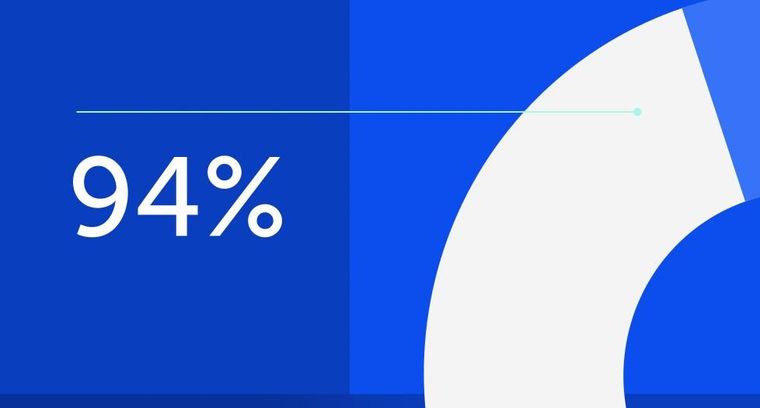
94% of researchers rate our articles as excellent or good
Learn more about the work of our research integrity team to safeguard the quality of each article we publish.
Find out more
ORIGINAL RESEARCH article
Front. Microbiol., 15 May 2023
Sec. Microorganisms in Vertebrate Digestive Systems
Volume 14 - 2023 | https://doi.org/10.3389/fmicb.2023.1148466
This article is part of the Research TopicLatest Perspective on Microbes Detection: From Laboratory to On-spot SensorView all 8 articles
This study aimed to evaluate the difference in gut microbiomes between preterm and term infants using third-generation long-read sequencing (Oxford Nanopore Technologies, ONT) compared with an established gold standard, Illumina (second-generation short-read sequencing). A total of 69 fecal samples from 51 term (T) and preterm (P) infants were collected at 7 and 28 days of life. Gut colonization profiling was performed by 16S rRNA gene sequencing using ONT. We used Illumina to validate and compare the patterns in 13 neonates. Using bioinformatic analysis, we identified features that differed between P and T. Both T1 and P1 microbiomes were dominated by Firmicutes (Staphylococcus and Enterococcus), whereas sequentially showed dominant transitions to Lactobacillus (p < 0.001) and Streptococcus in T2 (p = 0.001), and pathogenic bacteria (Klebsiella) in P2 (p = 0.001). The abundance of beneficial bacteria (Bifidobacterium and Lactobacillus) increased in T2 (p = 0.026 and p < 0.001, respectively). These assignments were correlated with the abundance at the species-level. Bacterial α-diversity increased in T (p = 0.005) but not in P (p = 0.156), and P2 showed distinct β-diversity clustering than T2 (p = 0.001). The ONT reliably identified pathogenic bacteria at the genus level, and taxonomic profiles were comparable to those identified by Illumina at the genus level. This study shows that ONT and Illumina are highly correlated. P and T had different microbiome profiles, and the α- and β-diversity varied. ONT sequencing has potential for pathogen detection in neonates in clinical settings.
Perturbations in the infant gut microbiome during early life affect growth, development, and long-term health (Sherman, 2010; Carl et al., 2014; Tarr and Warner, 2016). Preterm infants have a physiologically and anatomically immature gastrointestinal tract that is known to be more permeable than that of term infants, and their microbiota colonization is challenged by environmental factors, such as antibiotic use, hospital stay, and enteral feeding (Dominguez-Bello et al., 2010; Fallani et al., 2010; DiGiulio, 2012; Healy et al., 2022). Although previous studies have continuously attempted to elucidate the microbial communities of neonatal gut (Schwiertz et al., 2003; Palmer et al., 2007; Korpela et al., 2018), the overall understanding of the gut microbiome in preterm infants cared for in the neonatal intensive care unit (NICU) remains inadequate because of a relatively scarce attempt at sample analysis and the small number of participants studied. For decades, meconium has been considered sterile (sterile womb hypothesis), but some studies using molecular techniques suggest that meconium contains a complex microbiota (in-utero colonization hypothesis) (Hansen et al., 2015; Koleva et al., 2015). Recently, noteworthy studies have demonstrated again that meconium does not have detectable microbiome taxa due to insufficient bacterial read, and too low concentrations of DNA extraction (Kennedy et al., 2021; Martí et al., 2021; Klopp et al., 2022).
Next-generation sequencing (NGS) has revolutionized the profiling of environmental and clinical microbial communities in recent years (Leggett et al., 2020). Currently, second-generation NGS platforms, such as Illumina (San Diego, CA, USA), have been used for clinical in vitro testing with US FDA approval. However, the high cost of Illumina platforms and their large spatial footprint can make their application in clinical settings challenging. Short-read NGS technology-based 16S rRNA analysis is limited to a maximum of ~450 bp of 16S rRNA hypervariable regions, namely V3–V4 and V4–V5 (Biol-Aquino et al., 2019; Fadeev et al., 2021), among others. Depending on the variability in 16S rRNA regions between phylogenetically closely related microbes, such innate limitations can cause difficulties in identifying different microbes at the species and strain levels and even for specific microbes that are practically identical in the target amplicon region. It has been reported that even subtle strain-level variations are vast in the case of closely related bee species (Ellegaard et al., 2020) and can cause pathogenicity within the human microbiome (Yan et al., 2020); short-read-based 16S rRNA analysis methods may overlook potential epidemiologic points of interest in some microbial analytical settings.
Oxford Nanopore Technologies (ONT) sequencing is an innovative third-generation long-read NGS method of single-molecule real-time (SMRT) sequencing. The MinION device by ONT (Oxford, United Kingdom), a pocket-sized sequencing platform capable of producing runs comparable to Illumina and PacBio (Leggett et al., 2020), represents an inexpensive and portable sequencing platform capable of producing long-read data. Using ONT sequencing is a cost-effective approach for bacterial identification at the species-level owing to its capital cost and the ability to sequence the full 16S rRNA gene region and more. Outbreak surveillance and characterization of low microbial biomass samples are required (Ashton et al., 2015; Greninger et al., 2015; Quick et al., 2016; Schmidt et al., 2016), as in NICU environments, which can be aided by the advantages of ONT sequencing. Given the methodological differences in the 16S rRNA target regions between Illumina (V3-V4) and ONT (V1-V9), their sequencing results and success rates can differ, especially at low DNA loads such as neonatal meconium.
This study aimed to compare two sequencing methods ONT MinION and Illumina in infants’ gut microbiome and to compare changes between term and preterm infants using ONT MinION. This is the first study to compare the gut microbiome of term and preterm infants using both sequencing methods and provides the first step toward using ONT platforms in NICU.
We prospectively enrolled infants who were hospitalized in the newborn nursery room (NB) or NICU of Hanyang University Hospital, Seoul, Korea, from July 2021 until January 2022. The term infants (T) group was the control that was defined as ≥37 weeks of gestation and they did not receive intravenous antibiotics after birth. Infants with a gestational age of <37 weeks constituted the preterm group (P). Healthy P infants were hospitalized in the NICU and divided into two subgroups according to gestational age (GA): very preterm (VP, 28+0 ≤ GA ≤31+6 weeks) and moderate-to-late preterm (LP, 32+0 ≤ GA ≤ 36+6 weeks). All infants were enrolled in the study within 2 days after birth. Fecal samples were collected by a trained pediatrician during two periods: within 7 days of birth (T1, P1) and at 28 ± 7 days (T2, P2). Infants with major congenital anomalies or malformations, unstable conditions including septic shock, and necrotizing enterocolitis (NEC) were excluded from the study. The study was approved by the Institutional Review Board of Hanyang University Medical Center (No. 2021-03-017). Written informed consent was obtained from the parents of the infants and all methods were performed in accordance with standard human research ethics guidelines (Declaration of Helsinki).
A total of 51 infants including preterm (n = 31) and term (n = 20) who were hospitalized in the NICU or nursery room were enrolled and 69 fecal samples (T1 = 20, T2 = 12; P1 = 17, P2 = 20) were collected from them. After DNA extraction, amplification, and sequencing, 31 fecal samples (T1 = 15, T2 = 2; P1 = 13, P2 = 1) from 17 infants (T = 8; P = 9) were excluded due to insufficient concentrations of gDNA or library amplicons. Using ONT, valid results from 38 fecal samples (T1 = 5, T2 = 10; P1 = 4, P2 = 19) from 34 infants (T = 12; P = 22) were obtained. Additionally, 15 overlapping samples (T1 = 1, T2 = 3; P1 = 4, P2 = 7) from 13 infants (T = 4; P = 9) were analyzed using Illumina to test the effectiveness of ONT sequencing (Figure 1).
Figure 1. Sampling protocol and analysis method for participants. The subjects were grouped according to gestational age (37 weeks). Fecal samples were collected at 7 and 28 days after birth. ONT was performed on 38 fecal samples obtained from 34 infants. To compare the sequencing results, 15 overlapping samples from 13 infants were analyzed using Illumina. T, Term infants; P, Preterm infants; 7D, day 7 of life after birth; 28D, day 28 of life; DNA, Deoxyribonucleic Acid; rRNA, Ribosomal ribonucleic acid; ONT, Oxford Nanopore Technologies.
Clinical data were extracted from the medical records, at the time of fecal sample collection. Demographic characteristics included sex, delivery mode, birth weight, Apgar scores at 1 and 5 min, maternal gestational diabetes mellitus (GDM), pregnancy-induced hypertension (PIH), acute chorioamnionitis, use of antenatal steroids, and maternal antibiotic exposure. Information on after birth parameters, such as the use of antibiotics (within 48 h or on the day of sample collection), the day of invasive ventilator administration, and breastmilk exposure at the time of sample collection, was also collected.
Up to 1 g of feces from diapers was collected in a sterilized Cary-Blair aqueous solution tube using a sterilized microbiological sampling swab in the FecalSwab Sampling Kit (470 CE, Copan, Italy) and stored in a cryogenic freezer at −80°C. In cases where fecal sample collection was not feasible, feces were collected using a rectal swab. If it was difficult to store the samples immediately in the cryogenic freezer, they were first stored in the sample freezer at −20°C and transferred within a week to a cryogenic freezer for storage.
A DNeasy PowerSoil Pro Kit (QIAGEN, Hilden, Germany) was used for genomic DNA extraction from the microorganisms in the sample, according to the manufacturer’s instructions, except for the initial bead homogenization conditions. A bead homogenizer (Bead Ruptor Elite, OMNI International, Kennesaw, GA, USA), with bead-beating conditions of 1-min homogenization at 6 m/s, 5-min rest, with a total of 3-min homogenization time, using a ceramic bead mixture of diverse sizes was used. The sample input was 100–150 μL, and in case when the extraction was not processed properly, additional improvement measures were applied; the pellet from a 300–400 μL sample centrifuged at 15,000 g was used and the elution reagent was heated to 60–70°C before final DNA elution. The elution volume ranged from 50 to 100 μL, and slightly fewer amounts of elute were recovered for each sample. The concentration and purity of the extracted DNA were checked using a Nanodrop Lite spectrophotometer (Thermo Fisher Scientific, Waltham, USA), which ranged from 1.4 to 138.5 ng/μL (mean value 21.7 ng/μL) for dsDNA concentration and 1.68–2.08 (mean 1.89) for dsDNA A260/A280 ratio. The extracted gDNA samples were stored at −80°C for subsequent processing.
The extracted gDNA was then prepared for prokaryotic metagenome sequencing using the 16S Barcoding Kit and 16S Barcoding Kit 0–24 (SQK-RAB204 and SQK-16S024, Oxford Nanopore Technologies, Oxford, UK), according to the manufacturer’s protocol, using 10 ng of the extracted gDNA per sample. The PCR reaction was performed on the full 16S hypervariable region (V1-V9) (Frank et al., 2008) with 27F forward primer (5′-ATCGCCTACCGTGAC – barcode – AGAGTTTGATCMTGGCTCAG – 3′) and 1492R reverse primer (5′ – ATCGCCTACCGTGAC – barcode – CGGTTACCTTGTTACGACTT – 3′) by injecting each multiplexing barcode included in the 16S Barcoding Kit 0–24 into 10 ng of each extracted DNA under the following conditions: initial 30 s denaturation at 98°C (Stage 1), 25 cycles of 10 s denaturation at 98°C, 30 s annealing at 55°C, 90 s extension at 65°C (Stage 2), and 5 min final extension at 65°C (Stage 3), with NEBNext® Ultra™ II Q5® Master Mix (New England Biolabs, Ipswich, MA, USA) as the PCR polymerase reagent mixture. The 16S V1–V9 amplicons were subsequently purified using Agencourt AMPure XP (Beckman Coulter, Brea, CA, USA) magnetic beads with a PCR reaction mix to magnetic bead ratio of 5:3 and washed twice with freshly prepared 70% ethanol. The final elution of purified DNA was performed by adding 12 μL of 10 mM Tris–HCl pH 8.0 with 50 mM NaCl, incubating for 2 min at room temperature, and recovering 10 μL of the elute from each tube. The concentration of each purified 16S V1–V9 amplicon was confirmed with an Invitrogen Qubit 4 fluorometer (Thermo Fisher Scientific, Waltham, MA, USA), and the samples were pooled with a total concentration of 100 fmol in 10 μL of the final library.
Sequencing libraries were prepared according to Illumina 16S Metagenomic Sequencing Library protocols to amplify the V3 and V4 regions of the 16S rRNA gene. The input of 2 ng gDNA was PCR-amplified with 5x reaction buffer, 1 mM dNTP mix, 500 nM each of the universal F/R PCR primers, 5 pM each of the pRNA, mRNA oligo, and Herculase II fusion DNA polymerase (Agilent Technologies, Santa Clara, CA, USA). The cycle conditions for the 1st PCR were 3 min at 95°C for heat activation and 25 cycles of 30 s at 95°C, 10 s at 78°C, 60 s at 50°C, and 60 s at 72°C, followed by a 5-min final extension at 72°C. The universal primer pair with Illumina adapter overhang sequences used for amplification was as follows: V3-F:5′-TCGTCGGCAGCGTCAGATGTGTATAAGAGACAGCCTACGGGNGGCWGCAG-3′, V4-R:5′-GTCTCGTGGGCTCGGAGATGTGTATAAGAGACAGGACTACHVGGGTATCTAATCC-3′, based on the V3–V4 universal primers (Klindworth et al., 2013). The first PCR product was purified using AMPure XP beads (Beckman Coulter). Following purification, 2 μL of the purified eluate was again PCR-amplified for final library construction containing the index using the NexteraXT Indexed Primer. The cycle conditions for the second PCR were the same as those for the first PCR, except for the number of cycles which was 10. The PCR products were purified using AMPure XP beads. To verify the size of PCR-enriched fragments, the template size distribution was checked using a TapeStation D1000 Screen Tape (Agilent Technologies, Waldbronn, Germany).
For the prepared ONT library sequencing, MinION Flow Cell (R9.4.1, FLO-MIN-106D, Oxford Nanopore Technologies, Oxford, UK) with MinKNOW software (v21.06.13, Oxford Nanopore Technologies) was utilized. FAST5 raw data were collected for up to 48 h for each run, simultaneously processing the FAST5 data to the FASTQ file using a GPU-based ont-guppy basecaller (v5.0.16, Oxford Nanopore Technologies) in super-accurate mode with barcode and adaptor trimming, until the number of passed reads for the barcode of the lowest value exceeded 100,000. As the sequencing capacity of MinION Flow Cells was much higher than the maximum of 24 samples that could be multiplexed with the SQK-16S024 barcoding kit, after reaching the desired throughput for a sequencing run, the MinION cells were washed with the Flow Cell Wash Kit (EXP-WSH004, Oxford Nanopore Technologies) after each run, stored, and reused until the pores were depleted. For the Illumina V3–V4 library, the MiSeq platform was used to sequence the index-attached V3–V4 amplicons under 300 × 2 paired-end read conditions using standard Illumina 16S Metagenomic Sequencing Library protocols to obtain FASTQ data of read count ranging from 121,470 to 609,040.
For ONT sequencing, after the sequencing run, the FASTQ files that passed the quality filter were concatenated into one file per sample. Subsequently, the fastp package (Chen et al., 2018) was used to filter out the reads further to remove contaminants and artifacts that hinder the downstream processing of the data. The remaining reads were subsampled with 50,000 reads per sample using the seqtk package for taxonomic analysis and 15,000 reads per sample for phylogenetic tree alignment under the available computational resources. The reliability of the subsampled read numbers was verified. Prior to subjecting the full dataset to taxonomic analysis, three random samples from the ONT sequencing were subsampled to 30,000 reads and 100,000 reads per sample to ensure adequate read depth was achieved. The results showed negligible differences of less than 0.1% in all taxonomic levels, showing that the read depth of 30,000 reads per sample was sufficient for detecting minor constituents of the 16S metagenome. For the main taxonomic analysis, 50,000 reads per sample were used to further ensure adequate read depth. For diversity analysis, the alpha rarefaction curves of various alpha diversity parameters were checked to verify the plateau of the curves.
Illumina sequencing data were obtained in the FASTQ format and demultiplexed by each barcode. Paired-end reads were merged, and chimeric reads were eliminated. All the obtained raw reads were subjected to taxonomic pipeline analysis, after the adapter and low-quality sequences of the reads were trimmed using Trimmomatic (v0.39).
For taxonomic analysis of the ONT data, the full MetONTIIME pipeline was designed for use with CPU-based ont-guppy basecalling of the FAST5 data, and the MetONTIIME.sh shell script file was utilized for taxonomic analysis of the GPU-basecalled FASTQ data obtained previously. To compare the relative abundance of each sample and their corresponding groups, the QIIME2 platform (v2022.2) (Bolyen et al., 2019) and MetONTIIME pipeline were used for downstream processing of FASTQ data. The SILVA ribosomal RNA gene reference database version 138.1 NR99 (Quast et al., 2013) with curation methods provided by the RESCRIPt package (Robeson et al., 2021) was used for read mapping. First, sequences containing five or more ambiguous bases were removed and differentially filtered among kingdoms by discarding reads under 900 bp for archaea and under 1,200 bp for bacteria. The filtered sequences were dereplicated further to create a consensus taxonomy, extracted with 27F-1492R primers to obtain the amplicon region, and finally dereplicated again from an optimized reference database for more efficient taxonomic analysis. The default parameters provided by the QIIME2 pipelines were utilized unless otherwise specified. The FASTQ reads were dereplicated using vsearch (Rognes et al., 2016) and clustered into de novo operational taxonomic units (OTUs) to avoid reference bias. The clustered OTUs were taxonomically classified using vsearch global alignment with an identity threshold of 85% and query coverage parameter of 0.8. The generated taxonomy file was tabulated for each taxonomic level of interest from the phylum to the species-level, and the relative abundance of the taxa was determined for comparison.
QIIME2 platform was likewise utilized for Illumina V3–V4 data; however, amplicon sequence variants (ASVs) were constructed with trimmed Illumina reads using DADA2 method (Callahan et al., 2016) as an advanced alternative available in short-reads compared to OUT generation, and then taxonomically classified with the reference database using 97% identity threshold (Pichler et al., 2018). The same SILVA 138.1 NR99 database was used, with V3-F and V4-R primer sequences being utilized to optimize the database for analysis. The generated taxonomy was tabulated from the phylum to the genus level, and the relative abundance was calculated.
For ONT reads, the subsampled FASTQ files were dereplicated and clustered de novo with the same parameters as in the taxonomic analysis, and the clustered OTUs were used for phylogenetic tree alignment using the MAFFT (Katoh et al., 2002) alignment program with the FFT-NS-2 alignment method along with FastTree (Price et al., 2010) software. Subsequently, using the generated rooted tree phylogeny, alpha diversity metrics and rarefaction curves of up to 15,000 reads were generated, and beta diversity analysis of Bray–Curtis dissimilarity and weighted/unweighted UniFrac distances (Lozupone et al., 2011) were ordinated using principal coordinate analysis (PCoA) with q2-diversity plugin in the QIIME2 platform.
All data were analyzed using Statistical System 9.4 (SAS Institute, Cary, NC, USA), and R statistical software version 4.0.3 (R Foundation). Clinical characteristics were carried out by analysis of variance, and linear correlation between ONT and Illumina was estimated as Pearson correlation. Significant differences between treatments were defined at p < 0.05, and p < 0.01 levels, and adjusted P (Q value) < 0.05 was considered as significant differences in diversity analysis.
The T and P groups differed in gestational age (38.8 ± 0.9 vs. 32.3 ± 2.8, p < 0.001) and birth weight (3190 ± 229.4 vs. 1688 ± 600.2, p < 0.001), and delivery mode (p = 0.037). However, there were no differences in sex, delivery mode, the start of feeding day, or current breastfeeding. T, the control group was not exposed to antibiotics. However, according to the NICU guidelines of Hanyang University Hospital, most preterm infants are exposed to antibiotics. Except for GA and birth weight, there were no significant differences in the use of antibiotics, duration of antibiotics, delivery mode, and current breastfeeding between VP and LP groups (Supplementary Table 1). Baseline characteristics and infant outcomes are detailed in Table 1.
The dominant phylum identified in T1 was Firmicutes (99.05%), followed by Actinobacteria (0.68%), Proteobacteria (0.22%), and Bacteroidetes (0.05%) (Supplementary Table 2). In P1, Firmicutes was the most abundant (96.05%), followed by Proteobacteria (3.86%) and Actinobacteria (0.06%). No Bacteroidetes were present (Figure 2A).
Figure 2. Relative abundance of the most dominant bacterial communities, analyzed by ONT. (A) Relative abundance at the phylum level. (B) Relative abundance at the genus level. (C) The specific bacterial population at the genus level (Bifidobacterium, Lactobacillus, Streptococcus, Klebsiella) (D) Relative abundance at the species-level. (E) The specific bacterial population at the species-level (Enterococcus faecalis, Staphylococcus epidermidis, Enterococcus faecium, Staphylococcus haemolyticus, Bifidobacterium longum, Lactobacillus gasseri, Streptococcus salivarius, Klebsiella pneumoniae). Treatment groups: T1, term group in period 1; T2, term group in period 2; P1, preterm group in period 1; P2, preterm group in period 2. *, ** indicate significant differences between groups (p < 0.05, and p < 0.01, respectively). a,b Mean values within a row having different superscript letters are significantly different (p < 0.05).
Over time, Proteobacteria increased significantly in P2 (P1 vs. P2, p < 0.011), almost at the same level as Firmicutes. By contrast, Actinobacteria barely increased in abundance. There were statistically significant increases in the abundances of Firmicutes, Proteobacteria, and Actinobacteria (p < 0.001, p < 0.001, and p < 0.001, respectively) at 28 days (Figure 2A).
Within the same Firmicutes phylum, Staphylococcus (40.33%) was the most abundant in T1, followed by Enterococcus (39.76%) and Lactobacillus (0.41%), whereas, in P1, Enterococcus (65.58%) was dominant, followed by Staphylococcus (27.01%) and Lactobacillus (0.09%) (Figure 2B).
There were no significant differences between T and P at the genus level after 7 days of life. However, at 28 days, we observed a noticeable increase in the relative abundance of Klebsiella, a potential bacterial pathogen, (p = 0.002) in P2, and Streptococcus and beneficial Bifidobacterium and Lactobacillus in T2 (p = 0.001, p = 0.026, p < 0.001, respectively) (Figure 2C).
Firmicutes remained the most abundant phylum from T1 to T2. However, the dominant genera changed from Staphylococcus (40.33%), Enterococcus (39.76%), and Lactobacillus (0.41%) to Streptococcus (37.55%), Lactobacillus (22.07%), and Enterococcus (13.50%) (Supplementary Table 3).
The T1 showed higher abundance of Enterococcus faecalis (p = 0.081) and Staphylococcus epidermidis (p = 0.004), whereas in P1, Enterococcus faecium (p < 0.001) and Staphylococcus hemolyticus (p = 0.034) were more abundant (Figures 2D,E).
Bifidobacterium longum, Lactobacillus gasseri, and Streptococcus salivarius significantly increased in T2 (p = 0.017, p = 0.001, p < 0.001, respectively), whereas in P2, Klebsiella pneumoniae was increased, but there was no statistical difference (p = 0.096) (Supplementary Table 4).
Bacterial α-diversity was measured using Pielou’s evenness index (microbial evenness) and Faith’s phylogenetic diversity index (species richness). A statistically significant increase was observed in both evenness and richness in T (T1 vs. T2; p < 0.002 and T1 vs. T2; p < 0.002, respectively), but P did not show difference in both evenness and richness (P1 vs. P2; p = 0.156 and P1 vs. P2; p = 0.156, respectively) (Figure 3A).
Figure 3. Difference in α- and β - diversity of gut microbiome between T and P. (A) Species evenness index (Pielou’s evenness index) and species richness index (Faith’s phylogenetic diversity). (B) Principal coordinate analysis (PCoA) ordination plots of microbial communities in T1, T2, P1, and P2 based on weighted UniFrac distance. p-value shows significant differences between groups. q-value shows FDR, adjusted p-value.
Subsequently, we evaluated the similarity of the gut microbiota (β-diversity) for both bacterial composition and phylogenetic relationships of the components. There was a significant difference in the β-diversity between T1 and T2 (p = 0.005), but no differences were observed between P1 and P2 (p = 0.050). Samples from T1 and P1 were dispersed and intermingled, suggesting that the bacterial composition of T1 was largely similar to that of P1 (p = 0.327). However, there was a statistically significant difference between T2 and P2 (p = 0.001) (Figure 3B).
To investigate the impact of the degree of prematurity on the gut microbiome of premature infants, we divided them into two groups (VP and LP). VP infants had the greatest abundance of Klebsiella pneumoniae (Klebsiella genus/Proteobacteria phylum) (p = 0.014), whereas LP, later GA premature infants, had the greatest abundance of Enterococcus faecium (Enterococcus genus/Firmicutes phylum) (p = 0.008) (Figures 4A–C). Across all time points, in T, Lactobacillus gasseri (Lactobacillus genus/Firmicutes phylum) (p = 0.023) and Streptococcus salivarius (Streptococcus genus/Firmicutes phylum) (p = 0.013) were significantly increased.
Figure 4. Difference in relative abundance of the most dominant bacterial communities between VL and LP, as analyzed by ONT. (A) Relative abundance at the phylum level. (B) Relative abundance at the genus level. (C) Relative abundance at the species-level. Treatment groups: T, term group; VP, very preterm infants; LP, moderate to late preterm infants. *, ** indicate significant differences between groups (p < 0.05, and p < 0.01, respectively).
We sequenced using Illumina for identical samples from 13 infants and compared them with ONT sequencing results up to the genus level. This approach showed that the ONT sequencing depth was sufficient to capture the bacterial diversity in the infants’ fecal samples. Taxonomic assignments using ONT versus Illumina sequencing data were largely consistent in both phylum (log-transformed Pearson’s r2 = 0.905) and genus (log-transformed Pearson’s r2 = 0.926) (Figures 5A,B). As well Firmicutes and Proteobacteria (Figure 5C), especially Enterococcus, Escherichia, Klebsiella, Raoultella, Staphylococcus, and Streptococcus were well consistent (Figure 5D).
Figure 5. Correlation analysis between ONT and Illumina sequencing methods in phylum and genus level at D7 or D28. (A) Correlation plot of gut microbial abundance in phylum level of ONT (x-axis) and Illumina (y-axis) data at 7D or 28D (Pearson’s r2 = 0.905, Total; Pearson’s r2 = 0.961, D7; Pearson’s r2 = 0.819, 28D). The region on either side of the dotted line represents the 95% CIs. (B) Correlation plot of gut microbial abundance in genus level of ONT (x-axis) and Illumina (y-axis) data at 7D or 28D (Pearson’s r2 = 0.926, Total; Pearson’s r2 = 0.925, 7D; Pearson’s r2 = 0.904, 28D). The region on either side of the dotted line represents the 95% CIs. (C) Taxonomic profiles of gut microbiome abundance at 7D or 28D in phylum level. The top row corresponds to the results from ONT, and the bottom row shows the results from Illumina. (D) Taxonomic profiles of gut microbiome abundance at 7D or 28D in genus level. The top row corresponds to the results from ONT, and the bottom row shows the results from Illumina. 7D, day 7 of life after birth; 28D, day 28 of life; ONT, Oxford Nanopore Technologies.
Two sequencing reads correlated well, showing that the Firmicutes phylum was the most dominant, followed by Proteobacteria in T1 and P1, Firmicutes was still dominant in T2, and Proteobacteria abundance was increased in P2. However, the relative abundance of Actinobacteria (and the associated genus Bifidobacterium) was higher according to Illumina results, and it was also the same in T2 and P2 (T1 vs. T2; p = 0.073, P1 vs. P2; p = 0.099, respectively) (Supplementary Table 5). Although highly correlative, the total reads mapped for the ONT sequencing tended to exhibit greater read counts with a higher percentage of mapped reads compared to the results obtained using Illumina.
In this study, we evaluated the evolution of the gut microbiome during the first 28 days of life in T and P using ONT and Illumina sequencing combined with bioinformatics tools that could be used to gather clinically relevant data. ONT sequencing data were comparable in discriminatory power to Illumina data, allowing microbiome and abundance profiling and exploring species-specific profiles. Our data revealed a statistically significant increase of pathogenic bacteria (Klebsiella pneumoniae, Enterococcus faecium) in P; by contrast, few beneficial bacteria (Lactobacillus gasseri, Bifidobacterium longum) were found in this group. This difference was more evident at 28 days than at 7 days after birth.
This study demonstrated that both sequencing techniques performed comparably up to the genus level, but some bacterial proportions were different. There are several reasons for this difference. Firstly, Illumina sequencing generally exhibited more significant read counts with a lower percentage of mapped reads; however, this did not affect detection limits compared to ONT sequencing (Claesson et al., 2010; Stefan et al., 2022). This may explain the overexpression of Actinobacteria, which showed a low abundance in our study. Secondly, the principles of both sequencing methods are completely different (Claesson et al., 2010; Winand et al., 2019). While Illumina sequences the V3–V4 hypervariable regions of the 16 s rRNA gene (Klindworth et al., 2013) and yields >99% read accuracy using the sequencing-by-synthesis method, ONT sequences the whole hypervariable regions of 16S rRNA genes from V1 to V9 using the nanopore (pore-forming protein) method and yields around 91–92% read accuracy (R9.4.1 nanopore). The advantages and disadvantages of each method are described in detail (Supplementary Table 6). The lower read accuracy of ONT might require the read depth to be higher and the quality control of the produced reads to be more thorough to mitigate the erroneous reads that may cause identifying a same DNA sequence as different sequences (Claesson et al., 2010; Stefan et al., 2022). Therefore, the misclassification of Klebsiella oxytoca as Enterobacter can occur even if the same reference is used for taxonomy (Stefan et al., 2022). Thirdly, this difference can be seen in the case of low-complexity samples such as infant feces (Winand et al., 2019).
Despite the current limitations, the expected advantages of ONT sequencing are as follows; (1) it provides near real-time analysis of DNA sequences when coupled with cloud-based software, which can save time by identifying antibiotic-resistant bacteria, (2) it can enable the rapid selection of appropriate antibiotics in a clinical setting such as NICU (Ashton et al., 2015; Leggett et al., 2020; Stefan et al., 2022), and (3) in comparison to short-read data such as Illumina, bacterial identification at the species-level works well. At phylum level, the high accuracy of Illumina reads demonstrated superb classification performance, being able to use higher percent identity in the alignment compared to ONT data. However, it was comparably similar in genus level analysis, and in species-level, only ONT data was utilizable as majority of V3–V4 Illumina reads were not assigned. The specific details of unassigned portion of the reads are provided in detail (Supplementary Table 7).
The empirical differences in taxonomic analysis based on Illumina and ONT reads are based on their innate differences in resolving power in case of 16S rRNA gene-based analysis. The error rate of ONT sequencing, for the R9.4.1 pores and the corresponding chemistry, typically averages in the range of Phred Q-score of 10–15, which corresponds to 90.0 to 96.8% read accuracy. Thus, the lower percent identity threshold of 85% was implemented, as higher parameter has a possibility to erroneously assign the reads. Nevertheless, we observed that the ONT sequencing reads were able to be discerned down to species-level. This phenomenon can be described as follows. In terms of theoretical background, typically ~97% identity is applied for high-accuracy short-reads (i.e., Illumina, Ion Torrent, etc.), as they have very high read accuracy, and because their read length is short, which usually spans just one or two hypervariable regions of the 16S rRNA gene. Even with the longest short-read target region of V3-V4 (341F & 805R), which is a popular universal primer set employed for prokaryotic microbiome analysis, the amplicon size is 460 bp. Here, with 97% identity, around 445 bp is used to resolve the taxonomy. On the other hand, ONT-based full 16S hypervariable region analysis (V1-V9) has an amplicon size of 1,465 bp, and with an identity threshold of 85%, around 1,170 bp is utilized to resolve the taxonomy. This requires the percent identity threshold to be lower than the read accuracy, thus the parameter of 85% was used for the taxonomic analysis of the ONT reads. Nevertheless, even with the lower percent identity threshold, the ONT reads have around 2.6 times higher amplicon templates to resolve the read (445 bp vs. 1,170 bp). With the V3-V4 amplicon reads successfully resolving most of the reads down to genus level, we observed that V1-V9 amplicon reads, even when the percent identity of 85%, were able to be effectively discerned down to species-level, which was reported to be achievable with the older version of nanopores (Benítez-Páez et al., 2016; Shin et al., 2016), and now being more reliable with the updated pore version of R9.4.1 with higher read accuracy. The species-level analysis using ONT reads on 16S rRNA gene full-region has been continuously being reported with successful results (Nygaard et al., 2020; Huggins et al., 2022; Rozas et al., 2022), also in clinical targets (Komiya et al., 2022), even bringing forth the developments of ONT-dedicated species-level analysis workflows such as NanoCLUST (Rodríguez-Pérez et al., 2021).
Generally, the microbiome of healthy T and P infants after birth consists of four phyla: Firmicutes, Proteobacteria, Actinobacteria, and Bacteroidetes (Arboleya et al., 2015). Bacterial colonization in the gut changes rapidly after birth, especially for aerotolerant microbes (Unger et al., 2015). Infants have a primarily aerobic gastrointestinal tract, which promotes the appearance of facultative anaerobic bacteria, such as Firmicutes (Enterococcus, Staphylococcus, Streptococcus) and Proteobacteria (Enterobacter, Escherichia) (Senn et al., 2020). P infants show low diversity with increased colonization of potentially pathogenic bacteria such as Proteobacteria (Klebsiella and Enterococcus) and reduced levels of strict anaerobes such as Bifidobacterium, Bacteroides, and Atopobium (Rinninella et al., 2019). Consistent with the results of previous studies, our study also identified that T and P infants’ guts were dominated by Firmicutes (Arboleya et al., 2012, 2015), and P infants had higher Proteobacteria and fewer Actinobacteria at the phylum level (Tanaka et al., 2009; Fouhy et al., 2012). It also showed that preterm birth results in inadequate bacterial colonization for 28 days.
At the genus level, P infants had a significantly low abundance of protective commensal anaerobic bacteria such as Bifidobacterium and Lactobacillus. As Bifidobacterium has been associated with the expression of inflammatory response genes and the stimulation of genes that promote the integrity of the mucosal barrier, the risk of NEC in preterm infants increases with a decline in Bifidobacterium, resulting in an exaggerated inflammatory response (Esaiassen et al., 2017). Lactic acid bacteria such as Lactobacillus gasseri elicit various health benefits through their antimicrobial activity, bacteriocin production, and immunomodulation of innate and adaptive systems (Rodrigues da Cunha et al., 2012; Selle and Klaenhammer, 2013). In this study, over time, the abundance of Bifidobacterium and Lactobacillus increased in T infants but was significantly lower than that in P infants. Furthermore, P infants had excessive growth of potentially pathogenic bacteria, such as Klebsiella and Enterococcus over time. This finding is consistent with the results of previous studies (Tanaka et al., 2009; Zhu et al., 2017; Zou et al., 2018; Lu et al., 2020; Chang et al., 2021).
This study showed a significant increase in Klebsiella in P infants within 28 days, and among the Klebsiella, K. pneumoniae was dominant at the species-level. Klebsiella is a gram-negative bacterium, and one of the most common species is K. pneumoniae. Klebsiella is important in P infants, because Klebsiella overgrowth in the gut is highly associated with brain damage in EP infants and also associated with NEC, nosocomial infections, and late-onset sepsis (Carlisle et al., 2011; Zhuang et al., 2019; Chen et al., 2020; Paveglio et al., 2020; Ma et al., 2021; Seki et al., 2021). Gestational age remains a major determinant of gut microbiota colonization (Rinninella et al., 2019) and immunity. Younger individuals have a more immature immune system and a higher intestinal mucosal permeability (Sevastiadou et al., 2011; Strunk et al., 2011; Arboleya et al., 2015; Ananth et al., 2018). Compared with the gut microbiota of T infants, that of P infants is always characterized by decreased microbial diversity (Henderickx et al., 2019), but in our study, there was no difference in the evenness and richness of the microbiota in T and P infants. T showed significantly higher evenness and richness of gut microbiota over time, but there was no difference in P, which correlates with the results of previous studies (Arboleya et al., 2015; Chernikova et al., 2018; Chang et al., 2021; Kim et al., 2021).
Many studies have shown that the use of antibiotics reduces diversity and leads to dysbiosis of the microbiome. Previous studies have shown a decrease in Actinobacteria (including Bifidobacteria), and Bacteroidetes. In contrast, an increase was observed in Proteobacteria (including Klebsiella and Escherichia), and Enterococcus (Arboleya et al., 2015; Lu et al., 2020). Our study also showed consistent results with previous studies with decreased Bifidobacteria and increased Klebsiella. However, in this study, most preterm infants were exposed to antibiotics after birth, so it is difficult to know exactly whether the above results are due to antibiotics or gestational age. Other recent studies showed different results. Kim et al. (2021) showed that Actinobacteria were rather more abundant in the antibiotic-treated group. Moreover, the type, duration of usage, and combination of antibiotics may be more important factors than antibiotics use (Zhu et al., 2017).
Gut microbiome is affected by delivery mode. Cesarean section delayed the establishment of gut microbiota such as Bifidobacteria and Bacteroidetes (Kim et al., 2020). However, most high-risk neonates such as preterm infants are very often placed in situations where they were inevitably forced to be delivered by cesarean section to improve their survival (Ananth and Vintzileos, 2011). Consequently, we could not adjust this factor between two groups. Additionally, some studies have shown that the mode of delivery could influence the first colonizing microbes of the gut, but has less effect on the gut microbiome with time (Chu et al., 2017). La Rosa et al. (2014) showed preterm infants with a patterned progression of the gut bacterial community that is only minimally influenced by delivery modes, antibiotics, or feeds.
This study’s strength is in comparing the gut microbiome of P and T infants at two different time points using ONT and Illumina sequencing. Although there have been studies comparing the gut microbiome of T and P infants using Illumina (Chernikova et al., 2018; Jia et al., 2022), and studies identifying the gut microbiome of P infants using ONT (Ashton et al., 2015; Leggett et al., 2020), to our knowledge, this is the first study of gut microbiome comparison between T and P infants using ONT and Illumina together.
Nonetheless, this study has some limitations that need to be addressed in further studies. First, the number of samples is small, and there are missing data due to gDNA extraction failure or amplicons generation from 7-day samples. Some meconium 7-day samples did not show detectable microbiomes (Kennedy et al., 2021), which may be associated due to insufficient microbiota (Klopp et al., 2022). Moreover, Illumina sequencing platform was utilized for some 7-day samples, while other samples were analyzed on ONT. The differences in detection rates may have resulted from the innate differences of the 16S rRNA gene target regions of the two universal primer sets used in Illumina (341F-805R for V3-V4 region) and ONT (27F-1492R for V1-V9 region), as previously reported before the sample target region sets (Strube, 2021) and others such as V3-V4 to V4-V5 (Biol-Aquino et al., 2019; Fadeev et al., 2021) and V4-V5 to V5-V8 (McNichol et al., 2021) among many others. Therefore, the data not shown in our analysis are not missing at random, which can lead to comparison bias. In the future, finding from the above study can be helpful in designing amplicon sequencing strategies for 16S rRNA gene variable region. Finally, most preterm infants were given prophylactic antibiotics and delivered with Cesarean section, the condition of which has presumably affected the lower microbial abundance in the collected samples.
Our results demonstrate for the first time that gestational age affects the evolution of gut microbiota in terms of composition and diversities, using ONT and Illumina together. The ONT sequencing was shown to have a high correlation with Illumina sequencing and may prove useful in clinical settings, particularly for infants’ microbial analysis and tailored intervention.
The data presented in the study are deposited in the NCBI Sequence Read Archive repository, accession number PRJNA871395.
The studies involving human participants were reviewed and approved by the Institutional Review Board of Hanyang University Medical Center (No. 2021-03-017). Written informed consent to participate in this study was provided by the participants’ legal guardian/next of kin.
H-KP and B-HJ conceptualized, designed, and supervised the study. HK, M-JK, and JYP were involved in the data collection and initial statistical analysis. TC and JK performed the computational analyses, developed the algorithms, and interpreted the data. TC, JK, and H-KP wrote and edited the manuscript. All authors have reviewed and approved the revised manuscript.
This research was funded by the Hanyang University (MEB) Global Center for Developmental Disorders (HY-202100000002865), Bio & Medical Technology Development Program of the National Research Foundation of Korea (NRF-2019M3E5D1A01069363).
We would like to acknowledge all our colleagues at the Hanyang University MEB (Medicine-Engineering-Bio) center. The authors gratefully acknowledge the first president Dong-Hyun Ahn of the Hanyang Inclusive Clinic for Developmental Disorders in Hanyang University College of Medicine for his helpful discussions, as well as CEO Gang Pyo Lee, CTO JP, and researcher Hee Yeon Jin of Int-Gen, Seoul, Republic of Korea, which conducts microbiome and genetic research.
The authors declare that the research was conducted in the absence of any commercial or financial relationships that could be construed as a potential conflict of interest.
All claims expressed in this article are solely those of the authors and do not necessarily represent those of their affiliated organizations, or those of the publisher, the editors and the reviewers. Any product that may be evaluated in this article, or claim that may be made by its manufacturer, is not guaranteed or endorsed by the publisher.
The Supplementary material for this article can be found online at: https://www.frontiersin.org/articles/10.3389/fmicb.2023.1148466/full#supplementary-material
ONT, Oxford Nanopore Technologies; NICU, neonatal intensive care unit; NGS, next generation sequencing; T, term infants; P, preterm infants; GA, gestational age; VP, very preterm infants; LP, moderate to late preterm; NEC, necrotizing enterocolitis; GDM, gestational diabetes mellitus; PIH, pregnancy-induced hypertension; OTUs, operational taxonomic units; ASVs, amplicon sequence variants.
Ananth, C. V., Goldenberg, R. L., Friedman, A. M., and Vintzileos, A. M. (2018). Association of temporal changes in gestational age with perinatal mortality in the United States, 2007-2015. JAMA Pediatr. 172, 627–634. doi: 10.1001/jamapediatrics.2018.0249
Ananth, C. V., and Vintzileos, A. M. (2011). Trends in cesarean delivery at preterm gestation and association with perinatal mortality. Am. J. Obstet. Gynecol. 204:505.e1-8. doi: 10.1016/j.ajog.2011.01.062
Arboleya, S., Binetti, A., Salazar, N., Fernández, N., Solís, G., Hernandez-Barranco, A., et al. (2012). Establishment and development of intestinal microbiota in preterm neonates. FEMS Microbiol. Ecol. 79, 763–772. doi: 10.1111/j.1574-6941.2011.01261.x
Arboleya, S., Sánchez, B., Milani, C., Duranti, S., Solís, G., Fernández, N., et al. (2015). Intestinal microbiota development in preterm neonates and effect of perinatal antibiotics. J. Pediatr. 166, 538–544. doi: 10.1016/j.jpeds.2014.09.041
Ashton, P. M., Nair, S., Dallman, T., Rubino, S., Rabsch, W., Mwaigwisya, S., et al. (2015). Min ION nanopore sequencing identifies the position and structure of a bacterial antibiotic resistance island. Nat. Biotechnol. 33, 296–300. doi: 10.1038/nbt.3103
Benítez-Páez, A., Portune, K. J., and Sanz, Y. (2016). Species-level resolution of 16S rRNA gene amplicons sequenced through the min ION™ portable nanopore sequencer. Gigascience 5:4. doi: 10.1186/s13742-016-0111-z
Biol-Aquino, M. A., Perdiz, C. J., Borlagdan, M., Alcantara, J. D., and Mallillin, A. (2019). Differences in the bacterial profiles of infant gut by birth process, milk diet, and choice of 16S rRNA gene target region. Hum. Microbiome J. 13:100062. doi: 10.1016/j.humic.2019.100062
Bolyen, E., Rideout, J. R., Dillon, M. R., Bokulich, N. A., Abnet, C. C., Al-Ghalith, G. A., et al. (2019). Reproducible, interactive, scalable and extensible microbiome data science using QIIME 2 (vol 37, pg 852, 2019). Nat. Biotechnol. 37:1091. doi: 10.1038/s41587-019-0252-6
Callahan, B. J., Mcmurdie, P. J., Rosen, M. J., Han, A. W., Johnson, A. J. A., and Holmes, S. P. (2016). DADA2: high-resolution sample inference from Illumina amplicon data. Nat. Methods 13, 581–583. doi: 10.1038/nmeth.3869
Carl, M.A., Ndao, I.M., Springman, A.C., Manning, S.D., Johnson, J.R., and Johnston, B.D., Burnham, C.-a. D., Weinstock, E.S., Weinstock, G.M., and Wylie, T.N. (2014). Sepsis from the gut: the enteric habitat of bacteria that cause late-onset neonatal bloodstream infections. Clin. Infect. Dis. 58, 1211–1218. doi: 10.1093/cid/ciu084
Carlisle, E. M., Poroyko, V., Caplan, M. S., Alverdy, J. A., and Liu, D. (2011). Gram negative bacteria are associated with the early stages of necrotizing enterocolitis. PLoS One 6:e18084. doi: 10.1371/journal.pone.0018084
Chang, H.-Y., Chiang Chiau, J.-S., Ho, Y.-H., Chang, J.-H., Tsai, K.-N., Liu, C.-Y., et al. (2021). Impact of early empiric antibiotic regimens on the gut microbiota in very low birth weight preterm infants: an observational study. Front. Pediatr. 9:514. doi: 10.3389/fped.2021.651713
Chen, Y., Brook, T. C., Soe, C. Z., Oneill, I., Alcon-Giner, C., Leelastwattanagul, O., et al. (2020). Preterm infants harbour diverse Klebsiella populations, including atypical species that encode and produce an array of antimicrobial resistance-and virulence-associated factors. Microb. Genom. 6:e000377. doi: 10.1099/mgen.0.000377
Chen, S. F., Zhou, Y. Q., Chen, Y. R., and Gu, J. (2018). fastp: an ultra-fast all-in-one FASTQ preprocessor. Bioinformatics 34, i884–i890. doi: 10.1093/bioinformatics/bty560
Chernikova, D. A., Madan, J. C., Housman, M. L., Zain-Ul-Abideen, M., Lundgren, S. N., Morrison, H. G., et al. (2018). The premature infant gut microbiome during the first 6 weeks of life differs based on gestational maturity at birth. Pediatr. Res. 84, 71–79. doi: 10.1038/s41390-018-0022-z
Chu, D. M., Ma, J., Prince, A. L., Antony, K. M., Seferovic, M. D., and Aagaard, K. M. (2017). Maturation of the infant microbiome community structure and function across multiple body sites and in relation to mode of delivery. Nat. Med. 23, 314–326. doi: 10.1038/nm.4272
Claesson, M. J., Wang, Q., O'sullivan, O., Greene-Diniz, R., Cole, J. R., Ross, R. P., et al. (2010). Comparison of two next-generation sequencing technologies for resolving highly complex microbiota composition using tandem variable 16S rRNA gene regions. Nucleic Acids Res. 38:e200. doi: 10.1093/nar/gkq873
DiGiulio, D. B. (2012). Diversity of microbes in amniotic fluid. Semin. Fetal Neonatal Med. 17, 2–11. doi: 10.1016/j.siny.2011.10.001
Dominguez-Bello, M. G., Costello, E. K., Contreras, M., Magris, M., Hidalgo, G., Fierer, N., et al. (2010). Delivery mode shapes the acquisition and structure of the initial microbiota across multiple body habitats in newborns. Proc. Natl. Acad. Sci. 107, 11971–11975. doi: 10.1073/pnas.1002601107
Ellegaard, K. M., Suenami, S., Miyazaki, R., and Engel, P. (2020). Vast differences in strain-level diversity in the gut microbiota of two closely related honey bee species. Curr. Biol. 30, 2520–2531.e7. doi: 10.1016/j.cub.2020.04.070
Esaiassen, E., Fjalstad, J. W., Juvet, L. K., Van Den Anker, J. N., and Klingenberg, C. (2017). Antibiotic exposure in neonates and early adverse outcomes: a systematic review and meta-analysis. J. Antimicrob. Chemother. 72, 1858–1870. doi: 10.1093/jac/dkx088
Fadeev, E., Cardozo-Mino, M. G., Rapp, J. Z., Bienhold, C., Salter, I., Salman-Carvalho, V., et al. (2021). Comparison of two 16S rRNA primers (V3–V4 and V4–V5) for studies of arctic microbial communities. Front. Microbiol. 12:637526. doi: 10.3389/fmicb.2021.637526
Fallani, M., Young, D., Scott, J., Norin, E., Amarri, S., Adam, R., et al. (2010). Intestinal microbiota of 6-week-old infants across Europe: geographic influence beyond delivery mode, breast-feeding, and antibiotics. J. Pediatr. Gastroenterol. Nutr. 51, 77–84. doi: 10.1097/MPG.0b013e3181d1b11e
Fouhy, F., Guinane, C. M., Hussey, S., Wall, R., Ryan, C. A., Dempsey, E. M., et al. (2012). High-throughput sequencing reveals the incomplete, short-term recovery of infant gut microbiota following parenteral antibiotic treatment with ampicillin and gentamicin. Antimicrob. Agents Chemother. 56, 5811–5820. doi: 10.1128/AAC.00789-12
Frank, J. A., Reich, C. I., Sharma, S., Weisbaum, J. S., Wilson, B. A., and Olsen, G. J. (2008). Critical evaluation of two primers commonly used for amplification of bacterial 16S rRNA genes. Appl. Environ. Microbiol. 74, 2461–2470. doi: 10.1128/AEM.02272-07
Greninger, A. L., Naccache, S. N., Federman, S., Yu, G., Mbala, P., Bres, V., et al. (2015). Rapid metagenomic identification of viral pathogens in clinical samples by real-time nanopore sequencing analysis. Genome Med. 7, 1–13. doi: 10.1186/s13073-015-0220-9
Hansen, R., Scott, K. P., Khan, S., Martin, J. C., Berry, S. H., Stevenson, M., et al. (2015). First-pass meconium samples from healthy term vaginally-delivered neonates: an analysis of the microbiota. PLoS One 10:e0133320. doi: 10.1371/journal.pone.0133320
Healy, D. B., Ryan, C. A., Ross, R. P., Stanton, C., and Dempsey, E. M. (2022). Clinical implications of preterm infant gut microbiome development. Nat. Microbiol. 7, 22–33. doi: 10.1038/s41564-021-01025-4
Henderickx, J. G., Zwittink, R. D., Van Lingen, R. A., Knol, J., and Belzer, C. (2019). The preterm gut microbiota: an inconspicuous challenge in nutritional neonatal care. Front. Cell. Infect. Microbiol. 9:85. doi: 10.3389/fcimb.2019.00085
Huggins, L. G., Colella, V., Atapattu, U., Koehler, A. V., and Traub, R. J. (2022). Nanopore sequencing using the full-length 16S rRNA gene for detection of blood-borne Bacteria in dogs reveals a novel species of hemotropic mycoplasma. Microbiol. Spectr. 10:e03088-22. doi: 10.1128/spectrum.03088-22
Jia, Q., Yu, X., Chang, Y., You, Y., Chen, Z., Wang, Y., et al. (2022). Dynamic changes of the gut microbiota in preterm infants with different gestational age. Front. Microbiol. 13:923273. doi: 10.3389/fmicb.2022.923273
Katoh, K., Misawa, K., Kuma, K., and Miyata, T. (2002). MAFFT: a novel method for rapid multiple sequence alignment based on fast Fourier transform. Nucleic Acids Res. 30, 3059–3066. doi: 10.1093/nar/gkf436
Kennedy, K. M., Gerlach, M. J., Adam, T., Heimesaat, M. M., Rossi, L., Surette, M. G., et al. (2021). Fetal meconium does not have a detectable microbiota before birth. Nat. Microbiol. 6, 865–873. doi: 10.1038/s41564-021-00904-0
Kim, G., Bae, J., Kim, M. J., Kwon, H., Park, G., Kim, S.-J., et al. (2020). Delayed establishment of gut microbiota in infants delivered by cesarean section. Front. Microbiol. 11:2099. doi: 10.3389/fmicb.2020.02099
Kim, C. S., Grady, N., Derrick, M., Yu, Y., Oliphant, K., Lu, J., et al. (2021). Effect of antibiotic use within first 48 hours of life on the preterm infant microbiome: a randomized clinical trial. JAMA Pediatr. 175, 303–305. doi: 10.1001/jamapediatrics.2020.4916
Klindworth, A., Pruesse, E., Schweer, T., Peplies, J., Quast, C., Horn, M., et al. (2013). Evaluation of general 16S ribosomal RNA gene PCR primers for classical and next-generation sequencing-based diversity studies. Nucleic Acids Res. 41:e1. doi: 10.1093/nar/gks808
Klopp, J., Ferretti, P., Meyer, C. U., Hilbert, K., Haiß, A., Marißen, J., et al. (2022). Meconium microbiome of very preterm infants across Germany. mSphere 7:e00808-21. doi: 10.1128/msphere.00808-21
Koleva, P. T., Kim, J. S., Scott, J. A., and Kozyrskyj, A. L. (2015). Microbial programming of health and disease starts during fetal life. Birth Defects Res. C. Embryo Today 105, 265–277. doi: 10.1002/bdrc.21117
Komiya, S., Matsuo, Y., Nakagawa, S., Morimoto, Y., Kryukov, K., Okada, H., et al. (2022). Min ION, a portable long-read sequencer, enables rapid vaginal microbiota analysis in a clinical setting. BMC Med. Genet. 15, 1–10. doi: 10.1186/s12920-022-01218-8
Korpela, K., Blakstad, E. W., Moltu, S. J., Strømmen, K., Nakstad, B., Rønnestad, A. E., et al. (2018). Intestinal microbiota development and gestational age in preterm neonates. Sci. Rep. 8, 1–9. doi: 10.1038/s41598-018-20827-x
La Rosa, P. S., Warner, B. B., Zhou, Y., Weinstock, G. M., Sodergren, E., Hall-Moore, C. M., et al. (2014). Patterned progression of bacterial populations in the premature infant gut. Proc. Natl. Acad. Sci. 111, 12522–12527. doi: 10.1073/pnas.1409497111
Leggett, R. M., Alcon-Giner, C., Heavens, D., Caim, S., Brook, T. C., Kujawska, M., et al. (2020). Rapid min ION profiling of preterm microbiota and antimicrobial-resistant pathogens. Nat. Microbiol. 5, 430–442. doi: 10.1038/s41564-019-0626-z
Lozupone, C., Lladser, M. E., Knights, D., Stombaugh, J., and Knight, R. (2011). Uni Frac: an effective distance metric for microbial community comparison. ISME J. 5, 169–172. doi: 10.1038/ismej.2010.133
Lu, S., Huang, Q., Wei, B., and Chen, Y. (2020). Effects of β-lactam antibiotics on gut microbiota colonization and metabolites in late preterm infants. Curr. Microbiol. 77, 3888–3896. doi: 10.1007/s00284-020-02198-7
Ma, L., Peng, Q., Wang, W., Yang, Y., Chen, Y., Wang, L., et al. (2021). Late-onset sepsis in very low birth weight preterm infants: 7 years' experience at a tertiary hospital in China. Pediatr. Neonatol. 62, 529–535. doi: 10.1016/j.pedneo.2021.05.011
Martí, M., Spreckels, J. E., Jenmalm, M. C., and Abrahamsson, T. (2021). A protocol for characterization of extremely preterm infant gut microbiota in double-blind clinical trials. STAR Protoc. 2:100652. doi: 10.1016/j.xpro.2021.100652
Mcnichol, J., Berube, P. M., Biller, S. J., and Fuhrman, J. A. (2021). Evaluating and improving small subunit rRNA PCR primer coverage for bacteria, archaea, and eukaryotes using metagenomes from global ocean surveys. mSystems 6:e0056521. doi: 10.1128/mSystems.00565-21
Nygaard, A. B., Tunsjø, H. S., Meisal, R., and Charnock, C. (2020). A preliminary study on the potential of nanopore min ION and Illumina MiSeq 16S rRNA gene sequencing to characterize building-dust microbiomes. Sci. Rep. 10:3209. doi: 10.1038/s41598-020-59771-0
Palmer, C., Bik, E. M., Digiulio, D. B., Relman, D. A., and Brown, P. O. (2007). Development of the human infant intestinal microbiota. PLoS Biol. 5:e177. doi: 10.1371/journal.pbio.0050177
Paveglio, S., Ledala, N., Rezaul, K., Lin, Q., Zhou, Y., Provatas, A. A., et al. (2020). Cytotoxin-producing Klebsiella oxytoca in the preterm gut and its association with necrotizing enterocolitis. Emerg. Microbes Infect. 9, 1321–1329. doi: 10.1080/22221751.2020.1773743
Pichler, M., Coskun, Ø. K., Ortega-Arbulú, A. S., Conci, N., Wörheide, G., Vargas, S., et al. (2018). A 16S rRNA gene sequencing and analysis protocol for the Illumina Mini Seq platform. Microbiology 7:e00611. doi: 10.1002/mbo3.611
Price, M. N., Dehal, P. S., and Arkin, A. P. (2010). Fast tree 2-approximately maximum-likelihood trees for large alignments. PLoS One 5:e9490. doi: 10.1371/journal.pone.0009490
Quast, C., Pruesse, E., Yilmaz, P., Gerken, J., Schweer, T., Yarza, P., et al. (2013). The SILVA ribosomal RNA gene database project: improved data processing and web-based tools. Nucleic Acids Res. 41, D590–D596. doi: 10.1093/nar/gks1219
Quick, J., Loman, N. J., Duraffour, S., Simpson, J. T., Severi, E., Cowley, L., et al. (2016). Real-time, portable genome sequencing for Ebola surveillance. Nature 530, 228–232. doi: 10.1038/nature16996
Rinninella, E., Raoul, P., Cintoni, M., Franceschi, F., Miggiano, G. A. D., Gasbarrini, A., et al. (2019). What is the healthy gut microbiota composition? A changing ecosystem across age, environment, diet, and diseases. Microorganisms 7:14. doi: 10.3390/microorganisms7010014
Robeson, M. S., O'rourke, D. R., Kaehler, B. D., Ziemski, M., Dillon, M. R., Foster, J. T., et al. (2021). RESCRIPt: reproducible sequence taxonomy reference database management. PLoS Comput. Biol. 17:e1009581. doi: 10.1371/journal.pcbi.1009581
Rodrigues Da Cunha, L., Ferreira, C. L. F., Durmaz, E., Goh, Y. J., Sanozky-Dawes, R., and Klaenhammer, T. (2012). Characterization of Lactobacillus gasseri isolates from a breast-fed infant. Gut Microbes 3, 15–24. doi: 10.4161/gmic.19489
Rodríguez-Pérez, H., Ciuffreda, L., and Flores, C. (2021). Nano CLUST: a species-level analysis of 16S rRNA nanopore sequencing data. Bioinformatics 37, 1600–1601. doi: 10.1093/bioinformatics/btaa900
Rognes, T., Flouri, T., Nichols, B., Quince, C., and Mahe, F. (2016). VSEARCH: a versatile open source tool for metagenomics. PeerJ 4:e2584. doi: 10.7717/peerj.2584
Rozas, M., Brillet, F., Callewaert, C., and Paetzold, B. (2022). Min ION™ nanopore sequencing of skin microbiome 16S and 16S-23S rRNA gene amplicons. Front. Cell. Infect. Microbiol. 11:1317. doi: 10.3389/fcimb.2021.806476
Schmidt, K., Mwaigwisya, S., Crossman, L., Doumith, M., Munroe, D., Pires, C., et al. (2016). Identification of bacterial pathogens and antimicrobial resistance directly from clinical urines by nanopore-based metagenomic sequencing. J. Antimicrob. Chemother. 72, 104–114. doi: 10.1093/jac/dkw397
Schwiertz, A., Gruhl, B., Löbnitz, M., Michel, P., Radke, M., and Blaut, M. (2003). Development of the intestinal bacterial composition in hospitalized preterm infants in comparison with breast-fed, full-term infants. Pediatr. Res. 54, 393–399. doi: 10.1203/01.PDR.0000078274.74607.7A
Seki, D., Mayer, M., Hausmann, B., Pjevac, P., Giordano, V., Goeral, K., et al. (2021). Aberrant gut-microbiota-immune-brain axis development in premature neonates with brain damage. Cell Host Microbe 29, 1558–1572.e6. doi: 10.1016/j.chom.2021.08.004
Selle, K., and Klaenhammer, T. R. (2013). Genomic and phenotypic evidence for probiotic influences of Lactobacillus gasseri on human health. FEMS Microbiol. Rev. 37, 915–935. doi: 10.1111/1574-6976.12021
Senn, V., Bassler, D., Choudhury, R., Scholkmann, F., Righini-Grunder, F., Vuille-Dit-Bille, R. N., et al. (2020). Microbial colonization from the fetus to early childhood—a comprehensive review. Front. Cell. Infect. Microbiol. 10:573735. doi: 10.3389/fcimb.2020.573735
Sevastiadou, S., Malamitsi-Puchner, A., Costalos, C., Skouroliakou, M., Briana, D. D., Antsaklis, A., et al. (2011). The impact of oral glutamine supplementation on the intestinal permeability and incidence of necrotizing enterocolitis/septicemia in premature neonates. J. Matern. Fetal Neonatal Med. 24, 1294–1300. doi: 10.3109/14767058.2011.564240
Sherman, M. P. (2010). New concepts of microbial translocation in the neonatal intestine: mechanisms and prevention. Clin. Perinatol. 37, 565–579. doi: 10.1016/j.clp.2010.05.006
Shin, J., Lee, S., Go, M.-J., Lee, S. Y., Kim, S. C., Lee, C.-H., et al. (2016). Analysis of the mouse gut microbiome using full-length 16S rRNA amplicon sequencing. Sci. Rep. 6:29681. doi: 10.1038/srep29681
Stefan, C. P., Hall, A. T., Graham, A. S., and Minogue, T. D. (2022). Comparison of Illumina and oxford nanopore sequencing technologies for pathogen detection from clinical matrices using molecular inversion probes. J. Mol. Diagn. 24, 395–405. doi: 10.1016/j.jmoldx.2021.12.005
Strube, M. L. (2021). RibDif: can individual species be differentiated by 16S sequencing? Bioinform. Adv. 1:vbab020. doi: 10.1093/bioadv/vbab020
Strunk, T., Currie, A., Richmond, P., Simmer, K., and Burgner, D. (2011). Innate immunity in human newborn infants: prematurity means more than immaturity. J. Matern. Fetal Neonatal Med. 24, 25–31. doi: 10.3109/14767058.2010.482605
Tanaka, S., Kobayashi, T., Songjinda, P., Tateyama, A., Tsubouchi, M., Kiyohara, C., et al. (2009). Influence of antibiotic exposure in the early postnatal period on the development of intestinal microbiota. FEMS Immunol. Med. Microbiol. 56, 80–87. doi: 10.1111/j.1574-695X.2009.00553.x
Tarr, P. I., and Warner, B. B. (2016). Gut bacteria and late-onset neonatal bloodstream infections in preterm infants. Semin. Fetal Neonatal Med. 21, 388–393. doi: 10.1016/j.siny.2016.06.002
Unger, S., Stintzi, A., Shah, P., Mack, D., and O'connor, D. L. (2015). Gut microbiota of the very-low-birth-weight infant. Pediatr. Res. 77, 205–213. doi: 10.1038/pr.2014.162
Winand, R., Bogaerts, B., Hoffman, S., Lefevre, L., Delvoye, M., Van Braekel, J., et al. (2019). Targeting the 16s rRNA gene for bacterial identification in complex mixed samples: comparative evaluation of second (illumina) and third (oxford nanopore technologies) generation sequencing technologies. Int. J. Mol. Sci. 21:298. doi: 10.3390/ijms21010298
Yan, Y., Nguyen, L. H., Franzosa, E. A., and Huttenhower, C. (2020). Strain-level epidemiology of microbial communities and the human microbiome. Genome Med. 12:71. doi: 10.1186/s13073-020-00765-y
Zhu, D., Xiao, S., Yu, J., Ai, Q., He, Y., Cheng, C., et al. (2017). Effects of one-week empirical antibiotic therapy on the early development of gut microbiota and metabolites in preterm infants. Sci. Rep. 7, 1–10. doi: 10.1038/s41598-017-08530-9
Zhuang, L., Chen, H., Zhang, S., Zhuang, J., Li, Q., and Feng, Z. (2019). Intestinal microbiota in early life and its implications on childhood health. Genomics Proteomics Bioinformatics 17, 13–25. doi: 10.1016/j.gpb.2018.10.002
Keywords: gut microbiome, neonates, Illumina MiSeq®, Nanopore MinION, preterm infants
Citation: Cha T, Kim HH, Keum J, Kwak M-J, Park JY, Hoh JK, Kim C-R, Jeon B-H and Park H-K (2023) Gut microbiome profiling of neonates using Nanopore MinION and Illumina MiSeq sequencing. Front. Microbiol. 14:1148466. doi: 10.3389/fmicb.2023.1148466
Received: 20 January 2023; Accepted: 17 April 2023;
Published: 15 May 2023.
Edited by:
Palmiro Poltronieri, National Research Council (CNR), ItalyReviewed by:
Kelvin Li, University of Pittsburgh, United StatesCopyright © 2023 Cha, Kim, Keum, Kwak, Park, Hoh, Kim, Jeon and Park. This is an open-access article distributed under the terms of the Creative Commons Attribution License (CC BY). The use, distribution or reproduction in other forums is permitted, provided the original author(s) and the copyright owner(s) are credited and that the original publication in this journal is cited, in accordance with accepted academic practice. No use, distribution or reproduction is permitted which does not comply with these terms.
*Correspondence: Byong-Hun Jeon, YmhqZW9uQGhhbnlhbmcuYWMua3I=; Hyun-Kyung Park, bmVvcGFya0BoYW55YW5nLmFjLmty
†These authors have contributed equally to this work
Disclaimer: All claims expressed in this article are solely those of the authors and do not necessarily represent those of their affiliated organizations, or those of the publisher, the editors and the reviewers. Any product that may be evaluated in this article or claim that may be made by its manufacturer is not guaranteed or endorsed by the publisher.
Research integrity at Frontiers
Learn more about the work of our research integrity team to safeguard the quality of each article we publish.