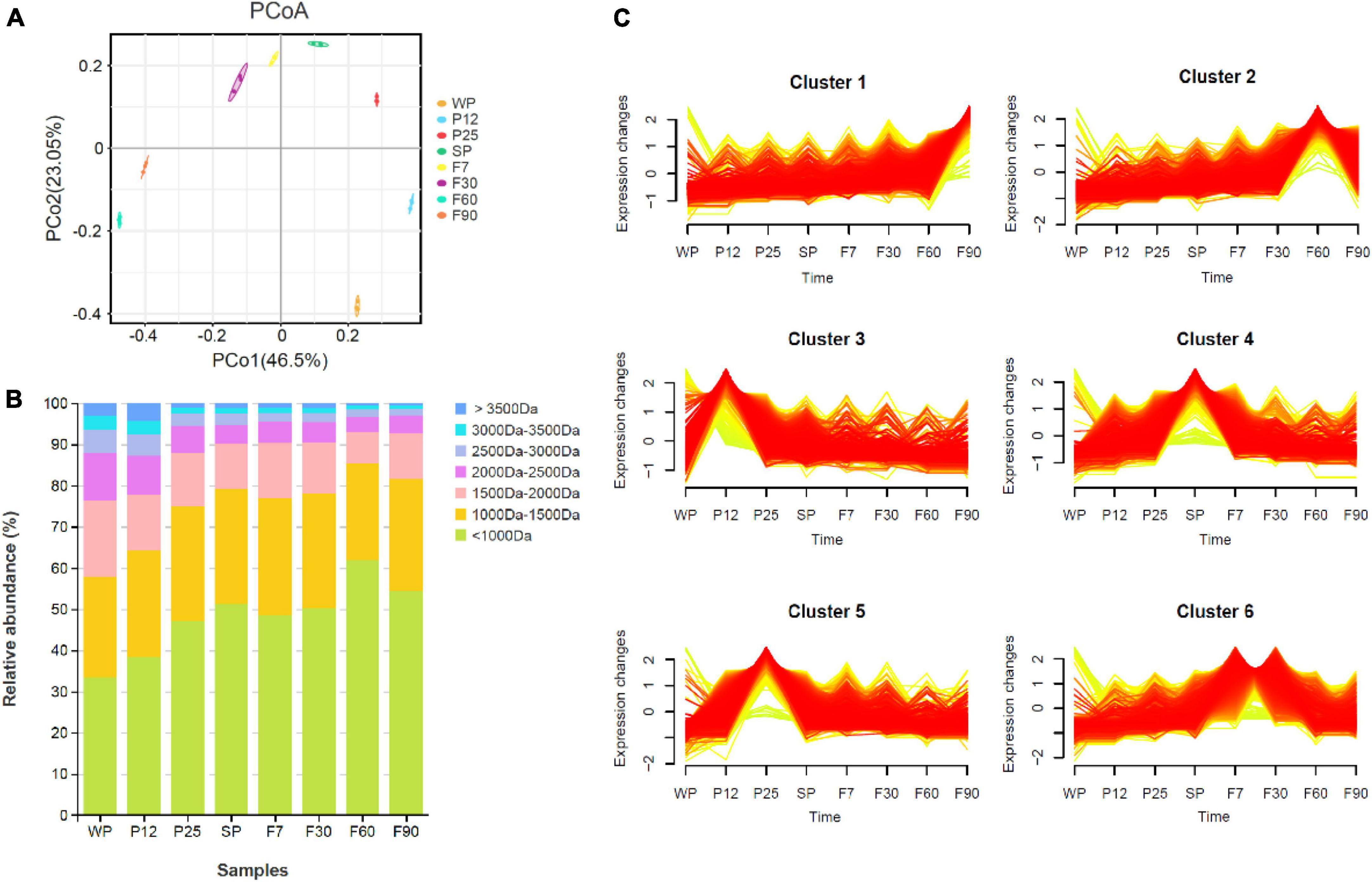
Figure 2. Peptide analysis during fermentation of sufu. (A) PCA analysis. (B) Molecular weight distribution of total peptides. (C) Trend analysis of differentially expressed peptides.
3.1.2. Identification of differential peptides and function prediction of precursor protein
In order to learn the role of peptides during sufu fermentation, WP was used as the control group to analyze the differential peptides. A total of 9,081 differential peptides were identified in this study. To clarify the changes in endogenous peptides and the functions of precursor proteins throughout the fermentation process, Mfuzz C-Means Clustering was used for temporal trend analysis of differential peptide expression. Mfuzz is able to identify underlying time-series patterns of expression profiles and cluster peptides with similar patterns, which can help us understand the connection between dynamic patterns of peptides and their functions (Dunn, 1973). As shown in Figure 2C, six clusters of peptides with different expression patterns were obtained. Cluster 1 displayed an ascending tendency over time, and includes 937 differential peptides. GO analysis showed that for the most part, the precursor proteins for differential peptides were annotated into the groups of cellular protein catabolic process, cysteine-type endopeptidase activity, peptidase activity, acting on L-amino acid peptides, and hydrolase activity (Supplementary Table 1). The KEGG pathway enrichment analysis was also conducted. The result indicated that the differential peptides were mainly involved in seven different pathways, including carbon metabolism, protein processing in endoplasmic reticulum, glycolysis/gluconeogenesis, biosynthesis of amino acids, and glyoxylate and dicarboxylate metabolism (Supplementary Table 2). COG annotation results show that the main functions of precursor proteins include post translational modification, protein turnover, chaperones, amino acid transport and metabolism, carbohydrate transport and metabolism, secondary metabolites biosynthesis, transport, and catabolism (Supplementary Table 3).
3.1.3. Prediction of umami peptides in sufu
During sufu fermentation, complexes formed by degradation of soybean protein can generate a strong umami taste (Yoshida, 1998; Hajeb and Jinap, 2015). Umami peptides, which have attracted increasing attention, can greatly improve food flavor and enhance taste perception (Zhang et al., 2017, 2019). The study of umami peptides not only provides reference for the quality improvement of food products, but also the development of new seasonings. In this study, three machine learning methods (iUmami-SCM, UMPred-FRL, Umami_YYDS) based on computational techniques were used for rapid screening of large-scale umami peptides (Charoenkwan et al., 2020, 2021; Cui et al., 2023). Among 40,479 endogenous peptides, 3,470, 3,245, and 4,381 peptides were identified as umami by three models respectively (Figure 3A). After filtering and taking the intersection, 183 high-quality candidate umami peptides were obtained. Based on the results of differential peptide trend analysis (Figure 2C), it was surprising to find that 26 umami peptides showed an upward trend consistently in the umami taste during sufu fermentation (Figure 3B). The precursor proteins of these peptides were mainly lipoxygenase, phospholipase D, glycinin G4, and phosphopyruvate hydratase (Supplementary Table 4). Studies had shown that these precursor proteins were related to the formation of umami flavor in food. Overall, the umami peptides we screened had the potential to produce a strong umami taste, which had great significance for the development and application of subsequent umami peptide products.
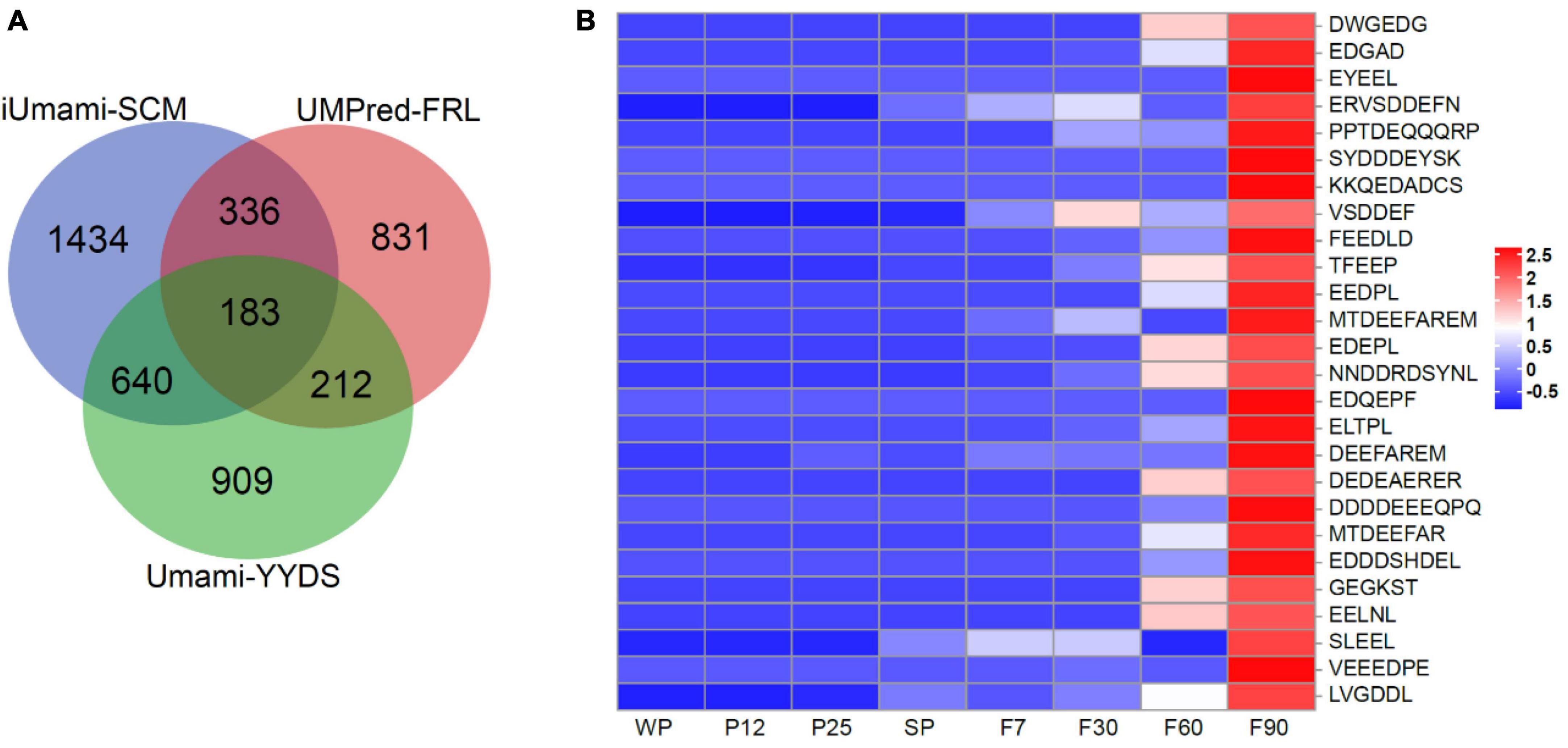
Figure 3. Umami peptides in sufu. (A) Venn analysis for the identification of umami peptides. (B) Heatmap analysis of umami peptides during fermentation of sufu.
3.2. Microbiota profile during sufu production
3.2.1. Similarity in microbiome composition at different stages
Microorganisms in sufu metabolize and synthesize flavor substances by secreting various kinds of enzymes. Its community composition plays an important role in regulating the flavor of sufu, and is considered to be a key factor in determining the quality of final products (Tan et al., 2020). In order to evaluate the variation and similarity in microbiome composition at different fermentation stages of sufu, beta diversity was analyzed by principal coordinate analysis (PCoA) plots. As shown in Figure 4, each sufu sample was represented by one dot, and samples in each group were clustered by an ellipse. The closer the two groups were ordinated, the more similar their microbiota profile. The PCoA plot for bacteria indicated the clear difference between WP, P25 and F90 due to their long distance. It was also noticed that the ellipses of WP and P12 were highly overlapped, indicating their high similarity in microbiome composition. Besides, the high similarity was also shown between SP and F7; F60 and F90. During the early stage of fermentation, sufu bacteria community changed the most after inoculation with Mucor for 12–25 h. And since the distance between F7 and F30 was longer than that between F30 and F60, F60 and F90, it can be concluded that the bacteria community of sufu mainly changed during the first 30 days rather than the later 60 days. According to Figure 4B, for fungi, P12 and F60 exhibited the most difference in community structures due to their longest distance. F7 and F30 were highly similar due to the large overlap, which was different from the pattern of the bacteria community.
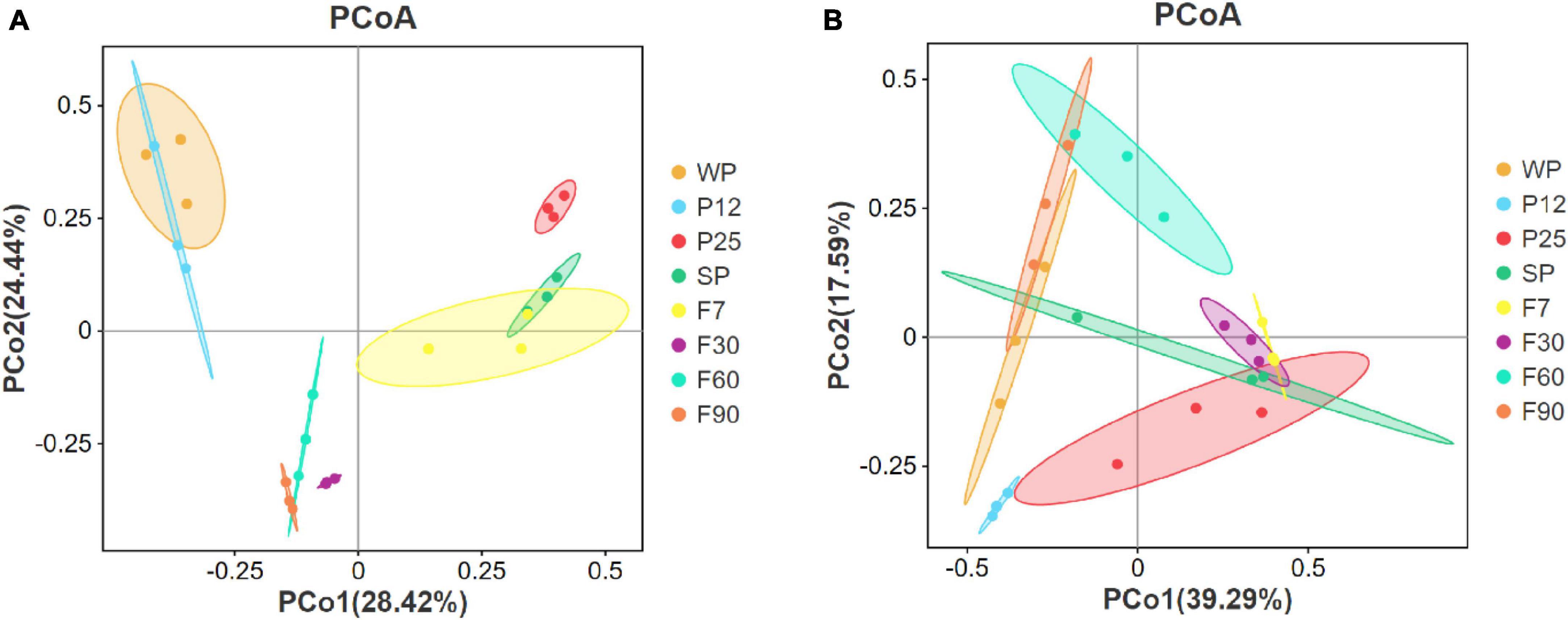
Figure 4. Principal coordinate analysis (PCoA) of the bacterial (A) and fungal (B) communities based on Bray-Curtis distances.
3.2.2. Abundance and diversity of microbial communities
Alpha diversity indices were used to evaluate the abundance and diversity of microbial communities during sufu production (Figure 5). While the Chao1 index and PD tree positively correlated with the abundance or richness of species, Simpson and Shannon index measured the degree of diversity within the community. As shown in Figure 5A, the abundance and diversity of bacterial communities fluctuated over time. It was noticed that Chao1 and PD tree indices significantly increased (P < 0.05) from F7 to F30, and continuously decreased after reaching its highest value at F30. Such trend indicated that the abundance of bacterial communities was significantly influenced by the addition of dressing, and was consistent with previous studies (Huang et al., 2018; He and Chung, 2020). The decrease in both abundance and diversity of bacterial communities after ripening for 30 days may due to the depletion of oxygen in the bottle. For fungal communities (Figure 5B), Chao1 and PD tree indices significantly decreased (P < 0.05) from WP to P12. These results indicated that the inoculation with Mucor significantly decreased the abundance of fungal communities. Simpson and Shannon indices decreased continuously before reaching their lowest value at F7, and kept increasing at the ripening stages. This suggested long ripening time of 90 days contributed to the diversity of fungal communities, which was also supported by previous studies (He and Chung, 2020; Li et al., 2022). It was also noticed that compared with fungal communities, bacterial communities were significantly richer during the whole production process. Such microbial community structure was consistent with other sufu products fermented by mold.
3.2.3. Composition of microbial community
Third-generation sequencing technology has been less studied in the fermentation process of sufu. Compared with second-generation sequencing, full-length sequencing avoids systematic errors due to PCR while improving read length, and therefore restoring the community structure of microorganisms more realistically at the species level (Shin et al., 2016; Callahan et al., 2019; Tedersoo and Anslan, 2019). In order to improve the efficiency and accuracy, full-length ITS and 16S rRNA gene amplicon analysis was used in this study. The composition of bacteria and fungi communities at both the genus and the species levels were compared in Figure 6.
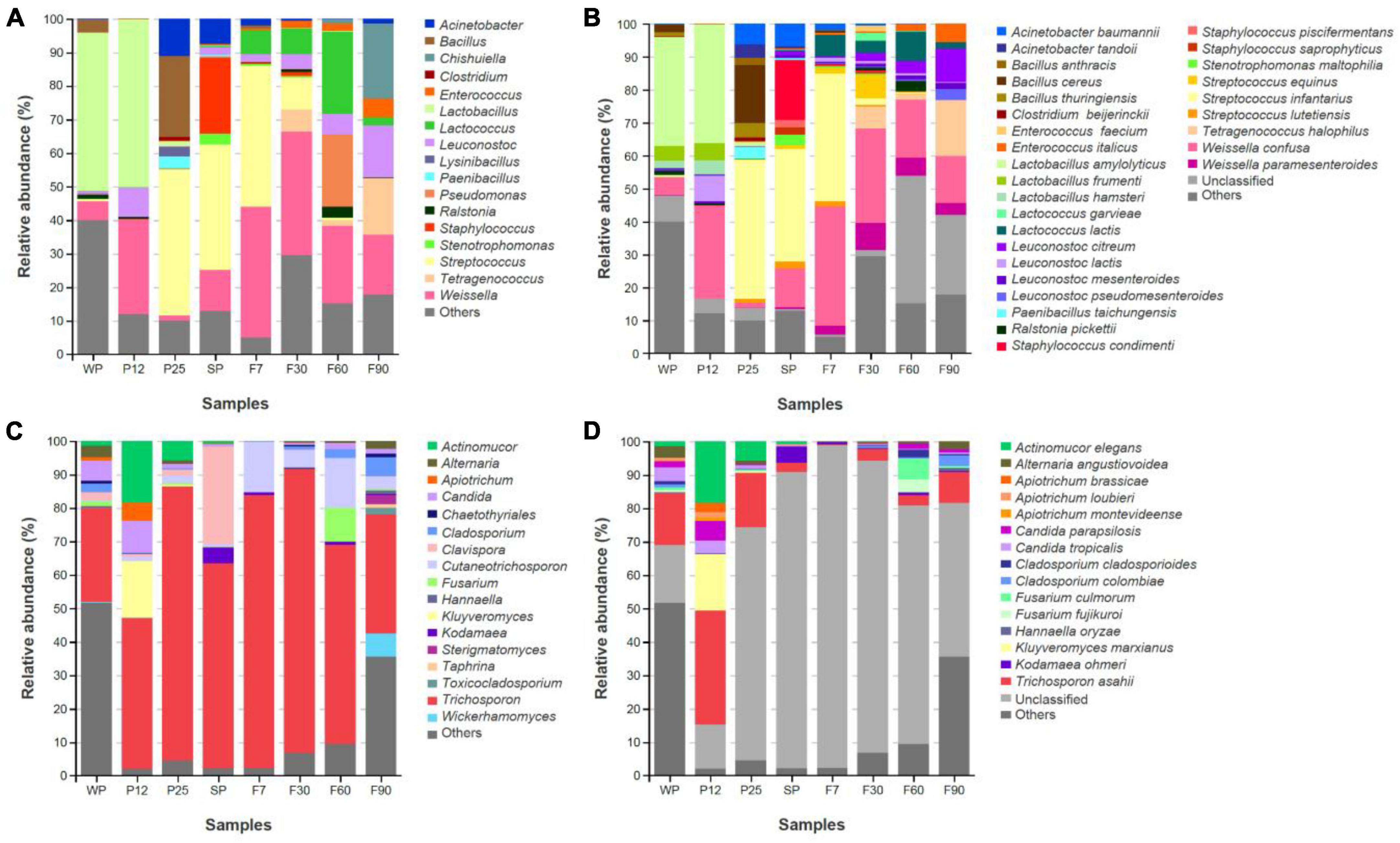
Figure 6. Structure and succession of microbial community during fermentation of bacteria (A) and fungi (C) at the genus level; structure and succession of microbial community during fermentation of bacteria (B) and fungi (D) at the species level.
For the bacteria community, a total of 18 genera and 31 species with an abundance greater than 1% were identified from samples at all stages. At the genus level (Figure 6A), the top three bacterial genera across the whole production process were Weissella, Lactobacillus and Streptococcus. Unlike Weissella, which was widely distributed at all stages, Lactobacillus was predominant before solid fermentation for 25 h, and Streptococcus was prevailing at P25, SP, and F7. At the species level (Figure 6B), Lactobacillus amylolyticus was identified as the predominant bacterial at WP (33.1%) and P12 (36.1%), but rapidly decreased and became a minor community member after incubating with Mucor for 25 h. The other two major bacterial communities at P12, Weissella confusa (28.1%) and Leuconostoc lactis (7.8%), also experienced a sharp decline in abundance at P25. The massive proliferation of Acinetobacter baumannii (6.2%), Bacillus cereus (17.6%), and Streptococcus infantarius (42.2%) at P25 might inhibit their growth. For salted phetz, Staphylococcus condimenti (18.2%) was assigned to the most abundant bacteria apart from Streptococcus infantarius (34.3%). The rapid growth of Staphylococcus condimenti might attribute to its perfect salt tolerance. For the ripening stages, it was interesting to find that Leuconostoc citreum kept growing during the whole ripening process, while the abundance of Weissella confusa and Streptococcus infantarius had steadily declined. This might be due to their different tolerance to the dressing mixture containing ethanol.
For fungal communities, Trichosporon was dominant at all stages. Before salt was added to molded pehtze at SP, its abundance kept increasing (Figure 6C). As the salt concentration reduced from SP to F7, Trichosporon showed upregulation, and then gradually descended after ripening in the dressing for 30 days. The pervious study also proved the decrease of Trichosporon during sufu ripening (Wei et al., 2021). During solid-state fermentation, Actinomucor elegans and Kluyveromyces marxianus were introduced to the community, with the abundance of 18.4 and 17.0% at P12, but a descending tendency later (Figure 6D). This phenomenon was consistent with previous studies that demonstrated salt could inhibit the growth of Mucor as well as other salt-sensitive microorganisms (Han et al., 2004a; Xie et al., 2018b; He and Chung, 2020).
Compared with previous studies in sufu, significant differences occurred in the microbial communities. The predominant genus such as Debaryomyces, Candida, and Aspergillus (Xu et al., 2020; Wei et al., 2021; Li et al., 2022) detected in previous studies appeared to be minor or undetected in this study. This might be originated from the difference in the starter culture and detection technology. Moreover, microorganisms obtained from the environment also made a great contribution to the microbial profile.
3.3. Core functional microorganisms related to formation of umami peptides
Microbial fermentation is one of the main production methods of soy peptides. As microorganisms carry out their own metabolic activities, mixed enzymes were produced to hydrolyze soy protein into peptides with different lengths and sequences (de Oliveira et al., 2015). Meanwhile, utilizing the nutrients in peptide hydrolyzate facilitates the growth and enzyme production capabilities of microorganisms, which significantly improves the hydrolysis efficiency (Cruz-Casas et al., 2021). In this study, the correlation between 26 umami peptides and identified microbial species including 29 bacterial and 15 fungi was analyzed using Pearson’s correlation tests. The core functional microorganisms related to the formation of umami peptides were selected based on the standard that average Pearson correlation coefficient r > 0.75 and P < 0.05. As shown in the correlation heatmap (Figure 7), 5 bacterial species and 2 fungi species were found significantly correlated with the 26 umami peptides. The correlation network was further analyzed in Figure 8. For fungi communities, only Cladosporium Colombia and Hannaella oryzae showed a significantly positive association with most of the umami peptides. However, their function on flavor and umami taste of fermented foods was seldom reported. For bacterial, the core functional genera related to umami peptides production were Enterococcus italicus, Leuconostoc citreum, Leuconostoc mesenteroides, Leuconostoc pseudomesenteroides and Tetragenococcus halophilus, which showed significant positive correlations with more than 20 umami peptides (Figure 8A). It was noticed that all five functional bacterial species belonged to lactic acid bacteria (LAB). They had been widely discovered from fermented foods such as cheese, kimchi, and soy sauce, and made great contributions to the flavor and taste (Nguyen et al., 2013). Leuconostoc citreum had been reported to exhibit glutaminase-producing activity in a traditional Thai fermented food (Thongsanit et al., 2009). Tetragenococcus halophilus was also proven to improve the flavor of fish sauce and increase the umami taste in soy sauce (Udomsil et al., 2011; Zhang et al., 2020). The genome sequences of five bacterial strains correlated with the formation of umami peptides were downloaded from NCBI. The specific information was shown in Supplementary Table 5. KEGG functional annotation was performed using eggNOG.9 The results showed that amino acid metabolism was the second important enrichment pathway (Figure 8B), which verified their umami peptides production ability.
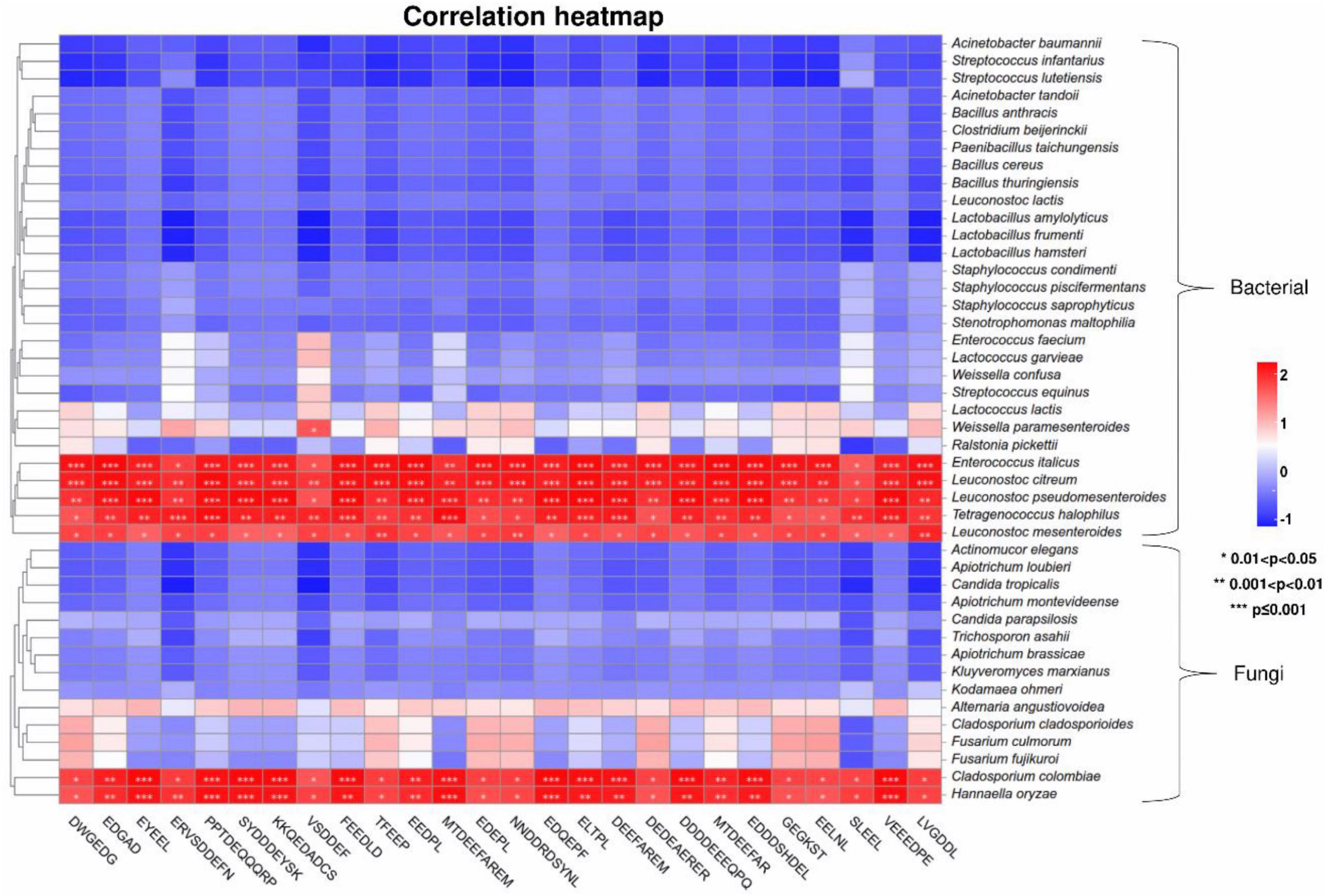
Figure 7. Clustering heatmaps based on Pearson’s correlation tests between the umami peptides and microorganisms based on species level.
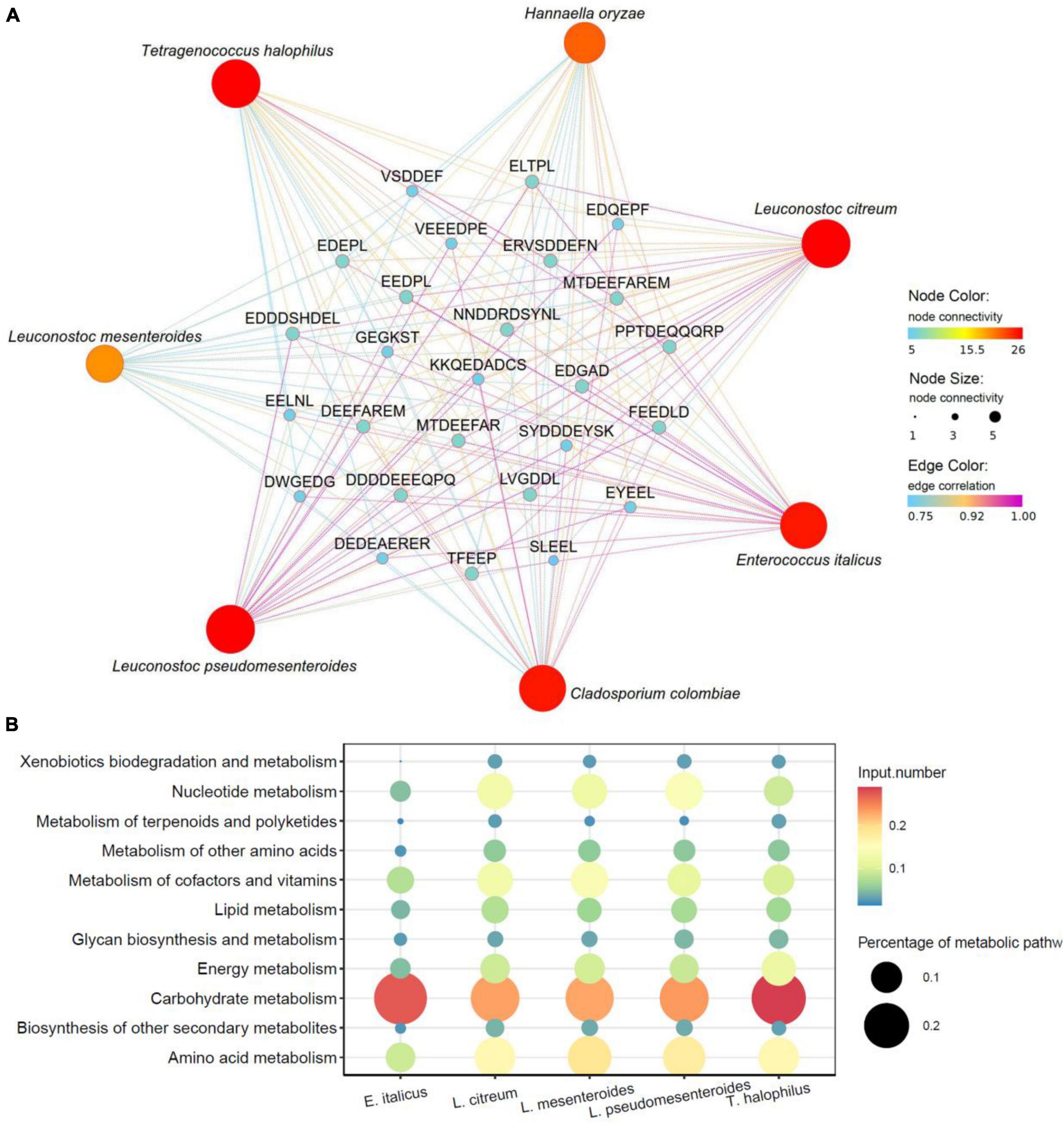
Figure 8. Correlation of microorganisms and peptides. (A) Correlation network maps based on Pearson’s correlation tests between the umami peptides and microorganisms based on species level. (B) KEGG enrichment analysis from E. italicus, L. citreum, L. mesenteroides, L. pseudomesenteroides and T. halophilus.
4. Conclusion
Overall, this study clarified the changes in endogenous peptides, the functions of their precursor proteins, and the succession of microorganisms throughout the 8 stages of sufu fermentation. 26 novel umami peptides with increasing expression patterns were identified. Also, five lactic acid bacteria that facilitated umami peptides formation were discovered at the species level for the first time. Our results have certain guiding significance for the subsequent development and utilization of biological resources. By regulating the core functional microorganisms, it was possible to improve the flavor and quality of commercial sufu products. Also, the discovery of novel umami peptides might provide new ideas for the development of food seasonings in the condiment industry. Further studies should be performed to verify strain’s umami peptide producing ability through isolation and cultivation. The umami intensity of novel peptides should also be examined through sensory evaluation and electric tongue analysis.
Data availability statement
The original contributions presented in this study are included in the article/Supplementary material, further inquiries can be directed to the corresponding author.
Author contributions
JM: conceptualization, data curation, visualization, writing – original draft, and writing – review and editing. ZZ: resources and writing – review and editing. HY: supervision, methodology, writing – review and editing, and resources. All authors contributed to the article and approved the submitted version.
Funding
This work was supported by the National Key R&D Program of China (2021YFD2100102-4).
Conflict of interest
The authors declare that the research was conducted in the absence of any commercial or financial relationships that could be construed as a potential conflict of interest.
Publisher’s note
All claims expressed in this article are solely those of the authors and do not necessarily represent those of their affiliated organizations, or those of the publisher, the editors and the reviewers. Any product that may be evaluated in this article, or claim that may be made by its manufacturer, is not guaranteed or endorsed by the publisher.
Supplementary material
The Supplementary Material for this article can be found online at: https://www.frontiersin.org/articles/10.3389/fmicb.2023.1181588/full#supplementary-material
Footnotes
- ^ https://www.ncbi.nlm.nih.gov/research/cog-project/
- ^ http://www.expasy.ch/sprot
- ^ http://www.genome.jp/kegg
- ^ http://www.geneontology.org/
- ^ http://camt.pythonanywhere.com/iUmami-SCM
- ^ http://pmlabstack.pythonanywhere.com/UMPred-FRL
- ^ http://tastepeptides-meta.com/cal
- ^ https://lima.how/
- ^ http://eggnog-mapper.embl.de/
References
Allen, G. C., Flores-Vergara, M., Krasynanski, S., Kumar, S., and Thompson, W. (2006). A modified protocol for rapid DNA isolation from plant tissues using cetyltrimethylammonium bromide. Nat. Protoc. 1, 2320–2325. doi: 10.1038/nprot.2006.384
Arai, S., Yamashita, M., and Fujimaki, M. (1972). Glutamyl oligopeptides as factors responsible for tastes of a proteinase-modified soybean protein. Agric. Biol. Chem. 36, 1253–1256. doi: 10.1080/00021369.1972.10860400
Ault, A. (2004). The monosodium glutamate story: The commercial production of MSG and other amino acids. J. Chem. Educ. 81:347. doi: 10.1021/ed081p347
Bolyen, E., Rideout, J. R., Dillon, M. R., Bokulich, N. A., Abnet, C. C., Al-Ghalith, G. A., et al. (2019). Reproducible, interactive, scalable and extensible microbiome data science using QIIME 2. Nat. Biotechnol. 37, 852–857. doi: 10.1038/s41587-019-0209-9
Bu, Y., Liu, Y., Luan, H., Zhu, W., Li, X., and Li, J. (2021). Characterization and structure–activity relationship of novel umami peptides isolated from Thai fish sauce. Food Funct. 12, 5027–5037. doi: 10.1039/D0FO03326J
Cai, H., Dumba, T., Sheng, Y., Li, J., Lu, Q., Liu, C., et al. (2021). Microbial diversity and chemical property analyses of sufu products with different producing regions and dressing flavors. LWT Food Sci. Technol. 144:111245. doi: 10.1016/j.lwt.2021.111245
Cai, R. C., Li, L., Yang, M., Cheung, H. Y., and Fu, L. (2016). Changes in bioactive compounds and their relationship to antioxidant activity in white sufu during manufacturing. Int. J. Food Sci. Technol. 51, 1721–1730. doi: 10.1111/ijfs.13149
Callahan, B. J., Wong, J., Heiner, C., Oh, S., Theriot, C. M., Gulati, A. S., et al. (2019). High-throughput amplicon sequencing of the full-length 16S rRNA gene with single-nucleotide resolution. Nucleic Acids Res. 47, e103–e103. doi: 10.1093/nar/gkz569
Charoenkwan, P., Nantasenamat, C., Hasan, M. M., Moni, M. A., Manavalan, B., and Shoombuatong, W. (2021). UMPred-FRL: A new approach for accurate prediction of umami peptides using feature representation learning. Int. J. Mol. Sci. 22:13124. doi: 10.3390/ijms222313124
Charoenkwan, P., Yana, J., Nantasenamat, C., Hasan, M. M., and Shoombuatong, W. (2020). iUmami-SCM: A novel sequence-based predictor for prediction and analysis of umami peptides using a scoring card method with propensity scores of dipeptides. J. Chem. Inf. Model. 60, 6666–6678. doi: 10.1021/acs.jcim.0c00707
Chen, Y. P., Wang, M., Blank, I., Xu, J., and Chung, H. Y. (2021). Saltiness-enhancing peptides isolated from the chinese commercial fermented soybean curds with potential applications in salt reduction. J. Agric. Food Chem. 69, 10272–10280. doi: 10.1021/acs.jafc.1c03431
Cruz-Casas, D. E., Aguilar, C. N., Ascacio-VALDéS, J. A., RODRíGUEZ-Herrera, R., CHáVEZ-GONZáLEZ, M. L., and Flores-Gallegos, A. C. (2021). Enzymatic hydrolysis and microbial fermentation: The most favorable biotechnological methods for the release of bioactive peptides. Food Chem. 3:100047. doi: 10.1016/j.fochms.2021.100047
Cui, Z., Zhang, Z., Zhou, T., Zhou, X., Zhang, Y., Meng, H., et al. (2023). A TastePeptides-Meta system including an umami/bitter classification model Umami_YYDS, a TastePeptidesDB database and an open-source package Auto_Taste_ML. Food Chem. 405:134812. doi: 10.1016/j.foodchem.2022.134812
Dallas, D. C., Guerrero, A., Parker, E. A., Robinson, R. C., Gan, J., German, J. B., et al. (2015). Current peptidomics: Applications, purification, identification, quantification, and functional analysis. Proteomics 15, 1026–1038. doi: 10.1002/pmic.201400310
Dang, Y., Gao, X., Ma, F., and Wu, X. (2015). Comparison of umami taste peptides in water-soluble extractions of Jinhua and Parma hams. LWT Food Sci. Technol. 60, 1179–1186. doi: 10.1016/j.lwt.2014.09.014
Dang, Y., Hao, L., Cao, J., Sun, Y., Zeng, X., Wu, Z., et al. (2019). Molecular docking and simulation of the synergistic effect between umami peptides, monosodium glutamate and taste receptor T1R1/T1R3. Food Chem. 271, 697–706. doi: 10.1016/j.foodchem.2018.08.001
de Oliveira, C. F., CORRêA, A. P. F., Coletto, D., Daroit, D. J., Cladera-Olivera, F., and Brandelli, A. (2015). Soy protein hydrolysis with microbial protease to improve antioxidant and functional properties. J. Food Sci. Technol. 52, 2668–2678. doi: 10.1007/s13197-014-1317-7
Dunn, J. C. (1973). A fuzzy relative of the ISODATA process and its use in detecting compact well-separated clusters. J. Cybern. 3, 32–57. doi: 10.1080/01969727308546046
Edgar, R. C. (2010). Search and clustering orders of magnitude faster than BLAST. Bioinformatics 26, 2460–2461. doi: 10.1093/bioinformatics/btq461
Edgar, R. C. (2016). UCHIME2: Improved chimera prediction for amplicon sequencing. bioRxiv [Preprint]. doi: 10.1101/074252
Gardes, M., and Bruns, T. D. (1993). ITS primers with enhanced specificity for basidiomycetes - application to the identification of mycorrhizae and rusts. Mol. Ecol. 2, 113–118. doi: 10.1111/j.1365-294X.1993.tb00005.x
Hajeb, P., and Jinap, S. (2015). Umami taste components and their sources in Asian foods. Crit. Rev. Food Sci. Nutr. 55, 778–791. doi: 10.1080/10408398.2012.678422
Han, B.-Z., Rombouts, F. M., and Nout, M. R. (2001b). A Chinese fermented soybean food. Int. J. Food Microbiol. 65, 1–10. doi: 10.1016/S0168-1605(00)00523-7
Han, B.-Z., Beumer, R. R., Rombouts, F. M., and Nout, M. R. (2001a). Microbiological safety and quality of commercial sufu–a Chinese fermented soybean food. Food Control 12, 541–547. doi: 10.1016/S0956-7135(01)00064-0
Han, B.-Z., Cao, C.-F., Rombouts, F. M., and Nout, M. J. R. (2004a). Microbial changes during the production of Sufu—-a Chinese fermented soybean food. Food Control 15, 265–270. doi: 10.1016/S0956-7135(03)00066-5
Han, B.-Z., Rombouts, F. M., and Nout, M. J. R. (2004b). Amino acid profiles of sufu, a Chinese fermented soybean food. J. Food Composit. Anal. 17, 689–698.
He, W., and Chung, H. Y. (2020). Exploring core functional microbiota related with flavor compounds involved in the fermentation of a natural fermented plain sufu (Chinese fermented soybean curd). Food Microbiol. 90:103408.
Huang, X., Yu, S., Han, B., and Chen, J. (2018). Bacterial community succession and metabolite changes during sufu fermentation. LWT Food Sci. Technol. 97, 537–545. doi: 10.1016/j.lwt.2018.07.041
Kamran, F., and Reddy, N. (2018). Bioactive peptides from legumes: Functional and nutraceutical potential. Recent Adv. Food Sci. 1, 134–149.
Lane, D. (1991). “16S/23S rRNA sequencing,” in Nucleic acid techniques in bacterial systematics, eds E. Stackebrandt and M. Goodfellow (New York, NY: John Wiley and Sons), 115–175.
Li, K., Tang, J., Zhang, Z., Wu, Z., Zhong, A., Li, Z., et al. (2022). Correlation between flavor compounds and microorganisms of Chaling natural fermented red sufu. LWT Food Sci. Technol. 154:112873. doi: 10.1016/j.lwt.2021.112873
Lindemann, B., Ogiwara, Y., and Ninomiya, Y. (2002). The discovery of umami. Chem. Sens. 27, 843–844. doi: 10.1093/chemse/27.9.843
Lioe, H. N., Takara, K., and Yasuda, M. (2006). Evaluation of peptide contribution to the intense umami taste of Japanese soy sauces. J. Food Sci. 71, S277–S283. doi: 10.1111/j.1365-2621.2006.tb15654.x
Liu, L., Chen, X., Hao, L., Zhang, G., Jin, Z., Li, C., et al. (2022). Traditional fermented soybean products: Processing, flavor formation, nutritional and biological activities. Crit. Rev. Food Sci. Nutr. 62, 1971–1989.
Liu, Z., Zhu, Y., Wang, W., Zhou, X., Chen, G., and Liu, Y. (2020). Seven novel umami peptides from Takifugu rubripes and their taste characteristics. Food Chem. 330:127204. doi: 10.1016/j.foodchem.2020.127204
Ma, B., Zhang, K., Hendrie, C., Liang, C., Li, M., Doherty-Kirby, A., et al. (2003). PEAKS: Powerful software for peptide de novo sequencing by tandem mass spectrometry. Rapid Commun. Mass Spectrom. 17, 2337–2342. doi: 10.1002/rcm.1196
Martin, M. (2011). Cutadapt removes adapter sequences from high-throughput sequencing reads. EMBnet J. 17, 10–12. doi: 10.14806/ej.17.1.200
Nguyen, D. T. L., Van Hoorde, K., Cnockaert, M., De Brandt, E., Aerts, M., and Vandamme, P. (2013). A description of the lactic acid bacteria microbiota associated with the production of traditional fermented vegetables in Vietnam. Int. J. Food Microbiol. 163, 19–27. doi: 10.1016/j.ijfoodmicro.2013.01.024
Nilsson, R. H., Larsson, K.-H., Taylor, A. F. S., Bengtsson-Palme, J., Jeppesen, T. S., Schigel, D., et al. (2019). The UNITE database for molecular identification of fungi: Handling dark taxa and parallel taxonomic classifications. Nucleic Acids Res. 47, D259–D264. doi: 10.1093/nar/gky1022
Quast, C., Pruesse, E., Yilmaz, P., Gerken, J., Schweer, T., Yarza, P., et al. (2012). The SILVA ribosomal RNA gene database project: Improved data processing and web-based tools. Nucleic Acids Res. 41, D590–D596. doi: 10.1093/nar/gks1219
Raikos, V., and Dassios, T. (2014). Health-promoting properties of bioactive peptides derived from milk proteins in infant food: A review. Dairy Sci. Technol. 94, 91–101. doi: 10.1007/s13594-013-0152-3
Rhyu, M.-R., and Kim, E.-Y. (2011). Umami taste characteristics of water extract of Doenjang, a Korean soybean paste: Low-molecular acidic peptides may be a possible clue to the taste. Food Chem. 127, 1210–1215. doi: 10.1016/j.foodchem.2011.01.128
Schrader, M., Schulz-Knappe, P., and Fricker, L. D. (2014). Historical perspective of peptidomics. EuPA Open Proteom. 3, 171–182. doi: 10.1016/j.euprot.2014.02.014
Shim, J., Son, H. J., Kim, Y., Kim, K. H., Kim, J. T., Moon, H., et al. (2015). Modulation of sweet taste by umami compounds via sweet taste receptor subunit hT1R2. PLoS One 10:e0124030. doi: 10.1371/journal.pone.0124030
Shin, J., Lee, S., Go, M.-J., Lee, S. Y., Kim, S. C., Lee, C.-H., et al. (2016). Analysis of the mouse gut microbiome using full-length 16S rRNA amplicon sequencing. Sci. Rep. 6:29681. doi: 10.1038/srep29681
Tan, G., Hu, M., Li, X., Pan, Z., Li, M., Li, L., et al. (2020). High-throughput sequencing and metabolomics reveal differences in bacterial diversity and metabolites between red and white sufu. Front. Microbiol. 11:758. doi: 10.3389/fmicb.2020.00758
Tedersoo, L., and Anslan, S. (2019). Towards PacBio-based pan-eukaryote metabarcoding using full-length ITS sequences. Environ. Microbiol. Rep. 11, 659–668. doi: 10.1111/1758-2229.12776
Thongsanit, J., Tanikawa, M., Yano, S., Tpachiki, T., and Wakayam, M. (2009). Identification of glutaminase-producing lactic acid bacteria isolated from Nham, a traditional Thai fermented food and characterisation of glutaminase activity of isolated Weissella cibaria. Ann. Microbiol. 59, 715–720. doi: 10.1007/BF03179213
Tran, N. H., Qiao, R., Xin, L., Chen, X., Liu, C., Zhang, X., et al. (2019). Deep learning enables de novo peptide sequencing from data-independent-acquisition mass spectrometry. Nat. Methods 16, 63–66. doi: 10.1038/s41592-018-0260-3
Udomsil, N., Rodtong, S., Choi, Y. J., Hua, Y., and Yongsawatdigul, J. (2011). Use of Tetragenococcus halophilus as a starter culture for flavor improvement in fish sauce fermentation. J. Agric. Food Chem. 59, 8401–8408. doi: 10.1021/jf201953v
Wang, W., Zhou, X., and Liu, Y. (2020). Characterization and evaluation of umami taste: A review. TrAC Trends Anal. Chem. 127:115876. doi: 10.1016/j.trac.2020.115876
Wei, G., Regenstein, J. M., and Zhou, P. (2021). The aroma profile and microbiota structure in oil furu, a Chinese fermented soybean curd. Food Res. Int. 147:110473. doi: 10.1016/j.foodres.2021.110473
White, T. J., Bruns, T., Lee, S., and Taylor, J. (1990). Amplification and direct sequencing of fungal ribosomal RNA genes for phylogenetics. PCR protoc. 18, 315–322. doi: 10.1016/B978-0-12-372180-8.50042-1
Xie, C., Zeng, H., Li, J., and Qin, L. (2018a). Comprehensive explorations of nutritional, functional and potential tasty components of various types of Sufu, a Chinese fermented soybean appetizer. Food Sci. Technol. 39, 105–114. doi: 10.1590/fst.37917
Xie, C., Zeng, H., Wang, C., Xu, Z., and Qin, L. (2018b). Volatile flavour components, microbiota and their correlations in different sufu, a Chinese fermented soybean food. J. Appl. Microbiol. 125, 1761–1773. doi: 10.1111/jam.14078
Xie, X., Dang, Y., Pan, D., Sun, Y., Zhou, C., He, J., et al. (2023). The enhancement and mechanism of the perception of saltiness by umami peptide from Ruditapes philippinarum and ham. Food Chem. 405:134886.
Xu, D., Wang, P., Zhang, X., Zhang, J., Sun, Y., Gao, L., et al. (2020). High-throughput sequencing approach to characterize dynamic changes of the fungal and bacterial communities during the production of sufu, a traditional Chinese fermented soybean food. Food Microbiol. 86:103340. doi: 10.1016/j.fm.2019.103340
Xu, N., Liu, Y., Hu, Y., Zhou, M., Wang, C., and Li, D. (2016). Autolysis of Aspergillus oryzae mycelium and effect on volatile flavor compounds of soy sauce. J. Food Sci. 81, C1883–C1890. doi: 10.1111/1750-3841.13396
Yamaguchi, S. (1967). The synergistic taste effect of monosodium glutamate and disodium 5’-inosinate. J. Food Sci. 32, 473–478. doi: 10.1111/j.1365-2621.1967.tb09715.x
Yamaguchi, S., and Ninomiya, K. (1998). What is umami? Food Rev. Int. 14, 123–138. doi: 10.1080/87559129809541155
Yoshida, Y. (1998). Umami taste and traditional seasonings. Food Rev. Int. 14, 213–246. doi: 10.1080/87559129809541158
Yu, X., Zhang, L., Miao, X., Li, Y., and Liu, Y. (2017). The structure features of umami hexapeptides for the T1R1/T1R3 receptor. Food Chem. 221, 599–605. doi: 10.1016/j.foodchem.2016.11.133
Zhang, J., Sun-Waterhouse, D., Su, G., and Zhao, M. (2019). New insight into umami receptor, umami/umami-enhancing peptides and their derivatives: A review. Trends Food Sci. Technol. 88, 429–438. doi: 10.1016/j.tifs.2019.04.008
Zhang, L., Zhang, L., and Xu, Y. (2020). Effects of Tetragenococcus halophilus and Candida versatilis on the production of aroma-active and umami-taste compounds during soy sauce fermentation. J. Sci. Food Agric. 100, 2782–2790. doi: 10.1002/jsfa.10310
Zhang, Y., Venkitasamy, C., Pan, Z., and Wang, W. (2013). Recent developments on umami ingredients of edible mushrooms–A review. Trends Food Sci. Technol. 33, 78–92. doi: 10.1016/j.tifs.2013.08.002
Zhang, Y., Venkitasamy, C., Pan, Z., Liu, W., and Zhao, L. (2017). Novel umami ingredients: Umami peptides and their taste. J. Food Sci. 82, 16–23.
Zhao, C. J., Schieber, A., and GäNZLE, M. G. (2016). Formation of taste-active amino acids, amino acid derivatives and peptides in food fermentations–A review. Food Res. Int. 89, 39–47. doi: 10.1016/j.foodres.2016.08.042
Zhao, Y., Zhang, M., Devahastin, S., and Liu, Y. (2019). Progresses on processing methods of umami substances: A review. Trends Food Sci. Technol. 93, 125–135. doi: 10.1016/j.tifs.2019.09.012
Zhu, W., Luan, H., Bu, Y., Li, J., Li, X., and Zhang, Y. (2021). Changes in taste substances during fermentation of fish sauce and the correlation with protease activity. Food Res. Int. 144:110349. doi: 10.1016/j.foodres.2021.110349
Keywords: sufu, solid-state fermentation, umami peptide, microbiome, interaction network
Citation: Mao J, Zhou Z and Yang H (2023) Microbial succession and its effect on the formation of umami peptides during sufu fermentation. Front. Microbiol. 14:1181588. doi: 10.3389/fmicb.2023.1181588
Received: 07 March 2023; Accepted: 30 March 2023;
Published: 17 April 2023.
Edited by:
Yu Ding, Jinan University, ChinaReviewed by:
Hai Yu, Yangzhou University, ChinaTingting Liang, Guangdong Academy of Sciences, China
Copyright © 2023 Mao, Zhou and Yang. This is an open-access article distributed under the terms of the Creative Commons Attribution License (CC BY). The use, distribution or reproduction in other forums is permitted, provided the original author(s) and the copyright owner(s) are credited and that the original publication in this journal is cited, in accordance with accepted academic practice. No use, distribution or reproduction is permitted which does not comply with these terms.
*Correspondence: Hongshun Yang, hongshunyang@hotmail.com