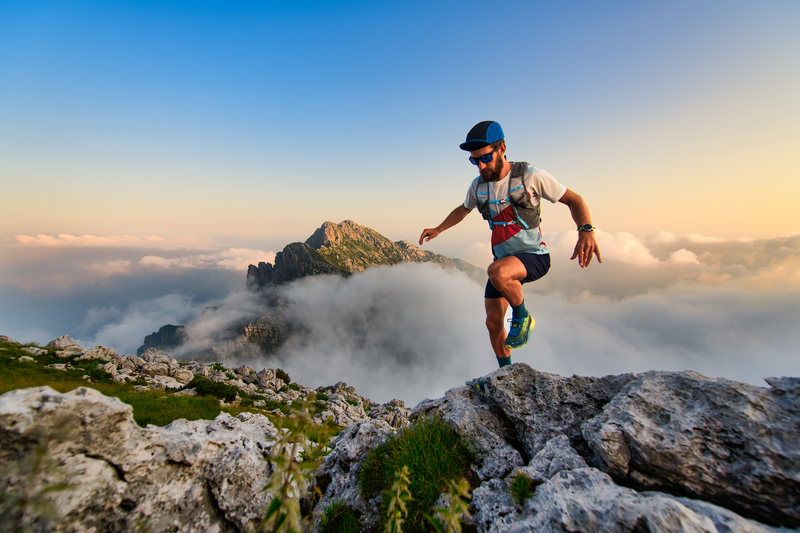
95% of researchers rate our articles as excellent or good
Learn more about the work of our research integrity team to safeguard the quality of each article we publish.
Find out more
ORIGINAL RESEARCH article
Front. Microbiol. , 25 May 2023
Sec. Microbe and Virus Interactions with Plants
Volume 14 - 2023 | https://doi.org/10.3389/fmicb.2023.1181245
This article is part of the Research Topic Plants surviving in extreme environment: Harnessing Soil-Plant–Microbial relationship to enhance crop health and productivity View all 21 articles
Introduction: Maize is the largest crop produced in China. With the growing population and the rapid development of urbanization and industrialization, maize has been recently cultivated in reclaimed barren mountainous lands in Zhejiang Province, China. However, the soil is usually not suitable for cultivation because of its low pH and poor nutrient conditions. To improve soil quality for crop growth, various fertilizers, including inorganic, organic, and microbial fertilizers, were used in the field. Among them, organic fertilizer-based sheep manure greatly improved the soil quality and has been widely adopted in reclaimed barren mountainous lands. But the mechanism of action was not well clear.
Methods: The field experiment (SMOF, COF, CCF and the control) was carried out on a reclaimed barren mountainous land in Dayang Village, Hangzhou City, Zhejiang Province, China. To systematically evaluate the effect of SMOF on reclaimed barren mountainous lands, soil properties, the root-zone microbial community structure, metabolites, and maize response were investigated.
Results: Compared with the control, SMOF could not significantly affect the soil pH but caused 46.10%, 28.28%, 101.94%, 56.35%, 79.07%, and 76.07% increases in the OMC, total N, available P, available K, MBC, and MBN, respectively. Based on 16S amplicon sequencing of soil bacteria, compared with the control, SMOF caused a 11.06–334.85% increase in the RA of Ohtaekwangia, Sphingomonas, unclassified_Sphingomonadaceae, and Saccharibacteria and a 11.91–38.60% reduction in the RA of Spartobacteria, Gemmatimonas, Gp4, Flavisolibacter, Subdivision3, Gp6, and unclassified_Betaproteobacteria, respectively. Moreover, based on ITS amplicon sequencing of soil fungi, SMOF also caused a 42.52–330.86% increase in the RA of Podospora, Clitopilus, Ascobolus, Mortierella, and Sordaria and a 20.98–64.46% reduction in the RA of Knufia, Fusarium, Verticillium, and Gibberella, respectively, compared with the control. RDA of microbial communities and soil properties revealed that the main variables of bacterial and fungal communities included available K, OMC, available P, MBN, and available K, pH, and MBC, respectively. In addition, LC-MS analysis indicated that 15 significant DEMs belonged to benzenoids, lipids, organoheterocyclic compounds, organic acids, phenylpropanoids, polyketides, and organic nitrogen compounds in SMOF and the control group, among which four DEMs were significantly correlated with two genera of bacteria and 10 DEMs were significantly correlated with five genera of fungi. The results revealed complicated interactions between microbes and DEMs in the soil of the maize root zone. Furthermore, the results of field experiments demonstrated that SMOF could cause a significant increase in maize ears and plant biomass.
Conclusions: Overall, the results of this study showed that the application of SMOF not only significantly modified the physical, chemical, and biological properties of reclaimed barren mountainous land but also promoted maize growth. SMOF can be used as a good amendment for maize production in reclaimed barren mountainous lands.
Maize (Zea mays L.) originated in the highlands of Mexico ~8,700 years ago (Piperno et al., 2009), is the second most plentiful crop globally (Ort and Long, 2014); it is also the largest crop produced in China (Gong et al., 2015). However, with the growing population and the rapid development of urbanization and industrialization, achieving grain supply security on limited arable land is a major challenge in contemporary times (Gong et al., 2015). In recent years, the barren mountainous areas were reclaimed for agricultural use in Zhejiang Province, China, to meet the demand for cultivated land (Wang et al., 2017). However, in most situations, reclaimed barren mountainous lands were not suitable for cultivation due to their acidic soil and nutrient-poor conditions (Li et al., 2023). Therefore, to develop the mountainous maize industry on reclaimed mountainous lands in Zhejiang Province, China, it is necessary to find effective measures to improve soil quality for the growth of maize.
In the last decades, to meet the requirements of crops, many farmers have resorted to using inorganic fertilizers (including commercial compound fertilizers, CCF). However, prolonged and excessive use of inorganic fertilizers has resulted in soil acidification, hardening, ecosystem degradation, environmental pollution, and so on (Lam et al., 2012; Hu et al., 2014; Roy et al., 2014; Gregorich et al., 2015; Yan et al., 2021). It is well-known that the quality of soil is affected by various factors (including physical, chemical, and biological properties). In the case of newly reclaimed lands, the quality of the soil can be greatly improved by increasing the use of organic fertilizers (such as crop residues, livestock manure, biogas liquid, and so on), which not only modify the physical and chemical properties of the soil but also have a great influence on the microbial communities (Li et al., 2021b, 2022a,b). For example, Li et al. (2021b) indicated that the soil quality was ameliorated by commercial organic fertilizers (COF), which caused a significant increase in pH, organic matter contents (OMC), available phosphorus (P), total nitrogen (N), alkaline hydrolysis N, total bacterial numbers, microbial biomass carbon (MBC), and a greatly increased height and total dry weight of maize seedlings in newly reclaimed lands compared with the control. Li et al. (2022b) showed that two kinds of COF, including PMMR-OF and SM-OF, not only significantly increased the weight of air-dried corn on newly reclaimed lands but also significantly changed the microbiota and chemical properties of corn soil. Li et al. (2022a) discovered that COF, SM, and MR not only significantly changed the pH of the soil but also increased the OMC considerably (in particular SM) and also caused a differential change in the bacterial and fungal community of the newly reclaimed lands.
Sheep manure organic fertilizer (SMOF) has recently been widely used on reclaimed barren mountainous lands in Hangzhou City, Zhejiang Province, China. Based on our previous research, organic fertilizer-based sheep manure had a beneficial effect on the growth of maize by improving the soil quality of newly reclaimed lands (Li et al., 2022b). However, the specific relationships among soil, microbes, and maize on reclaimed barren mountainous lands after applying SMOF were unclear. Thus, the aim of the study was to systematically evaluate the effect of SMOF on maize growth, root-zone soil properties, microbial community structure, and metabolites in reclaimed barren mountainous lands. In addition, we examined the correlation between soil properties, the microbial community structure, the correlation between differentially expressed metabolites (DEMs), and related microbes. This study provided a scientific basis for applying SMOF to promote maize cultivation on reclaimed barren mountainous lands in the future.
The field experiment was carried out on a reclaimed barren mountainous land in Dayang Village (29°25′36″N; 119°28′52″E; 200 m above sea level), Hangzhou City, Zhejiang Province, China. The soil type was acidic red with gravel, based on the soil classification system of the FAO-UNESCO, and the initial properties of the top 20 cm of soil were as follows: pH 5.94, OMC 1.49%, total N 0.98 g/kg, available P 50.64 mg/kg, and available K 144.85 mg/kg.
Three kinds of fertilizers were used in the field experiment: (1) sheep mature organic fertilizer (SMOF, mostly consisting of sheep manure, NPK ≥ 4%, OMC ≥ 30%) was provided by Hangzhou Nanwuzhuang Soil Fertilizer Co., Ltd. (Hangzhou, China); (2) commercial organic fertilizer (COF, mostly consisting of chicken manure and mushroom residues, NPK ≥ 4%, OMC ≥ 30%) was provided by Jiande Kairong Soil Fertilizer Co., Ltd. (Hangzhou, China); and (3) chemical compound fertilizer (CCF, N-P-K, 16-16-16, NPK = 48%, containing nitrate N and sulfate K) was provided by Shenzhen Batian Ecological Engineering Co., Ltd. (Shenzhen, China). The dosage of SMOF, COF, and CCF in this study was set according to the local farmers' habits. In detail, through the application of different fertilizers to the reclaimed barren mountainous land, the field experiment consisted of four different treatments: (1) SMOF at 1.50 kg/m2; (2) COF at 1.50 kg/m2; (3) CCF at 75 g/m2; and (4) the treatment without any fertilizer was applied as the control.
The field experiment was randomly designed and conducted from 6 April to 2 July 2022. The area of each plot was 24 m2 (length 7.5 m and width 3.2 m, respectively), and the planting density of maize was 35 × 50 cm. On 6 April, the top 0–20 cm of the soil of the experimental field was mixed with different fertilizers before planting, and then the seedlings of maize (cultivar “qianjiangtian 1”, provided by Hangzhou Academy of Agricultural Sciences, Hangzhou, China) were planted in the above-mentioned field. In detail, the maize seeds were placed into 50-cell seedling trays containing reclaimed barren mountainous land soil on 1 March and then kept at 25°C and 65−75% relative humidity for 5 weeks. Fresh maize ears and plants were harvested and measured after 87 days of planting. Each treatment had three replicates.
To evaluate the impact of SMOF on the biomass of maize in the reclaimed barren mountainous land, the fresh weight of maize ears and plants was measured using a digital scale (TCS-50, Shanghai Hento Industrial Co., Ltd., Shanghai, China) after 87 days of planting. Growth promotion efficacy (GPE%) was calculated using the following formula: GPE% = (treatment – control)/control × 100%.
Moreover, soil pH, OMC, total N, available P, available K, MBC, and microbial biomass nitrogen (MBN) were detected as previously described (Jackson, 1973; Brookes et al., 1985; Vance et al., 1987; Baran et al., 2019). In detail, when maize ears and plants from each plot were collected, ~1.0 kg of fresh root-zone soil (5–20 cm) was sampled simultaneously. After drying at room temperature and passing through a 0.45-mm sieve to remove fine roots and debris, the soil properties were measured. In brief, soil pH was measured at a soil/distilled water suspension ratio of 1:5 (g/mL) with a pH meter (FE28, Mettler-Toledo, Zurich, Switzerland); the content of organic matter was determined by the K2Cr2O7 oxidation external heating method; total N was determined using an automatic Kjeldahl distillation-titration unit; available P was determined by hydrochloric acid–ammonium fluoride extraction molybdenum–antimony anti–colorimetry; available K was extracted with 1 M ammonium acetate and determined by a flame photometer; MBC and MBN were determined using the chloroform fumigation extraction method. All the treatments had three replicates.
When maize ears and plants were collected on 2 July 2022, each plot sampled 20 g of maize root-zone soil and stored it at −80°C. DNA was extracted from the soil samples using the E.Z.N.ATM Mag–Bind Soil DNA Kit (OMEGA, Norcross, GA, USA) according to the manufacturer's protocols. The DNA quality was assessed using a Qubit® 3.0 fluorometer (ThermoFisher Scientific, USA).
The V3–V4 region of maize root-zone bacterial 16S rRNA genes and the ITS1 region of fungal ITS genes were amplified using the universal primers 341F (5′-CCT ACG GGN GGC WGC AG−3′) and 805R (5′-GAC TAC HVG GGT ATC TAA TCC−3′) (Wu et al., 2015), and ITS1F (5′-CTT GGT CAT TTA GGA AGT AA−3′) and ITS2 (5′-GCT GCG TTC TTC ATC GAT GC−3′) (Adams et al., 2013), respectively. The PCR assay volume was 30 μl, including 15 μl 2 × Hieff® Robust PCR Master Mix, 1 μl (10 μM) of each universal primer, 1 μl of DNA template, and 12 μl of ddH2O. The PCR thermal protocol generally consisted of an initial denaturation at 94°C for 3 min, 25 cycles of denaturation at 94°C for 30 s, annealing at 55°C for 30 s, extension at 72°C for 30 s, and a final extension at 72°C for 5 min. After the PCR amplicons were purified with Hieff NGS™ DNA selection beads (Yeasen, Shanghai, China), they were pooled in equal amounts, and pair-end (2 × 250 bp) sequencing was accomplished using the Illumina MiSeq™/Hiseq™ system (Sangon Biotechnology Co., Ltd., Shanghai, China).
The bioinformatics analysis of the microbe was conducted following the methodology used in our previous studies (Jiang et al., 2022; Li et al., 2023). In other words, to ensure high data quality, the raw data were preprocessed using PRINSEQ to remove low-quality reads (average quality score <20) (Schmieder and Edwards, 2011) and were merged using PEAR (v0.9.8) (Zhang et al., 2014). After the primers were trimmed with Cutadapt (v1.18) (Martin, 2011), clean reads were analyzed using Usearch with a 97% similarity cutoff to generate operational taxonomic units (OTUs) (Edgar, 2013, 2016). After selection of the representative read of each OTU using the QIIME package (v2020.06) (Caporaso et al., 2010), all 16S rDNA and ITS representative reads were annotated by blasting against the RDP database using the RDP classifier (Wang et al., 2007; Quast et al., 2013), and the UNITE database using BLAST (Altschul et al., 1997; Urmas et al., 2013), respectively.
Maize root-zone soil samples were collected on 2 July 2022, and 10 g of soil from each plot was stored at −80°C. After thawing at 4°C, 0.1 g of each soil sample was extracted in a 1 ML extract solution (methanol: H2O = 3:1, with the isotopically labeled internal standard mixture), homogenized at 35 Hz for 4 min, and sonicated for 5 min in an ice-water bath (repeat three times). Then, the samples were incubated at −40°C for 1 h, centrifuged at 4°C for 15 min (12,000 rpm), and the supernatant was transferred into a new glass vial for further analysis. Indeed, the liquid chromatography-mass spectrometry (LC-MS) analyses were performed using a UHPLC system (Vanquish, Thermo Fisher Scientific), and the conditions were set as follows: chromatographic column: waters ACQUITY UPLC HSS T3 column (1.8 μm, 2.1 mm × 100 mm); mobile phase A: 5 mM ammonium acetate and 5 mM acetic acid in the water; mobile phase B: acetonitrile; column temperature: 4°C, injection volume: 2 μl; gradient program: 0–0.5 min, 95% B; 0.5–7 min, 95% B; 7–8 min, 65−40% B; 8–9 min, 40% B; 9–9.1 min, 40−95% B; 9.1–12 min, 95% B. The ESI source conditions were set as follows: sheath gas flow rate, 50 Arb; aux gas flow rate, 15 Arb; capillary temperature, 320°C; full MS resolution, 60,000; MS/MS resolution, 15,000 collision energy as 10/30/60 in NCE mode; spray voltage, 3.8 kV (positive) or 3.4 kV (negative), respectively. The repeatability and reliability of the analysis process were evaluated by inserting one quality control (QC) sample, which was prepared by mixing an equal aliquot of the supernatants from all samples. All the treatments had six replicates. The Orbitrap Exploris 120 mass spectrometer (Orbitrap MS, Thermo) was used to acquire MS/MS spectra, and the obtained data in this study were converted to the mzXML format using ProteoWizard and processed with an in-house program, then an in-house MS2 database (SANGON) was applied for metabolite annotation.
The SPSS software (v16.0, SPSS Inc., Chicago, IL, USA) was used to perform one-way variance analysis (ANOVA). Origin software (v2023, Hampton, MA, USA) was employed for analyzing OTUs and alpha diversity indices (including Chao1, Shannon, and the Simpson index). The principal component analysis (PCA) based on beta diversity metrics from the Bray–Curtis metrics was utilized to observe the structural variation of root-zone soil microbes across samples used in this study (Ramette, 2007). The differences in root-zone soil microbes among different samples were tested using permutational multivariate ANOVA (PERMANOVA), with 999 permutations used to calculate p-values (Dixon, 2003). To further observe the differentially abundant microbes between groups, linear discriminant analysis effect size (LEfSe) was conducted to discover the differential biomarkers and estimate the biomarkers' effect size (Segata et al., 2011). To investigate the impact of different environmental factors (such as pH, OMC, total N, available P, available K, MBC, and MBN) on microbial community structure, redundancy discriminant analysis (RDA) was performed using Origin (v2022, Hampton, MA, USA). To investigate the impact of different fertilizers on microbial co-occurrence patterns, a Sparcc correlation coefficient among maize root-zone soil microbial communities was measured based on the relative abundance (RA) of OTUs at different treatments (SMOF, COF, CCF, and control). The high RA (>1%) and statistically significant correlations (p < 0.01, Sparcc's correlation N > 0.5 or <-0.5) among OTU levels were built into the network analysis. To observe the topology of the co-occurrence networks, the network graph analysis was performed based on these measurements, including average degree, modularity, nodes, and edges. To investigate the effect of SMOF on the metabolites, orthogonal projections to latent structures-discriminant analysis (OPLS-DA), volcano plots, heat maps, chord plots, and matchstick analyses on four different treatments were conducted with the MetaboAnalyst 4.0 platform. The screening thresholds for DEMs were set as variable importance in the projection (VIP) > 1 and p < 0.05. To investigate the association between DEMs and differential microbes in different treatment groups, a Spearman correlation coefficient among the high RA of maize root-zone soil microbes (top 40 bacteria or fungi at the genus level) and significant DEMs (the largest VIP value, p < 0.05) was measured by clustering a hot map (Hollander et al., 2008).
To evaluate the impacts of SMOF on maize production in the reclaimed barren mountainous land, the fresh weight of maize ears and plants was measured after 87 days of planting when harvested. The results showed that SMOF significantly promoted the growth of maize under field conditions (Table 1). Compared to the control, SMOF caused a 12.29 and 10.53% increase in the fresh weight of maize ears and plants, respectively, while COF caused a 9.72 and 21.57% increase, and CCF resulted in a 0.83% reduction and 15.74% increase, respectively. Furthermore, the fresh weight of maize ears was found to be 1.13-fold higher in the SMOF treatment, while in the COF treatment, it was 1.11-fold higher compared to the CCF treatment. These results suggest that SMOF had a greater effect on promoting the growth of maize ears compared to the other treatments (including COF, CCF, and the control).
The results indicated that there was no significant difference in the pH of the soil between SMOF and the control, but SMOF significantly increased the OMC, total N, available P, available K, MBC, and MBN (Table 2). In other words, compared with the control, SMOF caused a 0.50% increase, while COF and CCF caused a 1.67 and 7.18% reduction in the soil pH; the soil OMC was significantly increased by 46.10%, 26.24%, and 40.43% by SMOF, COF, and CCF, respectively; SMOF, COF, and CCF caused a significant increase by 28.28%, 20.20%, and 26.26% in total N, respectively; the content of available P and available K was significantly increased by SMOF, COF, and CCF, with a 101.94%, 67.49%, 53.51%, and 56.35%, 20.80%, and 31.80% increase, respectively. In addition, compared to the control, the MBC and MBN values were unaffected by CCF but significantly increased by SMOF and COF, with 79.09%, 66.01% (for MBC), and 76.07%, 63.81% (for MBN) increases, respectively.
The original microbial sequence data of all soil samples from four different treatments (SMOF, COF, CCF, and the control) were quality-controlled, and a total of 2,109,642 high-quality 16S rRNA gene sequences were obtained from the high-throughput amplicon sequencing. Among them, the high-quality sequences of each sample range from 154,878 to 193,807. A total of 92,480 OTUs from 13 bacterial phyla were identified, and the distribution of OTUs across all four treatments is shown in Figure 1A. Compared with the control, the number of bacterial OTUs was significantly increased by SMOF, COF, and CCF, with a 11.03%, 4.68%, and 6.50% increase, respectively. Similarly, after the raw data were quality-controlled, a total of 4,417,896 high-quality ITS gene sequences were obtained from all soil samples from the four treatments. Among them, the high-quality sequences of each sample range from 215,206 to 475,487. A total of 14,768 OTUs from six fungal phyla were identified, and the distribution of OTUs across all four treatments is shown in Figure 1B. The fungal OTU number was slightly increased (1.70%, 0.67%, and 8.31%, respectively) by SMOF, COF, and CCF compared with the control. Overall, the average bacterial OTU number was 6.65-, 6.33-, 5.99-, and 6.09-fold greater than that of fungi in SMOF, COF, CCF, and the control, respectively. Compared to the control, SMOF caused a greater ratio of bacterial to fungal OTU distribution.
Figure 1. Impacts of SMOF on the OTU distribution, Chao1 richness index, Shannon's diversity index, and Simpson's diversity index of microbes in maize root-zone soil. (A) Bacteria, (B) fungi. Different lowercase letters above columns indicate statistical differences (p < 0.05).
The RA of the top 10 bacterial classes (Figure 2A) and the whole eight fungal classes (Figure 2C) were observed across all the maize soil samples. Moreover, the top 10 bacterial genera (Figure 2B) and fungal genera (Figure 2D) with an average RA > 1% were highlighted across all the maize soil samples. The results showed that the application of SMOF resulted in significant changes in the microbial community composition of maize root-zone soil in the reclaimed barren mountainous land at the class and genus levels compared to the control. In other words, compared with the control, SMOF significantly increased the RA of Cytophagia (153.37%), Gammaproteobacteria (39.71%), Bacilli (37.97%), Deltaproteobacteria (12.47%), Alphaproteobacteria (12.20%), and Saccharibacteria (11.06%) while also reducing the RA of Anaerolineae (66.20%), Spartobacteria (35.67%), Gemmatimonadetes (30.65%), Acidobacteria_Gp4 (22.46%), Subdivision3 (18.35%), and Acidobacteria_Gp6 (14.27%) at the class level of bacteria. Furthermore, SMOF significantly increased the RA of Ohtaekwangia (334.85%), Sphingomonas (54.90%), unclassified_Sphingomonadaceae (17.76%), and Saccharibacteria (11.06%) while reducing the RA of Spartobacteria (38.60%), Gemmatimonas (30.65%), Gp4 (27.99%), Flavisolibacter (20.17%), Subdivision3 (18.58%), Gp6 (14.27%), and unclassified_Betaproteobacteria (11.91%) at the genus level of bacteria. Similarly, compared with the control, SMOF significantly increased the RA of Mortierellomycetes (70.79%), Sordariomycetes (21.02%), Agaricomycetes (13.93%), and Pezizomycetes (11.19%) while reducing the RA of Rozellomycotina (82.16%), Eurotiomycetes (63.72%), Dothideomycetes (48.33%), and Tremellomycetes (15.41%) at the class level of the fungus. Additionally, SMOF significantly increased the RA of Podospora (330.86%), Clitopilus (86.46%), Ascobolus (85.81%), Mortierella (74.15%), and Sordaria (42.52%) and also reduced the RA of Knufia (64.46%), Fusarium (42.84%), Verticillium (36.22%), and Gibberella (20.98%) at the genus level of the fungus. All these results indicated that the changes in the number of specific bacteria and fungi in different treatments might be due to the different nutrients under different fertilizer conditions, and different fertilizers, including SMOF, could affect the abundance of bacteria and fungi in the root-zone soil of maize to regulate the composition of the microbial community.
Figure 2. Relative abundance (RA) of the top 10 dominant bacterial (A, B) and fungal (C, D) classes and genera in four different treatments, respectively.
The alpha diversity analysis evaluated microbial species richness and diversity within each maize soil sample based on three indices, including Chao1, Shannon, and Simpson (Figure 1). Compared with the control, the bacterial Chao1 index was significantly increased by SMOF (7.91%) and CCF (5.60%), but no significant change was observed in the Shannon and Simpson index of bacterial community among all four different treatments. Moreover, no significant difference was found in the Chao1, Shannon, and Simpson index of fungal community among all four treatments. The results revealed that the bacterial Chao1 index was 5.60-, 5.83-, 5.77-, and 5.65-fold, and the bacterial Shannon index was 1.95-, 1.83-, 1.81-, and 1.78-fold greater than that of fungi in SMOF, COF, CCF, and the control, respectively. The bacterial Simpson index was 0.06-, 0.08-, 0.07-, and 0.05-fold less than fungi in SMOF, COF, CCF, and the control, respectively. Compared to the control, SMOF caused a greater ratio of bacterial and fungal diversity index (Shannon index), but the microbial richness index (Chao1 index) and diversity index (Simpson index) were differentially affected by different treatments. Overall, SMOF could significantly increase the microbial richness of maize root-zone soil in the reclaimed barren mountainous land.
To further study SMOF on maize root-zone microbial communities, principal component analysis (PCA) at the OTU level based on the Bray–Curtis distance was performed (Figure 3). The PCA analysis revealed that the soil root-zone bacterial communities of SMOF, COF, CCF, and the control formed four different groups, and SMOF was well separated from the other three treatments. The first principal component (PCA1) revealed 15.63% of the variability in the bacterial community, and the second principal component (PCA2) explained 12.11%. A PERMANOVA on samples of all four treatments also showed that different fertilizers explained 19.3% of the variation (p = 0.026) (Figure 3A). In addition, the PCA analysis of fungal community structure indicated that all samples from four treatments were divided into four groups. However, there was an overlap among all four treatments (Figure 3B). PCA1 and PCA2 explained 15.29 and 13.01% of the total variation in the fungal community, respectively. The bacterial community structure of maize root-zone soil was significantly changed by SMOF, but no significant change was observed in fungal community structure diversity.
Figure 3. Principal component analysis (PCA) of the maize root-zone bacterial (A) and fungal (B) communities based on OTU abundance. Ellipses have been drawn for each treatment with a confidence limit of 0.95.
Linear discriminant analysis (LDA) effect size (LEfSe) was used to identify the biomarkers with significant differences between the maize root-zone soil microbial communities under SMOF, COF, CCF, and the control conditions (Figure 4). Results showed that 11 bacterial biomarkers were found in SMOF, CCF, and the control (Figure 4A). In other words, SMOF was enriched with two types of Sphingomonadales. CCF was enriched with Opitutus, Opitutaceae, Opitutales, and Opitutae. The control was enriched with Pseudonocardiaceae, Microvirga, Methylobacteriaceae, Methylobacillus, and Frateuria. In addition, a total of 15 fungal biomarkers were obtained in SMOF, COF, CCF, and the control (Figure 4B). Specifically, SMOF was enriched with Ascobolus and Podospora, COF was enriched with two types of Cephalotrichum, two types of Dipodascus, Entoloma, and two types of Dendrosporium, CCF was enriched with Claroideoglomus, Thanatephorus, Psedophialophora, and Schizothecium, and the control was enriched with Penicillium and Herpotrichiellaceae.
Figure 4. Linear discriminant analysis (LDA) effect size (LEfSe) of the bacterial (A) and fungal (B) taxa, which identifies the most differentially abundant taxa among SMOF, COF, CCF, and the control in different treatments. Only bacterial taxa with LDA values greater than two and fungal taxa with LDA values >2.5 (p < 0.05) are shown.
Furthermore, the difference in RA composition (class and genus level) of the root-zone microbial community under SMOF, COF, CCF, and the control was visually explained through heat maps (Figure 5). At the class level of bacteria, SMOF was enriched with Gammaproteobacteria, Cytophagia, Deltaproteobacteria, and Alphaproteobacteria but was reduced with Spartobacteria, Anaerolineae, and Gemmatimonadetes (p < 0.05); COF was enriched with Acidobacteria_Gp1, Acidobacteria_Gp3, Saccharibacteria, but was reduced with Spartobacteria, and Anaerolineae (p < 0.05); CCF was enriched with Acidobacteria_Gp2, Planctomycetia, and Bacilli but was reduced with Sphingobacteriia and Acidobacteria_Gp6 (p < 0.05). At the genus level of bacteria, SMOF was enriched with Sphingomonas, Ohtaekwangia, and unclassified_Alphaproteobacteria but was reduced with Spartobacteria and Gemmatimonas (p < 0.05); COF was enriched with Gp1, Gp3, unclassified_Rhizobiales, and Saccharibacteria but was reduced with WPS-1 (p < 0.05); CCF was enriched with Gp2 and Gp4 but was reduced with unclassified_Chitinophagaceae, Gp6, and Flavisolibacter (p < 0.05). Furthermore, at the class level of the fungus, SMOF was enriched with Sordariomycetes and Mortierellomycetes but was reduced with Dothideomycetes, Eurotiomycetes, and Rozellomycotina (p < 0.05); COF was enriched with Tremellomycetes but was reduced with Pezizomycetes (p < 0.05); CCF was enriched with Agaricomycetes but was reduced with Pezizomycetes (p < 0.05). At the genus level of the fungus, SMOF was enriched with Podospora, Cristinia, Sordaria, and Mortierella but was reduced with Leptosphaeria, Knufia, and Fusarium (p < 0.05); COF was enriched with Aspergillus but was reduced with Pulvinula and Sodiomyces (p < 0.05); CCF was enriched with Clitopilus, Gibberella, and Trichoderma but was reduced with Chaetomium and Zopfiella (p < 0.05). The results demonstrated that different fertilizer treatments, such as SMOF, COF, and CCF, had an impact on the presence or absence of specific microbial species, ultimately altering the community structure of the maize root-zone soil.
Figure 5. Hierarchical clustering analysis and heat maps of dominant bacterial and fungal community abundance at class (A, B) and genus (C, D) levels, respectively. The tree plot represents a clustering analysis of the dominant bacteria and fungi at class and genus levels according to their Pearson correlation coefficient matrix and relative abundance, and the upper tree plot represents a clustering analysis of soil samples according to the Euclidean distance of the data, respectively.
Redundancy discriminant analysis (RDA) was used to examine the correlation between environmental factors and microbial communities in maize root-zone soil (Figure 6; Table 3). The results showed that soil properties influenced the composition of microbial communities at the genus level. In addition, 53.42 and 47.40% of the cumulative variance of the root-zone microbial community-factor correction occurred at the bacterial (Figure 6A) and fungal (Figure 6B) genus levels, respectively. The contributions of the four main variables, available K, OMC, available P, and MBN, explained 25.64%, 25.36%, 25.15%, and 23.38% of the bacterial community at the genus level, respectively, while the contributions of the three main variables, available K, pH, and MBC, explained as 32.96%, 23.49%, and 22.67% of the fungal community at the genus level, respectively. All those suggested that available K, OMC, available P, MBN, and available K, pH, MBC were the main factors influencing the bacterial and fungal communities, respectively (Table 3). The results also showed a complex relationship between microbial growth and soil nutrient elements because different soil properties significantly affected microbial community compositions in maize root-zone soil.
Figure 6. Redundancy discriminant analysis (RDA) of the root-zone microbial community compositions at genus levels with soil properties. (A) Bacteria. Gem, Gemmatimonas; Sph, Sphingomonas; Sub, Subdivision3; Ter, Terrimonas. (B) Fungi. Asc, Ascobolus; Cha, Chaetomium; Cli, Clitopilus; Fus, Fusarium; Gib, Gibberella; Knu, Knufia; Mor, Mortierella; Pod, Podospora; Pre, Preussia; Sor, Sordaria. OMC, organic matter contains; TN, total N; AP, available P; AK, available K; MBC, microbial biomass carbon; MBN, microbial biomass nitrogen. Arrows indicate the direction and magnitude of soil properties (pH, OMC, total N, AP, AK, MBC, and MBN) associated with the different bacterial and fungal genera.
Co-occurrence networks were constructed to explore the complexity of connections between maize root-zone soil microbial communities in SMOF, COF, CCF and the control. Moreover, the topological properties of the co-occurrence network were calculated to characterize the differences between different treatments (Figure 7). The bacterial network of SMOF consisted of 175 nodes and 205 edges (97 positive edges, 108 negative edges, and an average degree of 2.343), with modularity of −16.046. The COF, CCF, and control were 175 (positive: 71, negative: 104, average degree 2.500), 79 (positive: 79, negative: 0, average degree 1.756), and 225 (positive: 101, negative: 124, average degree 2.885), consisting of edges and 140, 90, and 156 nodes, respectively, and the modularity was −2.578, 0.798, and −6.410, respectively. Moreover, the fungal network of SMOF consisted of 125 nodes and 207 edges (103 positive edges, 104 negative edges, and an average degree of 3.312), with a modularity of −320.674. The COF, CCF, and control were 189 (positive: 90, negative: 99, average degree 2.953), 205 (positive: 113, negative: 92, average degree 2.929), and 217 (positive: 103, negative: 114, average degree 3.417), consisting of edges and 128, 140, and 127 nodes, respectively, and the modularity was −144.934, 3.317, and 1,311.843, respectively.
Figure 7. Impacts of SMOF on the co-occurrence patterns of soil bacterial (A) and fungal (B) communities. Networks were constructed at the OTU level. The size of the nodes (OTUs) represented the relative abundance (RA) of the microbe, and the nodes were colored according to phylum. The red and green lines represented positive and negative correlations, respectively.
A total of 10,514 peaks were screened in maize root-zone soil from four different treatments, among which 277 metabolites were identified using LC-MS analysis. Furthermore, a score map of metabolites was constructed using OPLS-DA (Figures 8A–C; Table 4). Results showed that the distribution of different treatments could be effectively separated between SMOF, COF, CCF, and the control. Figure 8A presents the sampling distributions of SMOF treatment and the control in the positive and negative areas of t[1]P, respectively, while the model values of SMOF and the control were R2X(cum) = 0.431, R2Y(cum) = 0.990, and Q2(cum) = 0.306. Similarly, Figure 8B presents the sampling distributions of COF treatment and the control in the positive and negative areas of t[1]P, respectively, while the model values of COF and the control were R2X(cum) = 0.364, R2Y(cum) = 0.987, and Q2(cum) = 0.269. Meanwhile, Figure 8C presents the sampling distributions of CCF treatment and the control in the positive and negative areas of t[1]P, respectively, while the model values of CCF and the control were R2X(cum) = 0.310, R2Y(cum) = 0.990, and Q2(cum) = 0.519. In view of the obvious separation of samples, it could be inferred that the metabolites in control root-zone soil were significantly changed by the application of the SMOF, COF, and CCF. Furthermore, the volcano plot (based on VIP > 1 and p < 0.05) also showed that the metabolites in the SMOF, COF, and CCF treatments were significantly different from the control (Figures 8D–F).
Figure 8. Orthogonal projections to latent structures-discriminant analysis (OPLS-DA) score map of maize root-zone soil of the SMOF (A), COF (B), and CCF (C) treatment. Volcano plot of differentially accumulated metabolites in SMOF vs. the control (D), COF vs. the control (E), and CCF vs. the control (F). Each point represents a metabolite with VIP > 1 and p < 0.05. A blue point indicates metabolites that are downregulated, a red point indicates metabolites that are upregulated, and a gray point indicates non-significant metabolites.
All the 277 metabolites mainly refer to lipids and lipid-like molecules (38.99%), organoheterocyclic compounds (14.44%), benzenoids (13%), organic acids and derivatives (9.75%), organic oxygen compounds (9.39%), organic nitrogen compounds (5.05%), and so on (Figure 9A). The results showed differences between the DEMs' numbers of SMOF, COF, or CCF and the control (Figures 9C–E). Indeed, there were 1,221 DEMs in the groups of SMOF and the control, with 1,107 upregulated and 114 downregulated (Figure 9C); 601 DEMs in the group of COF and the control, with 380 upregulated and 221 downregulated (Figure 9D); and 1,241 DEMs in the group of CCF and the control, with 854 upregulated and 387 downregulated (Figure 9E). Furthermore, to observe the change rules for metabolites, the metabolites with significant differences were normalized and shown in the hierarchical clustering heat map (Figure 10). Indeed, compared with the control, 34 DEMs (29 increased by 15.43−132.11%, five decreased by 16.91−73.92%), 12 DEMs (six increased by 14.28−61.68%, six decreased by 25.21−65.13%), and 29 DEMs (20 increased by 5.91−160.39%, nine decreased by 25.51−81.17%) were significantly changed by SMOF, COF, and CCF, respectively (Figures 9B, 10; Table 5).
Figure 9. Donut plot of metabolite classification and proportion (A), Venn analysis for three groups (B). The number of differentially expressed metabolites (DEMs) in the groups of SMOF and the control (C), COF and the control (D), and CCF and the control (E).
Figure 10. Heat map of hierarchical clustering analysis for groups SMOF, COF, or CCF vs. the control. The tree plot represents a clustering analysis of the differentially expressed metabolites (DEMs) significantly changed by SMOF, COF, or CCF according to their Person correlation coefficient matrix and relative abundance.
Table 5. A list of differentially expressed metabolites (DEMs) by relative content, VIP, FC, and a p-value.
The results also showed that three DEMs [tetramethylpyrazine, (3beta,5beta,8beta,22E,24xi)-Ergosta-6,22-diene-3,5,8-triol, daucol] were common metabolites in all three treatment groups. One DEM (N-Ethylglycine) was a common metabolite in the groups of SMOF or COF and the control; 11 DEMs [(10E,12Z)-(9S)-9-Hydroperoxyoctadeca-10,12-dienoic acid, scopolamine, sphingosine, stearidonic acid, (E)-3-(2-Hydroxyphenyl)-2-propenal, N-Ethylacetamide, docosapentaenoic acid (22n-6), (R)-Apiumetin glucoside, 9-HOTE, estriol-16-glucuronide, and 2-(Methylamino)benzoic acid] were common metabolites in the groups of SMOF or CCF and the control; four DEMs (betaine, cholesterol sulfate, PC(20:5(5Z,8Z,11Z,14Z,17Z)/18:4(6Z,9Z,12Z,15Z)), 24-Methylenecholesterol) were common metabolites in the group of COF or CCF and the control. Nineteen DEMs (rotenone, taraxacoside, 8,15-DiHETE, xi-2,3-Dihydro-3,5-dihydroxy-6-methyl-4H-pyran-4-one, morpholine, Na,Na-Dimethylhistamine, hypogeic acid, 2-Pyrrolidinone, triethylamine, D-lactic acid, cytosine, nortriptyline, prostaglandin E2, edulan I, phenylethylamine, S-Nitrosoglutathione, N-[(4-Hydroxy-3-methoxyphenyl)methyl]octanamide, falcarinone, and [2,2-Bis(2-methylpropoxy)ethyl]benzene) were unique metabolites in the groups of SMOF and the control; four DEMs (3-Hydroxybutyric acid, oleamide, (±)-(Z)-2-(5-Tetradecenyl)cyclobutanone, allodesmosine) were unique metabolites in the group of COF and the control; and 11 DEMs (1-Butylamine, 25-Hydroxyvitamin D2, cloversaponin I, 5,7alpha-Dihydro-1,4,4,7a-tetramethyl-4H-indene, histamine, 4-Aminobutyraldehyde, carvyl propionate, PE(P-16:0e/16:0), 5,8,11-Eicosatrienoic acid, 5,7-Dihydro-2-methylthieno[3,4-d]pyrimidine, and annosquamosin B) were unique metabolites in the group of CCF and the control. All those results indicated that these metabolites might play a crucial role in the response of maize to SMOF, COF, or CCF (Figures 9B, 10; Table 5).
Furthermore, chord plot analysis and matchstick analysis were used to visualize the enrichment analysis of these DEMs in three different treatment groups (SMOF, COF, or CCF, and the control) (Figure 11). The results showed that different metabolites were mostly related to each other. Some were positive correlations, whereas some were negative correlations (Figures 11A, C, E). In detail, there were 10 DEMs significantly upregulated and five DEMs significantly downregulated in the groups of SMOF and the control (Figure 11B); six DEMs significantly upregulated, and the other six DEMs significantly downregulated in the group of COF and the control (Figure 11D); 10 DEMs significantly upregulated and nine DEMs significantly downregulated in the group of CCF and the control (Figure 11F). More attention should be paid to those significant DEMs.
Figure 11. Chord plot analysis for the groups of SMOF and the control (A), COF and the control (C), CCF and the control (E), respectively. Nodes represent variables; text color is associated with different metabolites, and chords represent correlations. Matchstick analysis for the groups of SMOF and the control (B), COF and the control (D), and CCF and the control (F), respectively. The color of the dot represents the size of the VIP value, *represents 0.01 < p < 0.05, **represents 0.001 < p < 0.01, and ***represents p < 0.001.
To further analyze correlative relationships between microbes and metabolites, those metabolites with significant differences were normalized, and the clustering heat map was drawn (Figure 12). The results indicated that in the groups of SMOF and the control, four DEMs (N-[(4-Hydroxy-3-methoxyphenyl)methyl]octanamide, tetramethylpyrazine, Dimethylhistamine, and Nitrosoglutathione) were significantly correlated with two genera of bacteria (Saccharibacteria, unclassified_Rhizobiales); meanwhile, 10 DEMs (edulan I, stearidonic acid, (10E,12Z)-(9S)-9-Hydroperoxyoctadeca-10,12-dienoic acid, rotenone, (3beta,5beta,8beta,22E,24xi)-Ergosta-6,22-diene-3,5,8-triol, S-Nitrosoglutathione, 2-Pyrrolidinone, tetramethylpyrazine, [2,2-Bis(2-methylpropoxy)ethyl]benzene, and hypogeic acid) were significantly correlated with five genera of fungi (Cheilymenia, Paraphoma, Sodiomyces, Dipodascus, and Cephalotrichum). In the group of COF and the control, two DEMs (PC(20:5(5Z,8Z,11Z,14Z,17Z)/18:4(6Z,9Z,12Z,15Z)), and 24-Methylenecholesterol) were significantly correlated with one genus of bacteria (Rhodopseudomonas). Meanwhile, 12 DEMs [3-Hydroxybutyric acid, betaine, cholesterol sulfate, (±)-(Z)-2-(5-Tetradecenyl)cyclobutanone, Allodesmosine, (3beta,5beta,8beta,22E,24xi)-Ergosta-6,22-diene-3,5,8-triol, oleamide, 24-Methylenecholestero, PC(20:5(5Z,8Z,11Z,14Z,17Z)/18:4(6Z,9Z,12Z,15Z)), tetramethylpyrazine, daucol, and N-Ethylglycine] were significantly correlated with eight genera of fungi (Gibberella, Scutellinia, Bisifusarium, Didymella, Cephalotrichum, Dipodascus, unclassified_Pyronemataceae, and Zopfiella). In the group of CCF and the control, 13 DEMs [(R)-Apiumetin glucoside, 9-HOTE, stearidonic acid, 25-Hydroxyvitamin D2, (10E,12Z)-(9S)-9-Hydroperoxyoctadeca-10,12-dienoic acid, cholesterol sulfate, 5,8,11-eicosatrienoic acid, carvyl propionate, daucol, tetramethylpyrazine, PC(20:5(5Z,8Z,11Z,14Z,17Z)/18:4(6Z,9Z,12Z,15Z)), 24-Methylenecholesterol, and annosquamosin B] were significantly correlated with nine genera of bacteria (Adhaeribacter, Lysobacter, Ohtaekwangia, Micromonospora, Rhodopseudomonas, unclassified_Bacteria, Saccharibacteria, Subdivision3, and Gemmatimonas); meanwhile, 16 DEMs [25-Hydroxyvitamin D2, PE(P-16:0e/16:0), (10E,12Z)-(9S)-9-Hydroperoxyoctadeca-10,12-dienoic acid, cholesterol sulfate, 9-HOTE, 2-(Methylamino)benzoic acid, cloversaponin I, (R)-Apiumetin glucoside, 5,8,11-eicosatrienoic acid, carvyl propionate, betaine, annosquamosin B, tetramethylpyrazine, daucol, estriol-16-Glucuronide, and (3beta,5beta,8beta,22E,24xi)-Ergosta-6,22-diene-3,5,8-triol] were significantly correlated with nine genera of fungi (Cladorrhinum, Paraphoma, Podospora, Cheilymenia, Sodiomyces, Trichoderma, Fusarium, Cephalotrichum, and Dipodascus). Taken overall, the DEMs (organic acids, organoheterocyclic compounds, lipids, and secondary metabolites) produced by the application of different fertilizers (including SMOF) in the root-zone soil of maize may enrich the soil microbe and help coordinate the root-zone microbe.
Figure 12. Correlation heat map between DEMs and the related microbe in different treatment groups. Based on the top 40 genera of bacteria: SMOF vs. the control (A), COF vs. the control (C), and CCF vs. the control (E); based on the top 40 genera of fungi: SMOF vs. the control (B), COF vs. the control (D), and CCF vs. the control (F). *Indicated a significant correlation at p < 0.05, **indicated a significant correlation at p < 0.01.
Cultivated land is the lifeblood and main carrier of food production (Zhou et al., 2021). Cultivated land security is an important guarantee and foundation for food security, social stability, and sustainable development (Zhou et al., 2021). However, with considerable economic development and population growth, various cities have been undergoing rapid urbanization (Zhao, 2016). As the world's most populous country, cultivated lands are occupied on a massive scale in the urban expansion process in China (Tan et al., 2005). Land reclamation is regarded as a viable option to balance occupation and compensation (Li et al., 2023). This practice has been widely adopted worldwide to accommodate urbanization and economic development, especially in developing countries such as China (Hu et al., 2021). However, the soil of reclaimed land usually cannot meet the growth requirements of plants due to poor soil fertility, low soil pH, and high gravel content (Li et al., 2021b).
In most cases, the production capacity of reclaimed land is only 10−30% of the occupied cultivated land in China (Wang et al., 2017), and it often needs 7–10 years of fertilization to reach the level of occupied cultivated land, including acidification adjustment, returning straw to the field, planting green manure, and comprehensive utilization of COF and animal manure (Rendana et al., 2018; Teixeira et al., 2019; Li et al., 2021b). In other words, the content of soil organic matter is highly associated with soil suitability, and the quality of new reclamation land can be effectively improved by increasing the use of organic fertilizers. Indeed, after decades of practice, organic fertilizers have been recognized as valuable soil improvers that could not only improve the physical, chemical, and biological properties of the soil but also increase crop yields (Koocheki and Seyyedi, 2015; Yarami and Sepaskhah, 2015; Qiu et al., 2016; Li et al., 2021b; Yan et al., 2021). In our previous studies, considering the massive consumption in agriculture production, mushroom residue, biogas liquid, vegetable cake, COF, PMMR-OF (organic fertilizer-based pig manure and mushroom residue), and SM-OF (organic fertilizer-based sheep manure) were selected to be applied to new reclamation land, and the results showed that all these organic fertilizers, especially SM-OF, could not only effectively improve the soil quality of new reclamation land by increasing the soil organic matter but also have a greater effect on sweet potato and corn growth compared to the control (Li et al., 2021b, 2022a,b). According to Therios (1996), animal manures differ in nutrient content. It is common knowledge that sheep manure is referred to as cold manure because of its low nitrogen (N) content, and it is also known as a natural slow-release fertilizer with high phosphorus (P), potassium (K), and other essential elements for plant growth. Recently, sheep manure organic fertilizer (SMOF) has been widely used in the agriculture industry on reclaimed barren mountainous land in Dayang Village, Hangzhou City, Zhejiang Province, China. However, the systematically evaluated SMOF on reclaimed barren mountainous land soil properties, root-zone microbial community structure, metabolites, and maize response is very limited.
In this study, SMOF generally significantly promoted maize growth compared to CCF and the control. Moreover, some previous studies also reported that the use of sheep manure could improve the production of plants. For example, El Gammal and Salama (2016) showed that increasing the sheep manure application rate could induce a progressive enhancement of guava trees' growth and fruit set. Amanullah et al. (2019) revealed that the application of N in the form of 50% urea and 50% organic sources (including sheep manure) could significantly increase total rice biomass. Li et al. (2022a) reported that the application of sheep manure resulted in a significantly positive effect on sweet potato production on the new reclamation land.
The soil properties in maize fields were differentially affected by different treatments (SMOF, COF, CCF, and the control), and the effects differed depending on the soil parameters and types of fertilizer. In detail, the soil pH was slightly increased by SMOF but significantly decreased by CCF. A similar observation was observed by Han et al. (2016), which may be due to the H+ released into the soil after used by the plants (Magdoff et al., 1997); the leaching of basic cations such as magnesium (Mg), calcium (Ca), and potassium (K) from the soil (Han et al., 2016); and the proportion of soil microaggregates (<0.25 mm) decreased by the widespread use of CCF (Li et al., 2023). Huang et al. (2009) reported that the soil OMC and total N were widely used as the main parameters for evaluating soil fertility because of their heterogeneous mixtures of organic substances. In our study, the soil OMC and total N were significantly increased by SMOF, COF, and CCF compared with the control. Similar results were obtained by Dong et al. (2012), which may be because the application of organic and chemical fertilizers can promote plant growth and thus return more root residues to the soil (Hyvönen et al., 2008); organic and chemical fertilizers are beneficial to the accumulation of soil organic matter and thus improve the soil's fertility (Dong et al., 2012). The results also showed that available soil P and K contents were significantly affected by different fertilizer treatments (SMOF, COF, and CCF). In particular, SMOF, COF, and CCF led to significantly higher values of soil available P (99.94, 82.89, and 75.97 mg/kg, respectively) and available K (229.68, 177.45, and 193.62 mg/kg, respectively) than the control (49.49, 146.90 mg/kg, respectively). Previous studies have also shown that the application of manure could significantly increase available P (Huang et al., 2010), while available K was the highest in NPK treatments (Dong et al., 2012). In our study, available K was higher in the SMOF treatment than in the other treatments (including CCF), which may be due to the higher concentration of K+ in sheep manure (Zhang et al., 2015; Alhrout et al., 2018). Furthermore, MBC and MBN were significantly increased by SMOF and COF compared with the control, but there was no significant difference between CCF and the control. Soil microbial biomass is widely regarded as an important ecological indicator of changes in soil quality and acts as a source of nutrients available for plant uptake and growth (Haripal and Sahoo, 2014; Li et al., 2016). Overall, it can be inferred that SMOF is potentially an effective fertilizer to improve the quality of reclaimed barren mountainous land soil.
Microbial communities inhabiting the maize root zone of three fertilizer treatments and the control were examined using 16S rRNA and ITS gene high-throughput sequencing. We used the number of OTUs, the RA of the top 10 classes, and genera to measure the bacterial and fungal abundance and communities under four different treatment conditions. The results showed that the bacterial OTU number was significantly increased by SMOF, followed by CCF and COF, while the fungal OTU number was slightly increased by CCF, SMOF, and COF, as compared with the control. The results also showed that compared with the control, SMOF significantly increased the RA of Ohtaekwangia, Sphingomonas, unclassified_Sphingomonadaceae, and Saccharibacteria and reduced the RA of Spartobacteria, Gemmatimonas, Gp4, Flavisolibacter, Subdivision3, Gp6, and unclassified_Betaproteobacteria at the bacterial genus level; meanwhile, SMOF also significantly increased the RA of Podospora, Clitopilus, Ascobolus, Mortierella, and Sordaria, and reduced the RA of Knufia, Fusarium, Verticillium, and Gibberella at the fungal genus level. The change in the number of specific microbes in four different treatments may be due to the different nutrients in different fertilizers. Indeed, more attention should be paid to Ohtaekwangia, Sphingomonas, Sphingomonadacea, Saccharibacteria, Podospora, and Mortierella. Previous studies have reported that Ohtaekwangia showed a significant negative correlation with Ralstonia solanacearum (Deng et al., 2021); Sphingomonas possessed multifaceted functions, including remediation of environmental contaminations, producing highly beneficial phytohormones, degradation of organometallic compounds, and improving plant growth during stress conditions (Asaf et al., 2020); Sphingomonadacea could decompose organic pollutants (Timoshenko et al., 2021); Saccharibacteria was pointed out to be involved in hydrocarbon degradation (Figuroa-Gonzalez et al., 2020); Podospora produced a wide variety of carbohydrate-active enzymes, including xylanases, cellulases, and lytic polysaccharide monooxygenases (Silar, 2013; Fanuel et al., 2017); Mortierella was reported as a plant growth-promoting fungus (PGPF) by mobilizing P from insoluble forms and producing siderophores and phytoregulators (Srinivasan et al., 2012; Wani et al., 2017; Ceci et al., 2018; Ozimek and Hanaka, 2020). Furthermore, we used alpha diversity (Chao1, Shannon, and the Simpson index) and beta diversity (PCA) to measure the microbial species richness and diversity within each maize root-zone soil sample under four different fertilizer treatment conditions. The results showed that SMOF significantly increased the bacterial Chao1 index, but no significant difference was observed in the Chao1 index of fungi and the Shannon and Simpson index of bacteria and fungi. Meanwhile, the PCA analysis revealed that the bacterial community structure of the maize root-zone soil was significantly changed by SMOF (PERMANOVA, p < 0.05), and the different fertilizers explained 19.3% (p = 0.026) of the variation, but no significant change was observed in fungal community structure diversity. In other words, the richness of the microbe was increased by SMOF, but the diversity was differentially affected by SMOF. The current results align with those of Li et al. (2023), who reported that different fertilizers altered the composition of the pakchoi rhizosphere soil microbial community. Similar results were also obtained by Li et al. (2022c), who reported that three kinds of microbial fertilizer (CMA, TMF, and SMF) could increase the OTU number and bacterial Chao1 index of corn rhizosphere soil in newly reclaimed land, but no significant difference was observed in the Shannon index. Taken overall, the application of SMOF could favor the diversity of beneficial microbes and reshape the root zone microbial abundance distribution by enriching specific soil microbes in maize in a reclaimed barren mountainous land.
To further elucidate the impact of SMOF on microbial groups, LEfSe (LDA > 2 for bacteria or LDA > 2.5 for fungi, p < 0.05) was carried out to explore the role of specific microbes in the fertility improvement of reclaimed barren mountainous land soil. A total of 11 bacterial biomarkers were obtained in SMOF, CCF, and the control. Furthermore, the heat map indicated that SMOF could significantly enrich Sphingomonas, Ohtaekwangia, and unclassified_Alphaproteobacteria. Moreover, a total of 15 fungal biomarkers were collected from four different treatments. The heat map revealed that SMOF could significantly enrich Podospora, Cristinia, Sordaria, and Mortierella. It is worth noting that some species of Sphingomonas, Ohtaekwangia, Podospora, and Mortierella play an important role in improving plant growth, enhancing resilience to plant pathogens and producing a variety of carbohydrate-active enzymes (Silar, 2013; Asaf et al., 2020; Ozimek and Hanaka, 2020; Deng et al., 2021). In our previous studies, four fungal and five bacterial strains were isolated from new reclamation soil, and those strains had a great ability to improve the soil fertility and promote plant growth by solubilizing P, fixing N, producing siderophores, and indole acetic acid (Li et al., 2021a,c). Those microbes may have greater potential to colonize and affect soil physicochemical properties and microbial communities in reclaimed barren soil.
To test whether some soil physicochemical properties influenced microbial composition dispersion, we used RDA to examine the correlation between microbial communities and environmental factors. The results indicated that a total of 53.42% of the cumulative variance of the root-zone bacterial community-factor correction was at the genus level, and the bacterial communities could be significantly influenced by available K, OMC, available P, and MBN (explained as 25.64%, 25.36%, 25.15%, and 23.38% of the bacterial community, respectively) in all four different treatments. Additionally, a total of 47.40% of the cumulative variance of the root-zone fungal community-factor correction at the genus level, and the fungal communities could be significantly influenced by available K, pH, and MBC (explained as 32.96%, 23.49%, and 22.67% of the fungal community, respectively) in all four different treatments. Previous studies have also revealed that the growth of soil microbes could be affected by a variety of environmental factors. For example, Li et al. (2023) found that the growth of pakchoi soil microbes was affected by available P, AHN, pH, OMC, and total N; Tian et al. (2017) reported that soil organic content exerted the largest effect on the distribution of bacterial communities.
Correlation networks of co-occurring microorganisms allow the visual summary of lots of information, and co-occurrence networks offer new insights into microbial interaction analysis (Chaffron et al., 2010; Jiang et al., 2022). In this study, we applied correlation-based network results to analyze the interaction between OTUs of different treatments (Sparcc's correlation N > 0.5 or <−0.5, p < 0.01). It is well-known that more nodes and edges and higher community diversity represent a complex and stable network structure (Hernandez et al., 2021), and high modularity is also an indicator of the network's structural stability (Ma et al., 2021). In our study, four different treatments had different microbial co-occurrence network structures. However, compared with the control, in the SMOF treatment, the number of bacterial network nodes increased, but the edges, the average degree, and the modularity decreased; meanwhile, the number of fungal network nodes, edges, and the average degree decreased, but the modularity increased. Strikingly, most of the relationships between the bacterial and fungal communities in the SMOF treatment were positive, which indicated that most bacteria and fungi had similar guilds or niches and were mutually beneficial rather than competitive (Deng et al., 2016).
A total of 1,054 peaks were screened from maize root-zone soil in four different treatments. The results of OPLS-DA revealed that the metabolites in control root-zone soil were significantly changed by SMOF, COF, and CCF, which was also verified by the volcano plot. A total of 277 metabolites were identified by LC-MS analysis, which mainly belongs to lipids and lipid-like molecules, organoheterocyclic compounds, benzenoids, organic acids and derivatives, organic oxygen compounds, and so on. In the SMOF and the control groups, there were 1,221 DEMs screened, with 1,107 upregulated and 114 downregulated. Compared to the control, 34 DEMs were significantly changed by SMOF, among which 10 DEMs (S-Nitrosoglutathione, edulan I, tetramethylpyrazine, 8,15-DiHETE, hypogeic acid, rotenone, 2-Pyrrolidinone, daucol, N-[(4-Hydroxy-3-methoxyphenyl)methyl]octanamide, [2,2-Bis(2-methylpropoxy)ethyl]benzene) were significantly upregulated, and five DEMs (stearidonic acid, (10E,12Z)-(9S)-9-Hydroperoxyoctadeca-10,12-dienoic acid, 9-HOTE, (3beta,5beta,8beta,22E,24xi)-Ergosta-6,22-diene-3,5,8-triol, Na,Na-Dimethylhistamine) were significantly downregulated. As we know, benzenoids, lipids, organoheterocyclic compounds, organic acids, phenylpropanoids, polyketides, and other secondary metabolites are essential to life, playing a vital role in the metabolism of all living cells. Previous reports have shown that a number of different benzenoid compounds are rapidly produced in plants or microbes in response to insects, pathogens, or stress (Keen and Taylor, 1975; Herrmann, 1995); lipids are essential for the integrity of cells and organelles by acting as a hydrophobic barrier for the membrane (Kim, 2020); organic acids play significant and varied roles in rhizosphere acidification and mineral weathering, contributing protons and serving as ligands for complex metals; they can promote redox reactions with electron-deficient metals (a rhizosphere-promoted process considered in the next section on redox cycling) (Daniel et al., 2007). To further explore the correlation between microbes and DEMs, a clustering heat map was drawn. The results showed that in the groups of SMOF and the control, there were four DEMs significantly correlated with two genera of bacteria, among which Saccharibacteria was significantly negatively correlated with two DEMs and positively correlated with one DEM, and unclassified_Rhizobiales was significantly negatively correlated with one DEM; meanwhile, 10 DEMs significantly correlated with five genera of fungi, among which Cheilymenia was significantly positively correlated with one DEM, Paraphoma was significantly negatively correlated with two DEMs, and positively correlated with one DEM, Sodiomyces was significantly positively correlated with one DEM, Dipodascus was significantly positively correlated with one DEM, and negatively correlated with four DEMs, and Cephalotrichum was significantly negatively correlated with four DEMs. The results revealed complicated interactions between microbes and DEMs in maize root zone soil.
In conclusion, our results showed that SMOF not only significantly improved the soil quality (including the soil's physical, chemical, and biological properties) but also promoted maize growth. This suggests that SMOF can be a good amendment for maize production in a reclaimed barren mountainous land. Compared with the control, SMOF caused a differential change in the microbial community of reclaimed barren mountainous land. Some specific beneficial microbes, such as Ohtaekwangia, Sphingomonas, Sphingomonadacea, Saccharibacteria, Podospora, and Mortierella, have been found to be closely related to the soil improvement by SMOF, indicating the relationships among microbes, fertilizer, and soil. Furthermore, RDA results showed that the composition of bacterial and fungal communities in maize root-zone soil was significantly affected by available K, OMC, P, MBN, and available K, pH, and MBC, respectively. In SMOF treatment, most microbes had similar guilds or niches and were mutually beneficial rather than competitive. In addition, SMOF resulted in a significant change in the kinds and relative contents of metabolites in the root-zone soil. The correlation heat map showed a significant correlation at the genus level between related microbial groups and DEMs of maize root-zone soil in SMOF. All these results revealed that SMOF could influence the interaction among soil properties, microbial communities, and secondary metabolites and improve maize growth. We believe that these may play an important role in improving soil quality and promoting maize production in the reclaimed barren mountainous land.
The datasets presented in this study can be found in online repositories. The names of the repositories are NCBI and CNGBdb, with accession number PRJNA951893, PRJNA951350 and CNP0004202, respectively.
Conceptualization: XL, DW, QL, ZT, and JY. Methodology and investigation: XL, DW, and JY. Software: XL. Validation: XL, QL, ZT, and JY. Formal analysis, resources, and visualization: XL, QL, and JY. Data curation, supervision, project administration, and funding acquisition: QL and JY. Writing—original draft preparation and writing—review and editing: XL and JY. All authors have read and agreed to the published version of the manuscript.
This research was funded by the Key Research and Development Program of Zhejiang Province (2019C02035) and the Science and Technology Innovation and Promotion Demonstration Project of Hangzhou Academy of Agricultural Sciences (2022HNCT-13, 2022HNCT-07, and 2022HNCT-02).
The authors declare that the research was conducted in the absence of any commercial or financial relationships that could be construed as a potential conflict of interest.
All claims expressed in this article are solely those of the authors and do not necessarily represent those of their affiliated organizations, or those of the publisher, the editors and the reviewers. Any product that may be evaluated in this article, or claim that may be made by its manufacturer, is not guaranteed or endorsed by the publisher.
Adams, R. I., Miletto, M., Taylor, J. W., and Bruns, T. D. (2013). Dispersal in microbes: fungi in indoor air are dominated by outdoor air and show dispersal limitation at short distances. ISME J. 7, 1262–1273. doi: 10.1038/ismej.2013.28
Alhrout, H. H., Akash, M. W., and Hejazin, R. K. (2018). Effect of farm yard manure and NPK on the yield and some growth components of tomato (Lycopersicum esculentum). Res. Crops 19, 655–658. doi: 10.31830/2348-7542.2018.0001.43
Altschul, S. F., Madden, T. L., Schäffer, A. A., Zhang, J., Zhang, Z., Miller, W., et al. (1997). Gapped BLAST and PSI-BLAST: a new generation of protein database search programs. Nucleic Acids Res. 25, 3389–3402. doi: 10.1093/nar/25.17.3389
Amanullah, I., Hidayatullah, K., Jan, A., Shah, Z., Jamal Khan, M., Parmar, B., et al. (2019). “Organic carbon sources and nitrogen management improve biomass of hybrid rice (Oryza sativa L.) under nitrogen deficient condition,” in Advances in Rice Research for Abiotic Stress Tolerance, eds M. Hasanuzzaman, M. Fujita, and K. Nahar (Amsterdam: Elsevier), 447–467. doi: 10.1016/B978-0-12-814332-2.00022-8
Asaf, S., Numan, M., Khan, A. L., and AI-Harrasi, A. (2020). Sphingomonas: from diversity and genomics to functional role in environmental remediation and plant growth. Crit. Rev. Biotechnol. 40, 138–152. doi: 10.1080/07388551.2019.1709793
Baran, A., Mierzwa-Hersztek, M., Gondek, K., Tarnawski, M., Szara, M., Gorczyca, O., et al. (2019). The influence of the quantity and quality of sediment organic matter on the potential mobility and toxicity of trace elements in bottom sediment. Environ. Geochem. Health 41, 2893–2910. doi: 10.1007/s10653-019-00359-7
Brookes, P. C., Landman, A., Pruden, G., and Jenkinson, D. S. (1985). Chloroform fumigation and the release of soil nitrogen: a rapid direct extraction method to measure microbial biomass nitrogen in soil. Soil. Boil. Biochem. 17, 837–842. doi: 10.1016/0038-0717(85)90144-0
Caporaso, J. G., Kuczynski, J., Stombaugh, J., Bittinger, K., Bushman, F. D., Costello, E. K., et al. (2010). QIIME allows analysis of high-throughput community sequencing data. Nat. Methods 7, 335–336. doi: 10.1038/nmeth.f.303
Ceci, A., Pinzari, F., Russo, F., Maggi, O., and Persiani, A. M. (2018). Saprotrophic soil fungi to improve phosphorus solubilisation and release: in vitro abilities of several species. Ambio 47(Suppl.1), S30–S40. doi: 10.1007/s13280-017-0972-0
Chaffron, S., Rehrauer, H., Pernthaler, J., and von Mering, C. (2010). A global network of coexisting microbes from environmental and whole-genome sequence data. Genome Res. 20, 947–959. doi: 10.1101/gr.104521.109
Daniel de, D. R., Oh, N. H., Fimmen, R., and Jackson, J. (2007). Chapter 8-The rhizosphere and soil formation. The Rhizosphere, eds A. G. Cardon, J. L. Whitbeck, and M. A, Burlington (Elsevier Academic Press), 179–220. doi: 10.1016/B978-012088775-0/50010-0
Deng, X., Zhang, N., Shen, Z., Zhu, C., Liu, H., Xu, Z., et al. (2021). Soil microbiome manipulation triggers direct and possible indirect suppression against Ralstonia solanacearum and Fusarium oxysporum. NPJ Biofilms Microbiomes 7, 33. doi: 10.1038/s41522-021-00204-9
Deng, Y., Zhang, P., Qin, Y., Tu, Q., Yang, Y., He, Z., et al. (2016). Network succession reveals the importance of competition in response to emulsified vegetable oil amendment for uranium bioremediation. Environ. Microbiol. 18, 205–218. doi: 10.1111/1462-2920.12981
Dixon, P. (2003). VEGAN, a package of R functions for community ecology. J. Veg. Sci 14, 927–930. doi: 10.1111/j.1654-1103.2003.tb02228.x
Dong, W., Zhang, X., Wang, H., Dai, X., Sun, X., Qiu, W., et al. (2012). Effect of different fertilizer application on the soil fertility of paddy soils in red soil region of Southern China. PLOS ONE 7, e44504. doi: 10.1371/journal.pone.0044504
Edgar, R. C. (2013). UPARSE: highly accurate OTU sequences from microbial amplicon reads. Nat. Methods 10, 996–998. doi: 10.1038/nmeth.2604
Edgar, R. C. (2016). SINTAX: a simple non-Bayesian taxonomy classifier for 16S and ITS sequences. bioRxiv. 1–20. doi: 10.1101/074161
El Gammal, O. H. M., and Salama, A. S. M. (2016). Effect of sheep manure application rate and method on growth, fruiting and fruit quality of Balady guava trees grown under mid-sinai conditions. IOSR-JAVS 9, 2319–2380. doi: 10.9790/2380-09115972
Fanuel, M., Garajova, S., Ropartz, D., McGregor, N., Brumer, H., Rogniaux, H., et al. (2017). The Podospora anserina lytic polysaccharide monooxygenase Pa LPMO9H catalyzes oxidative cleavage of diverse plant cell wall matrix glycans. Biotechnol. Biofuels 10, 63. doi: 10.1186/s13068-017-0749-5
Figuroa-Gonzalez, P. A., Bornemann, T. L. V., Adam, P. S., Plewka, J., Révész, F., von Hagen, C. A., et al. (2020). Saccharibacteria as organic carbon sinks in hydrocarbon-fueled communities. Front. Microbiol. 11, 587782. doi: 10.3389/fmicb.2020.587782
Gong, F., Wu, X., Zhang, H., Chen, Y., and Wang, W. (2015). Making better maize plants for sustainable grain production in a changing climate. Front. Plant Sci. 6, 835. doi: 10.3389/fpls.2015.00835
Gregorich, E., Janzenx, H. H., Helgason, B., and Ellert, B. (2015). Nitrogenous gas emissions from soils and greenhouse gas effects. Adv. Agron. 132, 39–74. doi: 10.1016/bs.agron.2015.02.004
Han, S. H., An, J. Y., Hwang, J., Kim, S. B., and Park, B. B. (2016). The effects of organic manure and chemical fertilizer on the growth and nutrient concentrations of yellow poplar (Liriodendron tulipifera Lin.) in a nursery system. Forest Sci. Technol. 12, 137–143. doi: 10.1080/21580103.2015.1135827
Haripal, K., and Sahoo, S. (2014). Microbial biomass carbon, nitrogen, and phosphorus dynamics along a chronosequence of abandoned tropical agroecosystems. Int. J. Curr. Microbiol. App. Sci 3, 956–970. Available online at: http://www.ijcmas.com.
Hernandez, D. J., David, A. S., Menges, E. S., Searcy, C. A., and Afkhami, M. E. (2021). Environmental stress destabilizes microbial networks. ISME J. 15, 1722–1734. doi: 10.1038/s41396-020-00882-x
Herrmann, K. M. (1995). The shikimate pathway: early steps in the biosynthesis of aromatic compounds. Plant Cell 7, 907–919. doi: 10.2307/3870046
Hollander, M., Wolfe, D. A., and Chicken, E. (2008). “Nonparametric statistical methods” in Biostatistics and Microbiology: A Survival Manual, ed. S. P. Daryl (New York, NY: Springer), 121–162. doi: 10.1007/978-0-387-77282-0_7
Hu, G. C., Chen, D. H., Huang, X. P., Zhou, C. H., Yu, G. R., and Pan, J. L. (2014). The research about balance system of farm land in occupation and supplement: a demonstration from Lin'an city. J. Agr. 4, 112–115.
Hu, T., Fan, J., Hou, H., Li, Y., Li, Y., and Huang, K. (2021). Long-term monitoring and evaluation of land development in a reclamation area under rapid urbanization: a case-study in Qiantang New District, China. Land Degrade. Dev. 2, 3259–3271. doi: 10.1002/ldr.3980
Huang, Q. R., Hu, F., Huang, S., Li, H. X., Yuan, Y. H., Pan, G. X., et al. (2009). Effect of long-term fertilization on organic carbon and nitrogen in a subtropical paddy soil. Pedosphere 19, 727–734. doi: 10.1016/S1002-0160(09)60168-5
Huang, S., Zhang, W., Yu, X., and Huang, Q. (2010). Effects of long-term fertilization on corn productivity and its sustainability in an Ultisol of southern China. Agric. Ecosyst. Environ. 138, 44–50. doi: 10.1016/j.agee.2010.03.015
Hyvönen, R., Persson, T., Andersson, S., Olsson, B., Ågren, G. I., and Linder, S. (2008). Impact of long-term nitrogen addition on carbon stocks in trees and soils in northern Europe. Biogeochemistry 89, 121–137. doi: 10.1007/s10533-007-9121-3
Jiang, H., Lv, L., Ahmed, T., Jin, S., Shahid, M., Noman, M., et al. (2022). Nanoparticles-mediated amelioration of tomato bacterial wilt disease by modulating the rhizosphere bacterial community. Int. J. Mol. Sci. 23, 414. doi: 10.3390/ijms23010414
Keen, N. T., and Taylor, O. (1975). Ozone injury in soybeans: isoflavonoid accumulation is related to necrosis. Plant Physiol. 55, 731–733. doi: 10.1104/pp.55.4.731
Koocheki, A., and Seyyedi, S. M. (2015). Relationship between nitrogen and phosphorus use efficiency in saffron (Crocus sativus L.) as affected by mother corm size and fertilization. Ind. Crop. Prod. 71, 128–137. doi: 10.1016/j.indcrop.2015.03.085
Lam, S. K., Chen, D., Norton, R., Armstrong, R., and Mosier, A. R. (2012). Nitrogen dynamics in grain crop and legume pasture systems under elevated atmospheric carbon dioxide concentration: a meta-analysis. Glob. Chang. Biol. 18, 2853–2859. doi: 10.1111/j.1365-2486.2012.02758.x
Li, X., Li, D., Jiang, Y., Xu, J., Ren, X., Zhang, Y., et al. (2023). The effects of microbial fertilizer based Aspergillus brunneoviolaceus HZ23 on pakchoi growth, soil properties, rhizosphere bacterial community structure, and metabolites in newly reclaimed land. Front. Microbiol. 14, 1091380. doi: 10.3389/fmicb.2023.1091380
Li, X., Li, D., Lu, Q., Wang, D., Ren, X., Lv, L., et al. (2022a). Effects of different organic fertilizers on sweet potato growth and rhizosphere soil properties in newly reclaimed land. Agronomy 12, 1649. doi: 10.3390/agronomy12071649
Li, X., Li, D., Yan, J., Zhang, Y., Wang, H., Zhang, J., et al. (2021a). Effect of plant-growth-promoting fungi on eggplant (Solanum melongena L.) in new reclamation land. Agriculture 11, 1036. doi: 10.3390/agriculture11111036
Li, X., Lu, Q., Li, D., Wang, D., Ren, X., Yan, J., et al. (2022b). Effects of two kinds of commercial organic fertilizers on growth and rhizosphere soil properties of corn on new reclamation land. Plants 11, 2553. doi: 10.3390/plants11192553
Li, X., Lu, Q., Li, D., Wang, D., Ren, X., Yan, J., et al. (2022c). Effects of different microbial fertilizers on growth and rhizosphere soil properties of corn in newly reclaimed land. Plants 11, 1978. doi: 10.3390/plants11151978
Li, X., Su, Y., Ahmed, T., Ren, H., Javed, M. R., Yao, Y., et al. (2021b). Effects of different organic fertilizers on improving soil from newly reclaimed land to crop soil. Agriculture 11, 560. doi: 10.3390/agriculture11060560
Li, X., Yan, J., Li, D., Jiang, Y., Zhang, Y., Wang, H., et al. (2021c). Isolation and molecular characterization of plant-growth-promoting bacteria and their effect on eggplant (Solanum melongena) growth. Agriculture 11, 1258. doi: 10.3390/agriculture11121258
Li, Y., Wu, J., Shen, J., Liu, S., Wang, C., Chen, D., et al. (2016). Soil microbial C: N ratio is a robust indicator of soil productivity for paddy fields. Sci. Rep. 6, 35266. doi: 10.1038/srep35266
Ma, W., Yang, Z., Liang, L., Ma, Q., Wang, G., and Zhao, T. (2021). Characteristics of the fungal communities and co-occurrence networks in hazelnut tree root endospheres and rhizosphere soil. Front. Plant Sci. 12, 749871. doi: 10.3389/fpls.2021.749871
Magdoff, F., Lanyon, L., and Liebhardt, B. (1997). Nutrient cycling, transformations and flows: implications for a more sustainable agriculture. Adv Agron. 60, 1–73. doi: 10.1016/S0065-2113(08)60600-8
Martin, M. (2011). CUTADAPT removes adapter sequences from high-throughput sequencing reads. EMBnet J. 17, 10–12. doi: 10.14806/ej.17.1.200
Ort, D. R., and Long, S. P. (2014). Limits on yields in the corn belt. Science 344, 484–485. doi: 10.1126/science.1253884
Ozimek, E., and Hanaka, A. (2020). Mortierella species as the plant growth-promoting fungi present in the agricultural soils. Agriculture 11, 7. doi: 10.3390/agriculture11010007
Piperno, D. R., Ranereb, A. J., Holstb, I., Iriarted, J., and Dickauc, R. (2009). Starch grain and phytolith evidence for early ninth millennium B.P. maize from the Central Balsas River Valley, Mexico. Proc. Natl. Acad. Sci. U.S.A. 106, 5019–5024. doi: 10.1073/pnas.0812525106
Qiu, S., Gao, H., Zhu, P., Hou, Y., Zhao, S., Rong, X., et al. (2016). Changes in soil carbon and nitrogen pools in a Mollisol after long-term fallow or application of chemical fertilizers, straw or manures. Soil Till. Res. 163, 255–265. doi: 10.1016/j.still.2016.07.002
Quast, C., Pruesse, E., Yilmaz, P., Gerken, J., Schweer, T., Yarza, P., et al. (2013). The SILVA ribosomal RNA gene database project: improved data processing and web-based tools. Nucleic Acids Res. 41, 590–596. doi: 10.1093/nar/gks1219
Ramette, A. (2007). Multivariate analyses in microbial ecology. FEMS Microbiol. Ecol. 62, 142–160. doi: 10.1111/j.1574-6941.2007.00375.x
Rendana, M., Idris, W. M. R., Rahim, S. A., Rahman, Z. A., Lihan, T., and Jamil, H. (2018). Reclamation of acid sulphate soils in paddy cultivation area with organic amendments. Aims Agr. Food 3, 358–371. doi: 10.3934/agrfood.2018.3.358
Roy, A. K., Wagner-Riddle, C., Deen, B., Lauzon, J., and Bruulsema, T. (2014). Nitrogen application rate, timing and history effects on nitrous oxide emissions from corn (Zea mays L.). Can. J. Soil Sci. 94, 563–573. doi: 10.4141/cjss2013-118
Schmieder, R., and Edwards, R. (2011). Quality control and preprocessing of metagenomic datasets. Bioinformatics 27, 863–864. doi: 10.1093/bioinformatics/btr026
Segata, N., Izard, J., Waldron, L., Gevers, D., Miropolsky, L., Garrett, W. S., et al. (2011). Metagenomic biomarker discovery and explanation. Genome Biol. 12, R60. doi: 10.1186/gb-2011-12-6-r60
Silar, P. (2013). “Podospora anserina: from laboratory to biotechnology,” in Genomics of Soil-and Plant-Associated Fungi. Soil Biology 36, eds B. A. Horwitz, P. K. Mukherjee, M. Mukherjee, and C. P. Kubicek (Berlin: Springer), 283–309. doi: 10.1007/978-3-642-39339-6_12
Srinivasan, R., Yandigeri, M. S., Kashyap, S., and Alagawadi, A. R. (2012). Effect of salt on survival and P-solubilization potential of phosphate solubilizing microorganisms from salt affected soils. Saudi J. Biol. Sci. 19, 427–434. doi: 10.1016/j.sjbs.2012.05.004
Tan, M., Li, X., and Liu, C. (2005). Urban land expansion and arable land loss of the major cities in China in the 1990s. Sci. China Ser. D: Earth Sci. 48, 1492–1500. doi: 10.1360/03yd0374
Teixeira, R. S., Souza, I. F., Santos, R. S., Gomes, L. C., Borges, S. R., Vergutz, L., et al. (2019). Impact of fertilization on cover crops and microbial community on a bauxite-mined soil undergoing reclamation. J. Plant Nutr. Soil Sci. 182, 515–523. doi: 10.1002/jpln.201800586
Therios, I. (1996). Mineral Nutrition of Plants. Dedousi publications: Thessaloniki, Greece. (in Greek).
Tian, Q., Taniguchi, T., Shi, W., Li, G., Yamanaka, N., and Du, S. (2017). Land-use types and soil chemical properties influence soil microbial communities in the semiarid Loess Plateau region in China. Sci. Rep. 7, 45289. doi: 10.1038/srep45289
Timoshenko, A., Kolesnikov, S., Raiput, V. D., and Minkina, T. (2021). “Chapter 14 - Effects of zinc-oxide nanoparticles on soil microbial community and their functionality,” in Zinc-Based Nanostructures for Environmental and Agricultural Applications, Nanobiotechnology for Plant Protection, ed K. A. Abd-Elsalam (Amstrdam: Elsevier Science), 267–284. doi: 10.1016/B978-0-12-822836-4.00029-X
Urmas, K., Nilsson, R. H., Abarenkov, K., Tedersoo, L., Tayloy, A. F. S., Bahram, M., et al. (2013). Towards a unified paradigm for sequence-based identification of fungi. Mol. Ecol. 22, 5271–5277. doi: 10.1111/mec.12481
Vance, E. D., Brookes, P. C., and Jenkinson, D. S. (1987). An extraction method for measuring soil microbial biomass C. Soil. Boil. Biochem. 19, 703–707. doi: 10.1016/0038-0717(87)90052-6
Wang, Q., Garrity, G. M., Tiedje, J. M., and Cole, J. R. (2007). Naive Bayesian classifier for rapid assignment of rRNA sequences into the new bacterial taxonomy. Appl. Environ. Microbiol. 73, 5261–5267. doi: 10.1128/AEM.00062-07
Wang, Z., Shen, Q. Y., Shen, J. G., Zhu, J., Ma, W. H., and Yang, Y. M. (2017). Investigation on soil physical and chemical properties of new cultivated land in Yuhang District and improvement measures. Zhejiang Agric. Sci. 58, 1467–1470.
Wani, Z. A., Kumar, A., Sultan, P., Bindu, K., Riyaz-Ul-Hassan, S., and Ashraf, N. (2017). Mortierella alpina CS10E4, an oleaginous fungal endophyte of Crocus sativus L. enhances apocarotenoid biosynthesis and stress tolerance in the host plant. Sci. Rep. 7, 8598. doi: 10.1038/s41598-017-08974-z
Wu, L. Y., Wen, C. Q., Qin, Y. J., Yin, H. Q., Tu, Q. C., Nostrand, J. D. V., et al. (2015). Phasing amplicon sequencing on Illumina Miseq for robust environmental microbial community analysis. BMC Microbiol. 15, 125. doi: 10.1186/s12866-015-0450-4
Yan, J. L., Zhang, M. K., and Wang, D. Z. (2021). Different amendments: effect on soil physical properties of newly reclaimed land in low hilly region. Chin. Agr. Sci. Bull. 37, 67–73.
Yarami, N., and Sepaskhah, A. (2015). Saffron response to irrigation water salinity, cow manure and planting method. Agric. Water Manag. 150, 57–66. doi: 10.1016/j.agwat.2014.12.004
Zhang, J., Kobert, K., Flouri, T., and Stamatakis, A. (2014). PEAR: a fast and accurate Illumina Paired-End reAd mergeR. Bioinformatics 30, 614–620. doi: 10.1093/bioinformatics/btt593
Zhang, Y., Yang, S., Fu, M., and Cai, J. (2015). Sheep manure application increases soil exchangeable base cations in a emi-arid steppe of Inner Mongolia. J. Arid Land 7, 361–369. doi: 10.1007/s40333-015-0004-5
Zhao, P. J. (2016). Planning for social inclusion: the impact of socioeconomic inequities on the informal development of farmland in suburban Beijing. Land Use Policy 57, 431–443. doi: 10.1016/j.landusepol.2016.06.010
Keywords: SMOF, soil property, microbial community, metabonomics, maize, reclaimed barren mountainous land
Citation: Li X, Wang D, Lu Q, Tian Z and Yan J (2023) Effects of SMOF on soil properties, root-zone microbial community structure, metabolites, and maize (Zea mays L.) response on a reclaimed barren mountainous land. Front. Microbiol. 14:1181245. doi: 10.3389/fmicb.2023.1181245
Received: 07 March 2023; Accepted: 02 May 2023;
Published: 25 May 2023.
Edited by:
Dilfuza Egamberdieva, Leibniz Center for Agricultural Landscape Research (ZALF), GermanyReviewed by:
Murugan Kumar, National Bureau of Agriculturally Important Microorganisms (ICAR), IndiaCopyright © 2023 Li, Wang, Lu, Tian and Yan. This is an open-access article distributed under the terms of the Creative Commons Attribution License (CC BY). The use, distribution or reproduction in other forums is permitted, provided the original author(s) and the copyright owner(s) are credited and that the original publication in this journal is cited, in accordance with accepted academic practice. No use, distribution or reproduction is permitted which does not comply with these terms.
*Correspondence: Qiujun Lu, bHVxaXVqdW4xOUBnbWFpbC5jb20=; Jianli Yan, eWFuamlhbmxpMDBAZ21haWwuY29t
Disclaimer: All claims expressed in this article are solely those of the authors and do not necessarily represent those of their affiliated organizations, or those of the publisher, the editors and the reviewers. Any product that may be evaluated in this article or claim that may be made by its manufacturer is not guaranteed or endorsed by the publisher.
Research integrity at Frontiers
Learn more about the work of our research integrity team to safeguard the quality of each article we publish.