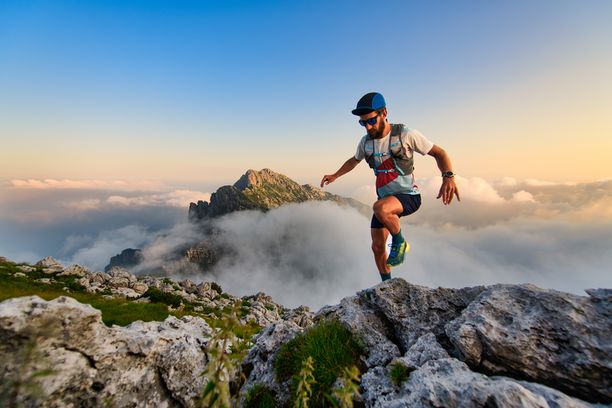
95% of researchers rate our articles as excellent or good
Learn more about the work of our research integrity team to safeguard the quality of each article we publish.
Find out more
MINI REVIEW article
Front. Microbiol., 30 March 2023
Sec. Systems Microbiology
Volume 14 - 2023 | https://doi.org/10.3389/fmicb.2023.1163741
This article is part of the Research TopicArtificial Intelligence in Forensic MicrobiologyView all 10 articles
Forensic microbiology has been widely used in the diagnosis of causes and manner of death, identification of individuals, detection of crime locations, and estimation of postmortem interval. However, the traditional method, microbial culture, has low efficiency, high consumption, and a low degree of quantitative analysis. With the development of high-throughput sequencing technology, advanced bioinformatics, and fast-evolving artificial intelligence, numerous machine learning models, such as RF, SVM, ANN, DNN, regression, PLS, ANOSIM, and ANOVA, have been established with the advancement of the microbiome and metagenomic studies. Recently, deep learning models, including the convolutional neural network (CNN) model and CNN-derived models, improve the accuracy of forensic prognosis using object detection techniques in microorganism image analysis. This review summarizes the application and development of forensic microbiology, as well as the research progress of machine learning (ML) and deep learning (DL) based on microbial genome sequencing and microbial images, and provided a future outlook on forensic microbiology.
Microorganisms, including viruses, bacteria, and fungi, are ubiquitously distributed and form diverse and rich communities (Oliveira and Amorim, 2018). Forensic microbiology is a discipline that deals with the study of microbiology in the context of forensic investigation (Kuiper, 2016). Its applications mainly focus on the diagnosis of cause of death, inference of crime location, estimation of postmortem interval (PMI), and individual identification.
Numerous studies have explored the application of microorganisms in forensic practice. Certain specific microorganisms have been proven to contribute to the determination of various causes of death, including drowning (Lucci et al., 2008; Kakizaki et al., 2010; Tie et al., 2010; Huys et al., 2012; Lee et al., 2016, 2017; Rácz et al., 2016; Wang et al., 2020), poisoning (Butzbach, 2010; Skopp, 2010; Grad et al., 2012; Han et al., 2012; Butzbach et al., 2013; Sastre et al., 2017), and hospital-acquired infections (Klevens et al., 2007; Sodhi et al., 2013; Lax et al., 2015; Khan et al., 2017). Considering the distinctive microbial community in soil obtained from a crime scene and other intermediary sites, soil microbiome provides evidence for inferring the geolocation (Meyers and Foran, 2008; Costello et al., 2009; Jesmok et al., 2016; Habtom et al., 2019; Yang et al., 2021). Moreover, since microorganisms contribute to postmortem decomposition and have a succession that follows a predictable pattern (Hauther et al., 2015; Javan et al., 2016; DeBruyn and Hauther, 2017; Lutz et al., 2020; Scott et al., 2020), forensic researchers began to explore the feasibility of microbiome succession for inferring the postmortem interval over the past few years. Some studies used machine learning and other technologies to establish models for PMI estimation based on microorganisms, which actually improve the accuracy of PMI inference (Pechal et al., 2014; Johnson et al., 2016; Liu et al., 2020; Zou et al., 2020). In addition, the microbiome composition in humans varies in body location (Kong and Segre, 2012); host characteristics, such as sex, age, and lifestyle (Ross et al., 2017); and skin care status (Bouslimani et al., 2019). Therefore, individuals may be identified using skin, hair, and body fluid microbiomics (Lax et al., 2014; Bäckhed et al., 2015; Wu et al., 2016; Schmedes et al., 2017; Willis et al., 2018).
In this review, we aim to summarize the developmental progress of forensic microbiology from classical methods to high-throughput data combined with artificial intelligence technologies and discuss the outlook for the future.
Forensic microbiology first gained global recognition in 2001 as a result of the Bacillus anthracis attacks through the USA postal service. In previous studies on forensic microbiology, forensic microbiological technologies were not specifically described, except for agar cultures for bacteria and fungi combined with PCR for certain microorganisms (Aoyagi et al., 2009; Huys et al., 2012; Uchiyama et al., 2012; Tuomisto et al., 2013; Can et al., 2014; Hauther et al., 2015; Yu et al., 2021a,b). To date, though more than 2,460 different species are presented in The Ribosomal Database Project stores (Maidak et al., 2000), most microbes in the environment have not been described and accessed for biotechnology. Few viable bacteria can be cultivated on artificial media (Kimura and Nobutada, 2006; Cecchini et al., 2012). Furthermore, traditional microbial culture highly relies on culture conditions and has limitations in the analysis of microbial community composition.
The 16/18S rRNA is the common marker for microbial classification and identification. Fluorescence in situ hybridization (FISH) (Langendijk, 1995) and denaturing gradient gel electrophoresis (DGGE) (Muyzer et al., 1993) are used to detect the specific 16S rRNA and gain access to unculturable microbes. Sanger sequencing technology (Sanger et al., 1977), also known as first-generation sequencing technology, allows for the wide use of 16S rRNA gene sequencing in bacterial taxonomy and leads to the discovery of a large number of new microbial taxa. Next-generation sequencing technology (NGS) can measure tens of thousands to millions of DNA simultaneously and can provide a high-throughput microbiome database (Metzker, 2010). With the development of sequencing technology, third-generation sequencing technology has more advantages in the study of community diversity due to its ultra-long sequencing reading (Franzén et al., 2015). However, the 16S rRNA test results are assembled into operational taxonomic units (OTUs). Most high-throughput sequencing microbiome data could not be identified and classified due to the limitation of the referenced genomes and genetic datasets (Yooseph et al., 2013; Afshinnekoo et al., 2015). Using targeted 16S rRNA with short amplifiers could not achieve reliable resolution at the species level, and full-length 16S rRNA sequences do not necessarily reduce this limitation (Forney et al., 2004). In addition, whole-genome shotgun (WGS) targets all gene content in microbial ecosystems and can differentiate microbial species and taxa to a greater extent than 16S rRNA amplicons (Schloissnig et al., 2013; Franzosa et al., 2015).
Though the expanding role of NGS and WGS combined with artificial intelligence will likely be a routine tool in forensic microbiology, the isolation, detection, and confirmation of specific microbes (pathogens or colonies) and the use of nucleic acid sequencing remain less relevant in resolving forensic challenges. Some investigators suggest the use of nanotechnology to design biosensors for the identification of foreign pathogens. Recently, microbial computer image analysis technology combined with machine learning and deep learning elucidated the identification of specific microbiomes (Ma et al., 2022). Moreover, some challenges related to standardization, validation, and procedural and bioinformatic pipelines persist in the study of forensic microbiology (Roesch et al., 2009; Lauber et al., 2010; Wu et al., 2010). Further advancements in technology will continue to improve the application of microbiology in forensic medicine. The trend in forensic microbiology is summarized in Figure 1.
Establishing a prognostic model and predicting the dynamic succession of microbial communities have improved research in forensic microbiology. High-throughput microbiotic datasets combined with machine learning extend the application of microbiomes in forensic issues. Traditional statistical methods could only determine the general composition of a microbial community and its basic succession, whereas machine learning models achieve quantitative analysis and accurate prediction (Zou et al., 2020). Currently, different machine learning models, including random forest (RF), support vector machine (SVM), and AdaBoost, are used in the field of forensic microbiology, and they have become a promising strategy for several forensic events.
One main use of forensic microbiology is human individual identification (Fierer et al., 2010; Lax et al., 2015; Leake et al., 2016; Williams and Gibson, 2017; Yang et al., 2019). In cases without blood or tissue evidence, a microbiological approach provides a breakdown of situations with the detection of the microbiome on skin, saliva, hair, or objects that have been touched. UniFrac metric (Fierer et al., 2010) and hierarchical clustering (Leake et al., 2016) are used in determining skin-associated bacteria and salivary microbiomes. In an RF model used for investigating pubic hair bacteria, the accuracy of individual identification was 2.7 ± 5.8%, and the gender accuracy was 1.7 ± 5.2% (Williams and Gibson, 2017).
In addition, machine learning can be used for PMI estimation in microbial forensics (Johnson et al., 2016; Liu et al., 2020, 2021; Cao et al., 2021; Cartozzo et al., 2021; Randall et al., 2021; Zhang et al., 2021; Kaszubinski et al., 2022; Zhao et al., 2022). RF models are widely used for PMI estimation and postmortem submersion interval (PMSI) diagnosis (Metcalf et al., 2013; Pechal et al., 2014; Cartozzo et al., 2021; Randall et al., 2021; Zhang et al., 2021; Kaszubinski et al., 2022; Zhao et al., 2022). In 2013, Metcalf et al. (2013) first proposed the concept of a “microbial clock” to infer PMI by setting up regression models using an RF. In addition, artificial neural network (ANN) models are used to infer PMI based on the microbiotic OTU data. In 2020, Liu et al. combined microbial community characterization and machine learning algorithms (RF, SVM, and ANN) to investigate microbial succession patterns during corpse decomposition and estimate PMI with a mean absolute error (MAE) of 1.5 ± 0.8 h within 24 h.
Available evidence demonstrates that forensic microbiology with machine learning can be used to infer geolocation to a certain extent (McNulty et al., 2004; Kersulyte et al., 2010; Blaser et al., 2013; Nagasawa et al., 2013; Tyakht et al., 2013; Escobar et al., 2014; Li et al., 2014; Suzuki and Worobey, 2014; Huang et al., 2020). Human gut microbiota plays a significant role in geolocation inference, which is supported by correlations based on traditional statistical methods, such as Wilcoxon's rank-sum test (Suzuki and Worobey, 2014) and analysis of similarities (ANOSIM) (Escobar et al., 2014). In 2014, UniFrac, network, and ANOSIM were used to analyze the human saliva microbiome. The variance between individuals was 6.75–10.21% (Li et al., 2014). In 2020, Huang et al. (2020) applied a machine learning framework to determine geolocations with an accuracy of 86%.
As previously stated, machine learning can be used to establish models for tissue/body fluid identification (Costello et al., 2009; Benschop et al., 2012; Lopez et al., 2019, 2020; Salzmann et al., 2021). Lopez et al. (2019) used ANN to identify different human epithelial biomaterials with AUC values of 0.99, 0.99, and 1 for skin, oral, and vaginal secretions, respectively. Later in 2020, deep neural network (DNN) was additionally used to identify human forensically relevant blood samples successfully (Lopez et al., 2020).
Investigative forensic microbiology with machine learning has practical applications in other aspects. Concerns related to drowning diagnosis (Huys et al., 2012; Wang et al., 2020), postmortem toxicology (Kaszubinski et al., 2020), and disease diagnosis are summarized in Table 1. Although the expanding effect of machine learning on NGS and WGS databases is without much doubt, some models derived from different machine learning models still mean less to the actual situation, which limits their application in forensic practice. The current challenges of machine learning are insufficient training samples and an imperfect microbial databank.
Compared with machine learning, deep learning can realize automatic feature learning through advanced network structure by combining several simple modules (Lecun et al., 2015; Ma et al., 2022). The deep learning process generally needs a large amount of training data followed by the formation of suitable neural networks. Previous studies have proven that convolutional neural networks (CNNs) and their derivative models allow for accurate tissue-type classification and microbiome identification, which could further extend the diagnosis of microbially caused death and personal identification in forensic science. A recent study constructed a CNN model through micorbiome analysis to classify three different human epithelial materials of skin, oral, and vaginal origins (Lopez et al., 2019). Other researchers proposed and designed the CNN based on a system for detecting fungi, parasite ova, and bacteria (Akintayo et al., 2016; Tahir et al., 2018). The accuracy for identifying fungi, soybean cyst nematode eggs, and bacteria was 94.8% (Tahir et al., 2018), 94.33% (Akintayo et al., 2016), and 96% (Treebupachatsakul and Poomrittigul, 2019), respectively.
Recent studies have demonstrated that CNN models could differentiate bacteria and algae based on microbiome images. The application of microbial computer image analysis mainly focuses on the segmentation, clustering, classification, and counting of microorganisms (Ma et al., 2022). The CNN models based on images for microbiome identification are supported by the study of Panicker (Oomman et al., 2018), which could detect tuberculosis in microscopic sputum smear images with a precision of 78.4%. In addition, deep learning on image analysis is mainly focused on the identification of diatoms and algae, which would provide a potential strategy for the diagnosis of drowning. In 2018, Bueno et al. (2018) compared the functions of R-CNN and you only look once (YOLO) in diatom detection; the F-measure of YOLO was 84%. In 2019, Huang et al. applied and trained a CNN model based on the GoogLeNet Inception V3 architecture to identify diatoms with a validation rate of 97.33% (Zhou et al., 2019, 2020), which indicates that DL is an efficient and low-cost automated diatom detection technology. In 2020, YOLO and SegNet were compared by Salido et al. (2020). In 2020, Ruis-Santaquiteria et al. (2020) found that Mask-RCNN and SegNet models are capable of segment diatoms from the same raw images used for manual identification, without any cropping or preprocessing step. With the assistance of necessary operation systems, such as the Center for Microbial Ecology Image Analysis System (Dazzo and Niccum, 2015), image analysis of microorganisms would contribute more to forensic microbiology due to its convenience and operational speed (Ma et al., 2022).
Forensic microbiology is still in its infancy. Advanced technologies, such as NGS and WGS, have provided a sufficient dataset that could not be imagined previously. Further advancements in technology will continue to improve the capacity for forensic microbial investigations. In addition, artificial intelligence has promoted the technological innovation of forensic microbiology in the past decades. With the accumulation of genomic sequencing datasets and microbial images, deep learning will exert greater power in achieving better prognostic models with higher accuracy. However, there are several issues that deserve further discussion.
1. Advantages and defects of AI
Based on the current microbiota database, ML and DL have the quick and automatic capability of relatively accurate prediction compared with the conditional methods. The “black box” of AI methods would not completely and theoretically characterize the real results. Although the new algorithms of AI will shed light on microbiotic analysis for forensic purposes, the basic strategy is focused on the bigger microbiota database to promote AI to work better.
2. Key points for establishing the forensic microbiota database
Considering the variation of microbial communities, the development of a forensic microbial bank is urgently needed. The sampling and sequencing procedures of forensic microorganisms still need to be further standardized. In addition, due to the complex influence of sample types, locations, environmental factors, and postmortem changes, combining the microbiome data from the experimental animals, human samples, and certain materials from the crime scene should be considered. Investigators should carry out multi-center cooperation.
3. Microbiome combined with other methods to solve forensic problems
The selection of differential microbes by bioinformatic technologies could better disclose microbial markers. AI could establish models for predicting forensic issues. Based on the specific microbes that could characterize the different body fluids and environments, even individual identification, targeted PCR testing of the selected microorganisms could be explored to improve the efficiency and accuracy of forensic problems in future.
RZ and DG designed the manuscript and edited the manuscript. HY and ZiW wrote the manuscript. ZhW and FZ searched, edited, and reviewed the literature. All authors have read and commented on the manuscript. All authors contributed to the article and approved the submitted version.
This research was supported by the National Natural Science Foundation of China (Grant No: 81971793), the Natural Science Foundation of Liaoning Province (Grant No: 2022-YGJC-74), and the Development Program of China (Grant No: 2022YFC3302002).
The authors declare that the research was conducted in the absence of any commercial or financial relationships that could be construed as a potential conflict of interest.
All claims expressed in this article are solely those of the authors and do not necessarily represent those of their affiliated organizations, or those of the publisher, the editors and the reviewers. Any product that may be evaluated in this article, or claim that may be made by its manufacturer, is not guaranteed or endorsed by the publisher.
Afshinnekoo, E., Meydan, C., Chowdhury, S., Jaroudi, D., Boyer, C., Bernstein, N., et al. (2015). Geospatial resolution of human and bacterial diversity with city-scale metagenomics. Cell Syst. 1, 72–87. doi: 10.1016/j.cels.2015.01.001
Akintayo, A., Lee, N., Chawla, V., Mullaney, M., and Sarkar, S. (2016). An end-to-end convolutional selective autoencoder approach to Soybean Cyst Nematode eggs detection. arXiv [Preprint]. arXiv: 1603.07834. doi: 10.48550/arXiv.1603.07834
Aoyagi, M., Iwadate, K., Fukui, K., Abe, S., Sakai, K., Maebashi, K., et al. (2009). A novel method for the diagnosis of drowning by detection of Aeromonas sobria with PCR method. Leg. Med. 11, 257–259. doi: 10.1016/j.legalmed.2009.07.003
Bäckhed, F., Roswall, J., Peng, Y., Feng, Q., Jia, H., Kovatcheva-Datchary, P., et al. (2015). Dynamics and stabilization of the human gut microbiome during the first year of life. Cell Host Microbe 17, 690–703. doi: 10.1016/j.chom.2015.04.004
Baek, S. S., Pyo, J. C., Pachepsky, Y., Park, Y., and Cho, K. H. (2020). Identification and enumeration of cyanobacteria species using a deep neural network. Ecol. Indicat. 115, 106395. doi: 10.1016/j.ecolind.2020.106395
Benschop, C. C. G., Quaak, F. C. A., Boon, M. E., Sijen, T., and Kuiper, I. (2012). Vaginal microbial flora analysis by next generation sequencing and microarrays; can microbes indicate vaginal origin in a forensic context? Int. J. Legal Med. 126, 303–310. doi: 10.1007/s00414-011-0660-8
Blaser, M. J., Dominguez-Bello, M. G., Contreras, M., Magris, M., Hidalgo, G., Estrada, I., et al. (2013). Distinct cutaneous bacterial assemblages in a sampling of South American Amerindians and US residents. ISME J. 7, 85–95. doi: 10.1038/ismej.2012.81
Bouslimani, A., da Silva, R., Kosciolek, T., Janssen, S., Callewaert, C., Amir, A., et al. (2019). The impact of skin care products on skin chemistry and microbiome dynamics. BMC Biol. 17, 47. doi: 10.1186/s12915-019-0660-6
Bueno, G., Déniz, O., Ruiz-Santaquiteria, J., Olenici, A., and Borrego-Ramos, M. (2018). “Lights and pitfalls of convolutional neural networks for diatom identification,” in Optics, Photonics and Digital Technologies for Imaging Applications (Washington, DC: SPIE). doi: 10.1117/12.2309488
Butzbach, D. M. (2010). The influence of putrefaction and sample storage on post-mortem toxicology results. For. Sci. Med. Pathol. 6, 35–45. doi: 10.1007/s12024-009-9130-8
Butzbach, D. M., Stockham, P. C., Kobus, H. J., Sims, D. N., Byard, R. W., Lokan, R. J., et al. (2013). Bacterial degradation of risperidone and paliperidone in decomposing blood. J. For. Sci. 58, 90–100. doi: 10.1111/j.1556-4029.2012.02280.x
Can, I., Javan, G. T., Pozhitkov, A. E., and Noble, P. A. (2014). Distinctive thanatomicrobiome signatures found in the blood and internal organs of humans. J. Microbiol. Methods 106, 1–7. doi: 10.1016/j.mimet.2014.07.026
Cao, J., Li, W. J., Wang, Y. F., An, G. S., Lu, X. J., Du, Q. X., et al. (2021). Estimating postmortem interval using intestinal microbiota diversity based on 16S rRNA high-throughput sequencing technology. Fa Yi Xue Za Zhi 37, 621–626. doi: 10.12116/j.issn.1004-5619.2020.400708
Cartozzo, C., Singh, B., Swall, J., and Simmons, T. (2021). Postmortem submersion interval (PMSI) estimation from the microbiome of sus scrofa bone in a freshwater lake. J. For. Sci. 66, 1334–1347. doi: 10.1111/1556-4029.14692
Cecchini, F., Iacumin, L., Fontanot, M., Comi, G., and Manzano, M. (2012). Identification of the unculturable bacteria Candidatus arthromitus in the intestinal content of trouts using dot blot and Southern blot techniques. Vet. Microbiol. 156, 389–394. doi: 10.1016/j.vetmic.2011.11.020
Costello, E. K., Lauber, C. L., Hamady, M., Fierer, N., Gordon, J. I., and Knight, R. (2009). Bacterial community variation in human body habitats across space and time. Science 326, 1694–1697. doi: 10.1126/science.1177486
Dazzo, F. B., and Niccum, B. C. (2015). Use of CMEIAS image analysis software to accurately compute attributes of cell size, morphology, spatial aggregation and color segmentation that signify in situ ecophysiological adaptations in microbial biofilm communities. Computation 3, 72–98. doi: 10.3390/computation3010072
DeBruyn, J. M., and Hauther, K. A. (2017). Postmortem succession of gut microbial communities in deceased human subjects. Peerj 5, 3437. doi: 10.7717/peerj.3437
Escobar, J. S., Klotz, B., Valdes, B. E., and Agudelo, G. M. (2014). The gut microbiota of Colombians differs from that of Americans, Europeans and Asians. BMC Microbiol. 14, 311. doi: 10.1186/s12866-014-0311-6
Fierer, N., Lauber, C. L., Zhou, N., McDonald, D., Costello, E. K., and Knight, R. (2010). Forensic identification using skin bacterial communities. Proc. Natl. Acad. Sci. U. S. A. 107, 6477–6481. doi: 10.1073/pnas.1000162107
Forney, L. J., Zhou, X., and Brown, C. J. (2004). Molecular microbial ecology: land of the one-eyed king. Curr. Opin. Microbiol. 7, 210–220. doi: 10.1016/j.mib.2004.04.015
Franzén, O., Hu, J., Bao, X., Itzkowitz, S. H., Peter, I., and Bashir, A. (2015). Improved OTU-picking using long-read 16S rRNA gene amplicon sequencing and generic hierarchical clustering. Microbiome 3, 43. doi: 10.1186/s40168-015-0105-6
Franzosa, E. A., Huang, K., Meadow, J. F., Gevers, D., Lemon, K. P., Bohannan, B. J. M., et al. (2015). Identifying personal microbiomes using metagenomic codes. Proc. Natl. Acad. Sci. U. S. A. 112, E2930–E2938. doi: 10.1073/pnas.1423854112
Grad, Y. H., Lipsitch, M., Feldgarden, M., Arachchi, H. M., Cerqueira, G. C., FitzGerald, M., et al. (2012). Genomic epidemiology of the Escherichia coli O104:H4 outbreaks in Europe, 2011. Proc. Natl. Acad. Sci. U. S. A. 109, 3065–3070. doi: 10.1073/pnas.1121491109
Habtom, H., Pasternak, Z., Matan, O., Azulay, C., Gafny, R., and Jurkevitch, E. (2019). Applying microbial biogeography in soil forensics. For. Sci. Int. Genet. 38, 195–203. doi: 10.1016/j.fsigen.2018.11.010
Han, E., Kim, E., Hong, H., Jeong, S., Kim, J., In, S., et al. (2012). Evaluation of postmortem redistribution phenomena for commonly encountered drugs. For. Sci. Int. 219, 265–271. doi: 10.1016/j.forsciint.2012.01.016
Hauther, K. A., Cobaugh, K. L., Jantz, L. M., Sparer, T. E., and DeBruyn, J. M. (2015). Estimating time since death from postmortem human gut microbial communities. J. For. Sci. 60, 1234–1240. doi: 10.1111/1556-4029.12828
Huang, L. H., Xu, C. Q., Yang, W. X., and Yu, R. S. (2020). A machine learning framework to determine geolocations from metagenomic profiling. Biol. Direct 15, 278. doi: 10.1186/s13062-020-00278-z
Hung, J., Ravel, D., Lopes, S. C. P., Rangel, G., Nery, O. A., Malleret, B., et al. (2017). Applying faster R-CNN for object detection on malaria images. Confer. Comput. Vis. Pattern Recogn. Workshops 2017, 808–813. doi: 10.1109/CVPRW.2017.112
Huys, G., Coopman, V., Van Varenbergh, D., and Cordonnier, J. (2012). Selective culturing and genus-specific PCR detection for identification of Aeromonas in tissue samples to assist the medico-legal diagnosis of death by drowning. For. Sci. Int. 221, 11–15. doi: 10.1016/j.forsciint.2012.03.017
Javan, G. T., Finley, S. J., Can, I., Wilkinson, J. E., Hanson, J. D., and Tarone, A. M. (2016). Human thanatomicrobiome succession and time since death. Sci. Rep. 6, 598. doi: 10.1038/srep29598
Jesmok, E. M., Hopkins, J. M., and Foran, D. R. (2016). Next-generation sequencing of the bacterial 16S rRNA gene for forensic soil comparison: a feasibility study. J. For. Sci. 61, 607–617. doi: 10.1111/1556-4029.13049
Johnson, H. R., Trinidad, D. D., Guzman, S., Khan, Z., Parziale, J. V., DeBruyn, J. M., et al. (2016). A machine learning approach for using the postmortem skin microbiome to estimate the postmortem interval. PLoS ONE 11, 370. doi: 10.1371/journal.pone.0167370
Kakizaki, E., Kozawa, S., Matsuda, H., Muraoka, E., Uchiyama, T., Sakai, M., et al. (2010). Freshwater bacterioplankton cultured from liver, kidney and lungs of a decomposed cadaver retrieved from a sandy seashore: possibility of drowning in a river and then floating out to sea. Leg. Med. 12, 195–199. doi: 10.1016/j.legalmed.2010.03.008
Kaszubinski, S. F., Pechal, J. L., Smiles, K., Schmidt, C. J., Jordan, H. R., Meek, M. H., et al. (2020). Dysbiosis in the dead: human postmortem microbiome beta-dispersion as an indicator of manner and cause of death. Front. Microbiol. 11, 5347. doi: 10.3389/fmicb.2020.555347
Kaszubinski, S. F., Receveur, J. P., Nestle, E. D., Pechal, J. L., and Benbow, M. E. (2022). Microbial community succession of submerged bones in an aquatic habitat. J. For. Sci. 67, 1565–1578. doi: 10.1111/1556-4029.15036
Kersulyte, D., Kalia, A., Gilman, R. H., Mendez, M., Herrera, P., Cabrera, L., et al. (2010). Helicobacter pylori from peruvian amerindians: traces of human migrations in strains from remote Amazon, and genome sequence of an amerind strain. PLoS ONE 5, 5076. doi: 10.1371/journal.pone.0015076
Khan, H. A., Baig, F. K., and Mehboob, R. (2017). Nosocomial infections: epidemiology, prevention, control and surveillance. Asian Pac. J. Trop. Biomed. 7, 478–482. doi: 10.1016/j.apjtb.2017.01.019
Kimura, N., and Nobutada, S. (2006). Metagenomics: access to unculturable microbes in the environment. Microb. Environ. 21, 201–215. doi: 10.1264/jsme2.21.201
Klevens, R. M., Edwards, J. R., Richards, C. L., Horan, T. C., Gaynes, R. P., Pollock, D. A., et al. (2007). Estimating health care-associated infections and deaths in US hospitals, 2002. Public Health Rep. 122, 160–166. doi: 10.1177/003335490712200205
Kong, H. H., and Segre, J. A. (2012). Skin microbiome: looking back to move forward. J. Invest. Dermatol. 132, 933–939. doi: 10.1038/jid.2011.417
Kuiper, I. (2016). Microbial forensics: next-generation sequencing as catalyst. Embo Rep. 17, 1085–1087. doi: 10.15252/embr.201642794
Langendijk, P. S. (1995). Quantitative fluorescence in situ hybridization of Bifidobacterium spp. with genus-specific 16S rRNA-targeted probes and its application in fecal samples. Appl. Environ. Microbiol. 61, 3069–3075. doi: 10.1128/aem.61.8.3069-3075.1995
Lauber, C. L., Zhou, N., Gordon, J. I., Knight, R., and Fierer, N. (2010). Effect of storage conditions on the assessment of bacterial community structure in soil and human-associated samples. FEMS Microbiol. Lett. 307, 80–86. doi: 10.1111/j.1574-6968.2010.01965.x
Lax, S., Hampton-Marcell, J. T., Gibbons, S. M., Colares, G. B., Smith, D., Eisen, J. A., et al. (2015). Forensic analysis of the microbiome of phones and shoes. Microbiome 3, 82. doi: 10.1186/s40168-015-0082-9
Lax, S., Smith, D. P., Hampton-Marcell, J., Owens, S. M., Handley, K. M., Scott, N. M., et al. (2014). Longitudinal analysis of microbial interaction between humans and the indoor environment. Science 345, 1048–1052. doi: 10.1126/science.1254529
Leake, S. L., Pagni, M., Falquet, L., Taroni, F., and Greub, G. (2016). The salivary microbiome for differentiating individuals: proof of principle. Microb. Infect. 18, 399–405. doi: 10.1016/j.micinf.2016.03.011
Lecun, Y., Bengio, Y., and Hinton, G. (2015). Deep learning. Nature 521, 436. doi: 10.1038/nature14539
Lee, D.-G., Yang, K. E., Hwang, J. W., Kang, H.-S., Lee, S.-Y., Choi, S., et al. (2016). Degradation of kidney and psoas muscle proteins as indicators of post-mortem interval in a rat model, with use of lateral flow technology. PLoS ONE 11, 557. doi: 10.1371/journal.pone.0160557
Lee, S. Y., Woo, S. K., Lee, S. M., Ha, E. J., Lim, K. H., Choi, K. H., et al. (2017). Microbiota composition and pulmonary surfactant protein expression as markers of death by drowning. J. For. Sci. 62, 1080–1088. doi: 10.1111/1556-4029.13347
Li, J., Quinque, D., Horz, H. P., Li, M. K., Rzhetskaya, M., Raff, J. A., et al. (2014). Comparative analysis of the human saliva microbiome from different climate zones: Alaska, Germany, and Africa. BMC Microbiol. 14, 316. doi: 10.1186/s12866-014-0316-1
Liu, R., Gu, Y., Shen, M., Li, H., Zhang, K., Wang, Q., et al. (2020). Predicting postmortem interval based on microbial community sequences and machine learning algorithms. Environ. Microbiol. 22, 2273–2291. doi: 10.1111/1462-2920.15000
Liu, R., Wang, Q., Zhang, K., Wu, H., Wang, G., Cai, W., et al. (2021). Analysis of postmortem intestinal microbiota successional patterns with application in postmortem interval estimation. Microb. Ecol. 12, 1–16. doi: 10.21203/rs.3.rs-411962/v1
Lopez, C. D., Gonzalez, D. M., Haas, C., Vidaki, A., and Kayser, M. (2020). Microbiome-based body site of origin classification of forensically relevant blood traces. For. Sci. Int. Genet. 47, 2280. doi: 10.1016/j.fsigen.2020.102280
Lopez, C. D., Vidaki, A., Ralf, A., Gonzalez, D. M., Radjabzadeh, D., Kraaij, R., et al. (2019). Novel taxonomy-independent deep learning microbiome approach allows for accurate classification of different forensically relevant human epithelial materials. For. Sci. Int. Genet. 41, 72–82. doi: 10.1016/j.fsigen.2019.03.015
Lucci, A., Campobasso, C. P., Cirnelli, A., and Lorenzini, G. (2008). A promising microbiological test for the diagnosis of drowning. For. Sci. Int. 182, 20–26. doi: 10.1016/j.forsciint.2008.09.004
Lutz, H., Vangelatos, A., Gottel, N., Osculati, A., Visona, S., Finley, S. J., et al. (2020). Effects of extended postmortem interval on microbial communities in organs of the human cadaver. Front. Microbiol. 11, 569630. doi: 10.3389/fmicb.2020.569630
Ma, P., Li, C., Rahaman, M. M., Yao, Y., Zhang, J., Zou, S., et al. (2022). A state-of-the-art survey of object detection techniques in microorganism image analysis: from classical methods to deep learning approaches. Artif. Intell. Rev. 12, 1–72. doi: 10.1007/s10462-022-10209-1
Maidak, B. L., Cole, J. R., Lilburn, T. G., Parker, C. T. Jr., Saxman, P. R., Stredwick, J. M., et al. (2000). The RDP (ribosomal database project) continues. Nucl. Acids Res. 28, 173–174. doi: 10.1093/nar/28.1.173
McNulty, S. L., Mole, B. M., Dailidiene, D., Segal, I., Ally, R., Mistry, R., et al. (2004). Novel 180- and 480-base-pair insertions in African and African–American strains of Helicobacter pylori. J. Clin. Microbiol. 42, 5658–5663. doi: 10.1128/JCM.42.12.5658-5663.2004
Metcalf, J. L., Parfrey, L. W., Gonzalez, A., Lauber, C. L., Knights, D., Ackermann, G., et al. (2013). A microbial clock provides an accurate estimate of the postmortem interval in a mouse model system. Elife 2, 1104. doi: 10.7554/eLife.01104
Metzker, M. L. (2010). Sequencing technologies: the next generation. Nat. Rev. Genet. 11, 31–46. doi: 10.1038/nrg2626
Meyers, M. S., and Foran, D. R. (2008). Spatial and temporal influences on bacterial profiling of forensic soil samples. J. For. Sci. 53, 652–660. doi: 10.1111/j.1556-4029.2008.00728.x
Muyzer, G., Dewaal, E. C., and Uitterlinden, A. G. (1993). Profiling of complex microbial populations by denaturing gradient gel electrophoresis analysis of polymerase chain reaction-amplified genes coding for 16S rRNA. Appl. Environ. Microbiol. 59, 695–700. doi: 10.1128/aem.59.3.695-700.1993
Nagasawa, S., Motani-Saitoh, H., Inoue, H., and Iwase, H. (2013). Geographic diversity of Helicobacter pylori in cadavers: forensic estimation of geographical origin. For. Sci. Int. 229, 7–12. doi: 10.1016/j.forsciint.2013.02.028
Oliveira, M., and Amorim, A. (2018). Microbial forensics: new breakthroughs and future prospects. Appl. Microbiol. Biotechnol. 102, 10377–10391. doi: 10.1007/s00253-018-9414-6
Oomman, P. R., Kalmady, K. S., Jeny, R., and Sabu, M. K. (2018). Automatic detection of tuberculosis bacilli from microscopic sputum smear images using deep learning methods. Biocybernet. Biomed. Eng. 38, 691–699. doi: 10.1016/j.bbe.2018.05.007
Pechal, J. L., Crippen, T. L., Benbow, M. E., Tarone, A. M., Dowd, S., and Tomberlin, J. K. (2014). The potential use of bacterial community succession in forensics as described by high throughput metagenomic sequencing. Int. J. Legal Med. 128, 193–205. doi: 10.1007/s00414-013-0872-1
Rácz, E., Könczöl, F., Tóth, D., Patonai, Z., Porpáczy, Z., Kozma, Z., et al. (2016). PCR-based identification of drowning: four case reports. Int. J. Legal Med. 130, 1303–1307. doi: 10.1007/s00414-016-1359-7
Randall, S., Cartozzo, C., Simmons, T., Swall, J. L., and Singh, B. (2021). Prediction of minimum postmortem submersion interval (PMSImin) based on eukaryotic community succession on skeletal remains recovered from a lentic environment. For. Sci. Int. 323, 784. doi: 10.1016/j.forsciint.2021.110784
Roesch, L. F. W., Casella, G., Simell, O., Krischer, J., Wasserfall, C. H., Schatz, D., et al. (2009). Influence of fecal sample storage on bacterial community diversity. Open Microbiol. J. 3, 40–46. doi: 10.2174/1874285800903010040
Ross, A. A., Doxey, A. C., and Neufeld, J. D. (2017). The skin microbiome of cohabiting couples. mSystems 2, 43. doi: 10.1128/mSystems.00043-17
Ruis-Santaquiteria, J., Bueno, G., Deniz, O., Vallez, N., and Cristobal, G. (2020). Semantic versus instance segmentation in microscopic algae detection-ScienceDirect. Eng. Appl. Artif. Intell. 87, 103271. doi: 10.1016/j.engappai.2019.103271
Sajedi, H., Mohammadipanah, F., and Rahimi, S. (2019). Actinobacterial strains recognition by machine learning methods. Multimedia Tools Appl. 78, 20285–20307. doi: 10.1007/s11042-019-7379-9
Salido, J., Sánchez, C., Ruiz-Santaquiteria, J., Cristóbal, G., Blanco, S., and Bueno, G. (2020). A low-cost automated digital microscopy platform for automatic identification of diatoms. Appl. Sci. 10, 6033. doi: 10.3390/app10176033
Salzmann, A. P., Arora, N., Russo, G., Kreutzer, S., Snipen, L., and Haas, C. (2021). Assessing time dependent changes in microbial composition of biological crime scene traces using microbial RNA markers. For. Sci. Int. Genet. 53, 2537. doi: 10.1016/j.fsigen.2021.102537
Sanger, F., Nicklen, S., and Coulson, A. R. (1977). DNA sequencing with chain-terminating inhibitors. Proc. Natl. Acad. Sci. U. S. A. 74, 5463–5467. doi: 10.1073/pnas.74.12.5463
Sastre, C., Bartoli, C., Baillif-Couniou, V., Leonetti, G., and Pelissier-Alicot, A. L. (2017). Post mortem redistribution of drugs: current state of knowledge. Curr. Pharm. Des. 23, 5530–5541. doi: 10.2174/1381612823666170622111739
Schloissnig, S., Arumugam, M., Sunagawa, S., Mitreva, M., Tap, J., Zhu, A., et al. (2013). Genomic variation landscape of the human gut microbiome. Nature 493, 45–50. doi: 10.1038/nature11711
Schmedes, S. E., Woerner, A. E., and Budowle, B. (2017). Forensic human identification using skin microbiomes. Appl. Environ. Microbiol. 83, 1672. doi: 10.1128/AEM.01672-17
Scott, L., Finley, S. J., Watson, C., and Javan, G. T. (2020). Life and death: a systematic comparison of antemortem and postmortem gene expression. Gene 731, 144349. doi: 10.1016/j.gene.2020.144349
Skopp, G. (2010). Postmortem toxicology. For. Sci. Med. Pathol. 6, 314–325. doi: 10.1007/s12024-010-9150-4
Sodhi, K., Shrivastava, A., Arya, M., and Kumar, M. (2013). Knowledge of infection control practices among intensive care nurses in a tertiary care hospital. J. Infect. Public Health 6, 269–275. doi: 10.1016/j.jiph.2013.02.004
Suzuki, T. A., and Worobey, M. (2014). Geographical variation of human gut microbial composition. Biol. Lett. 10, 1037. doi: 10.1098/rsbl.2013.1037
Tahir, M. W., Zaidi, N. A., Rao, A. A., Blank, R., Vellekoop, M. J., and Lang, W. (2018). A fungus spores dataset and a convolutional neural network based approach for fungus detection. IEEE Trans. Nanobiosci. 17, 281–290. doi: 10.1109/TNB.2018.2839585
Tie, J., Uchigasaki, S., Haseba, T., Ohno, Y., Isahai, I., and Oshida, S. (2010). Direct and rapid PCR amplification using digested tissues for the diagnosis of drowning. Electrophoresis 31, 2411–2415. doi: 10.1002/elps.200900754
Treebupachatsakul, T., and Poomrittigul, S. (2019). “Bacteria classification using image processing and deep learning,” in Proceedings of the 2019 34th International Technical Conference on Circuits/Systems, Computers and Communications (ITC-CSCC) (Jeju: IEEE). doi: 10.1109/ITC-CSCC.2019.8793320
Tuomisto, S., Karhunen, P. J., Vuento, R., Aittoniemi, J., and Pessi, T. (2013). Evaluation of postmortem bacterial migration using culturing and real-time quantitative PCR. J. For. Sci. 58, 910–916. doi: 10.1111/1556-4029.12124
Tyakht, A. V., Kostryukova, E. S., Popenko, A. S., Belenikin, M. S., Pavlenko, A. V., Larin, A. K., et al. (2013). Human gut microbiota community structures in urban and rural populations in Russia. Nat. Commun. 4, 3469. doi: 10.1038/ncomms3469
Uchiyama, T., Kakizaki, E., Kozawa, S., Nishida, S., Imamura, N., and Yukawa, N. (2012). A new molecular approach to help conclude drowning as a cause of death: simultaneous detection of eight bacterioplankton species using real-time PCR assays with TaqMan probes. For. Sci. Int. 222, 11–26. doi: 10.1016/j.forsciint.2012.04.029
Wang, L. L., Zhang, F. Y., Dong, W. W., Wang, C. L., Liang, X. Y., Suo, L. L., et al. (2020). A novel approach for the forensic diagnosis of drowning by microbiological analysis with next-generation sequencing and unweighted UniFrac-based PCoA. Int. J. Legal Med. 134, 2149–2159. doi: 10.1007/s00414-020-02358-1
Williams, D. W., and Gibson, G. (2017). Individualization of pubic hair bacterial communities and the effects of storage time and temperature. For. Sci. Int. Genet. 26, 12–20. doi: 10.1016/j.fsigen.2016.09.006
Willis, J. R., González-Torres, P., Pittis, A. A., Bejarano, L. A., Cozzuto, L., Andreu-Somavilla, N., et al. (2018). Citizen science charts two major “stomatotypes” in the oral microbiome of adolescents and reveals links with habits and drinking water composition. Microbiome 6, 218. doi: 10.1186/s40168-018-0592-3
Wu, G. D., Lewis, J. D., Hoffmann, C., Chen, Y.-Y., Knight, R., Bittinger, K., et al. (2010). Sampling and pyrosequencing methods for characterizing bacterial communities in the human gut using 16S sequence tags. BMC Microbiol. 10, 206. doi: 10.1186/1471-2180-10-206
Wu, J., Peters, B. A., Dominianni, C., Zhang, Y., Pei, Z., Yang, L., et al. (2016). Cigarette smoking and the oral microbiome in a large study of American adults. ISME J. 10, 2435–2446. doi: 10.1038/ismej.2016.37
Yang, J. Y., Tsukimi, T., Yoshikawa, M., Suzuki, K., Takeda, T., Tomita, M., et al. (2019). Cutibacterium acnes (Propionibacterium acnes) 16S rRNA genotyping of microbial samples from possessions contributes to owner identification. Msystems 4, 594. doi: 10.1128/mSystems.00594-19
Yang, T., Shi, Y., Zhu, J., Zhao, C., Wang, J., Liu, Z., et al. (2021). The spatial variation of soil bacterial community assembly processes affects the accuracy of source tracking in ten major Chinese cities. Sci. China Life Sci. 64, 1546–1559. doi: 10.1007/s11427-020-1843-6
Yooseph, S., Andrews-Pfannkoch, C., Tenney, A., McQuaid, J., Williamson, S., Thiagarajan, M., et al. (2013). A metagenomic framework for the study of airborne microbial communities. PLoS ONE 8, 862. doi: 10.1371/journal.pone.0081862
Yu, Z., Xie, Q., Zhao, Y., Duan, L., Qiu, P., and Fan, H. (2021a). NGS plus bacterial culture: a more accurate method for diagnosing forensic-related nosocomial infections. Leg. Med. 52, 101910. doi: 10.1016/j.legalmed.2021.101910
Yu, Z., Xu, Q., Xiao, C., Li, H., Wu, W., Du, W., et al. (2021b). SYBR green real-time qPCR method: diagnose drowning more rapidly and accurately. For. Sci. Int. 321, 720. doi: 10.1016/j.forsciint.2021.110720
Zhang, J., Wang, M., Qi, X., Shi, L., Zhang, J., Zhang, X., et al. (2021). Predicting the postmortem interval of burial cadavers based on microbial community succession. For. Sci. Int. Genet. 52, 2488. doi: 10.1016/j.fsigen.2021.102488
Zhao, X., Zhong, Z., and Hua, Z. (2022). Estimation of the post-mortem interval by modelling the changes in oral bacterial diversity during decomposition. J. Appl. Microbiol. 133, 3451–3464. doi: 10.1111/jam.15771
Zhou, Y., Cao, Y., Huang, J., Deng, K., Ma, K., Zhang, T., et al. (2020). Research advances in forensic diatom testing. For. Sci. Rese. 5, 98–105. doi: 10.1080/20961790.2020.1718901
Zhou, Y., Zhang, J., Huang, J., Deng, K., and Huang, P. (2019). Digital whole-slide image analysis for automated diatom test in forensic cases of drowning using a convolutional neural network algorithm. For. Sci. Int. 302, 109922. doi: 10.1016/j.forsciint.2019.109922
Keywords: forensic microbiology, forensic medicine, machine learning, deep learning, artificial intelligence
Citation: Yuan H, Wang Z, Wang Z, Zhang F, Guan D and Zhao R (2023) Trends in forensic microbiology: From classical methods to deep learning. Front. Microbiol. 14:1163741. doi: 10.3389/fmicb.2023.1163741
Received: 11 February 2023; Accepted: 08 March 2023;
Published: 30 March 2023.
Edited by:
Chen Li, Northeastern University, ChinaReviewed by:
Dong Zhao, China University of Political Science and Law, ChinaCopyright © 2023 Yuan, Wang, Wang, Zhang, Guan and Zhao. This is an open-access article distributed under the terms of the Creative Commons Attribution License (CC BY). The use, distribution or reproduction in other forums is permitted, provided the original author(s) and the copyright owner(s) are credited and that the original publication in this journal is cited, in accordance with accepted academic practice. No use, distribution or reproduction is permitted which does not comply with these terms.
*Correspondence: Dawei Guan, ZHdndWFuQGNtdS5lZHUuY24=; Rui Zhao, cnpoYW9AY211LmVkdS5jbg==
†These authors have contributed equally to this work and share first authorship
Disclaimer: All claims expressed in this article are solely those of the authors and do not necessarily represent those of their affiliated organizations, or those of the publisher, the editors and the reviewers. Any product that may be evaluated in this article or claim that may be made by its manufacturer is not guaranteed or endorsed by the publisher.
Research integrity at Frontiers
Learn more about the work of our research integrity team to safeguard the quality of each article we publish.