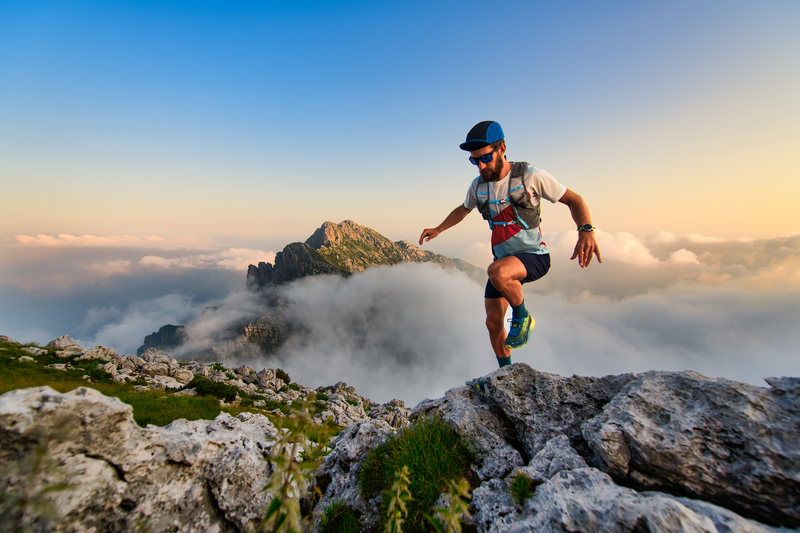
94% of researchers rate our articles as excellent or good
Learn more about the work of our research integrity team to safeguard the quality of each article we publish.
Find out more
ORIGINAL RESEARCH article
Front. Microbiol. , 09 March 2023
Sec. Infectious Agents and Disease
Volume 14 - 2023 | https://doi.org/10.3389/fmicb.2023.1138928
Gastric microbiome has been shown to contribute to gastric carcinogenesis, understanding how alterations in gastric microbiome is helpful to the prevention and treatment of gastric cancer (GC). However, few studies have focused on the change of microbiome during the gastric carcinogenesis. In this study, the microbiome of gastric juice samples from healthy control (HC), gastric precancerous lesions (GPL) and gastric cancer (GC) was investigated by 16S rRNA gene sequencing. Our results showed that the alpha diversity of patients with GC was significantly lower than other groups. Compared to other groups, some genera in GC group were shown to be up-regulated (e.g., Lautropia and Lactobacillus) and down-regulated (e.g., Peptostreptococcus and Parvimonas). More importantly, the emergence of Lactobacillus was closely related to the occurrence and development of GC. Moreover, the microbial interactions and networks in GPL exhibited higher connectivity, complexity and lower clustering property, while GC showed the opposite trend. Taken together, we suggest that changes in the gastric microbiome are associated with GC and perform a key function in maintaining the tumor microenvironment. Therefore, our findings will provide new ideas and references for the treatment of GC.
Gastric cancer (GC) is the fifth most frequently diagnosed cancer and the fourth-leading cause of cancer-related deaths worldwide (Sung et al., 2021). Although the incidence and mortality rates are declining with the advancement of therapeutic, GC is still a global medical burden (Thrift and El-Serag, 2020). Therefore, it is necessary to understand the mechanism of GC to better treat and prevent the occurrence of this disease. The development of GC can be described as a series of sequential stages: from chronic superficial gastritis, chronic atrophic gastritis, intestinal metaplasia, dysplasia to GC (Correa, 1992). The microbiome is involved in the development of GC and the composition of gastric microorganisms varies in individuals at different stages of the stomach (Stewart et al., 2020). Previous studies have suggested that many factors are associated with the development of GC. In addition to genetic predisposition, environmental factors including microbial community interactions have been shown to contribute to GC (Kwon et al., 2021).
Microbiome is considered a significant component of the tumor microenvironment. The human gastrointestinal tract contains a large number of microbiomes, including bacteria, fungi and viruses. Helicobacter pylori (HP) infection is a fundamental factor for gastric lesions (Vinasco et al., 2019). However, the presence of HP is not the only factor involved in gastric carcinogenesis, as only a minority of HP infected individuals develop GC, implying that other factors also perform a key function (Bjorkholm et al., 2003). Naturally, more attention has been paid to the function of gastric microbiome diversity in the development of GC. Experiments in the insulin-gastrin transgenic (INS-GAS) mouse model have demonstrated that the synergy among bacterial communities promotes gastric tumorigenesis (Lertpiriyapong et al., 2014; Whary et al., 2014). Later, gastric microbiome was also found to produce carcinogenic N-nitroso compounds and secondary amines by metabolizing food, which strengthened the role of gastric microbiome in the development of GC (Tseng et al., 2016). A study found the relative abundance and diversity of microbiome in the GC group decreased compared to patients with chronic gastritis (Ferreira et al., 2018). In addition, changes in gastric microbiome in patients with GC have been detected by using 16S rRNA gene sequencing in several studies recently, and gastric carcinogenesis was revealed to be associated with microbial dysbiosis (Chen et al., 2019; Gantuya et al., 2020; Zhang et al., 2021). Together, these findings highlighted the potential role of microbial community structure diversity in the development of GC.
Some studies have been devoted to revealing the differences in the microbial composition from noncancerous to GC. However, there is no consensus on the microbiome changes in the pathological stages of GC. In this study, gastric juice samples from patients with healthy control (HC) gastric precancerous lesions (GPL) and GC were collected, aiming to explore the differential distribution profile of microbiome in different stages of gastric lesions by using 16S rRNA gene sequencing. Our findings will help explore the role of gastric microbiome in carcinogenesis.
Total 60 participants, including 22 of HC, 22 of GPL and 16 of GC, were recruited at the Xiangya Hospital, Changsha, Hunan, China from October 2015 to November 2016. The demographic characteristics of participants were shown in Table 1. Among them, the HC group mainly consisted of patients with gastritis. Gastritis and gastric precancerous lesions patients were diagnosed by the gastrointestinal endoscopes Department of Xiangya Hospital according to the clinical practices (Chinese Medical Association, 2006; Medical Secretary, M.O.H., People's Republic of China, 2012). In addition, GPL refers to a kind of pathological change of gastric mucosa liable to cancerization. The inclusion criteria of GPL were atrophic gastritis, intestinal metaplasia or adenomatous polyp. The study was approved by the independent Ethics Committee of Xiangya Hospital of Central South University following the ethical guidelines of the Declaration of Helsinki (No. 038, 2015). Participation was voluntary and written informed consent was obtained from all participants.
Exclusion criteria were as follows: age under 18 years; the presence of a serious illness such as severe cardiopulmonary, renal, or metabolic diseases; prior medication history of antibiotics, acid drugs (proton pump inhibitors and H2 receptor antagonists), probiotics, or anti-inflammatory drugs (aspirin, nonsteroidal and steroids) for past one month; A large amount of alcohol and smoke for past a month. All patients were subject to endoscopy or biopsy.
The patients were treated according to clinical requirements and then undergo gastrointestinal endoscopy. Approximately, 10 mL of gastric juice was collected from the gastroscope with sterile syringe, then filtered by the double sterile gauze to remove food debris and stored in sterile 10–15 mL tubes. The tubes were kept at 0°C for no more than 12 h prior to DNA isolation. DNA isolation of the bacterial sediments was performed using the QIAamp® FAST DNA Stool Mini Kit (QIAGEN) according to the manufacturer’s protocol after centrifuging at 12,000 rpm, 4°C, for 10 min.
The V4 region of the 16S rRNA gene was amplified by polymerase chain reaction (PCR) with a universal forward primer and a unique barcoded fusion reverse primer (515\u00B0F: 5′-gtgccagcmgccgcggtaa-3′ and 806 R: 5′-ggactachvgggtwtctaat-3′). PCR was performed using 30 ng of genome DNA, V4 Dual-index Fusion PCR Primer Cocktail and PCR Master Mix (NEB Phusion High-Fidelity PCR Master Mix) for per reaction. The melting temperature is 56°C and PCR cycle is 30. The PCR products were purified with AmpureXP beads (AGENCOURT) to remove the unspecific products. The library was quantitated by determination of the average molecule length using the Agilent 2100 bioanalyzer instrument (Agilent DNA 1000 Reagents) and quantification of the library by real-time quantitative PCR (EvaGreenTM). Then, the qualified libraries were sequenced by the way of pair-end on the Illumina MiSeq System with the sequencing strategy PE250 (MiSeq Reagent Kit) by Beijing Genomics Institute (BGI, Wuhan, China).
After sequencing, the reads were de-multiplexed into samples according to the barcodes. Fitter sequences were imported to the Quantitative Insights into Microbial Ecology (QIIME2, 2022.2)1 (Bolyen et al., 2019). The raw data were filtered to eliminate the adapter pollution and low-quality reads to obtain clean reads, and then use DADA2 denoise-paired to dereplicate sequence data and create a feature table and feature representative sequences (Callahan et al., 2016). Taxonomic classifiers use plugin feature-classifier, classify-consensus-blast, based on Silva 138 reference sequence (MD5: a914837bc3f8964b156a9653e2420d22) and taxonomy files (MD5: e2c40ae4c60cbf75e24312bb24652f2c) (Camacho et al., 2009; Bokulich et al., 2018; Robeson et al., 2021). Use plugin taxa removal of non-bacterial sequences and mitochondrial chloroplast contamination.
All statistical analyses were performed using the R V4.1.2 environment (R Core Team, 2022). No special instructions, the statistical results were visualized using the “ggplot2” package (Wickham, 2009). Alpha diversity was measured using the function diversity in the package “Vegan” based on a flat taxonomy table. Gini-Simpson diversity index was obtained by subtracting the value of the classical Simpson index from 1. Beta diversity was compared using principal coordinate analysis (PCoA). Bacterial community composition across all samples based on Bray-Curtis distances. Redundancy analysis (RDA) was also conducted using Vegan (Lai et al., 2022). Package rdacca.hp. was used to obtain conditional effect base on Hierarchical Partitioning (Oksanen et al., 2022). Beta diversity across sample groups was compared by PERMANOVA with permutations of 999. ANOSIM was chosen to test for significance between groups (Wang et al., 2008), R > 0, p < 0.05 was considered significant. The DEseq2 package was used to analyze abundance difference and Marker genus (Topper et al., 2017). The differential expression matrix and the p-value matrix of species composition were obtained through the function DESeqDataSetFromMatrix. The significant level was p < 0.05 and the absolute FoldChange value greater than 2. The coexistence network of three groups was established based on Spearman correlation matrix and corrected p-value matrix using the igraph package; the Benjamini and Hochberg false discovery rate (FDR) were used to correct the p value; modules were divided according to the high intra-module connectivity and the low inter-module connectivity; Spearman correlation coefficient and corrected p values were 0.8 and 0.05, respectively, (Yuan et al., 2021). Gephi software2 is used to calculate the network topological properties and the hub network. Among them, clustering coefficient is a measure of the degree of clustering property of microorganism. Abundance ratio greater than 0.05 genus abundance heatmap was created using the pheatmap package, and the abundance information was transformed by adding one, then taking the logarithm of ten (Kolde, 2019). Phylum level Manhattan plot computed using edgeR package based on taxonomy information (Robinson et al., 2010).
In addition, biomarkers of sample groups were discovered by Linear Discriminant Analysis (LDA) Effect Size (LEfSe)3 (Segata et al., 2011). The strategy for multi-class analysis was set one-against-all, and the threshold on the logarithmic LDA score for discriminative features was set to 2.0.
The demographic characteristics of the HC, GPL, and GC groups are shown in Table 1. There is no difference in gender, smoking history, and drinking history, but difference in median age was detected (p < 0.05) among the three groups. On the one hand, RDA analysis showed that gender and age of all participants accounted for only 3.37% of the composition and distribution of microorganisms in gastric juice (Supplementary Figure S1A). On the other hand, the conditional effect of age on microorganisms in gastric juice is 0.70% (Supplementary Figure S1B). Moreover, the clinical data of the GC group population were shown in Table 1. The condition of patients with HP infection was shown in Supplementary Tables S1, S2.
Diversity rarefaction curves of all sample species tended to be parallel to the X-axis, indicating that there is a significant difference (Supplementary Figure S2). The alpha diversity indices Richness (Figure 1A), Gini-Simpson (Figure 1B) and Shannon Wiener (Figure 1C) decreased gradually with disease progression among HC, GPL, and GC groups, there are significant differences among Richness groups (p < 0.05). Compared by using principal coordinate analysis (PCoA), and the ANOSIM test (R = 0.077, p < 0.05), beta diversity demonstrated significant differences among groups. The PCoA results showed that clustered within groups with a smaller area in the HC group than GPL and GC groups, where the Bray-Curtis distances between HC and GPL were closer than HC and GC groups (Figure 1D; Supplementary Figure S3). The difference between groups was greater than that within groups, implying a significant difference in diversity among HC, GPL and GC groups (Figure 1D).
Figure 1. The microbial diversity of microbial communities. Alpha diversity index analysis richness (A), Gini-Simpson (B), and shannon-wiener (C) (kruskal–wallis test, *p < 0.05, **p < 0.01). (D) Beta diversity PCoA analysis (ANOSIM R = 0.077, p < 0.05), the ellipse contains 85% of the samples in each group. The difference of composition in phylum (E), genus (F) level between three groups. HC, healthy control; GPL, gastric precancerous lesions; GC, gastric cancer.
There were commonalities and differences in bacterial composition among the HC, GPL and the GC group. At the phylum level, 14 phyla were in the HC and GC group while 13 phyla were in the GPL group (Figure 1E). At the genus level, 157 genera were in the HC group, 150 in the GPL group, 131 in the GC group, only 95 genera common to all groups (Figure 1F). The differences in composition at the genus level among the three groups were greater than those at the phylum level. To clarify the bacterial differences among the groups, we compared the differentially abundant bacteria among the three groups at the phylum and the genus level, respectively. At the phylum level, the results indicated that the composition of the three groups was similar, but the relative abundance of components varied (Figures 2A,B). We analyzed the bacterial alterations between the GPL, GC, and HC groups. Comparison with the HC group, the decreased abundance of bacterial species was greater than the increased abundance in the GPL and GC groups (Figure 2C). Similar patterns of variation were also found in the comparison of GC and GPL groups (Supplementary Figure S4). Further analysis of bacterial abundance differences at the phylum level showed the same results. With the course of gastric disease intensified (HC, GPL, and GC), phylum Proteobacteria (Figure 2D) and Spirochaetota (Figure 2F) showed a significant down-regulation of gastric juice microbiome, while Firmicutes phylum (Figure 2E) showed a significant up-regulation (p < 0.05). Genus level abundance heatmap showed the distribution of abundance at the genus level for each sample. Prevotella, Alloprevotella, Haemophilus, Neisseria, Fusobacterium were the high-abundance genus (Supplementary Figure S5).
Figure 2. The relative abundances of taxonomy at the phylum level. (A) The relative abundances of taxonomy at the phylum level in all samples (60). (B) The relative abundances in phylum level in three groups. (C) Demonstration of precancerous lesions gastric cancer (GPL/GC) versus bacterial group with healthy control (HC). X-axis for ASVs, alphabetically ordered by taxonomic phylum level; Y-axis p value values for the comparison of the two groups, taken as loge (P), i.e., natural logarithmic transformation; the size of the nodes in the graph represents the relative abundance of that ASV, taken as log2 (CPM), the logarithm of 2; CPM is an abbreviation for count per million, which being fractions of a million; different nodes colors represent different phylum; the shape of the nodes in the graph marks the type of its change, whether it is up-regulated enriched (positive solid triangle), down-regulated depleted (inverted hollow triangle), or no significant difference change nosig (solid nodes). Differences abundance at the phylum level among groups (Wilcox. test, * p < 0.05, ** p < 0.01), Proteobacteria (D), Firmicutes (E), Spirochaetota (F).
To better elucidate the gastric juice microbial biomarkers among the HC, GPL, GC groups, we used three methods to analyze the markers. The results of LEfSe showed that the identified intergroup biomarker genera echoed the previous intergroup differences at the phylum level (Figure 3A), and Treponema, Campylobacter, Neisseria, Sphingomonas, Vulcaniibacterium, and Lactobacillus were biomarker genera (Figure 3B). Furthermore, GC and GPL showed decreased abundance of the genera Treponema, Campylobacter, and Neisseria, while Lactobacillus abundance increased compared to the HC group. Among them, genera Vulcaniibacterium, Sphingomonas were commonly found in the HC group but largely undetectable in the GC and GPL groups (Figure 3C). Lautropia was up-regulated and Escherichia-Shigella was down-regulated in both GC and GPL compared to the HC group, and the same result was observed in the GC group compared to the GPL group (Figures 4A,B). Compared with the GPL group, the abundance of Mycoplasma, Treponema was down-regulated in the GC group. The metastatic showed the GC group had significantly higher abundance in Streptococcus and Lactobacillus compared with the GPL and HC (Figure 4C). To further compare the commonalities and differences of the biomarkers discovered by several methods, we plotted the upsets. Interestingly, we found the biomarkers jointly identified based on LEfSe, DESeq2 and Metastats analysis methods have an intersection, which is Lactobacillus (Figure 4D).
Figure 3. LEfSe analysis of taxonomy with significant differences in abundance among groups. (A) Evolutionary branching diagram. The circles radiating from the inside to the outside represent taxonomic levels from the phylum to the genus. Each small circle at different taxonomic levels represents a taxon at that level, and the diameter size of the small circles is proportional to the relative abundance size; species without significant differences are uniformly colored in chartreuse, and the difference species Biomarker follows the group for coloring, red nodes indicate microbial taxa that play an important role in the HC group, green nodes indicate microbial taxa that play an important role in the GC group, purple nodes indicate microbial taxa that play an important role in the GPL group. The names of the species indicated by letters in the figure are shown in the legend on the right. (B) Histogram of LDA value, taxon with significantly different abundances in different groups are shown, and the length of the bar graph represents the effect size of the significantly. (C) Comparison of the abundance of biomarkers in each sample among HC, GPL and GC groups. HC, healthy control; GPL, gastric precancerous lesions; GC, gastric cancer.
Figure 4. Differential abundance analysis at the species level. The volcano shows significantly up-regulated and down-regulated in the gastric precancerous lesions (GPL) and gastric cancer (GC) group compared with the healthy control (HC) group (A); GC compared with the GPL group (B). The color of the nodes in the volcano marks the type of its change, blue indicates down-regulation, red indicates up-regulation, and gray indicates no significant difference between groups. (C) Differential abundance analysis at the genus level base on meta, LDA, DESeq2 and hub genus in co-occurrence network. The different relative abundance among three groups based on metastats. Significant differences among groups are indicated by alphabetic letters above the bars, determined by multiple comparison LSD-T test (p < 0.05). (D) This upset diagram shows the biomarkers found based on Metastats, LDA and DESeq2.
We performed a network co-occurrence analysis to unravel the relationships among microorganisms. With the same network construction parameters, the HC group network (Figure 5A) had 88 nodes and 177 edges, the GPL group (Figure 5B) had 91 nodes and 329 edges, while 84 nodes and 157 edges in GC group network (Figure 5C). In addition, HC group and GC groups are all clustered into 23 modules, while GPL group has only 19 modules (Table S3). The results suggested that microbial networks were composed of tightly connected nodes and formed a kind of “small-world” topology (Supplementary Figure S6; Supplementary Table S3). Compared with the topological properties of the random network with the same number of nodes and edges (Supplementary Figure S6; Supplementary Table S4), the network of the HC, GPL and GC groups exhibited a scale-free characteristic (p < 0.001, Supplementary Figure S7), indicating that the network structure was non-random. Correspondingly, we also analyzed the network properties for each group of networks. The average degree of the HC and GC groups were 3.078 and 4.023, which were lower than that of the GPL group (7.231, p < 0.001, Figure 5D), and the number of sides forming triangles was also lower (p < 0.001, Figure 5E). This suggests that total connectivity and complexity between gastric juice microbiome was higher in the GPL group than in the HC and GC group. The network Cluster of the GC group was significantly higher than that of the GPL group (p < 0.01, Figure 5F). These results manifested that the average “clustering property” of the whole network between gastric microorganisms in the GPL group was lower than that in the HC and GC groups. To understand each network in three groups deeply, we extracted the microbial hub network. Among the three groups of hub networks, Anaerococcus was the highest abundance in the HC group (Figure 5G), Cupriavidus was the highest abundant in the GPL group (Figure 5H), and in the GC group hub network, Moraxella was the highest abundant genus (Figure 5I). Overall, the GPL group hub network has the highest number of nodes and the highest agglomeration. The above findings can be concluded that there are differences in the gastric juice microorganism interaction network among the HC, GPL, and GC groups. Compared with the HC and GC groups, the GPL group network has the highest connectivity and complexity and the lowest clustering property.
Figure 5. Co-occurrence network in three groups. (A) healthy control (HC) network, (B) gastric precancerous lesions (GPL) network and (C) gastric cancer (GC) network. Comparison of network topology properties among groups, weighted degree (D), triangles (E), cluster (F). Wilcoxon test, **p < 0.01; ***p < 0.001. Co-occurrence hub network, HC (G), GPL (H), GC (I).
Gastric juice as samples were investigated in the present study, which was different from other studies using gastric mucosa (Yu et al., 2017; Ferreira et al., 2018; Png et al., 2022). Compared to gastric mucosa, gastric juice had two advantages in the study of gastric microbiota its homogeneity and noninvasiveness.
Previous studies have demonstrated that the gastric microbiome structure changes during carcinogenesis (Lam et al., 2017). It has been thought that HP was closely related to the development of GC, but the results of the available studies indicate that the risk of GC was not proportional to the degree of HP infection (Diaz et al., 2018). HP penetrates the gastric mucosa through flagella and mainly adheres to the surface of gastric epithelial cells. Therefore, other microbiome in gastric juice may be of greater importance for GC development (Hessey et al., 1990; Ruch and Engel, 2017). Our study pointed out that the gastric microbial composition and network of patients with GC have changed compared to the patients with HC and GPL. We identified the differences in the gastric microbiome among GC group and other groups, explored potential correlations, and also revealed the interaction between the gastric microbiome. The alpha diversity of GC group was significantly lower than other groups, and the alpha diversity indexes decreased gradually with the progression of gastric lesions. The microbial composition of the patients with GC gradually deviates from other groups. In addition, in the microbial interactions of the three groups, we found that networks of the GPL group exhibited higher connectivity, complexity and lower clustering property, which in the GC group showed the opposite.
The occurrence of GC starts from superficial gastritis, but the key factors promoting the development of GC are still unclear (Correa, 1992; Li et al., 2022). The microbial community is generally recognized as an important biological factor in the development of GC. Previous studies have shown that the structure of gastric microbial diversity was constantly changing in the process of gastric lesions (Wang et al., 2018). In the case of GC patients, the microbial community diversity was quite different from other groups (Coker et al., 2018). Our results were consistent with these showed that the alpha diversity of patients with GC was significantly lower than other groups. In addition, the PCoA analysis of the microbiome composition revealed that there were significant differences in the community structure between the GC group and other groups. This is in keeping with previous findings that the composition of the microbial community changed as the stomach disease gradually deepened (Wang et al., 2018). Therefore, our findings suggested that changes in microbial diversity were critical to the development of GC. The dysregulation of the gastric microbial community may increase the likelihood of developing cancer. Focusing on microbial diversity contributes to a better understanding of GC course and supports GC prevention and treatment.
Helicobacter pylori is the earliest microorganism reported to be associated with GC. Apart from HP, there are many other microbiome in the gastric, and their interactions are related to the development of GC (Duan et al., 2022). A Portuguese study showed that bacteria such as Streptococcus, Prevotella, Clostridium, and Lactobacillus were significantly increased in GC patients compared with patients with chronic gastritis (Ferreira et al., 2018). In addition, a recent animal study showed that the increasing of Lactobacillus abundance contributed to the development of GC (Dai et al., 2021). In this study, analysis of group differences at the phylum level showed that while the abundance of Firmicutes increased in the GC group, Proteobacteria, and Treponema decreased. To search for potential taxonomies that may be associated with carcinogenesis, we used different methods to identify the most significantly correlated taxa among the three groups. Through LEfSe analysis, we found that Treponema, Campylobacter, Vulcaniibacterium and Neisseria were increased in HC group at the genus level, while Lactobacillus and Streptococcus were significantly enriched in GC group. Neisseria is symbiosis bacteria in the mouth and esophagus, but the translocation and expansion of oral bacteria may be involved in the development of inflammation (Han and Wang, 2013). Campylobacter is closely related to HP, and despite a normal oral bacteria, numerous studies have found that its high transcriptional activity in gastric acid verified its role as a potential pathogen in the gastric (von Rosenvinge et al., 2013; Liu et al., 2018; Cui et al., 2019). Its ability to cause tissue damage and disease has been attributed to the production of virulence factors, leading to changes in epithelial permeability and local tissue destruction (Istivan and Coloe, 2006; Hess et al., 2012). Although there is no direct evidence that the occurrence of gastritis is associated with Campylobacter infection, chronic pathological changes ultimately result from the adhesion and colonization of Campylobacter. The presence of oral microbiome in the HC group suggested that our oral microbiome gradually play a cautionary role in the early stages of gastric lesions. The oral cavity severs as the beginning of the digestive system, its microbiome affects the health of the digestive system. Vulcaniibacterium, a moderate thermophilic bacterium (Yu et al., 2013; Niu et al., 2020), was first found in the stomach, suggesting that it may be closely related to the occurrence of gastritis. Meanwhile, we also observed enrichment of Streptococcus in GC group, which was consistent with previous findings (Sun et al., 2018; Huang et al., 2021). The abundance of Streptococcus has also been reported in several types of cancer, such as colorectal adenocarcinoma (Abdulamir et al., 2011). Taken together, the results suggest that Streptococcus may be involved in gastric carcinogenesis. More attention can be paid to the relationship between this bacteria and GC in the future.
Then, we further observed that compared with the HC group by differential abundance analysis. The up-regulated bacteria in cancer patients are Rotobacter, while down-regulated bacteria include Streptococcus pepticus and Micromonas, which are common oral microflora. Lautropia has been reported to be more abundant in patients with periodontal disease (Papapanou et al., 2020). It is also the predominant microorganism isolated from the sputum of cystic fibrosis patients (Ben Dekhil et al., 1997) and the oral cavity of children infected with human immunodeficiency virus (Rossmann et al., 1998). However, the possible pathogenic mechanism of the bacteria is still unclear. Our study found that this bacteria is up-regulated in GC patients, and its pathogenic mechanism may serve as a key target for future research on the relationship between oral bacteria and human health. In addition, a study on salivary microbiome found that Parvimonas was shown to be inversely associated with the development of GC (Huang et al., 2021), the bacteria were also found in CRC patients (Nakatsu et al., 2015). In the comparison between GPL and GC, we found that Lactobacillus also showed up-regulation while Mycoplasma and Treponema were down-regulated. First, Mycoplasma in gastric juice is down-regulated, which may be due to the ability of its lipoprotein P37 periplasmic transport system to promote cell motility and invasion (Gong et al., 2008; Gomersall et al., 2015). It allows Mycoplasma to colonize on the gastric mucosa by breaking through the mucosal barrier of gastric juice, eventually leading to Mycoplasma decreased. Secondly, Treponema can weaken MMPs’ regulation to tumor cell tissue invasion and exocytosis because the mucosal invasiveness of its major virulence factor chymotrypsin-like protease (Td-CTLP) and the hydrolysis of host-derived matrix metalloproteinases (MMPs), which has major impact on the tumor microenvironment (Kessenbrock et al., 2010; Marttila et al., 2014). Our results reconfirm the relationship between Treponema and gastric cancer.
Lactobacillus is a common probiotic that converts lactose to lactic acid, leading to acidification of the gastric mucosa, which can adapt to growth in gastric juice due to its acidophilic properties (Han et al., 2015). The up-regulation of Lactobacillus in GC has been verified in previous studies on gastric mucosa. Interestingly, we found that several differential analyses indicated that Lactobacillus presents closely related to the occurrence and development of gastric carcinogenesis. Therefore, the existence of Lactobacillus can be regarded as the key bacteria involved in the occurrence of GC. Although Lactobacillus acts as a probiotic, in the context of cancer, its metabolite lactic acid can perform an energy source function for tumor cells. It is also a key participant in many carcinogenesis processes, such as metastasis, angiogenesis, metabolism, and immunosuppression (Hirschhaeuser et al., 2011; Hayes et al., 2021). After the Warburg effect was proposed (Warburg et al., 1927), subsequent studies found that lactate, as an immune destroyer of the tumor microenvironment, could directly mediate its effects on cells, such as by blocking cytotoxicity, motility. Its effects can also be indirectly mediated on cells by inducing immunosuppressive cell types such as Tregs, TAMs, and MDSCs. Immune escape driven by lactate within the tumor microenvironment is a major contributor to cancer growth, progression and metastasis (Brand et al., 2016; Corbet and Feron, 2017). In tumor microenvironment, Lactobacilli do not end up with the Warburg effect of lactic acid production, but as lactate is continuously released from transformed cells to initiate carcinogenesis in susceptible cells and tissues (Brizel et al., 2001; San-Millan and Brooks, 2017). Therefore, Lactobacillus showed an upward trend in the GC group, revealing that it plays an important role in GC. A study using network co-occurrence analysis pointed out that there is an interaction between Lactobacillus and other gastric microbiome (Wang et al., 2020). The increase of Lactobacillus may lead to ecological dysregulation of the gastric fluid by interaction with other bacteria, thus enhancing the carcinogenic potential of the gastric microbiome. Therefore, we can state that Lactobacillus is an important factor for the next studies on gastric cancer prevention and treatment.
Nowadays, a wealth of evidence suggests that changes in the microbiome were related to carcinogenesis. Dysbiosis of the gastric microbiome was reflected not only at the level of changes in the abundance of microbiome members but also in the altered relationships of microbial interactions (Chen et al., 2020; Zhang et al., 2020). Many studies have pointed out that there is widespread competition between bacteria instead of cooperation in networks (Palmer and Foster, 2022). Our network analysis showed that the GPL group had higher network connectivity and complexity and lower aggregation, while the GC group had significantly sparser network connectivity. The high degree of cooperation of GPL group microbial community was closely related to Cupriavidus. It was a multifunctional microorganism found in soil and water, which is resistant to heavy metals and has been studied from environmental samples as well as human samples (Vaneechoutte et al., 2004). In previous studies, Cupriavidus has been reported associated with invasive human infections, such as bacteremia, pneumonia, immunocompromised patients and cystic fibrosis (CF) patients (Coenye et al., 2005; Kobayashi et al., 2016; Bianco et al., 2018). Here, we found that Cupriavidus played a key role in the stage of GPL. We hypothesize that Cupriavidus was associated with the development of GC. Moreover, Pathogenic bacteria breaching the mucosal protective barrier may make contributions to the decreased aggregation in the GC group, thereby promoting a decrease in the degree of microbial interaction in gastric juice (Krishnan et al., 2020). Compared with HC, the gastric microbiome in the GPL and GC groups changed in composition, ecological network, and function. These changes may be used as relevant factors for predicting carcinogenesis in the future.
In conclusion, our research provides evidence for the imbalance in gastric microbiome in GC patients, clarifies the differences in gastric juice microbiome among HC, GPL, and GC groups, elucidates the changes of gastric microbiome during the development of GC. Our study shows that Lactobacillus is an important indicator strain in the course of gastric cancer. The changes in gastric juice microbial community interactions are indicative of the development of gastric cancer. For now, gastric cancer is still a difficult clinical problem. The genus Lactobacillus is composed of more than 100 species. The biological behavior of different species varies greatly. At the same time, the interactions between Lactobacillus and other microbiome should not be underestimated. Although we propose a key role for Lactobacillus, its specific mechanism of action on gastric cancer remains to be further investigated, this will lay the foundation for the study of gastric cancer.
The 16S rRNA gene data reported in this paper have been deposited in the Sequence Read Archive (https://www.ncbi.nlm.nih.gov/sra), under accession number PRJNA849572 (https://www.ncbi.nlm.nih.gov/bioproject/PRJNA849572/).
The studies involving human participants were reviewed and approved by the independent Ethics Committee of Xiangya Hospital of Central South University following the ethical guidelines of the Declaration of Helsinki (No. 038, 2015). The patients/participants provided their written informed consent to participate in this study.
LC and ZY designed the experiments. SY carried out experiments. XP analyzed data prepared the figures. XP and SY drafted the manuscript. YZ, HC, and JH participation in discussion and revised the manuscript. All authors contributed to this manuscript, read, and approved the final manuscript.
This work was funded by the National Natural Science Foundation of China (32170071 and 32000054).
The authors declare that the research was conducted in the absence of any commercial or financial relationships that could be construed as a potential conflict of interest.
All claims expressed in this article are solely those of the authors and do not necessarily represent those of their affiliated organizations, or those of the publisher, the editors and the reviewers. Any product that may be evaluated in this article, or claim that may be made by its manufacturer, is not guaranteed or endorsed by the publisher.
The Supplementary material for this article can be found online at: https://www.frontiersin.org/articles/10.3389/fmicb.2023.1138928/full#supplementary-material
Abdulamir, A. S., Hafidh, R. R., and Abu Bakar, F. (2011). The association of Streptococcus bovis/gallolyticus with colorectal tumors: the nature and the underlying mechanisms of its etiological role. J. Exp. Clin. Cancer Res. 30:11. doi: 10.1186/1756-9966-30-11
Ben Dekhil, S. M., Peel, M. M., Lennox, V. A., Stackebrandt, E., and Sly, L. I. (1997). Isolation of Lautropia mirabilis from sputa of a cystic fibrosis patient. J. Clin. Microbiol. 35, 1024–1026. doi: 10.1128/jcm.35.4.1024-1026.1997
Bianco, G., Boattini, M., Audisio, E., Cavallo, R., and Costa, C. (2018). Septic shock due to meropenem-and colistin-resistant Cupriavidus pauculus. J. Hosp. Infect. 99, 364–365. doi: 10.1016/j.jhin.2018.03.025
Bjorkholm, B., Falk, P., Engstrand, L., and Nyren, O. (2003). Helicobacter pylori: resurrection of the cancer link. J. Intern. Med. 253, 102–119. doi: 10.1046/j.1365-2796.2003.01119.x
Bokulich, N. A., Kaehler, B. D., Rideout, J. R., Dillon, M., Bolyen, E., Knight, R., et al. (2018). Optimizing taxonomic classification of marker-gene amplicon sequences with QIIME 2 ' s q2-feature-classifier plugin. Microbiome 6:90. doi: 10.1186/s40168-018-0470-z
Bolyen, E., Rideout, J. R., Dillon, M. R., Bokulich, N., Abnet, C. C., Al-Ghalith, G. A., et al. (2019). Reproducible, interactive, scalable and extensible microbiome data science using QIIME 2. Nat. Biotechnol. 37, 852–857. doi: 10.1038/s41587-019-0209-9
Brand, A., Singer, K., Koehl, G. E., Kolitzus, M., Schoenhammer, G., Thiel, A., et al. (2016). LDHA-associated lactic acid production blunts tumor immunosurveillance by T and NK cells. Cell Metab. 24, 657–671. doi: 10.1016/j.cmet.2016.08.011
Brizel, D. M., Schroeder, T., Scher, R. L., Walenta, S., Clough, R. W., Dewhirst, M. W., et al. (2001). Elevated tumor lactate concentrations predict for an increased risk of metastases in head-and-neck cancer. Int. J. Radiat. Oncol. Biol. Phys. 51, 349–353. doi: 10.1016/s0360-3016(01)01630-3
Callahan, B. J., McMurdie, P. J., Rosen, M. J., Han, A. W., Johnson, A. J. A., and Holmes, S. P. (2016). DADA2: high-resolution sample inference from Illumina amplicon data. Nat. Methods 13:581-+. doi: 10.1038/nmeth.3869
Camacho, C., Coulouris, G., Avagyan, V., Ma, N., Papadopoulos, J., Bealer, K., et al. (2009). BLAST plus: architecture and applications. Bmc Bioinformat. 10:421. doi: 10.1186/1471-2105-10-421
Chen, L., Collij, V., Jaeger, M., van den Munckhof, I. C. L., Vila, A. V., Kurilshikov, A., et al. (2020). Gut microbial co-abundance networks show specificity in inflammatory bowel disease and obesity. Nature. Communications 11:17840. doi: 10.1038/s41467-020-17840-y
Chen, X.-H., Wang, A., Chu, A.-N., Gong, Y.-H., and Yuan, Y. (2019). Mucosa-associated microbiota in Gastric cancer tissues compared with non-cancer tissues. Front. Microbiol. 10:1261. doi: 10.3389/fmicb.2019.01261
Chinese Medical Association (2006). Consensus opinion on chronic gastritis in China. Gastroenterology 11, 674–684.
Coenye, T., Spilker, T., Reik, R., Vandamme, P., and LiPuma, J. J. (2005). Use of PCR analyses to define the distribution of Ralstonia species recovered from patients with cystic fibrosis. J. Clin. Microbiol. 43, 3463–3466. doi: 10.1128/jcm.43.7.3463-3466.2005
Coker, O. O., Dai, Z., Nie, Y., Zhao, G., Cao, L., Nakatsu, G., et al. (2018). Mucosal microbiome dysbiosis in gastric carcinogenesis. Gut 67, 1024–1032. doi: 10.1136/gutjnl-2017-314281
Corbet, C., and Feron, O. (2017). Tumour acidosis: from the passenger to the driver's seat. Nat. Rev. Cancer 17, 577–593. doi: 10.1038/nrc.2017.77
Correa, P. (1992). Human gastric carcinogenesis: a multistep and multifactorial process--first American Cancer Society award lecture on cancer epidemiology and prevention. Cancer Res. 52, 6735–6740.
Cui, J., Cui, H., Yang, M., Du, S., Li, J., Li, Y., et al. (2019). Tongue coating microbiome as a potential biomarker for gastritis including precancerous cascade. Protein Cell 10, 496–509. doi: 10.1007/s13238-018-0596-6
Dai, D., Yang, Y., Yu, J., Dang, T., Qin, W., Teng, L., et al. (2021). Interactions between gastric microbiota and metabolites in gastric cancer. Cell Death Dis. 12:1104. doi: 10.1038/s41419-021-04396-y
Diaz, P., Valenzuela Valderrama, M., Bravo, J., and Quest, A. F. G. (2018). Helicobacter pylori and Gastric cancer: adaptive cellular mechanisms involved in disease progression. Front. Microbiol. 9:5. doi: 10.3389/fmicb.2018.00005
Duan, X., Chen, P., Xu, X., Han, M., and Li, J. (2022). Role of Gastric microorganisms other than helicobacter pylori in the development and treatment of Gastric diseases. Biomed. Res. Int. 2022, 6263423–6263411. doi: 10.1155/2022/6263423
Ferreira, R. M., Pereira-Marques, J., Pinto-Ribeiro, I., Costa, J. L., Carneiro, F., Machado, J. C., et al. (2018). Gastric microbial community profiling reveals a dysbiotic cancer-associated microbiota. Gut 67, 226–236. doi: 10.1136/gutjnl-2017-314205
Gantuya, B., El Serag, H. B., Matsumoto, T., Ajami, N. J., Uchida, T., Oyuntsetseg, K., et al. (2020). Gastric mucosal microbiota in a Mongolian population with gastric cancer and precursor conditions. Aliment. Pharmacol. Ther. 51, 770–780. doi: 10.1111/apt.15675
Gomersall, A. C., Huy Anh, P., Iacuone, S., Li, S. F., and Parish, R. W. (2015). The mycoplasma hyorhinis p37 protein rapidly induces genes in fibroblasts associated with inflammation and cancer. PLoS One 10:e0140753. doi: 10.1371/journal.pone.0140753
Gong, M., Meng, L., Jiang, B., Zhang, J., Yang, H., Wu, J., et al. (2008). p37 from mycoplasma hyorhinis promotes cancer cell invasiveness and metastasis through activation of MMP-2 and followed by phosphorylation of EGFR. Mol. Cancer Ther. 7, 530–537. doi: 10.1158/1535-7163.Mct-07-2191
Han, K. J., Lee, N.-K., Park, H., and Paik, H.-D. (2015). Anticancer and anti-inflammatory activity of probiotic Lactococcus lactis NK34. J. Microbiol. Biotechnol. 25, 1697–1701. doi: 10.4014/jmb.1503.03033
Han, Y. W., and Wang, X. (2013). Mobile microbiome: Oral bacteria in extra-oral infections and inflammation. J. Dent. Res. 92, 485–491. doi: 10.1177/0022034513487559
Hayes, C., Donohoe, C. L., Davern, M., and Donlon, N. E. (2021). The oncogenic and clinical implications of lactate induced immunosuppression in the tumour microenvironment. Cancer Lett. 500, 75–86. doi: 10.1016/j.canlet.2020.12.021
Hess, D. L. J., Pettersson, A. M., Rijnsburger, M. C., Herbrink, P., van den Berg, H. P., and Ang, C. W. (2012). Gastroenteritis caused by campylobacter concisus. J. Med. Microbiol. 61, 746–749. doi: 10.1099/jmm.0.032466-0
Hessey, S. J., Spencer, J., Wyatt, J. I., Sobala, G., Rathbone, B. J., Axon, A. T., et al. (1990). Bacterial adhesion and disease activity in helicobacter associated chronic gastritis. Gut 31, 134–138. doi: 10.1136/gut.31.2.134
Hirschhaeuser, F., Sattler, U. G. A., and Mueller-Klieser, W. (2011). Lactate: a metabolic key player in cancer. Cancer Res. 71, 6921–6925. doi: 10.1158/0008-5472.Can-11-1457
Huang, K., Gao, X., Wu, L., Yan, B., Wang, Z., Zhang, X., et al. (2021). Salivary microbiota for Gastric cancer prediction: an exploratory study. Front. Cell. Infect. Microbiol. 11:640309. doi: 10.3389/fcimb.2021.640309
Istivan, T. S., and Coloe, P. J. (2006). Phospholipase a in gram-negative bacteria and its role in pathogenesis. Microbiol. SGM 152, 1263–1274. doi: 10.1099/mic.0.28609-0
Oksanen, J., Simpson, G., Blanchet, F., Kindt, R., Legendre, P., Minchin, P., et al. (2022). _Vegan: Community Ecology Package_. R package version 2.6-2. Available at: https://CRAN.R-project.org/package=vegan
Kessenbrock, K., Plaks, V., and Werb, Z. (2010). Matrix metalloproteinases: regulators of the tumor microenvironment. Cells 141, 52–67. doi: 10.1016/j.cell.2010.03.015
Kobayashi, T., Nakamura, I., Fujita, H., Tsukimori, A., Sato, A., Fukushima, S., et al. (2016). First case report of infection due to Cupriavidus gilardii in a patient without immunodeficiency: a case report. BMC Infect. Dis. 16:493. doi: 10.1186/s12879-016-1838-y
Kolde, R. (2019). _pheatmap: Pretty Heatmaps_. R package version 1.0.12. Available at: https://CRAN.R-project.org/package=pheatmap
Krishnan, V., Lim, D. X. E., Hoang, P. M., Srivastava, S., Matsuo, J., Huang, K. K., et al. (2020). DNA damage signalling as an anti-cancer barrier in gastric intestinal metaplasia. Gut 69, 1738–1749. doi: 10.1136/gutjnl-2019-319002
Kwon, S.-K., Park, J. C., Kim, K. H., Yoon, J., Cho, Y., Lee, B., et al. (2021). Human gastric microbiota transplantation recapitulates premalignant lesions in germ-free mice. Gut 71, 1266–1276. doi: 10.1136/gutjnl-2021-324489
Lai, J., Zou, Y., Zhang, J., and Peres-Neto, P. (2022). Generalizing hierarchical and variation partitioning in multiple regression and canonical analysis using the rdacca.hp R package. Methods Ecol. Evol. 13, 782–788. doi: 10.1111/2041-210X.13800
Lam, S. Y., Yu, J., Wong, S. H., Peppelenbosch, M. P., and Fuhler, G. M. (2017). The gastrointestinal microbiota and its role in oncogenesis. Best Pract. Res. Clin. Gastroenterol. 31, 607–618. doi: 10.1016/j.bpg.2017.09.010
Lertpiriyapong, K., Whary, M. T., Muthupalani, S., Lofgren, J. L., Gamazon, E. R., Feng, Y., et al. (2014). Gastric colonisation with a restricted commensal microbiota replicates the promotion of neoplastic lesions by diverse intestinal microbiota in the helicobacter pylori INS-GAS mouse model of gastric carcinogenesis. Gut 63, 54–63. doi: 10.1136/gutjnl-2013-305178
Li, Y., Huang, X., Tong, D., Jiang, C., Zhu, X., Wei, Z., et al. (2022). Relationships among microbiota, gastric cancer, and immunotherapy. Front. Microbiol. 13:987763. doi: 10.3389/fmicb.2022.987763
Liu, F., Ma, R., Wang, Y., and Zhang, L. (2018). The clinical importance of campylobacter conscious and other human hosted campylobacter species. Front. Cell. Infect. Microbiol. 8:243. doi: 10.3389/fcimb.2018.00243
Marttila, E., Jarvensivu, A., Sorsa, T., Grenier, D., Richardson, M., Kari, K., et al. (2014). Intracellular localization of Treponema denticola chymotrypsin-like proteinase in chronic periodontitis. J. Oral Microbiol. 6:24349. doi: 10.3402/jom.v6.24349
Medical Secretary, M.O.H., People's Republic of China (2012). Gastric cancer treatment standards (electronic version). Chin. J. Front. Med.
Nakatsu, G., Li, X., Zhou, H., Sheng, J., Wong, S. H., Wu, W. K. K., et al. (2015). Gut mucosal microbiome across stages of colorectal carcinogenesis. Nat. Commun. 6:8727. doi: 10.1038/ncomms9727
Niu, X.-K., Rao, M. P. N., Dong, Z.-Y., Kan, Y., Li, Q.-R., Huang, J., et al. (2020). Vulcaniibacterium gelatinicum sp. nov., a moderately thermophilic bacterium isolated from a hot spring. Int. J. Syst. Evol. Microbiol. 70, 1571–1577. doi: 10.1099/ijsem.0.003934
Palmer, J. D., and Foster, K. R. (2022). Bacterial species rarely work together. Science 376, 581–582. doi: 10.1126/science.abn5093
Papapanou, P. N., Park, H., Cheng, B., Kokaras, A., Paster, B., Burkett, S., et al. (2020). Subgingival microbiome and clinical periodontal status in an elderly cohort: the WHICAP ancillary study of oral health. J. Periodontol. 91, S56–S67. doi: 10.1002/jper.20-0194
Png, C. W., Lee, W. J. J., Chua, S. J., Zhu, F., Gastric, C., Yeoh, K. G., et al. (2022). Mucosal microbiome associates with progression to gastric cancer. Theranostics 12, 48–58. doi: 10.7150/thno.65302
R Core Team (2022). R: A language and environment for statistical computing. R Foundation for Statistical Computing, Vienna, Austria. Available at: https://www.R-project.org/
Robeson, M. S. 2nd, O'Rourke, D. R., Kaehler, B. D., Ziemski, M., Dillon, M. R., Foster, J. T., et al. (2021). RESCRIPt: Reproducible sequence taxonomy reference database management. PLoS Comput. Biol. 17:e1009581. doi: 10.1371/journal.pcbi.1009581
Robinson, M. D., McCarthy, D. J., and Smyth, G. K. (2010). edgeR: a Bioconductor package for differential expression analysis of digital gene expression data. Bioinformatics 26, 139–140. doi: 10.1093/bioinformatics/btp616
Rossmann, S. N., Wilson, P. H., Hicks, J., Carter, B., Cron, S. G., Simon, C., et al. (1998). Isolation of Lautropia mirabilis from oral cavities of human immunodeficiency virus-infected children. J. Clin. Microbiol. 36, 1756–1760. doi: 10.1128/jcm.36.6.1756-1760.1998
Ruch, T. R., and Engel, J. N. (2017). Targeting the mucosal barrier: how pathogens modulate the cellular polarity network. Cold Spring Harb. Perspect. Biol. 9:a027953. doi: 10.1101/cshperspect.a027953
San-Millan, I., and Brooks, G. A. (2017). Reexamining cancer metabolism: lactate production for carcinogenesis could be the purpose and explanation of the Warburg effect. Carcinogenesis 38, bgw127–bgw133. doi: 10.1093/carcin/bgw127
Segata, N., Izard, J., Waldron, L., Gevers, D., Miropolsky, L., Garrett, W. S., et al. (2011). Metagenomic biomarker discovery and explanation. Genome Biol. 12:R60. doi: 10.1186/gb-2011-12-6-r60
Stewart, O. A., Wu, F., and Chen, Y. (2020). The role of gastric microbiota in gastric cancer. Gut Microbes 11, 1220–1230. doi: 10.1080/19490976.2020.1762520
Sun, J.-H., Li, X.-L., Yin, J., Li, Y.-H., Hou, B.-X., and Zhang, Z. (2018). A screening method for gastric cancer by oral microbiome detection. Oncol. Rep. 39, 2217–2224. doi: 10.3892/or.2018.6286
Sung, H., Ferlay, J., Siegel, R. L., Laversanne, M., Soerjomataram, I., Jemal, A., et al. (2021). Global cancer statistics 2020: GLOBOCAN estimates of incidence and mortality worldwide for 36 cancers in 185 countries. CA Cancer J. Clin. 71, 209–249. doi: 10.3322/caac.21660
Thrift, A. P., and El-Serag, H. B. (2020). Burden of Gastric cancer. Clin. Gastroenterol. Hepatol. 18, 534–542. doi: 10.1016/j.cgh.2019.07.045
Topper, M. J., Vaz, M., Chiappinelli, K. B., Shields, C. E. D., Niknafs, N., Yen, R.-W. C., et al. (2017). Epigenetic therapy ties MYC depletion to reversing immune evasion and treating lung cancer. Cells 171:1284-+. doi: 10.1016/j.cell.2017.10.022
Tseng, C.-H., Lin, J.-T., Ho, H. J., Lai, Z.-L., Wang, C.-B., Tang, S.-L., et al. (2016). Gastric microbiota and predicted gene functions are altered after subtotal gastrectomy in patients with gastric cancer. Sci. Rep. 6:20701. doi: 10.1038/srep20701
Vaneechoutte, M., Kampfer, P., De Baere, T., Falsen, E., and Verschraegen, G. (2004). Wautersia gen. Nov., a novel genus accommodating the phylogenetic lineage including Ralstonia eutropha and related species, and proposal of Ralstonia Pseudomonas syzygii (Roberts et al. 1990) comb. nov. Int. J. Syst. Evol. Microbiol. 54, 317–327. doi: 10.1099/ijs.0.02754-0
Vinasco, K., Mitchell, H. M., Kaakoush, N. O., and Castano-Rodriguez, N. (2019). Microbial carcinogenesis: lactic acid bacteria in gastric cancer. Biochimica et Biophysica Acta Rev. Cancer 1872:188309. doi: 10.1016/j.bbcan.2019.07.004
von Rosenvinge, E. C., Song, Y., White, J. R., Maddox, C., Blanchard, T., and Fricke, W. F. (2013). Immune status, antibiotic medication and pH are associated with changes in the stomach fluid microbiota. ISME J. 7, 1354–1366. doi: 10.1038/ismej.2013.33
Wang, L., Dankert, H., Perona, P., and Anderson, D. J. (2008). A common genetic target for environmental and heritable influences on aggressiveness in drosophila. Proc. Natl. Acad. Sci. U. S. A. 105, 5657–5663. doi: 10.1073/pnas.0801327105
Wang, L. L., Liu, J. X., Yu, X. J., Si, J. L., Zhai, Y. X., and Dong, Q. J. (2018). Microbial community reshaped in gastric cancer. Eur. Rev. Med. Pharmacol. Sci. 22, 7257–7264. doi: 10.26355/eurrev_201811_16260
Wang, L., Xin, Y., Zhou, J., Tian, Z., Liu, C., Yu, X., et al. (2020). Gastric mucosa-associated microbial signatures of early Gastric cancer. Front. Microbiol. 11:1548. doi: 10.3389/fmicb.2020.01548
Warburg, O., Wind, F., and Negelein, E. (1927). The metabolism of tumors in the body. J. Gen. Physiol. 8, 519–530. doi: 10.1085/jgp.8.6.519
Whary, M. T., Muthupalani, S., Ge, Z., Feng, Y., Lofgren, J., Shi, H. N., et al. (2014). Helminth co-infection in helicobacter pylori infected INS-GAS mice attenuates gastric premalignant lesions of epithelial dysplasia and glandular atrophy and preserves colonization resistance of the stomach to lower bowel microbiota. Microbes Infect. 16, 345–355. doi: 10.1016/j.micinf.2014.01.005
Yu, G., Torres, J., Hu, N., Medrano-Guzman, R., Herrera-Goepfert, R., Humphrys, M. S., et al. (2017). Molecular characterization of the human stomach microbiota in Gastric cancer patients. Front. Cell. Infect. Microbiol. 7:302. doi: 10.3389/fcimb.2017.00302
Yu, T.-T., Zhou, E.-M., Yin, Y.-R., Yao, J.-C., Ming, H., Dong, L., et al. (2013). Vulcaniibacterium tengchongense gen. Nov., sp nov isolated from a geothermally heated soil sample, and reclassification of Lysobacter thermophilus Wei et al. 2012 as Vulcaniibacterium thermophilum comb. nov. Anton. Leeuw. Int. J. Gen. Mol. Microbiol. 104, 369–376. doi: 10.1007/s10482-013-9959-4
Yuan, M. M., Guo, X., Wu, L., Zhang, Y., Xiao, N., Ning, D., et al. (2021). Climate warming enhances microbial network complexity and stability. Nat. Clim. Chang. 11, 343–348. doi: 10.1038/s41558-021-00989-9
Zhang, X., Li, C., Cao, W., and Zhang, Z. (2021). Alterations of Gastric microbiota in Gastric cancer and precancerous stages. Front. Cell. Infect. Microbiol. 11:559148. doi: 10.3389/fcimb.2021.559148
Keywords: gastric carcinogenesis, microbiome, gastric cancer, microbial interactions, Lactobacillus
Citation: Peng X, Yao S, Huang J, Zhao Y, Chen H, Chen L and Yu Z (2023) Alterations in bacterial community dynamics from noncancerous to Gastric cancer. Front. Microbiol. 14:1138928. doi: 10.3389/fmicb.2023.1138928
Received: 06 January 2023; Accepted: 20 February 2023;
Published: 09 March 2023.
Edited by:
Alina Maria Holban, University of Bucharest, RomaniaReviewed by:
Congmin Xu, Georgia Institute of Technology, United StatesCopyright © 2023 Peng, Yao, Huang, Zhao, Chen, Chen and Yu. This is an open-access article distributed under the terms of the Creative Commons Attribution License (CC BY). The use, distribution or reproduction in other forums is permitted, provided the original author(s) and the copyright owner(s) are credited and that the original publication in this journal is cited, in accordance with accepted academic practice. No use, distribution or reproduction is permitted which does not comply with these terms.
*Correspondence: Zheng Yu, eXV6aGVuZ0Bjc3UuZWR1LmNu; Liyu Chen, Y2hlbmxpeXVAY3N1LmVkdS5jbg==
†These authors have contributed equally to this work
Disclaimer: All claims expressed in this article are solely those of the authors and do not necessarily represent those of their affiliated organizations, or those of the publisher, the editors and the reviewers. Any product that may be evaluated in this article or claim that may be made by its manufacturer is not guaranteed or endorsed by the publisher.
Research integrity at Frontiers
Learn more about the work of our research integrity team to safeguard the quality of each article we publish.