- 1Laboratory of Carbon Monitoring in Terrestrial Ecosystems, Institute of Physicochemical and Biological Problems in Soil Science, Pushchino, Russia
- 2Department of Soil Physics, Physical Chemistry and Biophysics, Agrophysical Research Institute, Saint Petersburg, Russia
- 3Center for Isotope Biogeochemistry, University of Tyumen, Tyumen, Russia
- 4Research Institute on Terrestrial Ecosystems, National Research Council, Porano, Italy
- 5National Biodiversity Future Center, Palermo, Italy
- 6Laboratory of Soil Carbon and Nitrogen Cycles, Institute of Physicochemical and Biological Problems in Soil Science, Pushchino, Russia
- 7Terrestrial Ecology Group, Institute of Zoology, University of Cologne, Cologne, Germany
- 8Soil Science Faculty, Lomonosov Moscow State University, Moscow, Russia
The patterns of change in bioclimatic conditions determine the vegetation cover and soil properties along the altitudinal gradient. Together, these factors control the spatial variability of soil respiration (RS) in mountainous areas. The underlying mechanisms, which are poorly understood, shape the resulting surface CO2 flux in these ecosystems. We aimed to investigate the spatial variability of RS and its drivers on the northeastern slope of the Northwest Caucasus Mountains, Russia (1,260–2,480 m a.s.l.), in mixed, fir, and deciduous forests, as well as subalpine and alpine meadows. RS was measured simultaneously in each ecosystem at 12 randomly distributed points using the closed static chamber technique. After the measurements, topsoil samples (0–10 cm) were collected under each chamber (n = 60). Several soil physicochemical, microbial, and vegetation indices were assessed as potential drivers of RS. We tested two hypotheses: (i) the spatial variability of RS is higher in forests than in grasslands; and (ii) the spatial variability of RS in forests is mainly due to soil microbial activity, whereas in grasslands, it is mainly due to vegetation characteristics. Unexpectedly, RS variability was lower in forests than in grasslands, ranging from 1.3–6.5 versus 3.4–12.7 μmol CO2 m−1 s−1, respectively. Spatial variability of RS in forests was related to microbial functioning through chitinase activity (50% explained variance), whereas in grasslands it was related to vegetation structure, namely graminoid abundance (27% explained variance). Apparently, the chitinase dependence of RS variability in forests may be related to soil N limitation. This was confirmed by low N content and high C:N ratio compared to grassland soils. The greater sensitivity of grassland RS to vegetation structure may be related to the essential root C allocation for some grasses. Thus, the first hypothesis concerning the higher spatial variability of RS in forests than in grasslands was not confirmed, whereas the second hypothesis concerning the crucial role of soil microorganisms in forests and vegetation in grasslands as drivers of RS spatial variability was confirmed.
1. Introduction
Soil respiration (RS) is one of the major fluxes of the global carbon (C) cycle affecting atmospheric CO2 concentrations. The process of RS potentially provides feedback to global climate change due to the large amount of C currently stored in soil organic matter (SOM), otherwise known as soil organic carbon (SOC) (Reichstein et al., 2003; Frey et al., 2013). SOC accounts for approximately 1,500 Pg of total C unequally distributed in the uppermost meter of the global soil layer, representing the largest terrestrial C pool (Kutsch et al., 2010; Schaufler et al., 2010; Mayer et al., 2020). The ratio between SOC accumulation in terrestrial ecosystems and SOC loss as CO2 efflux from soil determines whether an ecosystem serves as an atmospheric sink or source of C (Schlesinger and Andrews, 2000; Bolstad et al., 2004).
The processes and factors affecting RS has become a heavily scrutinized topic in the international research community in the broad context of its efforts to mitigate long-term climate change (Bu et al., 2012; Leifeld et al., 2013). However, the extremely high temporal and spatial variability of RS remains a challenge for the development of accurate regional and global models of the C cycle (Bond-Lamberty and Thomson, 2010). The temporal variation of the RS process is studied worldwide and can be effectively predicted by the dynamics of soil temperature and moisture (Luo and Zhou, 2006; Xu and Shang, 2016; Hursh et al., 2017; He et al., 2023). However, much remains unclear about the spatial variation of RS within different ecosystems, landscapes, and biomes, making it difficult to predict.
RS composed of autotrophic (mainly root respiration) and heterotrophic components hampers the quantification of spatial drivers controlling the total CO2 efflux from soil (Chen et al., 2017). The contribution of each of these components is characterized by high spatial heterogeneity (Subke et al., 2006). More specifically, the spatial variation of root respiration is affected by vegetation, i.e., by species composition, abundance of herbaceous species, and the corresponding density of fine roots in the upper soil layer (Rodeghiero and Cescatti, 2008). At the same time, changes in the microbial capacity to decompose SOM (enzymatic activity, basal respiration, microbial biomass abundance, etc.) from site to site determine the spatial distribution of heterotrophic respiration (Ananyeva et al., 2020). Consequently, the drivers of spatial distribution of different components of RS are interdependent, and it is quite difficult to determine which of these components are key.
Mountain landscapes occupy almost 25% of the global surface area (Kapos et al., 2000) and accumulate significant amounts of SOM (Canedoli et al., 2020); consequently, their contribution to the global С cycle can be considerable (Hansson et al., 2021). As for regional models describing the C cycle in mountains, the contribution of RS accounts for up to 21% of regional emissions estimates, exceeding even that of the C-rich soil of steppes (Kudeyarov et al., 2007). Nevertheless, there is a significant lack of data on RS in mountainous areas (Kudeyarov and Kurganova, 2005; Liu et al., 2014). These shortcomings are related to difficulties in logistics and equipment delivery, unfavorable climatic conditions, and measurement features (Lin et al., 2017). For instance, when measuring RS on mountain slopes, the correction factor on steepness should be applied (Xu and Shang, 2016). Therefore, researchers usually select a flat plot to determine RS, resulting in a lack of data about mountain landscapes with steep slopes. Hence, an extension of the regional database is necessary for better understanding the spatial patterns of RS in mountains and its drivers.
Most mountains (e.g., Himalayas, Alps, Caucasus) are covered with forests, which are replaced by grasslands at increased altitudes (Bardelli et al., 2017; Ahmad et al., 2020; Ivashchenko et al., 2021). Hence, the distribution patterns of RS change at higher altitudes. A higher variation coefficient in forests than in grasslands has been shown due to the high heterogeneity of soil conditions caused by gaps in forest canopies (Rodeghiero and Cescatti, 2008; Katayama et al., 2009; Qi et al., 2010; Darenova et al., 2016; Shi et al., 2019). Besides, considering that the ratio of heterotrophic to autotrophic respiration in forests is mainly higher than in grasslands as reviewed by Hanson et al. (2000), it would be reasonable to assume a different contribution of microbial and vegetation properties to the spatial variations of RS in these ecosystems. Despite a number of works having been conducted on the determination of drivers of spatial patterns of RS in forests (Luan et al., 2012; Jiang et al., 2020), there is still a lack of clear understanding on how they are combined in different ecosystem types. Such assessments face the substantial challenge of organizing simultaneous measurements of RS across various ecosystems to avoid the effect of temporal variability (Jiang et al., 2020). The spatial variability of RS could be assayed using manipulation experiments or monitoring natural environmental gradients in the field. Manipulation experiments in controlled environments negatively affect the ability to predict system responses to changing factors and, accordingly, the capacity to effectively schedule and implement conservation actions (Pressey et al., 2007; Reside et al., 2017; Ettinger et al., 2019). Interpreting the data from monitoring experiments performed in natural environments is a more challenging issue; nonetheless, it is a promising alternative to manipulation experiments as more realistic assessments of RS and its drivers could be achieved.
Thus, we developed an experimental design that allows for the mitigation of the effect of temporal variability via simultaneous measurements in various ecosystems along altitudinal gradient and only focused on spatial factors within forests and grasslands of the examined mountain slope. The following hypotheses were formulated:
i. The spatial variability of RS in forests is higher than in grasslands due to the high heterogeneity of environmental conditions (e.g., temperature and litter thickness) caused by canopy gaps compared to open grasslands.
ii. The spatial variability of RS in forests is attributable mainly to soil microbial activity, while spatial variability of RS in grasslands is attributable mainly to vegetation properties, taking into account different contributions of heterotrophic and autotrophic components to this process for the two ecosystem types.
2. Materials and methods
2.1. Study area and experimental design
The study was conducted in the Northwest Caucasus Mountains (43°40’N; 40°47′E) in Karachay-Cherkess Republic, South Russia. The mountain slope-to-test was the northeastern exposure and covered five vegetation belts: mixed forest at 1,200–18,00 m a.s.l., fir forest at 1,800–2,040 m a.s.l., deciduous forest at 2,040–2,290 m a.s.l., subalpine meadow at 2,290–2,300 m a.s.l., and alpine meadow at 2,300–2,500 m a.s.l. (Figure 1). The soil types were Cambisols, Umbrisols, and Leptosols formed on non-alkaline bedrock. The mean annual air temperature ranged from 3.5 to 5.9°C (our data for years 2018–2021; Table 1) and annual precipitation ranged from 800 to 1,850 mm (the nearest meteorological stations were located at 1,313 m a.s.l. and 2,006 m a.s.l.). The dominant vegetation species along the studied slope are shown in Table 1.
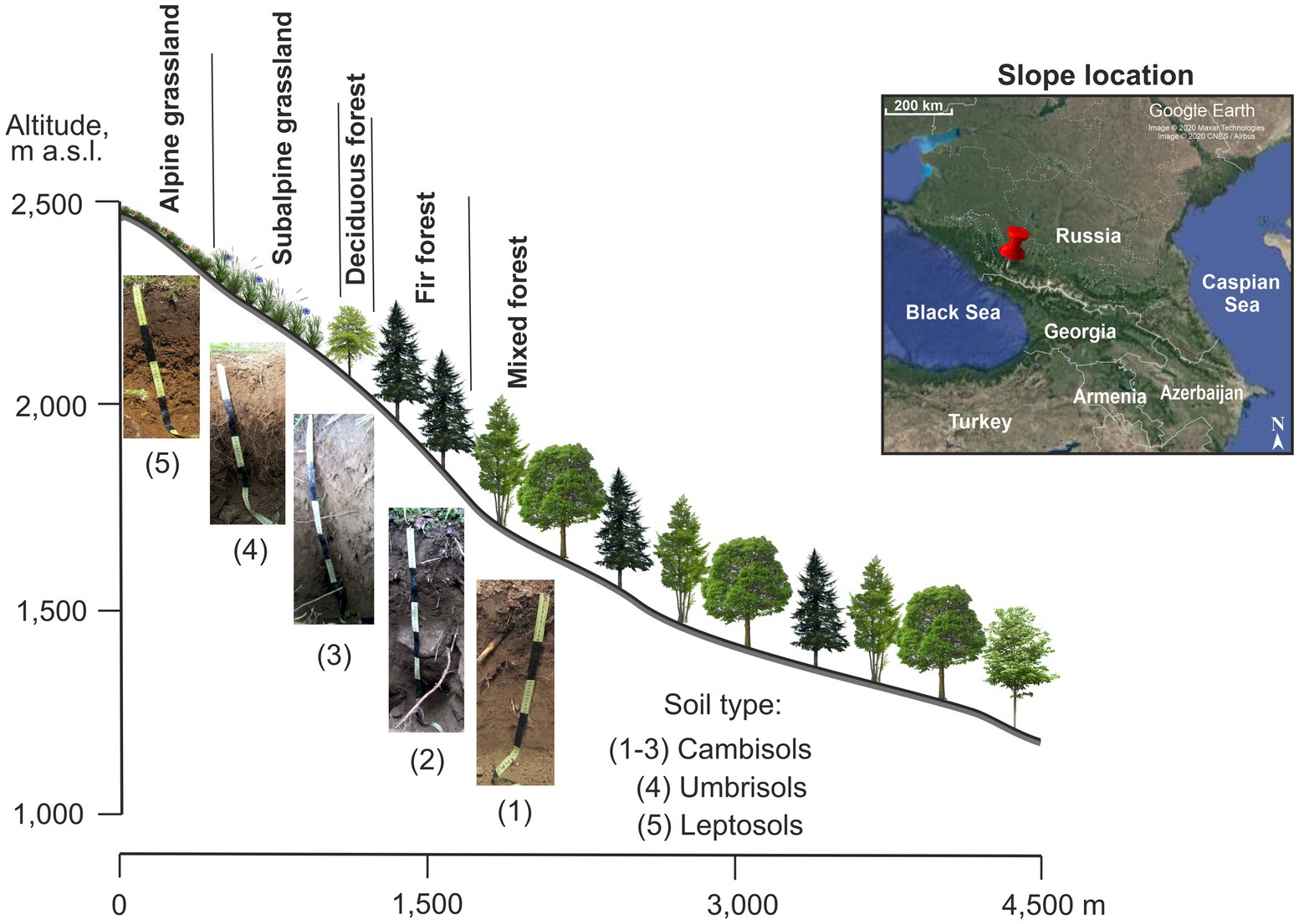
Figure 1. Scheme of the northeastern slope of Mt. Tkachiha (Northwest Caucasus, Karachay-Cherkess Republic, Russia).
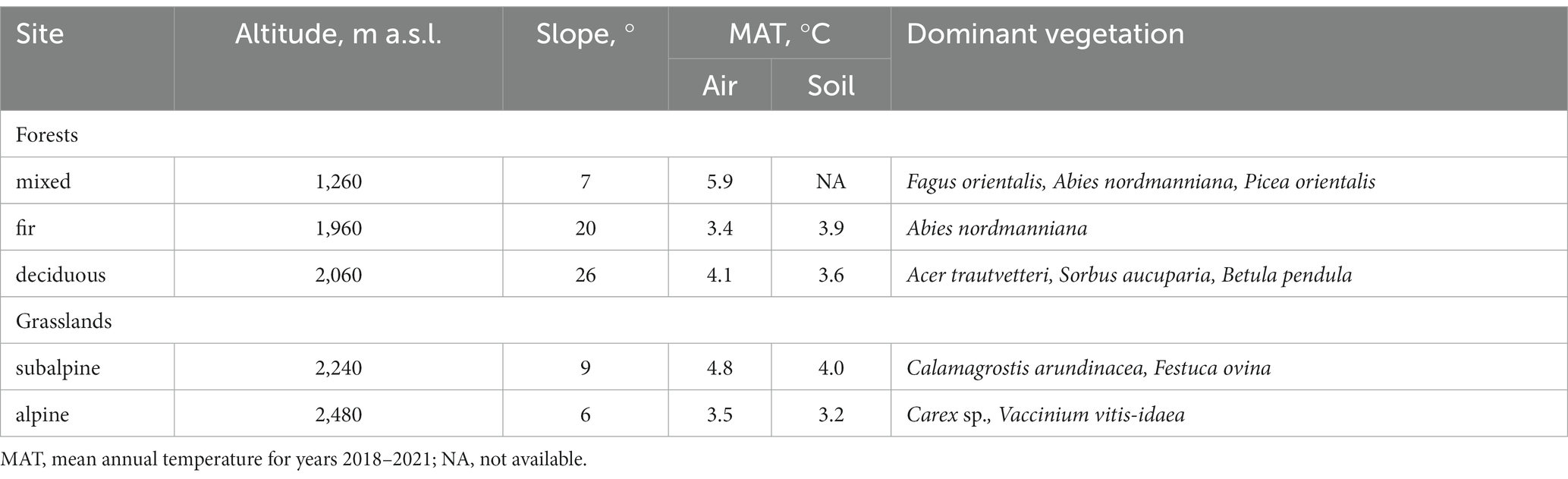
Table 1. General characteristics of studied forest and grassland sites along the northeastern slope of Mt. Tkachiha.
In each vegetation belt, 12 plots of 0.5 × 0.5 m were randomly established as described by Ivashchenko et al. (2021). In total, there were 60 plots along a 1.2 km altitudinal gradient. RS was measured simultaneously at all plots from 9:30 to 10:30 a.m. on August 11, 2018. This design was aimed at estimating the drivers of spatial variation of RS without considering the causes of its temporal changes. The measurement time (day and hour) was chosen so that the primary drivers of temporal variability of RS (i.e., plant phenology, soil temperature, and moisture) were close to representative throughout the altitudinal gradient. First, most plant species along the study slope reach their maximum phytomass and ripening stage in the first half of August. Second, on the eastern slope, there is enough light during the morning for photosynthesis, but there are still no large spatial fluctuations in soil temperature. On the measurement day, soil moisture across the slope was equally sufficient due to preceding rain events typical for the summer season at the location. Vegetation and soil physicochemical and microbial properties characterizing the sources, conditions, and mediators of CO2 formation in soil were considered as potential drivers of spatial variability of RS. In this case, only the upper 10-cm soil layer was taken into account, since (i) its microbial properties showed a close correlation with RS for different soils and ecosystems (Sushko S. et al., 2019; Sushko S. V. et al., 2019), and (ii) it provides main portion of surface CO2 fluxes from moist soils (Wiaux et al., 2015).
Vegetation surveys to identify plant species and their projective cover for the herbaceous layer were carried out at each 0.5 × 0.5 m plot before RS measurements. Simultaneously with the RS measurement, soil temperature was recorded at a 10 cm depth using the Checktemp sensor (Hanna Instruments, Germany), after which a single composite sample per plot (mixing 5 cores with Ø 5 cm) was taken from the upper 0–10 cm layer, placed in a plastic bag, and transported to the lab. Fresh samples (n = 60) were immediately sieved through a 2 mm mesh to exclude roots, debris, stones, and homogenized them. A portion of each soil sample was used to determine its moisture by the gravimetric method (8 h, 105°C). The remained soil was used for microbial and chemical analysis. Subsamples for microbial analysis were stored at 4°C for up to 2 weeks after sampling.
2.2. Soil respiration
Soil respiration (RS), i.e., CO2 efflux from the soil surface, was measured by the closed static chamber technique. For this, non-transparent PVC chambers (Ø 15.5 cm, volume 1.8 L) were inserted into the soil to a depth of 2–3 cm (above-ground grass was preliminarily cut) perpendicular to the slope surface. Three air samples (20 ml) were taken from each chamber through a rubber stopper with a gas-tight syringe and analyzed with an infrared gas analyzer (SBA-5, PP system, USA). The first zero-time sample was collected immediately after installing the chamber, while the other two were collected at an interval of 3–5 min. The day before, preliminary measurements were performed with gas sampling at nine time points (0, 1, 2, 3, 4, 5, 10, 15, 20 min) to determine the period of initial linear increasing of CO2 concentration inside the closed chamber at each site. It was found that 5 min is a representative time for all ecosystems during which the gas increases linearly (mean R2 = 0.98 ± 0.03). Additionally, we recorded atmospheric pressure along the altitudinal gradient simultaneously with RS measurements using a meteorological barometer. The RS rate (μmol CO2 m−2 s−1) was calculated according to the following equation:
where V is chamber volume (m3), P is air chamber pressure (Pa), R is gas constant (8.314 m3 Pa K−1 mol−1), S is soil surface area (m2), T is chamber air temperature (K), and ∂С/∂t is change of CO2 concentration inside the chamber over time (μmol mol−1 s−1). The final RS rate was corrected for the surface slope angle in degrees (θ): RS/cos θ (Xu and Shang, 2016).
2.3. Soil and vegetation analysis
Soil total C and N contents were determined by the dry combustion method using a CHNS analyzer (Leco Corp., USA). Dissolved organic carbon (DOC) and dissolved total nitrogen (DTN) were determined in 0.05 M K2SO4 extracts (5 g soil: 20 mL solution) using a Shimadzu TOC-VCPN analyzer (Shimadzu Corp., Japan) (Makarov et al., 2015). The pH was measured in a soil:water suspension (1:2.5 ratio) with a conductivity Sartorius Basic Meter (Germany). Microbial biomass carbon (MBC) was measured by the substrate-induced respiration method (Anderson and Domsch, 1978; International Organization for Standardization, 1997). Basal respiration (BR) was measured as the rate of soil CO2 release using gas chromatography (KrystaLLyuks-4,000 M; Meta-Chrom, Russia) (International Organization for Standardization, 2002). The MBC and BR values were determined under optimum hydrothermal conditions for the microorganisms: 22°C and 65% water holding capacity. Three hydrolytic C-and N-acquiring enzymes (β-D-glucosidase, chitinase, and leucine aminopeptidase) were measured using fluorogenic substrates (Marx et al., 2005; International Organization for Standardization/Technical Specification, 2019) as detailed by Ivashchenko et al. (2021). The soil samples for MBC, BR, and enzyme activities were pre-incubated at 22°C for 72 h (Loeppmann et al., 2016).
We chose characteristics of the herbaceous layer as a potential driving factor along the altitudinal gradient because: (i) it is a uniform ecological plant group for forest and grassland sites; (ii) despite a small contribution to overall forest biomass, this plant strata significantly affect N, P, K, and Mg cycles and mediate C dynamics at the ecosystem level (Gilliam, 2007). For each of the plots studied, the number of species (richness) and total projective coverage for graminoids and forbs were estimated (Ivashchenko et al., 2021). These functional plant groups were the most abundant and presented throughout the altitudinal gradient. In addition, the Shannon-Wiener plant diversity index (Hplant) for the herbaceous layer was calculated using the following equation:
where pi is the ratio of i species projective cover to total projective cover of all species per plot.
2.4. Statistical analysis
The spatial variability of the studied properties within forests and grasslands was quantified by the coefficient of variation (CV, %), calculated as the ratio of the standard deviation to the mean. Additionally, boxplots combined with dot plots were used to show the variability of RS across the studied sites. The significance of differences in variables between two or more independent groups was tested by Welch’s t-test or one-factor analysis of variance (ANOVA), respectively. Principal component analysis (PCA) was used to show variations and relationships between the studied environmental variables, as well as to illustrate the difference between forest and grassland sites. To reveal possible drivers of RS spatial variability within forests and grasslands, we used forward stepwise regression and path analysis. First, significant factors within each group of variables (soil physicochemical, microbial, and vegetation) were identified by forward stepwise regression using permutation tests. Afterward, the causal relation between the identified predictors was examined using path analysis. The pre-analysis and preparation of data included (1) checking the normality of distribution with a histogram plot and Shapiro–Wilk’s test, (2) log-transformation of some variables to achieve a normal distribution (i.e., C, N, C:N, DOC, BR, enzyme activity), and (3) centering and scaling to unit variance. All analyses and visualizations were performed in the R software system (version 4.1.2, RStudio Team, 2023) using the following packages: “ggplot2” for boxplots (Wickham, 2016), “FactoMineR” (Lê et al., 2008) and “factoextra” (Kassambara and Mundt, 2020) for PCA, “packfor” for forward stepwise regression (Dray et al., 2016), and “lavaan” for path analysis (Rosseel, 2012).
3. Results
3.1. Environmental characteristics in forests and grasslands
As expected, soil environments essentially differed between mountain forests and grasslands (Table 2). Grasslands located at higher altitudes than forests accumulated more organic matter (in the C, N, DOC, DTN forms) in soils characterized by a lower C:N ratio. Under both types of vegetation, the soils were strongly acidic (pH 5.0–5.2). Soil temperature in forests varied noticeably higher than in grasslands; its average values were 14.1, 11.3, 11.6, 13.7, and 13.0°C for mixed, fir, deciduous forests, subalpine and alpine grasslands, respectively. Conversely, water content was more homogenous in forests than in grasslands, averaging 39.3, 38.8, 39.4, 45.3 and 61.2% for the above listed ecosystems, respectively. Among the physicochemical properties studied in forest and grassland soils, the DTN content showed the highest spatial variability (CV 55–63%).
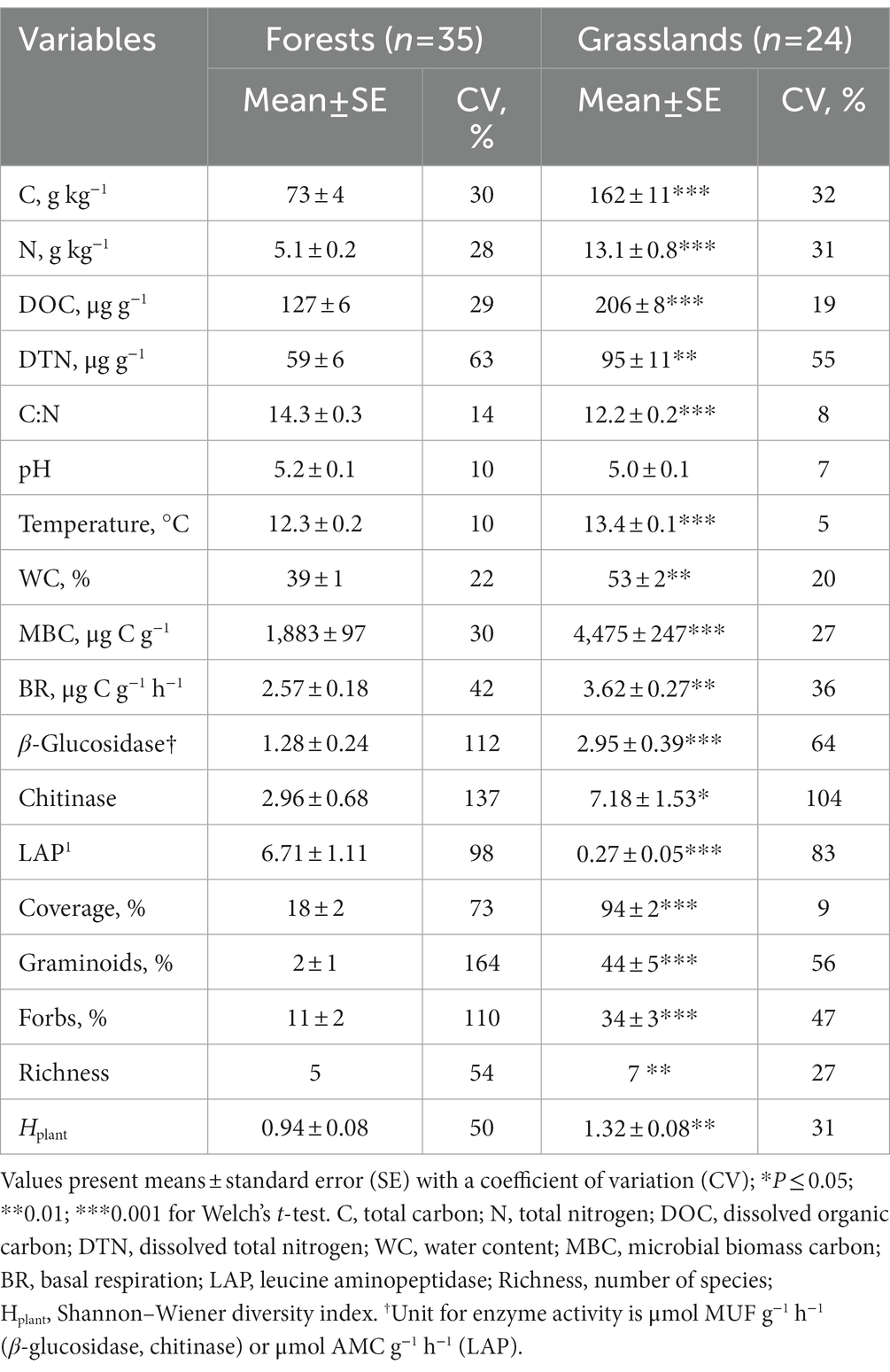
Table 2. Topsoil (0–10 cm) and vegetation (herbaceous layer) characteristics of forest and grassland sites along the studied mountain slope.
Differences in contents of total and dissolved forms of C and N between the forest and grassland soils led to significant differences in their microbial properties. Basically, indices of microbial activity in grasslands exceeded those in forests, except for leucine aminopeptidase (LAP) activity. Among the soil microbial properties, the activity of enzymes had the highest spatial variation within both forests and grasslands (CV 64–137%).
The forest understory (18% projective coverage) was formed mostly by forbs (11%). By contrast, the projective coverage (>90%) of grasslands almost equally consisted of graminoids and forbs. As a result, the number of species and the homogeneity of their distribution within a plot (Hplant) were higher in grasslands than in forests. In general, the spatial variation of all of the vegetation properties studied was considerably higher in forests than in grasslands (CV 50–164% vs. 9–56%).
The PCA analysis for all measured characteristics showed a clear grouping of studied sites by vegetation type, i.e., forests and grasslands (Figures 2A,B). Among the soil physicochemical parameters, the organic matter (in the C, N, DOC, DTN forms) and water contents mostly differed between forest and grassland soils (allocation of points along axis 1; r2 = 0.64–0.92) (Figure 2A). It is worth noting that the temperature and pH value of forest soils were characterized by extremely high variance (allocation of points along axis 2; r2 = 0.64–0.65), while for grassland soils they were more homogeneous. Equally high variation for soil microbial parameters was found within both forests and grasslands (Figure 2B). At the same time, soils under these vegetation types were separately grouped along axis 1, mainly according to MBC content and LAP activity (r2 = 0.76 and 0.59, respectively). In terms of herbaceous layer features, forest and grassland sites were clearly separated from each other mainly along axis 1, which is associated with variations in almost all of the studied characteristics (total and forbs projective coverage, number of species, Hplant; r2 = 0.60–0.76) (Figure 2C).
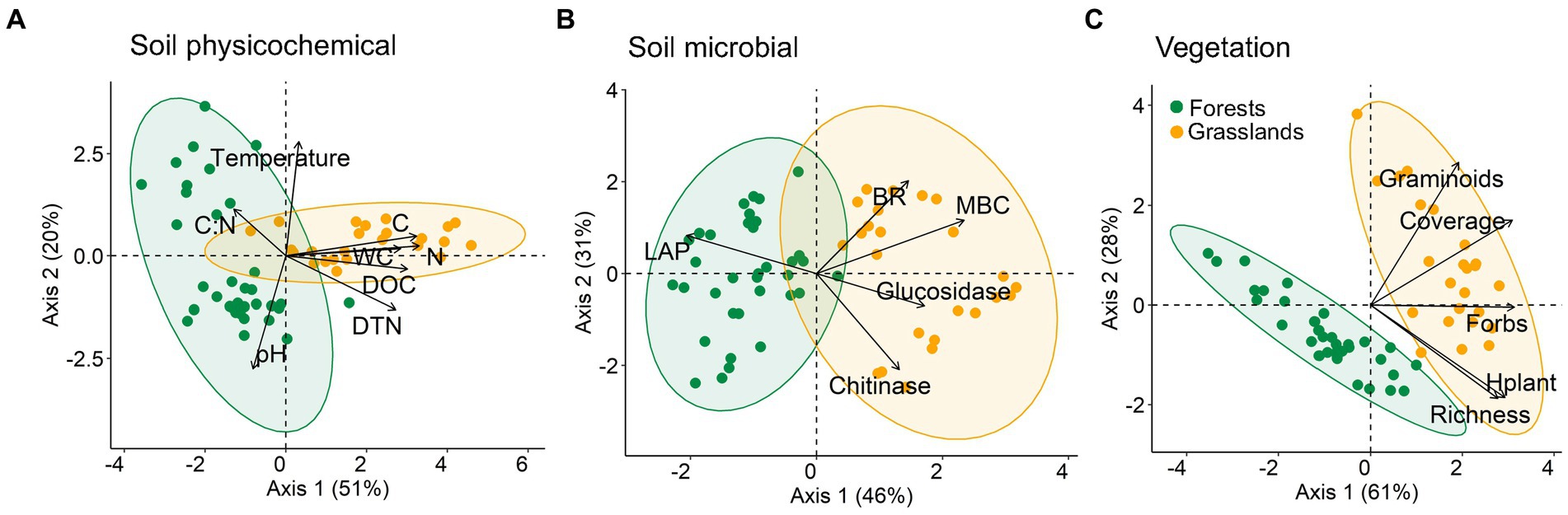
Figure 2. Principal component analysis (PCA) ordination triplot for soil physicochemical (A), soil microbial (B), and vegetation (C) characteristics of mountain forest and grassland sites. See variable abbreviations in Table 2.
3.2. Spatial variation of soil respiration and its possible drivers
The RS rate in the forests was, on average, half of that in the grasslands, reaching 3.7 and 7.3 μmol CO2 m−1 s−1, respectively (Figure 3). Within forests, RS increased significantly in the following order: fir < deciduous < mixed forests with average values of 3.0, 3.6 and 4.6 μmol CO2 m−1 s−1, respectively (p < 0.001 for one-way ANOVA). At the same time, RS did not differ between subalpine and alpine grasslands with average values of 8.1 and 6.4 μmol CO2 m−1 s−1, respectively (p = 0.09 for Welch’s t-test). In general, the spatial variation of RS in forests was lower than that in grasslands (CV 28 and 33%, respectively).
Difference in RS rate between forests and grasslands was associated with their divergence in soil (C, N, DOC, temperature, MBC and enzyme activities; R2 = 0.23–0.45, p ≤ 0.001) and vegetation properties (coverage and graminoid abundance; R2 = 0.49 and 0.55, p ≤ 0.001) (Supplementary Figure S1). Notably, the relationship with vegetation were stronger than with soil conditions, indicating its primary role in determining RS rate across ecosystem types.
Stepwise regression analysis showed that RS spatial variation in forests was best predicted by soil temperature, chitinase activity, and species richness of the herbaceous layer (explained variation 29–50%) (Table 3). The best predictors for grasslands included chitinase activity and graminoid abundance (explained variation 19 and 27%, respectively).
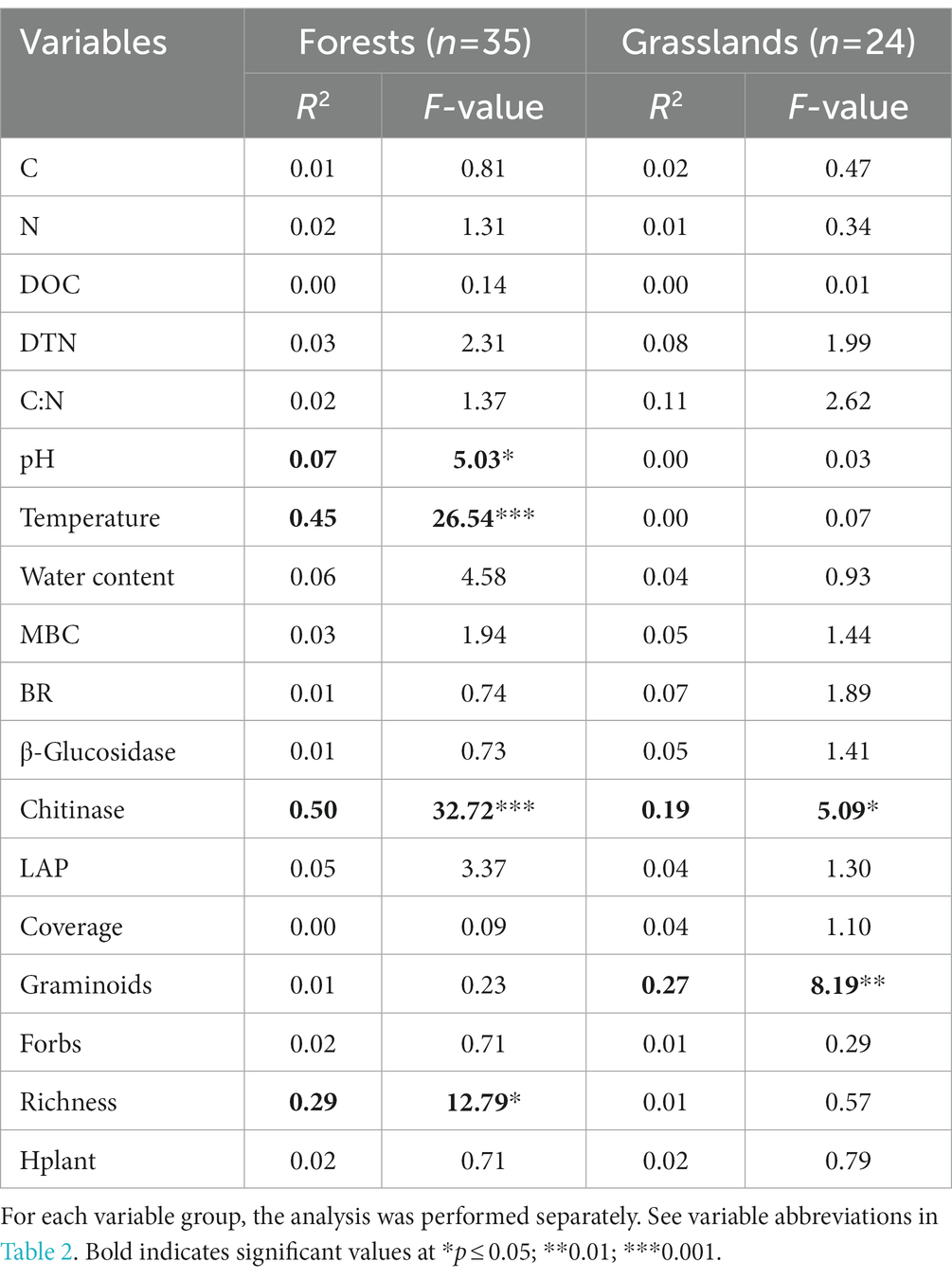
Table 3. Results of stepwise regression for Rs dependence on soil and vegetation characteristics in mountain forest and grassland sites.
Subsequent path analysis was used to explore the direct or indirect effects of the identified predictors on RS spatial variation. For forests, soil temperature was included in the path model both as (i) a direct factor, due to changing physical components of RS, e.g., gas pressure and its diffusive transport throughout pore space, and (ii) an indirect factor, acting through the regulation of chitinase activity. The plant species richness was considered only as an indirect factor since it determines the quality of the organic substrate entering the soil (root exudates, above-and below-ground litter), which, in turn, can affect chitinase activity. In general, the path model explained 50% of RS variation across forest sites (Figure 4A). In turn, soil temperature affected RS indirectly through the regulation of chitinase activity. Plant species richness did not affect chitinase, showing only a strong negative correlation with temperature.
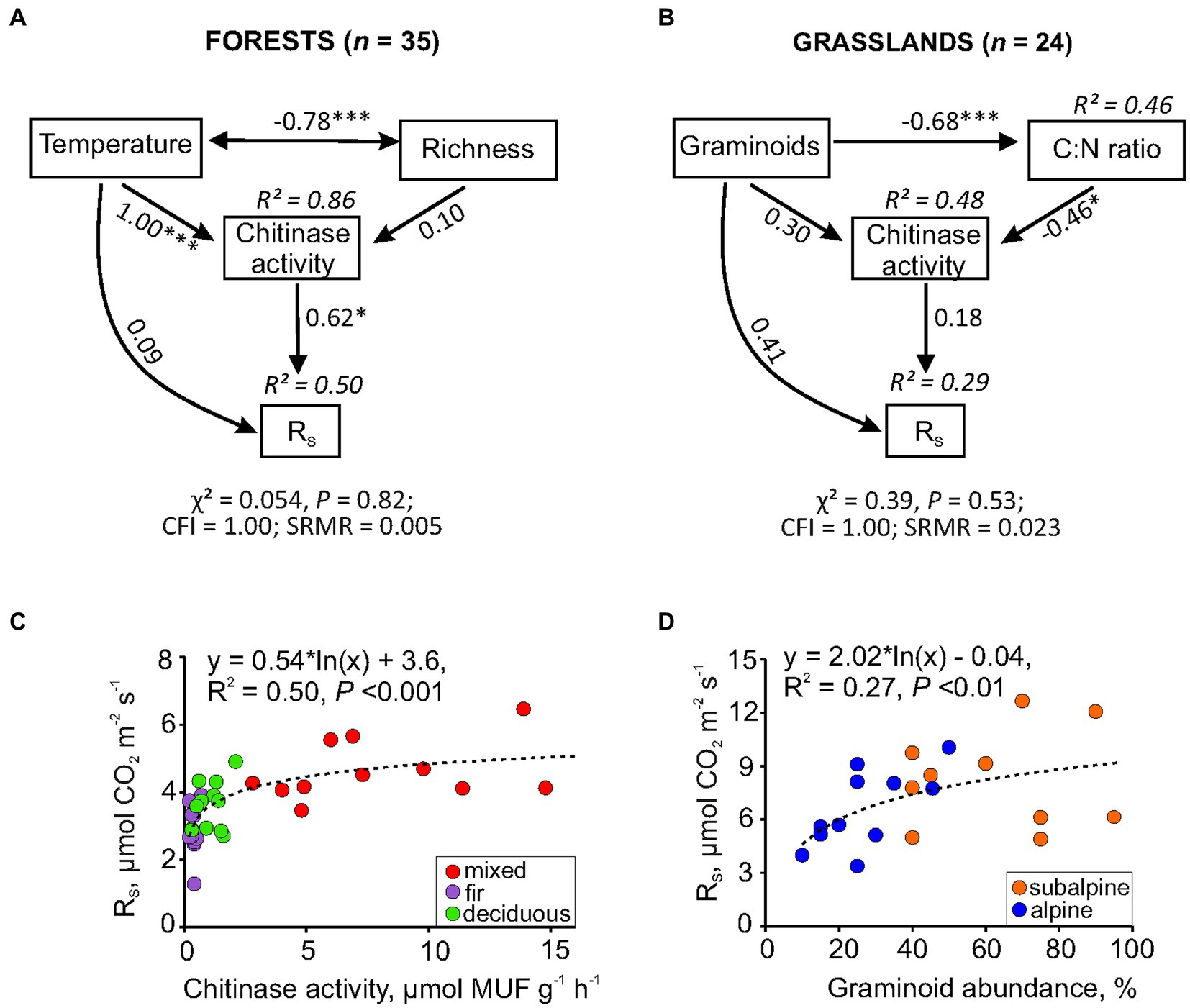
Figure 4. Path models and simple regression revealed the effects of soil (temperature, chitinase activity, C:N ratio) and vegetation factors (species richness, graminoid abundance) on RS spatial variability in mountain forests (A,C) and grasslands (B,D). In the path model, numbers within double-headed arrows are correlation coefficients between variables, numbers within one-way arrows are standardized path coefficients indicating the size effect of the causal relationship among variables (*p ≤ 0.05; **0.01; ***0.001); CFI, comparative fit index; SRMR, standardized root mean square residual.
For grasslands, chitinase activity, graminoid abundance, and, additionally, C:N ratio as their linking factor (indicated on the base correlation among all variables; see Supplementary Table S1) were included in the path model. As a result, the proposed model explained 29% of the RS variation in grasslands (Figure 4B). As expected, graminoids affected chitinase activity via changes in the soil C:N ratio. However, the significance of direct or indirect effects of graminoids and chitinase activity on RS was not confirmed by the model despite its overall satisfactory goodness-of-fit. Nevertheless, the largest standardized path coefficient (0.41) was found for the direct effect of graminoids on RS. This fact can likely explain the more pronounced effect of vegetation features on grassland RS variability than soil characteristics associated with the microbial decomposition of SOM (e.g., chitinase activity).
Summarizing the results of all of the analyses performed, one can conclude that the spatial changes in RS within mountain forests were mainly driven by soil chitinase activity alone, while spatial changes in RS in grasslands were associated with graminoid abundance. These relationships have been additionally demonstrated using a simple regression (Figures 4C,D).
4. Discussion
4.1. Spatial variability of soil respiration in mountain forests and meadows
Our results showed a higher RS rate in grasslands than in forests, which is consistent with the earlier reported difference between these ecosystem types (Raich and Tufekciogul, 2000; Kao and Chang, 2009). Meanwhile, intra-ecosystem RS variability was also higher in grasslands than in forests (Figure 3), which contradicts both the data reported for other mountain regions (Rodeghiero and Cescatti, 2008; Darenova et al., 2016) and our first hypothesis. On the one hand, this discrepancy can be attributed to the different time scales of measurements and, accordingly, different sources of RS variation. Specifically, our data show only the spatial variation in RS at individual time points without including its fluctuations during a season or year (i.e., combined spatio-temporal variability), unlike that in the above-mentioned studies. On the other hand, high altitude grasslands adopt accelerated photorespiration to complete growth and development within a short growing season (Streb and Cornic, 2012). Alpine species reach maximum photosynthetic capacity at a wide range of light intensity (i.e., 530–3,000 μmol photons m−2 s−1) with a higher interspecific variation than in lowland plants (Korner and Diemer, 1987). This phenomenon, along with a species-specific time lag between photosynthesis and root-derived CO2 efflux from soil (Kuzyakov and Gavrichkova, 2010), could have caused high spatial variability in RS within grasslands in our study.
4.2. Drivers of spatial variability in soil respiration in mountain forests and meadows
In our study, the drivers of spatial variability in RS were fundamentally different between forests and grasslands. In forests, both biotic and abiotic factors influenced the spatial variability of RS. A primary biotic driver was soil chitinase activity (Figure 4). The chitinase is one of the key enzymes of the C-and N-cycling, catalyzing degradation of chitin and peptidoglycan to carbohydrates and inorganic N available for soil microorganisms and plants (Andersson et al., 2004). With an increase in the availability of substrates for maintenance and growth of microorganisms and plants, their enzyme production would diminish, and vice versa (Allison et al., 2010). The relationship between chitinase activity and RS in forests could be explained by soil N deficiency, which is confirmed by significantly low contents of total and dissolved N forms, as well as by a high C:N ratio (Table 2). At the same time, the N-related enzyme LAP did not affect RS, unlike chitinase (Table 3). A reasonable explanation for this finding is that LAP catalyzes the hydrolysis of amino acids (Sinsabaugh et al., 2009), which is a less important source of N than amino sugars (i.e., chitin) contained in microbial necromass (Buckeridge et al., 2022). The main abiotic driver of the spatial variability in forest RS was soil temperature, which acted indirectly through the regulation of chitinase (Figure 4). The lower temperatures stimulate microbial necromass accumulation that would decompose primarily with increase in temperature, in particular by the chitinase (Bai et al., 2016; Wang et al., 2021). Moreover, a dominant portion of microbial necromass in forest topsoil is represented by fungi, which is an essential source of chitin and other amino sugars (Ni et al., 2020; Wang et al., 2021). Accordingly, in our study, a strong relationship between temperature and chitinase was found at colder soil conditions in forests compared to grasslands (Table 2). Thus, chitinase activity and its dependence on biotic and abiotic factors is critically important considering the inter-relations between C-and N-cycling in forest soils.
In grasslands, spatial variability in RS was related to vegetation structure, especially graminoid abundance, rather than chitinase activity (Table 3 and Figure 4). Overall, weaker relationships between potential drivers and RS in grasslands unlike in forests can be attributed to the difference in the heterogeneity of the above-and below-ground vegetation structure, biomass, and C allocation. Below-ground C allocation of photosynthates in grasslands is high, especially for some graminoids (Liu et al., 2021), which can eventually cause a considerable fraction of autotrophic (root-derived) respiration in RS (Hanson et al., 2000). Therefore, the relationship between RS and graminoid abundance was the most pronounced in studied grasslands. An important role of the vegetation structure and functioning in spatial heterogeneity of RS in grasslands was also confirmed by previous studies (e.g., Bahn et al., 2008; Fóti et al., 2016). At the same time, abiotic factors would be crucial for RS changes at unfavorable temperature and water conditions, e.g., in cold and drought periods. Therefore, we can expect an enormous variation in the importance of a number of spatial drivers of RS depending on season, as earlier reported by Shi and colleagues (Shi et al., 2020) for grassland ecosystems.
4.3. Prospects to study and forecast spatial variability of soil respiration
Monitoring RS is a complicated task due to its high variability in space and time (Rodeghiero and Cescatti, 2008). The spatial variation coefficient of RS can also be higher than that of RS temporal variation (Rodeghiero and Cescatti, 2008; Jiang et al., 2020). Therefore, the optimization of RS measurements to decrease uncertainties caused by spatial factors is an urgent matter. A sample size for RS measurements could be optimized using multiple probability simulation (Rodeghiero and Cescatti, 2008). However, for such a model, comprehensive information on RS spatial variability and its site-specific drivers is needed. Previous studies of RS spatial variability were combined with examinations of its temporal dynamics (Martin and Bolstad, 2005; Kosugi et al., 2007; Luan et al., 2012; Jiang et al., 2020). Under such conditions, spatial and temporal variability are overlapped since the measurements for different plots by closed chamber methods take several hours to even several days (Jiang et al., 2020). Consequently, high temporal variability in RS contributes to uncertainty in its spatial variability. The most accurate measurements are provided by long-term systems for multiplexed soil CO2 flux measurements, which are able to evaluate both spatial and temporal flux variations without overlapping across a large footprint (LI-COR, 2023). However, this measurement system is expensive and difficult to install on steep slopes. In our study, we sought to minimize RS temporal variability in mountain ecosystems, and thus focused on its spatial variability, which makes our research relatively novel for such kinds of field experiments. Simultaneous air sampling from closed chambers in five ecosystems took approximately 1 h and allowed for the analysis of CO2 concentrations on the same day despite the time spent for climbing and descent. However, extrapolating our results in time should be performed with care because seasonal variability in RS was not considered in the current set-up. Nevertheless, splitting the drivers for spatial patterns of RS between forests and grasslands constitutes a useful integration for C-cycle models (Jackson et al., 2017). Moreover, projecting our results to the consequences of climate change allowed us to assay not only C turnover changes but also drivers of its spatial variability at treeline shifts in mountains; therefore, further research in this direction appears to be a promising endeavor (Chen et al., 2022).
5. Conclusion
This study provides a comprehensive analysis of vegetation and soil factors along the altitudinal gradients to identify potential drivers of RS spatial variability. The results have shown principal differences in RS spatial patterns and their drivers between forest and grassland ecosystems. A higher spatial variability of this process was found in grasslands than in forests, which contradicts our first hypothesis. Soil microbial activity (i.e., chitinase) contributed greatly to spatial variability of RS in forests, while vegetation (graminoid abundance) was more considerable factors in grasslands, which fully confirmed our second hypothesis. These findings highlighted how CO2 fluxes in mountain forest and grassland ecosystems are regulated by a number of biotic and abiotic factors, emphasizing the most probable mechanisms of mutual regulations of C-and N-cycles.
Data availability statement
The raw data supporting the conclusions of this article will be made available by the authors, without undue reservation.
Author contributions
SS and KI: conception of the study. SS, KI, LO, AK, MK, and AZ: field measurements and laboratory analysis. SS, KI, LO, OG, SB, and IY: data interpretation, result discussion, and writing original draft. All authors contributed to the article and approved the submitted version.
Funding
Analytical processing and modeling were supported by state assignment No. 122111000095–8. Data survey was supported by Russian Science Foundation No. 22–74-10124.
Acknowledgments
We thank Kristina Radchenko, Alexander Tronin, Evgeny Ivashchenko, Anton Rogovoy, and Elvira Shtain for helping in field measurements and soil sampling. We are grateful to Vadim Smirnov for the discussion of the soil sampling design, to Viacheslav Vasenev for sharing equipment, to Valentin Lopes de Gerenyu for analyzing total carbon and nitrogen in the soil samples. We also thank the staff of Caucasian State Nature Biosphere Reserve for allowing us to carry out our investigation and technical support.
Conflict of interest
The authors declare that the research was conducted in the absence of any commercial or financial relationships that could be construed as a potential conflict of interest.
Publisher’s note
All claims expressed in this article are solely those of the authors and do not necessarily represent those of their affiliated organizations, or those of the publisher, the editors and the reviewers. Any product that may be evaluated in this article, or claim that may be made by its manufacturer, is not guaranteed or endorsed by the publisher.
Supplementary material
The Supplementary material for this article can be found online at: https://www.frontiersin.org/articles/10.3389/fmicb.2023.1165045/full#supplementary-material
References
Ahmad, M., Uniyal, S. K., Batish, D. R., Singh, H. P., Jaryan, V., Rathee, S., et al. (2020). Patterns of plant communities along vertical gradient in Dhauladhar Mountains in lesser Himalayas in North-Western India. Sci. Total Environ. 716:136919. doi: 10.1016/j.scitotenv.2020.136919
Allison, S. D., Wallenstein, M. D., and Bradford, M. A. (2010). Soil-carbon response to warming dependent on microbial physiology. Nat. Geosci. 3, 336–340. doi: 10.1038/ngeo846
Ananyeva, N. D., Sushko, S. V., Ivashchenko, K. V., and Vasenev, V. I. (2020). Soil microbial respiration in subtaiga and forest-steppe ecosystems of European Russia: field and laboratory approaches. Eurasian Soil Sci. 53, 1492–1501. doi: 10.1134/S106422932010004X
Anderson, J. P. E., and Domsch, K. H. (1978). A physiological method for the quantitative measurement of microbial biomass in soils. Soil Biol. Biochem. 10, 215–221. doi: 10.1016/0038-0717(78)90099-8
Andersson, M., Kjøller, A., and Struwe, S. (2004). Microbial enzyme activities in leaf litter, humus and mineral soil layers of European forests. Soil Biol. Biochem. 36, 1527–1537. doi: 10.1016/j.soilbio.2004.07.018
Bahn, M., Rodeghiero, M., Anderson-Dunn, M., Dore, S., Gimeno, C., Drösler, M., et al. (2008). Soil respiration in European grasslands in relation to climate and assimilate supply. Ecosystems 11, 1352–1367. doi: 10.1007/s10021-008-9198-0
Bai, Y., Eijsink, V. G. H., Kielak, A. M., van Veen, J. A., and de Boer, W. (2016). Genomic comparison of chitinolytic enzyme systems from terrestrial and aquatic bacteria: comparison of bacterial chitinolytic systems. Environ. Microbiol. 18, 38–49. doi: 10.1111/1462-2920.12545
Bardelli, T., Gómez-Brandón, M., Ascher-Jenull, J., Fornasier, F., Arfaioli, P., Francioli, D., et al. (2017). Effects of slope exposure on soil physico-chemical and microbiological properties along an altitudinal climosequence in the Italian Alps. Sci. Total Environ. 575, 1041–1055. doi: 10.1016/j.scitotenv.2016.09.176
Bolstad, P. V., Davis, K. J., Martin, J., Cook, B. D., and Wang, W. (2004). Component and whole-system respiration fluxes in northern deciduous forests. Tree Physiol. 24, 493–504. doi: 10.1093/treephys/24.5.493
Bond-Lamberty, B., and Thomson, A. (2010). Temperature-associated increases in the global soil respiration record. Nature 464, 579–582. doi: 10.1038/nature08930
Bu, X., Ruan, H., Wang, L., Ma, W., Ding, J., and Yu, X. (2012). Soil organic matter in density fractions as related to vegetation changes along an altitude gradient in the Wuyi Mountains, southeastern China. Appl. Soil Ecol. 52, 42–47. doi: 10.1016/j.apsoil.2011.10.005
Buckeridge, K. M., Creamer, C., and Whitaker, J. (2022). Deconstructing the microbial necromass continuum to inform soil carbon sequestration. Funct. Ecol. 36, 1396–1410. doi: 10.1111/1365-2435.14014
Canedoli, C., Ferrè, C., Abu El Khair, D., Comolli, R., Liga, C., Mazzucchelli, F., et al. (2020). Evaluation of ecosystem services in a protected mountain area: soil organic carbon stock and biodiversity in alpine forests and grasslands. Ecosyst. Serv. 44:101135. doi: 10.1016/j.ecoser.2020.101135
Chen, W., Ding, H., Li, J., Chen, K., and Wang, H. (2022). Alpine treelines as ecological indicators of global climate change: who has studied? What has been studied? Ecol. Inform. 70:101691. doi: 10.1016/j.ecoinf.2022.101691
Chen, Z., Xu, Y., Fan, J., Yu, H., and Ding, W. (2017). Soil autotrophic and heterotrophic respiration in response to different N fertilization and environmental conditions from a cropland in Northeast China. Soil Biol. Biochem. 110, 103–115. doi: 10.1016/j.soilbio.2017.03.011
Darenova, E., Pavelka, M., and Macalkova, L. (2016). Spatial heterogeneity of CO2 efflux and optimization of the number of measurement positions. Eur. J. Soil Biol. 75, 123–134. doi: 10.1016/j.ejsobi.2016.05.004
Dray, S., Legendre, P., and Blanchet, G. (2016). Packfor: Forward selection with permutation (Canoco p.46). R Package Version 0.0-8/r136. Available at: https://R-Forge.R-project.org/projects/sedar/
Ettinger, A. K., Chuine, I., Cook, B. I., Dukes, J. S., Ellison, A. M., Johnston, M. R., et al. (2019). How do climate change experiments alter plot-scale climate? Ecol. Lett. 22, 748–763. doi: 10.1111/ele.13223
Fóti, S., Balogh, J., Herbst, M., Papp, M., Koncz, P., Bartha, S., et al. (2016). Meta-analysis of field scale spatial variability of grassland soil CO2 efflux: interaction of biotic and abiotic drivers. Catena 143, 78–89. doi: 10.1016/j.catena.2016.03.034
Frey, S. D., Lee, J., Melillo, J. M., and Six, J. (2013). The temperature response of soil microbial efficiency and its feedback to climate. Nat. Clim. Chang. 3, 395–398. doi: 10.1038/nclimate1796
Gilliam, F. S. (2007). The ecological significance of the herbaceous layer in temperate forest ecosystems. Bioscience 57, 845–858. doi: 10.1641/B571007
Hanson, P. J., Edwards, N. T., Garten, C. T., and Andrews, J. A. (2000). Separating root and soil microbial contributions to soil respiration: a review of methods and observations. Biogeochemistry 48, 115–146. doi: 10.1023/A:1006244819642
Hansson, A., Dargusch, P., and Shulmeister, J. (2021). A review of modern treeline migration, the factors controlling it and the implications for carbon storage. J. Mt. Sci. 18, 291–306. doi: 10.1007/s11629-020-6221-1
He, Y., Wang, Y., Jiang, Y., Yin, G., Cao, S., Liu, X., et al. (2023). Drivers of soil respiration and nitrogen mineralization change after litter management at a subtropical Chinese sweetgum tree plantation. Soil Use Manag. 39, 92–103. doi: 10.1111/sum.12823
Hursh, A., Ballantyne, A., Cooper, L., Maneta, M., Kimball, J., and Watts, J. (2017). The sensitivity of soil respiration to soil temperature, moisture, and carbon supply at the global scale. Glob. Change Biol. 23, 2090–2103. doi: 10.1111/gcb.13489
International Organization for Standardization (1997). Soil quality – Determination of soil microbial biomass – Part 1: substrate-induced respiration method (ISO standard no. 14240–1:1997). Geneva: International Organization for Standardization.
International Organization for Standardization (2002). Soil quality – Laboratory methods for determination of microbial soil respiration (ISO standard no. 16072:2002). Geneva: International Organization for Standardization.
International Organization for Standardization/Technical Specification (2019). Soil quality – Measurement of enzyme activity patterns in soil samples using fluorogenic substrates in micro-well plates (ISO standard no. 22939:2019). Geneva: International Organization for Standardization.
Ivashchenko, K., Sushko, S., Selezneva, A., Ananyeva, N., Zhuravleva, A., Kudeyarov, V., et al. (2021). Soil microbial activity along an altitudinal gradient: vegetation as a main driver beyond topographic and edaphic factors. Appl. Soil Ecol. 168:104197. doi: 10.1016/j.apsoil.2021.104197
Jackson, R. B., Lajtha, K., Crow, S. E., Hugelius, G., Kramer, M. G., and Piñeiro, G. (2017). The ecology of soil carbon: pools, vulnerabilities, and biotic and abiotic controls. Annu. Rev. Ecol. Evol. Syst. 48, 419–445. doi: 10.1146/annurev-ecolsys-112414-054234
Jiang, Y., Zhang, B., Wang, W., Li, B., Wu, Z., and Chu, C. (2020). Topography and plant community structure contribute to spatial heterogeneity of soil respiration in a subtropical forest. Sci. Total Environ. 733:139287. doi: 10.1016/j.scitotenv.2020.139287
Kao, W. Y., and Chang, K. W. (2009). Soil CO2 efflux from a mountainous forest-grassland ecosystem in Central Taiwan. Bot. Stud. 50, 337–342.
Kapos, V., Rhind, J., Edwards, M., Price, M. F., and Ravilious, C. (2000). “Developing a map of the world’s mountain forests”, in Forests in sustainable mountain development: a state of knowledge report for 2000. eds. M. F. Price and N. Butt (Wallingford: CABI Pub.), 4–9. doi: 10.1079/9780851994468.0004
Kassambara, A., and Mundt, F. (2020). Factoextra: extract and visualize the results of multivariate data analyses. R package version 1.0.7. Available at: http://www.sthda.com/english/rpkgs/factoextra
Katayama, A., Kume, T., Komatsu, H., Ohashi, M., Nakagawa, M., Yamashita, M., et al. (2009). Effect of forest structure on the spatial variation in soil respiration in a Bornean tropical rainforest. Agric. For. Meteorol. 149, 1666–1673. doi: 10.1016/j.agrformet.2009.05.007
Korner, C., and Diemer, M. (1987). In situ photosynthetic responses to light, temperature and carbon dioxide in herbaceous plants from low and high altitude. Funct. Ecol. 1:179. doi: 10.2307/2389420
Kosugi, Y., Mitani, T., Itoh, M., Noguchi, S., Tani, M., Matsuo, N., et al. (2007). Spatial and temporal variation in soil respiration in a southeast Asian tropical rainforest. Agric. For. Meteorol. 147, 35–47. doi: 10.1016/j.agrformet.2007.06.005
Kudeyarov, V., and Kurganova, I. (2005). Respiration of Russian soils: database analysis, long-term monitoring, and general estimates. Eurasian Soil Sci. 38, 983–992.
Kudeyarov, V. N., Zavarzin, G. A., Blagodatsky, S. A., Borisov, A. V., Voronin, P. U., Demkin, V. A., et al. (2007). Pools and fluxes of carbon in terrestrial ecosystems of Russia. Moscow: Nauka
Kutsch, W. L., Bahn, M., and Heinemeyer, A. (Eds.) (2010). Soil carbon dynamics: an integrated methodology. 1st Edn. Cambridge: Cambridge University Press.
Kuzyakov, Y., and Gavrichkova, O. (2010). Time lag between photosynthesis and carbon dioxide efflux from soil: a review of mechanisms and controls. Glob. Change Biol. 16, 3386–3406. doi: 10.1111/j.1365-2486.2010.02179.x
Lê, S., Josse, J., and Husson, F. (2008). FactoMineR: An R package for multivariate analysis. J. Stat. Softw. 25, 1–18. doi: 10.18637/jss.v025.i01
Leifeld, J., Bassin, S., Conen, F., Hajdas, I., Egli, M., and Fuhrer, J. (2013). Control of soil pH on turnover of belowground organic matter in subalpine grassland. Biogeochemistry 112, 59–69. doi: 10.1007/s10533-011-9689-5
LI-COR (2023). Long-term systems. Available at: https://www.licor.com/env/products/soil_flux/long-term (Accessed October 03, 2019).
Lin, J. C., Mallia, D. V., Wu, D., and Stephens, B. B. (2017). How can mountaintop CO2 observations be used to constrain regional carbon fluxes? Atmospheric Chem. Phys. 17, 5561–5581. doi: 10.5194/acp-17-5561-2017
Liu, Y., Xu, M., Li, G., Wang, M., Li, Z., and De Boeck, H. J. (2021). Changes of aboveground and belowground biomass allocation in four dominant grassland species across a precipitation gradient. Front. Plant Sci. 12:650802. doi: 10.3389/fpls.2021.650802
Liu, X. P., Zhang, W. J., Hu, C. S., and Tang, X. G. (2014). Soil greenhouse gas fluxes from different tree species on Taihang Mountain, North China. Biogeosciences 11, 1649–1666. doi: 10.5194/bg-11-1649-2014
Loeppmann, S., Semenov, M., Blagodatskaya, E., and Kuzyakov, Y. (2016). Substrate quality affects microbial-and enzyme activities in rooted soil. J. Plant Nutr. Soil Sci. 179, 39–47. doi: 10.1002/jpln.201400518
Luan, J., Liu, S., Zhu, X., Wang, J., and Liu, K. (2012). Roles of biotic and abiotic variables in determining spatial variation of soil respiration in secondary oak and planted pine forests. Soil Biol. Biochem. 44, 143–150. doi: 10.1016/j.soilbio.2011.08.012
Luo, Y., and Zhou, X. (2006). Soil respiration and the environment. Amsterdam; Boston: Elsevier Academic Press.
Makarov, M. I., Malysheva, T. I., Menyailo, O. V., Soudzilovskaia, N. A., Van Logtestijn, R. S. P., and Cornelissen, J. H. C. (2015). Effect of K2SO4 concentration on extractability and isotope signature (δ13 C and δ15 N) of soil C and N fractions: extractability, δ13 C and δ15 N of soil C and N fractions. Eur. J. Soil Sci. 66, 417–426. doi: 10.1111/ejss.12243
Martin, J. G., and Bolstad, P. V. (2005). Annual soil respiration in broadleaf forests of northern Wisconsin: influence of moisture and site biological, chemical, and physical characteristics. Biogeochemistry 73, 149–182. doi: 10.1007/s10533-004-5166-8
Marx, M.-C., Kandeler, E., Wood, M., Wermbter, N., and Jarvis, S. C. (2005). Exploring the enzymatic landscape: distribution and kinetics of hydrolytic enzymes in soil particle-size fractions. Soil Biol. Biochem. 37, 35–48. doi: 10.1016/j.soilbio.2004.05.024
Mayer, M., Prescott, C. E., Abaker, W. E. A., Augusto, L., Cécillon, L., Ferreira, G. W. D., et al. (2020). Tamm review: influence of forest management activities on soil organic carbon stocks: a knowledge synthesis. For. Ecol. Manag. 466:118127. doi: 10.1016/j.foreco.2020.118127
Ni, X., Liao, S., Tan, S., Peng, Y., Wang, D., Yue, K., et al. (2020). The vertical distribution and control of microbial necromass carbon in forest soils. Glob. Ecol. Biogeogr. 29, 1829–1839. doi: 10.1111/geb.13159
Pressey, R. L., Cabeza, M., Watts, M. E., Cowling, R. M., and Wilson, K. A. (2007). Conservation planning in a changing world. Trends Ecol. Evol. 22, 583–592. doi: 10.1016/j.tree.2007.10.001
Qi, Y. C., Dong, Y. S., Jin, Z., Peng, Q., Xiao, S. S., and He, Y. T. (2010). Spatial heterogeneity of soil nutrients and respiration in the desertified grasslands of inner Mongolia, China. Pedosphere 20, 655–665. doi: 10.1016/S1002-0160(10)60055-0
Raich, J. W., and Tufekciogul, A. (2000). Vegetation and soil respiration: correlations and controls. Biogeochemistry 48, 71–90. doi: 10.1023/A:1006112000616
Reichstein, M., Rey, A., Freibauer, A., Tenhunen, J., Valentini, R., Banza, J., et al. (2003). Modeling temporal and large-scale spatial variability of soil respiration from soil water availability, temperature and vegetation productivity indices. Glob. Biogeochem. Cycles 17, 15-1–15-15. doi: 10.1029/2003GB002035
Reside, A. E., Beher, J., Cosgrove, A. J., Evans, M. C., Seabrook, L., Silcock, J. L., et al. (2017). Ecological consequences of land clearing and policy reform in Queensland. Pac. Conserv. Biol. 23:219. doi: 10.1071/PC17001
Rodeghiero, M., and Cescatti, A. (2008). Spatial variability and optimal sampling strategy of soil respiration. For. Ecol. Manag. 255, 106–112. doi: 10.1016/j.foreco.2007.08.025
Rosseel, Y. (2012). Lavaan: An R package for structural equation modeling. J. Stat. Softw. 48, 1–36. doi: 10.18637/jss.v048.i02
RStudio Team (2023). RStudio: integrated development for R. RStudio, PBC, Boston, MA. Available at: http://www.rstudio.com/]
Schaufler, G., Kitzler, B., Schindlbacher, A., Skiba, U., Sutton, M. A., and Zechmeister-Boltenstern, S. (2010). Greenhouse gas emissions from European soils under different land use: effects of soil moisture and temperature. Eur. J. Soil Sci. 61, 683–696. doi: 10.1111/j.1365-2389.2010.01277.x
Schlesinger, W. H., and Andrews, J. A. (2000). Soil respiration and the global carbon cycle. Biogeochemistry 48, 7–20. doi: 10.1023/A:1006247623877
Shi, B., Hu, G., Henry, H. A. L., Stover, H. J., Sun, W., Xu, W., et al. (2020). Temporal changes in the spatial variability of soil respiration in a meadow steppe: the role of abiotic and biotic factors. Agric. For. Meteorol. 287:107958. doi: 10.1016/j.agrformet.2020.107958
Shi, B., Xu, W., Zhu, Y., Wang, C., Loik, M. E., and Sun, W. (2019). Heterogeneity of grassland soil respiration: antagonistic effects of grazing and nitrogen addition. Agric. For. Meteorol. 268, 215–223. doi: 10.1016/j.agrformet.2019.01.028
Sinsabaugh, R. L., Hill, B. H., and Follstad Shah, J. J. (2009). Ecoenzymatic stoichiometry of microbial organic nutrient acquisition in soil and sediment. Nature 462, 795–798. doi: 10.1038/nature08632
Streb, P., and Cornic, G. (2012). “Photosynthesis and antioxidative protection in alpine herbs” in Plants in alpine regions. ed. C. Lütz (Vienna: Springer Vienna), 75–97.
Subke, J. A., Inglima, I., and Francesca Cotrufo, M. (2006). Trends and methodological impacts in soil CO2 efflux partitioning: a metaanalytical review. Glob. Change Biol. 12, 921–943. doi: 10.1111/j.1365-2486.2006.01117.x
Sushko, S. V., Ananyeva, N. D., Ivashchenko, K. V., and Kudeyarov, V. N. (2019). Soil CO2 emission, microbial biomass, and basal respiration of Chernozems under different land uses. Eurasian Soil Sci. 52, 1091–1100. doi: 10.1134/S1064229319090096
Sushko, S., Ananyeva, N., Ivashchenko, K., Vasenev, V., and Kudeyarov, V. (2019). Soil CO2 emission, microbial biomass, and microbial respiration of woody and grassy areas in Moscow (Russia). J. Soils Sediments 19, 3217–3225. doi: 10.1007/s11368-018-2151-8
Wang, B., An, S., Liang, C., Liu, Y., and Kuzyakov, Y. (2021). Microbial necromass as the source of soil organic carbon in global ecosystems. Soil Biol. Biochem. 162:108422. doi: 10.1016/j.soilbio.2021.108422
Wiaux, F., Vanclooster, M., and Van Oost, K. (2015). Vertical partitioning and controlling factors of gradient-based soil carbon dioxide fluxes in two contrasted soil profiles along a loamy hillslope. Biogeosciences 12, 4637–4649. doi: 10.5194/bg-12-4637-2015
Wickham, H. (2016). Ggplot2: Elegant graphics for data analysis. 2nd ed. Cham: Springer International Publishing
Keywords: soil CO2 emission, altitudinal gradient, forest and grassland ecosystems, soil properties, plant community structure
Citation: Sushko S, Ovsepyan L, Gavrichkova O, Yevdokimov I, Komarova A, Zhuravleva A, Blagodatsky S, Kadulin M and Ivashchenko K (2023) Contribution of microbial activity and vegetation cover to the spatial distribution of soil respiration in mountains. Front. Microbiol. 14:1165045. doi: 10.3389/fmicb.2023.1165045
Edited by:
Yu Luo, Zhejiang University, ChinaReviewed by:
Qin Peng, Chinese Academy of Sciences (CAS), ChinaYing Wang, Northwest A&F University, China
Copyright © 2023 Sushko, Ovsepyan, Gavrichkova, Yevdokimov, Komarova, Zhuravleva, Blagodatsky, Kadulin and Ivashchenko. This is an open-access article distributed under the terms of the Creative Commons Attribution License (CC BY). The use, distribution or reproduction in other forums is permitted, provided the original author(s) and the copyright owner(s) are credited and that the original publication in this journal is cited, in accordance with accepted academic practice. No use, distribution or reproduction is permitted which does not comply with these terms.
*Correspondence: Kristina Ivashchenko, aXZhc2hjaGVua28ua3ZAZ21haWwuY29t