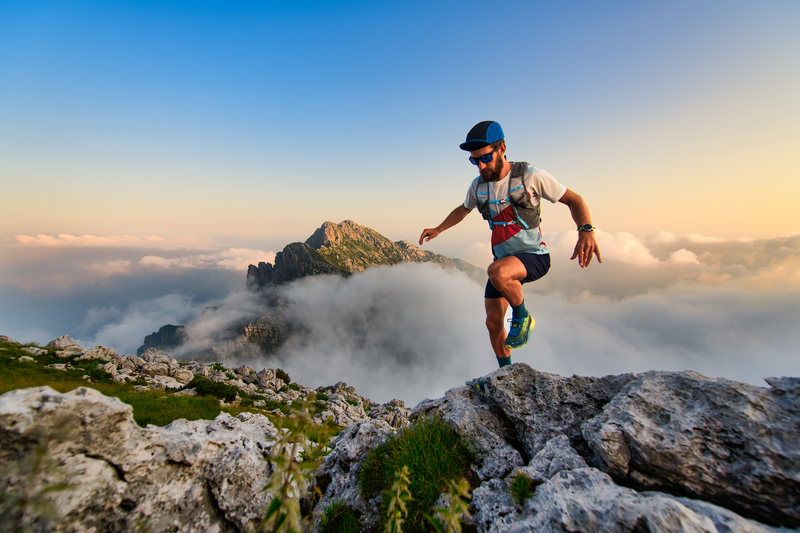
94% of researchers rate our articles as excellent or good
Learn more about the work of our research integrity team to safeguard the quality of each article we publish.
Find out more
ORIGINAL RESEARCH article
Front. Microbiol. , 06 April 2023
Sec. Food Microbiology
Volume 14 - 2023 | https://doi.org/10.3389/fmicb.2023.1141043
This article is part of the Research Topic Prevalence and Control Measures of Food-borne Pathogens View all 10 articles
The contamination of fresh produce with foodborne pathogens has been an on-going concern with outbreaks linked to these commodities. Evaluation of farm practices, such as use of manure, irrigation water source, and other factors that could influence pathogen prevalence in the farming environment could lead to improved mitigation strategies to reduce the potential for contamination events. Soil, water, manure, and compost were sampled from farms in Ohio and Georgia to identify the prevalence of Salmonella, Listeria monocytogenes (Lm), Campylobacter, and Shiga-toxin-producing Escherichia coli (STEC), as well as Arcobacter, an emerging human pathogen. This study investigated agricultural practices to determine which influenced pathogen prevalence, i.e., the percent positive samples. These efforts identified a low prevalence of Salmonella, STEC, and Campylobacter in soil and water (< 10%), preventing statistical modeling of these pathogens. However, Lm and Arcobacter were found in soil (13 and 7%, respectively), manure (49 and 32%, respectively), and water samples (18 and 39%, respectively) at a comparatively higher prevalence, suggesting different dynamics are involved in their survival in the farm environment. Lm and Arcobacter prevalence data, soil chemical characteristics, as well as farm practices and weather, were analyzed using structural equation modeling to identify which factors play a role, directly or indirectly, on the prevalence of these pathogens. These analyses identified an association between pathogen prevalence and weather, as well as biological soil amendments of animal origin. Increasing air temperature increased Arcobacter and decreased Lm. Lm prevalence was found to be inversely correlated with the use of surface water for irrigation, despite a high Lm prevalence in surface water suggesting other factors may play a role. Furthermore, Lm prevalence increased when the microbiome’s Simpson’s Diversity Index decreased, which occurred as soil fertility increased, leading to an indirect positive effect for soil fertility on Lm prevalence. These results suggest that pathogen, environment, and farm management practices, in addition to produce commodities, all need to be considered when developing mitigation strategies. The prevalence of Arcobacter and Lm versus the other pathogens suggests that multiple mitigation strategies may need to be employed to control these pathogens.
Foodborne illness associated with the consumption of fresh produce, particularly leafy greens, tomatoes, cantaloupes, tree fruit, and peppers, continues to be a major health concern with numerous outbreaks linked to these commodities (Iwu and Okoh, 2019). This diversity in product type suggests that common risk factors should be considered when evaluating farming practices or developing on-farm risk-mitigation approaches, not just produce-specific factors. Numerous studies have identified bacterial pathogens in agricultural environments, clearly indicating the potential for contamination of produce during cultivation and harvest (Cooley et al., 2007; Jeyaletchumi et al., 2011; Micallef et al., 2012; Strawn et al., 2013a,b; Marine et al., 2015; Belias et al., 2021). An evaluation of bacterial pathogens linked to foodborne illness, conducted by the Interagency Food Safety Analytics Collaboration (IFSAC) implicated four key bacterial pathogens, non-typhoidal Salmonella, E. coli O157, Listeria monocytogenes (Lm), and Campylobacter spp., based on frequency and severity of illness. These findings agree with other efforts identifying these four bacterial pathogens as the primary concerns for foodborne illness (Scallan et al., 2011; IFSAC, 2018).
Non-typhoidal Salmonella and Shiga-toxin-producing E. coli (STEC) are associated with a large proportion of the burden associated with foodborne outbreaks. Salmonella causes an estimated one million cases and is responsible for over a third of the hospitalizations, annually (Scallan et al., 2011). Furthermore, from data derived from an active and passive surveillance study between 2000 and 2008, it was estimated that STEC cause roughly 265,000 illnesses annually in the United States (US), resulting in over 3,600 hospitalizations and 30 deaths (Scallan et al., 2011). Many serovars of non-typhoidal Salmonella and STEC are also enteric pathogens to a wide range of wild and domesticated animals (Hoelzer et al., 2011; Persad and LeJeune, 2015). Due to this burden, research has evaluated the potential of these pathogens to survive in manure and in the field environment (Himathongkham et al., 1999; Sharma and Reynnells, 2016; Hruby et al., 2018; Gu et al., 2019; Sharma et al., 2019). The presence of animal hosts in the pre-harvest environment and the application of manure or compost as a soil amendment may facilitate the dissemination and survival of Salmonella, as well as other pathogens, in soil (Shah et al., 2019; Bardsley et al., 2021).
Lm is well-characterized as a saprophytic bacterium and considered ubiquitous in soil and the environment and the causative agent of invasive listeriosis, a comparatively rare illness that causes ∼19% of foodborne illness deaths (Ivanek et al., 2006; Scallan et al., 2011; Vivant et al., 2013; Iwu and Okoh, 2019). Due to its established role as a saprophytic organism, Lm may be better adapted to soil and soil microbiome competition than STEC and Salmonella (Freitag, 2009). Data have shown an association of Lm with wet soil environments and rain, but longitudinal studies evaluating Lm in agricultural fields are limited (Locatelli et al., 2013; Falardeau et al., 2018; Harrand et al., 2020). Watershed studies have also demonstrated that Lm, if found in water and sediment samples, could be introduced into the field environment if contaminated water is used for irrigation (Gorski et al., 2014; Stea et al., 2015). Additionally, it has been demonstrated that soil type can impact Lm survival and/or growth (Dowe et al., 1997; Jiang et al., 2004; Brennan et al., 2014). Furthermore, manure usage can further support Lm survival, depending on manure type (Dowe et al., 1997; Jiang et al., 2004; Brennan et al., 2014). These observations indicate the need for risk assessments using a variety of conditions to better understand the factors at play in Lm agricultural prevalence.
Campylobacter species are the leading cause of human bacterial gastroenteritis (EFSA and ECDC, 2021), and are predominantly associated with the consumption and handling of improperly cooked poultry, raw milk, and water. However, Campylobacter outbreaks have been associated with row crops and other produce, representing 6 and 1.7% of Campylobacter outbreaks, respectively (Gardner et al., 2011; IFSAC, 2018; Sher et al., 2021). Similarly, Arcobacter spp., which belong to the family Campylobacteraceae are classified as a serious hazard to human health by the International Commission on Microbiological Specifications for Foods (ICMSF) (Hoa et al., 2006; Ramees et al., 2017). Recently, Arcobacter prevalence was reported in fresh produce (Abay et al., 2022, Ma et al., 2022), and they are widely distributed among animals and environmental water (Collado and Figueras, 2011; Ramees et al., 2017; Niedermeyer et al., 2020). Furthermore, Arcobacter spp. have been associated with foodborne outbreaks (Lappi et al., 2013; EFSA and ECDC, 2021; Uljanovas et al., 2021). There are few longitudinal studies assessing Campylobacter prevalence in farm environments and in produce, and none for Arcobacter (Chai et al., 2009; Guevremont et al., 2017). Thus, there is limited understanding of the presence of these pathogens in the produce farm environment, and transmission to humans.
Farm practices, including irrigation, soil amendment types, pest control, and worker hygiene, have been highlighted as potential sources of pathogen introduction to the farm environment and/or produce. For example, biological soil amendments of animal origin (BSAAO) have been associated with higher risk of pathogen introduction, in particular, when not properly treated prior to use (i.e., composted), (Iwu and Okoh, 2019). In addition to the application of BSAAO to the field, dispersal of pathogen-containing fecal material in the produce preharvest environment could occur via surface water runoff and/or animal intrusion (Atwill et al., 2012; Pandey et al., 2014; Sharma and Reynnells, 2016; Alegbeleye and Sant’Ana, 2020). Enteric pathogens have also been detected in source water or distribution systems of irrigation water used in produce farms in multiple US coastal states (Gu et al., 2013, 2020; Draper et al., 2016; Allard et al., 2019; Weller et al., 2020).
This study evaluated pathogen prevalence and survival in the pre-harvest environment in two US geographic regions. These data provide critical information to compare and evaluate the role of soil characteristics, climate, and farm management practices on pathogen prevalence, and improve the understanding of pathogen ecology in agricultural settings. This knowledge, especially when coupled with commodity-specific risk assessments, may aid in the development of targeted mitigation strategies to enhance the microbial safety of fresh produce.
The study was initiated in the 2018 growing season as a small pilot study to evaluate potential regional differences in pathogen prevalence, alongside evaluating the effect of some farm management practices on pathogen prevalence. In 2019, the study was expanded to include more pathogens, increased sampling, and the contribution of irrigation water sources. The study ended in 2020 due to COVID-19 restrictions preventing monthly sampling, with the heaviest restrictions coinciding with amendment application.
Farms were recruited in both Georgia (GA) and Ohio (OH) by voluntary agreement of the farmers. All farms were blinded for the purposes of this study. Four farms in GA elected to participate in 2018, two of which also participated in 2019. Two new farms participated in GA in 2019, for a total of six participating farms in GA over both years. In each year a single, but different, farm was removed from the study due to circumstances unrelated to the study. All GA farms were certified organic farms, growing mixed commodities. During the 2018 growing season, limited sampling occurred on three GA farms/fields, with samples (amendment and soil) collected pre-amendment, post-amendment, mid-season and at harvest. In 2019, to expand the study as noted earlier, fields associated with certified organic farms were selected based on amendment type (BSAAO or green compost). Green compost was a BSA of non-animal origin, e.g., plant-based. BSAAO samples included both raw and composted manure samples, which were not analyzed separately in this study due to the limited number of composted manure samples. Composite samples were collected monthly from each field, starting prior to amendment application, and continuing through harvest. GA fields were subdivided into four sections that were sampled at each site visit. Some of these sections had differing amendment types but the sections were defined by the commodity grown within each section. Each of these sections were considered separate fields in the analysis.
In OH, 18 farms, using a variety of farm management approaches, were similarly recruited with six farms for each amendment type, dairy manure (DM)-amended, poultry manure (PM)-amended, and non-BSAAO-amended. The farm management approaches in OH ranged from conventional to organic with most using a hybrid approach. None of the OH farms were certified organic. The study included fields in rotation (i.e., fields that were cultivating a crop with the intention of restoring soil health). As these fields had crops growing and were not fallow, these fields are referred to as rotating fields. The OH farms are characterized as small, ranging from 80 to 120 acres; each grew mixed commodities on a staggered schedule, and often had animals residing on the farm, including work animals, or on neighboring farms.
Metadata on farm management practices were collected via an interview with each farmer at the end of the growing season. Observations were recorded where available but complete metadata were not always available due to differences in reporting by farmers. These metadata include the use of organic management practices, use and type of fertilizers and pesticides, presence of domestic animals, evidence of intrusion from wild animals, and other events of note.
Daily meteorological data, provided by the Ohio Agricultural Research and Development Center weather system, were obtained from a local weather station in OH, located within an ∼24 km radius of all sampled fields. The data included total liquid precipitation (inches of accumulation, collected midnight to midnight, melted in case of ice or snow), average/minimum/maximum air temperature (oF, average determined with the 24 hourly readings for each day), global solar radiation measured at 6 m (Langleys units, 1 Ly = 1 cal/cm2, sum of 5 min readings in 24 h, average/minimum/maximum soil temperature (oF, top 2 and top 4 inches) (Supplementary Table 1). Wind speed and relative humidity were excluded from this study due to errors in data collection.
In GA, daily meteorological data were collected from the University of Georgia Climatology Research Laboratory and included maximum rain gauge (inches), i.e., total precipitation, average air temperature (oF), average solar radiation (watts per square meter), and average 10 min wind gust (mph), i.e., wind speed (WS) (Supplementary Table 2). Soil temperature was not collected in GA. Solar radiation was measured differently in GA and OH. To correct for this, when comparing GA and OH weather, solar radiation data were standardized by subtracting the observed value from the observed state mean value and then dividing by the observed state standard deviation.
A single composite biological soil amendment (BSA, green compost) or BSAAO sample, consisting of 3–4 sub-samples, was taken from the compost or manure at the time of application to the field. DM, which was applied only to fields in OH, was comprised of a combination of straw/corn fodder and dairy heifer manure that had accumulated for several months, in the pen housing the animals, before being transferred to a manure spreader. Three to four sub-samples were collected from one of the manure spreaders and combined into a single composite sample for testing. PM was purchased by the farmers from a local broiler house and delivered to the farm as a pile with no covering. Time between delivery and sample collection varied from farm to farm. In the OH region, monthly DM samples were collected from farms using DM amendments to evaluate the longitudinal pathogen prevalence in the herds beyond the manure that was applied to the field. These samples were formed by combining 8–10 fresh manure pats into a composite DM sample and were often found in the housing facilities or grass pasture areas. Samples were shipped at temperatures consistent with environmental conditions to minimize changes. The received samples were aliquoted (25 g) aseptically into sterile, filter Whirl-Pak® bags and then processed for pathogen detection. The samples were tested for the presence of five pathogens, Lm, Arcobacter, Salmonella, STEC, and Campylobacter. STEC analyses were added in the 2018–2019 growing season as part of the expansion mentioned in Section “2.1 Farm characteristics.”
In OH and GA, water samples were collected in 2019 from the water source (pond, well, or creek/stream) and from the end of the drip tape. Stream water samples were collected within 3 to 4 ft from the edge of the stream and near the irrigation system’s pump. Pond water samples were collected 3 to 10 ft from the shore and near the irrigation pump. Well source water samples were either sampled at the well head, directly from the pump, or after the water had travelled through an irrigation pipe from the well to the field. While all GA fields used well water for irrigation, water samples were also collected from surface water adjacent to the GA farms.
Ten liters of source water were filtered using Modified Moore Swabs (MMS) for pathogen testing including Lm, Arcobacter, Campylobacter, Salmonella and STEC, as well as for 16S rRNA gene sequencing analysis. Additionally, two liters of water samples, collected from the end of the dripline, were filtered through MMS. The MMS were maintained at refrigeration prior to pathogen testing. All MMS were then bisected using a disposable sterile scalpel to ensure open exchange during processing. The bisected MMS were transferred to sterile, filter Whirl-Pak® bags and 250 mL of sterile distilled water was added to each. The MMS were manually massaged for 15–30 s to evenly distribute the swabs within the Whirl-Pak® bags and then stomached at 300 rpm for 2 min in 30 s increments, with manual massaging between intervals to redistribute the MMS. Aliquots of 25 mL were collected from each sample, with manual agitation between samples to redistribute any sediment that had settled and processed according to the appropriate pathogen protocol.
In the 2017–2018 season, four composite samples were collected from each field representing pre-amendment, post amendment, mid-season, and harvest time. Preliminary analysis indicated more frequent sampling was needed. For this reason, in the 2018–2019 growing season, one composite sample was collected monthly per field in OH and GA. Each composite sample was comprised of three random sub-samplings from different locations in the field, all collected from between the rows of growing crop, which were combined as a composite sample weighing ∼2 kg. Each sub-sample entailed collection of soil from an area of 6” x 6” x 1” (width x length x depth) by spade, treated with 70% ethanol between sampling, with visible debris removed.
The soil samples were shipped and processed for pathogen detection and 16S rRNA gene sequencing analysis in the same way described above for the BSA and manure samples. A portion (∼0.5 kg) of soil samples, collected pre-amendment, post-amendment, mid-season, and at harvest, was shipped to The Maine Agricultural and Forest Experiment Station Analytical Laboratory and Maine Soil Testing Service1 for analysis by their comprehensive soil test using the Mehlich 3 extraction method (Rutter et al., 2022). This comprehensive soil test assessed pH, total organic matter, available nitrogen (nitrate plus ammonium), phosphorus, potassium, calcium, magnesium, sulfur, boron, copper, iron, manganese, sodium, and zinc. Separately, 25 g samples were processed for pathogen detection and an additional 250 mg was used for DNA extraction for 16S rRNA sequencing gene analysis.
Cultural enrichment and detection of Lm largely followed ISO 11290, with one modification (Gnanou Besse et al., 2019). Ferric citrate, which serves as a screening tool, was omitted from the medium as early testing found limited association with Lm presence (data not shown) (Donnelly, 2002; Leclercq, 2004; Parsons et al., 2019). Antibiotics were obtained from MilliporeSigma (Sigma-Aldrich®, St. Louis, MO). Samples were processed according to ISO 11290 with manual massage, due to the presence of rocks. Detection was performed by plating 10 μL on Rapid L’mono (RLM) agar (Bio-Rad, Hercules, CA), according to manufacturer’s procedures, and then subcultured on RLM to mitigate media changes due to background flora. Presumptive Lm colonies were then cultured on Brain Heart Infusion (BHI) agar (Becton, Dickinson and Company, Sparks, MD) and confirmed via qPCR analysis (Burall et al., 2021).
Soil and manure samples were pre-enriched in 75 mL Bolton broth (ThermoFisher Scientific Oxoid limited, Hampshire, UK) containing amphotericin B (5 mg/L) in filter Whirl-Pak® bags at 37°C for 3–4 h. This was followed by enrichment of 3 mL pre-enriched sample in 7 mL Bolton broth containing 5 mg/L amphotericin B, 20 mg/L vancomycin, 5 mg/L cefsulodin, and 10 mg/L trimethoprim for 48 h under microaerobic (MA) conditions (5% O2, 7.6% CO2, 7.6% H2, 79.2% N2). Each MMS rinsate was enriched with 25 mL 2X Bolton broth containing antibiotics (as above), under MA conditions at 37°C for 48 h.
After enrichment, samples were screened microscopically for the presence of spiral to rod or curved shaped bacteria and by PCR using Campylobacter (16S rRNA)- and Arcobacter (23S rRNA)-specific primers (Linton et al., 1996; Kim et al., 2019). Dilutions of the presumptive positive samples were inoculated (100 μL) onto 0.65 μm mixed cellulose ester filters (Hiett, 2017) placed on Brucella Blood Agar (ThermoFisher Scientific Remel, Lenexa, KS) supplemented with lysed Horse blood (Lampire biological laboratories, Pipersville, PA) for 15 min and/or streaked (20 μL) onto modified charcoal-cefoperazone-deoxycholate (mCCDA) plates. Isolated colonies were screened microscopically and confirmed by PCR.
Pre-enrichment of samples was conducted by resuspending each sample in a Whirl-Pak® bag with 225 mL modified buffered peptone water. Each bag was slowly swirled for 1 h in a shaking incubator at 30°C before continuing incubation at 35°C for 24 h (± 2 h). Enrichment for Salmonella was performed as described in the FDA Bacteriological Analytical Manual (BAM) (Andrews et al., 2022) with Rappaport-Vassiliadis (RV) medium and Tetrathionate (TT) broth, followed by isolation of black single colonies on XLT-4 agar and confirmation using the VITEK® 2 system (BioMérieux, Inc, Marcy-l’Étoile, France).
Samples were pre-enriched by resuspending each sample in a filter bag with 225 mL modified buffered peptone water with pyruvate (mBPWp) and incubated at 37°C for 5 h (± 1 h). Acriflavin–Cefsulodin-Vancomycin supplement (3 mL) (Feng et al., 2020) was added and the samples were incubated at 42°C for 18 h (± 2 h). Then, two 1 mL replicate aliquots of the enrichments were collected from each sample. One was for screening for STEC by qPCR. The aliquot was centrifuged at 2,500 x g for 3 min for DNA extraction.
The other aliquot was for isolation. When tested positive by PCR, three 10 μL replicates from each culture were streaked onto ChromAgar STEC plates (CHROMagar™, Paris, France) and incubated at 37°C for 18 h. Pink colonies were selected for STEC verification. The colonies were grown in mBPWp in a 96-well plate at 37°C for 5–8 h. Half of the culture was used for DNA purification and the other half was saved as a seed culture for later use. DNA was purified and qPCR analysis was performed to detect STEC as described elsewhere (Li et al., 2017). Wells positive for STEC were tracked to the seed plate, which was used for secondary culture on CHROMagar™ STEC plates for further isolation and purification.
Water samples were centrifuged at 7,200 rpm for 30 min. Cell pellets were then resuspended in DNA/RNA Shield solution (Zymo Research, Irvine, CA) and stored before batch processing for DNA extraction. DNA was extracted from environmental samples with the ZymoBIOMICS DNA Miniprep kit (Zymo Research, Irvine, CA) utilizing the lysis bead bashing tubes and validated lysis protocols on either the FastPrep-24 5G homogenizer (MP Biomedicals, Santa Ana, CA) or a Vortex Genie with Horizontal-(24) Microtube Adaptor. 16S rRNA gene amplicon library preparation and sequencing was performed on the MiSeq benchtop sequencer (Illumina, San Diego, CA) targeting the V4 variable region of the 16S rRNA gene, following the manufacturer’s recommended protocol with the modification of using Omni Klentaq polymerase (DNA Polymerase Technology, St. Louis, MO) in place of KAPA HiFi HotStart Ready Mix, as described elsewhere (Daquigan et al., 2016). The amplification primers used were 515F-Y and 926R (Parada et al., 2016). Nextera XT dual indices were used to allow multiplex sequencing using 600 cycle V3 paired-end chemistry. Each sequencing run contained 60 to 80 multiplexed libraries and estimated equimolar library pools were sequenced at 8 pM. with a 15% phiX spike-in.
Taxonomic classification of 16S V4 amplicon microbiome datasets were processed using an in-house bioinformatics pipeline written in R, Bash, and Python. Preprocessing and classification of reads were performed as follows. First, paired-end reads were merged using FLASH (Magoc and Salzberg, 2011). In cases where reads could not be merged, either due to insufficient overlapping base pairs or poor quality within the overlapping region, read 1 was retained in the dataset. This approach allowed retention of more sequencing data. Next, the paired-merged and read 1 dataset was then processed using USEARCH (Edgar, 2010; Edgar and Flyvbjerg, 2015) to perform quality filtering and prepare reads for taxonomic classification. This included using the fastx_truncate option to remove the 16S rRNA PCR primers, fastq_filter to filter based on a minimum fragment length of 150 bp and ee (expected error) value of 1.0 quality, fastx_uniques to get unique sequences per sample, and unoise3 to check for and remove chimeric sequences. The resulting datasets were then classified with MAPseq (Matias Rodrigues et al., 2017), utilizing their default curated database. Briefly, this was created using NCBI GenBank and RefSeq reference sequence databases, extracting any sequences annotated as ribosomal RNA with 16S or 18S in the annotation. The data presented in the study are deposited in the NCBI repository, accession number PRJNA894200.
Associations between Lm, Arcobacter, Campylobacter, Salmonella, and STEC prevalence and type of soil amendment (DM, PM, and green compost) or water source (surface, including streams and ponds, or well) were assessed using Fisher’s exact test, performed in SAS 9.4 (SAS Institute, Cary, NC). Associations between pathogen prevalence and season were also assessed using Fisher’s exact test. Small sample sizes prohibited multivariate modeling analyses.
A structural equation model (SEM) was postulated specifying direct and/or indirect influence of local meteorological data, soil fertility data, farm management practices (soil amendment and source of irrigation water) and taxonomic diversity on the presence of the most prevalent pathogens, Lm and Arcobacter (Supplementary Tables 1, 2, and 5). The conceptual model, evaluating OH soil data, is outlined in Figure 1, specifying indicators and direction of causality. There were insufficient data to inform the model for Salmonella, Campylobacter, and STEC. Soil samples that were positive for Lm or Arcobacter after culture enrichment were considered “positive.” The farm management variables, soil amendment and irrigation water source, were ordinalized with increasing scores corresponding to greater observed Lm or Arcobacter prevalence. The water data were thus coded: 1 = non-irrigated, 2 = well water-irrigated, 3 = surface water-irrigated. Similarly, the manure data were coded as: 1 = no BSAAO, 2 = PM (including composted PM), and 3 = DM (including composted DM). To account for transition from soil amendment to no BSA amendment in a field from one year to another, i.e., if a field transitioned from PM or DM soil amendment in 2018 to no BSA amendment in 2019, the assigned amendment score was adjusted to 1.5 to account for possible lingering PM or DM effects. Similarly, if a field was irrigated with surface water in 2018 and then transitioned to no irrigation in 2019, that field was assigned an irrigation score of 1.5. This allowed the potential to capture carryover risk from one treatment to another during the SEM analysis.
Figure 1. Conceptual model for Lm and Arcobacter (Arco) prevalence in OH soil. Square boxes are observed variables, Farm management practices (soil amendment and irrigation source) and Simpson’s diversity index (SDI). Ovals are latent variables, soil fertility and local weather at time t. Arrows represent the flow of causality. Solid-line arrows indicate positive effects on the to variable, dash-line arrows indicate negative effects on the to variable. Double-headed curved arrows are covariances (i.e., no causality). Al, aluminum; B, boron; Ca, calcium; Cu, copper; Fe, iron; K, potassium; Mg, magnesium; Mn, manganese; Na, sodium; P, phosphorus; S, sulfur; Zn, zinc; LOI, loss-on-ignition; PM, poultry manure; DM, dairy manure; AT, air temperature; SR, solar radiation; PR, precipitation.
The soil elements tested in this study were known to influence plant and microbial growth and considered indicators of soil fertility Lines-Kelly, 1992 (Gardner, 1985). These elements, in the collective, are referred to as “soil fertility” in this manuscript, despite no direct assessment of fertility via crop growth or yield. Soil fertility was modeled as a latent variable. Soil fertility was defined as the underlying driver of the soil chemical profile values: soil pH, phosphorus, potassium, calcium, magnesium, aluminum, manganese, boron, copper, iron, sodium, ammonium nitrogen (NH4-N), nitrate nitrogen (NO3-N), sulfur, zinc, and loss-on-ignition, which estimates the organic matter content of the soil. Increasing values of nutrients and soil pH were considered as indicators of increased soil fertility (i.e., positive loadings). In a secondary analysis, both nitrogen analytes (NH4-N and NO3-N) were analyzed separately from soil fertility to examine their relationship with Nitrospira, Nitrosospira, Lm, and Arcobacter. The relative abundance of Nitrospira and Nitrosospira, the measured values of NH4-N and NO3-N, and the prevalence of both Lm and Arcobacter were plotted versus time to assess if prevalence tracked synchronically or asynchronically with the nitrogen analytes and nitrifying bacteria.
Weather was incorporated in the model as a latent variable observed through precipitation (PR), air temperature (AT), and solar radiation (SR), with increasing temperature and radiation values (i.e., positive loading) and decreasing precipitation (i.e., negative loading) representing increasing “weather” across time. Minima and maxima measures were not included to avoid collinearity issues with average daily measures and to reflect the center rather than the extremes of the data. Due to the high collinearity (corrPearson = 0.93) between soil and air temperatures, soil temperature was not included.
The literature indicated a delayed, or lagged, effect of weather on Lm prevalence, with a peak increase in Lm prevalence 24 h after a rain event but rain amounts over the preceding 2 days also increased Lm prevalence (Weller et al., 2015a,b; Harrand et al., 2020). Based on these data, a 48 h lag was used in this study to evaluate effects linked to precipitation. The term “lag” is used to indicate the timeframe prior to sample collection. The choice of lag (or no lag) or moving average span for the remaining weather parameters was informed by (1) published results and (2) visual examination of loess-smoothed plots.
There are two main components to an SEM. The structural component quantifies potential dependencies (pathways) between the variables in the model. The measurement component quantifies how well the latent variables are represented by the observed indicators (e.g., how well is soil fertility represented by the soil measurements Al, B, Ca, …, Zn). A SEM must have a sound theoretical basis and is only as successful as the researcher-hypothesized a priori model, based on researcher knowledge and/or published results.
The hypothesized OH conceptual model, guided by observed manure and water prevalence in Table 1 and prior research, is presented in Figure 1. As the SEM is a model approach driven by theory and prior research, it was postulated that the use of BSAAO, the use of surface water for irrigation, increasing soil fertility, decreasing AT and SR, increasing PR, and decreasing microbiome diversity would result in an increase in Lm prevalence. It was also hypothesized that the use of BSAAO, increasing soil fertility, increasing AT and SR, decreasing PR, and decreasing Simpson’s Diversity Index (SDI) would result in an increase in Arcobacter prevalence. SDI is a measure of alpha, or within-sample, diversity which takes into account the number of species present and the relative abundance of each species (Roswell et al., 2021). In both approaches, DM was hypothesized to have a larger effect than PM. Microbiome diversity, measured as SDI, was hypothesized to increase with increasing AT, SR, and soil fertility assuming nutrients in the soil can be utilized by bacteria and plants.
Diagonally weighted least squares was implemented using the sem function in the R lavaan package, version 0.6.9 (Rosseel, 2012) and the probit link function, Φ () where Φ is the cumulative standard normal distribution function. The model was fit by fixing the variances of the latent variables to unity. As observed weather and soil parameters, SDI, and prevalence data were on different scales, they were standardized to the same scale.
The fit of the SEM was assessed by the Bentler Comparative Fit Index (CFI) and the root mean square error of approximation (RMSEA). Covariances between the soil or weather manifest variables, as suggested by large modification indices (MI), MI > 10 (i.e., χ2df=1p<0.002), and fitting the model paradigm, were included in the model. The model was tuned by removing regression parameters and pathways with Wald z-test P-value > 0.15.
A more parsimonious SEM was conducted to model Lm prevalence in GA soil samples due to insufficient soil fertility panel data. No analysis was performed for the other pathogens, due to limited soil positive samples. Furthermore, all GA fields were well-irrigated, and no GA fields were amended with DM, eliminating irrigation source as a variable and reducing the soil amendment variable to two levels, 1 = green compost, 2 = PM. The hypothesized conceptual model, used to evaluate GA soil data, is presented in Figure 2A with local weather incorporated, as in the OH soil model, with the addition of WS as an indicator. Decreasing AT and SR, increasing PR and WS, and the use of PM amendment were hypothesized to result in increased Lm prevalence, similar to the model evaluating OH data.
Figure 2. (A) Conceptual model for Lm prevalence in GA soil. (B) Final estimated model for Lm prevalence in GA soil. (C) Weather effect sizes. GC, green compost. (D) Lm prevalence in GA soil by soil amendment type. PM, poultry manure; AT, air temperature; SR, solar radiation; PR, precipitation; WS, wind speed; CFI, comparative fit index; RMSEA, root mean square error of approximation.
Metataxonomic analysis of the bacterial community was conducted on OH soil samples, targeting the V4 region of the 16S rRNA gene. Families occurring in less than five of the samples were excluded. SDI was calculated, at the family level, using the R diversity function in the vegan package Oksanen et al., 2019. SDI is a measure of taxonomic diversity with 1 representing maximum diversity and 0 representing no diversity. SDI was included in the Lm and Arcobacter prevalence models as the measure of microbiome diversity.
Analyses of co-occurrence with Lm or Arcobacter were conducted to assess which soil genera correlated with the presence or absence of Lm or Arcobacter. Lm culture positive samples that were undetected in the 16S analysis were included by setting a value at half the minimum percent hit versus those positive by 16S analysis. The relative abundances of genera in the Lm+ (or Arcobacter+) OH soil samples were compared to those in Lm- (or Arcobacter-) OH soil samples. Non-parametric bootstrap 95% confidence intervals (CI) were calculated for the mean relative abundance of each genus for Lm- (or Arcobacter-) samples and Lm+ (or Arcobacter+) samples. CIs were calculated using the np.boot function in the R nptest package Helwig, 2021.
Amendment samples (n = 87) were tested for the prevalence of Salmonella, Arcobacter, Campylobacter, STEC, and Lm (Supplementary Table 3). When comparing pathogen prevalence in OH and GA, all five pathogens were lower in GA amendments (0–20% positive samples) than OH (3–51% positive samples) (P-values, not significant), though this may be due to the absence of DM in GA (Table 1). Lm, Salmonella, and Arcobacter were detected in DM and PM samples while Campylobacter was only detected in DM samples (Table 2). No pathogens were detected in the green (non-animal) compost samples. The presence of Campylobacter and Lm was significantly associated with manure type (PCampylobacter = 0.0002, PLm < 0.0001), being most prevalent in DM samples (Table 2). To assess seasonal trends in OH, pathogen prevalence was compared across season (winter, spring, summer and fall). Lm (P = 0.05) and Campylobacter (P = 0.001) were most prevalent in the summer and Arcobacter (P < 0.0001) was most prevalent in the fall, followed by the summer (Figure 3). There were comparatively fewer samples analyzed for STEC, as STEC was added in the second year, and a low prevalence was observed, reducing confidence in the observed results; however, we note that STEC was only detected in DM samples and appeared most prevalent in the fall.
Figure 3. Pathogen prevalence in amendment and water samples by season. Amendment includes the actual BSA applied to fields as well as dairy manure samples that were not associated with field application. Data is plotted to show the percent of the samples tested that were positive for the indicated pathogen evaluated within each season.
Water samples (n = 95) were tested for pathogen prevalence (Table 1, Supplementary Table 4). Thirty-nine samples (nOH = 31; nGA = 8) were collected from the end of the dripline, and 56 samples (nGA = 18, nOH = 38) were collected from the water source. Lm and Arcobacter were by far the most frequently detected pathogens. Comparisons of pathogen prevalence of GA and OH water samples, using Fisher’s exact test, found that Arcobacter was more prevalent in water from OH than GA (P = 0.05). However, no significant regional differences in prevalence for Lm, Salmonella, Campylobacter, and STEC were observed for water samples (Table 1).
Arcobacter was detected in both surface and well source water, with a higher prevalence in surface water (POverall < 0.0001, POH = 0.05), whereas Lm, Salmonella, Campylobacter, and STEC were detected only in surface water (pond or stream) (Table 2). Campylobacter and STEC were only detected in source samples whereas Lm, Salmonella and Arcobacter were detected in both source and dripline samples (Table 2).
To assess seasonal trends, pathogen prevalence in OH surface water was compared across seasons (spring, fall, or summer). Lm was significantly more prevalent in the summer OH water samples (P = 0.02; Figure 3). Some of the subgroups within Figure 3 had sample sizes too small for any conclusions to be drawn and are considered observational. No significant seasonal trend was observed for the other pathogens, either due to the absence of a significant difference or the dataset’s size preventing statistical analysis.
OH soil samples were tested for Salmonella (n = 310), Campylobacter (n = 260), STEC (n = 235), Lm (n = 311), and Arcobacter (n = 260; Table 1). These samples were collected from 21 farms/fields in 2018 and 29 farms/fields in 2019, for a total of 29 different farms/fields across both years with 20 of them sampled in both years. All OH soil samples were negative for Salmonella and Campylobacter, and only one was positive for STEC. Given the low yield for those three pathogens, prevalence analyses focused on Lm and Arcobacter. There were 154 (for Lm) and 144 (for Arcobacter) complete cases, i.e., samples which had complete data on soil fertility, weather, farm management data, and metagenomic to model the pathogen prevalence. Rotating fields (nOH = 13) were included in the analysis as non-amended fields (SA = 1) or previously amended fields (SA = 1.5) unless amendment was applied to the rotating field (n = 3, DM-amended). Fifteen percent of OH soil samples tested positive for Lm and 8% tested positive for Arcobacter (Table 1). However, when limited to the 154 (Lm) OH soil samples with complete data, 26 (20%) samples tested positive for Lm. Likewise, limiting the analysis to the 144 (Arcobacter) OH soil samples with complete data, 12 (8%) samples tested positive for Arcobacter.
Among the GA soil samples, 106 were tested for Lm and Salmonella, 89 for STEC, 84 for Campylobacter, and 67 for Arcobacter (Table 1). While no GA soil samples tested positive for Salmonella, Campylobacter, or STEC, one (2%) tested positive for Arcobacter and ten (9%) tested positive for Lm. Of the 10 Lm+ GA soil samples, seven were from PM-amended fields (14% of PM-amended fields) and 3 were from green compost-amended fields (6% of green compost-amended fields), indicating increased Lm prevalence in GA PM-amended fields versus green compost-amended fields (Table 2). There were 99 complete GA soil cases to model Lm prevalence.
Lm and Arcobacter prevalence of GA and OH soil samples were compared using Fisher’s exact test. Arcobacter was more prevalent in OH soil than GA soil (P = 0.06), while no differences were observed in Lm soil prevalence between the two regions.
Trends of OH soil Lm and Arcobacter prevalence and GA Lm prevalence versus weather were explored, with weather parameters observed at various hours (24, 48, 72, 96, and 120 h) prior to the day of soil collection or averaged across various moving averages (spanning 48, 72, 96, and 120 h) (data not shown). PR averaged over the 48 h prior to and including the day of soil collection correlated most closely with Lm prevalence in GA and OH soil and inversely correlated with Arcobacter prevalence in OH soil. AT and SR, also averaged over 48 h, inversely correlated with Lm prevalence in OH and GA soil and directly correlated with Arcobacter prevalence in OH soil. These findings largely agreed with prior literature results for Lm (Strawn et al., 2013a,b; Weller et al., 2015a; Harrand et al., 2020). WS averaged over the 48 h prior to and including the day of soil collection was observed to correlate most closely with Lm prevalence in GA soil.
Most soil characteristics analyzed in OH soil samples were good indicators of soil fertility, as defined in the methods, i.e., had large effect sizes (large, standardized regression coefficients), with the exceptions of Ca, Na, and S. Soil characteristics with larger size effects were considered as good indicators of soil fertility. All indicators had positive loadings, i.e., increasing values indicated increasing “fertility,” except for NO3-N and S, which had negative loadings, i.e., increasing NO3-N and S indicated decreasing fertility (Figure 4).
Figure 4. Effect sizes (z-statistic) of the observed soil chemistry variables composing the predicted (standardized) soil fertility latent variable for the Lm (A) and Arcobacter (B) models. Effect sizes (z-statistic) of the observed weather variables composing the predicted (standardized) Weather latent variable for the Lm (C) and Arcobacter (D) models. Blue dots are positive effect sizes and orange dots are negative effect sizes. The larger the dot, the larger the effect size, i.e., the more important the indicator. Al, aluminum; B, boron; Ca, calcium; Cu, copper; Fe, iron; K, potassium; Mg, magnesium; Mn, manganese; Na, sodium; P, phosphorus; S, sulfur; Zn, zinc; LOI, loss-on-ignition; AT, 24 h average of air temperature; PR, precipitation; SR, solar radiation.
The strongest indicators of local weather’s ability to predict Lm and Arcobacter prevalence in OH soil were AT and SR (Figures 4C, D), which is expected given seasonal patterns associated with changes in temperature due to increased solar radiation. AT and WS were significant indicators in predicting Lm prevalence in GA soil but SR and soil amendments were not (Figure 2). Lm prevalence in GA soil was directly impacted by weather (PGA = 0.04; Figure 2B) and, in OH, the impact of weather on soil Lm prevalence was mediated by soil fertility, with decreasing fertility correlating with decreasing Lm prevalence (P = 0.05; Figure 5). Observed Lm prevalence was highest during the coolest part of the year, winter and spring (Figure 6A and Supplementary Figure 1). Arcobacter prevalence in OH soil was directly impacted by weather (P = 0.04; Figure 5) but in an opposite direction, with observed Arcobacter prevalence highest during the warmest part of the year (summer and fall) (Figure 6A). The observed effect of PR was not nearly as prominent as AT or SR in OH or GA (effect sizeLm, = 0 in GA, effect sizeLm, = −1 and effect sizeArcobacter = −2 in OH).
Figure 5. Final (parsimonious) model for OH soil Lm and Arcobacter (Arco) prevalence. Numbers on single-headed arrows are the standardized regression path estimates (z-statistic) and p-values (in parentheses). The thickness of the arrow reflects the strength of the relationship, with solid-line arrows indicating positive relationships and dash-line arrows indicating negative relationships. For plot simplicity, the soil nutrient covariances are provided in Supplementary Figure 2. Al, aluminum; B, boron; Ca, calcium; Cu, copper; Fe, iron; K, potassium; Mg, magnesium; Mn, manganese; Na, sodium; P, phosphorus; S, sulfur; Zn, zinc; LOI, loss-on-ignition; PM, poultry manure; DM, dairy manure; AT, air temperature; SR, solar radiation; PR, precipitation; SDI, Simpson’s diversity index.
Figure 6. Seasonal trends in OH air temperature (AT), precipitation (PR), and solar radiation (SR). Overlaid are Lm soil prevalence, Arcobacter (Arco) prevalence, and Simpson’s diversity index (SDI) in OH soil. AT, PR, and SR are 48 h moving averages. All variables are standardized to the same scale using the R standardize function in the robustHD package (Alfons, 2019). Soil amendment groups are aggregated in (A) and grouped in (B). BSA, biological soil amendment; BSAAO, biological soil amendments of animal origin; PM, poultry manure; DM, dairy manure; N, not amended with BSA or BSAAO; AT, 24 h average of air temperature; SR, solar radiation; PR, precipitation; SDI, Simpson’s diversity index.
OH soil fertility itself was significantly impacted by both soil amendment (PLm = 0.02, PArcobacter = 0.01) and local weather (PLm < 0.0001, PArcobacter = 0.003; Figure 5). Soil fertility was highest when a DM-BSAAO was used, followed by PM-BSAAO. Increasing AT and SR and, to a lesser extent, decreasing PR were correlated with decreasing soil fertility in the summer. Soil fertility was highest in winter when AT was low and PR was largely in the form of snow and ice. Altogether, these factors contributed to improved soil fertility in the winter. AT and SR had a more pronounced effect on soil fertility than PR (Figure 4C and D). Soil fertility had a correlative effect on Lm but not Arcobacter prevalence, but had a direct effect on diversity (PLm = 0.005; Figure 5) in OH, where diversity decreased as soil fertility increased. Decreasing diversity, in turn, was correlated with higher Lm prevalence (P = 0.02), indicating an indirect effect of soil fertility on Lm prevalence. These results were in line with the expected results, suggesting the observations that informed the model overall were likely consistent with environmental factors.
While the role of plant nitrogen utilization could not be properly evaluated in this study, some intriguing patterns were observed between the levels of ammonium and nitrate, local weather, and soil amendment in OH with Lm showing an increased prevalence late in the year in PM-amended fields compared to DM- and non-BSAAO-amended fields (Figure 7). Evaluation of the presence of Nitrospira and Nitrosospira, along with Lm prevalence, nitrate levels, and ammonium levels, showed a potential relationship between these factors, possibly initiated by the large increase in ammonium levels observed only in PM-amended fields (Figure 7). Two genera associated with nitrification are Nitrospira and Nitrosospira (Daims, 2014). Their role in nitrogen processing may be affected by the nitrogen source provided based on the use of DM or PM, the latter with a higher content of ammonium Herbert et al.,2 and could explain the observed inverse relationship between the levels of ammonium (high to low) and nitrate (low to high) during the growing season in OH (Figure 7). PM, rich in urea and uric acids, was shown to boost ammonium levels, as expected, when (Figure 7). In these fields, an increase in Lm prevalence was observed late in the year, but not in non-BSAAO-amended fields, and was lower in DM-amended fields (Figures 6B, 7). Additionally, there are marked differences between these field groups when evaluating the relative abundance of Nitrospira and Nitrosospira.
Figure 7. Seasonal Trends in Lm and Arcobacter (Arco) prevalence, Nitrosospira relative abundance, Nitrospira relative abundance, ammonium-nitrogen and nitrate-nitrogen levels in Ohio soil, grouped by soil amendment. All variables are standardized to the same scale using the R standardize function in the robustHD package. BSA, biological soil amendment; BSAAO, biological soil amendments of animal origin; PM, poultry manure; DM, dairy manure; N, not amended with BSA or BSAAO.
To concentrate on regional differences in Lm soil prevalence, possibly due to different weather patterns, the GA and OH weather was compared. The AT and SR values for GA and OH (Figure 8A), and PR data for OH (Figure 8B) were cross-plotted. PR trends were similar cross year (2018, 2019) and were analogous cross both states (GA, OH). The SR trends were also similar cross year (2018, 2019) but the GA SR peaked around May, about two months before the OH SR peaked, around July. To ascertain when the prevalence of Lm in soil first significantly decreased, a structural change analysis was conducted. The mean monthly Lm prevalence in OH soil was calculated, over both years aggregated. Unlike OH, where soil was sampled throughout the year, the soil in GA was only sampled March through October, due to different amendment application times, resulting in insufficient longitudinal samples to conduct a structural change analysis in GA as mentioned earlier for other analyses.
Figure 8. (A) GA and OH monthly average air temperature (AT) and average solar radiation (SR) for study years 2018 and 2019. (B) GA and OH monthly average precipitation (PR) for study years 2018 and 2019. SR and PR were standardized to the same scale as they were measured differently in GA and OH.
The Quandt Likelihood Ratio (QLR) statistic (Quandt, 1960) was used to detect the presence of structural breaks in the OH data and was calculated using the Fstats function in the strucchange R package (Zeileis et al., 2002, 2003). To determine when the structural break(s) occurred, the breakpoints function in the same R package was used. The QLR tests detects structural changes of a regression of the Lm soil prevalence at month j regressed on the previous month’s (month j-1) prevalence, i.e., detects month-to-month prevalence changes. Only one structural break in OH was detected in March (PQLR = 0.05) and is evident in Figure 6A.
The final parsimonious Lm and Arcobacter model for OH revealed a causality effect associated with the type of soil amendment (PLm = 0.01, PArcobacter = 0.08) with prevalence highest for DM-amended fields (Figures 5, 9). Although the direct effect of soil amendment on Lm prevalence in GA was not significant, the observed Lm prevalence in PM-amended fields was marginally higher than for green compost-amended fields. A causal effect of irrigation source in OH (P = 0.06) on Lm prevalence was observed with prevalence highest for non-irrigated OH fields (Figures 5, 9A). Although, in OH, surface water samples presented the highest observed Lm prevalence and well water samples presented zero observed Lm prevalence (Table 2), soil samples from surface water-irrigated fields had similar Lm prevalence to well-irrigated fields, while the soil samples from non-irrigated fields had the highest Lm prevalence (Figure 9A). Since the decision regarding whether to irrigate may be influenced by known on-farm risk factors, such as resident animals, amendment practices were evaluated to determine if they might influence irrigation practices. When evaluating whether amendment choice might correlate with irrigation choice, it was found that: PM-amended fields were equally likely to be irrigated with surface or well water; DM-amended fields were more likely to be irrigated with well water; and non-amended fields were more likely to not be irrigated.
Figure 9. Distribution of Lm and Arcobacter (Arco) prevalence in OH soil by irrigation source (A,C) and soil amendment (B,D). BSA, biological soil amendment; BSAAO, biological soil amendments of animal origin; None, no irrigation of the field; Surface, irrigation performed with surface water; Well, irrigation via well water; N, not amended with BSA or BSAAO; PM, poultry manure; DM, dairy manure.
Metataxonomic sequence analysis was performed for 305 of the 311 OH soil samples. For purposes of informing the model, samples that were positive for Lm after enrichment (culture positive) were considered positive for Lm, even if the culture-independent sequencing results did not indicate the presence of Lm. In fact, an evaluation of the sensitivity of microbiome metataxonomics when detecting Lm in soil samples, only two OH soil samples were found to contain Lm by 16S rRNA sequencing analysis whereas 45 OH soil samples were culture-positive for Lm. Additionally, the two samples from which Lm levels were detected by metataxonomics, were culture-negative for Lm. These data highlight the need for culture enrichment of foodborne pathogens from environmental samples. Furthermore, the two samples which were Lm culture-negative but contained detectable levels of Lm in the metataxonomic analysis were included as Lm-containing samples in the analysis. The detection of Arcobacter using metataxonomics was also incongruous with culture results. Four OH soil samples had levels of Arcobacter detected by metataxonomics, three of which were culture-negative for Arcobacter and one of which was not cultured for Arcobacter. Of the 21 OH soil samples, which were culture-positive for Arcobacter, none had levels of Arcobacter detected by 16S rRNA gene amplicon sequencing. These 21 samples were included in the analysis as containing Arcobacter and the Arcobacter relative abundance (percent hit) was set to half the minimum relative abundance of the four Arcobacter containing metataxonomic samples, 0.002.
Diversity of the microbiome was calculated at the family level with the SDI ranging from 0.89 to 0.98. The type of soil amendment did not appear to influence bacterial diversity (Figure 6B). Although weather did not have a direct impact on bacterial diversity, diversity was visibly lower in the winter through spring, reaching its nadir in April/May (Figure 6A). This observation indicated that April/May represented an inflection point in bacterial diversity, perhaps due to increased temperatures that were disadvantageous for cold-adapted bacteria, while growth of more warm-adapted microorganisms had not yet occurred to levels detectable by metataxonomics. Diversity increased significantly with decreasing soil fertility in the Lm model (PLm = 0.005; Figure 5). As previously mentioned, fertility decreased with increasing AT and SR; as such, the effect of weather on diversity appeared mediated through soil fertility.
The top twenty most abundant genera in Lm and Arcobacter negative (–) samples were compared to the top twenty most abundant genera detected in Lm and Arcobacter positive (+) samples (Figure 10). In general, the top twenty genera are similarly abundant in positive and negative samples for Lm and Arcobacter (Figure 10) with two exceptions, mentioned below. As such, the profiles of dominant genera in soil appeared similar and their influence on pathogen prevalence did not appear substantial. The relative abundances of Flavobacterium and Gemmata, however, were significantly different (i.e., non-overlapping 95% CI, Table 3) between Lm+ and Lm-samples, with the former more abundant in Lm+ samples and the latter more abundant in Lm- samples. Regarding the genera with significantly different mean relative abundances between pathogen negative and positive samples in the Arcobacter co-occurrence analysis, the mean relative abundances (Figure 11A) were much lower (max∼0.5) than the mean relative abundances for Lm co-occurrence analysis (Figure 11B), max = 2.5); this signifies that for Arcobacter the genera differences observed between positive and negative samples were generated by genera that represented a smaller proportion of the soil microbiome than those genera associated with Lm presence or absence.
Figure 10. (A) Top (most abundant) twenty genera detected (16S) in Lm negative (–) samples vs. top 20 genera detected in Lm positive (+) samples in OH soil. (B) Top twenty genera detected in Arcobacter negative (–) samples vs. top 20 genera detected in Arcobacter positive (+) samples in OH soil. The darker the color, the more abundant the genus, i.e., the higher the relative abundance (%). An asterisk (*) denotes genera with significantly different mean relative abundance between positive and negative samples. RA, mean relative abundance.
Table 3. Top thirty genera whose mean relative abundance was most significantly different between (A) samples with no detectable Lm (Lm-) and samples with detectable Lm (Lm+) and (B) samples with no detectable Arcobacter (Arco-) and samples with detectable Arcobacter (Arco+).
Figure 11. Top thirty genera detected (16S) with significant differences between their mean relative abundance (%) when comparing Arcobacter negative (–) and positive (+) OH soil samples (A) and between Lm negative (–) and Lm positive (+) OH soil samples (B). Genera names were truncated at twenty characters. Bacteriodes RA in Lm+ samples was 0.000883. RA, mean relative abundance.
While green compost, PM, and DM were evaluated in this study, there was a higher prevalence of the evaluated pathogens in DM. Additionally, as green compost and PM were only collected around the time of application, analysis of seasonality trends was not possible. However, due to the collection of DM throughout the growing season, it was possible to determine the prevalence data for Arcobacter and Lm and evaluate potential patterns associated with season, leading to a possible explanation for the observed pattern. In the spring and summer, dairy herds associated with this study shifted to grazing in pastures around the farm. A similar shift likely occurred at other farms in the region. As a result, there was likely increased interaction of cattle with surface water that could lead to transmission of Lm to or from neighboring animals. This exposure could be by drinking contaminated surface water, which could occur independent of pasture access, as well as by the animals entering surface water that cross through the pasture, transmitting pathogens to and from pastures. This increased potential for exposure and transmission could explain the observed increase in Lm prevalence in OH DM in the spring that continued through the fall but declined in the winter (Figure 3) when herds were generally kept within barns, which could potentially reduce direct interaction with surface water. However, the possibility remains that dietary shifts associated with these seasonal shifts could be affecting Lm prevalence in DM as well. Although Lm DM prevalence was lowest in the winter, the regional herd, encompassing all cattle evaluated for Lm via manure testing, carried some level of Lm year-round. This presence of Lm in some cattle within the region would provide an opportunity for re-introduction of Lm to the agricultural region in the spring. Higher Lm prevalence in DM and surface water (Figure 3) samples during the summer was not observed in OH soil (Figure 6A). Soil Lm prevalence, instead, was higher in the spring than the summer. This reduced prevalence could be due to reduced runoff in the summer as precipitation was lowest in the summer and/or increased competition with other soil organisms in the warmer months. Similarly, Arcobacter prevalence in DM was much higher in warmer months than in the winter. Others have similarly reported higher frequency of Arcobacter in feces from cows in southern dairy herds than in those in the northern states, which may reflect higher transmission in warmer climates (Wesley et al., 2000).
Surface water had a higher pathogen prevalence when compared to well water. Although some ponds and streams evaluated were not used directly for agricultural irrigation, their relatively high Lm and Arcobacter prevalence should be considered since they could still be sources of pathogen introduction. Pathogens could be introduced into the field via animal movement and/or precipitation events could lead to flooding of surface water, introducing contaminated water into the field. This study did not examine surface water during the winter since it was not used for irrigation. However, an assessment of Lm prevalence in winter water samples could provide better information about how Lm survives and circulates in an agricultural region and how those factors contribute to the potential for Lm contamination of fresh produce. This is of particular interest after the observations of Lm prevalence in DM, indicating the potential for circulation between the regional herd and surface water. Lm prevalence in OH surface water was observed to peak in the summer when the domestic animals were more likely to be outside with possible direct interaction with surface water. This prevalence decreased to undetectable levels in the fall (Figure 3). Unlike Lm prevalence, Arcobacter presence in surface water was not significantly different in spring, summer and fall seasons. Similarly, others have shown that the prevalence of Arcobacter and five associated virulence genes in water samples from various sources were not impacted by weather in the Kathmandu Valley in Nepal (Ghaju Shrestha et al., 2019). These results, revealing differences in how each pathogen survives and persists in the agricultural environment, highlighted the need to consider pathogen-specific risk assessment models and mitigation strategies in agricultural practices.
The SEM approach used to evaluate factors associated with pathogen prevalence in soil is in line with the exploratory and confirmatory attitude of this study. Lam and Maguire provided a good description of SEM and its uses (Lam and Maguire, 2012). Lm and Arcobacter were both directly impacted by soil amendment, with pathogen prevalence being highest for DM amendment, second highest for PM amendment and lowest for no amendment (Figures 5, 9B, D). Lm presence in non-amended fields was marginally higher than Arcobacter presence (Table 2), suggesting Arcobacter prevalence in soil was primarily driven by BSAAO usage. BSAAO usage may serve as either the vehicle for Arcobacter introduction or provide a more favorable environment for pathogen survival. Other factors (e.g., irrigation source) appeared to have critical influence on Lm prevalence, though BSAAO usage did contribute to Lm prevalence. BSAAO serving as a primary driver for Arcobacter prevalence may explain the differences in prevalence observed between GA and OH farms in this study, with the former having reduced BSAAO usage and no use of DM and a concurrent reduction in Arcobacter prevalence. It is intriguing that this difference was also seen in surface water, suggesting added factors may be reducing Arcobacter prevalence in GA though it could simply be the absence of introduction from reduced BSAAO applications, potentially limited to the lack of DM.
Lm prevalence in soil was highest during the winter and spring when AT was lowest and precipitation amounts were marginally higher (Figures 6A, B). The impact of increasing AT (|effect size| ∼6) on decreasing Lm prevalence appeared more salient than the impact of increasing PR (|effect size| ∼1) on increasing Lm prevalence (Figure 4C). Although this study found, as others did (Strawn et al., 2013a,b; Weller et al., 2015a; Falardeau et al., 2018; Harrand et al., 2020), that Lm positivity increased with increased rainfall or soil moisture, AT appeared to suppress Lm more (i.e., higher effect size) than the moisture supported Lm. This observation is intriguing however, as GA weather had higher AT and similar PR compared to OH, but GA Lm prevalence was only slightly lower than that of OH in both soil and water samples. This suggests the possibility that AT alone may not be suppressing Lm or that temperatures during winter may provide a basal level of support for Lm prevalence throughout the year. However, it may also be that the microbiome population dynamics or other factors, such as soil texture, may vary between the different regions resulting in equivalent Lm prevalence despite the differences in temperature. SDI was highest during the summer and fall (Figure 6A), the same seasons where Lm prevalence is at its lowest, and the model (Figure 5) showed a direct role for diversity in the reduction of Lm, suggesting that competition played a role in suppression of Lm. It should be considered that AT, therefore, could impact Lm directly and indirectly.
Weather had a stronger direct effect on Arcobacter with prevalence increasing as AT increased and PR decreased (Figure 6A). A predictive model to measure the growth rate of A. butzleri showed that growth was directly proportional to increasing temperature, achieving maximum growth at a storage temperature of 40°C (Park and Ha, 2015). A more recent study has shown optimal growth of the three major human pathogenic Arcobacter species (A. butzleri, A. cryaerophilus, and A. skirrowii) at 35°C, followed by growth on agar plates at 30°C under microaerophilic conditions (Nguyen et al., 2021). Given average temperatures never exceeded 95°F (35°C), these prior data support the findings of this study, which indicated warmer temperatures supported Arcobacter prevalence, potentially by improved growth.
Soil fertility was directly impacted by both weather and soil amendment, as expected. Soil fertility decreased with rising AT and decreasing PR and was highest in DM-amended fields, with a slight decrease in PM-amended fields and a substantial decrease in non-amended fields. While some elements (anions) in the fertility panel may naturally leach out of soil through water percolating through soil column or surface erosion (water/wind whose speed increased through the growing season), all the elements in the panel are affected by weather through plant/crop uptake. Greater AT and SR enhance crop growth and thereby nutrient consumption, resulting in decreased soil fertility as the growing season progresses. While soil fertility was only correlated with Lm prevalence, the improvement of soil fertility associated with soil amendment usage could have indirectly affected Lm and Arcobacter prevalence via improved nutrient content. Although there are no studies to test the effect of soil fertility on the prevalence of Arcobacter, it is important to recognize its global presence in water bodies and various animals, including food and farm animals, domestic birds, wildlife, and zoo animals (Hsu and Lee, 2015).
The preliminary assessment, evaluating NO3-N and NH4-N separate from soil fertility, indicated the possibility of a relationship between ammonium, nitrate, Nitrospira, Nitrosospira, and Lm prevalence, though this observation requires further analysis since AT or other variables could play a role as well. However, within this study, given that manure was applied at a set time in the year and AT varies throughout the year and correlates with the season, it was not possible to disconnect these variables to evaluate their impact on nitrifying bacteria and Lm prevalence independently. The observed increase in Lm prevalence in PM-amended fields late in the year suggested the possibility that the ammonium increase associated with PM might impact nitrifying microorganisms, with one nitrifying genus, Nitrosospira, found to have a significant difference in relative abundance between Lm+ and Lm- fields (Figure 11A).
There are hypotheses that a healthy, robust soil, i.e., high soil fertility, may limit pathogen prevalence (Devarajan et al., 2021; Jayaraman et al., 2021). In fact, one recent study found that the use of cover crops and compost boosted soil diversity, improving suppression of Salmonella and Lm (Devarajan et al., 2021). However, in our study, while we found soils with higher SDI had reduced Lm prevalence, soils with higher soil fertility, which was improved with the use of BSAAO, which included both raw and composted manure, had a decreased SDI (Figure 5 and 6A). Additionally, the use of BSAAO was associated with an increased prevalence of Arcobacter and Lm, suggesting added complexity within these relationships. In particular, the Lm model showed that increased soil fertility resulted in decreased SDI, which could indirectly increase Lm prevalence as decreased SDI was correlated with higher Lm prevalence, though the same was not true for Arcobacter (Figure 5). Therefore, careful consideration of the attributes of a pathogen is important when testing how farm management practices could impact its prevalence.
This finding that there could be differing impacts on pathogens was further highlighted when evaluating the impact of irrigation on pathogen prevalence. Irrigation was found to have no impact on Arcobacter prevalence (Figures 5, 9C). However, irrigation source had a direct impact on Lm prevalence, though the result was counter to the expected results. Lm prevalence was highest for non-irrigated fields when compared to both well and surface water-irrigated fields (Figures 5, 9A). One explanation could be that farm management practices could have been impacted by observable risks, such as known proximity to animals, when deciding whether to use surface water for irrigation. Evaluation of farm practices found that non-BSA amended fields were more likely to not be irrigated, suggesting that other factors, such as economics or neighboring animals, may have influenced these decisions. However, metadata to evaluate what factors farmers used to inform their irrigation choice were not available. It is important to note, though, that the soil evaluated was not the soil directly irrigated but adjacent so it is possible that a different observation would have been made closer to the dripline. These possibilities suggest that more studies are needed to understand what could be creating the observed result, given that Lm prevalence was highest in surface water samples.
SEMs are uniquely suited for (1) identifying relationships (pathways) between latent variables (weather and soil fertility), (2) modeling complex (intermediary) relationships between factors and (3) explicitly identifying covariances between indicators and factors (Nachtigall et al., 2003). SEMs directly estimate both the effect sizes and direction of the effects, simultaneously for all factors, a notable strength of this analysis.
Some factors that indicated possible associations with soil pathogen prevalence but were not included in the soil analyses due to insufficient or incomplete data include comparing organic and non-organic farming, equipment hygiene, insecticide/fungicide application, presence of animal activity, water additives, and soil moisture measurements. The impact of these candidate factors would need a much larger study to be evaluated. Broader and more complete metadata collection was desirable for the study but attaining such on a voluntary basis was challenging, especially with potential for differences in memory to recall specific practices and/or events.
Year to year variability also cannot be eliminated as a factor in this study given 2018 and 2019 were not evaluated to determine if weather related factors were representative of overall weather trends.
Pathogen prevalence in soil, for both Lm and Arcobacter, was found to be associated with the use of BSAAO but not the use of surface water as an irrigation source. In fact, the Lm model indicated that not irrigating increased Lm prevalence, suggesting that other factors that might be linked to the decision to not irrigate, such as animals residing at neighboring farms, wildlife presence, equipment sanitation activities, land topography, or unidentified/unknown variables may be contributing to increased Lm prevalence in non-irrigated fields. As noted earlier, BSAAO usage was not correlated with the practice of not irrigating fields. This indirectly suggests that the presence of cattle on farms, which served as the source of DM, did not impact irrigation choices. AT appeared to play a more significant role on pathogen prevalence in soil rather than the use of BSAAO, although the effect of AT was in opposing directions for Arcobacter and Lm. Additionally, data showed key differences in how environmental conditions impacted pathogen prevalence, indicating that a one size fits all approach to risk mitigation would not control certain pathogens. While the sample size was small and metadata were limited, this real-world comparison of multiple farms in two regions based on BSAAO and irrigation provided critical data to inform and validate controlled studies. The results of this study have identified other factors, i.e., not irrigating could increase pathogen prevalence, that may need further study and the need to better understand how pathogens are established and circulated within the agricultural environment.
The data presented in the study are deposited in the NCBI repository, accession number PRJNA894200.
EL, GR, MK, CG, KJ, LB, CH, KB, LH, UB, BL, and AZ contributed to the conception and design of the study. CH and MF organized the database. JP, MF, CG, and KJ worked on bioinformatics and sequence analysis. MF performed the statistical analysis. CH, LB, CG, UB, LH, BL, AH, KB, JF, MK, KJ, and RR were involved in sample processing. MF, CH, CG, UB, LB, and BL wrote the manuscript. KB was responsible for the revision and proofreading of this work. All authors contributed to the article and approved the submitted version.
This work was funded by the U.S. Food and Drug Administration, Center for Food Safety and Applied Nutrition.
We thank Hugh Rand, at the U.S. Food and Drug Administration, for his helpful insight and input to this study and manuscript; Marianne Sawyer, Surasri Sahu, Mira Rakic-Martinez, and Mohammad S. Alam, at the U.S. Food and Drug Administration, for their assistance on soil sample preparation for fertility testing, and DNA extraction; and Jennifer Schrock and Nick Anderson, at the Ohio State University, for sample collection.
The authors declare that the research was conducted in the absence of any commercial or financial relationships that could be construed as a potential conflict of interest.
All claims expressed in this article are solely those of the authors and do not necessarily represent those of their affiliated organizations, or those of the publisher, the editors and the reviewers. Any product that may be evaluated in this article, or claim that may be made by its manufacturer, is not guaranteed or endorsed by the publisher.
The Supplementary Material for this article can be found online at: https://www.frontiersin.org/articles/10.3389/fmicb.2023.1141043/full#supplementary-material
Supplementary Figure 1 | Seasonal trends in GA air temperature (AT), precipitation (PR), solar radiation (SR), and wind speed (WS). Overlaid is Lm soil prevalence GA soil. AT, PR, SR and WS are 48 h moving averages. All variables are standardized to the same scale using the R standardize function in the robustHD package. Soil amendment groups are aggregated in (A) and grouped in (B). GC, green compost; PM, poultry manure; AT, 24 h average of air temperature; PR, precipitation; SR, solar radiation; WS, wind speed.
Supplementary Figure 2 | Covariances between the soil nutrient covariances included in the OH Lm and Arcobacter (Arco) soil prevalence models. Ca, calcium; Mg, magnesium; Mn, manganese; P, phosphorus; S, sulfur; Zn, zinc; LOI, loss-on-ignition; NO3_N, nitrate nitrogen; NH4_N, ammonium nitrogen.
AT, 24 h average of air temperature; BSA, biological soil amendment; BSAAO, biological soil amendments of animal origin; CFI, comparative fit index; h, hour; DM, dairy manure; LOI, loss-on-ignition; PM, poultry manure; PR, precipitation; QLR, Quandt likelihood ratio; RMSEA, root mean square error of approximation; SDI, Simpson’s diversity index; SEM, structural equation model; SR, solar radiation; VBNC, viable but non-culturable cells; WS, wind speed.
Abay, S., Yaman, A., Karakava, E., and Fuat, A. (2022). Prevalence and antibacterial susceptibilities of Arcobacter spp. and Campylobacter spp. from fresh vegetables. World J. Microbiol. Biotechnol. 38:132. doi: 10.1007/s11274-022-03315-3
Alegbeleye, O. O., and Sant’Ana, A. S. (2020). Manure-borne pathogens as an important source of water contamination: an update on the dynamics of pathogen survival/transport as well as practical risk mitigation strategies. Int. J. Hyg. Environ. Health 227:113524. doi: 10.1016/j.ijheh.2020.113524
Allard, S. M., Callahan, M. T., Bui, A., Ferelli, A. M. C., Chopyk, J., Chattopadhyay, S., et al. (2019). Creek to table: tracking fecal indicator bacteria, bacterial pathogens, and total bacterial communities from irrigation water to kale and radish crops. Sci. Total Environ. 666, 461–471. doi: 10.1016/j.scitotenv.2019.02.179
Andrews, W. H., Wang, H., Jacobson, A., Ge, B., Zhang, G., and Hammack, T. (2022). BAM Chapter 5: Salmonella. Silver Spring, MA: U.S. Food and Drug adminstration.
Atwill, E. R., Jay-Russell, M., Li, X., Vivas, E., Kilonzo, C., and Mandrell, R. (2012). “Methodological and epidemiological concerns when comparing microbial food safety risks from wildlife, livestock, and companion animals,” in Proceedings of the 25th Vertebrate Pest Conference, (Davis, CA: Western Center for Food Safety, University of California). doi: 10.5070/V425110514
Bardsley, C. A., Weller, D. L., Ingram, D. T., Chen, Y., Oryang, D., Rideout, S. L., et al. (2021). Strain, soil-type, irrigation regimen, and poultry litter influence Salmonella survival and die-off in agricultural soils. Front. Microbiol. 12:590303. doi: 10.3389/fmicb.2021.590303
Belias, A., Strawn, L. K., Wiedmann, M., and Weller, D. (2021). Small produce farm environments can harbor diverse Listeria monocytogenes and Listeria spp. populations. J. Food Prot. 84, 113–121. doi: 10.4315/JFP-20-179
Brennan, F. P., Moynihan, E., Griffiths, B. S., Hillier, S., Owen, J., Pendlowski, H., et al. (2014). Clay mineral type effect on bacterial enteropathogen survival in soil. Sci. Total Environ. 46, 302–305. doi: 10.1016/j.scitotenv.2013.08.037
Burall, L. S., Sepehri, S., Srinivasan, D., Grim, C. J., Lacher, D. W., Ferguson, M., et al. (2021). Development and validation of a quantitative pcr method for species verification and serogroup determination of Listeria monocytogenes isolates. J. Food Prot. 84, 333–344. doi: 10.4315/JFP-20-178
Chai, L. C., Ghazali, F. M., Bakar, F. A., Lee, H. Y., Suhaimi, L. R., Talib, S. A., et al. (2009). Occurrence of thermophilic Campylobacter spp. contamination on vegetable farms in Malaysia. J. Microbiol. Biotechnol. 19, 1415–1420. doi: 10.4014/jmb.0901.0002
Collado, L., and Figueras, M. J. (2011). Taxonomy, epidemiology, and clinical relevance of the genus Arcobacter. Clin. Microbiol. Rev. 24, 174–192. doi: 10.1128/CMR.00034-10
Cooley, M., Carychao, D., Crawford-Miksza, L., Jay, M. T., Myers, C., Rose, C., et al. (2007). Incidence and tracking of Escherichia coli O157:H7 in a major produce production region in California. PLoS One 2:e1159. doi: 10.1371/journal.pone.0001159
Daims, H. (2014). “The family nitrospiraceae,” in The Prokaryotes, eds D. E. F. Rosenberg, S. Lory, E. Stackebrandt, and F. Thompson (Berlin: Springer), 733–749. doi: 10.1007/978-3-642-38954-2_126
Daquigan, N., Grim, C. J., White, J. R., Hanes, D. E., and Jarvis, K. G. (2016). Early recovery of Salmonella from food using a 6-hour non-selective pre-enrichment and reformulation of tetrathionate broth. Front. Microbiol. 7:2103. doi: 10.3389/fmicb.2016.02103
Devarajan, N., McGarvey, J. A., Scow, K., Jones, M. S., Lee, S., Samaddar, S., et al. (2021). Cascading effects of composts and cover crops on soil chemistry, bacterial communities and the survival of foodborne pathogens. J. Appl. Microbiol. 131, 1564–1577. doi: 10.1111/jam.15054
Donnelly, C. W. (2002). Detection and isolation of Listeria monocytogenes from food samples: implications of sublethal injury. J. AOAC Int. 85, 495–500. doi: 10.1093/jaoac/85.2.495
Dowe, M. J., Jackson, E. D., Mori, J. G., and Bell, C. R. (1997). Listeria monocytogenes survival in soil and incidence in agricultural soils (dagger). J. Food Prot. 60, 1201–1207. doi: 10.4315/0362-028X-60.10.1201
Draper, A. D., Doores, S., Gourama, H., and LaBorde, L. F. (2016). Microbial survey of pennsylvania surface water used for irrigating produce crops. J. Food Prot. 79, 902–912. doi: 10.4315/0362-028X.JFP-15-479
Edgar, R. C. (2010). Search and clustering orders of magnitude faster than BLAST. Bioinformatics 26, 2460–2461. doi: 10.1093/bioinformatics/btq461
Edgar, R. C., and Flyvbjerg, H. (2015). Error filtering, pair assembly and error correction for next-generation sequencing reads. Bioinformatics 31, 3476–3482. doi: 10.1093/bioinformatics/btv401
EFSA, and ECDC (2021). The European union one health 2019 zoonoses report. EFSA J. 19:6406. doi: 10.2903/j.efsa.2021.6406
Falardeau, J., Walji, K., Haure, M., Fong, K., Taylor, G., Ma, Y., et al. (2018). Native bacterial communities and Listeria monocytogenes survival in soils collected from the Lower Mainland of British Columbia, Canada. Can. J. Microbiol. 64, 695–705. doi: 10.1139/cjm-2018-0115
Feng, P., Weagant, S. D., and Jinneman, K. (2020). “BAM: chapter 4a: diarrheagenic Escherichia coli,” in Bacteriological Analytical Manual, eds K. W. B. Jinneman, M. Davidson, P. Feng, B. Ge, G. Gharst, T. Hammack, et al. (Silver Spring, MA: U.S. FDA).
Freitag, N. E. (2009). Listeria monocytogenes – from saprophyte to intracellular pathogen. Nat. Rev. Microbiol. 7, 623–628. doi: 10.1038/nrmicro2171
Gardner, H. (1985). Soil Fertility, ed. O. Natural Resources Conservation Service (Washington, DC: United States Department of Agriculture).
Gardner, T. J., Fitzgerald, C., Xavier, C., Klein, R., Pruckler, J., Stroika, S., et al. (2011). Outbreak of campylobacteriosis associated with consumption of raw peas. Clin. Infect Dis. 53, 26–32. doi: 10.1093/cid/cir249
Ghaju Shrestha, R., Tanaka, Y., Sherchand, J. B., and Haramoto, E. (2019). Identification of 16S rRNA and virulence-associated genes of Arcobacter in water samples in the Kathmandu valley. Nepal. Pathogens 8:110. doi: 10.3390/pathogens8030110
Gnanou Besse, N., Lombard, B., Guillier, L., Francois, D., Romero, K., Pierru, S., et al. (2019). Validation of standard method EN ISO 11290 - Part 1 - detection of Listeria monocytogenes in food. Int. J. Food Microbiol. 288, 13–21. doi: 10.1016/j.ijfoodmicro.2018.03.024
Gorski, L., Walker, S., Liang, A. S., Nguyen, K. M., Govoni, J., Carychao, D., et al. (2014). Comparison of subtypes of Listeria monocytogenes isolates from naturally contaminated watershed samples with and without a selective secondary enrichment. PLoS One 9:e92467. doi: 10.1371/journal.pone.0092467
Gu, G., Luo, Z., Cevallos-Cevallos, J. M., Adams, P., Vellidis, G., Wright, A., et al. (2013). Occurrence and population density of Campylobacter jejuni in irrigation ponds on produce farms in the Suwannee River Watershed. Can. J. Microbiol. 59, 339–346. doi: 10.1139/cjm-2013-0027
Gu, G., Strawn, L. K., Ottesen, A. R., Ramachandran, P., Reed, E. A., Zheng, J., et al. (2020). Correlation of Salmonella enterica and Listeria monocytogenes in irrigation water to environmental factors, fecal indicators, and bacterial communities. Front. Microbiol. 11:557289. doi: 10.3389/fmicb.2020.557289
Gu, G., Strawn, L. K., Zheng, J., Reed, E. A., and Rideout, S. L. (2019). Diversity and dynamics of Salmonella enterica in water sources, poultry litters, and field soils amended with poultry litter in a major agricultural area of Virginia. Front. Microbiol. 10:2868. doi: 10.3389/fmicb.2019.02868
Guevremont, E., Lamoureux, L., Genereux, M., and Cote, C. (2017). Irrigation water sources and time intervals as variables on the presence of Campylobacter spp. and Listeria monocytogenes on romaine lettuce grown in muck soil. J. Food Prot. 80, 1182–1187. doi: 10.4315/0362-028X.JFP-16-551
Harrand, A. S., Strawn, L., Illas-Ortiz, P. M., Wiedmann, M., and Weller, D. (2020). Listeria monocytogenes prevalence varies more within fields than between fields or over time on conventionally farmed New York produce fields. J. Food Prot. 83, 1958–1966. doi: 10.4315/JFP-20-120
Helwig, N. E. (2021). nptest: Nonparametric Bootstrap and Permutation Tests. R package version 1.0-3.
Herbert, S., Hashemi, M., Chickering-Sears, C., Weis, S. M., Carlevale, J., Campbell-Nelson, K., et al. Conserving Ammonia in Manure. UMass Extension Crops, Dairy, Livestock and Equine Program. Available online at: https://ag.umass.edu/crops-dairy-livestock-equine/fact-sheets/conserving-ammonia-in-manure.
Hiett, K. L. (2017). Campylobacter jejuni isolation/enumeration from environmental samples. Methods Mol. Biol. 1512, 1–8. doi: 10.1007/978-1-4939-6536-6_1
Himathongkham, S., Bahari, S., Riemann, H., and Cliver, D. (1999). Survival of Escherichia coli O157:H7 and Salmonella typhimurium in cow manure and cow manure slurry. FEMS Microbiol. Lett. 178, 251–257. doi: 10.1111/j.1574-6968.1999.tb08684.x
Hoa, T. K. H., Lipman, L. J. A., and Gaastra, W. (2006). Arcobacter, what is known and unknown about a potential foodborne zoonotic agent! Vet. Microbiol. 115, 1–13. doi: 10.1016/j.vetmic.2006.03.004
Hoelzer, K., Moreno Switt, A. I., and Wiedmann, M. (2011). Animal contact as a source of human non-typhoidal salmonellosis. Vet. Res. 42:34. doi: 10.1186/1297-9716-42-34
Hruby, C. E., Soupir, M. L., Moorman, T. B., Pederson, C., and Kanwar, R. (2018). Salmonella and fecal indicator bacteria survival in soils amended with poultry manure. Water Air Soil Pollut. 229:32. doi: 10.1007/s11270-017-3667-z
Hsu, T. T., and Lee, J. (2015). Global distribution and prevalence of Arcobacter in food and water. Zoonoses Public Health 62, 579–589. doi: 10.1111/zph.12215
IFSAC (2018). Foodborne Illness Source Attribution Estimates for 2016 for Salmonella, Escherichia coli O157, Listeria monocytogenes, and Campylobacter using Multi-Year Outbreak Surveillance Data, United States, ed. T. I. F. S. A. Collaboration (Atlanda, GA: CDC).
Ivanek, R., Grohn, Y. T., and Wiedmann, M. (2006). Listeria monocytogenes in multiple habitats and host populations: review of available data for mathematical modeling. Foodborne Pathog. Dis. 3, 319–336. doi: 10.1089/fpd.2006.3.319
Iwu, C. D., and Okoh, A. I. (2019). Preharvest transmission routes of fresh produce associated bacterial pathogens with outbreak potentials: a review. Int. J. Environ. Res. Public Health 16:4407. doi: 10.3390/ijerph16224407
Jayaraman, S., Naorem, A. K., Lal, R., Dalal, R. C., Sinha, N. K., Patra, A. K., et al. (2021). Disease-suppressive soils-beyond food production: a critical review. J. Soil Sci. Plant Nutr. 21, 1437–1465. doi: 10.1007/s42729-021-00451-x
Jeyaletchumi, P., Tunung, R. S., Margaret, S., Chai, L. C., Radu, S., Farinazleen, M. G., et al. (2011). Evaluation of Listeria spp. and Listeria monocytogenes in selected vegetable farms. J. Trop. Agric. Fd Sci. 39, 255–266.
Jiang, X., Islam, M., Morgan, J., and Doyle, M. P. (2004). Fate of Listeria monocytogenes in bovine manure-amended soil. J. Food Prot. 67, 1676–1681. doi: 10.4315/0362-028X-67.8.1676
Kim, N. H., Park, S. M., Kim, H. W., Cho, T. J., Kim, S. H., Choi, C., et al. (2019). Prevalence of pathogenic Arcobacter species in South Korea: comparison of two protocols for isolating the bacteria from foods and examination of nine putative virulence genes. Food Microbiol. 78, 18–24. doi: 10.1016/j.fm.2018.09.008
Lam, T. Y., and Maguire, D. A. (2012). Structural equation modeling: theory and applications in forest management. Intl. J. For. Res. 2012:263953. doi: 10.1155/2012/263953
Lappi, V., Archer, J. R., Cebelinski, E., Leano, F., Besser, J. M., Klos, R. F., et al. (2013). An outbreak of foodborne illness among attendees of a wedding reception in Wisconsin likely caused by Arcobacter butzleri. Foodborne Pathog. Dis. 10, 250–255. doi: 10.1089/fpd.2012.1307
Leclercq, A. (2004). Atypical colonial morphology and low recoveries of Listeria monocytogenes strains on Oxford, PALCAM, Rapid’L.mono and ALOA solid media. J. Microbiol. Methods 57, 251–258. doi: 10.1016/j.mimet.2004.01.011
Li, B., Liu, H., and Wang, W. (2017). Multiplex real-time PCR assay for detection of Escherichia coli O157:H7 and screening for non-O157 Shiga toxin-producing E. coli. BMC Microbiol. 17:215. doi: 10.1186/s12866-017-1123-2
Lines-Kelly, R. (1992). Plant Nutrients in the Soil [Online]. Available online at: https://www.dpi.nsw.gov.au/agriculture/soils/improvement/plant-nutrients (accessed July 29 2021).
Linton, D., Owen, R. J., and Stanley, J. (1996). Rapid identification by PCR of the genus Campylobacter and of five Campylobacter species enteropathogenic for man and animals. Res. Microbiol. 147, 707–718. doi: 10.1016/S0923-2508(97)85118-2
Locatelli, A., Spor, A., Jolivet, C., Piveteau, P., and Hartmann, A. (2013). Biotic and abiotic soil properties influence survival of Listeria monocytogenes in soil. PLoS One 8:e75969. doi: 10.1371/journal.pone.0075969
Ma, Y., Ju, C., Zhou, G., Yu, M., Chen, H., He, J., et al. (2022). Genetic characteristics, antimicrobial resistance, and prevalence of Arcobacter spp. isolated from various sources in Shenzhen. China. Front. Microbiol. 13:1004224. doi: 10.3389/fmicb.2022.1004224
Magoc, T., and Salzberg, S. L. (2011). FLASH: fast length adjustment of short reads to improve genome assemblies. Bioinformatics 27, 2957–2963. doi: 10.1093/bioinformatics/btr507
Marine, S. C., Pagadala, S., Wang, F., Pahl, D. M., Melendez, M. V., Kline, W. L., et al. (2015). The growing season, but not the farming system, is a food safety risk determinant for leafy greens in the mid-Atlantic region of the United States. Appl. Environ. Microbiol. 81, 2395–2407. doi: 10.1128/AEM.00051-15
Matias Rodrigues, J. F., Schmidt, T. S. B., Tackmann, J., and von Mering, C. (2017). MAPseq: highly efficient k-mer search with confidence estimates, for rRNA sequence analysis. Bioinformatics 33, 3808–3810. doi: 10.1093/bioinformatics/btx517
Micallef, S. A., Rosenberg Goldstein, R. E., George, A., Kleinfelter, L., Boyer, M. S., McLaughlin, C. R., et al. (2012). Occurrence and antibiotic resistance of multiple Salmonella serotypes recovered from water, sediment and soil on mid-Atlantic tomato farms. Environ. Res. 114, 31–39. doi: 10.1016/j.envres.2012.02.005
Nachtigall, C., Kroehne, U., Funke, F., Steyer, R., and Schiller, F. (2003). (Why) should we use sem? pros and cons of structural equation modeling. MethPsychol Res. Online 8, 1–22.
Nguyen, P. T., JuArez, O., and Restaino, L. (2021). A new method for detection of Arcobacter butzleri, Arcobacter cryaerophilus, and Arcobacter skirrowii using a novel chromogenic agar. J. Food Prot. 84, 160–168. doi: 10.4315/JFP-20-245
Niedermeyer, J. A., Miller, W. G., Yee, E., Harris, A., Emanuel, R. E., Jass, T., et al. (2020). Search for Campylobacter spp. reveals high prevalence and pronounced genetic diversity of Arcobacter butzleri in floodwater samples associated with Hurricane Florence in North Carolina, USA. Appl. Environ. Microbiol. 86, e1118–e1120. doi: 10.1128/AEM.01118-20
Oksanen, J., Guillaume Blanchet, F., Friendly, M., Kindt, R., Legendre, P., McGlinn, D., et al. (2019). vegan: Community Ecology Package. R package version 2.5-6.
Pandey, P. K., Kass, P. H., Soupir, M. L., Biswas, S., and Singh, V. P. (2014). Contamination of water resources by pathogenic bacteria. AMB Express 4:51. doi: 10.1186/s13568-014-0051-x
Parada, A. E., Needham, D. M., and Fuhrman, J. A. (2016). Every base matters: assessing small subunit rRNA primers for marine microbiomes with mock communities, time series and global field samples. Environ. Microbiol. 18, 1403–1414. doi: 10.1111/1462-2920.13023
Park, S. Y., and Ha, S. D. (2015). Development of an absorbance-based response model for monitoring the growth rates of Arcobacter butzleri as a function of temperature, pH, and NaCl concentration. Poult. Sci. 94, 136–143. doi: 10.3382/ps/peu022
Parsons, C., Jahanafroozi, M., and Kathariou, S. (2019). Requirement of lmo1930, a gene in the menaquinone biosynthesis operon, for esculin hydrolysis and lithium chloride tolerance in Listeria monocytogenes. Microorganisms 7:539. doi: 10.3390/microorganisms7110539
Persad, A. K., and LeJeune, J. T. (2015). “Animal reservoirs of shiga toxin-producing Escherichia coli,” in Enterohemorrhagic Escherichia coli and Other Shiga Toxin-Producing E. coli, ed. V. H. Sperandio (Washington, DC: ASM Press), 211–230. doi: 10.1128/9781555818791.ch11
Quandt, R. E. (1960). Tests of the hypothesis that a linear regression system obeys two separate regimes. J. Am. Stat. Assoc. 55, 324–330. doi: 10.1080/01621459.1960.10482067
Ramees, T. P., Dhama, K., Karthik, K., Rathore, R. S., Kumar, A., Saminathan, M., et al. (2017). Arcobacter: an emerging food-borne zoonotic pathogen, its public health concerns and advances in diagnosis and control - a comprehensive review. Vet. Q. 37, 136–161. doi: 10.1080/01652176.2017.1323355
Rosseel, Y. (2012). lavaan: an r package for structural equation modeling. J. Stat. Softw. 48, 1–36. doi: 10.18637/jss.v048.i02
Roswell, M. E., Dushoff, J., and Winfree, R. (2021). A conceptual guide to measuring species diversity. Oikos 130, 321–338. doi: 10.1111/oik.07202
Rutter, E. B., Diaz, D. R., and Hargrave, L. M. (2022). Evaluation of Mehlich-3 for determination of cation exchange capacity in Kansas soils. Soil Sci. Soc. Am. J. 86, 146–156. doi: 10.1002/saj2.20354
Scallan, E., Hoekstra, R. M., Angulo, F. J., Tauxe, R. V., Widdowson, M. A., Roy, S. L., et al. (2011). Foodborne illness acquired in the United States-major pathogens. Emerg. Infect Dis. 17, 7–15. doi: 10.3201/eid1701.P11101
Shah, M. K., Bradshaw, R., Nyarko, E., Handy, E. T., East, C., Millner, P. D., et al. (2019). Salmonella enterica in soils amended with heat-treated poultry pellets survived longer than bacteria in unamended soils and more readily transferred to and persisted on spinach. Appl. Environ. Microbiol. 85:e334–19. doi: 10.1128/AEM.00334-19
Sharma, M., and Reynnells, R. (2016). Importance of soil amendments: survival of bacterial pathogens in manure and compost used as organic fertilizers. Microbiol. Spectr. 4. doi: 10.1128/microbiolspec.PFS-0010-2015
Sharma, M., Millner, P. D., Hashem, F., Vinyard, B. T., East, C. L., Handy, E. T., et al. (2019). Survival of Escherichia coli in manure-amended soils is affected by spatiotemporal, agricultural, and weather factors in the Mid-Atlantic United States. Appl. Environ. Microbiol. 85:e2392–18. doi: 10.1128/AEM.02392-18
Sher, A. A., Ashraf, M. A., Mustafa, B. E., and Raza, M. M. (2021). Epidemiological trends of foodborne Campylobacter outbreaks in the United States of America, 1998-2016. Food Microbiol. 97:103751. doi: 10.1016/j.fm.2021.103751
Stea, E. C., Purdue, L. M., Jamieson, R. C., Yost, C. K., and Truelstrup Hansen, L. (2015). comparison of the prevalences and diversities of Listeria species and Listeria monocytogenes in an urban and a rural agricultural watershed. Appl. Environ. Microbiol. 81, 3812–3822. doi: 10.1128/AEM.00416-15
Strawn, L. K., Fortes, E. D., Bihn, E. A., Nightingale, K. K., Grohn, Y. T., Worobo, R. W., et al. (2013a). Landscape and meteorological factors affecting prevalence of three food-borne pathogens in fruit and vegetable farms. Appl. Environ. Microbiol. 79, 588–600. doi: 10.1128/AEM.02491-12
Strawn, L. K., Grohn, Y. T., Warchocki, S., Worobo, R. W., Bihn, E. A., and Wiedmann, M. (2013b). Risk factors associated with Salmonella and Listeria monocytogenes contamination of produce fields. Appl. Environ. Microbiol. 79, 7618–7627. doi: 10.1128/AEM.02831-13
Uljanovas, D., Golz, G., Bruckner, V., Grineviciene, A., Tamuleviciene, E., Alter, T., et al. (2021). Prevalence, antimicrobial susceptibility and virulence gene profiles of Arcobacter species isolated from human stool samples, foods of animal origin, ready-to-eat salad mixes and environmental water. Gut Pathog. 13:76. doi: 10.1186/s13099-021-00472-y
Vivant, A.-L., Garmyn, D., and Piveteau, P. (2013). Listeria monocytogenes, a down-to-earth pathogen. Front. Cell Infect. Microbiol. 3:87. doi: 10.3389/fcimb.2013.00087
Weller, D., Belias, A., Green, H., Roof, S., and Wiedmann, M. (2020). Landscape, water quality, and weather factors associated with an increased likelihood of foodborne pathogen contamination of New York streams used to source water for produce production. Front. Sustain. Food Syst. 3:124. doi: 10.3389/fsufs.2019.00124
Weller, D., Wiedmann, M., and Strawn, L. K. (2015a). Spatial and temporal factors associated with an increased prevalence of Listeria monocytogenes in New York State spinach fields. Appl. Environ. Microbiol. 81, 6059–6069. doi: 10.1128/AEM.01286-15
Weller, D., Wiedmann, M., and Strawn, L. K. (2015b). Irrigation is significantly associated with an increased prevalence of Listeria monocytogenes in produce production environments in New York State. J. Food Prot. 78, 1132–1141. doi: 10.4315/0362-028X.JFP-14-584
Wesley, I. V., Wells, S. J., Harmon, K. M., Green, A., Schroeder-Tucker, L., Glover, M., et al. (2000). Fecal shedding of Campylobacter and Arcobacter spp. in dairy cattle. Appl. Environ. Microbiol. 66, 1994–2000. doi: 10.1128/AEM.66.5.1994-2000.2000
Zeileis, A., Kleiber, C., Kraemer, W., and Hornik, K. (2003). Testing and dating of structural changes in practice. Comput. Stat. Data Anal. 44, 109–123. doi: 10.1016/S0167-9473(03)00030-6
Keywords: farm management practices, fresh produce, Listeria, Salmonella, Campylobacter, STEC, Arcobacter, structural equation modeling
Citation: Ferguson M, Hsu C-K, Grim C, Kauffman M, Jarvis K, Pettengill JB, Babu US, Harrison LM, Li B, Hayford A, Balan KV, Freeman JP, Rajashekara G, Lipp EK, Rozier RS, Zimeri AM and Burall LS (2023) A longitudinal study to examine the influence of farming practices and environmental factors on pathogen prevalence using structural equation modeling. Front. Microbiol. 14:1141043. doi: 10.3389/fmicb.2023.1141043
Received: 09 January 2023; Accepted: 14 March 2023;
Published: 06 April 2023.
Edited by:
Stephen Forsythe, Foodmicrobe.com, United KingdomReviewed by:
Abasiofiok Mark Ibekwe, United States Department of Agriculture (USDA), United StatesCopyright © 2023 Ferguson, Hsu, Grim, Kauffman, Jarvis, Pettengill, Babu, Harrison, Li, Hayford, Balan, Freeman, Rajashekara, Lipp, Rozier, Zimeri and Burall. This is an open-access article distributed under the terms of the Creative Commons Attribution License (CC BY). The use, distribution or reproduction in other forums is permitted, provided the original author(s) and the copyright owner(s) are credited and that the original publication in this journal is cited, in accordance with accepted academic practice. No use, distribution or reproduction is permitted which does not comply with these terms.
*Correspondence: Laurel S. Burall, Laurel.Burall@fda.hhs.gov
†Present address: Christopher Grim, Karen Jarvis, Office of Regulatory Science, Center for Food Safety and Applied Nutrition, U.S. Food and Drug Administration, College Park, MD, United States
Disclaimer: All claims expressed in this article are solely those of the authors and do not necessarily represent those of their affiliated organizations, or those of the publisher, the editors and the reviewers. Any product that may be evaluated in this article or claim that may be made by its manufacturer is not guaranteed or endorsed by the publisher.
Research integrity at Frontiers
Learn more about the work of our research integrity team to safeguard the quality of each article we publish.